- Department of Materials Science and Engineering, Indian Institute of Technology Kanpur, Kanpur, India
The emergence of High Entropy Alloys (HEAs) in the world of materials has shifted the alloy design strategy based on a single principal element to the multi-principal elements where compositional space can cover almost the entire span of the higher dimensional phase diagrams. This approach can provide advanced materials with unique properties, including high strength with sufficient ductility and fracture toughness and excellent corrosion and wear resistance for a wide range of temperatures due to the concentrated alloying that cannot be obtained by traditional microalloying based on a single principal element. In addition, the alloy design approach provides new alloy systems in astronomical numbers with variety of microstructural attributes that can yield different properties, and hence conventional trial and error experimental methods for alloy development are redundant. With the help of high throughput experiments along with efficient computational tools, and artificial intelligence, mechanisms based mechanistic development of the multi-principal element alloys with tailored solid solution strengthening, stacking fault energy and microstructure is possible. The current review discusses the various design strategies based on multi-principal elements alloys in semblance with the desired mechanical properties dictated by the micro mechanisms associated with them to overcome the bottlenecks presented by the conventional approaches with possible breakthrough applications. The article will shed light on the current status as well as the future prospects of using these approaches to design novel HEAs.
Introduction
The millennia-old approach of alloy design based on the principal element doped with other elements in a minor amount for the improvement of the properties is almost getting exhausted in terms of synthesis of new alloy chemistry with a substantial change in the property, and most importantly, no new stable elements have been discovered for the last 100 years (Miracle, 2019). The surge of demand for new materials across the globe is ever increasing, including the intensive demands of high-temperature materials for energy productions and aerospace propulsions and low-cost, lightweight alloys for transportation and materials for strategic applications, including space and defence applications. Hence, a new alloy design strategy has been felt for the last 30 years, which will provide a new direction. In fact, this design approach can be considered a paradigm shift in alloy design and development of new materials, potentially leading to a mature technology. The advent of High Entropy Alloys (HEAs) (Miracle and Senkov, 2017; Sharma et al., 2018; George et al., 2019; Biswas et al., 2020) granted a new lifeline for alloys design technology where the development of new alloys is no longer uses the principal element-based approach. The HEAs attracted the materials science community owing to their unique multi principal elemental matrix, vast unexplored compositional space, unique structural and functional properties, bringing new frontiers in the material design. The vast compositional space offers the cosmic number of alloys systems, with some of these having promising properties such as excellent cryogenic temperature strength-ductility (Otto et al., 2013) and fracture toughness (Li W. et al., 2018), high-temperature strength (Feng et al., 2021), highly corrugated dislocation (Smith et al., 2016), remarkable irradiation resistance as self-healing is suggested mechanism (Xia et al., 2015), good parabolic oxidation resistance of than those of the conventional refractories alloys (Butler et al., 2017) as well as good wear resistance (Firstov et al., 2017; Yadav et al., 2018). In addition to their remarkable properties, HEAs continue to stimulate the scientific curiosity to understand fundamental material properties with new micro-mechanisms in a multicomponent space. The primary strengthening mechanism in the multicomponent alloys will involve multiple aspects: solid solution hardening, twin induced plasticity (TWIP), transformation induced plasticity (TRIP), bi-directional TWIP, controlled by compositional tunning. HEAs provide a vast compositional space; thus, compositional tuning can be considered a critical approach in the selection of the elements from the palette to achieve the desired properties. In this regard, stacking fault energy (SFE) plays a vital role in designing materials for structural applications. SFE is primarily driven by compositional tuning in terms of the type of the elements and their concentration, dictating the TWIP (De Cooman et al., 2018) and TRIP (Bhadeshia, 2002) effect in addition to the inherent solid solution strengthening, precipitate, and cluster strengthening at the nanoscale, and even recent study shows the atomic scale amorphous strengthening (Zhao et al., 2021). The synergic effect of all mechanisms mentioned above can provide the alloys system with the combination of strength, ductility, fracture toughness breaking the strength-ductility paradox. The properties of HEAs are further enhanced via microstructural engineering by the improvisation of novel processing such as equal-channel angular pressing (ECAP) (Furukawa et al., 2001), high pressure torsion (HPT) (Wu et al., 2017), and friction stir processing (FSP) (Ma, 2008), and recently, additive manufacturing along with conventional thermomechanical processes (rolling, forging followed by heat treatment) at different temperatures and time scales.
Thus, the selection of appropriate alloy chemistry from the cosmic number of alloys systems and their processing techniques that include synthesis and post-synthesis are challenging and require rapid screening by means of experimental findings aided by high throughput computational tools. For rapid throughput experimentation, the indention technique is only fast, reliable, and versatile in screening the mechanical properties from a small volume of the materials such as elastic modulus, hardness, and creep of materials.
Li et al. suggested that the high throughput experimental screening can even be enhanced by the rapid alloying prototyping, which mainly consists of the synthesis of at least five alloys systems of tuned composition in a single step in different copper mould and subsequently undergo the thermomechanical processing coupled with microstructural characterization (Li Z. et al., 2018). This mainly helps find out the suitable alloys system with outstanding mechanical properties. On the other hand, the generation of data is five times faster as compared other processing routes. The authors further suggested the use of diffusion multiple couples for quaternary and quinary alloy systems that helps to understand the intermixing of elements, their compositions, and types of phase formations. The structural and mechanical properties of these phases can also be determined by microscale techniques such as electron probe micro analyzer (EPMA), electron backscatter diffraction (EBSD), transmission electron microscopy (TEM), and atom probe tomography (APT) for phase analysis; nanoindentation and atomic force microscopy (AFM) for mechanical properties. Though these techniques involve time and computational prowess, it generates a large amount of data at a time, which can be fed back to computational tools such as CALcultion of PHAse Diagram (CALPHAD), Machine Learning (ML), and Artificial Intelligence (AI). It can thus lead to enhancing the accuracy of these tools. Figure 1 provides a schematic flow chart of multi scale modelling techniques for rapid alloy design. The computational tools such as CALPHAD, DFT calculation using VASP toolkit, ML)and AI play a vital role in screening of the alloys in terms of phases and their stability, structural and functional properties, and most importantly, the scientific understanding of the fundamental micro-mechanisms for the real-time applications of these class of materials (Kumar et al., 2018). In the following, we shall deliberate the use of the computational tools in enhancing the design of novel alloying with the betterment of mechanical properties. We shall focus on the mechanical properties of crystalline (FCC, BCC, HCP) and amorphous alloys.
Property Driven Alloy Design for HEAs
In practice, the conventional alloy design strategies revolve around tailoring the chemical compositions and associated microstructures of existing alloys to desired properties by considering deformation micro mechanisms, alloy prototyping using trial and error, and high-throughput calculations (Varvenne et al., 2016; Lilensten et al., 2017; Chen et al., 2020). After years of alloy design practice, various alloy systems have emerged from the laboratories and found significant value in a variety of engineering applications. As an emerging alloy family, all heritage alloy design methodologies of the conventional alloys have led to empirical rules-based design strategies that have proven successful in traditional alloy systems (Raturi et al., 2019). These are being utilized at the beginning to design HEAs (Zhang et al., 2008; Guo and Liu, 2011). Though, these strategies are effective to obtain the some of the new novel multicomponent alloys but are found to be not as effective in terms of their properties. In fact, as HEAs research advances, the disadvantages of this approach have become more apparent. Because HEAs contain several primary elements, they have a far greater variety of potential compositions than conventional alloys. The vast compositional space offers several opportunities to produce the necessary set of mechanical characteristics. Traditional approaches for designing HEAs, on the other hand, need a significant amount of computing time and power in order to traverse this considerable composition space. Furthermore, traditional alloy design procedures have been proven to be unable to incorporate many desired qualities into the fold when building new alloys. Even when taken into account, the crucial micro mechanisms responsible for improved mechanical behaviour of HEAs are frequently integrated in the last phases of alloy development (Guo et al., 2011; Ye et al., 2015; Tancret et al., 2017; Biswas et al., 2020). These constraints have hampered the extension of the HEAs space beyond Cantor alloy and its derivatives. Therefore, high-throughput computational tools and machine learning that can circumvent the reduced efficiency of earlier approaches have come to the fore to establish a new and advanced alloy design ecosystem for multicomponent alloys.
Computational thermodynamics using CALPHAD (an acronym for “calculation of phase diagrams”) is the most commonly used computational approach in designing HEAs, as their phase structures and phase equilibria are of particular relevance to desired properties and hence potential applications (Gao and Alman, 2013). The most significant advantage of CALPHAD is the use of free energy to analyse phase formation rather than entropy alone, permitting the understanding of the function of system enthalpy in the design of HEAs. It gives specific information on the phase as a function of composition, temperature, and pressure. It acts as a guidance diagram for new alloy design and development. Extensive research on HEAs has indicated that CALPHAD can effectively estimate primary phases present in HEAs (Senkov et al., 2013; Miracle and Senkov, 2017; Chen et al., 2019). It does not, however, yield enough screened HEAs with the required phases in the temperature range of interest. Despite this, the thermodynamic database has grown and will continue to grow as more experimental data becomes available, and the expected accuracy of CALPHAD for HEAs will improve even more. The advent of new HEAs databases in recent years, such as TCHEA3 and TCHEA5 (Chen et al., 2016), has aided in overcoming the previous issues. As a result, high-throughput CALPHAD simulations should become more dependable and helpful in creating and optimizing HEAs compositions for desired alloy properties.
