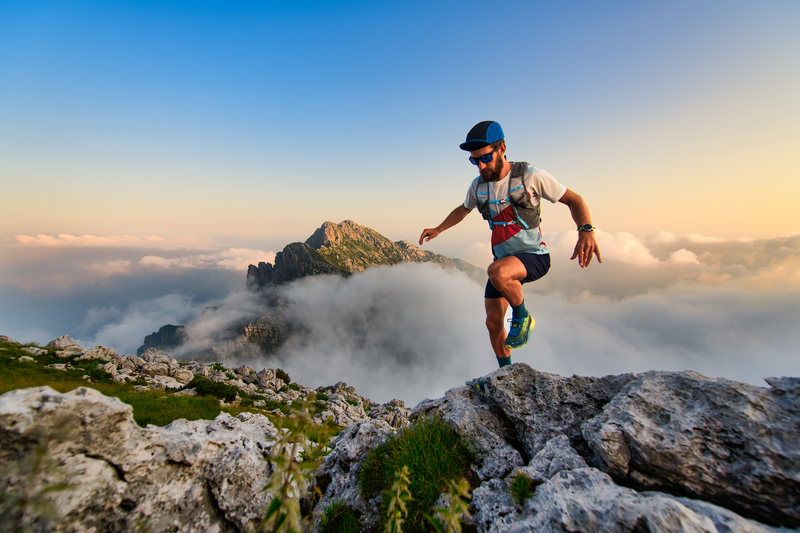
94% of researchers rate our articles as excellent or good
Learn more about the work of our research integrity team to safeguard the quality of each article we publish.
Find out more
ORIGINAL RESEARCH article
Front. Mar. Sci. , 11 December 2024
Sec. Ocean Solutions
Volume 11 - 2024 | https://doi.org/10.3389/fmars.2024.1499231
This article is part of the Research Topic Data-Driven Ocean Environmental Perception with its Applications View all 9 articles
Escort operation is an effective mean to ensure the safety of ship navigation in the Arctic ice area and expand the window period for ship navigation. At the same time, the operation mode between icebreaker and escorted ship may also causes collision accident. In order to scientifically reflect the complex coupling relationship in the escort operation system in Arctic waters and effectively manage the navigation risks. This study proposes to use the functional resonance analysis method (FRAM) to identify the risk factors of ship escort operation in Arctic waters, and uses the Bayesian network (BN) method to establish a risk assessment model for escort operation collision accident. The cloud model is used to process the uncertain data information. The proposed method is applied during the actual escort operation of a commercial ship on the Arctic Northeast Passage. According to the model simulation results, the risk performance of ship escort operation in Arctic waters is quantitatively analyzed, and the key risk causes are further analyzed. This study has positive significance for better understanding the risk evolution mechanism of ship escort operation in Arctic ice area and helping relevant management departments to take risk control measures.
The shipping industry undertakes the transportation of most commodities in the world’s foreign trade and has made great contributions to the industrial development of countries around the world (Otheitis and Kunc, 2015; Lenzen et al., 2023). In recent years, people have been seeking more economical and convenient ways of maritime transportation. The opening of the Arctic route has brought new opportunities for the development of the world’s shipping industry (Ryan et al., 2021). The Arctic route has greatly shortened the transportation distance from Asia to Europe, more and more commercial ships have begun to try to use the Arctic route for ocean-going cargo transportation. Due to the influence of extreme natural phenomena such as sea ice and strong winds in Arctic waters, there are great safety issues in conducting commercial navigation in Arctic waters. In order to ensure the safety of ships in Arctic waters, most ships will choose icebreaking escort services during navigation in the Arctic ice area. Under the leadership of icebreakers, ordinary commercial ships pass through the ice area by following, which greatly improves the navigation safety level of ships in the Arctic ice area (Zhang et al., 2017). However, in this operation mode, how to effectively avoid collisions between icebreaker and escorted ship becomes the key to escort operation. Therefore, it is necessary to introduce new theories and methods to scientifically analyze and manage the collision accident risk during escort operation in Arctic waters.
For the escort operation in Arctic waters, it consists of a series of operational tasks, and there exists a complex spatial and temporal correlation between different tasks, which is a typical complex system. Process safety is a commonly used safety analysis method in the industrial field. It emphasizes that the occurrence of a dangerous event will not only affect the current situation, but also the safety of the next link and subsequent processes. It is often used to analyze systemic risks (Amin et al., 2019; Behari, 2019). When quantitatively analyzing the risk of collision accident in ship escort operation in Arctic waters, it is necessary to combine the analytical ideas of process safety, introduce relevant complex system theoretical methods, characterize the complex coupling relationship in the ship escort operation system in Arctic waters, and clarify the law of changes in the navigation risks of ships during escort operations.
In order to scientifically analyze the complex coupling mechanism and risk quantification characteristics of ship escort operation in Arctic waters, this study proposes to combine the functional resonance analysis method (FRAM) with the Bayesian network (BN) to establish a quantitative analysis model for the collision accident risk of ship escort operation in Arctic waters, and adopts the cloud model (CM) for uncertain information processing to quantitatively analyze the risk of escort operation in specific scenarios.
This study is organized as follows. Section 2 analyzes and summarizes the current research status. Section 3 describes in detail the proposed method for quantitative analysis of collision accident risks of ship escort operation in Arctic waters. Section 4 applies the proposed method in combination with the specific scenario of ship escort operation in Arctic waters. Section 5 discusses the methods and results proposed in this study. Section 6 is the conclusion of this study.
As seasonal navigation in Arctic waters becomes a reality, the issue of ship navigation risks has received a lot of attention (Khan et al., 2020; Yao et al., 2024; Kandel and Baroud, 2024). In order to effectively expand the navigation window in Arctic waters, icebreaker escort operation has become a common mean (Moe and Brigham, 2017; Zhang et al., 2018). Relevant studies on the ship escort operation risks in Arctic waters has continued to increase. Zhang et al. (2019a) analyzed the safety risks of ship formation operations from the perspective of safe distance and speed in the multi-ship following mode of escort operations in Arctic ice area. Fu et al. (2022) combined failure mode and effects analysis (FMEA) and FRAM to simulate the evolution of ship traffic accident scenarios in Arctic waters, and further evaluated the changes in the probability of navigation risks of nuclear-powered icebreakers in Arctic waters under independent navigation and escort operation modes. Xu and Kim (2023) established a hybrid causal logic model for ship–ship and ship–ice collision accidents in Arctic waters and conducted a quantitative evaluation of the risks of collision accidents. Xu et al. (2023) used ship networking technology to develop an intelligent micro-model to analyze the stability of ship formations based on the movement trend and sea ice conditions of ship formations in Arctic waters to ensure the safety of ship formation operations in Arctic waters. Zhu et al. (2024) identified the risk factors affecting ship escort operations in Arctic waters from the perspective of complex system theory, and combined the BN analysis method to predict and analyze the risk characterization of escort operations. According to the current research status, compared with the study of navigation risk under the state of independent navigation of ships, escort operations need to consider traffic accidents between ship formations under the coordinated navigation of multiple ships. Due to the complexity of the task of escort operations in Arctic waters, in the research related to the risk of escort operations in Arctic waters, relevant methods suitable for complex system analysis are often used to analyze the causes of accidents (Fu et al., 2022; Zhu et al., 2024).
