- 1Water and Society Section, Norwegian Institute for Water Research (NIVA), Oslo, Norway
- 2Department of Biological, Geological and Environmental Sciences, University of Bologna, Bologna, Italy
- 3Institute for Biological Resources and Marine Biotechnology (IRBIM), National Research Council of Italy (CNR), Ancona, Italy
Fisheries are complex systems. Food web models are increasingly being used to study the ecological consequences of fisheries policies and environmental change on such systems around the world. Nonetheless, these consequences extend well into the social, economic, cultural, and political domains of such systems. The main goal of this contribution is to characterize how food web models are being used to study the socioeconomic consequences of management actions and environmental change. We conducted a systematic literature review covering research published between January 2010 and July 2023. Only 47 papers (out of an initial pool of 506 publications) met our research criteria. Based on this, it is evident that the body of literature has been increasing slowly and at a constant rate – a condition not shared with other emerging research fields. Modeled systems were mostly marine (87%), covering the waters of 38 countries across 19 Large Marine Ecosystems; albeit mostly in the Global North. The ecological components of the reviewed models (e.g., functional groups) were represented at a much finer scale than their socioeconomic counterparts. Most models were developed using Ecopath with Ecosim (68%) or Atlantis (21%) modeling software suites. Four key research foci were identified across the selected literature. These shaped the methodological approaches followed, as well as the models’ capabilities, the simulation drivers, the way food webs were integrated with bioeconomic models, and the performance metrics they used and reported. Nonetheless, less than half captured social concerns, only one-third addressed trade-offs among management objectives, and only a handful explicitly addressed uncertainty. The implications of these findings are discussed in detail with respect to resource managers needs for ecosystem-based fisheries management and ecosystem-based management. Our collective understanding of the interlinkages between the biophysical and socioeconomic components of aquatic systems is still limited. We hope this review is seen as a call for action and that the food web modeling community rises to the challenge of embracing interdisciplinarity to bridge existing knowledge silos and improve our ability to model aquatic systems across all their domains and components.
Introduction
Fisheries are complex systems that extend beyond fish populations and those who harvest them. Whether situated in freshwater habitats or the deep sea, fisheries function as intricate networks tied to aquatic ecosystems, global economies, and the societies in which they are situated (Garcia and Charles, 2008). Natural resource sustainability and economic viability is often the overall objective when managing fisheries. This begins from a human-based communication process involving various stakeholders, such as communities reliant on fishing-related livelihoods, scientists studying the human impact on marine ecosystems, and regulatory bodies responsible for sustainable management (Dybas, 2002; Armada et al., 2018; Bentley et al., 2019b).
Incorporating the social and economic components of fisheries is key for developing management strategies and fostering informed policy decisions (Costello et al., 2016; Stephenson et al., 2017; Bennett et al., 2021; Ouréns et al., 2022). Thus, explicitly addressing ecological, economic, and social trade-offs is necessary when drafting fisheries regulations, or when assessing their performance. This need is based on the interconnectedness of humans and the environment. On one hand, the fisheries sector is the main source of income for approximately 8% of the global population (Cheung and Sumaila, 2015). Seafood consumption is projected to increase to 182 million tons by 2030 (FAO, 2022). Hence, the increased pressure over these renewable resources and their effects on people’s livelihoods must be properly accounted for policies to be successful at protecting nature, the economy and global food security. On the other hand, fishers respond to economic and behavioral drivers (Russo et al., 2015; Wang et al., 2024). These, however, are dependent on how target stocks: (i) respond to harvesting, or (ii) are influenced by external drivers, such as environmental change or economic shocks, or (iii) react to altered food webs (Heymans et al., 2011; Kaplan and Leonard, 2012; Blenckner et al., 2015; Hodgson et al., 2018; Weijerman et al., 2018; Agnetta et al., 2022; Dowd et al., 2022).
The adoption of a systems, or holistic, approach to managing fisheries is now part of global legislation (Ramírez-Monsalve et al., 2016; Marshall et al., 2018; Haugen et al., 2024). Yet, the breadth of the system differs. In some cases, it is composed of a single target stock and its immediate environment (i.e., ecosystem approach to fisheries, EAF), while others include additional elements, such as multiple target stocks, trophic dynamics, species interactions (i.e., ecosystem-based fisheries management, EBFM), and others even include additional human activities affecting and being affected by fisheries (i.e., ecosystem-based management, EBM) (Patrick and Link, 2015). However, all frameworks recognize the need to comprehensively include socio-economic factors to inform decision-making processes (Stephenson et al., 2017; Marshall et al., 2018; Craig and Link, 2023).
Food web models are key tools for assessing the consequences of environmental and policy changes on fisheries systems (Craig and Link, 2023). They are representations of the complex network of feeding relationships and energy flows among species in an ecological community (Pimm et al., 1991; Plagányi, 2007). Models can be further categorized depending on the level of complexity that is explicitly addressed. End-to-end models aim to simulate the entire ecosystem, from primary producers to top predators, to provide comprehensive insights into ecosystem dynamics and the effects of fisheries management scenarios. These include Ecopath with Ecosim (EwE) (Christensen and Walters, 2004), Atlantis (Fulton et al., 2011; Audzijonyte et al., 2019), Object-oriented Simulator of Marine ecOSystem Exploitation (OSMOSE), Integrated Generic Bay Ecosystem Model (IGBEM), Dynamic Multi-Species Models or Minimum Realistic Models (Plagányi, 2007). Simple trophic models, on the other hand, focus on specific segments of the food web, analyzing only a few predator-prey relationships or trophic levels of interest - such as Models of Intermediate Complexity for Ecosystem assessments (MICE) (Thorson et al., 2019).
Food web models, however, are seldom applied to address environmental, ecological, and socio-economic issues together (Memmott, 2009; Wang et al., 2016; Bossier et al., 2018; Heijboer et al., 2018; Nielsen et al., 2018; Bauer et al., 2019). The literature using EwE, for example, the most widely used food web model in fisheries research (Plagányi, 2007; Bentley et al., 2024), has primarily addressed concerns regarding how ecosystems are affected by fisheries and vice versa. This has been done by simulating changes in fisheries policy and environmental drivers (e.g., sea surface temperature) and estimating their effects on target stocks, their predators, their prey, and the broader ecological community (Colléter et al., 2015; Keramidas et al., 2023). For example, these models have been successful at predicting: the consequences of harvesting prey on predators and non-target groups (Scotti et al., 2022), climate change impacts on fish biomass and energy transfer across the food web (Hodgson et al., 2018; Bauer et al., 2019; Agnetta et al., 2022), and the effects of implementing Marine Protected Areas (MPAs) on protected species (Ramirez et al., 2015). While these models conceptualize fisheries as agents of removal and sources of fishing mortality, a notable gap exists in the literature, with limited exploration into the implications of fisheries on human systems, such as how environmental changes may impact fleet revenue or a nation’s Gross Domestic Product (GDP). Moreover, as models are developed to address a particular question, their representation of the human subsystem is sometimes oversimplified (e.g., using a single fishing fleet as the source of fishing mortality in areas known to have multiple fisheries fleets operating simultaneously; Ramirez et al., 2015). While these models might be addressing important ecological questions, their usefulness for operationalizing EAF, EBFM and EBM require paying even more attention to the interplay between the biophysical and human components of these systems (Stephenson et al., 2017; Fulton, 2021; Craig and Link, 2023; Haugen et al., 2024).
The use of food web models to provide tactical advice for fisheries policy requires broadening the scope of research and how this is communicated to decision-makers and fisheries sector stakeholders (Fulton, 2021; Craig and Link, 2023). The end-users of the models are quite diverse and include government officials, fishers and their direct representatives, local businesses, seafood processors, distributors, wholesalers, and retailers, coastal communities, and seafood consumers – all key stakeholders whose choices and wellbeing are directly influenced by the information provided by ecological models (Schwermer et al., 2020). However, these stakeholders seek insights into the broader consequences of environmental change or fisheries policies on employment, salaries, fish prices, food security, availability of culturally significant resources, broader economic considerations, and gender issues. Addressing these objectives require adopting a language that resonates with their concerns and ensuring a close collaboration between policymakers and scientists (Stephenson et al., 2017; Galland et al., 2018; Craig and Link, 2023; Haugen et al., 2024).
This systematic review focuses on characterizing how food web models are being used to address the social and economic consequences of fisheries policies and environmental change. We first focus on characterizing the development of the field over time. Next, we focus on the questions addressed by the research and the methodological approaches undertaken by modelers. Finally, we discuss our findings within the broader literature, using examples from the reviewed literature to highlight interesting and novel approaches, while also drawing attention to topic areas that need greater consideration in future research.
Methods
The current review followed the PRISMA (Preferred Reporting Items for Systematic Reviews and Meta-Analyses) methodology (Moher et al., 2015). First, we developed a comprehensive research plan to set the boundaries of the analysis. Then we developed a multi-layer search string (Table 1) that was used on both Scopus (www.scopus.com) and Web of Science (www.webofknowledge.com) databases. A preliminary search of these databases resulted in very few papers meeting our criteria before the year 2011. Thus, we decided to focus only on peer-reviewed literature published since 2010. The search strategy (i.e., Scopus, Web of Science, and personal archive) resulted in 506 articles after duplicates were removed.