A variety of advanced computational approaches at different length and time scales, including quantum mechanics, molecular dynamics, mesoscale simulations, and continuum mechanics, have also been developed as robust newer high-throughput alloy design strategies. Quantum mechanics-based first-principles computations can compute the behaviour of atoms and electrons in condensed states and quantitatively define atomic bonding in an alloy (Lederer et al., 2018). As a result, the characteristics of any material can only be predicted using basic principles by solving the Schrödinger equation (Curtarolo et al., 2005; Curtarolo et al., 2012). The first principles method based on density functional theory (DFT) can predict physical attributes without the need for experimental data, relying solely on calculations based on charge, electronic mass, and Planck’s constant. This approach allows systematic analysis of HEAs from the standpoint of theoretical calculations, which is important for expanding knowledge of HEAs (Huang W. et al., 2019). The exact muffin-tin orbitals (EMTO) based on the framework of density functional theory defines the single-electron potential function and the total energy of the calculation system by employing the optimal overlapping potential sphere configuration and the charge density. This strategy not only increases computation efficiency but also ensures adequate calculation correctness. In first-principles calculations, the coherent potential approximation (CPA) can solve the disorder model of a multi-principal element replacement solid solution. As a result, the combined EMTO-CPA technique is one of the most successful ways for solving the first-principles calculation issue of HEAs.It has been argued, however, that multiphase structures are difficult to predict using these empirical principles, even though these alloys may be more enticing due to their multiphase nature and attractive properties.
Ab-initio molecular dynamics (AIMD) simulations, which are based on quantum mechanics, can anticipate the structure of liquid atoms and help better understand the solidification behaviour of complex alloys. AIMD is commonly used to assist in predicting the stability of alloy compositions, phase structures, as well as to screen candidate alloys with desired properties (Li et al., 2017). AIMD simulations, as opposed to classical molecular dynamics (MD) simulations, do not require elaborate experiments to get the interaction potential between atoms, making them more viable and easier to use. This approach has been successfully used to determine the elastic modulus and strength of several HEAs.Another method for understanding the microstructural development of HEAs for design involves the employment of phase-field modelling (Li et al., 2020). Similarly, several other mathematical modelling approaches, like statistical and strengthening modelling, may be used to filter a large pool of HEAs compositions for the desired set of properties (Tancret et al., 2017; Menou et al., 2018). These different computational approaches can potentially be linked together to develop a robust strategy to design HEAs with numerous target properties at the same time. Furthermore, these computational tools may be configured to run in a high-throughput mode, significantly reducing design cycles and computation time (Tancret et al., 2017; Menou et al., 2018).
Another high-throughput technology that can aid in the design of HEAs microstructures and properties is machine learning (ML). With the rapid acceptance of data science and informatics in the material science community, ML has become the front runner to guide alloy design from numerous perspectives, such as compositional design, phase prediction, and property-based alloy design. However, most of the studies in this direction have been concentrated on phase prediction in HEAs (Zhou et al., 2019; Abdoon Al-Shibaany et al., 2020; Kaufmann and Vecchio, 2020; Machaka, 2021). Mechanical property-focused alloy design with the assistance of ML finds limited investigations in HEAs. Nevertheless, more advanced forms of ML tools such as neural networks have shown light for high-throughput screening and determination of the composition-property relationships in HEAs in recent times. This has been further enhanced by combining ML with other high throughput computational tools to design HEAs based on their desired properties. Using the right ensemble and periodic-boundary conditions, molecular dynamics simulations can generate a property database. It can then be used in conjunction with ML to anticipate the mechanical characteristics of HEAs based on their elemental information (Wen et al., 2019; Klimenko et al., 2021). Therefore, various screening methods can be used in combination with ML to produce a complete forecast of the alloy systems based on the desired set of properties. This offers the potential to speed the screening of high-performance HEAs systems, allowing for the relationship between material composition, structure, and required attributes to be determined with high efficiency, low cost, and high reliability. Figure 2 shows a hypothetical environment wherein various design strategies can function in unison to accelerate property driven materials discovery.
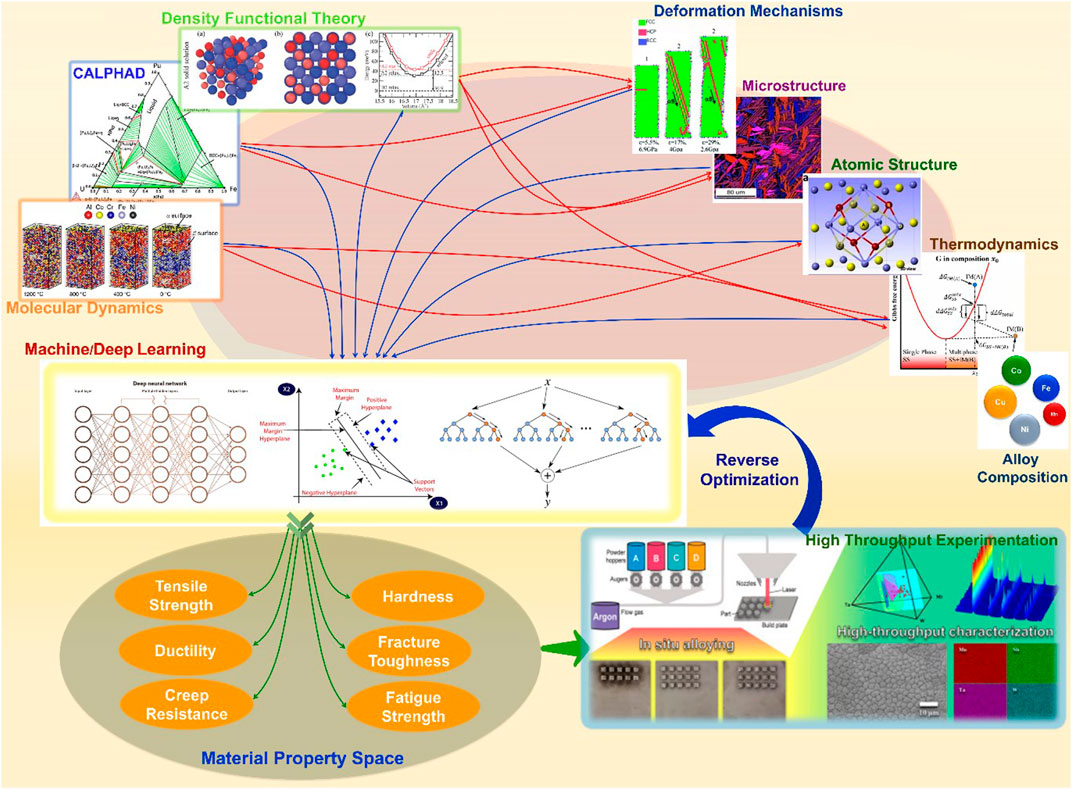
FIGURE 2. Schematic diagram showing the simultaneous utilization of various high throughput computational alloy design tools to create a closed-loop property driven design strategy for HEAs.
Overall, the utilization of these design approaches for HEAs is still very rudimentary because these methodologies are still in their infancy. Nonetheless, given the practically limitless possible chemistry, microstructures, and properties of HEAs, these high-throughput methodologies are strongly urged for the long-term development of rapid, accurate, and robust HEAs design procedures. This essentially entails the integration of crucial micromechanical elements and criteria that function in various types of HEAs (FCC, BCC, and HCP) and are responsible for their distinct mechanical properties.
Mechanical Properties of FCC Based High Entropy Alloys
Mechanical properties of high entropy alloys are immensely derived by their compositions and microstructures. Compositions define the elastic nature and atomic-scale interaction that decides the dislocation behaviour in materials in response to the applying stress at a different time (strain rate) and temperature (high temperature to cryogenic temperature) (Raturi et al., 2021). Compositions also contribute to the phase formation and volume fraction that determine the mechanical properties. So, phase and microstructure analyses are needed to correlate the mechanical properties. Cantor alloy and their subsets can be used as model HEAs to discuss the mechanical properties. The important parameters are YS, UTS, ductility, and fracture toughness.
At first glance, the most widely used mechanical characterization is hardness; which provides the initial stage of verification of mechanical properties. The room temperature hardness value of the equiatomic CoCrFeMnNi is higher than those of the FCC metals for different grain sizes (Otto et al., 2013; Sun et al., 2017). The corresponding Hall–Petch slope is also higher than the FCC metals, indicating that the Cantor alloy offer better slip resistance than the FCC metals at the grain boundaries (Huang YC. et al., 2019). However, the hardness base prediction of the yield strength (Hardness/3) of the Cantor alloy and their lower subset is insufficient because it is just a screening process to sort out the best alloy chemistry among several compositions to carry out further characterization. Besides, the hardness is generally measured at room temperature and only a small volume of material (a few microns thickness) is involved. Eventually, with the growing research in this area, a handful of uniaxial deformation data and thermomechanical processing are now becoming obtainable to compare the mechanical properties. The Cantor alloy show an abrupt fall in the strength (Y.S decreases from 571 to 360 MPa, grain size = 4.4 μm) from 77 to 300 K (Stepanov et al., 2015), while a moderate change in yield strength is observed for temperature range 300–800 K (Sun et al., 2019). The extraordinary mechanical properties at 77 K are due to formations of nano twin that contribute to a simultaneous increase in strength and ductility because of dynamic Hall–Petch strengthening. The room temperature yield strength of Cantor alloy (171 MPa) is relatively low, but a significant amount of work hardening is observed, which contributes to an excellent tensile strength (530 MPa) and ductility (57%). This is mainly attributed to the change in the deformation mechanisms from planer slip to dislocation cell structures (George et al., 2020).