When analyzing the risk of ship navigation, a combination of qualitative and quantitative analysis is often used. FRAM can effectively explain the deep logic of complex system accidents by analyzing system functions and focusing on the changes and coupling relationships of system functions when accidents occur, and is widely used in risk factor identification and correlation analysis (Tian et al., 2016; Li et al., 2019; Yousefi et al., 2019; Ma et al., 2023). França and Hollnagel (2023) used FRAM to model and analyze human factors in accidents when analyzing production accidents in the industrial field, and analyzed the main human factors affecting process safety. Liu et al. (2024a) combined FRAM and reinforcement learning to construct an emergency plan for blowout accidents, in which FRAM was used to simulate the emergency response process. Yu et al. (2024) analyzed the functions related to maritime accidents based on the maritime accident investigation report for grounding accidents in Arctic waters, and constructed a FRAM model to analyze the functional resonance of accidents. Zheng et al. (2024) designed a FRAM model consisting of four stages: understanding, designing, analyzing, and enhancing the response process, to provide a decision-making basis for emergency response to fuel storage accidents.
Ship navigation operations are a complex social system. Water traffic accidents have the characteristics of low probability and serious consequences, and the causes of accidents are often difficult to clarify. FRAM is suitable for analyzing the causes of complex system accidents and has been widely used in the study of water traffic accident risk analysis (Lee et al., 2020; Salihoglu and Besikci, 2021). The Arctic waters are well known for their harsh navigation environment (Abbassi et al., 2017; Yao et al., 2022; Li et al., 2023b). Ship escort operations increase the complexity of operational tasks in such harsh environments. FRAM can help analyze the occurrence process of escort operations in Arctic waters and clarify the complex interaction relationship between system modules when accidents occur.
The BN analysis method can effectively integrate various risk factors and carry out BN reasoning by combining various subjective and objective data information. It is widely used in the quantitative reasoning of ship navigation risks (Baksh et al., 2018; Zhang et al., 2019; Zhang et al., 2019b; Xu and Kim, 2023; Li et al., 2024). BN analysis methods have also been widely used in the quantitative analysis of ship navigation risks in Arctic waters. Vanhatalo et al. (2021) combined AIS data, satellite data, and accident data to predict the probability risk of ship ice entrapment accidents in the Arctic ice zone using the BN modeling method. Wang et al. (2022) collected relevant data sets and conducted a quantitative analysis of environmental risks on the Arctic Northwest Passage based on the establishment of a dynamic BN risk assessment model. Fu et al. (2023) used an object-oriented Bayesian network (OOBN) analysis method to quantitatively analyze the risks of multi-type ship traffic accidents in the Arctic ice area. Afenyo et al. (2023) proposed a hybrid method based on the Bayesian loss function to evaluate the losses caused by oil spill accidents in Arctic waters.Liu et al. (2024b) quantitatively analyzed the propagation mechanism of the Arctic navigation network under uncertain interference based on a data-driven BN model and proposed corresponding resilience enhancement strategies.
In the quantitative analysis of ship navigation risks, the uncertainty information band has a great impact on the quantification of risks (Nguyen et al., 2021). Due to the particularity of the navigation environment in Arctic waters, these uncertainties are more intense. The advantage of the BN analysis method is that it can integrate multi-source and multi-category information, and can perform network reasoning on a few basis. In addition, the Bayesian analysis method mostly adopts the form of network reasoning, which can effectively reflect the correlation between risk factors of complex systems. Therefore, it is appropriate to use the BN analysis method for various uncertainties in the risk quantitative analysis of ship escort operations in Arctic waters.
Since the risk factors affecting the occurrence of ship traffic accidents are diverse, many of which often have no direct data source, information uncertainty is the main obstacle to the quantification of ship navigation risks. In the process of quantifying uncertain information, cloud model is an uncertain artificial intelligence method that can realize the conversion between qualitative judgment and quantification, and has received extensive attention from relevant scholars in recent years (Peng et al., 2017; Ma et al., 2022). Wang et al. (2015) proposed a decision-making method based on cloud model for multi-criteria decision-making problems with unknown decision-makers’ weights. Guo et al. (2020) proposed a comprehensive evaluation method based on cloud model for the randomness and fuzziness of subjective information, which realized the simultaneous consideration of randomness and fuzziness in the process of quantification of subjective information. Cloud model has also been widely used in the study of water traffic accident risks. Wu et al. (2019) combined Markov chain and cloud model to quantitatively reason about the process risk of bauxite ships during maritime transportation. Liu et al. (2020) developed a ship collision accident risk reasoning system based on cloud model for the risk of ship collision accidents. Li et al. (2023a) used cloud model to quantify the uncertainty information in the evolution characteristics of the Maritime Autonomous Surface Ship navigation risk. Xi et al. (2024) integrated evidence theory and cloud model to fuse multi-source subjective information when analyzing human operational errors during ship icebreaking escort operations in Arctic waters. It can be seen that since cloud model can handle uncertain information with fuzziness and randomness, it is suitable for quantitative analysis of the uncertainty in ship navigation risks.
Ship escort operation in Arctic waters is a special operation mode. During the escort operation, the risk factors affecting different operation links are different, and there are complex coupling relationships between risk factors. These unique characteristics bring a series of new challenges to understanding the random phenomenon of system risks caused by the dynamic changes of risk factors and to quantitatively analyze the risks of escort operation processes. This study uses a system analysis method to analyze the dynamic behavior and nonlinear coupling effects in the process risks of complex technical systems in escort operations in Arctic waters. By introducing FRAM into escort operation in Arctic waters, key events or factors affecting the risks of escort operations are identified. Based on the network topological relationship between the risk factors of escort operations in Arctic waters, the cloud model is used to quantify the uncertain information, and the BN analysis method based on conditional probability reasoning is used to achieve quantitative analysis of escort operation process risks.
The main framework of the risk assessment method for escort operation in Arctic waters proposed by this study is shown in Figure 1. In this method, the complexity analysis method FRAM is used to identify risk factors, and the BN analysis method is used to establish a risk assessment model. For the uncertain information in the risk factors, the cloud model is proposed to achieve quantitative processing of subjective information. The main contents of the proposed method are divided into the following three steps.