Next, we proceeded to exclude articles based on the information included in their abstracts. The abstract screening process was implemented using the web-based systematic review platform HubMeta (Steel et al., 2023). All abstracts were screened by at least two researchers. A total of 406 publications were excluded in this stage (Figure 1). The main reasons for the exclusion of articles were that they: (i) did not use socio-economic drivers (model inputs) or indicators (model outputs) (44%), (ii) were not using food web models (19%), or (iii) were not primary sources of information (e.g., literature reviews, book chapters, synthesis reports) (5%). However, almost one third of the excluded articles were false positives (i.e., abstracts using keywords that matched our search string but whose content was not relevant for our research). As some abstracts were not informative, a second level of screening was required. This screening focused on the papers’ methods and results sections. After which only 47 publications were selected for data extraction and analysis (Figure 1).
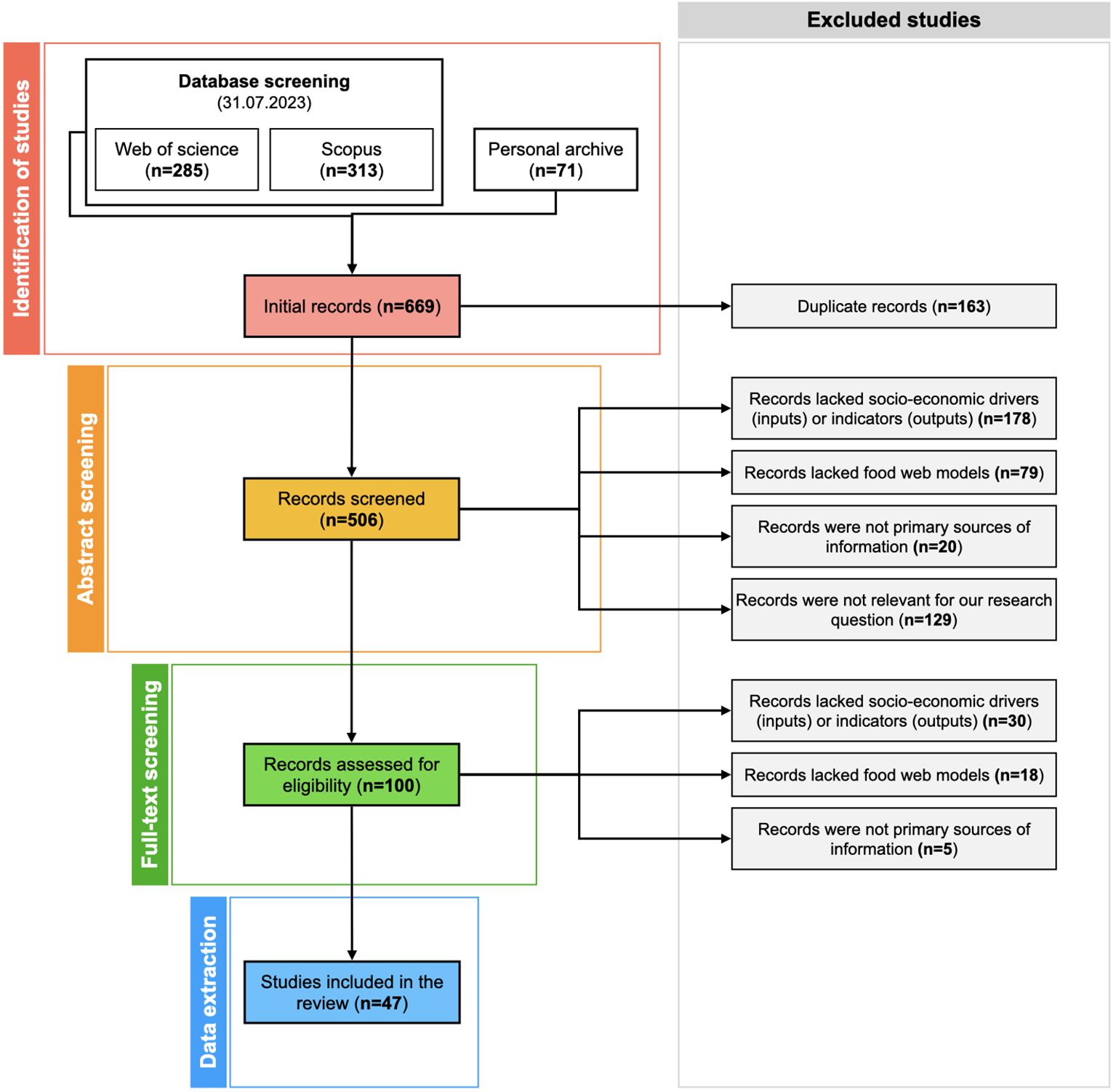
Figure 1. Flow diagram of the methodology and paper selection process based on the Preferred Reporting Items for Systematic Reviews (PRISMA) methodology (Moher et al., 2015).
Data was systematically extracted from each paper covering a series of standardized variables. These included general information about the articles (e.g., the year of publication, the research questions it addressed), the study area (e.g., the countries whose waters were included under the modeled area, the ecosystem type), the model (e.g., the modeling framework being used, the number of functional groups, the number of fishing fleets, the type of model integration procedures), the methodological approach used (e.g., if the study was simulation-based: what were the drivers used? If they were policy driven, was this achieved through input controls? Were fleet-dynamics explicitly addressed by the models? How did they measure the performance of the simulation outputs)?, and whether trade-offs and uncertainty were explicitly addressed and how. This information was the basis for assessing how food web models were being used to address the social and economic consequences of fisheries policies and environmental change. Variable descriptions and the resulting database are available for download (Supplementary Tables 1, 2).
Results
Field development
This field of study is relatively new with 47 peer-reviewed papers published between January 2010 and July 2023 matching our search criteria. While the literature has increased over time (Figure 2), the publishing rate has not (on average 3.4 papers yr-1). The research has mostly focused on modeling marine systems (87%), covering 38 countries across 19 Large Marine Ecosystems (LMEs) (Figure 3). However, these efforts are unevenly distributed. Most studies modeled LMEs found in North America (35%; mainly in the California Current, the Northeast US Continental Shelf and the Gulf of California), Europe (30%; mainly in the Baltic and Mediterranean seas) and Asia (15%; mainly in the South China and Sulu-Celebes seas). Brackish systems, like the Pearl River estuary in China (n=3), and freshwater systems, like Lake Erie shared by Canada and USA (n=2) and Lake Victoria shared by Kenya, Tanzania, and Uganda (n=1), have also been studied in detail.
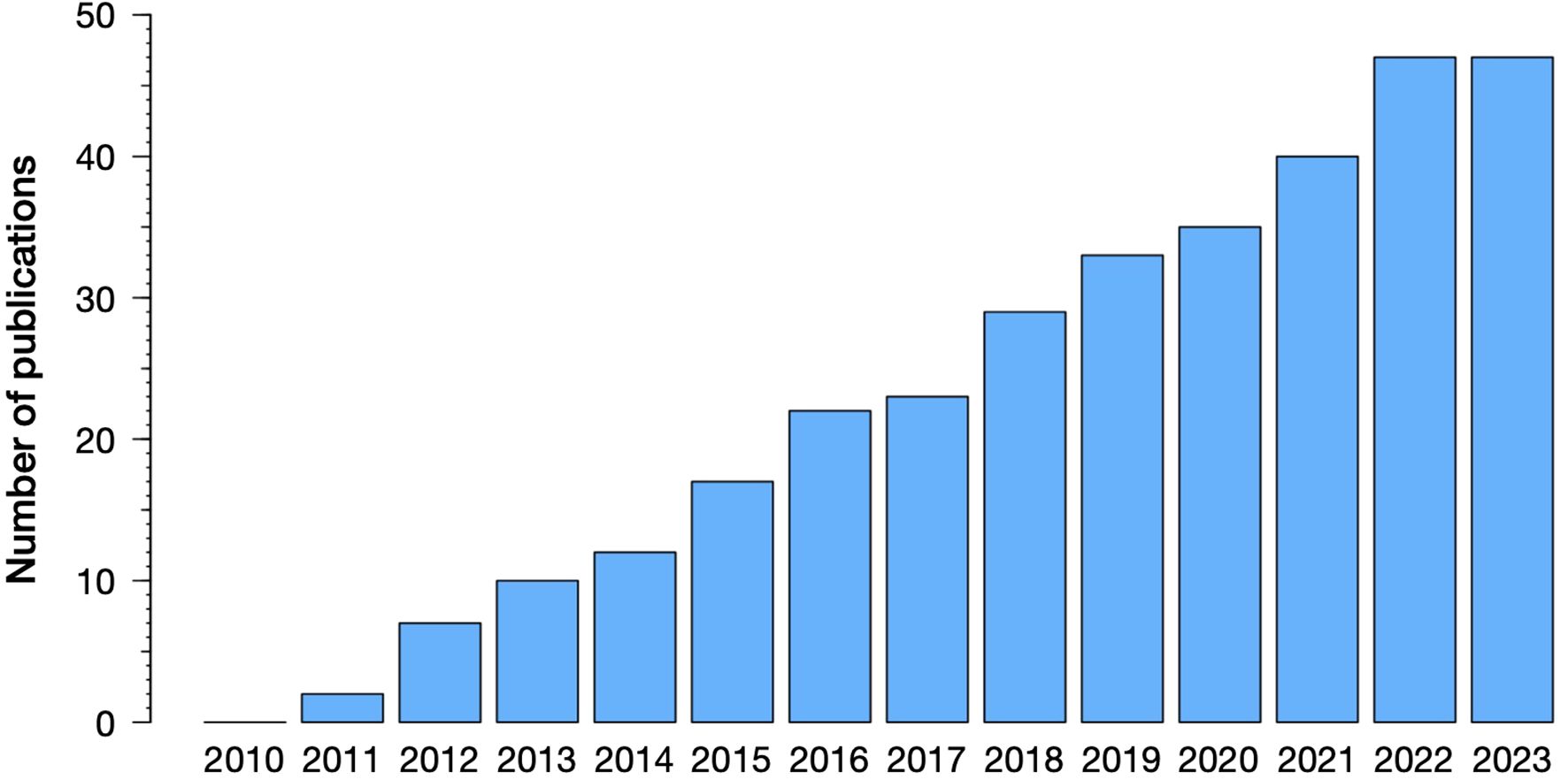
Figure 2. Cumulative frequency of publications evaluating the socioeconomic consequences of change on aquatic ecosystems using food web models across the studied period.
