- 1Natural Sciences Research Institute, College of Science, University of the Philippines Diliman, Quezon City, Philippines
- 2Institute of Biology, College of Science, University of the Philippines Diliman, Quezon City, Philippines
- 3Marine Science Institute, College of Science, University of the Philippines Diliman, Quezon City, Philippines
1 Introduction
Submarine groundwater discharges (SGDs) are conduits linking the marine and terrestrial environments allowing groundwater to flow from land into the ocean through continental margins (George et al., 2021). SGD-associated sites are recognized as biogeochemical hotspots, driven by diverse microorganisms that are influenced by fluctuating environmental conditions (Ruiz-González et al., 2021). Exploring these marine microorganisms is vital for understanding their ecological significance, contribution to environmental health (Adyasari et al., 2020), and potential biotechnological applications (Fenical, 2020; Shi et al., 2024).
In Mabini, Batangas, Philippines, SGD-influenced sites contained sedimentary rocks covered by microbial mats. Microbial mats are multi-layered and self-sustaining microbial communities typically thriving in aqueous environments (Rabus et al., 2015; Stal and Noffke, 2011). Mat-associated microbiota may play key roles in cycling essential elements such as carbon, hydrogen, nitrogen, oxygen, phosphorus, and sulfur and consequently, in ecosystem functioning in the marine environment (Glockner et al., 2012; Parkes et al., 2014). These marine microorganisms are potential sources of bioactive metabolites (Jensen et al., 2005; Magarvey et al., 2004), offering promising biotechnological, environmental, and pharmaceutical applications. Notable examples include Salinosporamide A and Didemnin B, which exhibit anticancer activity, Anthracimycin and Marinopyrrole A, identified as antibiotics, and Cyclomarin A, known for its anti-inflammatory properties (Fenical, 2020). A review by Shi et al. (2024) further underscores the diversity of bioactive compounds from marine microbes, highlighting their antibacterial, antiviral, antimalarial, anticancer, anti-inflammatory, and antibiofilm activities.
This data report presents a preliminary description of the diversity and functional profiles of marine microorganisms inhabiting the microbial mats of an SGD-influenced site in Mabini, Batangas, Philippines, as revealed through shotgun metagenomics. Using the metagenome data, metagenome-assembled genomes (MAGs) were recovered. Assembling genomes from metagenomic data, though putative, is a culture-independent approach that provides comprehensive and valuable insights into microbial diversity and functional potential (Wilkins et al., 2019). This approach enables the recovery of previously inaccessible genetic information from uncultivable microbes in SGD environments in the Philippines.
The paper presents the functional and structural metrics, identities, genes linked to nutrient metabolism, and BGCs of these MAGs. To the best of our knowledge, this is the first documentation of MAGs from a mat-associated microbial community in an SGD-influenced site in the Philippines. It addresses the dearth of data and knowledge on the microbial dimensions of SGD areas in the country and provides a baseline for future research using experimental or targeted approaches. Furthermore, investigating microbial mats in specific marine ecosystems can also offer valuable insights into climate change conditions, such as ocean acidification and warming (Mazière et al., 2023).
2 Methods
In July 2023, SCUBA divers collected microbial mats from sedimentary rocks in an SGD-influenced area in Acacia, Mabini, Batangas, Philippines, where small bubbles sparsely emerged between the rocks. These samples were taken from a depth of 8 to 12 meters, at approximate coordinates 13.727653° N and 120.883648° E. Microbial mats were carefully scraped off using a clean metal spatula and transferred into conical tubes underwater. Samples were then stored in a cooler with ice and transported to the Microbiological Research Services Laboratory, Natural Sciences Research Institute, at the University of the Philippines – Diliman, Quezon City. Upon arrival, the samples were stored in an ultra-low freezer (NuAire, USA) set at <-80°C until required for processing. The samples collected from the Acacia site were pooled for DNA extraction and processed as a single composite sample.
The total DNA was extracted from the microbial mats that were subsampled into four separate microcentrifuge tubes, each containing approximately 250 mg of the material. The extraction was carried out with the DNeasy PowerSoil® Pro Kit (QIAGEN, Netherlands), following the manufacturer’s protocol with minimal modifications. Lysis was performed individually on all four subsamples, and the resulting lysates were pooled to achieve a final DNA concentration of >100 ng/µL. All subsequent procedures were carried out according to the manufacturer’s protocol. The use of four subsamples was based on previous extractions from microbial mats where this approach consistently yielded sufficient DNA concentrations. DNA concentration was assessed using Denovix’s dsDNA Broad Range kit (DeNovix, USA) and fluorometer following the manufacturer’s protocol. The extracts were then sent to Macrogen, Inc. (South Korea) for shotgun metagenomic sequencing using the Illumina NovaSeq™ 6000 system with a throughput of 25 Gb at 150 bp paired-end setting.
Upon receipt of raw reads, the forward and reverse sequences underwent merging, trimming, assembly, and analysis, employing various bioinformatics tools, using KBase v2.7.11 (Arkin et al., 2018). The forward and reverse reads were interleaved during the importing stage and the interleaved reads were subsequently subjected to Trimmomatic v0.36 (Bolger et al., 2014) with a sliding window size of 4 trimming to a minimum quality of 30.
The Q30-trimmed reads were assembled using metaSPAdes v3.15.3 (Nurk et al., 2017; Prjibelski et al., 2020), MEGAHIT v.1.2.9 (Li et al., 2015), and IDBA-UD v.1.1.3 (Peng et al., 2012) with a minimum contig length of 2,000 bp. Quality Assessment Tool (QUAST) v.5.2.0 (Mikheenko et al., 2018) was used to evaluate the assemblies, and the detailed results can be found in Supplementary File S1. Among the three assemblies, metaSPAdes produced the longest assembly length at 250,173,012 bp, surpassing MEGAHIT by 60,524,281 bp and IDBA-UD by 132,767,228 bp, leading to its selection for assembling MAGs.
The metaSPAdes assembled reads were subjected to CONCOCT v1.1 (Alneberg et al., 2014), MaxBin2 v2.2.4 (Wu et al., 2016), and MetaBAT2 Contig Binning v1.7 (Kang et al., 2019) to cluster the assembled metagenomic sequences to putative genomes known as bins. All bins from the three different binning tools were optimized using the DAS tool v1.1.2, with DIAMOND as the gene identification tool (Sieber et al., 2018). The tool employed a score threshold of 0.5, a duplicate penalty of 0.6, and a megabin penalty of 0.5. The optimized bins were then filtered using CheckM v1.0.18 (Parks et al., 2015) to a completeness and contamination score of at least 90 and 5, respectively.
