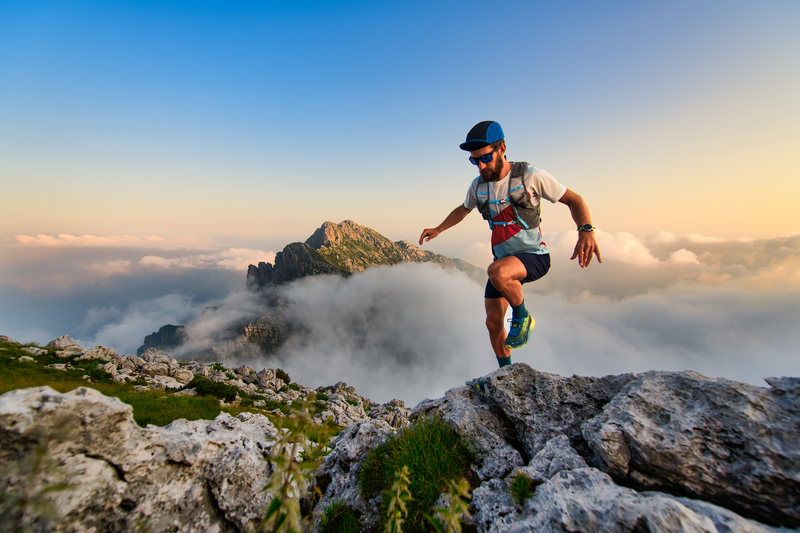
95% of researchers rate our articles as excellent or good
Learn more about the work of our research integrity team to safeguard the quality of each article we publish.
Find out more
REVIEW article
Front. Mar. Sci. , 07 March 2025
Sec. Ocean Observation
Volume 12 - 2025 | https://doi.org/10.3389/fmars.2025.1523585
Observing air-sea interactions on a global scale is essential for improving Earth system forecasts. Yet these exchanges are challenging to quantify for a range of reasons, including extreme conditions, vast and remote under-sampled locations, requirements for a multitude of co-located variables, and the high variability of fluxes in space and time. Uncrewed Surface Vehicles (USVs) present a novel solution for measuring these crucial air-sea interactions at a global scale. Powered by renewable energy (e.g., wind and waves for propulsion, solar power for electronics), USVs have provided navigable and persistent observing capabilities over the past decade and a half. In our review of 200 USV datasets and 96 studies, we found USVs have observed a total of 33 variables spanning physical, biogeochemical, biological and ecological processes at the air-sea transition zone. We present a map showing the global proliferation of USV adoption for scientific ocean observing. This review, carried out under the auspices of the ‘Observing Air-Sea Interactions Strategy’ (OASIS), makes the case for a permanent USV network to complement the mature and emerging networks within the Global Ocean Observing System (GOOS). The Observations Coordination Group (OCG) overseeing GOOS has identified ten attributes of an in-situ global network. Here, we discuss and evaluate the maturation of the USV network towards meeting these attributes. Our article forms the basis of a roadmap to formalise and guide the global USV community towards a novel and integrated ocean observing frontier.
The ocean plays a central role in the Earth’s cycles of energy, water, gases, and biogeochemistry, influencing weather and climate, biodiversity, and human activities. The ocean surface is an especially important part of the Earth system as it is the interface between the ocean and the atmosphere (Centurioni et al., 2019; Wanninkhof et al., 2019; Cronin et al., 2019). Here, momentum, energy (heat), freshwater, and gases (e.g., climate-critical greenhouse gases) are exchanged between the ocean and atmosphere. These air-sea fluxes act as a force on the ocean, driving ocean circulation and changing the environmental properties and chemistry of the marine biosphere, while at the same time influencing the atmosphere, with impacts on weather and climate. Quantifying these air-sea exchanges is essential to understanding the weather-climate system and the Earth’s energy budget, forecasting weather and climate, tracking the role of the ocean in sequestering anthropogenic carbon dioxide (CO2), and investigating a range of biological and biogeochemical processes. Despite its importance, the world’s air-sea exchanges remain minimally observed.
The surface ocean is prone to harsh sampling conditions from both the atmosphere (e.g., high winds, temperature, rain, snow, and ice) and the surface ocean (e.g., large waves, spray, sea ice, and strong currents) particularly in wintertime. These, plus the remoteness of the majority of the world’s oceans, have hindered data collection at the air-sea interface for decades. Air-sea interactions are complex to monitor, requiring measurements of multiple in-situ co-located state variables simultaneously by a suite of instrumentation positioned near the surface ocean and lower atmosphere. Air-sea fluxes often have high temporal and spatial variability that are difficult to sufficiently sample by lone ships or moorings; ocean heating of the atmosphere can lead to rapid convective processes, resulting in gustiness, cold-pool downdrafts, and highly-variable surface conditions (Wills et al., 2023). Likewise, oceanic or atmospheric fronts, and eddies and storms can result in disequilibrium between the ocean and atmosphere (Seo et al., 2023; Nicholson et al., 2022; Cronin et al., 2019; Swart et al., 2019), making it difficult to infer one from the other.
For over three decades, the Global Ocean Observing System (GOOS) has led a coordinated international effort to build global ocean observing capability (IOC 2018). This has included growing capacity for observing the air-sea interface. At present, there are only 25 air-sea flux moorings distributed globally as part of the OceanSITES GOOS network (Cronin et al., 2023). As an acknowledgment of the under-sampled air-sea interface, this is expected to increase as the Tropical Pacific Observing System (TPOS) begins to implement recommendations made by the international community (Cravatte et al., 2016). With advances in computational power and the applications of artificial intelligence and machine learning, higher spatio-temporal resolutions of the air-sea interface could deliver an ever-expanding list of insights and services than the existing global-scale air-sea flux coverage (Sloyan et al., 2018). Satellites and numerical models currently have resolutions that are too low to adequately capture or resolve detailed processes, which are required for weather-scale variability and validating assumptions across time and space (Gentemann et al., 2020a). The inherent challenge of quantifying air-sea fluxes lies in balancing the need for capturing high spatio-temporal resolution in variable conditions, with broad-scale global-mean coverage.
Uncrewed Surface Vehicles (USV; Figure 1) present a transformative solution to improving high-resolution observations in variable conditions, whilst delivering broad-scale coverage of the global ocean surface. Renewable-energy powered USVs can traverse tens of thousands of kilometres unassisted, simultaneously collecting data at high frequencies and thus solving the high-resolution and broad scale juxtaposition required for air-sea interaction observations. USVs enable navigable access to extreme environmental conditions such as tropical cyclones, winter storms and polar ice, which are typically under-sampled due to safety concerns and sparsely located (or absent) fixed moorings. Multiple oceanographic and atmospheric sensors can be remotely operated to simultaneously collect the essential ocean and climate variables necessary to calculate air-sea fluxes. Almost any instrument-based sensor can potentially be integrated, creating a multidisciplinary platform for ocean monitoring (Patterson et al., 2022), spanning ecology, biology, chemistry, physical oceanography, and atmospheric science. A USV’s position at the surface allows constant connectivity, near real-time data relays and access to wind, wave, and solar energy for propulsion and powering sensors. As USVs become more affordable, the implementation of multiple USVs used as force multipliers alongside other crewed or uncrewed vessels will significantly increase spatio-temporal efficiency, reducing the cost and increasing the accuracy of broad-scale surveys.
Figure 1. A sample of commercially available USVs, scaled to USV length, illustrating a wide-ranging ecosystem of high technology readiness level. These USVs are renewable powered, persistent, variable in cost and complexity that individually are suited to specific tasks and collectively to a range of different environments and variable conditions.
USVs have recently become a reliable way to access extreme and remote environments including tropical cyclones (Lenain and Melville, 2014; Mitarai and McWilliams, 2016; Ino et al., 2021), major hurricanes (Foltz et al., 2022; Zhang et al., 2023a, b; Yu et al., 2023), atmospheric cold pools (Wills et al., 2021, 2023), volcanically active areas where crewed ship operations are restricted (Tada et al., 2024), and in seasonally sea-ice covered polar seas (Wood et al., 2013; Swart et al., 2020; Chiodi et al., 2021; Du Plessis et al., 2022; Drushka et al., 2024; Sivam et al., 2024). USVs have also collected wave measurements in winter storms and hurricanes (Hole et al., 2016; Nickford et al., 2022; Zhang et al., 2023a), current profiles in the North Sea and Chukchi Sea (Wullenweber et al., 2022; Chi et al., 2023), wintertime storm-front interactions (Nickford et al., 2022; Toolsee et al., 2024), and have crossed entire ocean basins (Villareal and Wilson, 2014; Goebel et al., 2014) as well as circumnavigated Antarctica (Nicholson et al., 2022; Sutton et al., 2021). Even a single USV can be used to survey fronts and eddies, if air-sea flux measurements are combined to extrapolate sea surface temperature (SST) to a ‘foundational SST’ below the daytime stratification that occurs on sunny days with low winds (Cronin et al., 2024). USV adoption has also forged new disciplinary capabilities by providing reduced-noise platforms for multiple types of acoustic monitoring (Hildebrand et al., 2013, 2014; Pagniello et al., 2019), which is also complementary to fisheries research and operations (Mordy et al., 2017; Handegard et al., 2024), and have been used and designed for cost-effective maritime domain awareness (Nothacker, 2024), including for surveillance of remote marine protected areas (Angus et al., 2022; Molina-Molina et al., 2021). USVs have allowed an expanded footprint for ocean observing into extreme environments that challenge crewed vessels.
USV manufacturers, universities, and research institutions worldwide have pioneered groundbreaking USV capabilities, yielding valuable data and insights into this technology’s capabilities (Table 1). However, progress has been siloed within individual projects, reducing the potential for collective knowledge-building. The absence of standardised data collection, processing, disseminating and storage practices further hinders adoption and advancement of the USV industry for the benefit of society. Such complexities are not unique in ocean data collection, and previously, capacity development has been effectively progressed by taking a globally coordinated approach to data collection, management, and distribution. Such is the case with the 15 in-situ ocean observing networks within GOOS, including OceanSITES, Argo, drifters, OceanGliders, and Surface Ocean CO2 Observing Network (SOCONET), which approach global ocean observing under ten Observations Coordination Group (OCG) attributes to meet the needs of the global ocean observing community (GOOS, 2018).
Table 1. Scientific publications since 2010 that utilise USV datasets within nine generalised fields of study.
The scientific and USV industry communities have appealed for a globally coordinated approach to USV-based ocean monitoring (Clayson et al., 2023; Cronin et al., 2023; Whitt et al., 2020; Cronin et al., 2019; Wanninkhof et al., 2019; Centurioni et al., 2019; Meinig et al., 2019; Gille et al., 2016). Adopting a global network approach will transform the patchwork of independent USV projects into an established and trusted capability. As such, a ‘USV Network for GOOS’ has been established as an endorsed UN Ocean Decade project linked to the Observing Air-Sea Interaction Strategy (OASIS) UN Ocean Decade programme, to serve as a starting point for a permanent global USV network. This initiative aims to evolve the existing USV scientific data collection community into a coordinated entity with clear objectives and priorities, to be endorsed by the GOOS OCG as an emerging network. To achieve this, the USV Network for GOOS will demonstrate the network’s progress towards meeting the ten OCG attributes (Figure 2). Below, we discuss each attribute in detail in the context of the existing GOOS OCG networks.
Figure 2. Progress on the Observations Coordination Group Attributes of the Global Ocean Observing System networks. Attributes are listed in order of most progressed (green), progressing (yellow), and least progressed (red).
The primary purpose of the global USV network is to expand and complement the existing GOOS observing capability by improving ocean surface monitoring at small spatio-temporal scales unable to be captured by satellites and mooring arrays, whilst monitoring broadly scales of up to tens of thousands of kilometres. This will improve multidisciplinary observations within GOOS that are currently absent or cost prohibitive. The scope of the USV network will include prioritising core oceanographic and atmospheric observations associated with the energy, water, carbon, and life cycles needed to make transformational advances in weather and climate forecasting.
The network will complement the existing GOOS OCG networks through: (1) Increased observations of multiple co-located air-sea interaction and biogeochemical variables, including many under-sampled Essential Ocean Variables (EOVs) and Essential Climate Variables (ECVs); (2) Expanded sampling in dangerous weather and extreme events, including during high winds and variable sea states; (3) Targeted locational sampling to reach under-sampled areas such as high latitudes, remote tropics,continental shelf, and other areas that are cost-prohibitive to access; (4) Technological Advancement: Fostering a cooperative community to advance USV technology, sharing lessons on sensor integration and interoperability.