The ductility in FCC HEAs is inherently and sufficiently high from room temperature to cryogenic temperature. So, this parameter is no longer of much concern in the design strategy. However, the yield strength at room temperature in FCC HEAs is still challenging. So, the large size atoms such as V (Yin et al., 2020), Pd (Yin and Curtin, 2020) and Al (Kumar et al., 2020) have been doped in the Cantor alloy that contribute to a significant increase in yield strength. In all cases, a large misfit volume is created by the large size of these atoms causing massive solid solution hardening. Additionally, these atoms cause severe lattice distortion locally and globally, contributing to significant lattice friction to the dislocations motion. However, quantifying the lattice distortion used in the solid solution hardening model to predict the yield strength is very challenging. The conventional solid solution hardening model cannot predict the yield strength in HEAs. Even the newly developed solid solution hardening model is very efficient in predicting the yield strength in HEAs. However, they still lack quantification of lattice distortion locally, especially in HEAs, where each atom has a diverse atomic environment around its neighbour. The first principle calculation in the framework of DFT became an efficient tool to study the local atomic configuration in the alloys. Even the Varvennes solid solution hardening model that used the DFT calculation considers only the solute-dislocation interaction, not solute-solute interactions. It is important to describe here that atomic radius changes upon mixing of the elements due to the charge transfer that can alter the property locally. It would be more appropriate to develop a robust solid solution hardening model that efficiently gives an idea of selecting the type of element in conjugation with CALPHAD modelling. This can help us decide the required concentration of the selected elements in designing single phase HEAs from the strengthening perspective.
The addition of interstitial atoms such as carbon in Cantor alloy (0.5 atomic %) increases the yield strength from 165 to 225 MPa while ductility decreases by 20% (Su et al., 2019). A high degree of work hardening is observed at higher strain due to nano twin formations. The interstitial H atoms simultaneously increase the strength and ductility of Cantor alloy as it lowers the SFE, thus twin formations (Luo et al., 2017). Interestingly, it does not leads to contribute to interstitial hardening as no increase in yield strength is observed. SFE tailored engineering is well regarded in conventional alloys, which are also extended to HEAs. SFE not only depends upon the type of elements but also the compositions play a crucial role that leads to change in deformations mode from dislocation slip to TWIP (Deng et al., 2015) and TRIP (Varvenne et al., 2016). SFE engineering provides an insight into the selection of particular elements and their compositions in designing alloys systems. Liu et al. developed dual-phase (FCC + HCP) alloys by tuning the compositions through ab initio of quinary FeCoCrMnNi benefitted an increase in strength, ductility, and strain hardening (Liu et al., 2019). In another study, Nene et al. modified the SFE of quaternary FeMnCoCr by doping Si (Nene et al., 2018). The initial microstructures were FCC + HCP, and the volume fraction of HCP increases with an increase in strain. However, the calculations of SFE are very challenging. We can calculate the intrinsic stacking fault energy through experiments (TEM and X-ray powder diffractions). However, atomistic simulations can only determine extrinsic stacking fault energy and unstable twin fault energy, such as first principle calculations and molecular dynamic simulations. The computational base tools have become very efficient in predicting the compositional tuning and the trend of their physical properties, along with the experimental verifications, emerging as a powerful tool for intelligent alloy designs.
One of the most significant advantages of SFE engineering is associated with its microstructural heterogeneity through TWIP and TRIP that produces the synergic effect of strength and ductility. This microstructural heterogeneity can also be achieved without SFE engineering through microstructural entropy. Combined with CALPHAD for initial composition tuning and their phase (single and multi) and temperature prediction followed by conventional thermomechanical (rolling and heat treatment) processing, one can achieve multi modal grain distribution as a result of recovery and recrystallisation within the alloys, leading to the synergic effect of strength and ductility (Jha et al., 2021). The heterogeneous microstructure can be tailored by novel design microstructural engineering approaches such as HPT (High Pressure Torsion) and FSP (Friction Stir Processing) engineering. Nene et al. reported a drastic decrease in grain size from 100 to 6 µm of dual-phase Fe50Mn30Co10Cr10, thus increasing yield strength by two times when subjected to friction stir processing (Nene et al., 2017). The FSP not only contributed to the grain size reduction but also improved the FCC and HCP distribution that led to an increase in yield strength due to the presence of the second phase along with tensile strength and ductility. Thus, it is essential to use phase-field modelling (Moelans et al., 2008) to predict the microstructure and phase stability at different time, temperatures, compositions and length scales that can predict the exact conditions of the heat treatment process.
Phase-field modelling can also be used to design the precipitation-hardenable alloys to achieve good strength. In general, the alloys having FCC structure are quite soft, by inherent precipitation of the second phase in as - cast state (Shim et al., 2022) or can be precipitated in the matrix upon heat treatment, which can contribute to the pronounce effect of strengthening. Owing to the slow diffusion kinetics (but still under passionate debate), HEAs can form nano precipitate/the second phase in the as-cast state (Tong et al., 2005; Shun and Du, 2009; Singh et al., 2011; Lu et al., 2015), upon heat treatment for a prolonged period at 700°C (Tsai et al., 2013a) and even homogenized at 1,100°C followed by furnace cooling (Tsai et al., 2013b).
In this regard, many groups aimed to design HEAs by intentionally incorporated the second phase with controlled thermomechanical processing and tailored morphology to achieve the better combination of mechanical properties. He et al. achieved excellent tensile properties (YS = 645 MPa, UTS = 1,094 MPa, and ductility = 39%) as a result of nanosized reinforced phase, L12 Ni3(Ti, Al) in FCC matrix by introducing Ti and Al into the FeNiCoCr HEAs (He et al., 2016). Further thermomechanical process of the same alloys showed the yield strength of 1 GPa with elongations of 17%, which outperformed the existing advance high strength steels. In other studies, the AlCoCrxFeMo0.5Ni HEAs show a high hot hardness greater than Ni-base superalloys due to the presence of the sigma phase, which contributes to the resistance to softening along with the B2 phase, which imparts the strength (Hsu et al., 2011). Thus, it is necessary to use such a design strategy that predicts these phases and their morphology at different temperature and time scales that narrows down the screening process by reducing the time, which can be achieved by the phase-field modelling effectively in hybridization mode (with ICME, CALPHAD, DFT, and ML, etc.)
The phase-field modelling would be very effective in combination with CALPHAD modelling, DFT and ML in providing an insight into the type of phase, morphology (shape and size), volume fractions and their distributions with respect to the temperature and the compositions. It would be beneficiary if phase-field modelling could be coupled with some finite element method such as Ansys and COMSOL to predict the stress distribution so one can infer that passes during rolling and friction stir processing, the number of turns and load in HPT, can ultimately lead to desired hierarchical microstructures and very fast screening.
However, the novel design technique is always constrained by the cost and, more importantly, the geometry of the specimen. A recent study shows that the research is shifting towards in-situ hierarchical microstructural engineering in the as-cast state. Shim et al. reported that the in-situ hierarchical structure of CrFeNiMn0.5Cu0.5 shows the excellent combination of tensile strength and ductility (Shim et al., 2022).
Mechanical Properties of BCC High Entropy Alloys
Similar to FCC HEAs, alloy designing of refractory HEAs also need to utilize theoretical modelling and simulation methods to effectively search and discover the chemical composition and microstructure of the alloy to optimize the performance in the desired applications. However, for applicability in the high-temperature structural domain, the refractory HEAs must have distinct high resistance properties to thermal softening. Hence, the constituent elements in the refractory HEAs are selected using high-throughput CALPHAD and phase-field simulation considering the resultant strengthening mechanism, the deformation mechanism and phase transformation by microstructure modelling, and molecular dynamic (MD) simulation at elevated temperature. For instance, Steingrimsson et al. (2021) present a method of predicting the temperature-dependent ultimate strengths of BCC HEAs by introducing a bilinear log model to account for the underlying physics, particularly diffusion processes required to initiate phase transformations. Further, the evolution of the HEAs field from single phase microstructure to a large variety of architectural morphologies resultant from the order/disorder transformation, spinodal decomposition, precipitation, and massive transformation increase the need for a data-driven approach such as CALPHAD, first principles, molecular dynamics, finite element method, etc. in the designing of refractory HEAs.
In refractory HEAs, the performance of alloy and its mechanical properties are affected profoundly by its constituent elements. The atomic interactions of different elements tailor the elastic properties and dislocation behaviour. Zao et al. developed a model to interpret the contributions of lattice friction in material strengthening by conducting a systematic tensile test of Nb-based refractory HEAs (NbTiTaZrHf) (Zhao et al., 2019). The quantitative evaluation predicted that the lattice distortion does not increase directly with an increasing number of constituent elements. Instead, the ternary NbZrHf shows higher lattice distortion than the quinary (NbTiTaZrHf). The study can be extended further to use the model efficiently with machine learning (ML) algorithms and explore the effect of lattice distortion in the other alloy system in the designing phase. Also, the observed lattice distortion in the Nb-based bcc refractory HEAs is far more significant than the Ni-based FCC HEAs (NiCoCrFeMn) due to the smaller dislocation width of the constituent refractory elements. Thus, dislocation width can also act as a parameter to predict the alloy behaviour in the alloy design. Similarly, the interstitial elements deform alloy lattice and impede the dislocation motion that strengthens the alloy and affects its mechanical properties. Oxygen and nitrogen are commonly used in refractory HEAs and are found to promote strengthening when oxygen is added in the BCC ZrTiHfNb0.5Ta0.5 (Chen et al., 2018), and oxygen and nitrogen are added in the BCC TiZrHfNb HEA (Lei et al., 2018).