1. By analyzing the main behavioral characteristics of the escort operation system composed of icebreaker and escorted ship, the escort operation system is converted into multiple functional modules. The characteristics of each functional module are described from six aspects: I, O, C, P, R, and T. By analyzing the main factors leading to functional failure, the failure links between functional modules are determined, and the functional failure network diagram is completed by combining chain connections. In-depth analysis of each functional module is carried out to find out the potential key risk factors that lead to accident.
2. According to the risk factors identified by the FRAM model, with the help of expert knowledge or reference to other literature, the influence relationship between risk factors is judged. With risk factors as network nodes, the influence relationship between factors forms directed edges, and the risk assessment BN structure is preliminarily constructed. On this basis, according to the type of each node in the BN, the model input required for each risk factor is judged as a priori probability table or conditional probability table.
3. For the model input required for each risk factor in the risk assessment BN model, it is judged whether it has an objective data source. For risk factors with direct data sources, their prior probability tables are obtained through probability statistics. For risk factors without direct data sources, the cloud model is used to quantify expert knowledge, thereby further obtaining its prior probability table. On this basis, the BN reasoning is used to quantitatively assess the risk of escort operation collision accident in Arctic waters.
The risk of ship navigation is often understood as a combination of the possibility of a ship traffic accident and the severity of the accident consequences. In the research related to the risk assessment of ship navigation in polar waters, the probability of a certain type of accident is often quantitatively inferred (Afenyo et al., 2021). This probabilistic risk assessment method has been widely used. Escort operation in Arctic waters is a formation consisting of an icebreaker and a following escorted ship. During the escort operation, collision accident between ship formations is the main threat faced during navigation. The risk of collision accident is often caused by the complex coupling of multiple risk factors (Khan et al., 2020), which can be expressed by Equation 1. How to objectively reflect the complex correlation between risk factors and use quantitative analysis methods to evaluate the risk of collision accidents between ship formations is the key issue to be solved in this study.
FRAM is a method for describing and analyzing the functions and activities of socio-technical systems based on the perspective of functional resonance (Patriarca et al., 2017; Kim and Yoon, 2021). In the process of using FRAM for accident investigation and risk assessment, the focus is mainly on the connection between the subcomponents of the system. The system is often decomposed into multiple functional modules for identification and analysis, which is conducive to the positive control of system safety. The functional modules of FRAM are generally represented in the form of functional hexagons, representing six aspects of information: input (I), output (O), control (C), premise (P), resource (R) and time (T). These aspects of information are connected using chains according to the specific situation of the system to complete the construction of the FRAM model.
Safety-II focuses on the safety of the system and emphasizes how to make the system safer through various measures. As a system method from the Safety-II perspective, FRAM not only analyzes the complex nonlinear coupling between system functional modules, but also emphasizes the internal changes of the system based on human, technical and management factors, and can achieve good phenomenon expression. When using FRAM to analyze the collision accident risk of escort operations in Arctic waters, the construction of the FRAM model mainly follows the following steps.
1. Identify and describe the basic functions of the system. For the ship escort operation system in Arctic waters, it is converted into multiple functional modules through analysis. From the functional characteristics of I, O, C, P, R, and T, the characteristics of each functional module and the relationship between the functions are further described.
2. Analyze the potential changes of each functional module of the system. Analyze the main factors that may cause functional failure from the two aspects of the system inside and outside.
3. Draw a functional failure network diagram. Analyze the coupling relationship between the functional modules of the ship escort operation system in Arctic waters, determine the failure links, and complete the drawing of the functional failure network diagram by combining the chain connection.
4. Analyze the cause of the accident. Combined with the functional failure network diagram, deeply analyze each functional module to find out the key risk factors leading to collision accidents in Arctic waters escort operation.
A BN structure is a directed acyclic graph consisting of variable nodes and directed edges. It can intuitively reflect the causal relationship between factors, and can perform network learning and reasoning under limited information. It is often used to assess and predict the risk of ship traffic accidents (Sakar et al., 2021; Basnet et al., 2023). In the process of constructing a BN model, once the nodes, directed edges, and probability tables are determined, the BN model is established.
When constructing the BN model of escort operation collision accident risk in Arctic waters, the risk factors that affect the occurrence of accident represent nodes, and the coupling relationship between risk factors is represented by directed edges. The relationship strength between nodes is represented by a conditional probability table. Nodes without parent nodes need to use a bright probability table to express information. In this study, the nodes and directed edges in the BN can be obtained through the constructed FRAM model. The conditional probability table is generally obtained through logical judgment. The prior probability table often needs to be obtained through statistical calculations based on the objective discrete data set of risk factors. However, in practice, some risk factors often have missing data. Expert knowledge is the main means to make up for the missing data, but it is often necessary to use certain methods to convert subjective information into objective data information (Chen et al., 2022).
The cloud model is often used for the quantitative processing of uncertain information. It can complete the conversion between qualitative concepts and quantitative data. The cloud model uses three characteristic quantities: expectation (Ex), entropy (En) and hyperentropy (He) to express the randomness and fuzziness of qualitative concepts, and overall reflects the quantitative expression of qualitative concepts (Li et al., 2022). Among them, the mathematical expression of Ex is shown in Equation 2, which is similar to the concept of mean and represents its concentration. represents the subjective evaluation value of the expert on the uncertain information. n represents the number of evaluation values. The mathematical expression of En is shown in Equation 3, which represents the uncertainty of the target of interest. The larger its value, the wider the cloud droplet. The mathematical expression of He is shown in Equation 4, which represents the discreteness of En. The larger its value, the wider the thickness of the cloud droplet. In the field of ship navigation risk management research, cloud models are often used to quantify the uncertainty information of risk factors (Li et al., 2023a; Xi et al., 2024).
In order to complete the conversion from qualitative description to quantitative expression based on the three characteristic quantities of Ex, En and He, a forward cloud generator is often used to implement this function. For a random realization of a risk factor, its membership function needs to satisfy Equation 5. Through multiple random accumulations of cloud droplets, a large number of cloud droplets can be generated to form a cloud model.