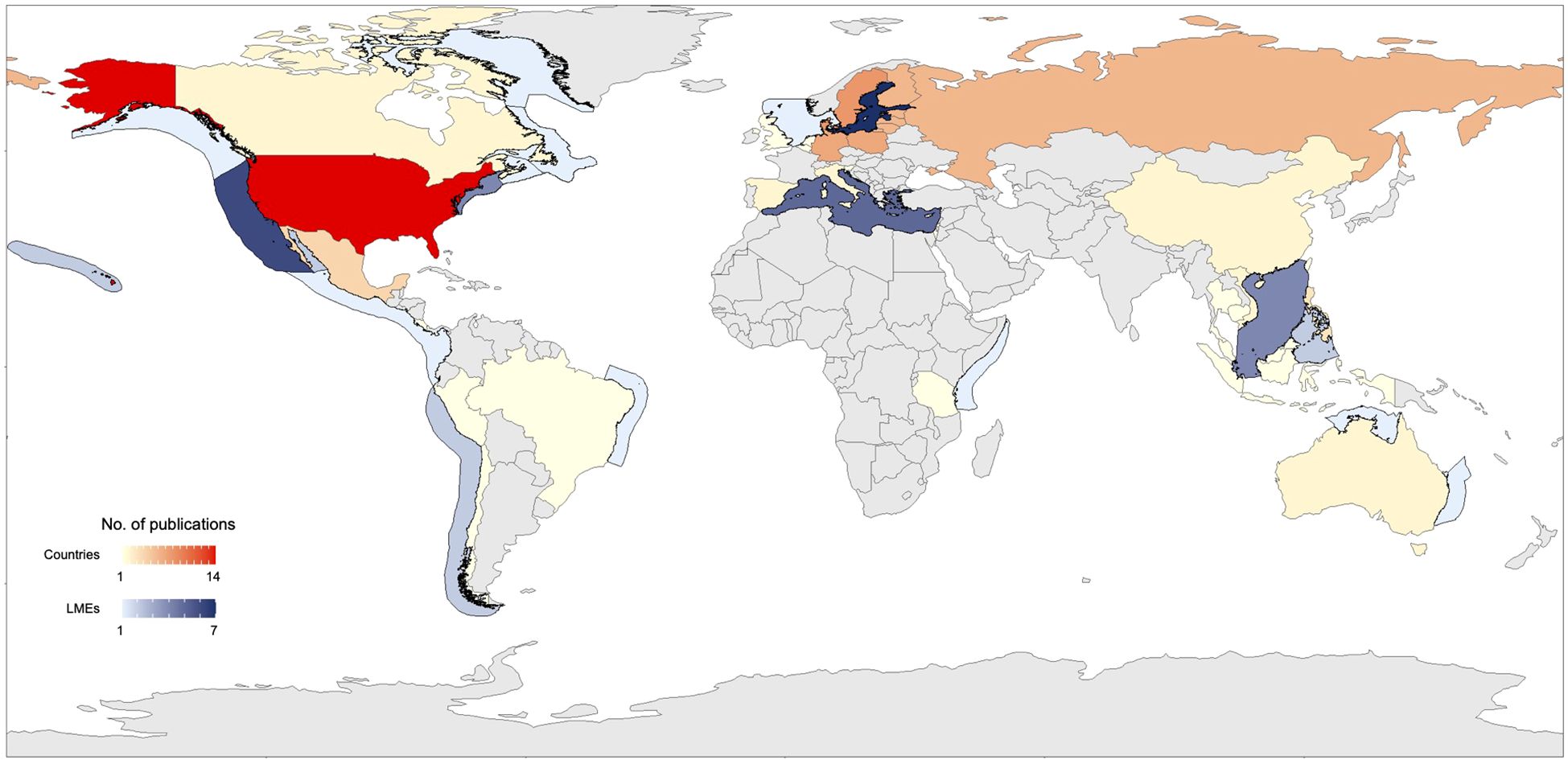
Figure 3. Spatial distribution of studies featuring marine fisheries systems. The map highlights the countries whose waters were modelled, as well as the Large Marine Ecosystems (LMEs) these belong to.
Food web model characteristics
Most publications (89%) used end-to-end models covering the entire food web, primarily developed using the EwE modeling software suite (Christensen and Walters, 2004) (n=30) or the Atlantis modeling framework (Audzijonyte et al., 2019) (n=8) or compared both modeling approaches (n=2). Alternative end-to-end models were also used in two studies (Jin et al., 2012; Koehn et al., 2017). Other types of food web models included MICE and multi-species bioeconomic models accounting for predator-prey interactions and their consequences (Wiedenmann et al., 2016; Voss et al., 2022).
Models provided a fine representation of the functional groups (i.e., species or groups of species) interacting in the food webs (Figure 4). On average, they included 35 ± 6 functional groups. Yet, their characterization of the fleets or métiers operating in the modeled systems had a much lower resolution. On average, models described 9 ± 2 fishing fleets. However, in 13% of the reviewed studies fisheries were lumped together into a single source of fishing mortality (e.g., Blenckner et al., 2015; Dowd et al., 2022). There were no statistically significant correlations between the year of publication and the number of functional groups, or fishing fleets, included in the models.
Research foci
Across the reviewed literature, five key research questions were addressed, with some articles covering more than one. Since these questions shaped the methodological approaches followed, we framed this overview around them. The food web models’ capabilities, simulation drivers, integration with bioeconomic models, and performance metrics discussed in the following sections are summarized in Figure 5.
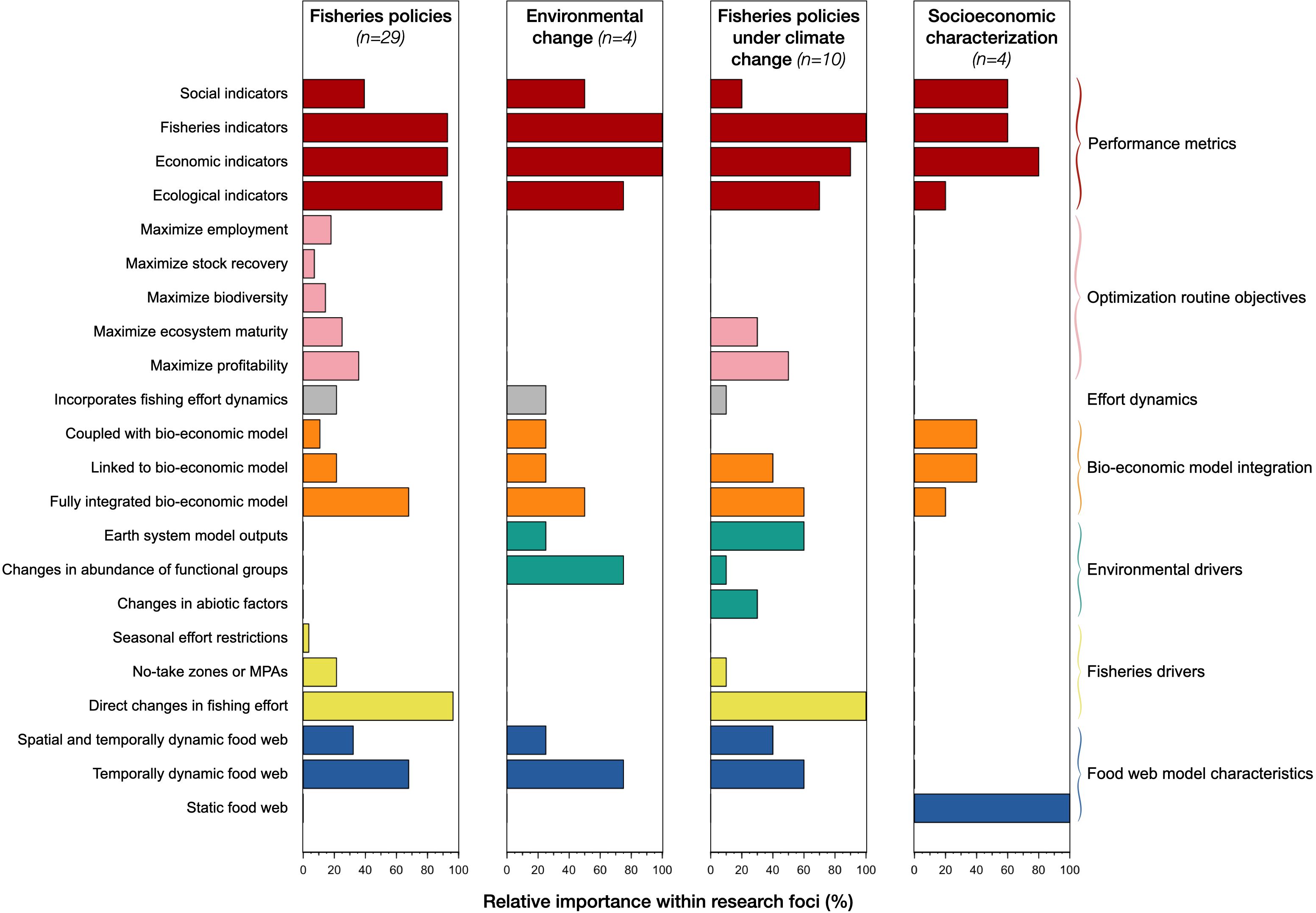
Figure 5. Relative importance of selected descriptors used to classify model capabilities across publications addressing the socioeconomic consequences of fisheries policies and environmental change.