Dislocation mechanisms are crucial for modifying the mechanical properties for the desired applications. A better understanding of all active deformation mechanisms and their interaction will be vital to design refractory HEAs with enhanced mechanical properties. Couzinié et al. (2015) (Raturi et al., 2022) examined the dislocation structure in deformed TiZrHfNbTa refractory HEAs and recognized that the motion of less mobile screw dislocation (b = a/2 <111>) was the predominant deformation mechanism initially. Later, Rao et al. (2017) investigated Co16.67Fe36.67Ni16.67Ti30 refractory HEAs and reported that the nonplanar core structure of a/2 <111> screw dislocation is responsible for higher lattice resistance or low mobility of the screw dislocation. The widespread acceptance of refractory HEAs as a structural material is also restricted by its limited plasticity. Although, incorporating the “metastability-engineering” approach such as TRIP in designing refractory HEAs improves the ductility without compromising the strength. For example, reducing the BCC stabilizer element Ta in the BCC TaxHfZrTi HEAs system result into TRIP effect with {110}BCC//{0002}HCP crystallographic orientation. Dynamic strain/stress partitioning between the BCC and HCP phases introduces the strain-hardening effect, which suppresses early cracking and contributes to continuous plastic deformation in the BCC matrix (Huang et al., 2017; Lilensten et al., 2017).
Compared to the HEAs, the commercial superalloy having medium to high configurational entropies are somewhat mature multicomponent alloy systems. Thus, the knowledge of commercial superalloys can provide clues for the designing of refractory high entropy superalloys (HES). Refractory HES containing Al with refractory elements have disordered body-centred cubic (BCC) and ordered B2 phases similar to superalloys γ-γ́ microstructure (Senkov et al., 2014). However, due to the lack of understanding of the reaction pathway of BCC + B2 microstructure, most of the alloy systems produce an inverted microstructure of discrete BCC phase within a continuous B2 phase. A recent study by Soni et al. (2020) on Al0.5NbTa0.8Ti1.5V0.2Zr.reported that the long-term annealing at 600°C phase leads to the inversion of microstructure into continuous BCC phase with discrete B2 precipitates. However, through better alloy designing, the process of flipping the inverted microstructure needs to be made universal, along with addressing a lot of enduring challenges in refractory superalloys.
Mechanical Properties of HCP High Entropy Alloys
Unlike the FCC and BCC, the HCP materials show the different characteristics in terms of lattice site occupations, anisotropy in microstructure, a limited number of slip systems. Therefore, they represent distinct microstructures, properties, and micro-mechanism than those of the FCC and BCC materials. Moreover, the c/a ratio in HCP materials plays a decisive role in the deformation mechanism. The involvement of non-basal pyramidal (c+a) slip and twinning mode is crucial to sustaining the higher strain rate along the c axis depending on c/a ratios. In contrast to the conventional HCP metals (Ti or Mg) where the c/a ratio is intrinsic in nature and highly depends on alloys compositions and does not vary during processing and deformations: the HEAs show unprecedented behaviour of change in the c/a ratio, which is very sensitive to deformation mode. Sinha et al. studied the change in c/a ratios when Co-Cr-Fe-Mn-Si alloys were subjected to friction stir processing leading to multiple deformation mechanisms with a better combination of strength and ductility (Sinha et al., 2019). In another study, Tracy et al. developed an equiatomic CoCrFeMnNi with FCC + HCP phases on the applications of the pressures. The HCP phase starts to form at a pressure greater than 14.6 GPa and is retained throughout the pressure range (14–56 GPa), while c/a ratios also change during pressure changes, unlike the conventional metals (Tracy et al., 2017).
However, the studies mentioned above are based on the deformation-induced technique base HCP phase in coexistence with the FCC phase. More importantly, they are not single phase HCP or HCP based alloys that form during synthesis. In this context, Takeuchi et al. designed a single-phase hcp high entropy alloys (Ir26Mo20Rh22.5Ru20W11.5 and Ir25.5Mo20Rh20Ru25W9.5) (Takeuchi et al., 2019) using a sandwich strategy where authors refer to a binary phase diagram of the constituents elements near equiatomic compositions that stabilizes the hcp phase in conjugation with the VEC of each element. Moreover, they successfully cast the alloys at ambient conditions (room temperature and atmospheric pressure). The work by Takeuchi et al. indicates that the conventional alloys strategy is still effective for designing the alloys at the first steps, especially for HCP base HEAs, whose exploration is negligible in comparison to FCC and BCC HEAs. In another study, Gao et al. developed HCP-based single-phase CoFeReRu high entropy alloys using the combinatorial strategy of CALPHD modelling and AIMD (ab initio molecular dynamic simulations) (Gao et al., 2016). The CALPHAD modelling helps out to understand the binary phase diagram of constituent elements in a quick manner that is further used to extrapolate the ternary and quaternary phase diagram as a screening process. On the other hand, the AIMD simulations provided quantitative data such as diffusion constant temperature without experimental efforts, thus saving time and energy. Rogal et al. developed rare earth element-based Hf25Sc25Ti25Zr25 HCP based high entropy alloys where DFT calculations were used to predict the phase and further verified with experiments (Rogal et al., 2016). The alloys show the dual HCP phase where the other HCP phase is minimal (not exceeding 6% by volume). The compressive strength of these HCP high entropy alloys is 1801 MPa and a high strain of 24%, while yield strength is 698 MPa compared to conventional Ti-Mo-Al-V alloys where compressive strength is 1,101 MPa. This is mainly attributed to massive solid solution hardening due to lattice distortions.
However, the reports on designing HCP-based high entropy alloys and scientific understanding of their deformation behaviour are negligible compared to the FCC and BCC-based high entropy alloys. On the other hand, the uses of pressure-assisted deformations and expansive rare earth elements limit the synthesis of HCP-based high-entropy alloys and make them very challenging. Figure 3 represents the mechanical properties of different High entropy Alloys along with the phases.
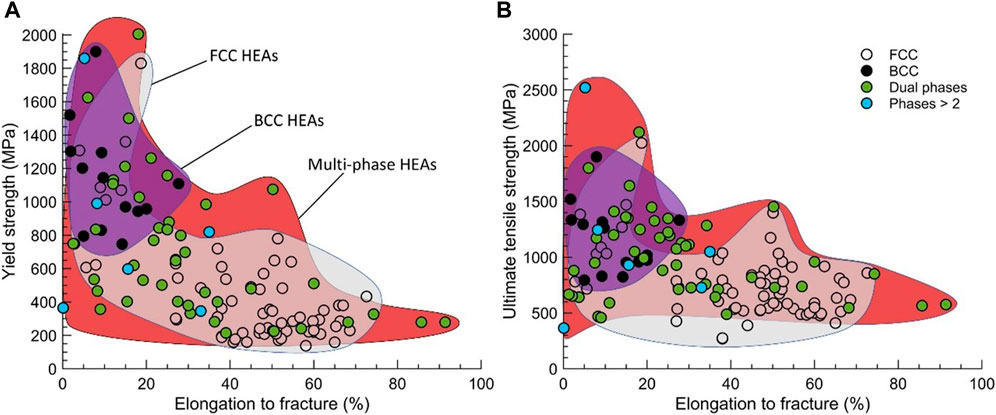
FIGURE 3. Property maps of (A) tensile yield strength and (B) ultimate tensile strength versus elongation to fracture of HEAs, grouped by phase structures (Li et al., 2021).
Mechanical Properties of Multicomponent Metallic Glasses
Unlike crystalline alloys, where the mechanical characteristics rely heavily on their crystalline structures, multicomponent metallic glasses (MMGs) display distinctive structural properties and non-conventional deformation processes due to their short range ordered structure and metastable state (Zhang et al., 2014; Bajpai et al., 2020). Nonetheless, the exceptional characteristics of MMGs are coupled with a lack of homogenous plastic deformation in the absence of dislocation-mediated crystallographic slip (Inoue, 2000). When metallic glasses deform under ambient conditions, the fundamental mechanism is the local atom rearrangement capable of accommodating shear stresses (Schuh et al., 2007). This involves the creation and propagation of the highly-localized shear bands in which a large amount of plastic strain accumulates in a minimal region (i.e., 10–20 nm). These localized regions are termed as Shear Transformation Zones (STZs). On the macroscopic scale, plastic deformation of metallic glasses is simply a biased build-up of local stresses caused by the activity of STZs and the redistribution of free volumes (Trexler and Thadhani, 2010). The working conditions profoundly affect the deformation micro-mechanisms in metallic glasses. The effect of temperature on mechanical properties of metallic glasses is determined by the dynamic equilibrium established between the formation of free volume owing to strain localization and its annihilation due to diffusional rearrangements as temperature rises (Trexler and Thadhani, 2010). Consequently, the deformation mechanism shifts from inhomogeneous to homogeneous flow as the glass transition temperature approaches. Thus, low strain rates and higher temperatures cause more widespread and sustained flow with elongations more than 100% before failure. The phenomena of stress- or pressure-induced phase transitions in MMGs is less well understood when compared to temperature dependent deformation behaviour. Nanocrystals generated by induced stress and pressure have been reported during nanoindentation and high-stress torsion straining of MMGs. The crystallisation process has been ascribed to flow dilatation in shear bands and dramatically increased diffusional mobility, resulting in the development of ordered atomic arrangements. Understanding these deformation and failure processes in metallic glasses can provide an insight into the design of alloy compositions and microstructures that can provide a combination of high strength, tensile ductility, and outstanding fracture toughness.
