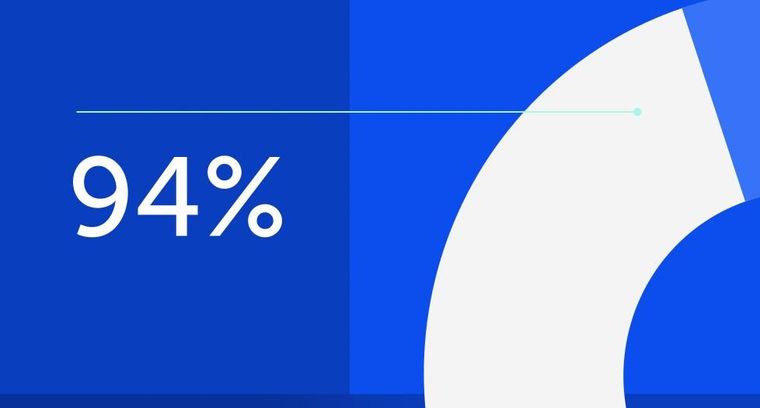
94% of researchers rate our articles as excellent or good
Learn more about the work of our research integrity team to safeguard the quality of each article we publish.
Find out more
ORIGINAL RESEARCH article
Front. Mar. Sci., 03 April 2025
Sec. Aquatic Microbiology
Volume 12 - 2025 | https://doi.org/10.3389/fmars.2025.1505754
This article is part of the Research TopicEffects of Human Activities on Microorganisms and Microbial Carbon Cycle in Coastal WatersView all 7 articles
Historically, oceanographic time-series have focused on long-term measurements of large open ocean gyres; yet, the coastal oceans, with their high productivity, tidal impacts, human feedbacks, and land-sea coupling, represent critical regions for predicting ocean dynamics and biogeochemistry under global change. The Piver’s Island Coastal Observatory (PICO) time-series, located in the second largest estuarine system on the US East Coast (Albemarle-Pamlico Sound), comprises more than a decade of weekly (or more frequent) measurements of core physical, chemical, and biological oceanographic variables. PICO provides insight into a coastal, mesotrophic ecosystem in an ecologically-diverse and biochemically-active region impacted by global change. Here, we report on a decade of observations focusing on pulse and press ecosystem changes. We observe strong mean annual cycles in environmental variables including temperature (10.1-28.9°C), pH (7.89-8.12), dissolved inorganic carbon (DIC: 1965 – 2088 µM), chlorophyll (2.54-5.77 mg Chl m-3), upon which are layered episodic disturbances (e.g., tropical cyclones) that dramatically and persistently (>1 month) impact this ecosystem. Among other variables, long term trends in pH (-0.004 ± 0.001 y-1; p<0.01), DIC (-9.8 ± 1.5 µM y -1; p<0.01) and chlorophyll (-0.17 ± 0.02 µg L-1 y-1; p<0.01) are exceeding those observed in the open ocean, suggesting an ecosystem in flux. These analyses provide a benchmark for future studies of the impact of changing climate and oceanographic climatology; further research will use this long-term research to developed targeted sampling and experimental manipulations to better understand ecosystem structure and function.
Despite the coastal ocean’s importance to global biogeochemistry and ecology; impact on fisheries, recreation, commerce and other human uses; and role as home for ~40% of the human population, coastal marine ecosystems are highly under-sampled, limiting our understanding of their temporal dynamics and sensitivity to environmental change. While proximity to land facilitates measurements, many nearshore environments exhibit high spatial and temporal variability, necessitating dense sampling (Hofmann et al., 2011; Zhao et al., 2022). Time-series, or repeated observations at the same location, allow us to characterize annual cycles in ecological and biogeochemical processes (Powell and Steele, 1995), and when sustained, capture interannual variability, pulse disturbances, as well as longer-term trends such as the impacts of changing climate (Keller et al., 2014).
However, most long-term marine time series are located in the open-ocean. For example, the continuous plankton recorder (1931) has tracked plankton abundance and diversity in the North Atlantic and shown, among other patterns, large scale shifts in species assemblages https://www.cprsurvey.org/. While hydrostation S (1954), now the Bermuda Atlantic Time-series Study (BATS: from 1988), has demonstrated strong annual patterns in hydrography, nutrients, particle flux, pigments and primary production, bacterioplankton abundance and production of the ecosystem (Steinberg et al., 2001) as well as the importance of episodic events like tropical cyclones (Bates et al., 1998). These sustained, high-precision measurements have captured the impacts of global change, including ocean acidification (Dore et al., 2003; Bates and Johnson, 2023). However, compared to their open-ocean counterparts, coastal time series are generally of more recent origin, but can be sampled more frequently; e.g., the San Pedro Ocean Time-series (Caron et al., 2017), Martha’s Vineyard Coastal Observatory (Hunter-Cevera et al., 2016) and Australia’s Integrated Marine Observing System (Brown et al., 2018). Further, in the last decade, advances in in situ instrumentation coupled with remote sensing enable monitoring of specific variables at high temporal resolution (Muller-Karger et al., 2018; Tilbrook et al., 2019). High-resolution measurements combined with open-ocean and near-coast time-series provide critical ongoing and historical benchmark data for global ocean assessment; however, there are critical gaps in our knowledge about the variability and pace of sustained change within and between coastal sites.