In this section we evaluate the ten attributes of a GOOS network as outlined by the OCG, highlighting current capabilities of the USV community network and where progress is needed.
Comprehensive global coverage of the open ocean using a range of USV archetypes is already making significant contributions to scientific ocean observations. To highlight this proliferation, we produced global USV coverage maps (Figure 3) using metadata (longitude, latitude, date, time) contributions from USV manufacturers, authors of published USV papers, and this paper’s co-authors’ professional networks. This has resulted in 200 USV datasets collected between 2011 and 2024 (Figure 3), although USV manufacturers indicated that significantly more data exist with commercial clients.
Figure 3. USV global ocean coverage maps from 2011 to 2024 as tracks (A) and density (B). Latitude-time heat map of count of daily USV observations in 5° latitude bins (C). (D) Cumulated count of USV observations as a function of latitude. Relative (left vertical axis) and cumulative (right vertical axis) counts of daily observations per month per 5° latitude (E).
The global coverage maps indicate tremendous potential to fill observational gaps in remote regions of the ocean, such as the Pacific Ocean and higher latitudes (Figures 3A, B). They also illustrate locations where USVs have not yet been used, such as in the Indian Ocean and South Atlantic. The steady increase in USV adoption over time (Figures 3C, E) is contrasted by large interannual variability of higher and lower sampling years (Figure 3E), indicating that USV observations are not yet sustained globally. Currently, about 80% of USV observations are located in the Northern Hemisphere (Figure 3C). This bias may be attributed to the relatively higher acquisition of funding in the Northern Hemisphere compared to the south, and large, dedicated process studies, such as the Tropical Pacific Observing System (TPOS; Smith et al., 2019), tropical Atlantic hurricane observations, the Salinity Processes in the Upper Ocean Regional Study (SPURS-2; SPURS project, 2015; Lindstrom et al., 2015) and the Sub-Mesoscale Ocean Dynamics Experiment (S-MODE; Farrar et al., 2020).
A unique aspect of USV technology is the ability to simultaneously monitor a significant range of EOVs and ECVs within the air-sea transition zone. Unlike many OCG networks that focus on measuring a small number of EOVs extensively, the USV network will complement the existing GOOS networks by collecting unprecedented co-located variables. This will effectively expand coverage of multidisciplinary studies, physical ocean-atmospheric observations, and physical ocean-ecological and biological observations.
Our review of USV literature found that of the 40 unique EOVs (https://goosocean.org/what-we-do/framework/essential-ocean-variables/) and ECVs (https://gcos.wmo.int/en/essential-climate-variables/table) 26 have been measured using USVs, plus a further five variables not listed as EOVs or ECVs (Table 2). The maximum number of variables monitored during a single USV deployment was 18, comprising wave height and period (sea state), skin temperature, subsurface temperature, salinity, currents, dissolved oxygen, biomass, ocean sound, atmospheric pressure, longwave and shortwave radiation, air temperature, humidity, wind velocity, seawater and air pCO2, coloured dissolved organic matter, and chlorophyll-a fluorometry (Zhang et al., 2019a). Sustained high-resolution data collection is possible due to USVs’ large payloads, large power capacity aided by renewable energy (e.g. wind and solar), sustained ample computing power, large data storage capacity for high-resolution data, and near real-time data relay typically packaged into 1-minute to 10-minute averages (Hodges et al., 2023; Foltz et al., 2022; Reeves Eyre et al., 2023).
The majority of USV studies in the literature aimed to quantify air-sea exchange of heat, momentum, freshwater, and CO2 (Table 1). These studies typically measure >8 covariables (Colbo and Weller, 2009) of the physical EOVs and ocean ECVs. High-resolution (>10 Hz) observations were collected in the majority of cases, which allowed for calculating turbulent fluxes via direct covariances, which are critical for air-sea interactions. Reducing the influence of the ship microclimate on air-sea boundary layer observations has been an important benefit of collecting EOVs and ECVs with USVs as opposed to ship-based observations. Crewed ships use variable air and sea intakes at 0.5 - 8 m above and below the ocean surface, whereas USV intakes can be varied more easily and are consistently closer to 0.5 - 5 m above and 0.1 - 7 m below the ocean surface, closer to the interface microlayer that governs fluxes (Drushka et al., 2024; Zhang et al., 2019a). The integration of an electronic precipitation gauge to a USV (Grare et al., 2021) is a promising step towards comprehensive measurements of air-sea interactions, though there are notable limitations to these approaches during high sea states due to interference from sea spray. The advantages of USVs for reaching high-resolution, fine-scale spatio-temporal physical processes and broadscale coverage is well documented (see Table 1).
A promising opportunity lies in the USV uptake for observing instrument-based ecological and biological variables such as eDNA (Preston et al., 2023), primary productivity (Hemsley et al., 2015), zooplankton (Pedersen et al., 2019; Guihen et al., 2014), phytoplankton biomass (Scott et al., 2020), phytoplankton abundance, community structure, and harmful algal blooms (Seegers et al., 2015). These are important and under-sampled components of GOOS (Koslow and Couture, 2015). The emerging instrument-based, underway net primary productivity measurements, enabled by gas tension devices (Cynar et al., 2022), also offers a promising pathway to expand USV ecological data collection.
USVs have been equipped with hydrophones to monitor and track seasonal changes in the distributions of sound producing marine mammals (Moore et al., 2007; Davis et al., 2016; Premus et al., 2022), occasionally other ocean life (Pagniello et al., 2019) and anthropogenic sound (Camus et al., 2021). Early studies using autonomous vehicles focused on documenting detections of marine animals, while more recent work has shifted toward examining how co-located oceanographic variables relate to observed patterns in presence, absence, or distribution (Baumgartner et al., 2014; Aniceto et al., 2020). These case-studies demonstrate USV capability to monitor co-located physical variables with: biogeochemical, biological and ecological variables. This co-located sampling has the potential to grow interdisciplinary marine studies and may help solve key scientific questions, such as determining the role of physical processes in marine movement behaviour (Hays et al., 2016).
The inherent ability of USVs to collect and store multidisciplinary data makes them highly versatile and ideal for opportunistic data collection, which is a major opportunity for the USV network. For example, a 2011 expedition that used four wave gliders yielded data for three different disciplines: surface ocean (Villareal and Wilson, 2014), acoustics (biomass; Goebel et al., 2014), and air-sea interactions (tropical cyclone; Lenain and Melville, 2014). During a study that was focused on assessing the presence of sea ice using camera footage (Meinig et al., 2015; Chiodi et al., 2021) and another of marine mammal and fish studies (Kuhn et al., 2020; Mordy et al., 2017), high-resolution (>10 Hz) 3-D wind velocity measurements were collected.
Table 2. Essential Ocean and Climate Variables (EOVs/ECVs) observed using USVs since 2010, for nine generalised fields of study, derived from a review of scientific literature describing the adoption of USVs for open-ocean observing (see Table 1).
USVs powered by renewable energy have entered the market in recent years due, in part, to the availability of low-cost and innovative battery technology and solar panels. Scientists have largely adopted these USVs, which are a subset of USVs that are inherently sustainable, leveraging a combination of renewable energy sources for propulsion, such as wind and wave power, or battery-electric motors charged by solar energy. Instrumentation and electronics are typically powered by solar-charged batteries, enabling USVs to operate in the open ocean for extended periods in the range of multiple months. USVs are typically integrated with large numbers of non-expendable oceanographic and meteorological instrumentation rendering these platforms high-value and therefore non-disposable. Moreover, high frequency ‘delayed data’ are usually stored onboard, greatly enhancing the value of a safe return to shore. Even after sustaining damage, USVs can be navigated to port for repair (Nickford et al., 2022; Sutton et al., 2021).
While the emergence of USVs powered by renewable energy is exciting for a range of sectors, this full reliance on renewable energy sources presents limitations, particularly regarding power availability and speed of propulsion. Studies have highlighted instances where the reliance on solar power alone for propulsion and instrument operation has led to gaps in data collection (Nickford et al., 2024; Chiodi et al., 2021; Levine et al., 2021). For example, an operational project using 20 wave gliders to monitor tide levels in Japanese waters, encountered consistent difficulties due to insufficient power (Ino et al., 2021). Other projects have reported USVs with a combination of wind and electric motor propulsion unable to fight strong currents (Chu et al., 2019; Tada et al., 2024), limiting their use in these environments. Power limitations can also lead to ambiguity regarding data transmission frequencies, affecting the quality of the data collected and reducing the efficacy of USV adoption. Mitarai and McWilliams (2016) expected real-time high frequency (20 Hz) data return, however, received data at 10-minute intervals in real-time and the high-frequency data once the platform had returned to port. A marginal sea ice study in the higher latitudes required cameras to be turned off during periods of low power (Chiodi et al., 2021), or reduced power duty cycles of a mini echosounder survey to as low as 25% as day time grew shorter with the changing season (de Robertis et al., 2019; Levine et al., 2021). The latter would also apply when cloudiness is persistent and there are larger solar angles. While relying solely on renewable energy sources in some environments introduces limitations, USV manufacturers are integrating hybrid fuel capabilities to extend operational capability and improve reliability for wintertime, high latitudes, during periods of high cloud cover, and in locations where there are strong currents. These integrations will be critical to allow the USV network to maintain environmental stewardship whilst also meeting network targets to operate in these challenging operational environments.
As with any in situ surface ocean data collection, biofouling is one of the limiting factors for USV deployment durations - a universal issue also affecting other platforms such as surface buoys and drifters. While adding anti-fouling to the instruments, sensors and the hull is one obvious solution, these typically contain copper. This can affect inductive salinity measurements if too close to the sensor (Johnson and Fassbender, 2023), so care must be taken to ensure an adequate sensor placement distance from the antifouling during the instrument integration process. Other anti-fouling options such as wipers and UV lights are theoretically more practical on a USV than on, say, a surface buoy or drifter because of the USVs larger power payloads (Ryan et al., 2020). One significant advantage of USVs is that they are recovered after missions, so all their sensors can be post-calibrated, unlike some of the expendable observing platforms. Comparing USV data with other platforms such as moored surface data at varying degrees of biofouling also provides an opportunity for better understanding the impact of biofouling on sensor data in general, following examples comparing fouled with un-fouled wave buoys (Thomson et al., 2015). Depending on where the USV is operating (e.g. warm, tropical environments versus higher latitudes), biofouling can present significant limitations on the duration of the platform deployment. Major benefits of the USV technology is that the data can be monitored in real-time, underwater cameras, if available, can be placed to monitor biofouling, and USVs can self-retrieve, or be swapped out with a recently serviced USV when biofouling (or indeed power or calibration limitations) becomes an issue.
A governance framework will set the network’s community of practice to drive implementation, development and long-term sustainment. A core steering committee comprising three leadership committees will lead the network. Each leadership committee will be made up of stakeholders across what we consider are the three crucial aspects to delivering ocean data using USVs: science, data management, and public-private partnerships. This multidisciplinary stakeholder-led governance structure will ensure that the network’s potential is fully realised and remains aligned with contemporary needs, including the recruitment and support of Early Career Ocean Professionals (ECOP) and ensuring the barriers to Justice, Inequality, Diversity, and Inclusion (JEDI) are broken (Johri et al., 2021). As such, open calls for participation, also following CARE principles (Collective benefit, Authority to control, Responsibility and Ethics; Carroll et al., 2020), will aid inclusive participation by individuals in under-represented regions and developing nations. A governance structure will be adopted that aligns with these principles and allows for equitable representation of the diverse stakeholders. If endorsed by GOOS OCG as an official emerging network, the USV network will benefit from OCG-facilitated discussions and opportunities aligned with these principles, and opportunity-sharing between the other OCG networks. USV network leadership committees will be required to provide transparency in decision-making and communications, and be required to declare conflicts of interest, especially when working with private companies. Guidance in implementing measures to maintain these standards will be drawn from other OCG networks.
To date, the network committee comprises the co-authors of this paper, and meets intermittently to share news, updates, ideas, and work on collaborative funding proposals. These meetings comprise a combination of recorded webinars (available at https://airseaobs.org/resources/usv-for-goos-webinar) and meetings to work on network activities and discuss funding opportunities to propel the network. A core committee will be formed within 12 months of this paper being published, and the steering committee will organise regular committee meetings to undertake tasks such as setting priorities for the network goals and activities, and developing funding pitches to work towards meeting the ten OCG attributes. Inter-sessional activities will be workshopped on relevant aspects of the network related to the ten attributes. The core steering committee will report annually to the GOOS OCG committee and provide input into GOOS OCG activities, and liaise with other relevant communities, such as OceanOPS (https://www.ocean-ops.org/), the Ocean Best Practices System (OBPS; https://www.oceanbestpractices.org/) and other OCG networks.