The filtered bins were extracted using MetagenomeUtils v1.1.1 (Arkin et al., 2018). Following extraction, these bins underwent taxonomic identification using the Genome Taxonomy Database (GTDB) toolkit v2.3.2 (Chaumeil et al., 2019). To further elucidate the relationships between the bins and other available GenBank genomes, a phylogenetic tree was reconstructed using SpeciesTreeBuilder v0.1.4 (Arkin et al., 2018). This tool leverages 49 clusters of orthologous groups to estimate relatedness and determine the nearest existing genomes to the bin set. The outgroup Thermoplasma acidophilum DSM 1728 (GCF 000195915.1), an archaean, was also included in the tree for reference.
All genomes were annotated using the Rapid Annotation using Subsystem Technology (RAST) through SEED Viewer v2.0 (Overbeek et al., 2013). The focus of this study was on genes related to nitrogen, phosphorus, potassium, iron acquisition and metabolism, and sulfur metabolism, as these pathways are crucial for growth, survival, and biogeochemical cycles.
Finally, biosynthetic gene clusters (BGCs) were identified using antiSMASH v7.0 (Blin et al., 2023) and PRISM 4 (Skinnider et al., 2020). This data report provides an initial overview of predicted biosynthetic gene clusters (BGCs) associated with putative microbes in a microbial mat influenced by submarine groundwater discharge (SGD) in the Philippines. The default settings of antiSMASH and PRISM 4 were used for BGC mining across all 17 MAGs. AntiSMASH was recognized to have a prediction accuracy of 97.7% (Medema et al., 2011) and PRISM 4 demonstrates a known prediction accuracy of 96% (Skinnider et al., 2020). All predicted BGCs were cataloged based on their types, regardless of their similarity scores with existing BGCs in antiSMASH and PRISM databases. This analysis was applied specifically to the bins to highlight the potential of these putative marine microorganisms as sources of various natural products. As this is a preliminary analysis, no experimental data confirming the expression of these BGCs is provided; the findings are intended solely as a baseline reference. The writing of this data report was improved with the aid of OpenAI's ChatGPT-4o.
3 Data and analysis
3.1 Data
3.1.1 Assembly characteristics of the 17 MAGs
3.1.1.1 Genome completeness and contamination
The assembly characteristics of the seventeen optimized and filtered bins extracted from the Q30-trimmed metaSPAdes assembly are presented in Figure 1A and Supplementary File S2. Extracted MAGs have CheckM completeness and contamination percentages of >90% and <5%, respectively following the standards on the minimum information about a metagenome-assembled genome (MIMAG) of Bowers et al. (2017). The analysis revealed that bins 017, 036, and 049 stand out with CheckM completeness scores exceeding 98%, with bin 049 achieving a completeness score of 100%. This indicates that these assembled genomes encompass a highly substantial portion of the marker genes required to define their positions within reference genomes (Parks et al., 2015). Moreover, bins 012, 035, and 039 exhibit 0% CheckM contamination, signifying minimal to no redundancy of marker genes, which are typically present as single copies within a genome (Parks et al., 2015). Additionally, Benchmarking Universal Single-Copy Orthologs (BUSCO) v5.4.6 (Simão et al., 2015) analysis revealed that 12 out of the 17 bins demonstrate completeness scores exceeding 90%, further affirming the quality of these genomic assemblies.
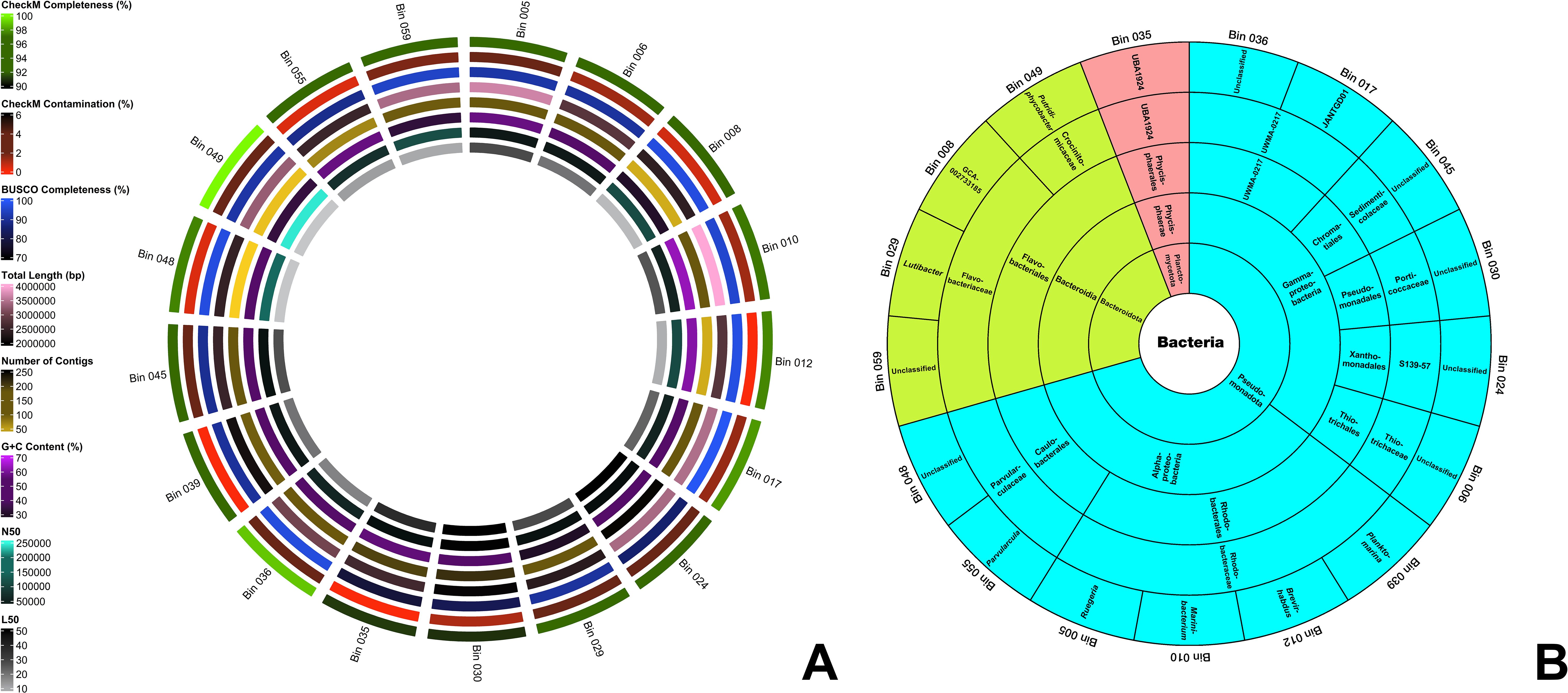
Figure 1. (A) Assembly features of the 17 MAGs. From the innermost to the outermost ring, the circles represent L50, N50, G+C content, the number of contigs, total genome length, BUSCO completeness, CheckM contamination, and CheckM completeness. (B) GTDB taxonomic classification of the 17 MAGs in a sunburst chart. The innermost circle indicates the domain, with subsequent rings displaying the phylum, class, order, family, and genus towards the outermost ring. MAGs shaded in blue belong to the phylum Pseudomonadota, those in green represent Bacteroidota, and those in red correspond to Planctomycetota.
