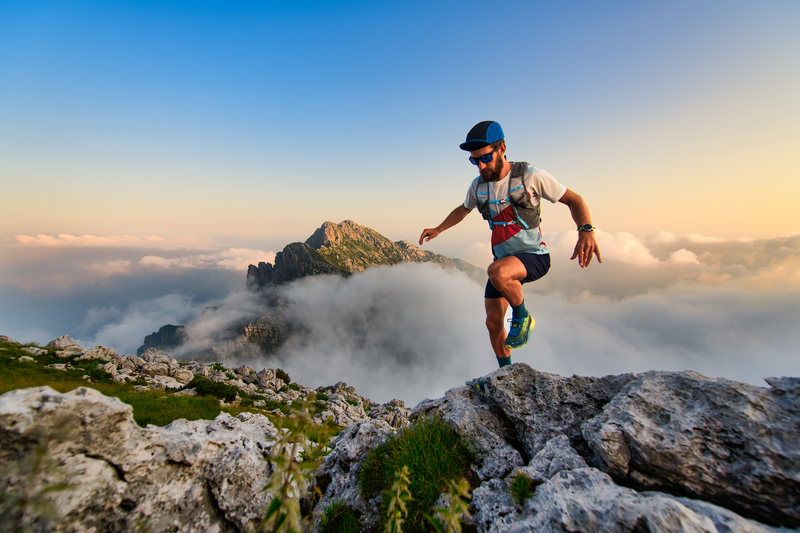
94% of researchers rate our articles as excellent or good
Learn more about the work of our research integrity team to safeguard the quality of each article we publish.
Find out more
ORIGINAL RESEARCH article
Front. Mar. Sci. , 10 February 2025
Sec. Marine Biogeochemistry
Volume 12 - 2025 | https://doi.org/10.3389/fmars.2025.1456825
Marine nitrogen cycle ultimately depends on the biological responses of oceanic microbial communities. It indirectly regulates primary production and influences the strength of the biological pump, which contributes to the oceanic uptake of atmospheric carbon dioxide (CO2). As the microbial community structure and functional capacities remain underestimated in terms of temporal and geographical coverage in the Baltic Sea, our understanding of the nitrogen cycle with respect to ecosystem functioning and climate change is limited. Therefore, in this study, we investigated the seasonal and spatial structure of microbial community abundance involved in the nitrogen loss (denitrification, anammox), reduction processes (dissimilatory nitrate reduction (DNR), dissimilatory nitrite reduction to ammonium (DNRA), and oxidation process (nitrification) in the Baltic Proper (Bornholm Deep, Gdańsk Deep, and Gotland Deep). Specifically, we focused on waters below the halocline at depths ranging from 75 to 135 m, characterized by changeable oxygen conditions. The potential of selected nitrogen processes was resolved by mapping raw reads against nitrogen cycle genes identified in de novo assembled metagenomes. Taxonomic analysis of bacterial and archaeal communities, based on paired-end raw reads, revealed that nitrification, DNR, and denitrification potential were primarily associated with the Nitrosopumilaceae and Thioglobaceae families within these phyla. Ammonia oxidation products likely fueled the production of nitrous oxide (N2O), with nitric oxide reductase (NOR)—an enzyme encoded by the Thioglobaceae genome—being responsible for further reduction. Anammox-related genes were not present within sites, thus denitrification pathway enzymes, namely, NOR and N2O reductase (NOS) were responsible for nitrogen loss. At all sites, genes encoding nitrogen reduction enzymes were most abundant, while the presence of NOS encoding genes was found in Bacteroidetes and Proteobacteria phyla within all sites. Our findings revealed no significant spatial variation, suggesting that the studied ecosystem exhibits a consistent nitrogen processing capacity across different locations. However, seasonality emerged as a key factor, as changes in nutrient and oxygen conditions throughout the year significantly influence microbial activity and the associated nitrogen-cycling processes.
Nitrogen (N) is an essential element for all living organisms and plays a critical role in the functioning of marine ecosystems. The largest nitrogen reservoir is the atmosphere; however, it is biologically available primarily to a limited yet diverse group of nitrogen-fixing bacteria and archaea. Other microorganisms must utilize bioavailable forms of nitrogen, such as ammonium and nitrate. The availability of these forms in the oceans regulates primary production and influences the strength of the biological pump, which contributes to the oceanic uptake of atmospheric carbon dioxide (CO2) (Galloway et al., 2008; Gruber and Galloway, 2008; Pajares and Ramos, 2019). The nitrogen biogeochemical cycle has been greatly disturbed by human activities (Fowler et al., 2013). The global supply of dissolved inorganic nitrogen (DIN) doubled during the last half of the twentieth century (Beusen et al., 2016). Anthropogenic inputs of nitrogen, estimated at ~160 Tg N year-¹, now nearly match the total natural nitrogen fixation in the ocean (~250 Tg N year-¹) and are expected to rise with global population growth, depending on societal efforts to limit nitrogen fluxes (Gruber and Galloway, 2008). This excess nitrogen has contributed to the degradation of many marine ecosystems by promoting harmful algal blooms, eutrophication, and the development of hypoxic and anoxic zones (Devlin and Brodie, 2023). Projections suggest that by 2050, human production of DIN could be approximately twice as high as it was in the 1990s (Malone and Newton, 2020). Currently, the global nitrogen balance appears to be shifting toward higher nitrogen losses (estimated at ~275–481 Tg N year-¹) compared to the combined natural and anthropogenic inputs (~265–294 Tg N year-¹) (Voss et al., 2013).
The primary natural source of nitrogen in the ocean is N2 fixation, while the major removal pathways are denitrification and anammox (Gruber and Galloway, 2008). Denitrification can be categorized into heterotrophic and autotrophic processes, with the Baltic Sea being particularly noted for its autotrophic denitrification, where bacteria utilize reduced inorganic compounds instead of organic carbon as electron donors (Burgin and Hamilton, 2007; Bonaglia et al., 2016; 2017; Kuliński et al., 2021). Several other nitrogen transformations play significant roles in the nitrogen cycle in the Baltic Sea, including ammonification, nitrification, dissimilatory nitrate reduction (DNR), dissimilatory nitrite reduction to ammonia (DNRA), complete ammonia oxidation to nitrate (comammox), and nitrate-/nitrite-dependent anaerobic methane oxidation (N-Damo).
The Baltic Sea exhibits unique and complex environmental conditions, creating natural gradients and variability that influence nitrogen transformations (Kuliński et al., 2021). A defining feature of the Baltic Proper is the presence of a permanent halocline, which restricts vertical mixing (Liblik et al., 2018). As a result, the deep waters are characterized by permanent anoxia, hypoxia, or fluctuating oxygen levels, shifting from oxic to hypoxic/anoxic states depending on the frequency and magnitude of inflows of oxygenated saline water from the North Sea (Liblik et al., 2018). In this context, hypoxia in the Baltic Sea is defined as oxygen concentrations below 90 µmol L-¹, while anoxia refers to oxygen levels below 2.4 µmol L-¹ (Liblik et al., 2018). Investigating these deep waters provides crucial insights into the nitrogen cycle and its variability under different oxygen gradients. This is especially relevant given the global decline in dissolved oxygen and the expansion of oxygen-deficient zones in the oceans, which have wide-ranging implications for biogeochemical cycles and aerobic organisms (Stramma et al., 2010). Several recent studies have explored microbial community composition and nitrogen cycle processes, primarily focusing on sediments (Bonaglia et al., 2017; Reyes et al., 2017; Zhang et al., 2022), with limited attention to water column studies (Mazur-Marzec et al., 2024). Nevertheless, water column denitrification has been identified below the halocline in the deep Baltic Sea—mostly Gotland Deep (Kuliński et al., 2021). Additionally, a close coupling between nitrification and denitrification, driven by Archaea (Thaumarchaeota, particularly Nitrosopumilus maritimus, Kraft et al., 2022), has been observed (Kuliński et al., 2021). In contrast, anammox appeared to play a less significant role in the redoxcline, likely due to the absence of an extended anoxic and sulfide-free zone, which is typical in habitats for anammox bacteria, such as the Black Sea (Hietanen et al., 2012; Kuliński et al., 2021). As the microbial community structure and functional capacities remain underestimated in terms of temporal and geographical coverage in the Baltic Sea, our understanding of the nitrogen cycle for ecosystem functioning and climate change is limited. Therefore, enhancing our understanding of the regulatory factors and key microbial communities involved in the nitrogen cycle is essential. This knowledge is crucial for the understanding and interpretation of how microbial communities might shift in their activity in a changing ocean like the Baltic Sea.