The Albemarle-Pamlico Estuarine (APE) System is the largest lagoonal estuary in the US and the second largest estuary on the US East Coast. The circulation of this shallow water system (~5 m mean depth) is driven by tidal and wind driven currents. The system is comprised of six river basins with a large, combined watershed (81,528 km2), and variability in the rate and composition of freshwater inputs (Harned and Davenport, 1990). Through temperature and light, seasonality plays an important role in environmental variability (Paerl et al., 2009), but episodic events (e.g., tropical cyclones) profoundly alter the system with impacts that can last over a year (Paerl et al., 2001). Allochthonous (watershed) and autochthonous (internal) processes are both important to the larger APE system (Hounshell et al., 2022); but near its outflows, open ocean waters also influence estuarine dynamics and environmental conditions. Adding further variability to this coastal region, the convergence of the warm South Atlantic Bight shelf and Gulf Stream waters with cooler Mid-Atlantic Bight shelf waters and additional oceanographic complexity (Seim et al., 2022; Gray et al., 2024) creates highly-localized biogeochemical (Selden et al., 2021) and diversity hotspots (Gronniger et al., 2023).
The Piver’s Island Coastal Observatory (PICO) is located at the southern end of the Pamlico Sound (North Carolina, USA) at the Beaufort Inlet and includes a broad suite of physical, chemical, and biological measurements, like open ocean time-series campaigns (e.g., HOT, BATS), to characterize microbially-mediated biogeochemistry. Because of its hydrographic complexity and temperate climate, PICO has a much wider parameter space than most coastal or open ocean time-series; however, this estuary lacks a major riverine freshwater source, and thus terrestrial influence is mediated by local runoff from tidal creeks and stormwater systems as well as influxes of groundwater, which cannot be easily quantified. Further, like the broader region, substantial impacts from reoccurring episodic disturbances such as tropical cyclones and other storms provide unique natural ‘experiments’ to explore the mechanisms structuring PICO’s chemistry and biology (Fenwick et al., 2024). Here, limited in situ instrumentation (e.g., temperature) coupled with a more extensive, weekly, discrete sampling generate an extensive dataset that helps to constrain the mechanisms of variability. In this study, we use the first decade of measurements to define the pulse and press of core biogeochemical state variables in this high- variability, temperate coastal marine system.
All samples were taken as part of the Piver’s Island Coastal Observatory (PICO) time-series, Beaufort, NC, USA at 34.7181°N 76.6707°W at a location with a mean low tide water column depth of ~4.5 m, and a mean tidal range of 0.95 m. Water was sampled weekly at 10:30 ± 30 min local time using a 5 L niskin bottle centered at 1 m with a bottle length of 0.7 m. The methodology for many variables is described by (Johnson et al., 2013) or (Ward et al., 2017). Briefly, DIC was measured in triplicate on mercuric chloride poisoned samples by acidification and subsequent quantification of released CO2 using a CO2 detector (Li-Cor 7000) (Dickson et al., 2007). pH was measured in triplicate spectrophotometrically with m-cresol purple (Clayton and Byrne, 1993) at standard temperature (25°C) and reported on the log total hydrogen ion scale (pHT,25°C). Both DIC and pH samples were collected following recommended procedures (Dickson et al., 2007) and measurements were calibrated against Certified Reference Materials purchased from Dr. A. G. Dickson of the Scripps Institution of Oceanography, University of California, San Diego. Nutrients (NO2, NO3, PO4, SiOH4) were measured in duplicate on 0.22 µm filtered samples stored at -80°C until later analysis using an Astoria-Pacific A2 autoanalyzer following the manufacturer’s recommended protocols or processed by Scripps Institute of Oceanography STS/ODF chemistry laboratory. Salinity was measured using a calibrated handheld conductivity, temperature, and depth instrument (YSI Castaway), on discrete samples using a refractometer calibrated against standards or using a Portasal 8410A salinometer. Turbidity was measured in duplicate on discrete samples using a calibrated handheld turbidimeter (Orion AQ4500). Duplicate chlorophyll pigment samples were extracted in MeOH and measured fluorometrically as previously described (Johnson et al., 2010b). Photosynthetic efficiency (Fv/Fm) was quantified with a FIRe (fluorescence induction and relaxation) fluorometer on samples kept in the dark for ≥15 min prior to assessment; data were processed with a custom MATLAB script based on (Kolber et al., 1998) and (Johnson, 2004). Heterotrophic bacteria, picocyanobacteria (non-orange fluorescing, picophytoplankton), Synechococcus (orange fluorescing picocyanobacteria) and eukaryotic phytoplankton were quantified using flow cytometry as previously described (Johnson et al., 2010a), or with an using Hoechst 34580 or Sybr Green I DNA stains using an Attune NxT with 405 nm excitation and 440 ± 25, 512 ± 13, 603 ± 24, 710 ± 25 nm emission and 488 nm excitation and 530 ± 15, 574 ± 13, 695 ± 20, 780 ± 30 nm emission. Production and respiration quantified using Winkler oxygen (Labasque et al., 2004) were measured using the light/dark bottle technique with 24 h incubations at ambient temperature in a sinusoidal incubator with ~1000 µmol quanta m-2 sec-1 peak PAR. In general, we maintained the same instrumentation throughout the time series; however, in some cases changing instrumentation was a necessity and often chosen to increase accuracy (e.g. Portasal over a refractometer to measure salinity). When measurements changed over the course of the time series we, when available, used Certified Reference Materials to determine that measurements were within expected tolerances and/or included an inter-comparison by running the same samples using both techniques.