3.1.1.2 Genome size, contigs, G+C content, N50, and L50
The genome size of the bins ranges from 2.0 Mbp to 4.0 Mbp. Bins 008, 012, 048, and 049 have less than 50 contigs, and bin 048 has the lowest number of contigs (21 contigs). All bins have an L50 value of less than 55. Bins 048 and 049 have an L50 value of 4 – the smallest L50 value. All bins have an N50 value of more than 10,000 and bin 049 has the highest N50 value (244,390). Ten out of 17 bins have more than 50% G+C content and bin 010 has the highest G+C content – 63.56%.
3.1.1.3 GTDB identities
The identities of the 17 MAGs are presented in Figure 1B. Through GTDB, all MAGs were determined to be under the Domain Bacteria and classified into three phyla, namely Pseudomonadota (12 bins), Bacteroidota (4 bins), and Planctomycetota (1 bin). The presence of three bacterial phyla—Pseudomonadota (syn. Proteobacteria), Bacteroidota (syn. Bacteroidetes), and Planctomycetota (syn. Planctomycetes)—was confirmed in the metagenome Q30-trimmed reads using both Kaiju v.1.3.4 (Menzel et al., 2016) and GOTTCHA2 v.0.0.7 (Freitas et al., 2015) analyses (Supplementary File S6). According to Kaiju, Pseudomonadota, Bacteroidota (part of the FCB group), and Planctomycetota (part of the PVC group) comprised approximately 68%, 16%, and 8% of the detected bacterial population, respectively. In contrast, GOTTCHA2 detected only Pseudomonadota and Bacteroidota, which were estimated to constitute 74% and 10% of the bacterial community in the metagenome reads, respectively. The assembled high-quality MAGs show a similar abundance ranking: Pseudomonadota, Bacteroidota, and Planctomycetota. Two classes were identified under the phylum Pseudomonadota, namely Alphaproteobacteria (6 bins) and Gammaproteobacteria (6 bins). All four bins under phylum Bacteroidota were all classified under class Bacteroidia. Bin 035 (UBA1924), the sole representative of the phylum Planctomycetota—the least abundant among the three phyla—was classified under the order Phycisphaerales. The identities of all MAGs up to the genus level are presented in Figure 1B. In Supplementary File S3, only bins 008 (GCA-002733185), 012 (Brevirhabdus sp.), 039 (Planktomarina sp.), and 049 (Putridiphycobacter sp.) were determined to have the closest placement ANI scores and the scores are 76.86, 78.25, 76.71, and 77.66, respectively. These ANI values indicate low similarity to their respective references (Jain et al., 2018). Such low ANI values, along with the absence of ANI placements for the other bins in the GTDB reference database (Supplementary File S3), suggest that these genomes may not have close representatives in GTDB. These observations may also highlight the potential novelty of these genomes, offering valuable insights into both characterized and yet-to-be-discovered microbes. It expands our understanding of microbial diversity in SGD ecosystems.
3.1.2 Phylogenetic insights and nutrient metabolism profiles of the 17 MAGs
A phylogenetic tree was reconstructed incorporating existing GenBank genome sequences. Additionally, genes involved in the metabolism of nitrogen, phosphorus, potassium, and sulfur and genes related to iron acquisition and metabolism were identified. The gene counts for all genomes are presented in Figure 2, while the specific roles in each nutrient metabolism are detailed in Supplementary File S4. Figure 2 was created in RStudio (RStudio Team, 2024) utilizing the ggplot (Wickham, 2016) and ggtree (Yu et al., 2016) packages.
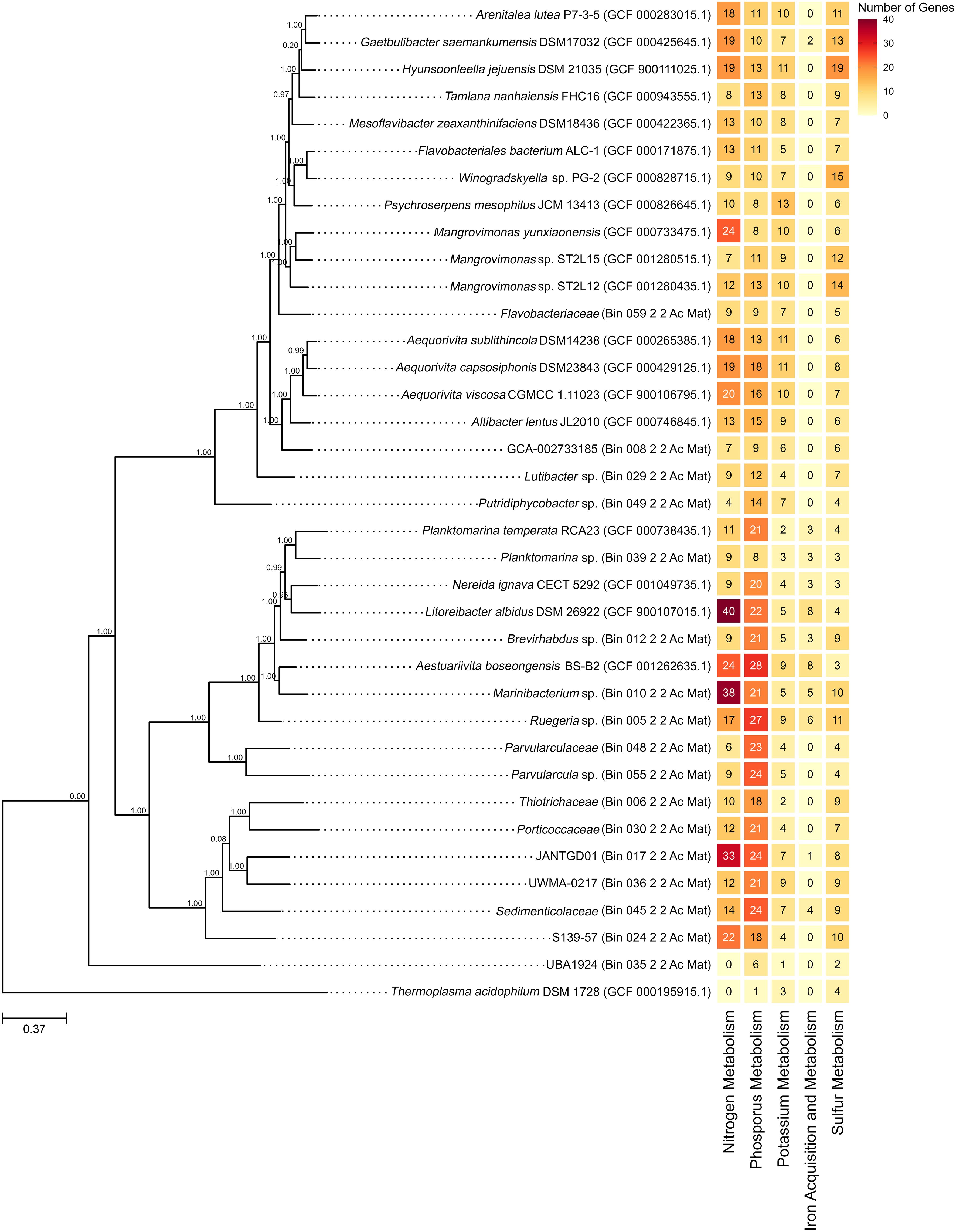
Figure 2. Reconstructed phylogenetic tree using SpeciesTreeBuilder with identified nutrient metabolism genes using RAST. The node values represent bootstrap support based on 1,000 replicates, while the scale bars indicate a genetic variation of 37% per unit length.