Understanding the consequences of implementing fisheries policies
Most researchers sought to quantify the ecological and socio-economic consequences of implementing fisheries policies (n=29; 62%). These papers generally involved models capturing temporal (n=20), or spatial and temporal food web dynamics (n=9), driven by input controls (Bellido et al., 2020) such as: (i) direct effort reductions (i.e., introducing restrictions on fishing methods over time; e.g., Forrest et al., 2015; Armada et al., 2018; Celic et al., 2018; Fay et al., 2019; Natugonza et al., 2020; Alms et al., 2022), (ii) the implementation of no-take zones or MPAs (e.g., Ainsworth et al., 2012; Kaplan et al., 2012; Kaplan and Leonard, 2012; Dichmont et al., 2013; Morzaria-Luna et al., 2013; Ramirez et al., 2015), or (iii) seasonal fishing effort restrictions (Ainsworth et al., 2012). In some cases, effort reductions resulted from broader economic (e.g., limiting subsidies, Heymans et al., 2011) or health policies (e.g., COVID-19 related restrictions; Coll et al., 2021), rather than fisheries policies. Most models (n=19) used capabilities already integrated in their modeling frameworks to estimate economic indicators. These were associated with fishing fleet performance (e.g., fleet revenue or profit). In other cases, the models were linked (unidirectional flow of information; n=7) to: (a) input-output (I-O) models (Kaplan and Leonard, 2012; Fay et al., 2019) or (b) social accounting matrices (SAM; Wang et al., 2015, 2016, 2020), to assess the broader socio-economic consequences of fisheries policies, or (c) bioeconomic models used for cost-benefit analysis (e.g., starting a mesopelagic fishery off California; Dowd et al., 2022). Finally, some food web models were coupled (allowing for feedback loops; n=3) to bioeconomic models. These were used: (i) in an equilibrium analysis evaluating the fleet and fishery system’s socio-economic consequences of fishing tuna at Maximum Sustainable Yield (MSY) in the South China Sea (Christensen et al., 2011); (ii) to evaluate the effects of spatial closures in the Gulf of Carpentaria (Dichmont et al., 2013); and (iii) to assess the consequences of single species management actions on fleet effort dynamics in Lake Erie (Lee et al., 2021).
Understanding the consequences of environmental change
A portion of the literature (n=4, 9%) sought to quantify the ecological effects and socio-economic consequences of environmental change. These covered topics such as ocean acidification in the California Current LME (Hodgson et al., 2018), changes in the extent of eelgrass beds in Puget Sound (Plummer et al., 2013), species invasions in Lake Erie (Apriesnig et al., 2022), or changes in predator/prey biomass driven by fish escaping from aquaculture installations in the Mediterranean Sea (Izquierdo-Gomez et al., 2016). These works also used models capturing temporal (n=3) or spatial and temporal food web dynamics (n=1) and relied on integrated modeling capabilities (n=2), linked bioeconomic models (n=1), and coupled bioeconomic models (n=1) to provide socio-economic indicators for fishing fleets. These indicators were mostly related to fleet revenue or profitability, with two studies quantifying fishers’ compensations and employment (Hodgson et al., 2018; Apriesnig et al., 2022).
Understanding the consequences of implementing fisheries policies under different climate change scenarios
Several studies (n=10; 21%) investigated the impacts of climate change alongside alternative fisheries management scenarios. These studies were prominent in the Baltic Sea (Blenckner et al., 2015; Bossier et al., 2018; Bauer et al., 2019; Tunca et al., 2019; Hyytiainen et al., 2021; Uusitalo et al., 2022), the Hawaiian archipelago (Weijerman et al., 2018, 2021) and the Mediterranean Sea (Agnetta et al., 2022). Most of these works used models capturing temporal food web dynamics (n=6), while the rest used spatio-temporally explicit food web models (n=4). Simulations were driven by input controls together with progressive changes in abiotic factors (e.g., water temperature, dissolved oxygen, salinity) or primary production. Two studies modeled the effect of environmental change based on explicit alternative societal development strategies by adjusting the nutrient load (Bossier et al., 2018; Hyytiainen et al., 2021). Most of these papers (n=6) followed best practices, as recommended by the Fisheries and Marine Ecosystem Model Intercomparison Project (Fish-MIP; Tittensor et al., 2021), and directly used the outputs of earth system models to drive simulations and standardize climate change scenarios with Representative Concentration Pathways (e.g., Bauer et al., 2019; Hyytiainen et al., 2021; Agnetta et al., 2022; Uusitalo et al., 2022). This approach enabled projection and propagation of bottom-up ecosystem changes rather than focusing solely on specific environmental drivers. Economic indicators were provided using integrated capabilities of food web modeling platforms (n=6) or by linking to bioeconomic models (n=4), with indicators mainly related to fisheries revenue or profit.
Socio-economic characterizations of fisheries systems
A fourth body of papers (n=4; 9%) sought to characterize the fisheries system in socio-economic terms by using the outputs of static food web models as inputs for linked or coupled bioeconomic models. This approach allowed users to estimate: (i) the profit per gear and per fisher, and the total number of fishers operating in Tanzania’s Chwaka Bay (Rehren et al., 2018), (ii) the direct, indirect and induced employment and income effects of alternative oyster farming scenarios in Narragansett Bay (USA; Byron et al., 2015) or of the whole New England (USA) coastal economy under alternative environmental scenarios (Jin et al., 2012), and (iii) the direct and downstream socio-economic contributions of fisheries along seafood value chains in Peru (Christensen et al., 2014) and Baía Fomosa (Northeastern Brazil; Bevilacqua et al., 2019). In the last two cases, value chain modeling highlighted differences in employment and income multipliers across fishing fleets and functional groups. Moreover, sex segregated data in the Peruvian case study allowed users to emphasize where men and women were employed along the chain and how different their incomes were (Christensen et al., 2014), while the modeling work in the Brazilian case study highlighted the value of subsistence fishing (Bevilacqua et al., 2019).
Searching for policies through optimizations
A subset of papers on fisheries policies issues (n=9) and on fisheries policies under climate change (n=4), also sought to identify fisheries policies that maximize a utility function using optimization routines. The utility function could be based on a single or multiple management objectives, being optimized independently or simultaneously, by modifying fishing effort levels. These papers addressed how fleet profitability varies when fishing effort is optimized to maximize system level profits versus ecological stability (Heymans et al., 2011; Wang et al., 2012; Viet Anh et al., 2014; Bossier et al., 2018; Bauer et al., 2019; Hyytiainen et al., 2021; Alms et al., 2022).
All optimization studies had economic objectives, such as maximizing the long-term system profits, expressed through the net present value (NPV) of the fishery (n=12), or maximizing the likelihood of sustaining profits above the multi-species maximum economic yield (MEY) (Voss et al., 2022). The second most common objectives were ecological, such as maximizing: (i) ecosystem maturity, expressed via the longevity-weighted biomass of the system (n=9), (ii) biodiversity, expressed through a modified version of Kempton’s Q index (n=4), or (iii) stock rebuilding, expressed through the likelihood of functional group biomasses remaining above pre-established thresholds (n=2) (Alms et al., 2022; Voss et al., 2022). Additionally, studies sought to maximize employment across fishing fleets (i.e., a social objective; n=5) and one study maximized a fishery objective, expressed through the likelihood of keeping yields above a multi-species MSY (Voss et al., 2022).
These papers required fleets’ cost-income structures as inputs for the optimizations and an understanding of how changes in fishing effort affected fisheries employment. Yet, their outputs also provided alternative estimates of fleet and system level revenue and profits.