To address this knowledge gap, we investigated the seasonal and spatial structure of microbial communities and gene abundance in deep water layers under variable oxygen conditions in the Baltic Proper, specifically in Bornholm Deep (IDEAL), Gdańsk Deep (P1) and Gotland Deep (BY15). We focused on nitrogen loss processes (denitrification and anammox), reduction processes [dissimilatory nitrate reduction (DNR), dissimilatory nitrite reduction to ammonium (DNRA)], and oxidation (nitrification). The objective of this study was to elucidate the distribution patterns of nitrogen-related microbial communities in the deep waters of the Baltic Proper, characterized by fluctuating oxygen conditions, and to assess the spatial and temporal dynamics of genes involved in nitrogen related processes.
The Baltic Sea is a semi-enclosed shelf sea located in northern Europe and is connected to the North Sea (and thus to the North Atlantic Ocean) through the narrow and shallow Danish Straits (Figure 1). The residence time of the Baltic Sea water is approximately 35 years (BACC II, Team A, 2015). Sporadic inflows of saline waters (Major Baltic Sea Inflow—MBI) from the North Sea and large riverine runoff make the Baltic Sea one of the largest brackish water bodies on Earth that has stratified water column. The depth of the halocline at Bornholm Deep, Gdańsk Deep, and Gotland Deep normally fluctuates between 60 and 80 m (e.g., Almroth-Rosell et al., 2021).
Figure 1. Map of the study sites located in Bornholm Deep (IDEAL), Gdańsk Deep (P1), and Gotland Deep (BY15).
Consequently, the Baltic Sea suffers from permanent or periodic hypoxia and/or anoxia caused by water column stratification (Schinke and Matthäus, 1998). During the stagnation periods, the Baltic Proper bottom water is hypoxic and can be anoxic, sometimes reaching sulfidic conditions. The characterized specific structure of the Baltic Sea makes it highly vulnerable to nutrient loads via river fluxes, atmospheric deposition, and point sources. Deep waters of Bornholm Deep and Gdańsk Deep can be characterized as hypoxic or oxygenated, while deep waters of Gotland Deep are predominantly anoxic (Liblik et al., 2018; Rak et al., 2020). In the Bornholm Deep, the long-term average bottom oxygen concentration is 38 µmol L-¹, indicating predominantly hypoxic conditions. Euxinic conditions with hydrogen sulfide (H2S) were recorded from 1980 to 2010; however, these conditions have not reappeared in the last decade (Kuliński et al., 2021). Similar trends have been observed in the Gdańsk Deep, while hydrogen sulfide is present in the Gotland Deep, depending on the sampling time, starting from a depth of 80 m (Kuliński et al., 2021). Additionally, the deep basins of the central Baltic Sea exhibit significant methane enrichments in stagnant anoxic water bodies (Schmale et al., 2010); however, in this study, methane was not measured.
Bornholm Deep (station IDEAL: 55°26.623′ N, 15°18.009′ E), Gdańsk Deep (station P1: 54°50.079′ N, 19°19.082′ E) and Gotland Deep (station BY15: 57°20.062′ N, 20°03.022′ E) (Figure 1) were sampled with r/v OCEANIA in 09_20, 04_21, 09_21, and 04_22. In addition, stations IDEAL and P1 were investigated in 01_22; however, station BY15 was skipped due to severe weather conditions. Niskin rosette system (Seabird SBE 32) equipped with CTD Seabird 9/11+ with electrical resistivity (converted to salinity), mechanical and acoustic sediment floor sensors were used to measure temperature (T), salinity (S), and dissolved oxygen (O2). The CTD system was placed for approximately 1–5 min in deep waters to allow the O2 signal to stabilize, and then, it was gently lifted towards the sampling depth (Table 1). Sampling depths oscillated from 75 to 135 m depending on the study site and oxygen conditions. Samples for chemical and DNA analyses and incubation experiments were collected below the halocline from different layers at each site depending on the CTD profile (Table 1).
Table 1. Physicochemical characterization of investigated water column layers in Bornholm Deep (IDEAL), Gdańsk Deep (P1), and Gotland Deep (BY15).
A volume of 10 ml of seawater for the nitrate (NO3−), nitrite (NO2−), ammonium (NH4+), and phosphate (PO43) was filtered using 0.45 µm cellulose acetate (Ca-45/25) 25-mm filters into polyethylene bottles and stored in −20°C for further analysis. A volume of 20 ml of seawater for DOC analysis was filtered through pre-combusted 0.45 μm MN GF-5 filters and transferred into the pre-combusted glass bottle and acidified to pH ~2 with HClconc.
In this study, we followed the bacterial sampling protocol “BACT-DNA: FILTRATION FOR DNA SEQUENCING AND METAGENOMICS” developed within the TARA OCEAN consortium by Silvia Gonzales-Acinas and Isabel Ferrera (Karsenti et al., 2011). During each sampling campaign, at all stations (IDEAL, P1, and BY15), approximately 30 L of seawater was collected from Niskin bottles and transferred into three sterile 10-L carboys, each wrapped in black tape to limit light exposure. Before use, the carboys were thoroughly washed with detergent and Milli-Q (MQ) water, then sterilized with ethanol and rinsed with MQ water.
The seawater samples were kept in a chilled water bath and filtered onsite immediately after collection. The filtration process used two 142-mm diameter filters: an initial 2-µm glass fiber filter (Millipore), followed by a target 0.2-µm hydrophilic polycarbonate membrane filter (Millipore) for nucleic acid extraction. Both filter holders were positioned inside a laminar flow cabinet equipped with a HEPA H14 filter (Cruma 670FL) to maintain sterility. The filtration pressure was controlled by a manometer placed between the two filter holders and kept below 1.5 bar. Once the filtration of the first three replicates was nearing completion, the rosette with Niskin bottles was deployed again to collect the next 30 L of seawater.
During the 9_20 sampling campaign, one 2-µm glass fiber filter and two 0.2-µm polycarbonate membrane filters were used for each site. In subsequent campaigns, only one 2-µm glass fiber filter and one 0.2-µm membrane filter were used per site. In the 9_20 campaign, the extracts from the two 0.2-µm filters were combined for analysis, while in later campaigns, the extract was obtained from a single 0.2-µm filter. Each 0.2-µm filter, and the corresponding extract, contained DNA material from approximately three to six replicates, depending on the total volume of seawater collected. This approach is consistent with a recent study comparing marine microbiome sampling protocols (Pascoal et al., 2023).
During the 9_20 campaign, a total of 60.1 L of seawater was filtered at the IDEAL station, 86.4 L at P1, and 67.3 L at BY15. For all subsequent campaigns, 100.0 L of seawater was filtered at each station. After filtration, the DNA-containing filters were transferred to sterile Falcon tubes and immediately stored at −80°C. Additionally, a blank sample was collected by filtering 100 L of Milli-Q water instead of seawater, following the same procedures for filtration and preservation.
Nutrient concentrations were determined using a SEAL AA500 Auto Analyzer (Seal Analytical) applying standard photometric methods. Quality control consisted of repeated measurements of two different CRMs (QC3179, Sigma Aldrich, and HAMIL-20.2, Environment Canada). Method detection limits were determined as 0.03 µmol L−1 for NO3−, 0.009 µmol L−1 for NO2−, 0.27 µmol L−1 for NH4+, and 0.1 µmol L−1 for PO43−. The accuracy of NO3−, NO2−, NH4+, and PO43− measurements was 98.6%, 98.8%, 98.7%, and 99.0%, respectively, while the precision (relative standard deviations/coefficient of variation) was 0.7%, 1.1%, 0.6%, and 0.5%, respectively. The dissolved organic carbon (DOC) analyses were done in a TOC-L analyzer (Shimadzu) using a high-temperature (680°C) oxidation method with Pt catalyst. The method was calibrated with certified reference materials provided by D. Hansell Laboratory (University of Miami, USA).
Total DNA was extracted from the whole filters using DNeasy® PowerWater® Kit (Qiagen) according to the manufacturer’s instructions, except for modification in a bead-beating step; instead of bead tubes provided in the kit, we used oak ridge 50-mL centrifuge tubes (Thermo Fisher Scientific, Waltham, MA, USA) to encompass filter diameter. To summarize, the bead beating step was performed using larger tubes (50 mL Oak Ridge tubes) instead of the kit-provided tubes, in order to accommodate the filter’s diameter. The beads supplied with the kit, consisting of two sizes (0.1 mm garnet and 0.7 mm garnet), were used. Garnet refers to a sand-like matrix that is irregular in both shape and size.