Among the 17 bins, 16 exhibited genes linked to nitrogen metabolism, with ammonia assimilation genes being the most prevalent among them (Supplementary File S4 Figure 1). Most GenBank genome sequences analyzed also contained genes related to nitrogen metabolism, with Litoreibacter albidus DSM 26922 exhibiting the highest number among them (Figure 2). L. albidus DSM 26922 formed a clade near bin 012 (Brevirhabdus sp.) with a bootstrap value of 1.0 and bin 039 (Planktomarina sp.) with a bootstrap value of 0.99 (Figure 2).
All bins contained genes associated with phosphorus metabolism, with the polyphosphate-related genes being the most prevalent (Supplementary File S4 Figure 2). Similarly, all GenBank genome sequences analyzed contained genes related to phosphorus metabolism (Supplementary File S4 Figure 2). Among these, Aestuariivita boseongensis BS-B2 had the most abundant phosphorus metabolism genes and formed a clade with bin 010 (Marinibacterium sp.) with a bootstrap value of 1.00 (Figure 2).
Genes related to potassium metabolism, specifically potassium homeostasis-related genes, were identified in all bins (Supplementary File S4 Figure 3). A similar trend was observed in all analyzed GenBank genomes (Supplementary File S4 Figure 3). Notably, Psychroserpens mesophilus JCM 13413 had the highest potassium metabolism genes (Figure 2). Phylogenetically, this genome was distant from other bins, with the closest bin being bin 059, identified within the family Flavobacteriaceae (Figure 2).
All bins demonstrated the presence of genes involved in sulfur metabolism, with thioredoxin-disulfide reductase genes being the most prevalent (Supplementary File S4 Figure 4). A similar pattern was seen in the GenBank genome sequences (Supplementary File S4 Figure 4). Hyunsoonleella jejuensis DSM 21035 had the highest number of sulfur metabolism genes and bin 059, under Flavobacteriaceae family, was determined to be the closest bin (Figure 2).
Only six bins were determined to possess genes associated with iron acquisition and metabolism (Figure 2). Of these, five bins exhibited iron acquisition genes similar to Streptococcus, showcasing its prevalence (Supplementary File S4 Figure 5). Similarly, only a few GenBank genome sequences exhibited genes associated with these functions (Supplementary File S4 Figure 5). Among these, L. albidus DSM 26922 and A. boseongensis BS-B2 exhibited the highest number of iron acquisition and metabolism-related genes, with bins 010 (Marinibacterium sp.), 012 (Brevirhabdus sp.), and 039 (Planktomarina sp.) identified to be the closest bin (Figure 2).
3.1.3 Detected biosynthetic gene clusters in the 17 MAGs
BGCs were identified through antiSMASH and PRISM, and detailed in Supplementary File S5. In antiSMASH, ribosomally synthesized and post-translationally modified peptides (RiPP)-like BGCs were prevalent, found in 11 of 17 bins. BGCs related to arylpolyene, betalactone, ectoine, heterocyst glycolipid synthase-like polyketide synthase (HgIE-KS), homoserine lactone, lassopeptide, non-ribosomal peptide synthetase (NRPS), NRPS-like, resorcinol, RRE-containing, type I polyketide synthase (T1PKS), terpene, and thiopeptide were also identified across various bins. Bin 005 had the highest number of BGCs, including betalactone, ectoine, homoserine lactone, RiPP-like, and terpene, while no BGCs were found in bin 048 (Parvularculaceae). PRISM detected polyketide BGCs in 12 of 17 bins, along with acyl homoserine lactone, ectoine, NRPs, lassopeptide, and class II/III bacteriocins. Bins 005 and 010 had the most BGCs, particularly for acyl homoserine and polyketides, with bin 005 also containing ectoine. No BGCs were found in bins 008, 029 (Lutibacter sp.), and 035 (UBA1924).
3.2 Analysis
All bins contained genes related to phosphorus, potassium, and sulfur metabolism, with most also possessing genes for nitrogen metabolism. However, only six bins had genes for iron metabolism and acquisition. These genes suggest a significant role for these microorganisms in cycling phosphorus, potassium, sulfur, nitrogen, and iron at the SGD-influenced site. Notably prevalent were genes involved in ammonia assimilation (nitrogen), polyphosphate (phosphorus), potassium homeostasis (potassium), thioredoxin-disulfide reductase (sulfur), and Streptococcus iron acquisition (iron acquisition and metabolism).