Food-web-based bioeconomic model structure
Most researchers (n=23, 49%) used existing bioeconomic modeling capabilities within core modules of the food web models to produce various indicators. These ranged from estimating the economic consequences of fisheries management actions (Ainsworth et al., 2012; Celic et al., 2018) to constructing physical and monetary accounts for Israeli fisheries in the Mediterranean (Michael-Bitton et al., 2022).
Other researchers developed custom food web models that integrated economic capabilities (n=5, 11%). For example, Tunca et al. (2019) developed a food-web-based bioeconomic model to test whether cooperative fisheries could better mitigate climate change impacts than non-cooperative ones in the Baltic Sea, while Koehn et al. (2017) and Sanchirico and Essington (2021) developed food-web-based bioeconomic models to assess the trade-offs of managing forage fisheries off California under an ecosystem-based approach.
The remaining researchers incorporated food web models in bio-economic modeling chains (i.e., ensemble modeling; n=19, 40%). In these cases, the core capabilities were extended by linking (n=13, 28%) or coupling (n=6, 13%) them with existing bioeconomic models. In some cases (n=5, 11%), the bioeconomic model was developed for the study. For example, Weijerman et al. (2016) linked the outputs of an Atlantis model of Guam’s coral reefs with two qualitative behavioral models for fishers and divers that incorporated economic parameters (e.g., fuel costs) to test eight different scenarios combining the effects of MPAs, fisheries management actions and land-based sources of pollution across various ecological and fisheries indicators. While Dichmont et al. (2013) coupled an Ecospace model of the Gulf of Carpentaria with a fisheries’ behavioral model to assess how different management strategies would affect the fleets’ effort dynamics and their ecological and economic performance. Yet, most bioeconomic modeling chains used models which had already been published (Table 2). For example, four publications linked food web model outputs with IMPLAN, an I-O model for the US economy (Kaplan and Leonard, 2012; Byron et al., 2015; Hodgson et al., 2018; Fay et al., 2019), while others (n=3, 6%) linked EwE outputs with SAMs for the Pearl River Delta (Wang et al., 2015, 2016, 2020), extending the capabilities of I-O models in the provision of socio-economic indicators, or used EwE’s value chain plug-in (n=3, 6%) to estimate socio-economic indicators as seafood flowed from the sea to the dinner plate (Christensen et al., 2011, 2014; Bevilacqua et al., 2019).
Performance metrics and trade-off analyses
All reviewed papers used various performance metrics or indicators to express model outcomes, classified into four domains: (1) ecological: used to highlight characteristics of the food webs or of functional groups not targeted by fisheries, (2) economic: used to express the economic and financial performance of the system, (3) fisheries: used to characterize fisheries performance in non-economic terms, and (4) social: used to express the systems’ contributions to employment and peoples livelihoods (Table 3). Indicators for the fisheries and economic domains were most prevalent, appearing in 94% and 91% of the selected papers, respectively. Ecological indicators were represented in 74% of the literature, while social indicators featured in only 40% of the papers.
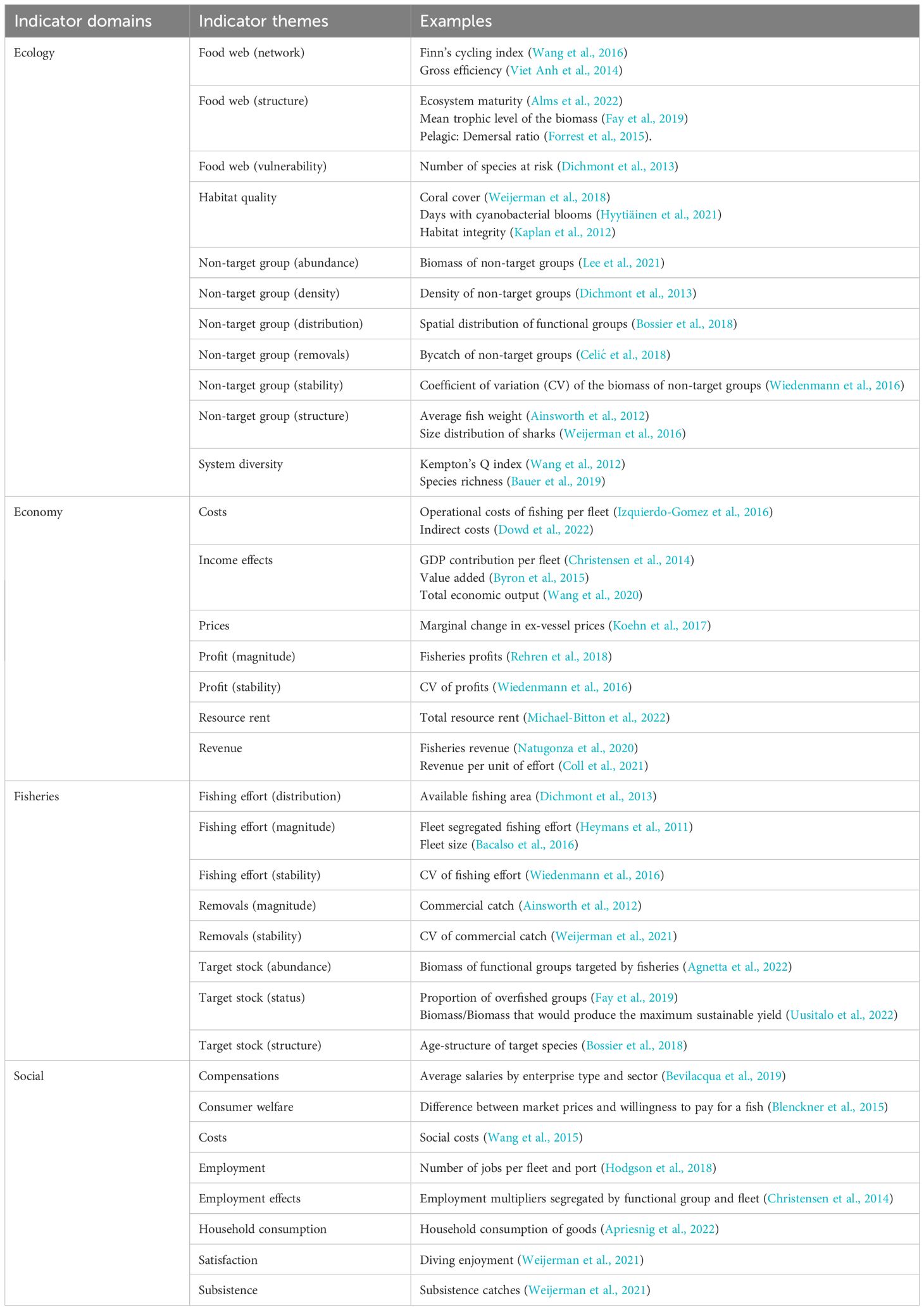
Table 3. Indicators’ domains, themes, and selected examples used to assess the performance of aquatic systems.
Ecological indicators belonged to eleven themes, primarily expressing information regarding non-target group abundance (74%; e.g., biomass of non-target groups or protected species), food web structure (49%; e.g., mean trophic level of the catch, average longevity, pelagic-demersal ratios), system diversity (40%; e.g., Kempton’s Q90 index, species richness, evenness), habitat quality (23%; e.g., coral cover, reef condition), food web network processes (20%; e.g., system throughput, gross efficiency, Finn’s cycling index) and non-target group removals (11%; e.g., bycatch). Economic indicators belonged to seven themes. The most common being: fleet or system level revenue (53%), fleet or system level profits (51%), income effects (28%; e.g., income multipliers by fleet or functional group, total economic output of the system) and costs (23%; e.g., fishing costs segregated by fleet or functional group). Indicators for the fisheries domain could be grouped into eight themes, mostly describing removals (86%; e.g., commercial catch), target stock abundance (72%; e.g., biomass of target groups or catches per unit effort), and fishing effort (23%, e.g., fishing days per year or fleet size). Finally, social indicators, covered eight themes and mostly focused on employment (80%; e.g., jobs segregated by fleet), compensations (20%; e.g., salaries segregated by enterprise type and sector) and employment effects (15%; employment multipliers segregated by functional group or fleet).
Over half of the reviewed literature used indicators from three domains, while only two papers focused exclusively on economic indicators (Figure 6). Nonetheless, trade-offs among indicators were not explicitly addressed in 32% of the papers. Most trade-offs analyses explored consequences of management action and/or environmental change between fisheries and ecological indicators (78%; e.g., by simulating the implementation of gear restrictions around coral reefs in Hawai’i and compering their effects on catch of target species and the abundance of apex predators; Weijerman et al., 2021), economic and ecological indicators (69%; e.g., by simulating the successful implementation of an illegal fishing ban in the central Philippines and comparing its effect on the abundance of non-target groups and the net profits of various fishing fleets; Bacalso et al., 2016), fisheries and economic indicators (53%; e.g., by simulating the effects of climate change, nutrient loading and fisheries policies in the Baltic Sea and comparing their impact on total yields and profitability; Uusitalo et al., 2022), or among economic indicators (38%; e.g., by simulating different fisheries management scenarios in the Pearl River estuary and comparing impacts on profitability and total income effects of multiple fleets; Wang et al., 2016).