Data distribution is a major challenge for the USV network due to nuances that are unique to the USV network, including the multiple and diverse platform types, manufacturers, and the multitude of USV data delivery mechanisms and options; USV data delivery needs to be considered differently from other networks. One aspect of data delivery stems from USVs’ unique capability to persistently relay Near Real-Time (NRT) data (e.g. roughly every 10 minutes) as well as very high-resolution Delayed Mode (DM) data (Figure 4). NRT data transmission configuration depends on the USV satellite communication subscription (e.g., bandwidth and data) which currently varies with USV make, model, and operators. Alongside this, the complexity of the Global Telecommunication System (GTS) and the transmission procedures for different data types (e.g., biological and ecological data) constrain data uploads to the GTS. It is possible for the USV network to borrow and adapt standards of existing networks to make it easier for USV end-users to make their data publicly available. For example, the Ships Observations Team (SOT) has recently formed a task team for Enhancement of Independent Class Observations (TT-EICO). This task team is designed to support the development and maintenance of new pilot projects to include gathering of data and metadata, and their quality control, from vessels where the information is not yet made available on the GTS (pers comm. Shawn Smith and Darin Figurskey). USV data handling and transmission will need to be considered independently of OceanGliders, drifters, and Argo platforms because of the large data storage payloads and their constant connection to satellites at the surface. For NRT transmission, the World Meteorological Organisation (WMO) GTS uses the BUFR (Binary Universal Form for the Representation of meteorological and oceanographic data) format and an existing template was developed to specifically support USV NRT data exchange. This template has been used over the last several years to exchange USV data on the GTS and is a strong starting point for implementation across the USV network. In this context, the GTS is currently evolving the WMO Information System (WIS; https://community.wmo.int/en/activity-areas/wis) so USV data infrastructure will be planned to meet the requirements of the WIS 2.0 as it replaces the GTS. The USV network is in an ideal position to lead the development of appropriate data and metadata formats, which will be made available online in the form of templates to the scientific community, USV manufacturers, and private USV users should they wish to make their data publicly available. The network will offer expertise and guidance to ensure data is disseminated according to the GOOS OCG attributes.
Figure 4. A proposed data flow diagram for ensuring quality and timely delivery of data to the global community.
The USV network is committed to promoting FAIR, CARE and TRUST principles, defined here: FAIR principles (Findable, Accessible, Interoperable, Reusable; Wilkinson et al., 2016; Tanhua et al., 2019) list the characteristics that facilitate data exchange; the TRUST principles (Transparency, Responsibility, User focus, Sustainability and Technology; Lin et al., 2020) focus on defining the criteria for best practices in digital preservation by repositories, and the CARE principles are people and purpose oriented, ensuring that Indigenous innovations and self-determination are not ignored, thus decreasing the power differentials and historical contexts. Communicating these data-norms and expectations is an important aspect of developing the community of practice. These principles will be communicated to the public and network as part of the USV community of practice (on a USV network website, which is currently being developed) so that data contributors are aware of the principles under which the network and associated data distribution operates.
The USV network will develop data processing strategies and best practices by harnessing community-agreed protocols and taking advantage of existing efforts and data distribution models from other GOOS networks and data delivery quality standards. As USVs are new technology, the network has a collective responsibility to ensure that appropriate quality controls on data processing and delivery are met. While these processes are not yet fully formed, we will draw from the learnings of other OCG networks introducing new technologies. For example, open source code will be encouraged in a similar fashion to that of the OceanGlider community (https://github.com/OceanGlidersCommunity) to promote open source code development at the community level. This coordinated approach will ensure methodologies and standard operating procedures will be developed amongst the USV community to avoid duplicitous efforts, siloed practices, and provide products that will benefit scientists and USV manufacturers alike. Key QARTOD (Quality Assurance/Quality Control of Real-Time Oceanographic Data) principles, such as quality descriptors and detailed quality flags will be developed following industry-standard codes and manuals (Bushnell, 2017; https://github.com/ioos/ioos_qc). A centralised system to curate and distribute data for stakeholders is essential for managing and disseminating data. For example, CUBEnet (http://oceancube.usm.edu/) has been able to streamline data access for stakeholders and enhance the utility of oceanographic data and address science-based questions in the Gulf of Mexico. By integrating these best practices and leveraging the experiences from the established systems and best practices discussed above, the USV network will develop a cohesive and efficient data management solution for the USV user community.
Each OCG network has a role in GOOS, and its progress towards its specific mission and targets must be tracked. The USV network defines its unique contribution to GOOS as its ability to observe multiple EOVs and ECVs at fine spatio-temporal scales, whilst monitoring broadly at scales of up to tens of thousands of kilometres from seconds to months. The USV network targets remote and difficult to access locational and disciplinary environments.
Recognising that air-sea fluxes are concentrated near fronts, Cronin et al. (2019) proposed that a global network of approximately 1000 platforms deployed as pairs or clusters within 10° by 10° boxes may be a reasonable target (368 boxes would cover the global ocean), playing a similar role to the Argo network target of one float profile per 3° by 3° box every 10 days (Roemmich et al., 2019) or the drifter network target of one drifter per 5° by 5° box (Centurioni, 2018). Alternatively, the USV network may want a target of having repeat transects that can capture the annual mean air-sea fluxes, enabling national and international stocktakes, such as called for by the 2015 Paris Accord (Wanninkhof et al., 2019). Such repeat transects could be referred to as GO-USV transects, as their targets are similar to those of the GO-SHIP network, albeit with a focus on air-sea interaction at more rapid timescales than the GO-SHIP focus on full water column variability over decadal timescales. If these transects were made as a USV cluster, the transect could act like a ‘mobile meso-net’, capturing the multi-scale variability of convective systems that drive much of the air-sea interactions. An example of this type of sampling is being tested in TPOS, where near-annual missions with 2-4 USVs sampling cold pools and convective mesoscale systems as USVs transect through the Inter-tropical convergence zone, and then sample submesoscale SST fronts as they travel into or out of the cold tongue of surface water on the equator (Cronin et al., 2024, 2023; Wills et al., 2023). Once demonstrated, the ‘mobile cluster’ or ‘force-multiplier’ approach to ocean data collection could be transformative to climate and weather science.
At minimum, the USV targets should track the attributes discussed in this section, including metrics related to coverage, number of EOVs and ECVs, applications addressed, and data sets. Defining targets and gaps for future goals requires active community discussion and in some part is likely to be regionally dependent, and progress over time.
Delivery of quality NRT or DM metadata is an essential aspect of a coordinated, global USV network. To date, the existing USV community has been independently managing data and metadata associated with individual projects; some data being stored privately, and other data being made freely available in online catalogues, such as the National Centers for Environmental Information (NCEI; https://www.ncei.noaa.gov/), Zenodo (https://zenodo.org/), European Marine Observation and Data Network (EMODnet; https://emodnet.ec.europa.eu/en), and USV manufacturers websites (https://www.saildrone.com/technology/data-sets).
The USV community recognises two key opportunities for delivering quality data and metadata in a frictionless way to global end users as outlined by the GOOS OCG (https://goosocean.org/who-we-are/observations-coordination-group/data-management/). These opportunities are to develop: (1) A central repository for global USV data that follows FAIR, TRUST, and CARE principles, and can be accessed from anywhere in the world (discussed further in Section 7); and (2) Standardisation of USV data and metadata, including various formats for NRT and DM, and for data storage and distribution, discussed below.
A major requirement for metadata quality and delivery is the development and adherence to standards. While there are currently no formal standards for USV metadata collection, the National Oceanic and Atmospheric Administration (NOAA) is working towards developing a data and metadata template for USVs (https://www.ncei.noaa.gov/products/uncrewed-system-metadata-templates) that, alongside parallel efforts in other nations and agencies, and across different manufacturers, could be harmonised to form standard templates for the network. The USV network will play a major role in establishing open communication lines between industry and scientists to help with the co-development of proposed standards. While reviewing literature for this paper, we found that USV data streams regularly omit important metadata, such as the distance from a sensor to the water level. Changes in the location of the sensor in relation to the water line or USV centre of gravity can alter the way the data should be interpreted. There are opportunities for the network to focus on standard metadata content and data formats for use across the community.
Best practices extend from standardising metadata collection (discussed in Attribute 7) to data collection methodologies from an ecosystem of USV archetypes, and approaches to industry data collection. Adopting standards across a USV network that encompasses industry- and science-operated vehicles and sensors is particularly important for building trust with future scientific and industry end users, including GOOS regional alliances, OCG networks, and industry (Parks et al., 2024). A significant aspect that is continually identified within the scientific USV end-user community has been the lack of certainty around data collected from a non-spherical, propelled platform. An important, and typically overlooked practice is to perform intercomparison studies to describe and account for uncertainties in the powered motion of the USV and the hydrodynamic responses of the hull, which differ compared to a moored spherical buoy, and may be specific to certain variables. This is particularly important for the measurement of wave spectra, which is an under-sampled ocean variable but a key variable for calculating air-sea fluxes (Thomson and Girton, 2017; Amador et al., 2023; Colosi et al., 2023).
Intercomparisons between USVs and established monitoring platforms of known precision and uncertainty, under a range of different conditions are a fundamental process for the trusted adoption of USVs by the scientific community. However, there are challenges associated with these intercomparisons, especially in remote locations, extreme conditions, and/or regions of high natural variability (Sabine et al., 2020; Zhang et al., 2019a). Moored buoys are likely the most useful for this purpose, as they remain in fixed locations and provide standard near-surface ocean and atmospheric measurements.
The USV community would benefit from a standardised USV intercomparisons methodology, and a common database of data intercomparisons across a wide range of ocean-atmosphere conditions and USV platform archetypes to determine strengths and weaknesses of different USV platforms and gain confidence in the use of the data for scientific analysis and data assimilation. The USV network will, in collaboration with manufacturers, develop standards for data processing and data quality control (QC) of the platforms and instruments, with the aim of ensuring that data published according to these guidance and standards are of the highest quality and can be used for multiple applications. An assessment of existing standards and recommended practices will be done and where applicable expanded. In order to ensure knowledge sharing and community uptake, the outcomes of this work will be published in OBPS (https://www.oceanbestpractices.org/) and maintained by the network. Developing standards and best practices will be an opportunity to work with USV manufacturers to ensure that the scientific needs of USVs for certain applications and environments are made available.
An important aspect of the USV network is its relative advantage in reaching remote and under-sampled locations, which aligns with the GOOS mission to promote feasible, high-impact observing programs. These regions often lack the resources to deploy traditional observatories, and USVs present a compelling option for extending coverage to the archipelagos of southeast Asia, central America, and the tropical Pacific and Indian Oceans. The existing data (Figure 3) show notable coverage in very remote locations, including prolific adoption in the tropical Pacific, and we can see that isolated USV deployments occur in other remote and under-sampled locations, such as Australia’s northern regions (the Timor and Arafura Seas), central America’s archipelagos, northern parts of South America, and the western African coastline, associated with voyages out of the Canary Islands. Notably, no USV tracks are available in the Indian Ocean, which may present an opportunity to extend multidisciplinary and interdisciplinary co-located observations for the Indian Ocean Observing System (IndOOS), as strongly noted in the 2019 IndOOS roadmap (Beal et al., 2019; Hermes et al., 2019; Beal et al., 2020).
There are substantial opportunities for USV capacity development via comprehensive training and capacity-building programs aimed at developing the technical skills in oceanographic data collection and management (McKenna et al., 2023). These programs are designed to cover various aspects of USV operation, data acquisition, data processing, and interpretation using advanced analytical tools to better position a changing workforce as well as specifically target a young audience in pursuit of ocean science education and employment. USV technological adoption empowers maritime professionals in enhancing operational capabilities and data handling proficiency as well as provides an on-ramp for educational, and vocational programs.
A sustained USV network will potentially be one of the greatest challenges, and a key component to sustainment will be a steady finance stream. Historically and in the near future, the existing missions have and will be individually funded through 2-5 year research grants. However, there is often synergy between intensive process studies (discussed in Attribute 1) and long-term monitoring that can provide seasonal-to-interannual cost recovery, which could help support a sustained USV network. Another strategy to obtain sustained observations might be to take advantage of transits from future USV service stations, particularly ones located in the global south. Ultimately through economies of scale, we can expect that a threshold will be reached where it is more economical to plan as a sustained observing network rather than as one-off missions. For example, USVs could be recovered and redeployed by refurbishing the platform and integrating fresh sensors, similar to how moorings are turned around in sustained long-term mooring networks.