For long term trends, data from 2011- 2021 were subsampled on a weekly frequency using nearest neighbor methods. Marine CO2 system variables, including total alkalinity, were calculated from measured pH and DIC using a MATLAB implementation of CO2SYS (Van Heuven et al., 2011). Time-series data was analyzed in MATLAB using custom scripts. Using resampled data, linear trends were calculated by minimizing the residuals in y. Power frequency was calculated using Fourier transform or the Climate Data Toolbox (Greene et al., 2019) and subsampled using a bandstop filter of 0.75-1.25× frequency peak. Time of emergence was calculated after (Turk et al., 2019). Breakpoint analyses were performed in R 4.3.2 by first segmenting the data: storm data includes all samples collected within 180 days following “wet” storms: Hurricanes Irene (2011), Florence (2018) and Dorian (2019), including daily sampling for days 6-41 and 2-20 following initial predicted impacts for hurricanes Florence and Dorian, respectively. The remainder of the data was categorized as non-storm data, even though “non-wet” storms occurred during this time frame. The non-storm data was first smoothed using a general additive model using the R mgcv 1.9-0 package with the cyclic cubic smoothing spline (Hastie, 2023). We used segmented linear regression to fit the difference between the gam prediction and observed data using the segmented 2.0-3 package (Muggeo, 2008) with the slope of the linear regression following the breakpoint set to zero (Asmala et al., 2021).
At PICO, we previously observed patterns of core biogeochemical variables across short-term (i.e., diurnal, tidal) and long-term repeatable cycles (i.e., seasonal/annual cycles) as well as responses to episodic events (Johnson et al., 2013; Gronniger et al., 2022). More than 10 years of weekly observations confirm the strong seasonal cycles across a range of variables; here, we identify how shifts in timing and long-term trends in environmental variables reveal complex anthropogenic impacts on the coastal ocean. In examining seasonal patterns, most measured environmental variables have consistent timing of their annual maxima (Figure 1, Table 1). While temperature and pH have consistently timed peaks, chlorophyll, DIC, salinity, silicate and turbidity are much more variable (Supplementary Figure 1), suggesting interannual differences in these factors. Further, compared to open ocean time series, PICO has much larger annual ranges; for example, temperature exhibits ~2×, pH ~3×, DIC ~3× and salinity ~10× the range observed at the Bermuda Atlantic Time Series (BATS), which is located at a similar latitude in the Atlantic Ocean (Bates and Johnson, 2023). These broader observed ranges are driven in part by close coupling with the terrestrial environment and enhanced biological activity, with chlorophyll concentrations an order of magnitude higher than in the open ocean (Johnson and Howd, 2000). PICO’s location at mouth of an estuary adjacent to the broad (and shallow) mid-Atlantic Bight shelf captures land-sea coupling, while retaining marine characteristics (e.g., generally high salinity >30 PSU). While enhanced ranges of physicochemical variables are common in the coastal ocean [e.g (Hofmann et al., 2011; Poppeschi et al., 2022)], here biological activity (gross productivity - ~0.5-2 mg O2 L-1 d-1) and photosynthetic biomass (e.g., chlorophyll – 2.5–5.8 µg L-1) are generally higher and less seasonal than open-ocean sites, likely due to more consistent availability of nutrients (e.g., NO3). Moreover, we predict that variation in the timing and magnitude of environmental factors will propagate to changes in the microbiome (Ward et al., 2017; Bolaños et al., 2022; Poppeschi et al., 2022).
Figure 1. Temporal patterns and trends (red lines) of temperature, salinity and nitrate at the Piver’s Island Coastal Observatory (PICO) study site based on weekly measurements. Right, histogram of observations and frequency spectra demonstrating major differences in the distribution and timing of variability among core biogeochemical variables. Upper picture: Sentinel-2 highlight optimized natural color image remote sensing image centered on PICO site from 20240827. Center picture: false color image of dissolved organic matter concentration satellite product following a major storm (after Jordon 2018/NASA). Bottom picture: false color image of chlorophyll concentration from Sentinel-2 Se2WaQ processing from 20240901.
Layered on interannual and episodic variability, we observe statistically-significant, long-term trends in several core environmental variables (Figure 2). For example, carbonate system variables pH and DIC are both decreasing (Table 1) more rapidly than in the open ocean (Dore et al., 2009; Bates and Johnson, 2023). Although anthropogenically-driven increases in atmospheric CO2 are predicted to propagate to the marine environment, here DIC is decreasing, demonstrating the dominance of local processes (e.g., primary production) in this system. Concurrently, we observe larger-than-expected declines in pH and total alkalinity (TA), which cannot be attributed to DIC, suggesting changes in runoff chemistry due to human- impacts such as accelerated weathering (Kaushal et al., 2017). These variables as well as decreasing turbidity, chlorophyll and Secchi depth are consistent with long-term, broader trends of decreased nutrient concentrations and increased water clarity in the Albemarle-Pamlico Estuary (Harned and Davenport, 1990) and in the US Southeast region (Hall et al., 2020). However, we do observe minor increases in NO3 and NH4 along with an apparent microbial shift toward cyanobacteria (Synechocccus and picocyanobacteria) and away from picoeukaryotes (Table 1), although we did not quantify the abundance of larger eukaryotic phytoplankton. Similarly, primary production and respiration rates (since 2015, via O2 production and consumption in incubations), are both decreasing (Table 1), indicating general declines in biological activity. Moreover, this system has chronic N:P <16 suggesting that it is typically N-limited, but low concentrations of nutrients coupled with high chlorophyll levels (for the marine environment) throughout the year suggests that nutrients are either rapidly recycled in the system or potentially that other factors control chlorophyll biomass. Thus overall, the environmental signals in this system indicate ecosystem complexity.
Figure 2. Seasonal Patterns and Annual trends for the PICO time series from 2011-2021. Weekly resampled ‘raw’ data and decomposed trend, annual cycles and residuals of pH (top) and chlorophyll a (bottom).