Extraction was performed under Labgard ES Energy Saver Class II, Type A2 Laminar Flow Biological Safety Cabinet NU-440-400E with a HEPA filter/HEPEX™ Plenum Pressure Monitoring system (NuAire) to avoid cross-contamination of the samples. The quantity and quality of the isolated DNA were checked spectrophotometrically using an Epoch Microplate Spectrophotometer (Agilent), measuring A260/A280 ratios and by agarose gel electrophoresis. After isolation, DNA samples were stored frozen at −20°C until further use. Before the library construction, DNA samples were also quality checked at sequencing facility, using Victor 3 fluorometry (Perkin Elmer) with picogreen (Invitrogen, Thermo Fisher Scientific) and also agarose gel electrophoresis. Metagenomic libraries were prepared using TruSeq DNA PCR-Free (Illumina) with 350 bp insert. Library construction for blank samples failed due to low concentration (<10ng/μL); thus, further analysis was dropped as this result rules out cross-contamination between samples. All libraries were subjected to shotgun sequencing using the NovaSeq6000 platform (Illumina) at Macrogen Europe B.V. (Netherlands), resulting in 150 bp paired-end read library, with approximately 52–105 M reads dependent on the sample.
Quality check, using Fastp v0.20.1 program, resulted in approximately 1% loss of total reads in all samples, mainly due to cleaning of low-quality reads. Reads were further assembled into contigs using MEGAHIT (Li et al., 2015), with standard parameters. Obtained contigs were further subjected to blastx alignment (Camacho et al., 2009) against databases containing protein sequences potentially engaged in N-cycle-related processes. Databases were constructed with regard to four N-cycle-related processes: anammox, denitrification, DNRA, and nitrification with the addition of a fifth database comprised of proteins engaged in DNR. Databases were manually curated, using CD-HIT (v 4.8.1) with -g 1 -s 0.8 -c 0.9 parameters, from sequences mainly obtained from NcycDB (Tu et al., 2018) at 100% identity cutoff. Blastx output with an e-value of 1e−4 pinpointed contigs exhibiting sequence similarities to N-cycle-related genes, which quantity was further narrowed down by bit score ≥ 50 (Pearson, 2013). Selected contigs were annotated using Prokka (Seemann, 2014), with –a metagenome flag, mainly to extract ORFs predicted by Prodigal (Hyatt et al., 2010) incorporated into the Prokka suite. Potential N cycle genes were retrieved by alignment of predicted ORFs against databases described previously. Obtained datasets were subjected to the prediction of evolutionarily conserved protein domains and motifs (Marchler-Bauer and Bryant, 2004), against the Conserved Domain Database (CDD database, https://www.ncbi.nlm.nih.gov/cdd/) with the expected value threshold set to 1e−4, to sift through genes bearing incomplete domain motifs. In case of NAP, NAR, HZS, and NOR protein-coding genes, as the subunits are found in operons, standalone genes were omitted. A different strategy was also implemented for NXR encoding genes, solely focusing on the identity of sequence to reference (7B04), as reference sequence encoding NXR (nxrA) did not contain a complete domain. Usage of the pfam13447 domain to distinguish HAO encoding genes was insufficient, as it covers up to seven CxxCH repeated sequence motifs while HAO requires eight. Sequences were searched manually for additional motifs outside of complete pfam13447 domain spans.
To allow comparison of gene abundance, the BWA-MEM algorithm containing the BWA software package (v0.7.17-r1188) was used to perform local alignment mapping raw reads from each season against their corresponding assembled metagenome, utilized as index file. Within spans of obtained gene sequences, the number of primary alignment reads was counted using samtools (v 1.11) view -c -F 260 flags using the BWA alignment output, BAM file, as an input. To account for the discrepancy in datasets size and gene lengths, the RPKM (reads per kilobase per million mapped reads) standardization (reads per kilobase per million sequenced reads) was used.
Datasets of raw reads were classified using the Kraken2 classification taxonomic system (Wood et al., 2019) utilizing Kraken standard library. Taxonomic assignments of N-cycle-related genes were performed on the whole nucleotide sequence of contigs bearing target genes, utilizing the aforementioned classification tool. Binning software MetaBAT2 (v2.10.2) was employed on contigs, using standard commands (Kang et al., 2019). Contigs within bins were searched with regard to SSU (16S rRNA) sequences by ssu_finder contained within CheckM suite (v1.1.6) (Karsenti et al., 2011). Found sequences were subjected to SINA Aligner (v1.2.11), aligning sequences according to SILVA taxonomy (Pruesse et al., 2012). Based on 16S rRNA sequence found within P1 iBP.35, Thioglobaceae classified bin was narrowed down to SUP05 clade sp000205985 (sequence identity, 97.8%) (GTDB database) (Spietz et al., 2019). The completeness of potential genomes and their contamination were estimated by CheckM. Contaminated bins (>10% of contamination) that included N-cycle-related genes were resolved by RefineM to assess if contigs bearing targeted genes were marked as contamination. Seawater de novo assembled metagenomes of IDEAL, P1, and BY15 are released under PRJNA906436 BioProject accession number. It is worth mentioning that throughout this paper, we have avoided referring to bins as organisms. Data presenting information on investigated nitrogen-cycle-related bins and gene characteristics across selected depths within the IDEAL, P1, and BY15 are published at Zenodo (Szymczycha et al., 2024). The genes investigated in this study included amoA, amoB, amoC, hao, and nxrA, which are associated with nitrification; napA, napB, napC, narG, narH, narI, narJ, and narY, which are involved in DNR; nrfA and otr, which are linked to DNRA; nirK, nirS, norB, norC, and nosZ, which are related to denitrification; and hzo, hdh, and hzsABC, which are indicative of anammox.
Dissimilatory nitrate reduction is the initial step in several nitrogen-related processes, including denitrification, dissimilatory nitrite reduction to ammonium (DNRA), anaerobic methane oxidation (DAMO), and anammox (e.g., Pajares and Ramos, 2019). However, DAMO has not yet been identified in the Baltic Sea waters (e.g., Mazur-Marzec et al., 2024). To compare nitrogen-related processes potential and accompanying microbial community abundance across different sites (Bornholm Deep, Gdańsk Deep, and Gotland Deep) and to account for seasonal variations, we have discussed nitrification, DNR, denitrification, DNRA, and anammox separately.
Kruskal–Wallis and Dunn’s tests were used in order to compare the obtained results. To identify significant differences, non-parametric Kruskal–Wallis tests were performed. For all parameters with significant Kruskal–Wallis test (α = 0.05), post-hoc pairwise comparisons were done using Dunn’s test. Pairwise comparisons of bins between depths were conducted by FastANI (v1.34), meaning pairwise comparisons were performed, with each site compared to two other sites. All calculations to measure biological diversity were done in R (v3.6.3), utilizing stats and vegan packages.
Salinity, temperature, and dissolved oxygen distributions in each station are presented in Figure 2 and Table 1. S, T, and O2 profiles show the typical seasonal distribution for the Baltic Sea depths. O2 starts to drop with depth below the halocline; however, the most rapid decline was noticed for BY15 within all seasons. The physicochemical characterization of each sampling layer is presented in Table 1. T and S at each site and sample depth had comparable values. Oxygenated conditions occurred only at the sampling depth located at P1 during two sampling periods in 9.21 and 4.22, while hypoxic conditions occurred (2.4 ≤ O2 ≤ 90 µmol L−1) at all other sampling depths within sampling sites and sampling periods. DOC, NO3−, NO2−, NH4+, and PO43− distribution (Table 1) did not show any pattern among seasons and stations.
Figure 2. Depth profiles of temperature (T), salinity (S) and oxygen (O2) in Bornholm Deep (IDEAL), Gdańsk Deep (P1), and Gotland Deep (BY15). Shaded areas correspond to the investigated water column layers (Table 1).
The datasets of raw reads varied across seasons and sampling sites, ranging from 68.9 to 105.8 million at IDEAL, 58.5 to 103.1 million at P1, and 52.6 to 104.5 million at BY15. Taxonomic assignment of bacterial and archaeal kingdoms, based on paired-end raw reads (above 0.02%), revealed the microbial community structure at the phylum level. Across all seasons and study sites, the phyla Thaumarchaeota and Proteobacteria dominated the taxonomic composition, while Bacteroidetes, Firmicutes, and Actinobacteria were present at lower abundances but consistently above 0.4% (Figure 3).
Figure 3. General characterization of prokaryotic raw reads at the phyla level. Water samples were collected in Bornholm Deep (IDEAL), Gdańsk Deep (P1) and Gotland Deep (BY15) between September 2020 (9_20) and April 2022 (4_22). The most abundant phyla (≥ 0.02%) were color-coded, with values representing the percentage of phyla raw reads relative to the entire dataset for each season.