The Northeast Passage in the Arctic is the busiest waters for commercial ships to conduct navigation operations in Arctic waters. The seasonal navigation characteristics of the Northeast Passage in the Arctic are obvious. It can be freely navigated in the summer months with the highest temperature. In the seasons close to the summer months, the ice conditions are good, and commercial ships reinforced with ordinary ice class can conduct commercial navigation under the escort of icebreaker. During the escort operation, the icebreaker in front has a higher icebreaking level, which can directly break the sea ice that hinders navigation and form a navigable channel. The escorted ship behind follows the navigation track of the icebreaker in front to complete the ice zone navigation operation. This method greatly improves the navigation efficiency and safety of ordinary ice class reinforced ships in the Arctic ice zone. However, the escort operation itself is still a high-risk water transportation activity. First of all, the width of the icebreaker and the escorted ship will affect the level of risk of the escort operation. If the width of the icebreaker is smaller than that of the escorted ship, the width of the channel formed by the icebreaker will not meet the navigation needs of the escorted ship, greatly increasing the navigation risk. Secondly, the closing speed of the channel formed by the icebreaker has a great impact on the risk of escort operations. If the ice channel closes quickly, the escorted ship will maintain a high speed, and the icebreaker and the escorted ship will also maintain a close distance, which will directly increase the risk of collision accidents. In addition, the size, strength and thickness of the remaining floating ice in the ice channel will also have a great impact on the risk of escort operations. Therefore, for high-risk water transportation activities such as escort operations, it is necessary to take scientific means to quantify its risk level.
In this study, a formation mode consisting of an icebreaker and an escorted ship is used to quantitatively analyze the safety risks of its operation process. The escort operation risk of the TIAN HUI ship in 2018 is taken as the research object. The ship departed from the Barents Sea on July 20 and arrived at the Bering Strait on August 3. During the Arctic voyage, the voyage from July 29 to August 2 was escorted by the icebreaker VAYGACH. In Figure 2, relying on AIS data, the route information of the ship from the departure point to the Bering Strait section and the changes in the ship’s position every day are shown.
According to the timing characteristics of ship escort operation in Arctic waters, it can be mainly divided into the preparatory stage before the operation, the icebreaking escort operation stage, and the end of the escort operation. In the preparatory stage before the operation, it is necessary to combine the weather forecast information to formulate a comprehensive navigation plan. Before the escort operation officially begins, it is also necessary to select a suitable icebreaker and conduct a comprehensive safety inspection. After the escort operation mission begins, the icebreaker must choose a suitable forward strategy in front to ensure that it can effectively break the sea ice and form a reliable navigable waterway. By maintaining timely and effective communication between the two ships, ensure that the ship speed remains within a safe and efficient range. At the same time, ensure that the distance between the two ships is not too long or too short. During the icebreaking escort operation stage, due to the dynamic adjustment of the ship movement between the two ships at any time, this stage is also a high-incidence period for collision accidents. It is necessary to analyze and judge the collision risk in a timely manner and make effective risk prevention and control strategies.
By analyzing the main operational tasks of each stage and further subdividing the key subtasks of each step, the escort operation system in Arctic waters can be mainly divided into nine functional modules: “Make a suitable sailing plan”, “Choosing the right icebreaker”, “Effectiveness of communication”, “Comprehensive safety inspection”, “Identify effective ice-breaking strategies”, “Maintain a suitable speed”, “Keep a safe distance”, “Judge the collision risk”, and “Risk control measures”. Combined with the main steps of FRAM model construction, the functional characteristics of each functional module are analyzed from six aspects: I, O, C, P, R, and T. Taking the functional module “Make a suitable sailing plan” as an example, its functional description is shown in Table 1.
On this basis, the relationship between different functional modules of the escort operation system in Arctic waters is further described, and the main factors that may lead to functional failure are analyzed from the two aspects of the system internal and external, as shown in Table 2. According to the correlation and potential changes between the functional modules, the failure links are analyzed to establish the functional failure network diagram of the ship icebreaking escort operation in Arctic waters, as shown in Figure 3.
According to the main factors leading to functional failure analyzed in Table 2 and the functional failure network of ship escort operation in Arctic waters described in Figure 3. Considering that in the Arctic waters escort operation system, abnormal changes in functional output will lead to an increase in the risk of collision accidents through the coupling resonance of upper and lower related functional modules. Through the functional resonance analysis between functional modules, combined with the specific task characteristics of ship escort operation, the risk factors leading to escort operation collision accident are further analyzed in depth. The analysis results are shown in Table 3.
Through FRAM, the basic functions of the system and potential changes of modules of ship escort operation in Arctic waters are analyzed, and the risk factors affecting the safety of escort operation are preliminarily obtained. Further, through the analysis of the coupling relationship between modules, a functional failure network diagram is drawn. On this basis, the key risk factors leading to collision accidents of escort operations in polar waters are identified. These analyses can provide a direct basis for the establishment of a BN model for risk assessment of ship escort operation in Arctic waters. Combined with the risk factors of collision accident of escort operation in Arctic waters obtained from the analysis in Table 3, according to the rules of establishing the BN model, the risk factors affecting the occurrence of collision accidents of escort operation in Arctic waters are taken as nodes, and the coupling relationship between risk factors is taken as directed edges, and the BN structure of collision accident during escort operation in Arctic waters is obtained, as shown in Figure 4.
In the established BN model for risk assessment of escort operation in Arctic waters, the node states are divided into two types: “yes” and “no”. The model inputs required in the model include two types: prior probability table and conditional probability table. The conditional probability table can be obtained through logical judgment, and the prior probability table needs to be obtained through statistical calculation based on the objective discrete data set of risk factors. In the model, the node that needs prior probability input is the root node, including “harsh environmental conditions” “too much broken ice in the waterway” “insufficient information” “cognitive bias” “inadequate professional skills” “inadequate look-out” “equipment damage” “insufficient reading of relevant materials” “violations” “relevant system is not perfect” “insufficient attention”. Nowadays, a variety of marine observation and prediction methods are commonly used to facilitate the acquisition of ship navigation data (Yin et al., 2023). Among them, the environmental risk factors can be statistically calculated through objective data. The environmental data used in this study are the fifth-generation reanalysis data provided by the European Centre for Medium-Range Weather Forecasts. This dataset combines model data with observational data from all over the world to form a globally complete and consistent dataset. The dataset contains data on a variety of meteorological and oceanographic environmental parameters, including temperature, air pressure, precipitation, snowfall, wind speed, wave height, sea ice, etc. Figure 5 shows the environmental distribution of Arctic waters, among which (Figure 5A) shows the overall environmental distribution of the entire Arctic waters. Here, sea ice concentration is used as an example for display. Due to space limitations, other environmental factors are not displayed one by one. In (Figures 5B–D), the dynamic distribution of temperature, sea ice and wind speed at the ship station of TIAN HUI on the eighth day is shown respectively. Referring to the classification of the impact of environmental factors in Arctic waters on ship navigation safety in Li et al. (2023b), these discrete environmental data sets can provide a reference for solving the prior probability of environmental risk factors such as “Too much broken ice in the waterway” and “harsh environmental conditions”. Taking the node “Too much broken ice in the waterway” as an example, when calculating the prior probability distribution of its ship station every day, the change of sea ice concentration at the location is extracted according to the grid where the longitude and latitude of its ship station are located, as shown in Figure 6. Considering the strong icebreaking performance of the icebreaker, the value of sea ice concentration of 0.55 is considered as the state threshold, and then the prior probability distribution table of the node “Too much broken ice in the waterway” at each ship station can be directly obtained by statistics, as shown in Table 4.