Ammonia assimilation is a pivotal component of the nitrogen cycle wherein ammonia is incorporated into organic compounds, that can be utilized by living organisms for survival and growth (Wright and Lehtovirta-Morley, 2023). Polyphosphate is a biopolymer implicated in cellular functions such as antibiotic resistance, biofilm formation, cell cycle control, energy storage, motility, stress response, and virulence (Akbari et al., 2021; Pokhrel et al., 2019). Potassium homeostasis is vital in adjusting membrane potential and electrical signaling, activating enzymes, maintaining pH levels, regulating osmotic pressure, and synthesizing proteins in bacteria (Stautz et al., 2021). The genes encoding potassium channels and transporters are essential, as they facilitate these critical functions. The bacterial enzyme thioredoxin-disulfide reductase is known to be involved in colonization, stress response, namely oxygen and disulfide stress, and virulence (Felix et al., 2021). Lastly, according to the study of Ge and Sun (2014), iron acquisition genes observed in Streptococcus are crucial for iron uptake, which are essential for activating oxygen, amino acid & nucleoside production, and electron transport that may impact the bacteria’s survival and virulence. The prevalence of the aforementioned genes may be influenced by the nutrients present in the water emitted by the SGD vents in Acacia, to which the microbial mats are continuously exposed.
The nutrient levels in the SGD vent water were analyzed, revealing the following average concentrations: 0.13 µM nitrite, 1.77 µM ammonium, 7.76 µM nitrate, 110.45 µM total dissolved nitrogen (TDN), 9.66 µM dissolved inorganic nitrogen (DIN), and 100.78 µM dissolved organic nitrogen (DON). For phosphorus compounds, the average concentrations were 0.04 µM phosphate, 0.29 µM total dissolved phosphorus (TDP), and 0.25 µM dissolved organic phosphorus (DOP). Additionally, an iron concentration of 0.03 ppm was detected. The nutrient data are also detailed in Supplementary File S7.
The varying levels of different nitrogen forms in the SGD vent water may indicate an environment where nitrogen cycling and various nitrogen-utilization pathways are crucial for the survival of the putative microbes in the microbial mats. This observation aligns with the detection of diverse nitrogen-metabolism genes across the MAGs. Additionally, the relatively low average concentrations of both phosphorus and iron may explain the prevalence of polyphosphate-related and iron acquisition-related genes among the MAGs. These genes enable microbes to store phosphate as polyphosphate, which can later serve as an energy source—an advantageous trait in environments with limited phosphate availability (Achbergerová and Nahálka, 2011). The ability to concentrate and store nutrients within the matrix is critical, as these microbes are anchored to surfaces, like rocks, and cannot relocate to nutrient-rich areas. Similarly, the presence of iron acquisition genes, similar to those found in Streptococcus species, suggests adaptation to environments with restricted free iron. These genes are known to be utilized by Streptococcus in low-iron conditions (Ge and Sun, 2014).
Based on the obtained nutrient and genomic data, the prevalence of specific genes, such as those involved in polyphosphate and iron acquisition, appears to correlate with the phosphorus- and iron-limited conditions of the SGD vent water. These genes, along with those associated with potassium homeostasis and thioredoxin-disulfide, supported by their known roles in growth and survival, are likely essential for the putative marine microbes to thrive in an SGD-influenced area. Additionally, the presence of various nitrogen metabolism genes across all MAGS suggests that these putative microbes may play a role in nitrogen cycling, supporting other marine life (Hunter-Cevera et al., 2005) and contributing to biogeochemical processes in this environment.
The GenBank genomes with the highest number of nutrient metabolism genes identified in the phylogenetic tree (Figure 2) belong to the families Flavobacteriaceae—including H. jejuensis DSM 21035, G. saemankumensis DSM 17032, and P. mesophilus JCM 13413—and Roseobacteraceae—including A. boseongensis BS-B2, L. albidus DSM 26922, N. ignava CECT 5292, and P. temperata RCA23. Both families are well-known for thriving in marine environments and playing critical roles in ocean nutrient cycling (Gavriilidou et al., 2020; Pujalte et al., 2014; Riedel et al., 2013).
In Figure 2, bins 010 (Marinibacterium sp.) and 039 (Planktomarina sp.) formed monophyletic clades with A. boseongensis BS-B2 (Park et al., 2014) and P. temperata RCA23 (Giebel et al., 2013), respectively, each with a bootstrap value of 1.00. Notably, bin 10 and A. boseongensis BS-B2, both under Rhodobacteraceae, share similar genome sizes (~4.0 Mbp and ~3.9 Mbp, respectively) and G+C content of 62.2% and 63.6%, respectively (Park et al., 2014; Figure 1B; Supplementary File S2). Similarly, bin 39 and P. temperata RCA23 share the same genus, with genome sizes of ~2.2 Mbp and ~3.3 Mbp, and G+C content of 52.0% and 53.5%, respectively (Giebel et al., 2013; Figure 1B; Supplementary File S2).
The high bootstrap values for these bins indicate a significant genomic similarity with the referenced GenBank genomes. Given these families are involved in ocean nutrient cycling, it is likely that bins 010 and 039 also contribute to nutrient cycling processes within the SGD-influenced environment of Acacia, Mabini, Batangas.
In addition to nutrient metabolism genes, BGCs were also identified in the majority of the MAGs. BGCs are groups of two or more neighboring genes that are encoded together to produce specific secondary metabolites (Medema et al., 2015). RiPP-like BGCs were determined to be the most prevalent class detected in the bins. This class, previously recognized as bacteriocin-encoding genes (Blin et al., 2021), has been extensively studied in relation to their anticancer, antibiotics, and biopreservative potential (Negash and Tsehai, 2020; Thapar and Salooja, 2023). In PRISM, BGCs associated with polyketides stood out to be the most abundant BGC among the assembled genomes. Polyketides are known to have promising applications in the field of medicine, such as antibiotics, immunosuppressants, and anticancer agents (Sanchez and Demain, 2011; Zhang and Liu, 2016), and biotechnology, such as hydrocarbon biofuels (Gayen, 2022). In an ecological context, the presence or prevalence of these types of BGCs likely represents a survival strategy by producing secondary metabolites that inhibit the growth of rival microorganisms, as highlighted by Chen et al. (2020). This prevalence may also be associated with adaptation to nutrient-limited environments, where competition for resources drives the need for such defensive mechanisms.