In this context, data driven approaches such as ML and atomistic simulations (MD and DFT) can be used to predict and design the property space for MMGs. ML has especially come to the fore in recent times to evaluate the mechanical properties of MMGs. Jeon et al. (2021) used an inverse design method combining an artificial neural network (ANN) model and particle swarm optimization (PSO) model to design Fe-based MMGs with excellent thermal and mechanical properties. Xiong et al. (2020) estimated the elastic properties, i.e., bulk and shear modulus, of MMGs using several linear and non-linear ML models. Li et al. (2022) used an ML approach to predict the magnetic properties of Fe-based MGs with consideration of glass forming ability. Xiong et al. (2019) used a Support Vector Regression (SVR) and Gaussian Process (GP) model to estimate the elastic modulus of MMGS in a separate study. Such advancements in predictive modelling and high throughput experimentation technology have permitted the development of several complicated Zr-, Ti-, Cu-, and Fe-based metallic glasses in dimensions large enough to allow detailed mechanical property research. In order to broaden the property design space for MMGs, an integration of atomistic simulation tools and ML is paramount, which can enable the incorporation of several micro-mechanical aspects of deformation, discussed earlier, into alloy design for a more efficient design of high performance MMGs. Figure 4 represents the high throughput alloy design and development strategy.
Challenges and Future Perspectives
High Entropy alloys with huge compositional space for designing novel materials require combinatorial and high throughput synthesis and processing methods to explore the suitable alloys chemistry. Their scientific understanding of the phase formations, phase stability, and microstructures at different time and temperature scales are the biggest challenges facing the material science community in carrying forward research on HEAs. The present scientific knowledge and understanding of the materials design are based on the collective effort of theoretical and experimental findings, which is more than 5 decades old. This includes thermodynamic data, phase transformations, defects engineering, diffusion data, kinetics model, deformation mechanism under different loading, microstructures, and property correlation as a function of chemistry. Nonetheless, this data is usually acquired for the materials based on principal elements and extrapolated to the HEAs that limit the benefits and scientific understanding of this new class of materials. For instance, the B2 precipitate can serve as an important strengthening phase in high-temperature materials like refractory HEAs. This phase is present in several alloys and can be used as a key parameter for designing a suitable refractory high entropy alloy for high-temperature applications using the CALPHAD model. However, the presence of B2 phases in the binary phase diagram involving refractory metals has not been observed (Miracle, 2019). So, the new data and thermodynamic model are essential in predicting the B2 phase formations to accelerate the progress in this direction. The extensive study primarily focuses on the study of mechanical properties of alloys such as CoCrFeMnNi and its lower subsets at cryogenic and room temperature and AlxCoCuCrFeNi (Santodonato et al., 2015). However, other astonishing properties and scientific aspects may be achieved by careful investigations of thermodynamic data or diffusional data or defect structures which can be helpful to accelerate the design strategy. The high throughput screening is the key parameter of this strategy. For example, the hardness test is an rapid screening method in designing structural alloys. However, the same materials show a greater degree of variations in properties when subjected to the conventional test, while the method with a great degree of accuracy is very slow and only limited to the few alloys system. The theoretical approach for the rapid screening of the alloy design using various computational tools such as first principle calculation, machine learning, and deep learning are becoming popular in predicting the phase and their stability; mechanical properties in the correlation of microstructures. In addition, the accuracy of theoretical screening depends upon the aspects of methodology and framework adopted. For example, the first principle calculation in the framework of DFT using SQS structures requires a large number of atoms in predicting the property locally and globally, which, in turn demands a large time for structures relaxations, slowing the screening process. ML tool, though, is very fast, but the lack of available data on HEAs limits its accuracy in predicting the properties. The lack of data for the development of new physical models is the biggest challenge for future scientific progress of these new classes of materials. In this regard, the National Science and Technology Council have come forward and established the Materials Genome Initiative (Interagency Group on Advanced Materials, 2011), proposing a common platform of data sharing and analyses that will provide knowledge and pieces of information to scientist and engineers for designing the new advanced materials. The main aim of this proposition is to reduce the time for new material discovery, development, and manufacturing more efficiently and economically through computational tools, data management and integrated approach materials science and engineering.
The alloy design based on MD, DFT and CALPHAD is an effective method for educated sampling of compositional space compared to the experimental method. However, there is a gap between the HEAs phase compositions obtained from experimental methods and the CALPHAD predictions. This deviation is because of the incomplete thermodynamic databases (Zhang et al., 2018). The higher order interaction effects beyond ternary are not available in CALPHAD, which can be obtained through high-quality experiments and theoretical methods. Therefore, there is a need for a materials library or database for HEAs, which will combine all the aspects of chemistry, processing, and property space. One of the methods to rapidly produce material library is the use of additive manufacturing process, with controlled composition and microstructure gradient, combined with computation methods. This library can then be used to develop more reliable databases to predict phases and their properties in HEAs using the CALPHAD method. The theories, models, and methods for traditional alloys cannot directly be applied to HEAs because there is no clear definition of solute or solvent entities in the latter. The correlation between chemistry, processing and property is required for this new field of materials. The materials library will help in an improved fundamental understanding of thermodynamics and mechanical behaviour of the alloys as well as formulate theories and models for such materials. The studies on HEAs have shown that this field has the potential to overcome the bottlenecks of traditional alloys and can be utilized in critical conditions. The potential applications include material for hydrogen storage, diffusion barriers, structural materials, cutting tools, etc. However, HEAs are not yet explored commercially. The field will remain open only for scientific curiosity without commercial success and practical use. Therefore, it is crucial to advance from lab scale studies towards designing prototypes to finally commercialization of HEAs. The challenge with the commercialization of HEAs is the expensive raw materials used to fabricate the alloys. Cost reduction from raw materials as well as processing needs to be considered without compromising the properties.
Summary
High entropy alloys have opened a vast compositional and microstructural space with novel properties. However, the traditional alloy designing strategy is not applicable for HEAs. Various design tools like AIMD simulations, CALPHAD modelling, machine learning (ML) need to be combined to accelerate the discovery of high-performance HEAs systems. In-situ hierarchical microstructural engineering, metastability engineering, advanced ML tool like neural networks, heterogeneous microstructural engineering techniques like conventional rolling, ECAP along with new techniques such as HPT and FSP are some of the approaches that can be incorporated in the HEAs field for better screening of alloys with target properties. The biggest challenge for the future scientific progress for these materials is the lack of data for developing new physical models. In this regard, Material Genome Initiative (MGI) provides a common platform for HEAs data sharing, which can be utilized by scientists and engineers to design advanced materials in a time and cost effective manner. This kind of materials library combines chemistry–processing–property space of HEAs and help in formulating new theories, models and methods. The real time application of HEAs is also a challenge, for which prototype needs to be developed, which will finally lead to commercialization of these alloys.
Data Availability Statement
The original contributions presented in the study are included in the article/supplementary materials, further inquiries can be directed to the corresponding authors.
Author Contributions
JK, SJ, AR, and AB have drafted the manuscript, made corrections, and prepared figures NG and KB have supervised them and corrected the manuscript.
Conflict of Interest
The authors declare that the research was conducted in the absence of any commercial or financial relationships that could be construed as a potential conflict of interest.
Publisher’s Note
All claims expressed in this article are solely those of the authors and do not necessarily represent those of their affiliated organizations, or those of the publisher, the editors and the reviewers. Any product that may be evaluated in this article, or claim that may be made by its manufacturer, is not guaranteed or endorsed by the publisher.
Acknowledgments
The authors would like to thank the funding agencies, SERB-DST, ISRO, BRNS, for funding and IIT Kanpur for characterization facilities to conduct research on high entropy alloys.
References
Abdoon Al-Shibaany, Z. Y., Alkhafaji, N., Al-Obaidi, Y., and Atiyah, A. A. (2020). Deep Learning-Based Phase Prediction of High-Entropy Alloys. IOP Conf. Ser. Mat. Sci. Eng. 987, 012025. doi:10.1088/1757-899x/987/1/012025
Bajpai, A., Bhatt, J., Biswas, K., and Gurao, N. P. (2020). A New Perspective to Thermodynamical Designing of High Entropy Bulk Metallic Glasses (HE-BMGs). Phys. B Condens. Matter 595, 412350. doi:10.1016/j.physb.2020.412350
Bhadeshia, H. K. D. H. (2002). TRIP-assisted Steels? ISIJ Int. 42, 1059–1060. doi:10.2355/isijinternational.42.1059
Biswas, K., Yeh, J.-W., Bhattacharjee, P. P., and Dehosson, J. T. M. (2020). High Entropy Alloys: Key Issues under Passionate Debate. Scr. Mater. 188, 54–58. doi:10.1016/j.scriptamat.2020.07.010
Butler, T. M., Chaput, K. J., Dietrich, J. R., and Senkov, O. N. (2017). High Temperature Oxidation Behaviors of Equimolar NbTiZrV and NbTiZrCr Refractory Complex Concentrated Alloys (RCCAs). J. Alloys Compd. 729, 1004–1019. doi:10.1016/j.jallcom.2017.09.164
Chen, H.-L., Mao, H., and Chen, Q. (2016). Calphad Database Development for HEMs. United Kingdom: Calphad.