Figure 5. Natural environmental conditions for navigation in Arctic waters. (A) shows the overall environment of the Arctic waters. (B) shows the temperature changes at fixed coordinate points. (C) shows the sea ice changes at fixed coordinate points. (D) shows the wind speed changes at fixed coordinate points.
Table 4. Prior probability distribution of “too much broken ice in the waterway” during ship navigation in Arctic waters.
In the BN risk assessment model, there are still some nodes that do not have direct data sources. According to the proposed cloud model quantitative analysis method, the cloud characteristic distribution value of the risk factor is obtained by relying on expert knowledge, and the random sample distribution of the risk factor is further obtained through cloud simulation. Here, five experts from related fields are invited to provide expert knowledge for the quantification of subjective information. Among them, three experts are professors from Shanghai Maritime University, who have been engaged in the research of risk management of ship navigation in Arctic waters for a long time. Two experts are captains with rich experience in driving polar commercial ships, from COSCO SHIPPING Special Transport Co., Ltd. For each risk factor, a subjective evaluation of the quantitative value of the factor is carried out based on their experience and knowledge. Based on the evaluation, the Ex, En, and He of risk factor are obtained by combining Equations 2–4, and the cloud simulation results are further obtained according to Equation 5.
Take the prior probability distribution calculation of the node “inadequate professional skills” as an example. During the voyage of the TIAN HUI ship in the Arctic waters, the voyage from July 29 to August 2 was escorted by the icebreaker VAYGACH. According to its icebreaking escort operation characteristics, its icebreaking escort voyage was divided into three stages, namely the first day of icebreaking escort operation (Stage 1), the middle stage of icebreaking escort operation (Stage 2), and the last day of icebreaking escort operation (Stage 3). For these three stages of the ship’s voyage in the Arctic waters, the three characteristic quantities of the risk factor “inadequate professional skills” in each stage are obtained through expert judgment, and its cloud droplet distribution is further obtained according to cloud simulation, as shown in Figure 7. Taking the quantitative value of 0.75 as the threshold for state division, the prior probability distribution of each stage of ship navigation operations in Arctic waters is calculated by counting the number of cloud droplets in each cloud droplet distribution, as shown in Table 5.
Figure 7. Quantitative cloud of information on risk factors for ship escort operations in Arctic waters.
According to the prior probability distribution and conditional probability distribution of each node, the quantitative assessment results of the navigation risk of the TIAN HUI ship during the icebreaker escort operation in the Arctic waters are obtained through BN reasoning, as shown in Figure 8. According to the results of the risk quantification assessment, during the icebreaker escort operation, the navigation risk of the ship showed a fluctuating downward trend. The average risk value per day during the ship’s icebreaker escort operation is 0.087. The highest risk time occurred on the second day of the escort operation, and the lowest risk time occurred on the last day. The difference between the highest and lowest risk quantitative values is 0.176. This shows that during the icebreaker escort operation in Arctic waters, the risk level fluctuates greatly. Carrying out icebreaker escort operations is a high-risk water transportation activity, and special attention should be paid to the safety threats brought by sudden risk events in the operation. Specifically, on the first day of the icebreaking escort operation, the risk of ship navigation is at a high level, with a quantitative value of 0.157. On the second day of the icebreaking escort operation, the risk of ship navigation reached the highest value, with a quantitative result of 0.185. Subsequently, the risk of ship navigation gradually decreased, and the risk level is the lowest on the last day of the escort operation, with a quantitative value of 0.009.
In order to further analyze the key risk factors during the escort operation in Arctic waters, the node “Level of collision risk” is set as the target node in the BN model, and the BN sensitivity analysis is carried out. The main risk factors affecting the occurrence of collision accident during the escort operation in Arctic waters are obtained, as shown in Figure 9. The top ten risk factors affecting the occurrence of collision accident are obtained through analysis, namely “Harsh environmental conditions (R1)” “Too much broken ice in the waterway (R2)” “Improper distance (R3)” “Error in judgement (R4)” “Insufficient information (R5)” “Cognitive bias (R6)” “Improper speed (R7)” “Inappropriate choice of icebreaker (R8)” “Unreasonable ice-breaking strategies (R9)” “Inadequate professional skills (R10)”. It can be seen that among the main risk factors affecting the occurrence of collision accident during escort operations in Arctic waters, the most important risk factor comes from the navigation environment, followed by inappropriate ship following distance and mistakes of ship operators.
It is a high-risk water traffic activity for ordinary commercial ships to carry out navigation in Arctic waters. In order to ensure navigation safety, escort operation is an effective operation mode. However, while this operation mode improves the navigation safety level of ships, it may cause collision accidents due to the following mode between icebreaker and escorted ship. This study quantitatively analyzes the risk level of collision accident during escort operation in Arctic waters. In order to verify the reliability of the risk assessment model established in this study and the effectiveness of escort operation on the navigation safety level in Arctic waters, it is necessary to conduct comparative verification analysis. During the five days when the TIAN HUI ship carried out escort operations, considering the impact of the icebreaking level of icebreakers on navigation risks, the risk assessment results were compared and analyzed. At a lower icebreaking level, the impact of sea ice on the navigation safety of ships will be greater. Assuming that the icebreaking performance of icebreakers is reduced, the possibility of sea ice threats increases by 20%. Therefore, in the BN risk assessment model in Figure 4, the model input of the node “too much broken ice in the waterway” is changed to analyze the navigation risk during escort operations. The results are shown in Figure 10.
From the quantitative results, it can be seen that with the decrease in icebreaking performance of icebreakers, the fluctuation trend of the escort operation risk in Arctic waters is consistent with the previous trend. However, under the strong condition of reduced icebreaking performance of icebreakers, the overall level of navigation risk is higher than that of icebreakers with high icebreaking levels. This is consistent with the actual navigation conditions of ships in Arctic waters, which shows the reliability of the model. Specifically, during the five days when TIAN HUI ship took icebreaking escort operations, the risk difference in the first two days was much higher than that in the last three days. By analyzing the sea ice conditions in Table 4, it was found that the value of ice cover in the first two days of icebreaking escort operations was much higher than that in the last three days. This also shows that when the sea ice conditions are more severe, it is very necessary to choose a reliable high-level icebreaker.