The identified BGCs in the MAGs not only provide a competitive advantage to the putative marine microbes but also are known to exhibit several health-related benefits, such as antimicrobial activity and cytotoxic properties (Kwon and Hovde, 2024). Although these microbes are often unculturable, BGCs could be harnessed for metabolite production using a molecular approach. For instance, BGCs can be synthesized and expressed in culturable hosts (Lin et al., 2020), potentially generating not only the intended metabolites but also novel variants. Nguyen et al. (2022) demonstrated this by co-expressing RiPP BGCs in Escherichia coli, yielding diverse metabolite forms. Such strategies can be applied to the BGCs identified in MAGs from SGD microbial mats in Acacia, Mabini, Batangas, opening avenues for future discoveries in natural products and synthetic biology, with promising implications for advancements in medicine and biotechnology.
Data availability statement
The datasets presented in this study can be found in online repositories. The names of the repository/repositories and accession number(s) can be found below: https://www.ncbi.nlm.nih.gov/bioproject/1111281 https://zenodo.org/records/14177018.
Author contributions
JV: Formal analysis, Project administration, Visualization, Writing – original draft, Writing – review & editing. PG: Conceptualization, Investigation, Methodology, Project administration, Writing – review & editing. LM: Conceptualization, Investigation, Methodology, Project administration, Writing – review & editing. AE: Investigation, Writing – review & editing. MS: Conceptualization, Funding acquisition, Methodology, Project administration, Resources, Supervision, Writing – review & editing.
Funding
The author(s) declare that financial support was received for the research, authorship, and/or publication of this article. The study is an output of the SGD Project 4: Probing Microbial Diversity in Submarine Groundwater Discharge (SGD) Areas under the program Biodiversity and Resilience of Coral Reefs and Associated Ecosystems in Submarine Groundwater Discharge Areas (BioRe CoARE SGD). The program was funded by the Department of Science and Technology-Philippine Council for Agriculture, Aquatic and Natural Resources Research and Development (DOST-PCAARRD).
Acknowledgments
The team extends its gratitude to Mishel Valery V. Rañada for her role as a SCUBA diver in collecting the samples featured in this report. The writing of this data report was improved with the aid of OpenAI's ChatGPT-4o.
Conflict of interest
The authors declare that the research was conducted in the absence of any commercial or financial relationships that could be construed as a potential conflict of interest.
Publisher’s note
All claims expressed in this article are solely those of the authors and do not necessarily represent those of their affiliated organizations, or those of the publisher, the editors and the reviewers. Any product that may be evaluated in this article, or claim that may be made by its manufacturer, is not guaranteed or endorsed by the publisher.
Supplementary material
The Supplementary Material for this article can be found online at: https://www.frontiersin.org/articles/10.3389/fmars.2024.1500350/full#supplementary-material
References
Achbergerová L., Nahálka J. (2011). Polyphosphate - an ancient energy source and active metabolic regulator. Microbial Cell Factories 10, 63. doi: 10.1186/1475-2859-10-63
Adyasari D., Hassenrück C., Montiel D., Dimova N. (2020). Microbial community composition across a coastal hydrological system affected by submarine groundwater discharge (SGD). PloS One 15. doi: 10.1371/journal.pone.0235235
Akbari A., Wang Z., He P., Wang D., Lee J., Han I., et al. (2021). Unrevealed roles of polyphosphate-accumulating microorganisms. Microbial Biotechnol. 14, 82–87. doi: 10.1111/1751-7915.13730
Alneberg J., Bjarnason B. S., de Bruijn I., Schirmer M., Quick J., Ijaz U. Z., et al. (2014). Binning metagenomic contigs by coverage and composition. Nat. Methods 11, 1144–1146. doi: 10.1038/nmeth.3103
Arkin A. P., Cottingham R. W., Henry C. S., Harris N. L., Stevens R. L., Maslov S., et al. (2018). KBase: the United States department of energy systems biology knowledgebase. Nat. Biotechnol. 36, 566–569. doi: 10.1038/nbt.4163
Blin K., Shaw S., Augustijn H. E., Reitz Z. L., Biermann F., Alanjary M., et al. (2023). Antismash 7.0: New and improved predictions for detection, regulation, chemical structures and visualisation. Nucleic Acids Res. 51. doi: 10.1093/nar/gkad344
Blin K., Shaw S., Kloosterman A. M., Charlop-Powers Z., van Wezel G. P., Medema M. H., et al. (2021). AntiSMASH 6.0: Improving cluster detection and comparison capabilities. Nucleic Acids Res. 49. doi: 10.1093/nar/gkab335
Bolger A. M., Lohse M., Usadel B. (2014). Trimmomatic: A flexible trimmer for Illumina sequence data. Bioinformatics 30, 2114–2120. doi: 10.1093/bioinformatics/btu170
Bowers R. M., Kyrpides N. C., Stepanauskas R., Harmon-Smith M., Doud D., Reddy T. B., et al. (2017). Minimum information about a single amplified genome (MISAG) and a metagenome-assembled genome (MIMAG) of bacteria and Archaea. Nat. Biotechnol. 35, 725–731. doi: 10.1038/nbt.3893
Chaumeil PA, Mussig AJ, Hugenholtz P, Parks DH. (2019). GTDB-TK: A toolkit to classify genomes with the Genome Taxonomy Database. Bioinformatics, 36(6), 1925–1927. doi: 10.1093/bioinformatics/btz848
Chen R., Wong H. L., Kindler G. S., MacLeod F. I., Benaud N., Ferrari B. C., et al. (2020). Discovery of an abundance of biosynthetic gene clusters in Shark Bay Microbial mats. Front. Microbiol. 11. doi: 10.3389/fmicb.2020.01950
Felix L., Mylonakis E., Fuchs B. B. (2021). Thioredoxin reductase is a valid target for antimicrobial therapeutic development against gram-positive bacteria. Front. Microbiol. 12. doi: 10.3389/fmicb.2021.663481
Fenical W. (2020). Marine Microbial Natural Products: The evolution of a new field of science. J. Antibiotics 73, 481–487. doi: 10.1038/s41429-020-0331-4
Freitas T. A. K., Li P.-E., Scholz M. B., Chain P. S. G. (2015). Accurate read-based metagenome characterization using a hierarchical suite of unique signatures. Nucleic Acids Res. 43. doi: 10.1093/nar/gkv180
Gavriilidou A., Gutleben J., Versluis D., Forgiarini F., van Passel M. W., Ingham C. J., et al. (2020). Comparative genomic analysis of Flavobacteriaceae: Insights into carbohydrate metabolism, gliding motility and secondary metabolite biosynthesis. BMC Genomics 21. doi: 10.1186/s12864-020-06971-7
Gayen K. (2022). Metabolic Engineering Approaches for high-yield hydrocarbon biofuels. Hydrocarbon Biorefinery, 253–270. doi: 10.1016/b978-0-12-823306-1.00005-4
Ge R., Sun X. (2014). Iron acquisition and regulation systems in Streptococcus species. Metallomics 6, 996. doi: 10.1039/c4mt00011k
George M. E., Akhil T., Remya R., Rafeeque M. K., Suresh Babu D. S. (2021). Submarine groundwater discharge and associated nutrient flux from southwest coast of India. Mar. pollut. Bull. 162, 111767. doi: 10.1016/j.marpolbul.2020.111767
Giebel H.-A., Kalhoefer D., Gahl-Janssen R., Choo Y.-J., Lee K., Cho J.-C., et al. (2013). Planktomarina temperata gen. nov., sp. nov., belonging to the globally distributed RCA Cluster of the marine Roseobacter clade, isolated from the German wadden sea. Int. J. Systematic Evolutionary Microbiol. 63, 4207–4217. doi: 10.1099/ijs.0.053249-0
Glockner F. O., Stal L. J., Sandaa R.-A., Gasol J. M., O’Gara F., Hernandez F., et al. (2012). “Marine microbial diversity and its role in ecosystem functioning and environmental change,” in Marine Board Position Paper 17. Eds. Claewaert J. B., McDonough N. (Marine Board-ESF, Ostend, Belgium).