Next, we sought to quantify the time of emergence, or when global change-driven shifts in environmental variables can be discerned within background of high temporal (Hofmann et al., 2011; Zhao et al., 2022). At PICO, we can estimate this time to emergence using existing data; O2 respiration, chlorophyll and NO3 have the shortest times of emergence (15.5, 21.1 and 23.2 years, respectively), supporting their longer-term trends (Table 1). Carbonate system parameters, pH and total alkalinity (TA) also have short times of emergence; and our estimate for pH (26.3 years) is statistically indistinguishable from the 23 ± 13 years estimated from North American coastal margins oceanographic measurements (Turk et al., 2019). Further, we sought to identify potential relationships between environmental variables using correlations between environmental factors; many variables reveal strong cross-correlations, but the lag period for the maximum correlation varies substantially (Table 2). For example, the seasonal variables day length and temperature are strongly correlated (r=0.94) with a 5-week lag. However, cross-correlations among the carbonate system variables are more complicated: TA is strongly correlated with DIC (r=0.98; 0 lag), but DIC is only weakly correlated with pH (r=0.33; 19-week lag). Further, these correlations can mask more complex relationships that offer insight into system drivers: for example, at short lags, TA is negatively correlated with pH, but at zero lag TA and pH are positively correlated (Supplementary Figure 2). Summarizing this data, if the carbonate system were only driven by inorganic processes (e.g., dissolution and speciation of CO2), the strongest correlations would be expected at zero lag, but here variation in the strength (and sign) with lag period demonstrate the presence of multiple drivers. Long-term trends in pH (decreasing) and DIC (decreasing) are inconsistent with expectations (and open ocean time series observations) of decreasing pH and increasing DIC due to anthropogenically-driven atmospheric invasion of CO2. Lagged correlation plots also demonstrate an uncoupling of DIC and pH on seasonal and episodic (zero lag) scales (Supplementary Figure S2). However, strong positive correlations of TA and DIC across lags and both variables’ correlations with salinity suggests the influx of freshwater sources with low DIC and TA. Interestingly, pH is not correlated with salinity at zero lag likely due to the variability in organic acid content of source waters. Taken together, seasonal patterns and long-term trends follow expected climatological forcing (e.g., temperature, chlorophyll) but others (e.g., carbonate system) have unexpected trends or complex relationships that require further sampling or experimentation to clarify. One source of poor correlations could be discrete events such as storms where factors other than long term drivers “break” expected relationships between environmental factors.
In addition to the press disturbances of climate change and human ecosystem modification, discrete (or pulse) events have the potential to alter coastal marine ecosystems. Here, some variables display episodic peaks (e.g., NO3-; Figure 1); however, the infrequency of these events makes it difficult to predict their source. We focus on the potential for storms to alter environmental conditions, as PICO is one of three proposed US hotspots for tropical cyclones with an estimated 2-year return time for hurricanes and tropical storms (Keim et al., 2007; Patrick et al., 2022). In this system, storm events serve as a repeated disturbance with potential short to medium term alteration of environmental variables. In contrast with pioneering research into tropical cyclone impacts on the adjacent Neuse River Estuary (Paerl et al., 2001), PICO’s location at a break in the barrier island system (Beaufort Inlet) with high tidal flushing reduces the persistence of direct storm impacts and terrestrial runoff compared to the lagoonal Neuse River Estuary (e.g., days- weeks rather than weeks-years) (Paerl et al., 2001). As tropical cyclones have distinct characteristics (e.g., antecedent conditions, rainfall, windspeed and duration), here, we focus on “wet” storms (>20 cm of rain), which are most likely to alter environmental factors (Asmala et al., 2021). Practically, therefore we focused on Hurricanes Irene (2011), Florence (2018) and Dorian (2019); for the latter two storms weekly sampling was supplemented with several weeks of daily sampling to better resolve the temporal impact of these storms on environmental factors. However, this intensive sampling biases the dataset toward these later two storms. All three wet tropical cyclones occurred during the hurricane season’s peak in the mid-Atlantic (Late August- mid September) and thus represent similar environmental conditions. However, as the baseline data during this period includes tropical cyclones that were not categorized as wet storms, our calculations likely underestimate the extent and persistence of these events.
Our break-point calculations reveal the persistence of environmental factors’ responses to “wet storm” disturbance, with altered conditions persisting for silicate, DIC, and salinity for 42-55 days (Figure 3). Although the watershed immediately adjacent to PICO is small (595 km2), the estuary is hydrologically connected to the Neuse River (Albemarle-Pamlico Sound system) through a canal system and thus receives freshwater inputs from this much large watershed over extended periods during high-flow conditions (Kirby-Smith and Costlow, 1989). We posit that these longer-term alterations in environmental parameters are mediated by continued introduction of low salinity, low DIC and high nutrient riverine and groundwaters. Although storms can introduce terrestrial nutrients, among those we quantify, only silicate had a statistically significant wet-storm signal, whereas NO3 and NH4 did not significantly deviate from the baseline. Silicate is above the analytical detection limit in our system (2011-2021 average concentration 4.8 µM), whereas NO3 exhibits intense peaks but is generally low to undetectable (Figure 1). As silicate is used by a more limited number of taxa (e.g., some eukaryotic phytoplankton, protists, and sponges), this micronutrient persists in the water column and can perhaps serve as a “conservative” nutrient tracer for influxes of other nutrients which may be rapidly assimilated into microbial biomass.
Figure 3. Time scale of environmental variable responses to wet tropical cyclones. (A) Panel showing post-storm deviation of environmental variable Dissolved Organic Carbon (DIC) from predicted seasonal norm for up to 180 days following three wet tropical cyclones that impacted the study site Irene (2011), Florence (2018) and Dorian (2019). Red line tracks the deviation from seasonally-expected values, the vertical black line indicates the predicted break point (days) with the shaded region showing the standard error (B) Table summarizing break point analysis for a suite of environmental factors. Bold rows indicate statistically-significant deviations from seasonal environmental conditions. Direction indicates whether the environmental factor is higher or lower than the expected seasonal condition.