In the context of meeting this attribute, the network is more likely to be sustained if the other attributes are met: data management, a community of practice, data and metadata standards and best practices, governance structure and the setting and delivery of network targets and metrics will all drive and ensure a sustained and burgeoning network.
As a network focused on air-sea interactions, the USV network will provide a critical and currently unmet capability within GOOS, complementing existing networks by providing high-resolution surface ocean and lower atmosphere observations over broad regions (Figure 5). Essentially, scientific USVs play a major role in measuring air-sea interactions during extreme conditions and capturing submesoscale processes dynamically, contributing multiple variables to valuable process-scale information for enhancing weather and climate research at previously unmet spatial and temporal scales.
Figure 5. Uncrewed Surface Vehicles’ complementary role in the GOOS networks, covering small-scale processes for up to 12 months and spanning oceans, adapted from https://goosocean.org/document/17466. Asterisked networks represent those which sample the interior ocean.
USVs have already provided complementary coverage and enhanced both the extent and variety of data collected as an integrated capability within existing GOOS OCG networks. For example, USVs have been paired with OceanGlider deployments, providing simultaneous surface ocean and lower atmospheric observations, adding critical surface observations to the collection of interior ocean observations (Nicholson et al., 2022; Kosaka et al., 2023; Zhang et al., 2023a). In some instances, USVs have been especially augmented to function as motherships for transport and release of OceanGliders (Siddle et al., 2021). Like the OceanGlider network, USVs can complement OceanSITES network time series by providing spatial gradient information needed for evaluating advective processes, and in this way enable closure of budget analyses governing variability at the time series stations (e.g. Fassbender et al., 2017). USVs have been deployed as GO-USV repeat transects that could complement the GO-SHIP transects, as discussed in Attribute 6. USV-observed CO2 fluxes will undoubtedly be an important component of the emerging SOCONET, supporting SOCONET’s mission to provide global ocean CO2 uptake information for annual national assessments and 5-year global stocktakes (Wanninkhof et al., 2019). Any network, such as SOCONET, that is defined by an EOV, will be reliant upon platform networks like the USV network, to make these measurements. A USV network will invariably make overlapping measurements with other platform networks, however these will likely have different spatial and temporal scales and provide an opportunity for understanding differences in data outputs between different platforms.
USVs offer a valuable complementary service to enhance satellite observations due to their high temporal resolution. For example, most wind-measuring satellites use sun synchronous orbits, meaning that they obtain measurements at best every 12 hours, along a swath with a width typically between 500 and 1500 km, depending on satellite specifications. USVs can provide valuable high-resolution data of satellite-inferred variables such as wind speed and direction, sea surface temperature, and salinity measurements. Satellites interpreted in concert with USV measurements offer a means to evaluate variability over spatio-temporal scales that would be inaccessible from satellites or USVs alone. USV data are already being used for satellite data validation (Ricciardulli et al., 2022; Yu et al., 2023). As the USV network grows and the data are more widely trusted and available in appropriate formats, we expect that USV data will be used more extensively to support satellite validation and calibration, consistent with the current usage of surface drifting and moored buoy data. Through webinars and workshops, OASIS sustains communication within the satellite air-sea flux community and supports links with the USV network. Continued interactions between the satellite and USV communities are necessary and will benefit both groups. For example, wind observations from a NOAA-Saildrone USV are being used to validate Synthetic Aperture Radar (SAR) winds in Atlantic hurricanes, while SAR data also provide a consistency check for USVs. Future satellite concepts offer the possibility of obtaining targeted measurements of air-sea fluxes. For example, air-sea turbulent heat fluxes could be inferred using bulk parameterisations by measuring near surface temperature and humidity as well as wind speed and sea surface temperature (Gentemann et al., 2020b). Air-sea momentum exchange could be inferred using a Doppler scatterometer to measure winds and surface currents simultaneously (Rodríguez et al., 2019), as has been done from aircraft during S-MODE (Farrar et al., 2020). All of these concepts will rely on in-situ surface measurements (e.g. from USVs) to support calibration and validation.
Building on their proven capabilities, the USV network has the potential to drive further complementary services and facilitate unrealised integrations within other GOOS OCG networks. As numerical weather prediction models begin to incorporate true coupled ocean-atmosphere assimilation schemes, USV and other platforms that measure coincident ocean and atmosphere variables will become ever more critical (Penny et al., 2019). Opportunistic data collection for non-scientific USV missions has the potential to become a realised component of the Volunteer Observing Ships (VOS) and Ships of Opportunity Programme (SOOP), and would require these volunteers to use the network data and metadata standards, and agree to the data principles outlined in Attribute 5.
GOOS regional alliances have also recognised the value and growing USV adoption. The GROOM II project focuses on fostering USV operations, providing 11 European countries and 20 member organisations with information and community resources in operationalising USVs. The USV network will work collaboratively with these and other existing regional networks to foster and build the global USV network. Other under-sampled regional alliances, such as the Southern Ocean, stand to benefit significantly from the USV network, which may be supported under the regional, community-driven Southern Ocean Observing System (SOOS), to address the environmental constraints of ocean observing in such a difficult environment.
This paper is a first step in gathering a community of interested individuals which can help set the foundations for a coordinated and collaborative global network. The following terms of reference, which may be adapted after establishing the steering committee, are proposed.
● Develop and implement a global network for air-sea interaction observations through focusing on sampling the following core EOVs/ECVs: air temperature, air pressure, humidity, skin temperature, sea surface temperature and salinity, current profiles, wind speed and direction, radiation (long-wave/short-wave), atmospheric pressure, seawater and air pCO2, dissolved oxygen, and chlorophyll. Focus on stand-alone USV missions in the network, and the integration of USVs within other OCG networks such as GO-USVs, USV VOS/SOOP, USV-OceanGlider pairings, and USVs in SOCONET.
● Develop an implementation plan for the coordinated collection of biological and ecological data using USVs.
● Coordinate delivery of NRT data to the GTS and quality-controlled data to a network of global data centres.
● Develop and systematically review data collection best practices, working with the OBPS and tools to reduce duplication of effort by learning from other networks.
● Work with the wider scientific community to develop standardised methods for performing intercomparisons and calculating derived variables, such as surface wave height spectra.
● Coordinate and exchange information with GOOS OCG on scientific and technical issues and to optimise the overall capability of GOOS.
● Collaborate with the USV manufacturing industry in a two-way dialogue to develop appropriate practices for sampling and data QC.
● Ensure FAIR, TRUST, CARE data practices, and JEDI principles across network governance structure.
● Promote coordination and partnerships with other ocean observing networks.
The wide range of unique USV platforms offers both advantages and challenges for global coverage and providing reliable and quality data. Diverse USV platform types will build resilience in sustaining GOOS observations, as was demonstrated by autonomous platforms during the COVID pandemic (Boyer et al., 2023), however the non-uniform platform shapes, sizes, and movement characteristics introduce complexity and challenges for developing standards and best practices.
Diversity in USV size and shape format will help improve the challenges that arise from the reliance on solar power alone for power generation (discussed in Attribute 3). This reliance is a recognised challenge across manufacturers and end-users. Technology accelerators and philanthropic organisations promoting sustainable marine technology solutions are funding opportunities for novel power generation alternatives to solar, such as wave- and hydro- generators or repackaged wind generators which would be considered for polar-adapted USVs. Although these solutions are in development, there is promising collective global momentum towards problem-solving. In the meantime, USV operators will require the tools, training and experience to work within the limitations of the technology and the environment. Given USVs are highly mobile and manoeuvrable, USV schedules (much like ship schedules) can be coordinated to ensure that operational failures due to low energy do not occur.
Widespread adoption of USVs for ocean science will be shaped by several practical considerations. Public-private partnerships will play a crucial role in this landscape, and the USV network will facilitate collaboration between scientific institutions and USV manufacturers. These partnerships can bridge gaps between scientific needs and commercial capabilities, potentially through dedicated science-business liaisons who understand both USV platforms and scientific methodologies. However, the diversity in USV platforms and the business models of their manufacturers presents challenges, with primary business models, owner-operator, product-as-a-service, and leases, each presenting unique challenges and benefits, influencing operational decision-making, data flow, and cost structures.
Regulatory compliance remains a complex issue due to the absence of standardised international regulations for USVs, which tend to come under the banner of ‘uncrewed maritime vehicles’, and include underwater uncrewed vehicles. The 1982 United Nations Convention on the Law of the Sea (UNCLOS) did not anticipate modern uncrewed technologies and this has led to a fragmented regulatory landscape. The classification of USVs as ‘ships’ or ‘not-ships’ significantly impacts their operational freedoms and restrictions. On the high seas, USVs classified as ‘ships’ enjoy the freedom of navigation as would any other ship. The definition of a USV as a ‘ship’ or ‘non-ship’ is governed by each individual nation (UNCLOS, Art. 91); there is currently no unified global approach for legal status of uncrewed vehicles.
In the context of sustained, long-term marine scientific research at a global scale (i.e. a focus of the GOOS), UNCLOS provides Part XIII Marine Scientific Research (MSR). Part XIII establishes rules to grant consent for MSR to be undertaken and to promote altruistic values such as: the obligations of international states and organisations to promote cooperation; favourable conditions for integrating the efforts of scientists conducting MSR; and data and knowledge needing to be shared and disseminated for the collective good of mankind. However, for the existing GOOS networks, which includes autonomous platforms such as OceanGliders and Argo floats, there are notable concerns and challenges about the practicalities of coordinating the observation networks and attaining MSR clearances, such as in disputed territorial waters, and within practical timelines for planning voyages and deployments (GOOS, 2021). Sovereign security is a major area of concern for coastal states and can substantially delay or prevent MSR clearances. A global USV network endorsed by GOOS will operate under UNCLOS Part XIII, like other GOOS networks (GOOS, 2021). While UNCLOS convention allows ‘the right of innocent passage’ through areas of national jurisdiction (UNCLOS, Art. 17), in reality the presence of an uncrewed system in foreign waters typically results in disputes (Chang et al., 2024), since many USVs are associated (or suspected to be) with military operations due to their dual use capabilities. Ultimately, the navigation of USVs undertaking MSR in areas of national jurisdiction falls under the jurisdiction of the coastal state, and USV users must obtain prior approval to operate within the jurisdiction of the coastal state (UNCLOS). This means that globally-roaming scientific USVs operating inside EEZs will be best operated in partnership with local collaborators who can navigate local governance structures and appeal to sovereign legal maritime authorities.
Growing observing capability using USVs, through new sensor integrations, prolonged endurance, improved manoeuvrability, unprecedented co-variable data collection capability, developing appropriate standards, and fine-scale and real-time data delivery will endure as a result of a coordinated and inclusive community. New developments in profiling capability such as towed and winched instruments, and the emergence of instruments designed specifically for USVs, is already resulting in enhanced environmental measurements. These include rapidly evolving structures connected to the physical and biogeochemical air-sea fluxes such as fronts, mixing, biomass patchiness, and lower marine boundary layer from winds bursts to weather. USVs are also beginning to make direct eddy covariance flux measurements (Reeves Eyre et al., 2023), which will result in better constraints of bulk formula methods and greatly expand direct observations spatially and temporally. Perhaps the most promising and regularly promoted aspect of USVs that have yet to be implemented at scale in science is the concept of force multipliers to measure a ‘pseudo-synoptic’ view of rapidly developing phenomena (Nickford et al., 2022; Nicholson et al., 2022; Toolsee et al., 2024), such as ocean fronts, tropical cyclones, marine heatwaves, or phytoplankton blooms. In other words, a few USVs working together can provide unprecedented 3-D or even 4-D perspectives of the ocean or atmosphere during an experiment.
New data science techniques are allowing us to integrate USV observations with various types of model simulations, such as weather forecast operational models, to high-resolution coupled model simulations, in order to understand and upscale the impact that fine-scale processes have on our weather, climate, and ecosystems (Swart et al., 2023). In general, there are several ways USV data can be applied to numerical modelling. First, USV data provide a ground truth to validate numerical products (reanalysis, simulations, and forecasts). Second, knowledge gained from USV data would help improve numerical model parameterisations (e.g., improved drag coefficient parameterisation under hurricane wind conditions). Third, when USV data are available to operational forecast centres in real time via GTS, their data assimilation systems can inject USV data into their forecast initialisation procedures. To enable this capability, the USV network and its nascent community has an important role to play in the development of agreed standards and best practices, guided and facilitated by OBPS, and digital infrastructure to globally disseminate USV data according to FAIR, CARE, and TRUST principles, and promote JEDI principles across the governance structure. Meeting these needs will facilitate data integration into ocean models and broader adoption by the research community and other users. This community-driven approach that supports scientists and manufacturers in sharing experiences, challenges, and solutions is much-needed across the USV end-user community. Every individual that was approached to join the network in writing this manuscript willingly provided data (published and unpublished) and intellectual contributions, demonstrating the collective drive and need for the network globally. These cohesive and altruistic characteristics of the network community aligns well with the prescribed UNESCO-oriented OCG attributes.