Ship navigation risk management often faces the problem of missing information, and how to solve the uncertainty in it has become one of the main problems to be solved in current research. The uncertainty of risk information often comes from several aspects. On the one hand, in the stage of risk identification, in the process of identifying risk factors and determining the logical relationship between them, different scholars have different logical thinking, and the various theoretical methods used are different, so the results often have strong uncertainty. On the other hand, in the process of quantitative analysis of ship navigation risk, there is often the problem of missing data sources for some risk factors, thus bringing uncertainty in data information. Compared with ordinary waters, the navigation environment in Arctic waters is more severe, and the task of escort operation is very complex, so the uncertainty faced in the process of quantitative analysis and management of navigation risk is even stronger, and new ideas are urgently needed to solve these problems.
In this study, to address the uncertainty in the risk modeling process, based on the functional resonance perspective, the FRAM method is used to describe and analyze the functions and activities of the escort operation system in Arctic waters, to establish a functional failure network of the ice-breaking escort operation in Arctic waters, and to analyze in depth the main risk factors that lead to collision accident during escort operation. This method effectively overcomes the problem of excessive subjectivity in risk factor identification under the traditional “man-machine-environment” perspective. Moreover, the logical relationship between the risk factors can be sorted out in a scientific and logical way, which can help to establish the risk network model of collision accident of escort operation in Arctic waters. In the process of quantitative risk analysis, this study proposes the use of FRAM and BN method effectively combined to establish a quantitative model for risk assessment, which has the advantage of integrating multi-source information and carrying out quantitative analysis of risk by means of network reasoning, and it is a reliable method of quantitative risk analysis for the characteristics of the escort operation in Arctic waters. In addition, in the processing of uncertain data information, this study proposes the use of a cloud model to realize the conversion of subjective experience to objective data, and cloud simulation through the eigenvalues of risk factors, thus providing a direct data source for BN risk inference. This approach brings new ideas for dealing with the uncertainty of data information in ship navigation risk management.
This study proposes an approach combining FRAM and BN to quantitatively analyze the risk of ship escort operation in Arctic waters. In the proposed method, to address the complexity of the ship escort operation system in Arctic waters, FRAM is used to model and analyze the complex coupling relationship between the systems, and the key risk factors in the system are further analyzed. Based on the correlation relationship between risk factors, a BN analysis method is used to establish a collision risk assessment model for ship escort operation in Arctic waters. Relying on multi-source data, the subjective information is quantified by combining the cloud model of uncertain information processing. For the specific scenarios of ice-breaking escort operation of ships in Arctic waters, the escort operation risk is quantitatively analyzed.
The results show that during the escort operation, the average value of ship navigation risk is 0.087, which is at a high level, and the maximum difference in risk reaches 0.176, with a large level of risk fluctuation during the escort operation. Among the main risk factors affecting the occurrence of collision accident during ship escort operation in Arctic waters, the risk factor with the greatest impact is “Harsh environmental conditions”, followed by “Too much broken ice in the waterway”. Taken together, among the main risk factors affecting the occurrence of collision accidents during escort operation in Arctic waters, the most important risk factor comes from the navigational environment, especially the influence of sea ice conditions. Unsuitable following distance of the ship and the error of the ship operator are also the key reasons affecting the collision accident. During ship escort operation in Arctic waters, it is essential to select icebreakers of the appropriate icebreaking class according to the sea ice conditions.
The method proposed in this study is effective in understanding the level of navigational risk during ship escort operation in Arctic waters, clarifying the key risk factors involved, and improving the safety level of escort operations. In future research, the model can be further expanded to introduce decision analysis methods to assess the effectiveness of various types of risk control measures by proposing them, so as to maximize the safety level of ship escort operations in Arctic waters.
The raw data supporting the conclusions of this article will be made available by the authors, without undue reservation.
ZL: Conceptualization, Data curation, Funding acquisition, Methodology, Writing – original draft, Writing – review & editing. XZ: Writing – review & editing, Data curation. SL: Data curation, Supervision, Writing – review & editing. KG: Writing – review & editing. SH: Writing – review & editing.
The author(s) declare that financial support was received for the research, authorship, and/or publication of this article. This work is financially supported by National Natural Science Foundation of China (NSFC) (Grant No. 52402422), by the Philosophy and Social Sciences Planning Project of Guangdong Province under Grant GD24XGL066. This work is also financially supported by the Zhanjiang Science and Technology Plan Project (Project No. 2024B01001), by the Business Administration Program Construction Project of Shenzhen Polytechnic University.
The authors gratefully acknowledge the reviewers for their valuable comments and suggestions.
The authors declare that the research was conducted in the absence of any commercial or financial relationships that could be construed as a potential conflict of interest.
All claims expressed in this article are solely those of the authors and do not necessarily represent those of their affiliated organizations, or those of the publisher, the editors and the reviewers. Any product that may be evaluated in this article, or claim that may be made by its manufacturer, is not guaranteed or endorsed by the publisher.