In contrast with the persistent deviations from the annual cycle for the above variables, storm-driven pH decreases were apparent for ~ 2 weeks (breakpoint 15.57 days, Figure 3), as in the long-term observations, likely due to organic compounds, which are increasingly recognized as important in controlling coastal pH (Kerr et al., 2023) (Figure 3). The only other variable with a significant storm signal was chlorophyll a (breakpoint 6 days); post-storm conditions include an initial wash out (as existing phytoplankton are diluted by freshwater fluxes or lysed due to low salinity conditions), followed by a phytoplankton bloom due to nutrient influxes, predominantly large, eukaryotic phytoplankton (Steichen et al., 2020; Gronniger et al., 2022). As temporal variability is inherently high within this system, especially for biological variables, detection of a phytoplankton bloom, albeit briefly, is somewhat surprising. A number of other variables did not exhibit significant post-storm breakpoint values (NH4, NO3, Secchi depth, turbidity, temperature), although these parameters are significant in other systems following storms (Steichen et al., 2020); however, values may differ due to the sensitivity of different metrics, distinct characteristics of ecosystems, as well as the availability of baseline data to constrain natural variability. As tropical cyclones are predicted to increase in intensity, and potentially in frequency, with global change (Knutson et al., 2021), wet storms with the potential for long-term alteration of environmental factors are likely to impact coastal ecosystems as well as the people and other organisms that depend on them.
The Piver’s Island Coastal Observatory has >1200 standardized time point observations now spanning nearly 15 years; continued observations will characterize the evolving pulse and press of this ecosystem as well as guide experimentation to test specific hypotheses, e.g., microbiome responses to increases in temperature and acidity (Wang et al., 2021). Several core variables already demonstrate statistically significant long-term trends and episodic-event-driven responses (e.g., pH, chlorophyll), but other core global change variables such as temperature have not yet emerged from the background environmental variability (Figure 1). Extended duration and/or higher frequency sampling through expanded in situ sensors or automated samplers may help to constrain both long term trends as well as shorter term ecological variability and disturbance response. For example, extended duration (1995-present) hourly in situ temperature measurements from this site show a significant slope (0.035 ± 0.002°C/y, p<0.01) that exceeds global mean increases (~0.007) but will need to be continued for another decade to break through the predicted time of emergence (Table 1). These approaches may also help to better constrain the persistence of storms impacts on biogeochemical (or ecological) variables as well as the mechanisms behind their differential recovery times. This study utilizes comprehensive weekly sampling at a single location, but complementary studies in the larger estuarine system that sample (1) high frequency observation of select variables at a single location (Paerl et al., 2009), (2) estuarine spatial variability at coarse temporal resolution (Asmala et al., 2021) or (3) offshore gradients at even coarser temporal resolution (Wang et al., 2019) all provide their own unique insights into environmental trends and their drivers. Coordinated sampling, especially following different kinds of disturbance (heat waves, winter storms, etc.) as well as synthesis across multiple research platforms could help to resolve the timing and spatial extent of the pulse and press of this system (Larkin et al., 2020; Patrick et al., 2022). Understanding how seasonal and episodic variability as well as longer term trends, many of which are in flux, impact the composition and function of this ecosystem is critically important to understand the biogeochemistry and ecology, impacts on fisheries, recreation, commerce or other human uses, and broader habitat of this large coastal ecosystem.
The datasets presented in this study can be found in online repositories. The names of the repository/repositories and accession number(s) can be found below: Data Deposition: Data are deposited in the BCO-DMO database: https://www.bco-dmo.org/project/2281 https://www.bco-dmo.org/project/941303 https://www.bco-dmo.org/project/941318.
ZJ: Conceptualization, Data curation, Formal Analysis, Investigation, Visualization, Writing – original draft, Funding acquisition, Project administration, Supervision, Writing – review & editing. DH: Data curation, Formal Analysis, Funding acquisition, Investigation, Visualization, Writing – original draft, Conceptualization, Project administration, Supervision, Writing – review & editing.
Wang, Guangyi; Tianjin University, Blinebry, Sara; Duke University Marine Lab, Xie, Ningdong; Duke University Marine Lab, Wheeler, Jay; Duke University Marine Lab, Ward, Christopher S.; Duke University Marine Lab, Yung, Cheuk-Man; Duke University Marine Lab, Gu, Junyao; Duke University Marine Lab, Juarez, Doris L.; Duke University Marine Lab, Duan, Yingbo; Tianjin University, Loftus, Sarah E.; Duke University Marine Lab, Gronniger, Jessica L.; Duke University Marine Lab, Davis, Katherine M.; Duke University Marine Lab, Lanasa, Mikayla; Duke University Marine Lab, Li, Jiaqian; Duke University Marine Lab, Wang, Zhao; Duke University Marine Lab, Givens, Laura; Duke University Marine Lab, Carlson, Christina; Duke University Marine Lab, Lin, Yajuan; Duke University Marine Lab, Swink, Courtney; Duke University Marine Lab, Keister, Elise; Duke University Marine Lab, Bittar, Thais, Duke University Marine Lab, Williams, Tiffany, Duke University Marine Lab, Dai, Qing; Duke University Marine Lab, Foley, Katherine; Duke University Marine Lab, Benavidas, James; Duke University Marine Lab, Izzo, Lisa; Duke University Marine Lab, Suzuki, Maya; Duke University Marine Lab, Xu, Youyi; Duke University Marine Lab, Estrada- Graf, Adrian; Duke University Marine Lab, Larkin, Alyse; Duke University Marine Lab, O’Brian, Bryce; Duke University Marine Lab, Lawrence, Alexandra; Duke University Marine Lab, Pruchniewski, Morgan; Duke University Marine Lab, Roberts, Sarah; Duke University Marine Lab.