De novo assemblies of metagenomes constructed from the 9_20 dataset (including all three sites) were used as references for other seasons. The total number of contigs was 601,521 at IDEAL, 594,764 at P1, and 853,241 at BY15, with 106, 91, and 112 contigs containing genes related to N cycle processes at these sites, respectively. Notably, anammox-related genes were absent in the 9_20 dataset and were excluded from the analysis of data for other seasons.
The relative abundance of nitrification, DNR, DNRA, and denitrification was determined based on protein-coding genes identified within continuous sequences. These abundances were calculated from the sum of raw reads mapped to target genes in each sample. To account for differences in dataset size and gene length, the abundance of nitrogen-cycle-related genes was normalized to the total mapped reads in each sample’s contigs and expressed in RPKM (reads per kilobase per million mapped reads). This allowed for a direct comparison of N-cycle-related protein-coding genes across the study sites (Figures 4, 5). Proteobacteria genes were present across all investigated nitrogen cycle processes, with Thaumarchaeota contributing to nitrification, Actinobacteria to DNR, and Firmicutes and Bacteroidetes to the DNRA process. All these phyla were also involved in denitrification. To explore the functional potential of microorganisms at different sites, metagenome binning was performed, resulting in the recovery of 57/130, 41/89, and 63/179 bins related to nitrogen cycle processes at IDEAL, P1, and BY15, respectively. In terms of abundance, the first quartile of bins at these sites contained 43, 17, and 25 potential nitrogen-cycle-related organisms.
Figure 4. Relative abundance of phyla calculated using the sum of gene RPKM (reads per kilobase per million mapped reads) to account for differences in gene length and dataset size across selected nitrogen cycle processes, including nitrification, DNR, DNRA, and denitrification. Genes associated with these processes were assigned to specific phyla at the study sites (Bornholm Deep - IDEAL, Gdańsk Deep - P1, Gotland Deep - BY15) and categorized by season (X_2X). The intensity of red color represents the abundance of genes involved in these processes. 'NA' indicates genes for which taxonomic classification could not be determined.
Figure 5. Total number of RPKM for each gene found assigned to each analyzed processes in three study sites (Bornholm Deep, IDEAL; Gdańsk Deep, P1; Gotland Deep, BY15). Phylum-assigned values were portrayed as a percent of the total RPKM for each gene in every process across all analyzed seasons.
Genes encoding proteins involved in nitrification, such as AMO, HAO, and NXR, showed no significant seasonal variation in RPKM values (Kruskal–Wallis; p > 0.05). However, genes related to denitrification (nirK, nirS, norBC, and nosZ) and DNR (napABC and narGHIJY) exhibited significant seasonal differences at all study sites (p ≤ 0.003). DNRA-related genes (nrfA and otr) showed significant seasonal variation only at P1 (Kruskal–Wallis; p < 0.001). Pairwise comparisons using Dunn’s test revealed that denitrification-related genes at IDEAL and P1 differed significantly between the 9_20 and 9_21 seasons (p = 0.034, p = 0.002, respectively) and between the 9_20 and 1_22 seasons (p = 0.012, p = 0.002, respectively) (Supplementary Tables S1, S2). At BY15, significant differences in denitrification genes were observed between 9_20 and 4_21 (p = 0.007) and between 9_20 and 4_22 (p = 0.007) (Supplementary Tables S1, S2). Interestingly, DNR-related gene abundances varied in the same seasons as denitrification (Supplementary Tables S1, S2).
Oxidation of ammonium to hydroxylamine (NH2OH) is catalyzed by the archaeal or bacterial ammonia monooxygenase (AMO). Among the nitrification-related genes, AMO-encoding genes displayed the highest abundance in the samples, predominantly found in contigs classified as Thaumarchaeota (Figure 5). Notably, clusters of archaeal AMO-encoding genes appeared twice, each located on separate contigs assigned to the Nitrosopumilaceae family. Similarly, bacterial amoABC genes were also identified in clusters, although they appeared singularly with low abundance in each sample. At the IDEAL and BY15 stations, bacterial AMO-encoding genes were primarily classified within the Nitrosomonadaceae family. However, at the P1 station, these genes were instead assigned to the Methylococcaceae family of Proteobacteria, based on nucleotide identity. Archaeal and bacterial AMOs overall sequence homology shows only approximately 40% amino acid identity (Lancaster et al., 2018); thus, they were quantified separately. As for bacterial AMOs, no previous distinction had been made; however, BLASTn did not show any significance against pmoA primers (Luesken et al., 2011). Contigs containing archaeal AMO-encoding genes were binned with nirK genes at the BY15 and IDEAL stations, based on similar genomic signatures. However, this was not observed at the P1 station, where the homologous bin to BY15 and IDEAL (IBp.17) lacked the nirK gene. The Average Nucleotide Identity (ANI) between the P1 bin and the BY15 and IDEAL bins was 97.85% and 83.83%, respectively (Figure 6). This observation was found consistent with the taxonomic assignment of the above-mentioned genes, as all contigs were classified to the same family of Thaumarchaeota phylum. At P1, however, AMO-encoding genes classified as Thaumarchaeota remained unbinned; thus, their alignment to their binned counterparts in other depths had shown 99% sequence identity (hence lower case letter in IBp.17 bin).
Figure 6. Potential genomes (y-axis) reconstructed from metagenomes found within the study sites (A) Bornholm Deep (IDEAL), (B) Gdańsk Deep (P1), and (C) Gotland Deep (BY15). The selected nitrogen cycle genes (x-axis) such as nitrification, DNR, DNRA, and denitrification were marked with different shapes accounting for the taxonomical assignment of contigs bearing appropriate genes. Name bin.XXX suggest that the hypothetical organism is found exclusively in the respective stations. I (IDEAL), B (BY15), and P (P1) letters in bin names mark which sampling site was found bearing a common bin, where small letters indicate a difference in bin composition in relation to its homologous organism, despite genes presence in respective depth. An asterisk indicates missing genes in datasets while comparing sites, despite presence of homologous bin. Bins with gray areas of heatmap hold columns with 16S rRNA genes found within bins. Proteobacteria were colorcoded with regard to Alpha (red), Beta (blue), and Gamma (gray) classes of phyla
The oxidation of hydroxylamine (NH2OH) to nitric oxide (NO), an essential intermediate, is catalyzed by hydroxylamine dehydrogenase (HAO). However, none of the recovered hao genes were found binned with AMO encoding genes. At P1 and BY15, hao genes were found in bin iBP.35 (ANI 99.4861), which also contained the napABC gene cluster related to dissimilatory nitrate reduction (DNR) and nor genes involved in denitrification. A corresponding hao gene sequence was identified at the IDEAL station on contig IDEAL_6474:2967-4427, but it remained unbinned. Bins containing hao were classified as Proteobacteria, consistent with other nitrogen-cycle-related genes and the 16S rRNA sequence, which assigned them to the Thioglobaceae family. Although a similar bin was found at the IDEAL station (iBP.35; ANI 98.755), it did not contain any genes associated with nitrification (Figure 6).
The final step of canonical nitrification, NO2− oxidation to NO3−, is performed by obligate aerobic bacteria possessing the nitrite oxidoreductase (nxr) gene. The gene encoding NXR subunit A (nxrA) was detected at the IDEAL and BY15 stations but was absent from P1. While most of the abundant nxrA subunits in these samples remained unclassified, they were binned alongside two nirK genes in bins classified as members of the Azospirillaceae Proteobacterial family [IB.18; (ANI) 99.6019] (Figure 6).
The first step of denitrification, namely, the reduction of nitrate (NO3−) to nitrite (NO2−), is mediated by two distinct classes of nitrate reductase: the membrane-bound NAR and periplasmic NAP encoded by the nar and nap operons, respectively. The most abundant genes in DNR process were primarily classified into Proteobacteria and Actinobacteria phyla (Figure 6). High RPKM values for NAP and NAR encoding genes assigned to Proteobacteria at the P1 and BY15 sites within all sampling campaigns were driven by contigs grouped into a single bin, iBP.35 (Figure 7). At the IDEAL station within all sampling campaigns, nar genes were similarly found in bin iBP.35, although homologous nap genes, while present, remained unbinned. In BY15 and P1 within all sampling campaigns, NAR-encoding genes of Actinobacteria were identified in bins, which also contained 16S rRNA sequences classified within the Sporichthyaceae family, specifically in BP.43, along with two additional bins in BY15 (bin.149 and bin.165) (Figure 6). While contigs from IDEAL were also classified within the Actinobacteria phylum, the most abundant bin, Ib.19, belonged to the Microbacteriaceae family. Notably, the Actinobacteria bins containing nar genes did not include any other nitrogen-cycle-related genes.
Figure 7. Potential genomes reconstructed from metagenomes found in (A) Bornholm Deep (IDEAL), (B) Gdańsk Deep (P1) and (C) Gotland Deep (BY15), sorted in accordance to means of gene abundances (RPKM) across seasons. Nitrogen related processes were colour coded where black, red, blue and purple indicate denitrification, DNR, DNRA, and nitrification, respectively.