Abbassi R., Khan F., Khakzad N., Veitch B., Ehlers S. (2017). Risk analysis of offshore transportation accident in Arctic waters. Int. J. Maritime Eng. 159, A213–A223. doi: 10.3940/rina.ijme.2017.a3.351
Afenyo M., Jiang C. M., Ng A. K. Y. (2023). A Bayesian-loss function-based method in assessing loss caused by ship-source oil spills in the arctic area. Risk Anal. 43, 1557–1571. doi: 10.1111/risa.14025
Afenyo M., Ng A. K. Y., Jiang C. M. (2021). A multiperiod model for assessing the socioeconomic impacts of oil spills during arctic shipping. Risk Anal. 9, 13773. doi: 10.1111/risa.13773
Amin M. T., Khan F., Amyotte P. (2019). A bibliometric review of process safety and risk analysis. Process Saf. Environ. Prot. 126, 366–381. doi: 10.1016/j.psep.2019.04.015
Baksh A. A., Abbassi R., Garaniya V., Khan F. (2018). Marine transportation risk assessment using Bayesian Network: Application to Arctic waters. Ocean Eng. 159, 422–436. doi: 10.1016/j.oceaneng.2018.04.024
Basnet S., Bahootoroody A., Chaal M., Lahtinen J., Bolbot V., Banda O. V., et al. (2023). Risk analysis methodology using STPA-based Bayesian network- applied to remote pilotage operation. Ocean Eng. 270, 113569. doi: 10.1016/j.oceaneng.2022.113569
Behari N. (2019). Assessing process safety culture maturity for specialty gas operations: A case study. Process Saf. Environ. Prot. 123, 1–10. doi: 10.1016/j.psep.2018.12.012
Chen P., Zhang Z., Huang Y., Dai L., Hu H. (2022). Risk assessment of marine accidents with Fuzzy Bayesian Networks and causal analysis. Ocean Coast. Manage. 228, 106323. doi: 10.1016/j.ocecoaman.2022.106323
França J. E. M., Hollnagel E. (2023). Analyzing human factors and complexities of mining and O&G process accidents using FRAM: Copiapo (Chile) and FPSO CSM (Brazil) cases. Process Saf. Prog. 42, S9–S18. doi: 10.1002/prs.12428
Fu S., Yu Y., Chen J., Han B., Wu Z. (2022). Towards a probabilistic approach for risk analysis of nuclear-powered icebreakers using FMEA and FRAM. Ocean Eng. 2022, 260. doi: 10.1016/j.oceaneng.2022.112041
Fu S. S., Zhang Y., Zhang M. Y., Han B., Wu Z. D. (2023). An object-oriented Bayesian network model for the quantitative risk assessment of navigational accidents in ice-covered Arctic waters. Reliability Eng. System Saf. 238, 109459. doi: 10.1016/j.ress.2023.109459
Guo P., Li X. F., Jia Y. L., Zhang X. (2020). Cloud model-based comprehensive evaluation method for entrepreneurs’ Uncertainty tolerance. Mathematics 8, 1491. doi: 10.3390/math8091491
Kandel R., Baroud H. (2024). A data-driven risk assessment of Arctic maritime incidents: Using machine learning to predict incident types and identify risk factors. Reliability Eng. system Saf. 243, 109779. doi: 10.1016/j.ress.2023.109779
Khan B., Khan F., Veitch B. (2020). A Dynamic Bayesian Network model for ship-ice collision risk in the Arctic waters. Saf. Sci. 130, 104858. doi: 10.1016/j.ssci.2020.104858
Kim Y. C., Yoon W. C. (2021). Quantitative representation of the functional resonance analysis method for risk assessment. Reliability Eng. System Saf. 214, 107745. doi: 10.1016/j.ress.2021.107745
Lee J., Yoon W. C., Chung H. (2020). Formal or informal human collaboration approach to maritime safety using FRAM. Cognition Technol. Work 22, 861–875. doi: 10.1007/s10111-019-00606-y
Lenzen M., Tzeng M., Floerl O., Anastasija Z. (2023). Application of multi-region input-output analysis to examine biosecurity risks associated with the global shipping network. Sci. Total Environ. 854, 158758. doi: 10.1016/j.scitotenv.2022.158758
Li P. C., Wang Y. H., Yang Z. L. (2024). Risk assessment of maritime autonomous surface ships collisions using an FTA-FBN model. Ocean Eng. 309, 118444. doi: 10.1016/j.oceaneng.2024.118444
Li W., Chen W. J., Hu S. P., Xi Y. T., Guo Y. L. (2023a). Risk evolution model of marine traffic via STPA method and MC simulation: A case of MASS along coastal setting. Ocean Eng. 281, 114673. doi: 10.1016/j.oceaneng.2023.114673
Li W. C., He M., Sun Y. B., Cao Q. G. (2019). A proactive operational risk identification and analysis framework based on the integration of ACAT and FRAM. Reliability Eng. System Saf. 186, 101–109. doi: 10.1016/j.ress.2019.02.012
Li Z., Hu S. P., Zhu X. M., Gao G. P., Yao C. Y., Han B., et al. (2022). Using DBN and evidence-based reasoning to develop a risk performance model to interfere ship navigation process safety in Arctic waters. Process Saf. Environ. Prot. 162, 357–372. doi: 10.1016/j.psep.2022.03.089
Li Z., Zhu X. M., Li R. H., Liao S. G., Gao K. X. (2023b). A comprehensive method for causation analysis of ship–ice collision risk in Arctic waters. Environ. Sci. pollut. Res. 31, 40366–40382. doi: 10.1007/s11356-023-28249-7
Liu Y., Ma X. X., Qiao W. L., Ma L. H., Han B. (2024b). A novel methodology to model disruption propagation for resilient maritime transportation systems-a case study of the Arctic maritime transportation system. Reliability Eng. System Saf. 241, 109620. doi: 10.1016/j.ress.2023.109620
Liu X., Meng H. X., An X., Xing J. D. (2024a). Integration of functional resonance analysis method and reinforcement learning for updating and optimizing emergency procedures in variable environments. Reliability Eng. System Saf. 241, 109655. doi: 10.1016/j.ress.2023.109655
Liu H., Zhang L., Liu S. (2020). Modeling of ship collision risk based on cloud model. IEEE Access 8, 221162–221175. doi: 10.1109/ACCESS.2020.3042811
Ma Q., Lu L., Li Q., Wang Z. P. (2022). Green construction grade evaluation of large channels based on uncertain AHP-multidimensional cloud model. Sustainability 14, 6143. doi: 10.3390/su14106143
Ma L. H., Ma X. X., Liu Y., Deng W. Y., Lan H. (2023). Risk assessment of coupling links in hazardous chemicals maritime transportation system. J. loss Prev. process industries 82, 105011. doi: 10.1016/j.jlp.2023.105011
Moe A., Brigham L. (2017). Organization and management challenges of Russia's icebreaker fleet. Geographical Rev. 107, 48–68. doi: 10.1111/j.1931-0846.2016.12209.x
Nguyen S., Chen P. S. L., Du Y. Q., Thai V. V. (2021). An operational risk analysis model for container shipping systems considering uncertainty quantification. Reliability Eng. System Saf. 209, 107362. doi: 10.1016/j.ress.2020.107362
Otheitis N., Kunc M. (2015). Performance measurement adoption and business performance An exploratory study in the shipping industry. Manage. Decision 53, 139–159. doi: 10.1108/MD-02-2014-0108
Patriarca R., Di Gravio G., Costantino F. (2017). A Monte Carlo evolution of the Functional Resonance Analysis Method (FRAM) to assess performance variability in complex systems. Saf. Sci. 91, 49–60. doi: 10.1016/j.ssci.2016.07.016