The author(s) declare that financial support was received for the research and/or publication of this article. Funding support was provide by the US National Science Foundation awards 1416665 (to DH and ZJ), 2033934 to DH, and 2224819 to DH. Additional support was provided by the US-Australia Fulbright Program to DEH.
The authors declare that the research was conducted in the absence of any commercial or financial relationships that could be construed as a potential conflict of interest.
The author(s) declare that no Generative AI was used in the creation of this manuscript.
All claims expressed in this article are solely those of the authors and do not necessarily represent those of their affiliated organizations, or those of the publisher, the editors and the reviewers. Any product that may be evaluated in this article, or claim that may be made by its manufacturer, is not guaranteed or endorsed by the publisher.
The Supplementary Material for this article can be found online at: https://www.frontiersin.org/articles/10.3389/fmars.2025.1505754/full#supplementary-material
Supplementary Figure 1 | Raw, series trend, annual variability and residual (raw-[trend + annual variability]) for each biogeochemical variable. Y-axis units are described in Table 2.
Supplementary Figure 2 | Weekly lagged cross-correlation for each combination of biogeochemical variables. Blue horizontal lines are 95% confidence bounds.
Asmala E., Osburn C. L., Paerl R. W., Paerl H. W. (2021). Elevated organic carbon pulses persist in estuarine environment after major storm events. Limnol. Oceanogr. Lett. 6, 43–50. doi: 10.1002/lol2.10169
Bates N. R., Johnson R. J. (2023). Forty years of ocean acidification observations, (1983–2023) in the Sargasso Sea at the Bermuda Atlantic Time-series Study site. Front. Mar. Sci. 10. doi: 10.3389/fmars.2023.1289931
Bates N. R., Knap A. H., Michaels A. F. (1998). Contribution of hurricanes to local and global estimates of air-sea exchange of CO2. Nature 395, 58–61. doi: 10.1038/25703
Bolaños L. M., Tait K., Somerfield P. J., Parsons R. J., Giovannoni S. J., Smyth T., et al. (2022). Influence of short and long term processes on SAR11 communities in open ocean and coastal systems. ISME. Commun. 2, 116. doi: 10.1038/s43705-022-00198-1
Brown M. V., Van De Kamp J., Ostrowski M., Seymour J. R., Ingleton T., Messer L. F., et al. (2018). Systematic, continental scale temporal monitoring of marine pelagic microbiota by the Australian Marine Microbial Biodiversity Initiative. Sci. Data 5, 180130. doi: 10.1038/sdata.2018.130
Caron D. A., Connell P. E., Schaffner R. A., Schnetzer A., Fuhrman J. A., Countway P. D., et al. (2017). Planktonic food web structure at a coastal time-series site: I. Partitioning of microbial abundances and carbon biomass. Deep. Sea. Res. Part I.: Oceanogr. Res. Papers. 121, 14–29. doi: 10.1016/j.dsr.2016.12.013
Clayton T. D., Byrne R. H. (1993). Spectrophotometric seawater pH measurements: total hydrogen ion concentration scale calibration of m-cresol purple and at-sea results. Deep. Sea. Res. Part I.: Oceanogr. Res. Papers. 40, 2115–2129.
Dickson A. G., Sabine C. L., Christian J. R. (2007). Guide to best practices for ocean CO2 measurements (PICES special publication 3). Dickson: North Pacific Marine Science Organization.
Dore J. E., Lukas R., Sadler D. W., Church M. J., Karl D. M. (2009). Physical and biogeochemical modulation of ocean acidification in the central North Pacific. Proc. Natl. Acad. Sci. 106, 12235–12240. doi: 10.1073/pnas.0906044106
Dore J. E., Lukas R., Sadler D. W., Karl D. M. (2003). Climate-driven changes to the atmospheric CO2 sink in the subtropical North Pacific Ocean. Nature 424, 754–757. doi: 10.1038/nature01885
Fenwick I. F., Heim K. C., Pershing A. J., Mills K. E., Lucey S. M., Nye J. A. (2024). Evaluating the influence of press and pulse disturbances on community dynamics of the Northeast US Large Marine Ecosystem. Mar. Ecol. Prog. Ser. 744, 1–16. doi: 10.3354/meps14663
Gray P. C., Savelyev I., Cassar N., Lévy M., Boss E., Lehahn Y., et al. (2024). Evidence for kilometer-scale biophysical features at the gulf stream front. J. Geophys. Res.: Oceans. 129, e2023JC020526. doi: 10.1029/2023JC020526
Greene C. A., Thirumalai K., Kearney K. A., Delgado J. M., Schwanghart W., Wolfenbarger N. S., et al. (2019). The climate data toolbox for MATLAB. Geochem. Geophys. Geosyst. 20, 3774–3781. doi: 10.1029/2019GC008392
Gronniger J. L., Gray P. C., Niebergall A. K., Johnson Z. I., Hunt D. E. (2023). A Gulf Stream frontal eddy harbors a distinct microbiome compared to adjacent waters. PloS One 18, e0293334. doi: 10.1371/journal.pone.0293334
Gronniger J. L., Wang Z., Brandt G. R., Ward C. S., Tsementzi D., Mu H., et al. (2022). Rapid changes in coastal ocean microbiomes uncoupled with shifts in environmental variables. Environ. Microbiol. 24, 4167–4177. doi: 10.1111/1462-2920.16086
Hall E. R., Wickes L., Burnett L. E., Scott G. I., Hernandez D., Yates K. K., et al. (2020). Acidification in the U.S. Southeast: causes, potential consequences and the role of the southeast ocean and coastal acidification network. Front. Mar. Sci. 7. doi: 10.3389/fmars.2020.00548
Harned D., Davenport M. (1990). “Water-quality trends and basin activities and characteristics for the Albemarle-Pamlico estuarine system, North Carolina and Virginia,” in Open-File Report (US Geological Survey).