Dissimilatory reduction of nitrite (NO2−) to ammonium, a reaction catalyzed by the ammonia-forming cytochrome c nitrite reductase, encoded by the nrfA gene, was detected in all samples (Figures 4, 5). All nrfA genes found in BY15 station had their homologous sequence within P1 within all sampling campaigns. Proteobacteria NRF subunit A encoding gene occurred singularly in IDEAL and had no counterparts in other samples. While IDEAL bin.33 is not homologous to BP.40 found in two other sites (ANI 99.408), all those bins contain NOS-encoding and nrfA genes. A high abundance of DNRA engaged Proteobacteria, within P1 and BY15 samples, was due to NRF subunit A encoding genes found within BP.51 bins (ANI 99.600) coupled with NAR encoding genes. Prevalent across seasons nrfA genes in P1 and BY15 were classified as Firmicutes; however, they remained unbinned (Figure 5).
In addition to nrfA, genes encoding octahaem tetrathionate reductase (OTR)—a potential alternative catalyst for the same reaction—were also analyzed. OTR-encoding genes were identified at all stations, primarily classified under the Proteobacteria phylum based on contig taxonomy. The most abundant OTR-encoding genes across all samples were located in the IBp.30 and IBp.25 bins, either standalone or co-located with NAP- and NIR-encoding genes, respectively (Figure 6).
In all samples, the most abundant denitrification-related genes were classified to Proteobacteria, Thaumarchaeota, and Bacteroidetes phyla (Figure 5). The reduction of nitrite (NO2−) to nitric oxide (NO) is carried out by two distinct nitrite reductases, encoded by the nirK and nirS genes. Throughout the seasons, Proteobacteria nirK genes were consistently found in bins together with norBC genes in the iBP.35 bins. Nitric oxide, produced during nitrite reduction, can be further reduced to nitrous oxide (N2O) by nitric oxide reductase (NOR) encoded by norBC genes. The capacity for nitrous oxide reduction conducted by norBC gene was also identified in the Bacteroidetes phylum. In the Bacteroidetes, norBC genes were accompanied by nosZ genes in both IDEAL bin.110, classified to the Flavobacteriaceae family, and BY15 bin.34, classified to the Sphingobacteriaceae family. An exception was the unclassified norBC cluster at P1, which remained unbinned. nirS encodes homodimeric cytochrome cd1 nitrite reductase that has been characterized in Gram-negatives. Proteobacteria bins bearing nirS genes were also found carrying NAP encoding genes. Out of those bins, BP.57 also bearing NOS was classified as Sulfurimonadaceae family and was deemed most abundant hypothetical organism converting N2O to N2 in BY15 sampling site, which is in line with other studies both in the Black Sea and Baltic Sea (Grote et al., 2007; Fuchsman et al., 2012, 2017, 2019; Rogge et al., 2017). Genes encoding nitrous oxide reductase (NOS), responsible for the final reduction of N2O to nitrogen gas (N2), revealed similar organisms as key potential N2 producers across all sampling sites. Although homologous nosZ genes were identified at multiple stations, their abundance varied between locations. Organisms encoding nosZ were primarily associated with the Proteobacteria and Bacteroidetes phyla. Notably, Bacteroidetes bins carrying nosZ genes with RPKM values above 5 were consistently classified within the Flavobacteriaceae family (Figure 5).
Key enzymes for anammox process, specifically hydrazine oxidoreductase (hzo) and hydrazine dehydrogenase (hdh), which oxidize hydrazine intermediate to dinitrogen gas, were not detected in any of the sites. Additionally, genes encoding hydrazine synthesis (hzsABC) were absent from all sites.
Marine water represents a vast habitat for pelagic habitats and plays essential roles in biogeochemical cycles, influencing global processes such as carbon sequestration and nutrient recycling. Among the unique marine environments, oxygen variable areas (changing from oxic via hypoxic sometimes reaching anoxic) stand out as critical regions for nitrogen cycling (Stramma et al., 2010; Dalsgaard et al., 2014; Bristow et al., 2016; Suenaga et al., 2018; Sun et al., 2021). Understanding the dynamics of microbial communities in these environments and their functional roles can provide insights into how marine environments respond to changes in oxygen concentration, nutrient availability, and climate change (Pajares and Ramos, 2019).
This study clearly identified Thaumarchaeota and Proteobacteria as the dominant phyla across various sampling depths at IDEAL, P1, and BY15 and within all sampling campaigns (seasons). Therefore, it seems that Thaumarchaeota and Proteobacteria phyla have the most significant impact on nitrogen cycle in the Baltic Sea. Similar findings have been reported in studies from other marine environments, such as the North Atlantic and coastal regions, where Proteobacteria frequently dominate due to their roles in nutrient cycling (Fuchsman et al., 2017). In addition, within all investigated seasons, all sites were predominantly inhabited by AOA, which is in line with other investigations where AOA was linked to oxic–anoxic interfaces in environments similar to the Baltic Sea, e.g., semi-enclosed with low salinity Black Sea, and Pacific Ocean (Francis et al., 2005). AMO complex requires O2 for expression, which negatively affects ammonia oxidation rates and its limiting growth of AOA, when its concentration is found in submicromolar range (Bristow et al., 2016). While inflows of North Sea water, bringing oxygenated waters, are limiting growth of AOA and are essential to sustain their biomass (Buchanan et al., 2023), numerical domination over AOB may not reflect their activity but rather be controlled by environmental variables such as salinity and sulfide concentrations (Caffrey et al., 2007). Crenarchaeota AMO subunit abundance was previously correlated with a decline in ammonium concentration (Wuchter et al., 2006), and a positive correlation between a number of Crenarchaeota/Thaumarchaeota cells and elevated levels of nitrite concentration along with ammonia depletion was also reported (Könneke et al., 2005). However, no correlation of such was found in our chemical results (Table 1). Moreover, while conditions across seasons varied, nitrification-related genes did not express any significant change in abundance between sites and seasons (Supplementary Tables S1, S2). The absence of a correlation between nitrification-related genes and ammonia or nitrite levels suggests additional environmental factors influencing microbial processes. In all sites, AOA outcompete AOB due to better adaptation of microbes to variable oxygen conditions. In this study, archaea AMO genes were contained in bin (IBp.17) bearing 16S rRNA sequence classified as either Crenarchaeota (SILVA taxa) or Thaumarchaeota (RDP taxa), further unanimously classified to Nitrosopumilaceae family. AOA species are equipped in a comprehensive array of genes, which allow them to use ammonia as nitrogen (assimilation) or energy source (oxidation) and help them survive under environmental pressures. What is more, under stress conditions, nitric oxide (NO) is crucial in ammonia oxidation by AOA, with NirK playing a key role in this pathway (Qin et al., 2020). Indirectly, the functional importance of NirK was confirmed in this study. At IDEAL, contigs that carried AMO-encoding genes were binned solely with nirK genes. However, the role of NirK in AOA ammonia catabolism is still not well understood by the scientific community. Hydroxylamine oxidoreductase (HAO) was not contained within bins carrying amo genes, confirming the suggestion of unidentified F420-dependent monooxygenases or Cu-containing metalloenzymes being involved in the oxidation of hydroxylamine (NH2OH) (Kerou et al., 2016).