Peng B., Zhou J. M., Peng D. H. (2017). Cloud model based approach to group decision making with uncertain pure linguistic information. J. Intelligent Fuzzy Syst. Appl. Eng. Technol. 32, 1959–1968. doi: 10.3233/JIFS-161473
Ryan C., Huang L. F., Li Z. Y., Ringsberg J. W., Thomas G. (2021). An Arctic ship performance model for sea routes in ice-infested waters. Appl. Ocean Res. 117, 102950. doi: 10.1016/j.apor.2021.102950
Sakar C., Toz A. C., Buber M., Koseoglu B. (2021). Risk analysis of grounding accidents by mapping a fault tree into a bayesian network. Appl. Ocean Res. 113, 1–12. doi: 10.1016/j.apor.2021.102764
Salihoglu E., Besikci E. B. (2021). The use of Functional Resonance Analysis Method (FRAM) in a maritime accident: A case study of Prestige. Ocean Eng. 219, 108223. doi: 10.1016/j.oceaneng.2020.108223
Tian J., Wu J. Y., Yang Q. B., Zhao T. D. (2016). FRAMA: A safety assessment approach based on Functional Resonance Analysis Method. Saf. Sci. 85, 41–52. doi: 10.1016/j.ssci.2016.01.002
Vanhatalo J., Huuhtanen J., Bergstrm M., Helle I., Mäkinen J., Kujala P., et al. (2021). Probability of a ship becoming beset in ice along the Northern Sea Route – A Bayesian analysis of real-life data. Cold Regions Sci. Technol. 184, 103238. doi: 10.1016/j.coldregions.2021.103238
Wang C. Y., Ding M. H., Yang Y. D., Wei T., Dou T. F. (2022). Risk assessment of ship navigation in the northwest passage: historical and projection. Sustainability 14, 5591. doi: 10.3390/su14095591
Wang J. Q., Peng J. J., Zhang H. Y., Liu T., Chen X. H. (2015). An uncertain linguistic multi-criteria group decision-making method based on a cloud model. Group Decision Negotiation 24, 171–192. doi: 10.1007/s10726-014-9385-7
Wu J. J., Hu S. P., Jin Y. X., Fei J. G., Fu S. S. (2019). Performance simulation of the transportation process risk of bauxite carriers based on the markov chain and cloud model. J. Mar. Sci. Eng. 7, 108. doi: 10.3390/jmse7040108
Xi Y. T., Zhang X., Han B., Zhu Y., Fan C. L., Kim E., et al. (2024). Advanced human reliability analysis approach for ship convoy operations via a model of IDAC and DBN: A case from ice-covered waters. J. Mar. Sci. Eng. 12, 1536. doi: 10.3390/jmse12091536
Xu S., Kim E. (2023). Hybrid causal logic model for estimating the probability of an icebreaker–ship collision in an ice channel during an escort operation along the Northeast Passage. Ocean Eng. 284, 115264. doi: 10.1016/j.oceaneng.2023.115264
Xu K. Y., Liu J. G., Meng H. (2023). Stability and energy consumption analysis of arctic fleet: modeling and simulation based on future motion of multi-ship. Environ. Sci. pollut. Res. 31, 40352–40365. doi: 10.1007/s11356-023-27787-4
Yao S. Y., Hu H., Yang J. B. (2022). A probabilistic safety assessment framework for arctic marine traffic using the evidential reasoning approach. Int. J. Shipping Transport Logistics 14, 266–301. doi: 10.1504/IJSTL.2022.122419
Yao S. Y., Wu Q. H., Qi K., Qi K., Chen Y. W., Lu Y., et al. (2024). An interpretable XGBoost-based approach for Arctic navigation risk assessment. Risk Anal. 44, 14175. doi: 10.1111/risa.14175
Yin J. C., Wang H. F., Wang N. N., Wang X. G. (2023). An adaptive real-time modular tidal level prediction mechanism based on EMD and Lipschitz quotients method. Ocean Eng. 289, 116297. doi: 10.1016/j.oceaneng.2023.116297
Yousefi A., Hernandez M. R., Pea V. L. (2019). Systemic accident analysis models: A comparison study between AcciMap, FRAM, and STAMP. Process Saf. Prog. 38, e12002. doi: 10.1002/prs.12002
Yu Y. R., Liu K. Z., Fu S. S., Chen J. H. (2024). Framework for process risk analysis of maritime accidents based on resilience theory: A case study of grounding accidents in Arctic waters. Reliability Eng. System Saf. 249, 110202. doi: 10.1016/j.ress.2024.110202
Zhang W. B., Goerlandt F., Kujala P., Qi Y. (2018). A coupled kinematics model for icebreaker escort operations in ice-covered waters. Ocean Eng. 167, 317–333. doi: 10.1016/j.oceaneng.2018.08.035
Zhang M. Y., Zhang D., Fu S. S., Yan X. P., Goncharov V. (2017). Safety distance modeling for ship escort operations in Arctic ice-covered waters. Ocean Eng. 146, 202–216. doi: 10.1016/j.oceaneng.2017.09.053
Zhang X. X., Zhang Q. N., Yang J., et al. (2019b). Safety risk analysis of unmanned ships in inland rivers based on a fuzzy bayesian network. J. advanced transportation, 4057195. doi: 10.1155/2019/4057195
Zhang W. B., Zou Z. Y., Goerlandt F., et al. (2019a). A multi-ship following model for icebreaker convoy operations in ice-covered waters. Ocean Eng. 180, 238–253. doi: 10.1016/j.oceaneng.2019.03.057
Zheng Q. H., Liu X. W., Yang M., Wang W. Z., Adriaensen A. (2024). Enhancing emergency response planning for natech accidents in process operations using functional resonance analysis method (FRAM): A case of fuel storage tank farm. Process Saf. Environ. Prot. 188, 514–527. doi: 10.1016/j.psep.2024.05.132
Keywords: risk performance, functional resonance accident model, Bayesian network, cloud model, escort operation, Arctic waters
Citation: Li Z, Zhu X, Liao S, Gao K and Hu S (2024) Risk performance analysis model of escort operation in Arctic waters via an integrated FRAM and Bayesian network. Front. Mar. Sci. 11:1499231. doi: 10.3389/fmars.2024.1499231
Received: 20 September 2024; Accepted: 26 November 2024;
Published: 11 December 2024.
Edited by:
Phoebe Koundouri, Athens University of Economics and Business, GreeceReviewed by:
Guanqiu Qi, Chung-Ang University, Republic of KoreaCopyright © 2024 Li, Zhu, Liao, Gao and Hu. This is an open-access article distributed under the terms of the Creative Commons Attribution License (CC BY). The use, distribution or reproduction in other forums is permitted, provided the original author(s) and the copyright owner(s) are credited and that the original publication in this journal is cited, in accordance with accepted academic practice. No use, distribution or reproduction is permitted which does not comply with these terms.
*Correspondence: Shiguan Liao, c2dsaWFvMDExM0BzenB1LmVkdS5jbg==
Disclaimer: All claims expressed in this article are solely those of the authors and do not necessarily represent those of their affiliated organizations, or those of the publisher, the editors and the reviewers. Any product that may be evaluated in this article or claim that may be made by its manufacturer is not guaranteed or endorsed by the publisher.
Research integrity at Frontiers
Learn more about the work of our research integrity team to safeguard the quality of each article we publish.