Hofmann G. E., Smith J. E., Johnson K. S., Send U., Levin L. A., Micheli F., et al. (2011). High-frequency dynamics of ocean pH: A multi-ecosystem comparison. PloS One 6, e28983. doi: 10.1371/journal.pone.0028983
Hounshell A. G., Fegley S. R., Hall N. S., Osburn C. L., Paerl H. W. (2022). Riverine discharge and phytoplankton biomass control dissolved and particulate organic matter dynamics over spatial and temporal scales in the neuse river estuary, North Carolina. Estuaries. Coasts. 45, 96–113. doi: 10.1007/s12237-021-00955-w
Hunter-Cevera K. R., Neubert M. G., Olson R. J., Solow A. R., Shalapyonok A., Sosik H. M. (2016). Physiological and ecological drivers of early spring blooms of a coastal phytoplankter. Science 354, 326–329. doi: 10.1126/science.aaf8536
Johnson Z. I. (2004). Development and application of the background irradiance gradient - single turnover fluorometer (BIG-STf). Mar. Ecol. Prog. Ser. 283, 73–80. doi: 10.3354/meps283073
Johnson Z., Howd P. (2000). Marine photosynthetic performance forcing and periodicity for the Bermuda Atlantic Time Series 1989-1995. Deep-Sea. Res. I. 47, 1485–1512. doi: 10.1016/S0967-0637(99)00111-9
Johnson Z. I., Shyam R., Ritchie A. E., Lin Y., Mioni C., Lance V. P., et al. (2010a). The effects of iron- and light-limitation on phytoplankton communities of deep chlorophyll maxima of the Western Pacific Ocean. J. Mar. Res. 68, 1–26. doi: 10.1357/002224010793721433
Johnson Z. I., Shyam R., Ritchie A. E., Mioni C., Lance V. P., Murray J. W., et al. (2010b). The effect of iron-and light-limitation on phytoplankton communities of deep chlorophyll maxima of the western Pacific Ocean. J. Mar. Res. 68, 283–308. doi: 10.1357/002224010793721433
Johnson Z. I., Wheeler B. J., Blinebry S. K., Carlson C. M., Ward C. S., Hunt D. E. (2013). Dramatic variability of the carbonate system at a temperate coastal ocean site (Beaufort, North Carolina, USA) is regulated by physical and biogeochemical processes on multiple timescales. PloS One 8, e85117. doi: 10.1371/journal.pone.0085117
Kaushal S. S., Duan S., Doody T. R., Haq S., Smith R. M., Newcomer Johnson T. A., et al. (2017). Human-accelerated weathering increases salinization, major ions, and alkalinization in fresh water across land use. Appl. Geochem. 83, 121–135. doi: 10.1016/j.apgeochem.2017.02.006
Keim B. D., Muller R. A., Stone G. W. (2007). Spatiotemporal patterns and return periods of tropical storm and hurricane strikes from Texas to Maine. J. Climate 20, 3498–3509. doi: 10.1175/JCLI4187.1
Keller K. M., Joos F., Raible C. C. (2014). Time of emergence of trends in ocean biogeochemistry. Biogeosciences 11, 3647–3659. doi: 10.5194/bg-11-3647-2014
Kerr D. E., Turner C., Grey A., Keogh J., Brown P. J., Kelleher B. P. (2023). OrgAlkCalc: Estimation of organic alkalinity quantities and acid-base properties with proof of concept in Dublin Bay. Mar. Chem. 251, 104234. doi: 10.1016/j.marchem.2023.104234
Kirby-Smith W. W., Costlow J. D. (1989). “The Newport River estuarine system,” in Sea Grant publication; UNC-SG-WP-89-04 (University of North Carolina Sea Grant Program, Raleigh, NC).
Knutson T. R., Chung M. V., Vecchi G., Sun J., Hsieh T.-L., Smith A. J. P (2021). “Science Brief Review: Climate change is probably increasing the intensity of tropical cyclones,” in Critical Issues in Climate Change Science. Eds. Quéré C., Liss P., Forster P.
Kolber Z. S., Prasil O., Falkowski P. G. (1998). Measurements of variable chlorophyll fluorescence using fast repetition rate techniques - defining methodology and experimental protocols. Biochim. Biophys. Acta 1367, 88–106. doi: 10.1016/S0005-2728(98)00135-2
Labasque T., Chaumery C., Aminot A., Kergoat G. (2004). Spectrophotometric Winkler determination of dissolved oxygen: re-examination of critical factors and reliability. Mar. Chem. 88, 53–60. doi: 10.1016/j.marchem.2004.03.004
Larkin A. A., Moreno A. R., Fagan A. J., Fowlds A., Ruiz A., Martiny A. C. (2020). Persistent El Niño driven shifts in marine cyanobacteria populations. PloS One 15, e0238405. doi: 10.1371/journal.pone.0238405
Muggeo V. (2008). Segmented: an R package to fit regression models with broken-line relationships. R. News 8, 20–25.