In the short-term, some administrative USV network costs have been provided in-kind by OASIS as an affiliated UN Decade of Ocean Science project. The network is actively pursuing government grants to support operational activities such as website development, data management services and administrative support. A formal endorsement as an emerging GOOS OCG network will increase network visibility and facilitate participation in international collaborations, research programs and regional alliances that pool resources and funding for ocean observing programs involving USVs. The network is pursuing international collaborative research grants to work on key and immediate needs, such as much-needed intercomparisons and the development of standards and best practices guidelines. These efforts are coordinated amongst the network participants, and updates are provided and knowledge shared in regular network meetings. In the medium to long term the network will require more sustained funding streams. Given the novelty of this technology, the USV network will pursue engagement with non-profit organisations, technology organisations (such as the Marine Technology Society) and pursue philanthropic funding. This may require the network to adapt to an organisational structure that is formally ‘not for profit’, which may also open doors to other end-users. The USV network has the potential to promote the value of data, technology and the private sector in ocean observing with shared interests in both the altruistic and commercial benefits of a global USV network.
RP: Conceptualization, Data curation, Formal analysis, Funding acquisition, Investigation, Methodology, Project administration, Resources, Software, Supervision, Validation, Visualization, Writing – original draft, Writing – review & editing. JoB: Conceptualization, Investigation, Writing – original draft, Writing – review & editing. MC: Conceptualization, Data curation, Funding acquisition, Investigation, Methodology, Project administration, Resources, Supervision, Writing – original draft, Writing – review & editing. JE: Data curation, Visualization, Writing – original draft, Writing – review & editing. JM: Writing – original draft, Writing – review & editing. JP: Conceptualization, Data curation, Investigation, Writing – original draft, Writing – review & editing. AP: Writing – original draft, Writing – review & editing. SS: Conceptualization, Funding acquisition, Investigation, Project administration, Resources, Supervision, Writing – original draft, Writing – review & editing. CA: Writing – original draft, Writing – review & editing. WB: Data curation, Writing – original draft, Writing – review & editing. PB: Writing – original draft, Writing – review & editing. JuB: Writing – review & editing, Writing – original draft. EB: Writing – original draft, Writing – review & editing. JaB: Writing – original draft, Writing – review & editing. LC: Data curation, Writing – original draft, Writing – review & editing. BD: Writing – original draft, Writing – review & editing. MD: Data curation, Writing – original draft, Writing – review & editing. MF: Data curation, Writing – original draft, Writing – review & editing. GF: Writing – original draft, Writing – review & editing. SG: Investigation, Writing – original draft, Writing – review & editing. LG: Data curation, Writing – original draft, Writing – review & editing. JH: Writing – original draft, Writing – review & editing. LH: Data curation, Writing – original draft, Writing – review & editing. MH: Data curation, Writing – review & editing. VH: Writing – review & editing. CKo: Writing – review & editing. NK: Data curation, Writing – review & editing. CKu: Data curation, Investigation, Writing – original draft, Writing – review & editing. LLe: Data curation, Writing – original draft, Writing – review & editing. LLo: Writing – original draft, Writing – review & editing. AM: Writing – original draft, Writing – review & editing. EM: Writing – original draft, Writing – review & editing. CRM: Conceptualization, Writing – original draft, Writing – review & editing. SM: Data curation, Writing – review & editing. CM: Data curation, Writing – original draft, Writing – review & editing. AN: Data curation, Writing – review & editing. SAN: Data curation, Writing – review & editing. S-AN: Data curation, Writing – review & editing. KO: Investigation, Writing – original draft, Writing – review & editing. DP: Data curation, Writing – review & editing. LP: Data curation, Writing – review & editing. VR: Data curation, Writing – original draft, Writing – review & editing. NR: Data curation, Writing – review & editing. ES: Data curation, Writing – original draft, Writing – review & editing. CS: Writing – original draft, Writing – review & editing. AS: Writing – original draft, Writing – review & editing. NT: Data curation, Writing – original draft, Writing – review & editing. JT: Writing – original draft, Writing – review & editing, Data curation. IU: Data curation, Writing – original draft, Writing – review & editing. LY: Writing – review & editing, Writing – original draft. CZ: Data curation, Writing – review & editing. DZ: Data curation, Writing – review & editing.
The author(s) declare financial support was received for the research, authorship, and/or publication of this article. This work occurred as an activity of the Observing Air-Sea Interactions Strategy (OASIS). We acknowledge funding provided by Elysium EPL and national committees of the Scientific Committee on Oceanic Research (SCOR) and from a grant to SCOR from the U.S. National Science Foundation (OCE-2140395) to support SCOR Working Group #162 (OASIS) activities. Working Group #162 (OASIS) activities. Elysium EPL were not involved in study design, data collection and analysis, decision to publish or preparation of the manuscript. SG acknowledges support from NASA (80NSSC24M0010). SS and MD have received funding from the European Union’s Horizon Europe ERC Synergy Grant programme under grant agreement No. 101118693 (WHIRLS). S-AN, SS, and MD have received funding from the European Union’s Horizon 2020 Research and Innovation Program under grant agreement No. 821001 (SO-CHIC). S-AN acknowledges support from the Department of Science and Innovation (DSI/CON C3184/2023) and from NRF SA National Antarctic Programme (SANAP230503101416). ES acknowledges support from the Natural Environment Research Council and the ARIES Doctoral Training Partnership [grant number NE/S007334/1].
We thank all those providing USV metadata in order to provide the global USV coverage maps and information. We thank Matt Donnelly for providing critical feedback on the manuscript. SG acknowledges support from NASA (80NSSC24M0010). This is PMEL publication #5684.
Author RP was employed by the company Elysium EPL. Author AP was employed by company Astraeus Ocean Systems. Authors LC and VR were employed by Akvaplan-niva. Author MF was employed by the company Seasats. Author DP was employed by company Offshore Sensing. Author NR was employed by the company Ocius Technology LTD. Author ES has a NERC CASE PhD studentship which receives financial support from AutoNaut Ltd.
The remaining authors declare that the research was conducted in the absence of any commercial or financial relationships that could be construed as a potential conflict of interest.
The author(s) declare that no Generative AI was used in the creation of this manuscript.
All claims expressed in this article are solely those of the authors and do not necessarily represent those of their affiliated organizations, or those of the publisher, the editors and the reviewers. Any product that may be evaluated in this article, or claim that may be made by its manufacturer, is not guaranteed or endorsed by the publisher.
Amador A., Merrifeild S. T., Terrill E. J. (2023). Assessment of atmospheric and oceanographic measurements from an autonomous surface vehicle. J. Atmos. Ocean. Technol. 40 (3), 305–326. doi: 10.1175/JTECH-D-22-0060.1
Angus J., Bouvier-Brown M., Robinson A. (2022). “Solar powered Uncrewed Surface Vehicles (USVs) for Marine Protected Area (MPA) monitoring,” in OCEANS 2022 MTS/IEEE, Hampton Roads, 17-20 Oct 2022. doi: 10.1109/OCEANS47191.2022.9977292
Aniceto A. S., Pedersen G., Primicerio R., Biuw M., Lindstrøm U., Camus L. (2020). Arctic marine data collection using oceanic gliders: Providing ecological context to cetacean vocalizations. Front. Mar. Sci. 7. doi: 10.3389/fmars.2020.585754
Bandara K., Basedow S. L., Pederson G., Tverberg V. (2022). Mid-summer vertical behaviour of a high latitude oceanic zooplankton community. J. Mar. Syst. 230, 103733. doi: 10.1016/j.jmarsys.2022.103733
Baumgartner M. F., Stafford K. M., Winsor P., Statscewich H., Fratantoni D. M. (2014). Glider-based passive acoustic monitoring in the Arctic. Mar. Technol. Soc. J. 48, 40–51. doi: 10.4031/MTSJ.48.5.2
Beal L. M., Vialard J., Roxy M. K. (2019). Full Report. IndOOS-2: A roadmap to sustained observations of the Indian Ocean for 2020-2030. CLIVAR-4/2019 GOOS-237, 206. doi: 10.36071/clivar.rp.4.2019
Beal L. M., Vialard J., Roxy M. K., Li J., Anres M., Annamalai H., et al. (2020). A road map to IndOOS-2: Better Observations of the rapidly warming Indian Ocean. Bull. Am. Meteorol. Soc. 101, E1891–E1913. doi: 10.1175/BAMS-D-19-0209.1
Berger J., Laske G., Babcock J., Orcutt J. (2016). An ocean bottom seismic observatory with near real-time telemetry. Earth Space Sci. 3, 68–77. doi: 10.1002/2015EA000137
Bingham B., Kraus N., Howe B., Freitag L., Ball K., Koski P., et al. (2012). Passive and active acoustics using an autonomous Wave Glider. J. Field Robot. 29 (6), 911–923. doi: 10.1002/rob.21424
Boyer T., Zhang H.-M., O-Brien K., Reagan J., Diggs S., Freeman E., et al. (2023). Effects of the pandemic of observing the global ocean. Am. Meteorol. Soc. 104, E389–E410. doi: 10.1175/BAMS-D-21-0210.1
Bushnell M. (Ed.) (2017). QARTOD Project Plan Accomplishments for 2012-2016 and Update for 2017-2021 (Silver Spring, MD, U.S: Department of Commerce, National Oceanic and Atmospheric Administration, National Ocean Service, Integrated Ocean Observing System), 48. doi: 10.7289/V5JQ0Z71
Camus L., Andrade H., Aniceto A. S., Aune M., Bandara K., Basedow S. L., et al. (2021). Autonomous surface and underwater vehicles as effective ecosystem monitoring and research platforms in the Arctic—The Glider Project. Sensors 21, 6752. doi: 10.3390/s21206752
Carroll S. R., Garba I., Figueroa-Rodrıguez O. L., Holbrook J., Lovett R., Materechera S., et al. (2020). The CARE principles for indigenous data governance. Data Sci. J. 19 (43), 1–12. doi: 10.5334/dsj-2020-043
Centurioni L. R. (2018). “Drifter technology and impacts for sea surface temperature, sea level pressure, and ocean circulation studies,” in Observing the Oceans in Real Time. Eds. Venkatesan R., Tandon A., D’Asaro E., Atmanand M. (Springer Oceanography. Springer, Cham). doi: 10.1007/978-3-319-66493-4_3
Centurioni L. R., Turton J., Lumpkin R., Braasch L., Brassington G., Chao Y., et al. (2019). Global in situ observation of essential climate and ocean variables at the air-sea interface. Front. Mar. Sci. 6. doi: 10.3389/fmars.2019.00419
Chang Y.-C., Zhang C., Wang N. (2024). The international legal status of the unmanned maritime vehicles. Mar. Pol. 113, 103830. doi: 10.1016/j.marpol.2020.103830
Chi N.-H., Zhang D., Zhang C. (2023). Validation of OSCAR surface currents in the western arctic marginal seas against saildrone observations. Earth Space Sci. 10, e2022EA002612. doi: 10.1029/2022EA002612
Chiodi A. M., Hristova H., Foltz G. R., Zhang J. A., Mordy C., Edwards C., et al. (2024). Surface ocean warming near the core of Hurricane Sam and its representation in forecast models. Front. Mar. Sci. 10. doi: 10.3389/fmars.2023.1297974
Chiodi A. M., Zhang C., Cokelet E. D., Yang Q., Mordy C. W., Gentemann C., et al. (2021). Exploring the Pacific arctic seasonal ice zone with saildrone USVs. Front. Mar. Sci. 8. doi: 10.3389/fmars.2021.640697
Chu D., Parker-Stetter S., Hufnagle L. C., Thomas R., Getsiv-Clemons J., Gauthier S. (2019). “2018 Unmanned Surface Vehicle (Saildrone) acoustic survey off the west coasts of the United States and Canada,” in OCEANS 2019 MTS/IEEE, Seattle, 27-31 Oct 2019. doi: 10.23919/OCEANS40490.2019.8962778
Clayson C. A., DeMott C. A., de Szoeke S. P., Chang P., Foltz G. R., Krishnamurthy R., et al. (2023). A new paradigm for observing and modeling of the air-sea interactions to advance earth system prediction (Washington DC: A US CLIVAR Report, US CLIVAR Project Office), 86. doi: 10.5065/24j7-w583
Cokelet E. D., Meinig C., Lowrence-Salvas N., Stabeno P. J., Mordy C. W., Tabisola H. M., et al. (2015). “The use of Saildrones to examine spring conditions in the Bering Sea,” in OCEANS 2015 MTS/IEEE, Washington, 19-22 Oct 2015. doi: 10.23919/OCEANS.2015.7404357
Colbo K., Weller R. A. (2009). Accuracy of the IMET sensor package in the subtropics. J. Atmos. Ocean. Technol. 26, 1867–1890. doi: 10.1175/2009JTECHO667.1
Colosi L., Pizzo N., Grare L., Statom N., Lenain L. (2023). Observations of surface gravity wave spectra from moving platforms. Am. Meteorol. Soc. 40, 1153–1169. doi: 10.1175/JTECH-D-23-0022.1
Crance J. L., Berchock C. L., Tabisola H. (2016). Saildrone Summer 2016 Bering Sea Mission—Final Report. Available online at: https://www.mmc.gov/wp-content/uploads/Saildrone-2017-MMC-Final-Report.pdf (Accessed September 3 2024). Submitted to the Marine Mammal Commission Under IA AKC-196.