The most abundant HAO-encoding genes were binned with nirK and norBC genes, as well as with napAB and narGHIJ clusters (iBP.35; Figure 7) at P1. A comparable set of genes is found in IDEAL and BY15, which likewise, in accordance to the contigs classification, were affiliated with the Thioglobaceae family of Proteobacteria phylum. These findings were comparable to those of Arora-Williams et al. (2022), where their MAG classified as Thioglobaceae contained the same set of genes as the bins mentioned above. The Thioglobaceae family in low oxygen conditions is capable of respiring nitrate instead of oxygen (Mattes et al., 2021). Interestingly, the hao family genes were found to be located immediately downstream of the NAP encoding gene clusters (Haase et al., 2017), as reported in this study in iBP.35 bins. Reduction in nitrite or hydroxylamine to ammonium may be carried out by HAO enzyme, which may be the case within those sites due to the fact that higher levels of NH4+ in seasons came with exhaustion of nitrate and nitrite pools, with higher abundance (RPKM < 100) of iBP.35 bins. Thus, this process is contributing to retention of nitrogen pool within sites. However, at oxygen concentrations approximately 1% of atmospheric levels (~2.8 µmol L-¹), nirK gene expression has been detected in cultured bacteria (Hartsock and Shapleigh, 2010). This suggests that the bacteria may be capable of adapting to denitrification under these conditions. At all sites, iBP.35. nirK gene was coupled with NOR, which is used in the reduction in nitric oxide (NO) formed as a result of nitrite reduction. These potential organisms can hypothetically produce nitrous oxide (N2O) as an end product (Sun et al., 2021). N2O release has also been documented in NRF-mediated DNRA process (Heo et al., 2020). The excess N2O stream can diffuse to the atmosphere and act as a greenhouse gas and ozone-depleting agent; however, N2O-reducing bacteria have a high affinity for N2O (Suenaga et al., 2018). Lacking any other N-cycle-related processes protein coding genes most abundant NOS proteins at all sites were assigned to the Flavobacteriaceae family of Bacteroidetes, as in Rasigraf et al. (2017) studies, conducted within the same basin. Vibrionaceae (IBP.08) and Sulfurimonadaceae (BP.57) families of Proteobacteria phylum were also found as potential NOS producers. Oxygen can inhibit the oxygen-sensitive nitric oxide synthase (NOS) enzyme, thereby reducing N2O consumption and potentially increasing its emissions. In contrast, the nitric oxide reductase (NOR) enzyme is comparatively less affected by O2 concentrations. In incubation experiments, partial inhibition of N2 and N2O emissions was deemed at nanomolar presence of O2 (Dalsgaard et al., 2014), while nitrogen loss rates during denitrification were held up when O2 levels reached 3 µmol L−1 (Babbin et al., 2014). According to those studies in situ only during handful of seasons, bacteria could potentially carry out final steps of denitrification process. Denitrification may occur in anoxic zones within particles at the oxygen concentrations sampled in this study (Bianchi et al., 2018; Fuchsman et al., 2019; Stief et al., 2016). Although particles were removed from these samples using a prefilter, bacteria originally associated with the particles could still be present in the water column. Thioglobaceae family and Nitrosopumilaceae can also grow by carbon fixation; however, DOC fluctuations across seasons did not reflect on above mentioned family abundance.
The absence of anammox genes in this study contrasts sharply with findings in other regions, such as the North Sea, where anammox has been identified as a significant process contributing to nitrogen removal in hypoxic environments (Thamdrup et al., 2006).
In our study, we investigated the nitrogen cycle by analyzing microbial abundance and associated functional genes below the halocline in the Baltic Sea, examining both seasonal and spatial variations. Our findings revealed no significant spatial variation, suggesting that the studied ecosystem exhibits a consistent nitrogen processing capacity across different locations. However, seasonality emerged as a key factor, as changes in nutrient and oxygen conditions throughout the year significantly influence microbial activity and the associated nitrogen cycling processes. This study contributes to the understanding and interpretation of how microbial communities might shift in their activity in a changing ocean like the Baltic Sea.
The study presents an analysis of the physicochemical and microbial dynamics across three sampling sites (below the halocline at depths ranging from 75 to 135 m) in the Baltic Sea, revealing important insights into seasonal variations in oxygen and nitrogen cycling processes. The hypoxic conditions across most sampling depths underline the potential ecological stressors present in these waters, which aligns with other findings in the Baltic Sea (Conley et al., 2009) and Black Sea (Grote et al., 2007). The analysis of microbial community structure revealed a predominance of Thaumarchaeota and Proteobacteria, consistent with previous studies indicating their essential roles in nitrogen cycling. The consistent presence of Bacteroidetes, Firmicutes, and Actinobacteria, albeit at lower abundances, highlights the complexity and diversity of microbial life in the Baltic Sea. The presence of nitrification-related genes, particularly those encoding ammonia monooxygenase (AMO), was substantial, especially from Thaumarchaeota, suggesting a critical role in ammonium oxidation.
Interestingly, all DNR genes (napA, napB, napG, narH, narI, and narJ), except for narY, were significantly more abundant than DNRA genes (nrfA and otr). This observation suggests that the dissimilatory reduction of nitrate to nitrite is inherently more efficient than the dissimilatory reduction of nitrite to ammonia. Conservatively, DNRA refers to nitrate reduction to ammonium; differentiating between dissimilatory nitrate reduction (DNR) and dissimilatory nitrite reduction to ammonium (DNRA) is essential for gaining deeper insights into the complexities of the nitrogen cycle, particularly in dynamic ecosystems like the Baltic Sea.
Our findings revealed no significant spatial variation, suggesting that the studied ecosystem exhibits a consistent nitrogen processing capacity across different locations, with the most potential for nitrification, DNR, and denitrification. However, seasonality emerged as a key factor, as changes in nutrient and oxygen conditions throughout the year significantly influence microbial activity and the associated nitrogen cycling processes.
The obtained results suggest that while certain microbial phyla are consistently present, the functional potential can vary significantly with seasonal changes and site-specific factors. Continued monitoring and detailed investigations into these processes will be crucial for predicting ecosystem responses to environmental changes and informing conservation strategies in the Baltic Sea region. The absence of certain key genes, such as those involved in the anammox process, also suggests potential gaps in the current understanding of nitrogen cycling dynamics that warrant further exploration. This study contributes to the understanding and interpretation of how microbial communities might shift in their activity in a changing ocean like the Baltic Sea.
All sequencing data obtained for this project were submitted to GenBank, and they are available under the BioProject accession number PRJNA906436. Data presenting information on investigated nitrogen cycle related bins, gene characteristics across selected depths within the IDEAL, P1, and BY15 and bin contigs characterization are published at Zenodo (Szymczycha et al., 2024).
MG: Conceptualization, Data curation, Formal analysis, Investigation, Methodology, Validation, Visualization, Writing – original draft, Writing – review & editing. EK: Writing – review & editing. AŁ: Writing – review & editing. KH: Writing – review & editing. GW: Writing – review & editing. BS: Conceptualization, Funding acquisition, Investigation, Methodology, Supervision, Writing – review & editing.
The author(s) declare financial support was received for the research, authorship, and/or publication of this article. The reported results were obtained within the framework of the statutory activities of the Institute of Oceanology of the Polish Academy of Sciences and the following research project: 2019/34/E/ST10/00217 funded by the Polish National Science Centre.
We would like to acknowledge Marta Borecka, Katarzyna Koziorowska-Makuch, Karol Kuliński, and Przemysław Makuch for their help in sample collections and Marc Silberberger for map preparation.
The authors declare that the research was conducted in the absence of any commercial or financial relationships that could be construed as a potential conflict of interest.
All claims expressed in this article are solely those of the authors and do not necessarily represent those of their affiliated organizations, or those of the publisher, the editors and the reviewers. Any product that may be evaluated in this article, or claim that may be made by its manufacturer, is not guaranteed or endorsed by the publisher.
The Supplementary Material for this article can be found online at: https://www.frontiersin.org/articles/10.3389/fmars.2025.1456825/full#supplementary-material
Almroth-Rosell E., Wåhlström I., Hansson M., Väli G., Eilola K., Andersson P., et al. (2021). A regime shift toward a more anoxic environment in a eutrophic sea in northern Europe. Front. Mar. Sci. 8. doi: 10.3389/fmars.2021.799936
Arora-Williams K., Holder C., Secor M., Ellis H., Xia M., Gnanadesikan A., et al. (2022). Abundant and persistent sulfur-oxidizing microbial populations are responsive to hypoxia in the Chesapeake Bay. Environ. Microbiol. 24, 2315–2332. doi: 10.1111/1462-2920.15976
Babbin A. R., Keil R. G., Devol A. H., Ward B. B. (2014). Organic matter stoichiometry, flux, and oxygen control nitrogen loss in the ocean. Science 344, 406–408. doi: 10.1126/science.1248364
BACC II, Team A (2015). Second assessment of climate change for the Baltic Sea basin (Cham, Switzerland: Springer Open).