Hunter-Cevera J., Karl D., Buckley M. (2005). Marine microbial diversity: The key to Earth's habitability (based on a colloquium sponsored by the American Academy of Microbiology, held April 8–10, 2005, in San Francisco, California). Washington, DC: American Society for Microbiology. Retrieved from https://www.ncbi.nlm.nih.gov/books/NBK559439/
Jain C., Rodriguez-R L. M., Phillippy A. M., Konstantinidis K. T., Aluru S. (2018). High throughput ANI analysis of 90k prokaryotic genomes reveals clear species boundaries. Nat. Commun. 9. doi: 10.1038/s41467-018-07641-9
Jensen P. R., Gontang E., Mafnas C., Mincer T. J., Fenical W. (2005). Culturable Marine Actinomycete diversity from tropical Pacific Ocean sediments. Environ. Microbiol. 7, 1039–1048. doi: 10.1111/j.1462-2920.2005.00785.x
Kang D. D., Li F., Kirton E., Thomas A., Egan R., An H., et al. (2019). MetaBAT 2: An adaptive binning algorithm for robust and efficient genome reconstruction from Metagenome Assemblies. PeerJ 7. doi: 10.7717/peerj.7359
Kwon T., Hovde B. T. (2024). Global characterization of biosynthetic gene clusters in non-model eukaryotes using domain architectures. Sci. Rep. 14. doi: 10.1038/s41598-023-50095-3
Li D., Liu C.-M., Luo R., Sadakane K., Lam T.-W. (2015). MEGAHIT: An ultra-fast single-node solution for large and complex metagenomics assembly via succinct de bruijn graph. Bioinformatics 31, 1674–1676. doi: 10.1093/bioinformatics/btv033
Lin Z., Nielsen J., Liu Z. (2020). Bioprospecting through cloning of whole natural product biosynthetic gene clusters. Front. Bioengineering Biotechnol. 8. doi: 10.3389/fbioe.2020.00526
Magarvey N. A., Keller J. M., Bernan V., Dworkin M., Sherman D. H. (2004). Isolation and characterization of novel marine-derived actinomycete taxa rich in bioactive metabolites. Appl. Environ. Microbiol. 70, 7520–7529. doi: 10.1128/aem.70.12.7520-7529.2004
Mazière C., Duran R., Dupuy C., Cravo-Laureau C. (2023). Microbial Mats as model to decipher climate change effect on microbial communities through a mesocosm study. Front. Microbiol. 14. doi: 10.3389/fmicb.2023.1039658
Medema M. H., Blin K., Cimermancic P., de Jager V., Zakrzewski P., Fischbach M. A., et al. (2011). antiSMASH: Rapid Identification, annotation and analysis of secondary metabolite biosynthesis gene clusters in bacterial and fungal genome sequences. Nucleic Acids Res. 39. doi: 10.1093/nar/gkr466
Medema M. H., Kottmann R., Yilmaz P., Cummings M., Biggins J. B., Blin K., et al. (2015). Minimum information about a biosynthetic gene cluster. Nat. Chem. Biol. 11, 625–631. doi: 10.1038/nchembio.1890
Menzel P., Ng K. L., Krogh A. (2016). Fast and sensitive taxonomic classification for metagenomics with Kaiju. Nat. Commun. 7. doi: 10.1038/ncomms11257
Mikheenko A., Prjibelski A., Saveliev V., Antipov D., Gurevich A. (2018). Versatile genome assembly evaluation with quast-LG. Bioinformatics 34, i142–i150. doi: 10.1093/bioinformatics/bty266
Negash A. W., Tsehai B. A. (2020). Current applications of bacteriocin. Int. J. Microbiol., 1–7. doi: 10.1155/2020/4374891
Nguyen N. A., Cong Y., Hurrell R. C., Arias N., Garg N., Puri A. W., et al. (2022). A silent biosynthetic gene cluster from a methanotrophic bacterium potentiates discovery of a substrate promiscuous proteusin cyclodehydratase. ACS Chem. Biol. 17, 1577–1585. doi: 10.1021/acschembio.2c00251
Nurk S., Meleshko D., Korobeynikov A., Pevzner P. A. (2017). metaSPAdes: A new versatile metagenomic assembler. Genome Res. 27, 824–834. doi: 10.1101/gr.213959.116
Overbeek R., Olson R., Pusch G. D., Olsen G. J., Davis J. J., Disz T., et al. (2013). The seed and the rapid annotation of microbial genomes using subsystems technology (RAST). Nucleic Acids Res. 42. doi: 10.1093/nar/gkt1226
Park S., Won S.-M., Kim H., Park D.-S., Yoon J.-H. (2014). Aestuariivita boseongensis gen. Nov., sp. nov., isolated from a tidal flat sediment. Int. J. Systematic Evolutionary Microbiol. 64, 2969–2974. doi: 10.1099/ijs.0.062406-0
Parkes R. J., Cragg B., Roussel E., Webster G., Weightman A., Sass H. (2014). A review of prokaryotic populations and processes in sub-seafloor sediments, including biosphere:geosphere interactions. Mar. Geol 352, 409–425. doi: 10.1016/j.margeo.2014.02.009
Parks D. H., Imelfort M., Skennerton C. T., Hugenholtz P., Tyson G. W. (2015). CheckM: Assessing the quality of microbial genomes recovered from isolates, single cells, and Metagenomes. Genome Res. 25, 1043–1055. doi: 10.1101/gr.186072.114
Peng Y., Leung H. C., Yiu S. M., Chin F. Y. (2012). IDBA-Ud: A de novo assembler for single-cell and metagenomic sequencing data with highly uneven depth. Bioinformatics 28, 1420–1428. doi: 10.1093/bioinformatics/bts174
Pokhrel A., Lingo J. C., Wolschendorf F., Gray M. J. (2019). Assaying for inorganic polyphosphate in bacteria. J. Visualized Experiments 143). doi: 10.3791/58818
Prjibelski A., Antipov D., Meleshko D., Lapidus A., Korobeynikov A. (2020). Using SPAdes de novo assembler. Curr. Protoc. Bioinf. 70. doi: 10.1002/cpbi.102