Cravatte S., Kessler W. S., Smith N., Wijffels S., Ando K., Cronin M., et al. (2016). TPOS 2020 project. First report. GOOS-215, TPOS 2020 200. Available online at http://tpos2020.org/first-report/ (Accessed February 5, 2025).
Crews L., Lee C. M., Rainville L., Thomson J. (2022). Direct observations of the role of lateral advection of sea ice meltwater in the onset of autumn freeze up. J. Geophys. Res. 127, e2021JC01777. doi: 10.1029/2021JC017775
Cronin M. F., Gentleman C. L., Edson J., Ueki I., Bourassa M., Brown S., et al. (2019). Air-sea fluxes with a focus on heat and momentum. Front. Mar. Sci. 6. doi: 10.3389/fmars.2019.00430
Cronin M. F., Swart S., Marandino C. A., Anderson C., Browne P., Chen S., et al. (2023). Developing and Observing Air-Sea Interaction Strategy (OASIS) for the global ocean. ICES J. Mar. Sci. 80, 367–373. doi: 10.1093/icesjms/fsac149
Cronin M. F., Zhang D., Wills S. M., Reeves Eyre J. E., Thompson L. A., Anderson N. (2024). Diurnal warming rectification in the tropical Pacific linked to sea surface temperature front. Nat. Geosci. 17, 316–322. doi: 10.1038/s41561-024-01391-8
Cynar H., Juranek L. W., Mordy C. W., Strausz D., Bell S. (2022). High-resolution biological net community production in the Pacific-influenced Arctic as constrained by O2/Ar and O2/N2 observations. Deep-Sea Res. Part II. 206, 105214. doi: 10.1016/j.dsr2.2022.105214
Daniel T., Manley J., Trenaman N. (2011). The Wave Glider: enabling a new approach to persistent ocean observation and research. Ocean Dynam. 61, 1509–1520. doi: 10.1007/s10236-011-0408-5
Davis R., Baumgartner M., Comeau A., Cunningham D., Davies K., Furlong A., et al. (2016). “Tracking whales on the Scotian Shelf using passive acoustic monitoring on ocean gliders,” in OCEANS 2016 MTS/IEEE, Monterey, 19-23 Sep 2016. doi: 10.1109/OCEANS.2016.7761461
de Robertis A., Lawrence-Salvas N., Jenkins R., Wangen I., Mordy C. W., Meinig C., et al. (2019). Long-term measurements of fish backscatter from Saildrone unmanned surface vehicles and comparison with observations from a noise-reduced research vessel. ICES J. Mar. Sci. 76, 2459–2470. doi: 10.1093/icesjms/fsz124
de Robertis A., Levine M., Lauffenburger N., Honkalehto T., Ianelli J., Monnahan C. C., et al. (2021). Uncrewed Surface Vehicle (USV) survey of walleye pollock, Gadus Chalcogrammus, in response to the cancellation of ship-based surveys. ICES J. Mar. Sci. 78, 2797–2898. doi: 10.1093/icesjms/fsab155
Drushka K., Westbrook E., Bingham F., Gaube P., Dickinson S., Fournier S., et al. (2024). Salinity and Stratification at the Sea Ice Edge (SASSIE): An oceanographic field campaign in the Beaufort Sea. Earth Sys. Sci. Data. 16 (9), 4209–4242. doi: 10.5194/essd-2023-406
Dunn M., Pederson G., Basedow S. L., Daase M., Falk-Peterson S., Bachelot L., et al. (2023). Inverse method applied to autonomous broadband hydroacoustic survey detects higher densities of zooplankton in near-surface aggregations than vessel-based net survey. Can. J. Fish. Aquat. Sci. 80, 451–467. doi: 10.1139/cjfas-2022-0105
Du Plessis M. D., Swart S., Biddle L. C., Giddy I. S., Monteiro P. M. S., Reason C. J. C., et al. (2022). The daily-resolved southern ocean mixed layer: regional contrasts assessed using glider observations. J. Geophys. Res.: Oceans. 127, e2021JC017760. doi: 10.1029/2021JC017760
Edholm J. M., Swart S., Plessis M. D., Nicholson A. (2022). Atmospheric rivers contribute to summer surface buoyancy forcing in the Atlantic sector of the southern ocean. Geophys. Res. Lett. 49, e2022GL100149. doi: 10.1029/2022GL100149
Farrar J. T., D’Asaro E., Rodriguez E., Shcherbina A., Czech E., Matthias P., et al. (2020). “S-MODE: The sub-mesoscale ocean dynamics experiment,” in IGARSS 2020 IEEE International Geoscience and Remote Sensing Symposium, Waikoloa, 26 Sep - 2 Oct 2020. doi: 10.1109/IGARSS39084.2020.9323112
Fassbender A. J, Sabine C. J., Cronin M. F., Sutton A. J. (2017). Mixed-layer carbon cycling at the Kuroshio extension observatory. Global Biogeochem. Cycles 30 (2), 272–228. doi: 10.1002/2016GB005547
Foltz G. R., Zhang C., Meinig C., Zhang J. A., Zhang D. (2022). An unprecedented view inside a hurricane. Eos 103. doi: 10.1029/2022EO220228
Foster J. H., Erickson T. L., Bingham B. (2020). Wave glider-enhanced vertical seafloor geodesy. Am. Meteorol. Soc. 37, 417–427. doi: 10.1175/JTECH-D-19-0095.1
Gentemann C., Clayson C. A., Brown S., Lee T., Parfitt R., Farrar J. T., et al. (2020a). FluxSat: Measuring the ocean-atmosphere turbulent exchange of heat and moisture from space. Remote Sens. 12, 1796. doi: 10.3390/rs12111796
Gentemann C. L., Scott J. P., Mazzini P. L. F., Pianca C., Akella S., Minnett P. J., et al. (2020b). Saildrone: adaptively sampling the marine environment. Bull. Am. Meteorol. Soc. 101, E744–E762. doi: 10.1175/BAMS-D-19-0015.1
Ghani M. H., Hole L. R., Fer I., Kourafalou V. H., Wienders N., Kang H., et al. (2014). The SailBuoy remotely-controlled unmanned vessel: Measurements of near surface temperature, salinity and oxygen concentration in the northern Gulf of Mexico. Methods Oceanogr. 10, 104–121. doi: 10.1016/j.mio.2014.08.001
Gille S., Josey S., Swart S. (2016). New approaches for air-sea fluxes in the Southern Ocean. Eos 97. doi: 10.1029/2016EO052243
Goebel N. L., Frolov S., Edwards C. A. (2014). Complementary use of Wave Glider and satellite measurements: Description of spatial decorrelation scales in Chl-a fluorescence across the Pacific basin. Methods Oceanogr. 10, 90–103. doi: 10.1016/j.mio.2014.07.001
GOOS (2018). Observations Coordination Group (OCG) network attributes, commitments and benefits – what it means to be a an OCG network, Report GOOS-266. Available online at: https://oceanexpert.org/document/24002 (Accessed January 14 2024).
GOOS (2021). Ocean observations in areas under national jurisdiction workshop. Report no. GOOS-246 (Paris: IOC/UNESCO). Available online at: https://goosocean.org/document/26607 (Accessed February 5 2025).
Grare L., Statom N. M., Pizzo N., Lenain L. (2021). Instrumented Wave Gliders for air-sea interaction and upper ocean research. Front. Mar. Sci. 8. doi: 10.3389/fmars.2021.664728
Guihen D., Fielding S., Murphy E. J., Heywood K. J., Griffiths G. (2014). An assessment of the use of ocean gliders to undertake acoustic measurements of zooplankton: the distribution and density of Antarctic krill (Euphausia superba) in the Weddell Sea. Limnol. Oceanogr.: Methods 12, 373–389. doi: 10.4319/lom.2014.12.373
Handegard N. O., de Robertis A., Holmin A. J., Johnsen E., Lawrence J., Bouffant N. L., et al. (2024). Uncrewed surface vehicles (USVs) as platforms for fisheries and plankton acoustics. ICES J. Mar. Sci., 1–12. doi: 10.1093/icesjms/fsae130
Hays G. C., Ferreira L. C., Sequeira A. M. M., Meekan M. G., Duarte C. M., Bailey H., et al. (2016). Key questions in marine megafauna movement ecology. Trends Ecol. Evol. 31, 463–474. doi: 10.1016/j.tree.2016.02.015
Hemsley V. S., Smyth T. J., Martin A. P., Frajka-Williams E., Thompson A. F., Damerell G., et al. (2015). Estimating oceanic primary production using vertical irradiance and chlorophyll profiles from ocean gliders in the North Atlantic. Environ. Sci. Technol. 49, 11612–11621. doi: 10.1021/acs.est.5b00608
Hermes J. C., Masumoto Y., Beal L. M., Roxy M. K., Vialard J., Andres M., et al. (2019). A sustained Ocean Observing System in the Indian Ocean for climate related scientific knowledge and societal needs. Front. Mar. Sci. 6. doi: 10.3389/fmars.2019.00355
Hildebrand J. A., D’Spain G. L., Wiggins S. M. (2014). Glider-based passive acoustic marine mammal detection. J. Acoust. Soc. America. 136, 2118. doi: 10.1121/1.4899628
Hildebrand J. A., Gentes Z. E., Johnson S. C., Frasier K. E., Merkens K., Thayre B. J., et al. (2013). Acoustic monitoring of cetaceans in the northern Gulf of Mexico using Wave Gliders equipped with high-frequency acoustic recording packages. Report for Research Agreement 20105138. Marine Physical Laboratory. Technical Memorandum 539. Available online at: https://www.cetus.ucsd.edu/docs/reports/MPLTM539-2013.pdf (Accessed February 5, 2025).
Hodges B. A., Grare L., Greenwood B., Matsuyoshi K., Pizzo N., Statom N. M., et al. (2023). Evaluation of ocean currents observed from autonomous surface vehicles. J. Atmos. Ocean. Technol. 40 (10), 1121–1136. doi: 10.1175/JTECH-D-23-0066.1
Hole L. R., Fer I., Peddie D. (2016). Directional wave measurements using an autonomous vessel. Ocean Dynam. 66, 1087–1098. doi: 10.1007/s10236-016-0969-4
Iinuma T., Kido M., Ohta Y., Fukuda T., Tomita F., Ueki I. (2021). GNSS-acoustic observations from seafloor crustal deformation using a Wave Glider. Front. Earth Sci. 9. doi: 10.3389/feart.2021.600946
Ino K., Sugiyama S., Takahiro K., Chikara T., Rika N., Hidemi O., et al. (2021). Operation and observations of the autonomous ocean vehicle (AOV). Report of Hydrographic and Oceanographic Researches. Available online at: https://aquadocs.org/handle/1834/41921 (Accessed October 16 2024).
IOC (Intergovernmental Oceanographic Commission) of UNESCO (2018). History of development of GOOS. Document code: IOC/INF-1361. Available online at: https://unesdoc.unesco.org/ark:/48223/pf0000264651 (Accessed October 30 2024).