Beusen A. H. W., Bouwman A. F., Van Beek L. P. H., Mogollón J. M., Middelburg J. J. (2016). Global riverine N and P transport to ocean increased during the 20th century despite increased retention along the aquatic continuum. Biogeosciences 13, 2441–2451. doi: 10.5194/bg-13-2441-2016
Bianchi D., Weber T. S., Kiko R., Deutsch C. (2018). Global niche of marine anaerobic metabolisms expanded by particle microenvironments. Nat. Geosci. 11, 263–268. doi: 10.1038/s41561-018-0081-0
Bonaglia S., Hylén A., Rattray J., Kononets M., Ekeroth N., Roos P., et al. (2017). The fate of fixed nitrogen in marine sediments with low organic loading: An in situ study. Biogeosciences 14, 285–300. doi: 10.5194/bg-14-285-2017
Bonaglia S., Klawonn I., De Brabandere L., Deutsch B., Thamdrup B., Brücher V. (2016). Denitrification and DNRA at the Baltic Sea oxic–anoxic interface: Substrate spectrum and kinetics. Limnol. Oceanogr. 61, 1900–1915. doi: 10.1002/lno.10343
Bristow L. A., Dalsgaard T., Tiano L., Mills D. B., Bertagnolli A. D., Wright J. J., et al. (2016). Ammonium and nitrite oxidation at nanomolar oxygen concentrations in oxygen minimum zone waters. Proc. Natl. Acad. Sci. 113, 10601–10606. doi: 10.1073/pnas.1600359113
Buchanan P. J., Sun X., Weissman J., McCoy D., Bianchi D., Zakem E. (2023). Oxygen intrusions sustain aerobic nitrite-oxidizing bacteria in anoxic marine zones. bioRxiv. doi: 10.1101/2023.02.22.529547
Burgin A. J., Hamilton S. K. (2007). Have we overemphasized the role of denitrification in aquatic ecosystems? A review of nitrate removal pathways. Front. Ecol. Environ. 5, 89–96. doi: 10.1890/1540-9295(2007)5[89:HWOTRO]2.0.CO;2
Caffrey J. M., Bano N., Kalanetra K., Hollibaugh J. T. (2007). Ammonia oxidation and ammonia-oxidizing bacteria and archaea from estuaries with differing histories of hypoxia. ISME. J. 1 (7), 660–662. doi: 10.1038/ismej.2007.79
Camacho C., Coulouris G., Avagyan V., Ma N., Papadopoulos J., Bealer K., et al. (2009). BLAST+: architecture and applications. BMC Bioinf. 10, 421. doi: 10.1186/1471-2105-10-421
Conley D. J., Björck S., Bonsdorff E., Carstensen J., Destouni G., Gustafsson B. G., et al. (2009). Hypoxia-related processes in the baltic sea. Environ. Sci. Technol. 43, 3412–3420. doi: 10.1021/es802762a
Dalsgaard T., Stewart F. J., Thamdrup B., De Brabandere L., Revsbech N. P., Ulloa O., et al. (2014). Oxygen at nanomolar levels reversibly suppresses process rates and gene expression in anammox and denitrification in the oxygen minimum zone off northern Chile. mBio 5, e01966–e01914. doi: 10.1128/mBio.01966-14
Devlin M., Brodie J. (2023). “Nutrients and eutrophication,” in Marine pollution – monitoring, management and mitigation. Ed. Reichelt-Brushett A. (Springer Nature Switzerland, Cham), 75–100.
Fowler D., Coyle M., Skiba U., Sutton M. A., Cape J. N., Shepard L. J., et al. (2013). The global nitrogen cycle in the twenty-first century. Philos. Trans. R. Soc. B.: Biol. Sci. 368, 20130164. doi: 10.1098/rstb.2013.0164
Francis C. A., Roberts K. J., Beman J. M., Santoro A. E., Oakley B. B. (2005). Ubiquity and diversity of ammonia-oxidizing archaea in water columns and sediments of the ocean. Proc. Natl. Acad. Sci. U.S.A. 102 (41), 14683–14688. doi: 10.1073/pnas.0506625102
Fuchsman C. A., Devol A. H., Sauders J. K., McKay C., Rocap G. (2017). Niche Partitioning of the N cycling microbial community of an offshore Oxygen Deficient Zone. Front. Microbiol. 8. doi: 10.3389/fmicb.2017.02384
Fuchsman C. A., Murray J. W., Staley J. T. (2012). Stimulation of autotrophic denitrification by intrusions of the Bosporus Plume into the anoxic Black Sea. Front. Microbiol. 3. doi: 10.3389/fmicb.2012.00257
Fuchsman C. A., Paul B., Staley J. T., Yakushev E. V., Murray J. W. (2019). Detection of transient denitrification during a high organic matter event in the Black Sea. Global Biogeoche. Cycles. 33, 143–162. doi: 10.1029/2018GB006032
Galloway J. N., Townsend A. R., Erisman J. W., Bekunda M., Cai Z., Freney J. R., et al. (2008). Transformation of the nitrogen cycle: Recent trends, questions, and potential solutions. Science 320, 889–892. doi: 10.1126/science.1136674
Grote J., Labrenz M., Pfeiffer B., Jost G., Jürgens K. (2007). Quantitative distributions of Epsilonproteobactena and a Sulfurimonas subgroup in pelagic redoxclines of the central Baltic Sea. Appl. Environ. Microbiol. 73, 7155–7161. doi: 10.1128/AEM.00466-07
Gruber N., Galloway J. N. (2008). An Earth-system perspective of the global nitrogen cycle. Nature 451, 293–296. doi: 10.1038/nature06592
Haase D., Hermann B., Einsle O., Simon J. (2017). Epsilonproteobacterial hydroxylamine oxidoreductase (ϵHao): Characterization of a ‘missing link’ in the multihaem cytochrome c family. Mol. Microbiol. 105, 127–138. doi: 10.1111/mmi.13690
Hartsock A., Shapleigh J. P. (2010). Mechanisms of oxygen inhibition of nirK expression in Rhodobacter sphaeroides. Microbiology 156, 3158–3165. doi: 10.1099/mic.0.0387030
Heo H., Kwon M., Song B., Yoon S. (2020). Involvement of NO3– in ecophysiological regulation of dissimilatory nitrate/nitrite reduction to ammonium (DNRA) is implied by physiological characterization of soil DNRA bacteria isolated via a colorimetric screening method. Appl. Environ. Microbiol. 86, e01054–e01020. doi: 10.1128/AEM.01054-20
Hietanen S., Jäntti H., Buizert C., Jürgens K., Labrenz M., Voss M., et al. (2012). Hypoxia and nitrogen processing in the baltic sea water column limnol. Oceanogr. 57, 325–337. doi: 10.4319/lo.2012.57.1.0325
Hyatt D., Chen G. L., LoCascio P. F., Land M. L., Larimer F. W., Hauser L. J. (2010). Prodigal: Prokaryotic gene recognition and translation initiation site identification. BMC Bioinf. 11, 119. doi: 10.1186/1471-2105-11-119
Kang D. D., Li F., Kirton E., Thomas A., Egan R., An H., et al. (2019). MetaBAT 2: An adaptive binning algorithm for robust and efficient genome reconstruction from metagenome assemblies. PeerJ 26, e7359. doi: 10.7717/peerj.7359
Karsenti E., Acinas S. G., Bork P., Bowler C., De Vargas C., Raes J., et al. (2011). A holistic approach to marine ecosystems biology. PloS Biol. 9, e1001177. doi: 10.1371/journal.pbio.1001177
Kerou M., Offre P., Valledor L., Abby S. S., Melcher M., Nagler M., et al. (2016). Proteomics and comparative genomics of Nitrososphaera viennensis reveal the core genome and adaptations of archaeal ammonia oxidizers. Proc. Natl. Acad. Sci. United. States America 113, E7937–E7946. doi: 10.1073/pnas.1601212113
Könneke M., Bernhard A., de la Torre A., Walker C. B., Waterbury J. B., Stahl D. A, et al. (2005). Isolation of an autotrophic ammonia-oxidizing marine archaeon. Nature 437 (7058), 543–546. doi: 10.1038/nature03911
Kraft B., Jehmlich N., Larsen M., Bristow L. A., Könneke M., Thamdrup B., et al. (2022). Oxygen and nitrogen production by an ammonia-oxidizing archaeon. Science 375, 97–100. doi: 10.1126/science.abe6733
Kuliński K., Rehder G., Asmala E., Bartosova A., Carstensen J., Gustafsson B., et al. (2021). Baltic earth assessment report on the biogeochemistry of the baltic sea. Earth Syst. Dynamics. 2021, 1–93. doi: 10.5194/esd-2021-33
Lancaster K. M., Caranto J. D., Majer S. H., Smith M. A. (2018). Alternative bioenergy: Updates to and challenges in nitrification metalloenzymology. Joule 2, 421–441. doi: 10.1016/j.joule.2018.01.018
Li D., Liu C.-M., Luo R., Sadakane K., Lam T.-W. (2015). MEGAHIT: An ultra-fast single-node solution for large and complex metagenomics assembly via succinct de Bruijn graph. Bioinformatics 31, 1674–1676. doi: 10.1093/bioinformatics/btv033