Chen, S. Y., Tong, Y., Tseng, K.-K., Yeh, J.-W., Poplawsky, J. D., Wen, J. G., et al. (2019). Phase Transformations of HfNbTaTiZr High-Entropy Alloy at Intermediate Temperatures. Scr. Mater. 158, 50–56. doi:10.1016/j.scriptamat.2018.08.032
Chen, Y.-T., Chang, Y.-J., Murakami, H., Gorsse, S., and Yeh, A.-C. (2020). Designing High Entropy Superalloys for Elevated Temperature Application. Scr. Mater. 187, 177–182. doi:10.1016/j.scriptamat.2020.06.002
Chen, Y., Li, Y., Cheng, X., Xu, Z., Wu, C., Cheng, B., et al. (2018). Interstitial Strengthening of Refractory ZrTiHfNb0.5Ta0.5Ox (X = 0.05, 0.1, 0.2) High-Entropy Alloys. Mater. Lett. 228, 145–147. doi:10.1016/j.matlet.2018.05.123
Couzinié, J. P., Lilensten, L., Champion, Y., Dirras, G., Perrière, L., and Guillot, I. (2015). On the Room Temperature Deformation Mechanisms of a TiZrHfNbTa Refractory High-Entropy Alloy. Mater. Sci. Eng. A 645, 255–263.
Curtarolo, S., Morgan, D., and Ceder, G. (2005). Accuracy of Ab Initio Methods in Predicting the Crystal Structures of Metals: A Review of 80 Binary Alloys. Calphad 29, 163–211. doi:10.1016/j.calphad.2005.01.002
Curtarolo, S., Setyawan, W., Hart, G. L. W., Jahnatek, M., Chepulskii, R. V., Taylor, R. H., et al. (2012). AFLOW: An Automatic Framework for High-Throughput Materials Discovery. Comput. Mater. Sci. 58, 218–226. doi:10.1016/j.commatsci.2012.02.005
De Cooman, B. C., Estrin, Y., and Kim, S. K. (2018). Twinning-induced Plasticity (TWIP) Steels. Acta Mater. 142, 283–362. doi:10.1016/j.actamat.2017.06.046
Deng, Y., Tasan, C. C., Pradeep, K. G., Springer, H., Kostka, A., and Raabe, D. (2015). Design of a Twinning-Induced Plasticity High Entropy Alloy. Acta Mater. 94, 124–133. doi:10.1016/j.actamat.2015.04.014
Feng, R., Feng, B., Gao, M. C., Zhang, C., Neuefeind, J. C., Poplawsky, J. D., et al. (2021). Superior High‐Temperature Strength in a Supersaturated Refractory High‐Entropy Alloy. Adv. Mat. 33, 2102401. doi:10.1002/adma.202102401
Firstov, S. A., Gorban’, V. F., Krapivka, N. A., Karpets, M. V., and Kostenko, A. D. (2017). Wear Resistance of High-Entropy Alloys. Powder Metall. Met. Ceram. 56, 158–164. doi:10.1007/s11106-017-9882-8
Furukawa, M., Horita, Z., Nemoto, M., and Langdon, T. G. (2001). Review: Processing of Metals by Equal-Channel Angular Pressing. J. Mater. Sci. 36, 2835–2843. doi:10.1023/a:1017932417043
Gao, M., and Alman, D. (2013). Searching for Next Single-phase High-Entropy Alloy Compositions. Entropy 15, 4504–4519. doi:10.3390/e15104504
Gao, M. C., Zhang, B., Guo, S. M., Qiao, J. W., and Hawk, J. A. (2016). High-Entropy Alloys in Hexagonal Close-Packed Structure. Metall Mat Trans A 47, 3322–3332. doi:10.1007/s11661-015-3091-1
George, E. P., Curtin, W. A., and Tasan, C. C. (2020). High Entropy Alloys: A Focused Review of Mechanical Properties and Deformation Mechanisms. Acta Mater. 188, 435–474. doi:10.1016/j.actamat.2019.12.015
George, E. P., Raabe, D., and Ritchie, R. O. (2019). High-entropy Alloys. Nat. Rev. Mater 4, 515–534. doi:10.1038/s41578-019-0121-4
Guo, S., and Liu, C. T. (2011). Phase Stability in High Entropy Alloys: Formation of Solid-Solution Phase or Amorphous Phase. Prog. Nat. Sci. Mater. Int. 21, 433–446.
Guo, S., Ng, C., Lu, J., and Liu, C. T. (2011). Effect of Valence Electron Concentration on Stability of Fcc or Bcc Phase in High Entropy Alloys. J. Appl. Phys. 109, 103505. doi:10.1063/1.3587228
He, J. Y., Wang, H., Huang, H. L., Xu, X. D., Chen, M. W., Wu, Y., et al. (2016). A Precipitation-Hardened High-Entropy Alloy with Outstanding Tensile Properties. Acta Mater. 102, 187–196.
Hsu, C.-Y., Juan, C.-C., Wang, W.-R., Sheu, T.-S., Yeh, J.-W., and Chen, S.-K. (2011). On the Superior Hot Hardness and Softening Resistance of AlCoCrxFeMo0.5Ni High-Entropy Alloys. Mater. Sci. Eng. A 528, 3581–3588. doi:10.1016/j.msea.2011.01.072
Huang, H., Wu, Y., He, J., Wang, H., Liu, X., An, K., et al. (2017). Phase‐Transformation Ductilization of Brittle High‐Entropy Alloys via Metastability Engineering. Adv. Mat. 29, 1701678. doi:10.1002/adma.201701678
Huang, W., Martin, P., and Zhuang, H. L. (2019a). Machine-learning Phase Prediction of High-Entropy Alloys. Acta Mater. 169, 225–236. doi:10.1016/j.actamat.2019.03.012
Huang, Y. C., Su, C. H., Wu, S. K., and Lin, C. (2019b). A Study on the Hall-Petch Relationship and Grain Growth Kinetics in FCC-Structured High/Medium Entropy Alloys. Entropy (Basel) 21. doi:10.3390/e21030297
Inoue, A. (2000). Stabilization of Metallic Supercooled Liquid and Bulk Amorphous Alloys. Acta Mater. 48, 279–306. doi:10.1016/s1359-6454(99)00300-6
Interagency Group on Advanced Materials (2011). Materials Genome Initiative for Global Competitiveness. Washington, D.C.: National Science and Technology Council.
Jeon, J., Seo, N., Kim, H.-J., Lee, M.-H., Lim, H.-K., Son, S. B., et al. (2021). Inverse Design of Fe-Based Bulk Metallic Glasses Using Machine Learning. Metals 11. doi:10.3390/met11050729
Jha, S. R., Biswas, K., and Gurao, N. P. (2021). Achieving High Strength and Ductility in Equimolar FeMnNi Medium Entropy Alloy by Tuning Microstructural Entropy. Mater. Sci. Eng. A 826, 141965.
Kaufmann, K., and Vecchio, K. S. (2020). Searching for High Entropy Alloys: A Machine Learning Approach. Acta Mater. 198, 178–222. doi:10.1016/j.actamat.2020.07.065
Klimenko, D., Stepanov, N., Li, J., Fang, Q., and Zherebtsov, S. (2021). Machine Learning-Based Strength Prediction for Refractory High-Entropy Alloys of the Al-Cr-Nb-Ti-V-Zr System. Materials 14, 7213. doi:10.3390/ma14237213
Kumar, J., Gurao, N. P., and Biswas, K. (2020). The Effect of Aluminium Addition on Solid Solution Strengthening in CoCrFeMnNi High Entropy Alloy: Experiment and Modelling. Cambridge: Cambridge University Press.