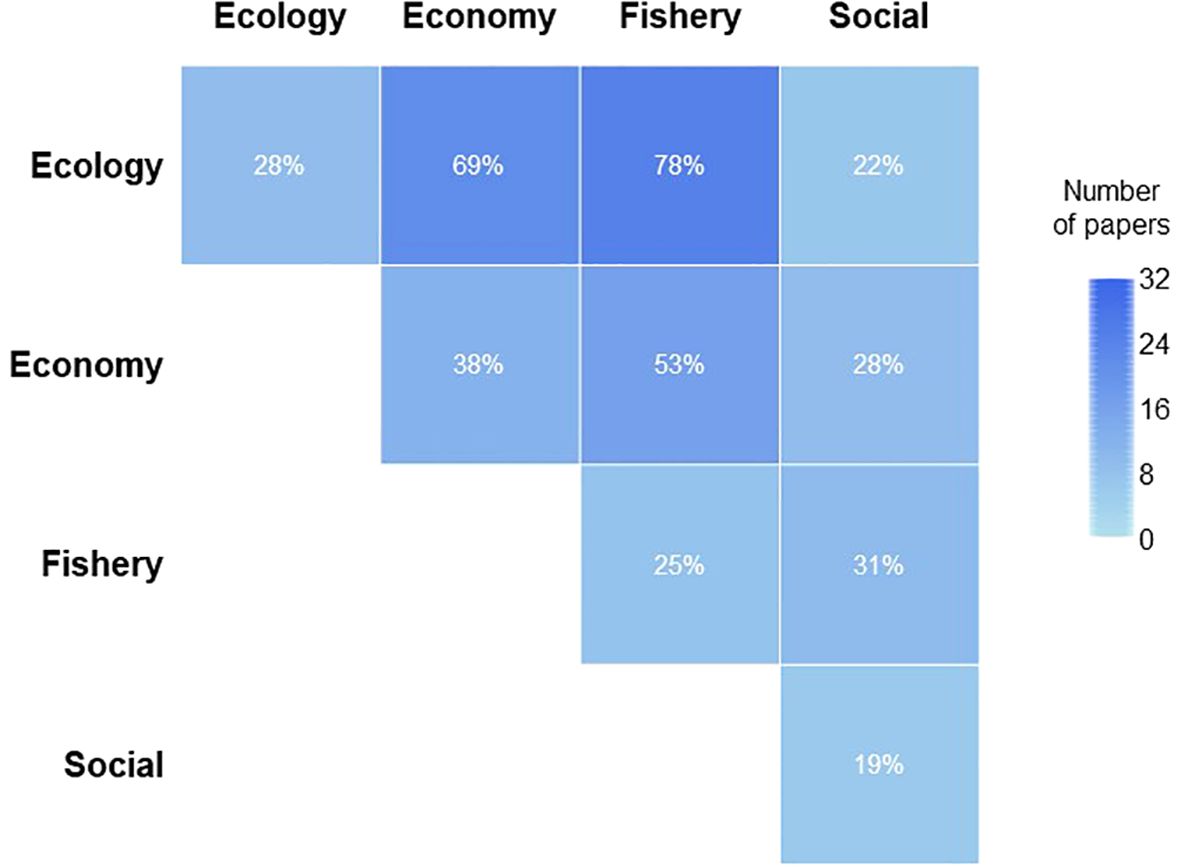
Figure 6. Most common indicator domain combinations (e.g., ecology vs fisheries) used across the reviewed literature to explore trade-offs among management objectives.
Uncertainty
Uncertainty is present in all modeling work, including parameter uncertainty (i.e., from sampling or measurement errors, or natural variability, affecting input values for used to parametrize the models), structural uncertainty (i.e., from model bias or faulty assumptions affecting how model components interact) or implementation error (i.e., from assumptions of full compliance while simulating policies) (Walters and Martell, 2004).
Over half of the reviewed articles (55%) did not explicitly consider uncertainty (Figure 7). The most commonly addressed was parameter uncertainty in the biophysical components of the food web model (34%), challenged through sensitivity analyses on model input parameters (i.e., explicitly addressed, n=10), indirectly addressed by comparing scenarios (i.e., using different input parameter values, n=3) or using alternative vulnerability schedules in EwE models (n=3). Only 15% of the literature considered parameter uncertainty in socio-economic components, addressed explicitly through sensitivity analyses (n=6), or indirectly through input parameter scenarios (n=1).
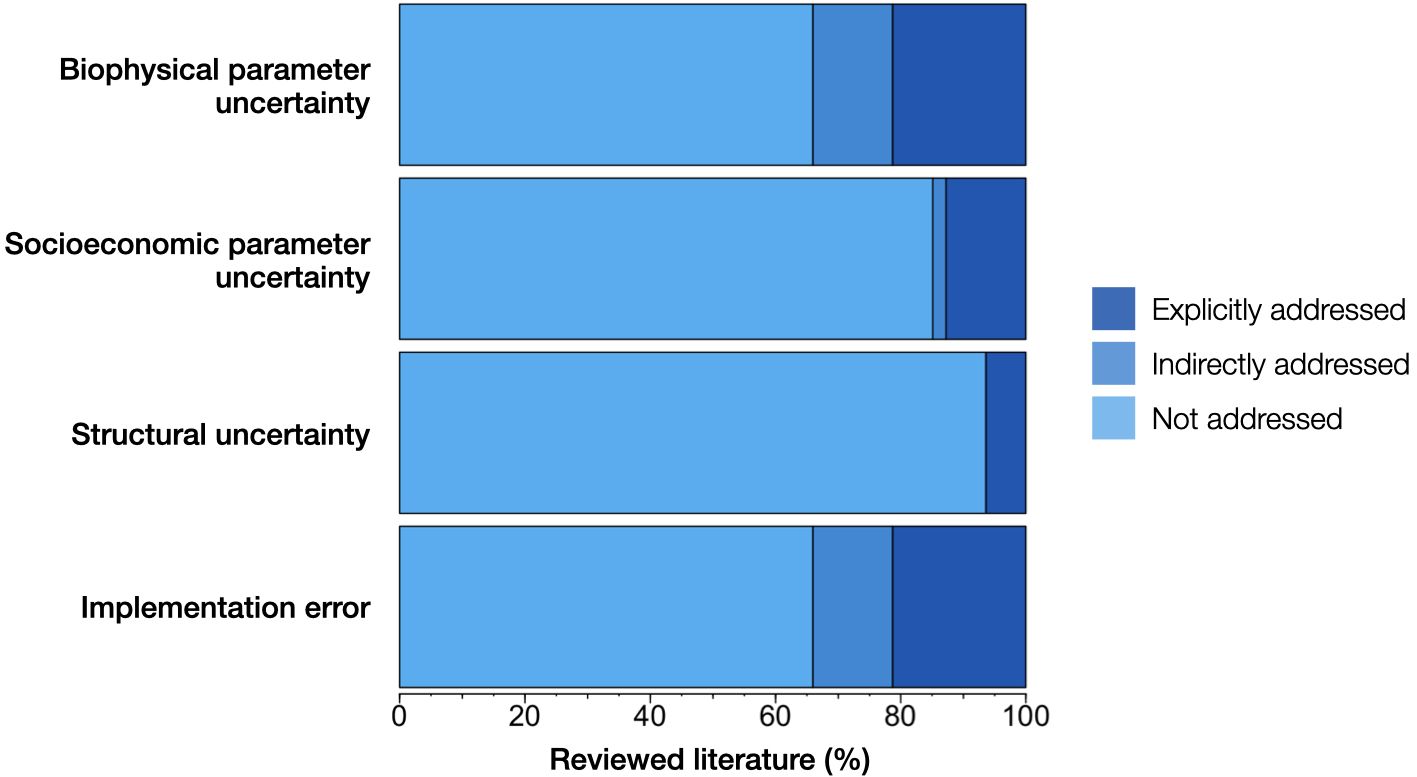
Figure 7. Publications addressing uncertainty as a fraction of the reviewed literature. Stacked bar charts correspond to the different types of uncertainty (e.g., biophysical parameter uncertainty). Colors denote if and how uncertainty was addressed.
Structural uncertainty was considered in 11% of the literature, mostly indirectly through scenarios (i.e., changing assumptions about how model components interact, n=3), or explicitly by comparing effects of model choice on simulation or optimization outputs (n=2). Only 6% of the literature addressed implementation error by simulating various levels of implementation and analyzing their effects on outputs.
Discussion
Food web models are great tools for integrating information, scenario testing and trade-off analyses (Fulton, 2021; Craig and Link, 2023; Rodriguez-Perez et al., 2023). These tools are being increasingly used for providing strategic and tactical advice to fisheries managers (Howell et al., 2021; Craig and Link, 2023). In this systematic review, we have found evidence that they were being used for characterizing the socio-economic importance of fisheries systems and simulating the socio-economic consequences of implementing fisheries policies and/or environmental change (including climate change) (Figure 5). Although great papers have been written in this field, food web models in general are not properly integrating the human dimensions of aquatic ecosystems.