Iyer S., Drushka K., Thompson E. J., Thompson J. (2022). Small-scale spatial variations of air-sea heat, moisture, and buoyancy fluxes in the tropical trade winds. J. Geophys. Res. Oceans. 127, 1–21. doi: 10.1029/2022JC018972
Jia C., Minnett P. J. (2023). Ocean warm skin signals observed by Saildrone at high latitudes. Geophys. Res. Lett. 50, 1–9. doi: 10.1029/2022GL102384
Jia C., Minnett P. J., Szczodrak M., Izaguirre M. (2023). High latitude sea surface skin temperatures derived from Saildrone infrared measurements. Trans. Geosci. Remote Sens. 61, 1–14. doi: 10.1109/TGRS.2022.3231519
Johnson G., Fassbender A. (2023). After two decades, argo at PMEL, looks to the future. Oceanography. 36 (2-3), 54–59. doi: 10.5670/oceanog.2023.223
Johri S., Carnevale M., Porter L., Zivian A., Kourantidou M., Meyer E., et al. (2021). Pathways to justice, equity, diversity and inclusion in marine science and conservation. Front. Mar. Sci. 8. doi: 10.3389/fmars.2021.696180
Kosaka N., Umemiya Y., Endou N., Kura T., Matsubara H., Hisada M., et al. (2023). Simultaneous observations of atmosphere and ocean directly under typhoons using autonomous surface vehicles. SOLA 19, 116–125. doi: 10.2151/sola.2023-016
Koslow A. J., Couture J. (2015). Pacific Ocean observation programs: Gaps in ecological time series. Mar. Pol. 51, 408–414. doi: 10.1016/j.marpol.2014.09.003
Krug M., Swart S., Gula J. (2017). Submesoscale cyclones in the Agulhas current. Geophys. Res. Lett. 44, 346–354. doi: 10.1002/2016GL071006
Kuhn C. E., de Robertis A., Sterling J., Mordy C. W., Meinig C., Lawrence-Slavas N., et al. (2020). Test of unmanned surface vehicles to conduct remote focal follow studies of a marine predator. Mar. Ecol. Prog. Series. 635, 1–7. doi: 10.3354/meps13224
Lenain L., Melville W. K. (2014). Autonomous surface vehicle measurements of the ocean’s response to tropical cyclone Freda. Am. Meteorol. Soc. 31, 2169–2190. doi: 10.1175/JTECH-D-14-00012.1
Levine R. M., de Robertis A., Grunbaum D., Woodgate R., Mordy C. W., Mueter F., et al. (2021). Autonomous vehicle surveys indicate that flow reversal retain juvenile fishes in a highly advective high-latitude ecosystems. Limnol. Oceanogr. 66, 1139–1154. doi: 10.1002/lno.11671
Lin D., Crabtree J., Dillo I., Downs R. R., Edmunds R., Giaretta D., et al. (2020). The TRUST Principles for digital repositories. Sci. Data. 7, 144. doi: 10.1038/s41597-020-0486-7
Lindstrom E., Bryan F., Schmitt R. (2015). SPURS: salinity process in the upper-ocean regional study - the North Atlantic experiment. Oceanography 28, 14–19. doi: 10.5670/oceanog.2015.01
McKenna J. R., Sunkara V., Kar S., Schmachtenberger C., Arguelles A., Arnold M. (2023). “Maritime workforce training for the new Blue Economy,” in OCEANS 2023 MTS/IEEE, Biloxi, 25-28 Sep 2024. doi: 10.23919/OCEANS52994.2023.10337396
Meinig C., Burger E. F., Cohen N., Cokelet E. D., Cronin M. F., Cross J. N., et al. (2019). Public private partnerships to advance regional ocean observing capabilities: A Saildrone and NOAA-PMEL case study and future considerations to expand to global scale observing. Front. Mar. Sci. 6. doi: 10.3389/fmars.2019.00448
Meinig C., Jenkins R., Lawrence-Slavas N., Tabisola H. (2015). “The use of Saildrones to examine spring conditions in the Bering Sea: vehicle specification and mission performance,” in OCEANS 2015 MTS/IEEE, Washington, 19-22 Oct 2015. doi: 10.23919/OCEANS.2015.7404348
Miles T. N., Zhang D., Foltz G., Zhang J., Meinig C., Bringas F., et al. (2021). Uncrewed ocean gliders and Saildrones support hurricane forecasting and research. Oceanography 34, 78–81. doi: 10.5670/oceanog.2021.supplement.02-28
Mitarai S., McWilliams J. C. (2016). Wave glider observations of surface winds and currents in the core of Typhoon Danas. Geophys. Res. Lett. 43, 11,312–11,319. doi: 10.1002/2016GL071115
Molina-Molina J. C., Salhaoui M., Guerrero-Gonzalez A., Arioua M. (2021). Autonomous marine robot based on AI recognition for permanent surveillance in marine protected areas. Sensors 21, 2664. doi: 10.3390/s21082664
Monteiro P. M., Gregor L., Lévy M., Maenner S., Sabine C. L., Swart S. (2015). Intraseasonal variability linked to sampling alias in air-sea CO2 fluxes in the Southern Ocean. Geophys. Res. Lett. 42, 8507–8514. doi: 10.1002/2015GL066009
Moore S. E., Howe B. M., Stafford K. M., Boyd M. L. (2007). Including whale call detection in standard ocean measurements: Application of acoustic seagliders. Mar. Technol. Soc. J. 41, 53–57. doi: 10.4031/002533207787442033
Mordy C. W., Cokelet E. D., de Robertis A., Jenkins R., Kuhn C. E., Lawrence-Slavas N., et al. (2017). Advances in ecosystem research: Saildrone surveys of oceanography, fish, and marine mammals in the Bering Sea. Oceanography 30, 113–115. doi: 10.5670/oceanog.2017.230
Mullsion J., Symonds D., Trenaman N. (2011).ADCP data collected from a Liquid Robotics Wave Glider (IEEE). Available online at: https://ieeexplore.ieee.org/document/5759563 (Accessed October 18 2024).
Nagano A., Ando K. (2020). Saildrone-observed atmospheric boundary layer response to winter mesoscale warm spot along the Kuroshio south of Japan. Prog. Earth Planet. Sci. 7, 1–16. doi: 10.1186/s40645-020-00358-8
Nagano A., Geng B., Richards K. J., Cronin M. F., Taniguchi K., Katsumata M., et al. (2022a). Coupled atmosphere-ocean variations on timescales of days observed in the Western Tropical Pacific warm pool during mid-March 2020. J. Geophys. Res. Oceans. 127, e2022JC019032. doi: 10.1029/2022JC019032
Nagano A., Hasegawa T., Ariyoshi K., Iinuma T., Fukuda T., Fujii N., et al. (2022b). USV-Observed turbulent heat flux induced by late spring cold dry air incursion over sub-mesoscale warm regions off Sanriku, Japan. Sensors 22, 9695. doi: 10.3390/s22249695
Nicholson S.-A., Whitt D. B., Fer I., Du Plessis M. D., Lebehot A. D., Swart S., et al. (2022). Storms drive outgassing of CO2 in the subpolar Southern Ocean. Nat. Commun. 14, 158. doi: 10.1038/s41467-021-27780-w
Nickford S., Palter J. B., Donohue K., Fassbender A. J., Gray A. R., Long J., et al. (2022). Autonomous wintertime observations of air-sea exchange in the Gulf Stream reveal a perfect storm for ocean CO2 uptake. Geophys. Res. Lett. 49, e2021GL096805. doi: 10.1029/2021GL096805
Nickford S., Palter J. B., Mu L. (2024). The importance of contemporaneous wind and pCO2 measurements for regional air-sea CO2 flux estimates. J. Geophys. Res. Oceans. 129, e2023JC020744. doi: 10.1029/2023JC020744
Nothacker J. S. (2024). (Massachusetts Institute of Technology). Available online at: https://dspace.mit.edu/handle/1721.1/155656 (Accessed February 5 2025).
Pagniello C. M. L. S., Cimino M. A., Terrill E. (2019). Mapping fish chorus distributions in southern California using an autonomous Wave Glider. Front. Mar. Sci. 6. doi: 10.3389/fmars.2019.00526
Parks J., Hanstein C., Kramp M., Strom K., Cabrie J., Rodriguez J. J., et al. (2024). Vessel recruiting best practices. v. 1.2. Available online at: https://repository.oceanbestpractices.org/bitstream/handle/11329/2583/Ship%20recruitment%20BP%201.2.pdf?sequence=3&isAllowed=y (Accessed October 7 2024).
Patterson R. G., Lawson E., Udyawer V., Brassington G. B., Groom R. A., Campbell H. A. (2022). Uncrewed surface vessel technological diffusion depends on cross-sectoral investment in open-ocean archetypes: A systematic review of USV applications and drivers. Front. Mar. Sci. 8. doi: 10.3389/fmars.2021.736984
Pedersen G., Peddie D., Falk-Petersen S., Dunlop K., Camus L., Daase M., et al. (2019). “Autonomous surface vehicles for persistent acoustic monitoring of zooplankton in a highly productive shelf area,” in OCEANS 2019 MTS/IEEE, Marseille, 17-20 Jun 2019. doi: 10.1109/oceanse.2019.8867089
Penna N. T., Morales Maqueda M. A., Martin I., Guo J., Fodin P. R. (2018). Sea surface height measurement using a GNSS Wave Glider. Geophys. Res. Lett. 45, 5609–5616. doi: 10.1029/2018GL077950
Penny S. G., Akella S., Balmaseda M. A., Browne P., Carto J. A., Chevallier M., et al. (2019). Observational needs for improving ocean and coupled reanalysis, S2S prediction, and decadal prediction. Front. Mar. Sci. 6. doi: 10.3389/fmars.2019.00391
Premus V. E., Abbot P. A., Kmelnitsky V., Gedney C. J., Abbot T. A. (2022). A wave glider-based, towed hydrophone array system for autonomous, real-time, passive acoustic marine mammal monitoring. J. Acoust. Soc. America. 152, 1814–1828. doi: 10.1121/10.0014169
Preston C., Yamahara K., Pargett D., Weinstock C., Birch J., Roman B., et al. (2023). Autonomous eDNA collection using an uncrewed surface vessel over a 4200-km transect of the eastern Pacific Ocean. Environ. DNA. 6, 1–18. doi: 10.1002/edn3.468
Reeves Eyre J. E. J., Cronin M. F., Zhang D., Thompson E. J., Fairall C. W., Edson J. B. (2023). Saildrone direct covariance wind stress in various wind and current regimes of the Tropical Pacific. J. Atmos. Ocean. Technol. 40, 503–517. doi: 10.1175/JTECH-D-22-0077.1
Ricciardulli L., Foltz G. R., Manaster A., Meissner T. (2022). Assessment of Saildrone extreme wind measurements in Hurricane Sam using MW satellite sensors. Remote Sens. 14 (12), 2726. doi: 10.3390/rs14122726
Rodríguez E., Bourassa M., Chelton D., Farrar J. T., Long D., Perkovic-Martin D., et al. (2019). The winds and currents mission concept. Front. Mar. Sci. 6. doi: 10.3389/fmars.2019.00438
Roemmich D., Alford M. H., Claustre H., Johnson K., King B., Moum J., et al. (2019). On the future of Argo: A global, full-depth, multidisciplinary array. Front. Mar. Sci. 6. doi: 10.3389/fmars.2019.00439
Ryan E., Turkmen S., Benson S. (2020). An Investigation into the application and practical use of (UV) ultraviolet light technology for marine antifouling. Ocean Eng. 216, 107690. doi: 10.1016/j.oceaneng.2020.107690
Sabine C., Sutton A., McCabe K., Lawrence-Slavas N., Alin S., Feely R., et al. (2020). Evaluation of a new carbon dioxide system for autonomous surface vehicles. J. Atmos. Ocean. Technol. 37, 1305–1317. doi: 10.1175/JTECH-D-20-0010.1
Sakic P., Chupin C., Ballu V., Coulombier T., Morvan P.-Y., Urvoas P., et al. (2021). Geodetic seafloor positioning using an unmanned surface vehicle - contribution of direction-of-arrival observations. Front. Earth Sci. 9. doi: 10.3389/feart.2021.636156
Schmidt K. M., Swart S., Reason C., Nicholson S.-A. (2017). Evaluation of satellite and reanalysis wind products with in situ wave glider wind observations in the Southern Ocean. J. Atmos. Ocean. Technol. 34, 2551–2568. doi: 10.1175/jtech-d-17-0079.1
Scott J. P., Crooke S., Cetinić I., Del Castillo C. E., Gentemann C. L. (2020). Correcting non-photochemical quenching of Saildrone chlorophyll-a fluorescence for evaluation of satellite ocean color retrievals. Optics Express. 28, 4274. doi: 10.1364/oe.382029
Seegers B. N., Birch J. M., Marin R., Scholin C. A., Caron D. A., Seubert E. L., et al. (2015). Subsurface seeding of surface harmful algal blooms observed through the integration of autonomous gliders, moored environmental sample processors, and satellite remote sensing in southern California. Limnol. Oceanogr. 60, 754–764. doi: 10.1002/lno.10082
Seo H., O’Niell L. W., Bourassa M. A., Czaja A., Drushka K., Edson J. B., et al. (2023). Ocean mesoscale and frontal-scale ocean-atmosphere interactions and influence on large-scale climate: A review. J. Climate. 36, 1981–2013. doi: 10.1175/JCLI-D-21-0982.1
Siddle E., Heywood K. J., Webber B. G. M., Bromley P. (2021). First measurements of ocean and atmosphere in the Tropical North Atlantic using Caravela, a novel uncrewed surface vessel. Weather 76, 200–204. doi: 10.1002/wea.4004
Sivam S., Zhang C., Zhang D., Yu L., Dressel I. (2024). Surface latent and sensible heat flux over the Pacific Sub-Arctic Ocean from Saildrone observations and three global reanalysis products. Front. Mar. Sci. 11. doi: 10.3389/fmars.2024.1431718
Sloyan B. M., Roughan M., Hill K. (2018). Chapter 3: the global ocean observing system. New Front. In Operat. Oceanogr, 75–90. Available online at: http://purl.flvc.org/fsu/fd/FSU_libsubv1_scholarship_submission_1536167723_386010b6 (Accessed February 5, 2025).