Liblik T., Naumann M., Alenius P., Hansson M., Lips U., Nausch G., et al. (2018). Propagation of impact of the recent Major Baltic inflows from the eastern Gotland Basin to the Gulf of Finland. Front. Mar. Sci. 5. doi: 10.3389/fmars.2018.00222
Luesken F. A., Zhu B., van Alen T. A., Butler M. K., Diaz M. R., Song B., et al. (2011). pmoA primers for detection of anaerobic methanotrophs. Appl. Environ. Microbiol. 77, 3877–3880. doi: 10.1128/AEM.02960-10
Malone T. C., Newton A. (2020). The globalization of cultural eutrophication in the coastal ocean: Causes and consequences. Front. Mar. Sci. 7. doi: 10.3389/fmars.2020.00670
Marchler-Bauer A., Bryant S. H. (2024). CD-search: protein domain annotations on the fly. Nucleic Acids Res. 32, W327–W331. doi: 10.1093/nar/gkh454
Mattes T. E., Ingalls A. E., Burke S., Morris R. M. (2021). Metabolic flexibility of SUP05 under low DO growth conditions. Environ. Microbiol. 23, 2823–2833. doi: 10.1111/1462-2920.15226
Mazur-Marzec H., Andersson A. F., Błaszczyk A., Dąbek P., Górecka E., Grabski M., et al. (2024). Biodiversity of microorganisms in the Baltic Sea: the power of novel methods in the identification of marine microbes. FEMS Microbiol. Rev. 48. doi: 10.1093/femsre/fuae024
Pajares S., Ramos R. (2019). Processes and microorganisms involved in the marine nitrogen cycle: Knowledge and gaps. Front. Mar. Sci. 6. doi: 10.3389/fmars.2019.00739
Pascoal F., Tomasino M. P., Piredda R., Quero G. M., Torgo L., Poulain J., et al. (2023). Inter-comparison of marine microbiome sampling protocols. ISME. Commun. 3, 84. doi: 10.1038/s43705-023-00278-w
Pearson W. R. (2013). An introduction to sequence similarity (“homology”) searching. Curr. Protoc. Bioinf. 3, 3.1.1–3.1.8. doi: 10.1002/0471250953.bi0301s42
Pruesse E., Peplies J., Glöckner F. O. (2012). SINA: Accurate high-throughput multiple sequence alignment of ribosomal RNA genes. Bioinformatics 28, 1823–1829. doi: 10.1093/bioinformatics/bts252
Qin W., Zheng Y., Zhao F., Wang Y., Urakawa H., Martens-Habbena W., et al. (2020). Alternative strategies of nutrient acquisition and energy conservation map to the biogeography of marine ammonia-oxidizing archaea. ISME. J. 14, 2595–2609. doi: 10.1038/s41396-020-0710-7
Rak D., Walczowski W., Dzierzbicka-Głowacka L., Shchuka S. (2020). Dissolved oxygen variability in the southern baltic sea in 2013–2018. Oceanologia 62, 525–537. doi: 10.1016/j.oceano.2020.08.005
Rasigraf O., Schmitt J., Jetten M. S. M., Lüke C. (2017). Metagenomic potential for and diversity of N-cycle driving microorganisms in the Bothnian Sea sediment. MicrobiologyOpen 6, e00475. doi: 10.1002/mbo3.475
Reyes C., Schneider D., Lipka M., Thürmer A., Böttcher M. E., Friedrich M. W. (2017). Nitrogen metabolism genes from temperate marine sediments. Mar. Biotechnol. 19, 175–190. doi: 10.1007/s10126-017-9741-0
Rogge A., Vogts A., Voss M., Jürgens K., Jost G., Labrenz M. (2017). Success of chemolithoautotrophic SUP05 and Sulfurimonas GD17 cells in pelagic Baltic Sea redox zones is facilitated by their lifestyles as K- and r-strategists. Environ. Microbiol. 19, 2495–2506. doi: 10.1111/1462-2920.13783
Schinke H., Matthäus W. (1998). On the causes of major Baltic inflows: An analysis of long time series. Continental. Shelf. Res. 18, 67–97. doi: 10.1016/S0278-4343(97)00071-X
Schmale O., Schneider von Deimling J., Gülzow W., Nausch G., Waniek J. J., Rehder G., et al (2010). Distribution of methane in the water column of the baltic sea. Geophys. Res. Lett. 37, L12604. doi: 10.1029/2010GL043115
Seemann T. (2014). Prokka: Rapid prokaryotic genome annotation. Bioinformatics 30, 2068–2069. doi: 10.1093/bioinformatics/btu153
Spietz R. L., Marshall K. T., Zhao X., Morris R. M. (2019). Complete genome sequence of “Candidatus thioglobus sp.” Strain NP1, an open-ocean isolate from the SUP05 clade of marine gammaproteobacteria. Microbiol. Resour. Announc. 8, e00097–e00019. doi: 10.1128/MRA.00097-19
Stief P., Kamp A., Thamdrup B., Glud R. N. (2016). Anaerobic nitrogen turnover by sinking diatom aggregates at varying ambient oxygen levels. Front. Microbiol. 7. doi: 10.3389/fmicb.2016.00098
Stramma L., Schmidtko S., Levin L. A., Johnson C. (2010). Ocean oxygen minimum expansion and their biological impacts. Deep-Sea. Res. Part I. 57, 587–595. doi: 10.1016/j.dsr.2010.01.005
Suenaga T., Riya S., Hosomi M., Terada A. (2018). Biokinetic characterization and activities of N2O-reducing bacteria in response to various oxygen levels. Front. Microbiol. 9. doi: 10.3389/fmicb.2018.00697
Sun X., Frey C., Garcia-Robledo E., Jayakumar A., Ward B. B. (2021). Microbial niche differentiation explains nitrite oxidation in marine oxygen minimum zones. ISME. J. 15, 1317–1329. doi: 10.1038/s41396-020-00852-3
Szymczycha B., Grabski M., Kotlarska E., Łuczkiewicz A., Węgrzyn G. (2024). Spatial and seasonal distribution of selected nitrogen cycle genes in deep waters of the Baltic Proper [Data set] (Geneva, Switzerland: Zenodo). doi: 10.5281/zenodo.13836219
Thamdrup B., Dalsgaard T., Jensen M. M., Ulloa O., Farías L., Escribano R. (2006). Anaerobic ammonium oxidation in the oxygen-deficient waters off northern Chile. Limnol. Oceanogr. 51, 2145–2156. doi: 10.4319/lo.2006.51.5.2145
Tu Q., Lin L., Cheng L., Deng Y., He Z. (2018). NCycDB: A curated integrative database for fast and accurate metagenomic profiling of nitrogen cycling genes. Bioinformatics 35, 1040–1048. doi: 10.1093/bioinformatics/bty741
Voss M. W., Heo S., Prakash R. S., Erickson K. I., Alves H., Chaddock L. (2013). The influence of aerobic fitness on cerebral white matter integrity and cognitive function in older adults: Results of a one-year exercise intervention. Hum. Brain Mapp. 34, 2972–2985. doi: 10.1002/hbm.22119
Wood D. E., Lu J., Langmead B. (2019). Improved metagenomic analysis with Kraken 2. Genome Biol. 20, 257. doi: 10.1186/s13059-019-1891-0
Wuchter C., Abbas B., Coolen M. J., Herfort L., van Bleijswijk J., Timmers P., et al. (2006). Archaeal nitrification in the ocean. Proc. Natl. Acad. Sci. U S A. 103 (33), 12317–12322. doi: 10.1073/pnas.0600756103
Keywords: nitrification, DNRA, DNR, denitrification, anammox, metagenome, oxygen deficiency, DNA
Citation: Grabski M, Kotlarska E, Łuczkiewicz A, Hryniewicz K, Węgrzyn G and Szymczycha B (2025) Spatial and seasonal distribution of selected nitrogen cycle genes in deep waters of the Baltic Proper. Front. Mar. Sci. 12:1456825. doi: 10.3389/fmars.2025.1456825
Received: 29 June 2024; Accepted: 16 January 2025;
Published: 10 February 2025.
Edited by:
Nicholas David Ward, Pacific Northwest National Laboratory (DOE), United StatesReviewed by:
Allison Moreno, University of California, Santa Cruz, United StatesCopyright © 2025 Grabski, Kotlarska, Łuczkiewicz, Hryniewicz, Węgrzyn and Szymczycha. This is an open-access article distributed under the terms of the Creative Commons Attribution License (CC BY). The use, distribution or reproduction in other forums is permitted, provided the original author(s) and the copyright owner(s) are credited and that the original publication in this journal is cited, in accordance with accepted academic practice. No use, distribution or reproduction is permitted which does not comply with these terms.
*Correspondence: Beata Szymczycha, YmVhdHN6QGlvcGFuLnBs
Disclaimer: All claims expressed in this article are solely those of the authors and do not necessarily represent those of their affiliated organizations, or those of the publisher, the editors and the reviewers. Any product that may be evaluated in this article or claim that may be made by its manufacturer is not guaranteed or endorsed by the publisher.
Research integrity at Frontiers
Learn more about the work of our research integrity team to safeguard the quality of each article we publish.