Muller-Karger F. E., Miloslavich P., Bax N. J., Simmons S., Costello M. J., Sousa Pinto I., et al. (2018). Advancing marine biological observations and data requirements of the complementary essential ocean variables (EOVs) and essential biodiversity variables (EBVs) frameworks. Front. Mar. Sci. 5. doi: 10.3389/fmars.2018.00211
Paerl H. W., Bales J. D., Ausley L. W., Buzzelli C. P., Crowder L. B., Eby L. A., et al. (2001). Ecosystem impacts of three sequential hurricanes (Dennis, Floyd, and Irene) on the United States’ largest lagoonal estuary, Pamlico Sound, NC. Proc. Natl. Acad. Sci. 98, 5655–5660. doi: 10.1073/pnas.101097398
Paerl H. W., Rossignol K. L., Guajardo R., Hall N. S., Joyner A. R., Peierls B. L., et al. (2009). FerryMon: Ferry-based monitoring and assessment of human and climatically driven environmental change in the Albemarle-Pamlico sound system. Environ. Sci. Technol. 43, 7609–7613. doi: 10.1021/es900558f
Patrick C. J., Kominoski J. S., Mcdowell W. H., Branoff B., Lagomasino D., Leon M., et al. (2022). A general pattern of trade-offs between ecosystem resistance and resilience to tropical cyclones. Sci. Adv. 8, eabl9155. doi: 10.1126/sciadv.abl9155
Poppeschi C., Charria G., Daniel A., Verney R., Rimmelin-Maury P., Retho M., et al. (2022). Interannual variability of the initiation of the phytoplankton growing period in two French coastal ecosystems. Biogeosciences 19, 5667–5687. doi: 10.5194/bg-19-5667-2022
Seim H. E., Savidge D., Andres M., Bane J., Edwards C., Gawarkiewicz G., et al. (2022). Overview of the processes driving exchange at cape hatteras program. Oceanography 35, 6–17. doi: 10.5670/oceanog.2022.205
Selden C. R., Chappell P. D., Clayton S., Macías-Tapia A., Bernhardt P. W., Mulholland M. R. (2021). A coastal N2 fixation hotspot at the Cape Hatteras front: Elucidating spatial heterogeneity in diazotroph activity via supervised machine learning. Limnol. Oceanogr. 66, 1832–1849. doi: 10.1002/lno.11727
Steichen J. L., Labonté J. M., Windham R., Hala D., Kaiser K., Setta S., et al. (2020). Microbial, physical, and chemical changes in galveston bay following an extreme flooding event, hurricane harvey. Front. Mar. Sci. 7. doi: 10.3389/fmars.2020.00186
Steinberg D. K., Carlson C. A., Bates N. R., Johnson R. J., Michaels A. F., Knap A. H. (2001). Overview of the US JGOFS Bermuda Atlantic Time-series Study (BATS): a decade-scale look at ocean biology and biogeochemistry. Deep. Sea. Res. Part II.: Topical. Stud. Oceanogr. 48, 1405–1447. doi: 10.1016/S0967-0645(00)00148-X
Tilbrook B., Jewett E. B., Degrandpre M. D., Hernandez-Ayon J. M., Feely R. A., Gledhill D. K., et al. (2019). An enhanced ocean acidification observing network: from people to technology to data synthesis and information exchange. Front. Mar. Sci. 6. doi: 10.3389/fmars.2019.00337
Turk D., Wang H., Hu X., Gledhill D. K., Wang Z. A., Jiang L., et al. (2019). Time of emergence of surface ocean carbon dioxide trends in the north american coastal margins in support of ocean acidification observing system design. Front. Mar. Sci. 6. doi: 10.3389/fmars.2019.00091
Van Heuven S., Pierrot D., Rae J. W. B., Lewis E., Wallace D. W. R. (2011). MATLAB Program Developed for CO2 System Calculations (Oak Ridge, Tennessee: Carbon Dioxide Information Analysis Center, Oak Ridge National Laboratory, U.S. Department of Energy).
Wang Z., Juarez D. L., Pan J.-F., Blinebry S. K., Gronniger J., Clark J. S., et al. (2019). Microbial communities across nearshore to offshore coastal transects are primarily shaped by distance and temperature. Environ. Microbiol. 21, 3862–3872. doi: 10.1111/1462-2920.14734
Wang Z., Tsementzi D., Williams T., Juarez D., Garcia N., Johnson Z., et al. (2021). Environmental stability impacts the differential sensitivity of marine microbiomes to increases in temperature and acidity. ISME. J. 15, 19–28. doi: 10.1038/s41396-020-00748-2
Ward C. S., Yung C.-M., Davis K. M., Blinebry S. K., Williams T. C., Johnson Z. I., et al. (2017). Annual community patterns are driven by seasonal switching between closely-related marine bacteria. ISME. J. 11, 1412–1422. doi: 10.1038/ismej.2017.4
Keywords: time series, microbiome, global change, coastal ocean, climate change, carbonate system
Citation: Johnson ZI, Hunt DE and the PICO Consortium (2025) The Piver’s Island Coastal Observatory – a decade of weekly+ observations reveal the press and pulse of a changing temperate coastal marine system. Front. Mar. Sci. 12:1505754. doi: 10.3389/fmars.2025.1505754
Received: 03 October 2024; Accepted: 26 February 2025;
Published: 03 April 2025.
Edited by:
Song Feng, Pacific Northwest National Laboratory (DOE), United StatesReviewed by:
Martin Johnson, Ecodiversity Ltd, IrelandCopyright © 2025 Johnson, Hunt and the PICO Consortium. This is an open-access article distributed under the terms of the Creative Commons Attribution License (CC BY). The use, distribution or reproduction in other forums is permitted, provided the original author(s) and the copyright owner(s) are credited and that the original publication in this journal is cited, in accordance with accepted academic practice. No use, distribution or reproduction is permitted which does not comply with these terms.
*Correspondence: Zackary I. Johnson, emlqQGR1a2UuZWR1; Dana E. Hunt, ZGFuYS5odW50QGR1a2UuZWR1
Disclaimer: All claims expressed in this article are solely those of the authors and do not necessarily represent those of their affiliated organizations, or those of the publisher, the editors and the reviewers. Any product that may be evaluated in this article or claim that may be made by its manufacturer is not guaranteed or endorsed by the publisher.
Research integrity at Frontiers
Learn more about the work of our research integrity team to safeguard the quality of each article we publish.