Kumar, N., Tiwary, C. S., and Biswas, K. (2018). Preparation of Nanocrystalline High-Entropy Alloys via Cryomilling of Cast Ingots. J. Mater Sci. 53, 13411–13423. doi:10.1007/s10853-018-2485-z
Lederer, Y., Toher, C., Vecchio, K. S., and Curtarolo, S. (2018). The Search for High Entropy Alloys: A High-Throughput Ab-Initio Approach. Acta Mater. 159, 364–383. doi:10.1016/j.actamat.2018.07.042
Lei, Z., Liu, X., Wu, Y., Wang, H., Jiang, S., Wang, S., et al. (2018). Enhanced Strength and Ductility in a High-Entropy Alloy via Ordered Oxygen Complexes. Nature 563, 546–550. doi:10.1038/s41586-018-0685-y
Li, J. L., Li, Z., Wang, Q., Dong, C., and Liaw, P. K. (2020). Phase-field Simulation of Coherent BCC/B2 Microstructures in High Entropy Alloys. Acta Mater. 197, 10–19. doi:10.1016/j.actamat.2020.07.030
Li, W., Liaw, P. K., and Gao, Y. (2018a). Fracture Resistance of High Entropy Alloys: A Review. Intermetallics 99, 69–83. doi:10.1016/j.intermet.2018.05.013
Li, W., Xie, D., Li, D., Zhang, Y., Gao, Y., and Liaw, P. K. (2021). Mechanical Behavior of High-Entropy Alloys. Prog. Mater. Sci. 118, 100777. doi:10.1016/j.pmatsci.2021.100777
Li, X., Shan, G., and Shek, C. H. (2022). Machine Learning Prediction of Magnetic Properties of Fe-Based Metallic Glasses Considering Glass Forming Ability. J. Mater. Sci. Technol. 103, 113–120. doi:10.1016/j.jmst.2021.05.076
Li, Z., Körmann, F., Grabowski, B., Neugebauer, J., and Raabe, D. (2017). Ab Initio assisted Design of Quinary Dual-phase High-Entropy Alloys with Transformation-Induced Plasticity. Acta Mater. 136, 262–270. doi:10.1016/j.actamat.2017.07.023
Li, Z., Ludwig, A., Savan, A., Springer, H., and Raabe, D. (2018b). Combinatorial Metallurgical Synthesis and Processing of High-Entropy Alloys. J. Mat. Res. 33, 3156–3169. doi:10.1557/jmr.2018.214
Lilensten, L., Couzinié, J.-P., Bourgon, J., Perrière, L., Dirras, G., Prima, F., et al. (2017). Design and Tensile Properties of a Bcc Ti-Rich High-Entropy Alloy with Transformation-Induced Plasticity. Mater. Res. Lett. 5, 110–116. doi:10.1080/21663831.2016.1221861
Liu, S. F., Wu, Y., Wang, H. T., Lin, W. T., Shang, Y. Y., Liu, J. B., et al. (2019). Transformation-reinforced High-Entropy Alloys with Superior Mechanical Properties via Tailoring Stacking Fault Energy. J. Alloys Compd. 792, 444–455. doi:10.1016/j.jallcom.2019.04.035
Lu, Z. P., Wang, H., Chen, M. W., Baker, I., Yeh, J. W., Liu, C. T., et al. (2015). An Assessment on the Future Development of High-Entropy Alloys: Summary from a Recent Workshop. Intermetallics 66, 67–76. doi:10.1016/j.intermet.2015.06.021
Luo, H., Li, Z., and Raabe, D. (2017). Hydrogen Enhances Strength and Ductility of an Equiatomic High-Entropy Alloy. Sci. Rep. 7, 9892. doi:10.1038/s41598-017-10774-4
Ma, Z. Y. (2008). Friction Stir Processing Technology: A Review. Metall Mat Trans A 39, 642–658. doi:10.1007/s11661-007-9459-0
Machaka, R. (2021). Machine Learning-Based Prediction of Phases in High-Entropy Alloys. Comput. Mater. Sci. 188, 110244. doi:10.1016/j.commatsci.2020.110244
Menou, E., Toda-Caraballo, I., Rivera-Díaz-del-Castillo, P. E. J., Pineau, C., Bertrand, E., Ramstein, G., et al. (2018). Evolutionary Design of Strong and Stable High Entropy Alloys Using Multi-Objective Optimisation Based on Physical Models, Statistics and Thermodynamics. Mater. Des. 143, 185–195. doi:10.1016/j.matdes.2018.01.045
Miracle, D. B. (2019). High Entropy Alloys as a Bold Step Forward in Alloy Development. Nat. Commun. 10, 1805. doi:10.1038/s41467-019-09700-1
Miracle, D. B., and Senkov, O. N. (2017). A Critical Review of High Entropy Alloys and Related Concepts. Acta Mater. 122, 448–511. doi:10.1016/j.actamat.2016.08.081
Moelans, N., Blanpain, B., and Wollants, P. (2008). An Introduction to Phase-Field Modeling of Microstructure Evolution. Calphad 32, 268–294. doi:10.1016/j.calphad.2007.11.003
Nene, S. S., Frank, M., Liu, K., Mishra, R. S., Mcwilliams, B. A., and Cho, K. C. (2018). Extremely High Strength and Work Hardening Ability in a Metastable High Entropy Alloy. Sci. Rep. 8, 9920. doi:10.1038/s41598-018-28383-0
Nene, S. S., Liu, K., Frank, M., Mishra, R. S., Brennan, R. E., Cho, K. C., et al. (2017). Enhanced Strength and Ductility in a Friction Stir Processing Engineered Dual Phase High Entropy Alloy. Sci. Rep. 7, 16167. doi:10.1038/s41598-017-16509-9
Otto, F., Dlouhý, A., Somsen, C., Bei, H., Eggeler, G., and George, E. P. (2013). The Influences of Temperature and Microstructure on the Tensile Properties of a CoCrFeMnNi High-Entropy Alloy. Acta Mater. 61, 5743–5755. doi:10.1016/j.actamat.2013.06.018
Rao, S. I., Varvenne, C., Woodward, C., Parthasarathy, T. A., Miracle, D., Senkov, O. N., et al. (2017). Atomistic Simulations of Dislocations in a Model BCC Multicomponent Concentrated Solid Solution Alloy. Acta Mater. 125, 311–320. doi:10.1016/j.actamat.2016.12.011
Raturi, A., Aditya C, J., Gurao, N. P., and Biswas, K. (2019). ICME Approach to Explore Equiatomic and Non-equiatomic Single Phase BCC Refractory High Entropy Alloys. J. Alloys Compd. 806, 587–595. doi:10.1016/j.jallcom.2019.06.387
Raturi, A., Biswas, K., and Gurao, N. P. (2021). A Mechanistic Perspective on the Kinetics of Plastic Deformation in FCC High Entropy Alloys: Effect of Strain, Strain Rate and Temperature. Scr. Mater. 197, 113809. doi:10.1016/j.scriptamat.2021.113809
Raturi, A., Biswas, K., and Gurao, N. P. (2022). Elastic and Plastic Anisotropy in a Refractory High Entropy Alloy Utilizing Combinatorial Instrumented Indentation and Electron Backscatter Diffraction. J. Alloys Compd. 896, 162902. doi:10.1016/j.jallcom.2021.162902
Rogal, Ł., Czerwinski, F., Jochym, P. T., and Litynska-Dobrzynska, L. (2016). Microstructure and Mechanical Properties of the Novel Hf25Sc25Ti25Zr25 Equiatomic Alloy with Hexagonal Solid Solutions. Mater. Des. 92, 8–17. doi:10.1016/j.matdes.2015.11.104
Santodonato, L. J., Zhang, Y., Feygenson, M., Parish, C. M., Gao, M. C., Weber, R. J. K., et al. (2015). Deviation from High-Entropy Configurations in the Atomic Distributions of a Multi-Principal-Element Alloy. Nat. Commun. 6, 5964. doi:10.1038/ncomms6964
Schuh, C., Hufnagel, T., and Ramamurty, U. (2007). Mechanical Behavior of Amorphous Alloys. Acta Mater. 55, 4067–4109. doi:10.1016/j.actamat.2007.01.052
Senkov, O. N., Senkova, S. V., and Woodward, C. (2014). Effect of Aluminum on the Microstructure and Properties of Two Refractory High-Entropy Alloys. Acta Mater. 68, 214–228. doi:10.1016/j.actamat.2014.01.029
Senkov, O., Zhang, F., and Miller, J. (2013). Phase Composition of a CrMo0.5NbTa0.5TiZr High Entropy Alloy: Comparison of Experimental and Simulated Data. Entropy 15, 3796–3809. doi:10.3390/e15093796
Sharma, A. S., Yadav, S., Biswas, K., and Basu, B. (2018). High-entropy Alloys and Metallic Nanocomposites: Processing Challenges, Microstructure Development and Property Enhancement. Mater. Sci. Eng. R Rep. 131, 1–42. doi:10.1016/j.mser.2018.04.003
Shim, S. H., Pouraliakbar, H., and Hong, S. I. (2022). Hierarchical Structured As-Cast CrFeNiMn0.5Cu0.5 High Entropy Alloy with Excellent Tensile Strength/ductility Properties. Scr. Mater. 210, 114473. doi:10.1016/j.scriptamat.2021.114473
Shun, T.-T., and Du, Y.-C. (2009). Microstructure and Tensile Behaviors of FCC Al0.3CoCrFeNi High Entropy Alloy. J. Alloys Compd. 479, 157–160. doi:10.1016/j.jallcom.2008.12.088
Singh, S., Wanderka, N., Murty, B. S., Glatzel, U., and Banhart, J. (2011). Decomposition in Multi-Component AlCoCrCuFeNi High-Entropy Alloy. Acta Mater. 59, 182–190. doi:10.1016/j.actamat.2010.09.023
Sinha, S., Nene, S. S., Frank, M., Liu, K., Agrawal, P., and Mishra, R. S. (2019). On the Evolving Nature of C/a Ratio in a Hexagonal Close-Packed Epsilon Martensite Phase in Transformative High Entropy Alloys. Sci. Rep. 9, 13185. doi:10.1038/s41598-019-49904-5