Pujalte M. J., Lucena T., Ruvira M. A., Arahal D. R., Macián M. C. (2014). The family rhodobacteraceae. Prokaryotes, 439–512. doi: 10.1007/978-3-642-30197-1_377
Rabus R., Venceslau S. S., Wöhlbrand L., Voordouw G., Wall J. D., Pereira I. A. C. (2015). A post-genomic view of the ecophysiology, catabolism and biotechnological relevance of sulphate-reducing prokaryotes. Adv. Microbial Physiol., 55–321. doi: 10.1016/bs.ampbs.2015.05.002
Riedel T., Fiebig A., Petersen J., Gronow S., Kyrpides N. C., Göker M., et al. (2013). Genome sequence of the Litoreibacter arenae Type Strain (DSM 19593T), a member of the Roseobacter clade isolated from Sea Sand. Standards Genomic Sci. 9, 117–127. doi: 10.4056/sigs.4258318
RStudio Team. (2024). RStudio: Integrated development environment for R. RStudio, PBC. Available at: https://www.rstudio.com
Ruiz-González C., Rodellas V., Garcia-Orellana J. (2021). The microbial dimension of submarine groundwater discharge: Current challenges and Future Directions. FEMS Microbiol. Rev. 45. doi: 10.1093/femsre/fuab010
Sanchez S., Demain A. L. (2011). Secondary metabolites. Compr. Biotechnol., 155–167. doi: 10.1016/b978-0-08-088504-9.00018-0
Shi T., Wang B., Zhao D.-L., Newman D. J. (2024). Editorial: The Discovery, identification and application of marine microorganisms derived natural products. Front. Mar. Sci. 11. doi: 10.3389/fmars.2024.1366379
Sieber C. M., Probst A. J., Sharrar A., Thomas B. C., Hess M., Tringe S. G., et al. (2018). Recovery of genomes from metagenomes via a dereplication, aggregation and scoring strategy. Nat. Microbiol. 3, 836–843. doi: 10.1038/s41564-018-0171-1
Simão F. A., Waterhouse R. M., Ioannidis P., Kriventseva E. V., Zdobnov E. M. (2015). Busco: Assessing genome assembly and annotation completeness with single-copy orthologs. Bioinformatics 31, 3210–3212. doi: 10.1093/bioinformatics/btv351
Skinnider M. A., Johnston C. W., Gunabalasingam M., Merwin N. J., Kieliszek A. M., MacLellan R. J., et al. (2020). Comprehensive prediction of secondary metabolite structure and biological activity from Microbial Genome sequences. Nat. Commun. 11. doi: 10.1038/s41467-020-19986-1
Stal L. J., Noffke N. (2011). Microbial mats. Encyclopedia Astrobiol, 1042–1045. doi: 10.1007/978-3-642-11274-4_986
Stautz J., Hellmich Y., Fuss M. F., Silberberg J. M., Devlin J. R., Stockbridge R. B., et al. (2021). Molecular mechanisms for bacterial potassium homeostasis. J. Mol. Biol. 433, 166968. doi: 10.1016/j.jmb.2021.166968
Thapar P., Salooja M. K. (2023). Bacteriocins: Applications in food preservation and therapeutics. Lactobacillus A Multifunctional Genus. doi: 10.5772/intechopen.106871
Wickham, H H (2016). ggplot2: Elegant Graphics for Data Analysis. Springer-Verlag New York. ISBN 978-3-319-24277-4, Available at: https://ggplot2.tidyverse.org
Wilkins L. G., Ettinger C. L., Jospin G., Eisen J. A. (2019). Metagenome-assembled genomes provide new insight into the microbial diversity of two thermal pools in Kamchatka, Russia. Sci. Rep. 9. doi: 10.1038/s41598-019-39576-6
Wright C. L., Lehtovirta-Morley L. E. (2023). Nitrification and beyond: Metabolic versatility of ammonia oxidising archaea. ISME J. 17, 1358–1368. doi: 10.1038/s41396-023-01467-0
Wu Y.-W., Simmons B. A., Singer S. W. (2016). MaxBin 2.0: An automated binning algorithm to recover genomes from multiple metagenomic datasets. Bioinformatics 32, 605–607. doi: 10.1093/bioinformatics/btv638
Yu G, Smith DK, Zhu H, Guan Y, Lam TT (2016). ggtree: An R package for visualization and annotation of phylogenetic trees with their covariates and other associated data. Methods in Ecology and Evolution, 8(1), 28–36. doi: 10.1111/2041-210x.12628
Keywords: biosynthetic gene clusters, metagenome-assembled genomes, microbial mats, shotgun sequencing, submarine groundwater discharge
Citation: Veluz JT, Gloria PCT, Mallari LAN, Enova AER and Siringan MAT (2025) MAGnificent microbes: metagenome-assembled genomes of marine microorganisms in mats from a Submarine Groundwater Discharge Site in Mabini, Batangas, Philippines. Front. Mar. Sci. 11:1500350. doi: 10.3389/fmars.2024.1500350
Received: 23 September 2024; Accepted: 12 December 2024;
Published: 06 January 2025.
Edited by:
Marialetizia Palomba, University of Tuscia, ItalyReviewed by:
Marinella Silva Laport, Federal University of Rio de Janeiro, BrazilBárbara Muñoz Palazón, University of Granada, Spain
Copyright © 2025 Veluz, Gloria, Mallari, Enova and Siringan. This is an open-access article distributed under the terms of the Creative Commons Attribution License (CC BY). The use, distribution or reproduction in other forums is permitted, provided the original author(s) and the copyright owner(s) are credited and that the original publication in this journal is cited, in accordance with accepted academic practice. No use, distribution or reproduction is permitted which does not comply with these terms.
*Correspondence: Joshua T. Veluz, anR2ZWx1ekB1cC5lZHUucGg=