Smith N., Kessler W. S., Cravatte S., Sprintall J., Wijffels S., Cronin M. F., et al. (2019). Tropical Pacific observing system. Front. Mar. Sci. 6. doi: 10.3389/fmars.2019.00031
Smith M., Thomson J. (2016). Scaling observations of surface waves in the Beaufort Sea. Elem.: Sci. Anthrop. 4, 97. doi: 10.12952/journal.elementa.000097
SPURS Project, Bingham F. (2015). SPURS-1 FIeld campaign Wave Glider data products. Ver. 1.0. PO.DAAC, CA, USA (Accessed September 27 2024).
Sutton A. J., Williams N. L., Tilbrook B. (2021). Constraining Southern Ocean CO2 flux uncertainty using uncrewed surface vehicle observations. Geophys. Res. Lett. 48, e2020GL091748. doi: 10.1029/2020GL091748
Swart S., Du Plessis M. D., Nicholson S.-A., Monteiro P. M. S., Dove L. A., Thomalla S., et al. (2023). The Southern Ocean mixed layer and its boundary fluxes: fine-scale observational progress and future research priorities. Philos. Trans. R. Soc. A. 381, 20220058. doi: 10.1098/rsta.2022.0058
Swart S., Du Plessis M. D., Thompson A. F., Biddle L. C., Giddy I., Linkders T., et al. (2020). Submesoscale fronts in the Antarctic marginal ice zone and their response to wind forcing. Geophys. Res. Lett. 47, e2019GL086649. doi: 10.1029/2019GL086649
Swart S., Gille S. T., Delille B., Josey S., Mazloff M., Newman L., et al. (2019). Constraining Southern Ocean air-sea-ice fluxes through enhanced observations. Front. Mar. Sci. 6. doi: 10.3389/fmars.2019.00421
Swart S., Zietsman J. J., Coetzee J. C., Goslett D. G., Hoek A., Needham D., et al. (2016). Ocean robotics in support of fisheries research and management. Afr. J. Mar. Sci. 38, 525–538. doi: 10.2989/1814232X.2016.1251971
Tada N., Nagano A., Tanaka S., Ichihara H., Suetsugu D., Matsuoka D., et al. (2024). Challenge for multifaceted data acquisition around active volcanoes using uncrewed surface vessel. Front. Mar. Sci. 11. doi: 10.3389/fmars.2024.1406381
Tanhua T., Pouliquen S., Jausman J., O’Brien K., Bricher P., de Bruin T., et al. (2019). Ocean FAIR data services. Front. Mar. Sci. 6. doi: 10.3389/fmars.2019.00440
Thomson J., Girton J. (2017). Sustained measurements of Southern Ocean air-sea coupling from a Wave Glider autonomous surface vehicle. Oceanogr. Mag. 30, 104–109. doi: 10.5670/oceanog.2017.228
Thomson J., Girton J., Jha R., Trapani A. (2018). Measurements of directional wave spectra and wind stress from a Wave Glider autonomous surface vehicle. J. Atmos. Ocean. Technol. 35, 347–363. doi: 10.1175/JTECH-D-17-0091.1
Thomson J., Talbert J., de Klerk A., Brown A., Schwendeman M., Goldsmith J., et al. (2015). Biofouling effects on the response of a wave measurement buoy in deep water. J. Atmos. Ocean. Technol. 32, 1281–1286. doi: 10.1175/JTECH-D-15-0029.1
Toolsee T., Nicholson S.-A., Monteiro P. M. S. (2024). Storm-driven pCO2 feedback weakens the response of air-sea CO2 fluxes in the sub-Antarctic Southern Ocean. Geophys. Res. Lett. 51, e2023GL107804. doi: 10.1029/2023GL107804
Vazquez-Cuervo J., Gomez-Valdes J., Bouali M., Miranda L. E., van der Stocken T., Tang W., et al. (2019). Using Saildrones to validate satellite-derived sea surface salinity and sea surface temperature along the California/Baja coast. Remote Sens. 11, 1964. doi: 10.3390/rs11171964
Villareal T. A., Wilson C. (2014). A comparison of the pac-X trans-pacific wave glider data and satellite data (MODIS, Aquarius, TRMM and VIIRS). PloS One 9, e92280. doi: 10.1371/journal.pone.0092280
Wanninkhof R., Pickers P. A., Omar A. M., Sutton A., Murata A., Olsen A., et al. (2019). A surface ocean CO2 reference network, SOCONET and associated marine boundary layer CO2 measurements. Front. Mar. Sci. 6. doi: 10.3389/fmars.2019.00400
Whitt C., Pearlman J., Polagye B., Caimi F., Muller-Karger F., Copping A., et al. (2020). Future vision for autonomous ocean observations. Front. Mar. Sci. 7. doi: 10.3389/fmars.2020.00697
Wiggins S. M. (2009). Integration and use of a High-frequency Acoustic Recording Package (HARP) on a Wave Glider. Internal Project Report MPL TM-528. Liquid Robotics. Available online at: https://www.kimerius.com/app/download/5785636992/Integration+and+use+of+a+high-frequency+acoustic+recording+package+on+a+wave+glider.pdf (Accessed November 1 2024).
Wilkinson M. D., Dumontier M., Aalbersberg I. J., Appleton G., Axton M., Baak A., et al. (2016). The FAIR Guiding Principles for scientific data management and stewardship. Sci. Data. 3), 160018. doi: 10.1038/sdata.2016.18
Wills S. M., Cronin M. F., Zhang D. (2021). Cold pools observed by uncrewed surface vehicles in the central and eastern Tropical Pacific. Geophys. Res. Lett. 48, e2021GL093373. doi: 10.1029/2021GL093373
Wills S. M., Cronin M. F., Zhang D. (2023). Air-sea heat fluxes associated with convective cold pools. J. Geophys. Res.: Atmos. 128, e2023JD039708. doi: 10.1029/2023JD039708
Wood K. R., Overland J. E., Salo S. A., Bond N. A., Williams W. J., Dong X. (2013). Is there a “new normal” climate in the Beaufort Sea? Polar Res. 32, 19552. doi: 10.3402/polar.v32i0.19552
Wullenweber N., Hole L. R., Ghaffari P., Graves I., Tholo H., Camus L. (2022). SailBuoy ocean currents: low-cost upper-layer ocean current measurements. Sensors 22, 5553. doi: 10.3390/s22155553
Yu L., Chen Y., Gonzalez A. O., Zhang C., Foltz G. R. (2023). Dry air outbreak and significant surface turbulent heat loss during Hurricane Ian: Satellite and saildrone observations. Geophys. Res. Lett. 50, e2023GL105583. doi: 10.1029/2023GL105583
Zeiden K., Thomson J., Girton J. (2023). Estimating profiles of dissipation rate in the upper ocean using acoustic doppler measurements made from surface following platforms. J. Atmos. Ocean. Technol. 40, 1571–1589. doi: 10.1175/JTECH-D-23-0027.1
Zhang D., Chiodi A., Zhang C., Foltz G. R., Cronin M. F., Mordy C. W., et al. (2023b). Observing extreme ocean and weather events using innovative Saildrone uncrewed surface vehicles. Oceanography 36, 70–77. doi: 10.5670/oceanog.2023.214
Zhang D., Cronin M. F., Meinig C., Farrar J. T., Jenkins R., Peacock D., et al. (2019a). Comparing air-sea flux measurements from a new unmanned surface vehicle and proven platforms during the SPURS-2 Field Campaign. Oceanography 32, 122–133. doi: 10.5670/oceanog.2019.220
Zhang C., Foltz G. R., Chiodi A. M., Mordy C. W., Edwards C. R., Meinig C., et al. (2023a). Hurricane observations by uncrewed systems. Bull. Am. Meteorol. Soc. 104, E1893–E1917. doi: 10.1175/BAMS-D-21-0327.1
Zhang C., Levine A. F., Wang M., Gentemann C., Mordy C. W., Cokelet E. D., et al. (2022). Evaluation of surface conditions from operational forecasts using in situ Saildrone observations in the Pacific Arctic. Month. Weather Rev. 150, 1437–1455. doi: 10.1175/MWR-D-20-0379.1
Zhang Y., Rueda C., Kieft B., Ryan J. P., Wahl C., O’Reilly T. C., et al. (2019b). Autonomous tracking of an oceanic thermal front by a Wave Glider. J. Field Robot. 36, 940–954. doi: 10.1002/rob.21862
Keywords: Uncrewed Surface Vehicle (USV), Autonomous Surface Vessel (ASV), Air-sea interactions, Global Ocean Observing System (GOOS), In situ ocean observing system, Essential Ocean Variables (EOV), Essential Climate Variables (ECV), weather observation
Citation: Patterson RG, Cronin MF, Swart S, Beja J, Edholm JM, McKenna J, Palter JB, Parker A, Addey CI, Boone W, Bhuyan P, Buck JJH, Burger EF, Burris J, Camus L, de Young B, du Plessis M, Flanigan M, Foltz GR, Gille ST, Grare L, Hansen JE, Hole LR, Honda MC, Hormann V, Kohlman C, Kosaka N, Kuhn C, Lenain L, Looney L, Marouchos A, McGeorge EK, McMahon CR, Mitarai S, Mordy C, Nagano A, Nicholson S-A, Nickford S, O’Brien KM, Peddie D, Ponsoni L, Ramasco V, Rozenauers N, Siddle E, Stienbarger C, Sutton AJ, Tada N, Thomson J, Ueki I, Yu L, Zhang C and Zhang D (2025) Uncrewed surface vehicles in the Global Ocean Observing System: a new frontier for observing and monitoring at the air-sea interface. Front. Mar. Sci. 12:1523585. doi: 10.3389/fmars.2025.1523585
Received: 06 November 2024; Accepted: 10 February 2025;
Published: 07 March 2025.
Edited by:
Gilles Reverdin, Centre National de la Recherche Scientifique (CNRS), FranceReviewed by:
Zhixuan Feng, East China Normal University, ChinaCopyright © 2025 Patterson, Cronin, Swart, Beja, Edholm, McKenna, Palter, Parker, Addey, Boone, Bhuyan, Buck, Burger, Burris, Camus, de Young, du Plessis, Flanigan, Foltz, Gille, Grare, Hansen, Hole, Honda, Hormann, Kohlman, Kosaka, Kuhn, Lenain, Looney, Marouchos, McGeorge, McMahon, Mitarai, Mordy, Nagano, Nicholson, Nickford, O’Brien, Peddie, Ponsoni, Ramasco, Rozenauers, Siddle, Stienbarger, Sutton, Tada, Thomson, Ueki, Yu, Zhang and Zhang. This is an open-access article distributed under the terms of the Creative Commons Attribution License (CC BY). The use, distribution or reproduction in other forums is permitted, provided the original author(s) and the copyright owner(s) are credited and that the original publication in this journal is cited, in accordance with accepted academic practice. No use, distribution or reproduction is permitted which does not comply with these terms.
*Correspondence: Ruth G. Patterson, cGF0dGVyc29uLnJ1dGhAZ21haWwuY29t
†Early Career Research Professional authors (within 10 yrs of completion of PhD)
‡Student Authors
Disclaimer: All claims expressed in this article are solely those of the authors and do not necessarily represent those of their affiliated organizations, or those of the publisher, the editors and the reviewers. Any product that may be evaluated in this article or claim that may be made by its manufacturer is not guaranteed or endorsed by the publisher.
Research integrity at Frontiers
Learn more about the work of our research integrity team to safeguard the quality of each article we publish.