ImageMech: From Image to Particle Spring Network for Mechanical Characterization
- 1Department of Civil Engineering, National Taiwan University, Taipei, Taiwan
- 2Physics Department, National Taiwan University, Taipei, Taiwan
- 3Institute of Physics, Academia Sinica, Taipei, Taiwan
- 4Physics Department, National Taiwan Normal University, Taipei, Taiwan
- 5Department of Biomedical Engineering, National Taiwan University, Taipei, Taiwan
In the original article, there was a mistake in Figure 7 as published. The original published figure does not include the total wall time of CPU version of CuLSM and of LAMMPS simulations with different neighbor settings. The corrected Figure 7 appears below.
Consequently, a correction has been made to Section 3 Results, sub-section 3.2 Benchmarks of CuLSM Acceleration, Paragraph 1.
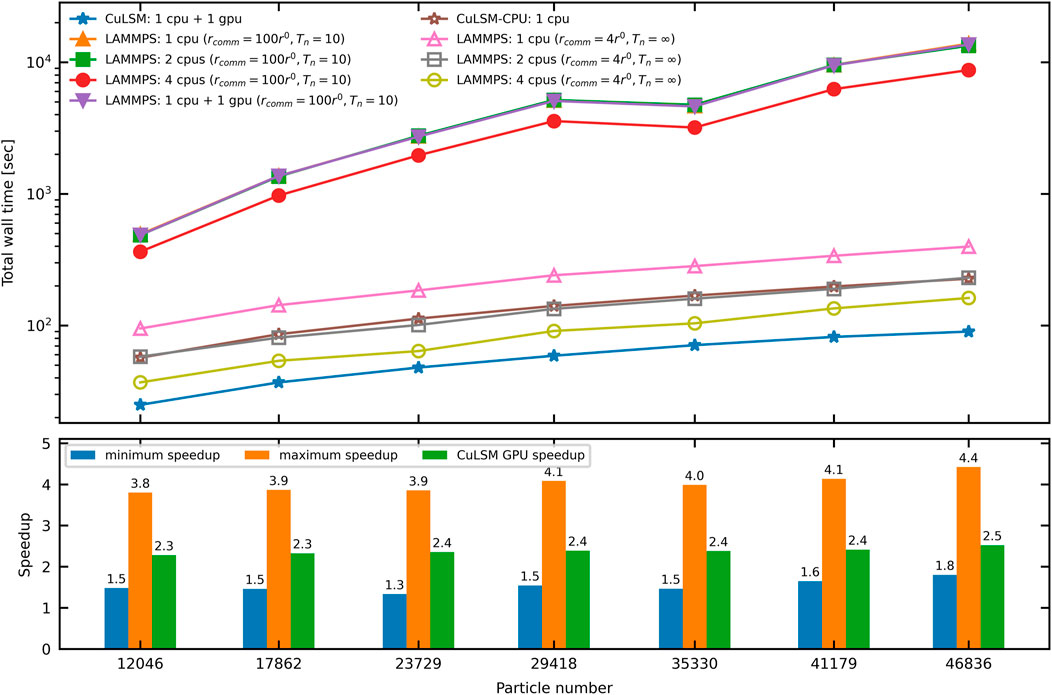
FIGURE 7. Comparison of the total computing wall time by CuLSM and LAMMPS. Note that LAMMPS: 1 CPU + 1 GPU does not support parallelism on the spring list. The bottom panel compares the maximum and minimum speedup of CuLSM against LAMMPS of rcomm = 4r0 and Tn = ∞. The maximum speedup compares CuLSM: 1 CPU + 1 GPU with LAMMPS: 1 CPU, and the minimum speedup compares CuLSM: 1 CPU + 1 GPU with LAMMPS: 4 CPUs. The GPU speedup of CuLSM (CuLSM: 1 CPU + 1 GPU versus CuLSM-CPU: 1 CPU) is presented.
“To benchmark the performance of CuLSM, we record the computing time of mode-I fracture simulations on Poisson composites of different sizes, as listed in Table 2. In Figure 7, we compare the total wall time of simulations by CuLSM (1 CPU + 1 GPU) and LAMMPS with 1 CPU, 2 CPUs, 4 CPUs, and 1 CPU + 1 GPU. With inter-processor communication cutoff rcomm = 100r0 and default step interval for neighbor list update Tn = 10, LAMMPS with 1 CPU can be one to two orders slower than CuLSM. With these settings, LAMMPS is unfavorably slow and the spatial decomposition scheme is incapable of accelerating the LSM simulation efficiently. Note that LAMMPS does not currently support GPU acceleration on bond potentials. Therefore, LAMMPS 1 CPU + 1 GPU shows no speedup compared to LAMMPS 1 CPU. With communication cutoff (rcomm = 4r0) and turning off the neighbor list update (Tn = ∞), the total wall time of LAMMPS scales in the same order as CuLSM with respect to the particle number. CuLSM can be up to 4.4 times faster than LAMMPS with 1 CPU and have around 1.5 speedup compared to LAMMPS with 4 CPUs. CuLSM-CPU with 1 CPU has comparable speed with LAMMPS with 2 CPUs. Note that the optimal neighbor setting depends on the simulation cases for the spatial decomposition scheme. The GPU speedup of CuLSM, i.e., the speedup of CuLSM 1 CPU + 1 GPU against CuLSM-CPU 1 CPU, is also presented in the bottom panel of Figure 7. On the machine with Intel i5-8400 and Nvidia GeForce GTX 1060, the GPU speedup of CuLSM is about 2.5. CuLSM reduces the total wall time (including input, output, and copying) by a considerable margin, with only 1 CPU and 1 GPU. The enhanced performance results from the parallelization on particle and spring lists. The input files for all the benchmarks andmore information can be found online at the link in Data Availability Statement”.
The authors apologize for this error and state that this does not change the scientific conclusions of the article in any way. The original article has been updated.
Publisher’s Note
All claims expressed in this article are solely those of the authors and do not necessarily represent those of their affiliated organizations, or those of the publisher, the editors, and the reviewers. Any product that may be evaluated in this article, or claim that may be made by its manufacturer, is not guaranteed or endorsed by the publisher.
Keywords: CUDA (compute unified device architecture), parallel computing, modeling and simulation, lattice spring model (LSM), mechanical characterisation
Citation: Chiang Y, Chiu TW and Chang SW (2022) Corrigendum: ImageMech: From Image to Particle Spring Network for Mechanical Characterization. Front. Mater. 9:883245. doi: 10.3389/fmats.2022.883245
Received: 24 February 2022; Accepted: 14 March 2022;
Published: 25 April 2022.
Edited and reviewed by:
Flavia Libonati, University of Genoa, ItalyCopyright © 2022 Chiang, Chiu and Chang. This is an open-access article distributed under the terms of the Creative Commons Attribution License (CC BY). The use, distribution or reproduction in other forums is permitted, provided the original author(s) and the copyright owner(s) are credited and that the original publication in this journal is cited, in accordance with accepted academic practice. No use, distribution or reproduction is permitted which does not comply with these terms.
*Correspondence: Shu-Wei Chang, changsw@ntu.edu.tw