Hundreds, if not thousands, of papers using food web models were published across the studied period (2010-2023). Yet only in 47 papers, models included socio-economic drivers or expressed their outputs using socio-economic indicators. This finding is not entirely surprising, as less than 5% of all ocean science literature involves social sciences (Partelow et al., 2023). However, the publication rate remained constant over time. This is a feature not shared by other emerging fields of study using food web models (e.g., offshore renewables), which shows that the one which we have reviewed is somewhat stagnant. As a modeling community it is important to change this.
The human dimensions of aquatic systems really matter. This is not something new, and an issue mentioned by both scientists and decision makers alike (Marshall et al., 2018; Barreto et al., 2020; Bennett et al., 2021; Ramírez-Monsalve et al., 2021; Tittensor et al., 2021; Melbourne-Thomas et al., 2022; Partelow et al., 2023; Rodriguez-Perez et al., 2023). Decision makers, resource users and stakeholders of global aquatic systems want to understand, and anticipate, the consequences of environmental or policy change not only on ecosystem functioning, but also on human well-being (Marshall et al., 2018; Fulton, 2021; Weiskopf et al., 2022; Craig and Link, 2023; Rodriguez-Perez et al., 2023; Thébaud et al., 2023). This requires the explicit consideration of the costs, benefits, and trade-offs over a wide array of ecological, social, economic, cultural, and political objectives at play. Failure to do so can lead to unsuccessful policies. For example, management decisions that do not account for the social and economic costs of their implementation can result in public dissatisfaction, lack of societal acceptance and reduced compliance (Stephenson et al., 2017; Weber et al., 2019; Craig and Link, 2023; Haugen et al., 2024). These consequences further limit management success while generating mistrust and hampering future collaboration and data sharing (among stakeholders and with regulators), as well as diminishing the legitimacy of future interventions. Moreover, these pathways can lead to pervasive, albeit resilient, cycles that foster controversial decisions, reduce policy options, hasten environmental degradation, and perpetuate social injustices, loss of cultural identity, and human rights abuses (Barreto et al., 2020; Bennett et al., 2022; Melbourne-Thomas et al., 2022; Villasante et al., 2022; Craig and Link, 2023; Partelow et al., 2023; Haugen et al., 2024).
Additionally, given that sustainability is the overarching goal of management interventions, it is important to remember that it operates under a triple bottom line (i.e., environmental, financial, and social) that permeates across both the biophysical and human dimensions of aquatic systems (Marshall et al., 2018; Rodriguez-Perez et al., 2023; Thébaud et al., 2023). If we work under the assumption that achieving sustainable fisheries will inevitably lead to social and economic well-being, then we will certainly fail. Positive socio-economic outcomes are not inevitable features of sustainable fisheries (Barclay et al., 2023; Partelow et al., 2023). Trade-offs between fisheries and ecosystem objectives, and conflict between resource users, may still occur even when fishing sustainably (Stephenson et al., 2017; Barclay et al., 2023; Craig and Link, 2023; Haugen et al., 2024). Nonetheless, only two thirds of the papers included in this review explicitly addressed trade-offs among management objectives and outputs.
To better illustrate this point, perhaps we can draw some examples from the reviewed literature. In Peru, research by Christensen et al. (2014) highlighted differences in the per ton contributions of functional group and fishing fleets to the country’s GDP and total employment. Hence, management measures seeking to improve stock status such as reductions in the total allowable catch of resources caught by multiple fleets (e.g., anchoveta or mackerels caught by both industrial and small-scale purse seiners) would impact resource users differently. Thus, it is likely that some stakeholder groups would oppose changes in management targets, that could be beneficial to them in the medium to long-term, if these did not include additional short-term assurances, such as socialized quota allocation among fleets (Walters and Martell, 2004). Similarly, research by Fay et al. (2019) or showed that effort reductions on the Northeast US Shelf caused greater change in employment than in sales, and that the consequences of management actions for individual sectors may be felt disproportionally through the region and across sectors. Finally, research by Celic et al. (2018) showed that the introduction of landing obligations, to reduce discards, in the Mediterranean Sea would have negative consequences on the ecosystem biomass, as well as on the fleets’ marketable landings and revenue. This work highlights that regulations with “good intentions” need to be validated and quantitatively assessed before being implemented as they could lead to unforeseen and unwanted consequences.
More work is required within the modeling community to frame and connect management actions with strategic goals related to social and economic objectives (Stephenson et al., 2017; Barclay et al., 2023; Partelow et al., 2023; Rodriguez-Perez et al., 2023; Thébaud et al., 2023; Haugen et al., 2024). Moreover, model outputs simulating the consequences of environmental or policy change also require to be expressed in meaningful socio-economic indicators for stakeholders and decision makers (e.g., fisher’s income, employment, access to seafood or recreation; Abedin et al., 2019; Bennett et al., 2021, 2022; Melbourne-Thomas et al., 2022; Barclay et al., 2023; Partelow et al., 2023; Thébaud et al., 2023; Haugen et al., 2024). These indicators, although likely sharing many similarities, should be context-specific (Bennett et al., 2021; Melbourne-Thomas et al., 2022; Partelow et al., 2023) and covering more than the most common attributes described in the reviewed papers (i.e., changes in biomass of target and not-target species, and changes in the average long-term yields by functional group and sector). Increased indicator diversity is needed particularly for the economic and social domains (Figure 6, Table 3). Many important, and viable, indicator candidates for these dimensions are available in the literature and could be linked to model outputs with relative ease (Barreto et al., 2020; Bennett et al., 2021, 2022; Barclay et al., 2023; Partelow et al., 2023; Thébaud et al., 2023). This is a task that requires modelers leaving their comfort zone and embracing interdisciplinarity (Weber et al., 2019; Fulton, 2021; Bennett et al., 2022; Weiskopf et al., 2022; Barclay et al., 2023; Craig and Link, 2023; Partelow et al., 2023; Rodriguez-Perez et al., 2023; Haugen et al., 2024).
Collecting comprehensive socio-economic data is crucial for developing standardized indicators that enable policymakers to monitor fishing activities effectively in both ecological and socio-economic contexts. Standardization can aid informed decision-making (Stephenson et al., 2017; Bennett et al., 2021), however reliance on a standardized set of indicators should not constrain the creativity and flexibility of existing modeling tools. It is important to mainstream their use while adapting models and indicators to specific local and stakeholder needs and contexts, ensuring that they remain relevant to diverse management objectives.
The inclusion of the human dimensions of aquatic systems will not only allow for more transparent debates and management advise, but it will also likely increase the uptake and usage of food web models in decision making processes (Weiskopf et al., 2022; Partelow et al., 2023; Haugen et al., 2024). Yet, this challenge is not homogenous across the globe. Greater efforts are required to increase representation of models for the Global South. Most publications featured in this review covered North American, European, and Australian case studies (Figure 3). Although this is also the case for Atlantis (CSIRO, 2024) and EwE (Ecopath Research and Development Consortium, 2024) models in general, the picture is less skewed towards the Global North when no socio-economic filters are applied. Raising the profile of case studies in the Global South is key, granted that these areas are already disproportionally affected by environmental injustices (Bennett et al., 2023). Moreover, given current needs, limitations, and trends, it is likely that the negative cumulative environmental, social, and economic effects of the blue acceleration, climate change, and local blue growth policies will continue to perpetuate existing injustices in the Global South (Jouffray et al., 2020; Melbourne-Thomas et al., 2022; Bennett et al., 2023).
Models that capture socio-economic dimensions provide policymakers with a more holistic understanding of the potential outcomes of management actions, allowing them to anticipate and mitigate negative impacts on communities reliant on fisheries (Fulton, 2021; Craig and Link, 2023). Trade-offs between ecological and socio-economic objectives can be assessed, supporting the development of inclusive policies objectives that strive for both healthy ecosystems and human communities. Food web modeling can thus play a pivotal role in providing information to strengthen local and global decision-making processes in favor of just, profitable, and sustainable futures (i.e., combating path dependency).
Another issue worth highlighting is the need to enhance the characterization of economic actors in the food web models. The food web modeling community has learnt from experience that there is no one-size-fits-all solution for representing food webs. Some questions require more complex models than others (Fulton, 2021; Craig and Link, 2023). Yet, food web models tend to oversimplify humans within aquatic systems. Our review found that functional groups were consistently represented at a much finer resolution than fishing fleets (Figure 4). In many cases fishing fleets were only seen as sources of fishing mortality, and in the most extreme situations all types of vessels and fishing operations were grouped under a single fishing fleet. This is not a serious representation of fishers or fisheries. Fleets group people whose decisions affect the system and who are affected by changes in the system. Thus, they need to be better characterized in the models so that drivers, trade-offs, and conflicts among them can be better explored (Ulrich et al., 2012; Weber et al., 2019; Schadeberg et al., 2021; Thébaud et al., 2023).