Smith, T. M., Hooshmand, M. S., Esser, B. D., Otto, F., Mccomb, D. W., George, E. P., et al. (2016). Atomic-scale Characterization and Modeling of 60° Dislocations in a High-Entropy Alloy. Acta Mater. 110, 352–363. doi:10.1016/j.actamat.2016.03.045
Soni, V., Gwalani, B., Alam, T., Dasari, S., Zheng, Y., Senkov, O. N., et al. (2020). Phase Inversion in a Two-phase, BCC+B2, Refractory High Entropy Alloy. Acta Mater. 185, 89–97. doi:10.1016/j.actamat.2019.12.004
Steingrimsson, B., Fan, X., Yang, X., Gao, M. C., Zhang, Y., and Liaw, P. K. (2021). Predicting Temperature-dependent Ultimate Strengths of Body-Centered-Cubic (BCC) High-Entropy Alloys. npj Comput. Mater 7, 152. doi:10.1038/s41524-021-00623-4
Stepanov, N., Tikhonovsky, M., Yurchenko, N., Zyabkin, D., Klimova, M., Zherebtsov, S., et al. (2015). Effect of Cryo-Deformation on Structure and Properties of CoCrFeNiMn High-Entropy Alloy. Intermetallics 59, 8–17. doi:10.1016/j.intermet.2014.12.004
Su, J., Raabe, D., and Li, Z. (2019). Hierarchical Microstructure Design to Tune the Mechanical Behavior of an Interstitial TRIP-TWIP High-Entropy Alloy. Acta Mater. 163, 40–54. doi:10.1016/j.actamat.2018.10.017
Sun, S. J., Tian, Y. Z., Lin, H. R., Dong, X. G., Wang, Y. H., Wang, Z. J., et al. (2019). Temperature Dependence of the Hall-Petch Relationship in CoCrFeMnNi High-Entropy Alloy. J. Alloys Compd. 806, 992–998. doi:10.1016/j.jallcom.2019.07.357
Sun, S. J., Tian, Y. Z., Lin, H. R., Dong, X. G., Wang, Y. H., Zhang, Z. J., et al. (2017). Enhanced Strength and Ductility of Bulk CoCrFeMnNi High Entropy Alloy Having Fully Recrystallized Ultrafine-Grained Structure. Mater. Des. 133, 122–127. doi:10.1016/j.matdes.2017.07.054
Takeuchi, A., Wada, T., and Kato, H. (2019). High-Entropy Alloys with Hexagonal Close-Packed Structure in Ir26Mo20Rh22.5Ru20W11.5 and Ir25.5Mo20Rh20Ru25W9.5 Alloys Designed by Sandwich Strategy for the Valence Electron Concentration of Constituent Elements in the Periodic Chart. Mat. Trans. 60, 1666–1673. doi:10.2320/matertrans.m2019037
Tancret, F., Toda-Caraballo, I., Menou, E., and Rivera Díaz-Del-Castillo, P. E. J. (2017). Designing High Entropy Alloys Employing Thermodynamics and Gaussian Process Statistical Analysis. Mater. Des. 115, 486–497. doi:10.1016/j.matdes.2016.11.049
Tong, C.-J., Chen, Y.-L., Yeh, J.-W., Lin, S.-J., Chen, S.-K., Shun, T.-T., et al. (2005). Microstructure Characterization of Al X CoCrCuFeNi High-Entropy Alloy System with Multiprincipal Elements. Metall Mat Trans A 36, 881–893. doi:10.1007/s11661-005-0283-0
Tracy, C. L., Park, S., Rittman, D. R., Zinkle, S. J., Bei, H., Lang, M., et al. (2017). High Pressure Synthesis of a Hexagonal Close-Packed Phase of the High-Entropy Alloy CrMnFeCoNi. Nat. Commun. 8, 15634. doi:10.1038/ncomms15634
Trexler, M. M., and Thadhani, N. N. (2010). Mechanical Properties of Bulk Metallic Glasses. Prog. Mater. Sci. 55, 759–839. doi:10.1016/j.pmatsci.2010.04.002
Tsai, M.-H., Yuan, H., Cheng, G., Xu, W., Jian, W. W., Chuang, M.-H., et al. (2013a). Significant Hardening Due to the Formation of a Sigma Phase Matrix in a High Entropy Alloy. Intermetallics 33, 81–86. doi:10.1016/j.intermet.2012.09.022
Tsai, M.-H., Yuan, H., Cheng, G., Xu, W., Tsai, K.-Y., Tsai, C.-W., et al. (2013b). Morphology, Structure and Composition of Precipitates in Al0.3CoCrCu0.5FeNi High-Entropy Alloy. Intermetallics 32, 329–336. doi:10.1016/j.intermet.2012.07.036
Varvenne, C., Luque, A., and Curtin, W. A. (2016). Theory of Strengthening in Fcc High Entropy Alloys. Acta Mater. 118, 164–176. doi:10.1016/j.actamat.2016.07.040
Wen, C., Zhang, Y., Wang, C., Xue, D., Bai, Y., Antonov, S., et al. (2019). Machine Learning Assisted Design of High Entropy Alloys with Desired Property. Acta Mater. 170, 109–117. doi:10.1016/j.actamat.2019.03.010
Wu, W., Song, M., Ni, S., Wang, J., Liu, Y., Liu, B., et al. (2017). Dual Mechanisms of Grain Refinement in a FeCoCrNi High-Entropy Alloy Processed by High-Pressure Torsion. Sci. Rep. 7, 46720. doi:10.1038/srep46720
Xia, S.-Q., Wang, Z., Yang, T.-F., and Zhang, Y. (2015). Irradiation Behavior in High Entropy Alloys. J. Iron Steel Res. Int. 22, 879–884. doi:10.1016/s1006-706x(15)30084-4
Xiong, J., Shi, S.-Q., and Zhang, T.-Y. (2020). A Machine-Learning Approach to Predicting and Understanding the Properties of Amorphous Metallic Alloys. Mater. Des. 187, 108378. doi:10.1016/j.matdes.2019.108378
Xiong, J., Zhang, T.-Y., and Shi, S.-Q. (2019). Machine Learning Prediction of Elastic Properties and Glass-Forming Ability of Bulk Metallic Glasses. MRS Commun. 9, 576–585. doi:10.1557/mrc.2019.44
Yadav, S., Sarkar, S., Aggarwal, A., Kumar, A., and Biswas, K. (2018). Wear and Mechanical Properties of Novel (CuCrFeTiZn)100-xPbx High Entropy Alloy Composite via Mechanical Alloying and Spark Plasma Sintering. Wear 410-411, 93–109. doi:10.1016/j.wear.2018.05.023
Ye, Y. F., Wang, Q., Lu, J., Liu, C. T., and Yang, Y. (2015). Design of High Entropy Alloys: A Single-Parameter Thermodynamic Rule. Scr. Mater. 104, 53–55. doi:10.1016/j.scriptamat.2015.03.023
Yin, B., and Curtin, W. A. (2020). Origin of High Strength in the CoCrFeNiPd High-Entropy Alloy. Mater. Res. Lett. 8, 209–215. doi:10.1080/21663831.2020.1739156
Yin, B., Maresca, F., and Curtin, W. A. (2020). Vanadium Is an Optimal Element for Strengthening in Both Fcc and Bcc High-Entropy Alloys. Acta Mater. 188, 486–491. doi:10.1016/j.actamat.2020.01.062
Zhang, W., Liaw, P. K., and Zhang, Y. (2018). Science and Technology in High-Entropy Alloys. Sci. China Mat. 61, 2–22. doi:10.1007/s40843-017-9195-8
Zhang, Y., Zhou, Y. J., Lin, J. P., Chen, G. L., and Liaw, P. K. (2008). Solid-Solution Phase Formation Rules for Multi-Component Alloys. Adv. Eng. Mat. 10, 534–538. doi:10.1002/adem.200700240
Zhang, Y., Zuo, T. T., Tang, Z., Gao, M. C., Dahmen, K. A., Liaw, P. K., et al. (2014). Microstructures and Properties of High-Entropy Alloys. Prog. Mater. Sci. 61, 1–93. doi:10.1016/j.pmatsci.2013.10.001
Zhao, S., Li, Z., Zhu, C., Yang, W., Zhang, Z., Armstrong, D. E. J., et al. (2021). Amorphization in Extreme Deformation of the CrMnFeCoNi High-Entropy Alloy. Sci. Adv. 7, eabb3108. doi:10.1126/sciadv.abb3108
Zhao, Y. Y., Lei, Z. F., Lu, Z. P., Huang, J. C., and Nieh, T. G. (2019). A Simplified Model Connecting Lattice Distortion with Friction Stress of Nb-Based Equiatomic High-Entropy Alloys. Mater. Res. Lett. 7, 340–346. doi:10.1080/21663831.2019.1610105
Keywords: multi-principal element alloys, solid solution strengthening, stacking fault energy, microstructural engineering, computational material science
Citation: Kumar J, Jha S, Raturi A, Bajpai A, Sonkusare R, Gurao NP and Biswas K (2022) Novel Alloy Design Concepts Enabling Enhanced Mechanical Properties of High Entropy Alloys. Front. Mater. 9:868721. doi: 10.3389/fmats.2022.868721
Received: 03 February 2022; Accepted: 28 March 2022;
Published: 06 June 2022.
Edited by:
Ravi Sankar Kottada, Indian Institute of Technology Madras, IndiaReviewed by:
Xiao Yang, Technical Institute of Physics and Chemistry (CAS), ChinaPraveen Sathiyamoorthi, Indian Institute of Technology (BHU), India
Copyright © 2022 Kumar, Jha, Raturi, Bajpai, Sonkusare, Gurao and Biswas. This is an open-access article distributed under the terms of the Creative Commons Attribution License (CC BY). The use, distribution or reproduction in other forums is permitted, provided the original author(s) and the copyright owner(s) are credited and that the original publication in this journal is cited, in accordance with accepted academic practice. No use, distribution or reproduction is permitted which does not comply with these terms.
*Correspondence: N. P. Gurao, bnBndXJhb0BpaXRrLmFjLmlu; Krishanu Biswas, a2Jpc3dhc0BpaXRrLmFjLmlu