Additionally, human processes should be better represented in food web models – including the feedback between ecosystem dynamics and peoples’ choices or behavior (Tittensor et al., 2021; Melbourne-Thomas et al., 2022; Thébaud et al., 2023). Fishers’ behavior is not only dependent on regulations or profit (Girardin et al., 2017; Schadeberg et al., 2021; Thébaud et al., 2023). However, understanding how changes in profit affect effort dynamics is a key issue which is often overlooked. Very few of the reviewed papers accounted for fishing effort dynamics in their models (Figure 5). Changes in fishing effort drove most models, yet not many considered how the outcomes of a level of effort on a given time step (e.g., the profitability of the fleet on year t) influenced fishing effort (or affected target species selection) in the next time step. Atlantis models can capture some aspects of fleet dynamics (e.g., Ainsworth et al., 2012), yet for food web models developed on EwE, capturing fleet dynamics seriously relies on ensemble modeling efforts (e.g., Dichmont et al., 2013; Lee et al., 2021; Apriesnig et al., 2022; Failler et al., 2022). In both cases, this task requires multiple additional steps for food web modelers, but it adds realism to predictions.
The ensemble modeling approaches (i.e., modeling chains) identified in the present review (those assessing fleet dynamics and beyond) are promising examples of how modeling efforts can help advance interdisciplinarity. Given that economists generally use the types of outputs these models generate, albeit with simpler biophysical model components, their mainstreaming can be a good path to increasing model development and uptake beyond the food web modeling community. Another promising example is EwE’s value chain plugin (Christensen et al., 2011), which is the tool that provides the most diverse set of socio-economic indicators among the works reviewed for this study, while also allowing for feedback between the biophysical and human components of the fisheries system. More case studies involving ensemble bio-economic modeling approaches using food web models at their cores are certainly needed and should be encouraged.
It is important to note, however, that modelers should be cautious of whether they are representing fleets (i.e., groups of vessels of similar characteristics) or métiers (i.e., groups of fishing operations with similar target species, fishing gears, areas, and seasonality) in their models. Although, fleets and métiers can be the same in some situations, the kind of questions that models can address, and the validity of the advice provided based on them, will vary depending on this distinction (Ulrich et al., 2012).
Furthermore, we need to understand how context shapes fishers’ interactions (among them and with nature) (Partelow et al., 2023). This requires modelers to be critical and sincere about which aspects of such contexts are dependent on processes that cannot be adequately predicted (e.g., changes in seafood demand and consumer preferences, technological developments, population growth, future fuel costs, changes in employment across sectors, or collaboration among governments; Pinnegar et al., 2005; Melbourne-Thomas et al., 2022; Thébaud et al., 2023). A possible solution to this challenge can come from co-designing (with stakeholders and regulators) and operationalizing scenarios for standardizing model runs (e.g., based on shared socio-economic pathways; Pinnegar et al., 2005; Kreiss et al., 2020; Hamon et al., 2021). In these exercises model users can develop an explicit shared understanding of their assumptions regarding the interplay of future environmental, social, economic, technological, political, and cultural conditions. Scenario development of this sort has been promoted in the European context through projects such as CERES (2024) and FutureMARES (2024) – the latter directly applying such scenarios on EwE models. More efforts, and financial support, is needed for such undertakings across the globe.
Knowledge co-production is increasingly being promoted in the food web modeling arena given that it leads to better model fits and increased trust about model predictions (Bevilacqua et al., 2016; Bentley et al., 2019a; Fulton, 2021; Craig and Link, 2023). Yet, we found very few examples of such model development among the papers reviewed for this publication (e.g., Armada et al., 2018; Bevilacqua et al., 2019; Alms et al., 2022). However, we also champion model co-development as it can lead to additional benefits when seeking to include socio-economic drivers or expressing model outputs in socio-economic terms. First, it allows modelers to integrate the human dimensions of the system early on the modeling process, potentially reshaping how they represent the system’s ecological dimensions – so models are better at highlighting attributes that are important for a broader user base (Melbourne-Thomas et al., 2022; Barclay et al., 2023). Second, they improve our collective understanding of the socio-ecological system, particularly on how (i) different stakeholder groups operate, (ii) their management objectives, desires, and goals, (iii) the way they measure the success of management actions, and (iv) the thresholds for what they find acceptable or desirable, as well as (v) what information is available and what are the persistent data needs and data collection priorities (Galland et al., 2018; Weber et al., 2019; Barreto et al., 2020; Bennett et al., 2021; Ramírez-Monsalve et al., 2021; Bennett et al., 2022; Melbourne-Thomas et al., 2022; Partelow et al., 2023; Rodriguez-Perez et al., 2023). Third, they can help modelers, resource users and regulators to communicate better, over a shared language, (a) reducing chances of misinterpreting findings or advice (Galland et al., 2018; Weiskopf et al., 2022), (b) diminishing existing biases about the use of food web models for guiding management actions (Weijerman et al., 2018; Fulton, 2021; Craig and Link, 2023), and (c) collectively prioritizing issues that should be at the top of decision makers’ agendas (Galland et al., 2018; Weber et al., 2019; Ramírez-Monsalve et al., 2021; Bennett et al., 2022; Partelow et al., 2023; Rodriguez-Perez et al., 2023; Haugen et al., 2024). Although this is key for ecosystem-based fisheries management initiatives, its relevance might even be greater when using food web models to address ecosystem-based management pursuits – where fisheries (and fishers) are just some of many groups being affected and affecting the system, and where cumulative impact assessments need to be robust and comprehensive on the social and economic fronts (Weber et al., 2019; Bennett et al., 2022; Melbourne-Thomas et al., 2022; Weiskopf et al., 2022; Frazão Santos et al., 2023; Partelow et al., 2023; Haugen et al., 2024).
Finally, the elephant in the room: uncertainty. All models rely on assumptions and are based on incomplete knowledge and imperfect data. Yet, it is uncommon for integrated ecological-economic fisheries models (whether they have a food web model at their core or not) to produce confidence intervals or report uncertainty (Nielsen et al., 2018). In this review we found that more than half of the papers did not explicitly address uncertainty in model parametrization, structure, or outputs (Figure 7).
Not talking about uncertainty is misleading, reduces model uptake in decision-making processes and creates mistrust about model outputs across their broader user base (Weiskopf et al., 2022; Craig and Link, 2023; Rodriguez-Perez et al., 2023; Haugen et al., 2024). Effectively communicating uncertainty is essential for informed decision-making. Transparency regarding the sources and magnitude of uncertainty allows policymakers to assess the risks associated with different management options (Craig and Link, 2023; Weiskopf et al., 2022). Utilizing clear visualizations and explanations can help convey complex information on uncertainty, improving stakeholders’ confidence in model predictions and ensuring decisions account for potential variability in outcomes. We acknowledge that communicating uncertainty effectively to decision-makers and resource users is challenging and requires creativity and out-of-the-box thinking (Galland et al., 2018; Fulton, 2021; Craig and Link, 2023; Rodriguez-Perez et al., 2023; Haugen et al., 2024). However, the papers included in this review have a very different target audience. For academia, being explicit about the sources of uncertainty in our work should be as common practice as the peer review process (Schindler and Hilborn, 2015). There are numerous tools available for addressing parameter uncertainty (e.g., running sensitivity analysis; Bracis et al., 2020; Rovellini et al., 2024), structural uncertainty (e.g., promoting model comparisons; Link et al., 2012; Forrest et al., 2015; Natugonza et al., 2020; Tittensor et al., 2021; Craig and Link, 2023), and implementation error (e.g., assessing the outcomes of proposed management interventions under different implementation success scenarios; Ainsworth et al., 2012; Kaplan et al., 2012; Natugonza et al., 2020). Yet, their use was limited and mostly focused on understanding how uncertainty on the biophysical dimensions of the models (e.g., diets, abundance, sensitivity to temperature) affected model outputs (e.g., non-target group biomass, fisheries yields and revenue). Uncertainty on socio-economic parameters was only addressed explicitly in six papers (Wang et al., 2015; Wiedenmann et al., 2016; Bauer et al., 2019; Sanchirico and Essington, 2021; Apriesnig et al., 2022; Dowd et al., 2022). The effects of misrepresenting the economic components of the models in these works were significant for both ecological and socio-economic outputs. This can be quite problematic for some ensemble modeling initiatives that assume static representations (or stationary parametrizations) of the socio-economic dimensions of their models. This a challenge faced by researchers working with food web models coupled or linked to value chains, input-output models, social accounting matrices or computable general equilibrium models (Table 2). Their socio-economic components are dynamic over time and hence require that multiple models be developed (or that they are updated as new information becomes available). This is best practice for food web models (Fulton, 2021; Weiskopf et al., 2022; Craig and Link, 2023; Haugen et al., 2024) and should also be the case for all components of their ensemble modeling chain.
We have been quite critical on how food web models are being used to characterize and simulate the socio-economic consequences of change in aquatic ecosystems. However, the works reviewed for this contribution are truly great, denote that progress is taking place, and that there is room for continued improvement. We hope that this paper is a call for action. Food web models, and the community of researchers that develop them, must embrace interdisciplinarity to better represent aquatic systems and the uncertainty across all modelled domains and components. Yet, it is important to recognize that there is no “free lunch” when seeking to seriously address the human dimensions of aquatic systems in the modelling arena. Extending data gathering and monitoring programs, training personnel, co-developing models, and actively engaging in multiple stakeholder forums and policy workshops is certainly a costly endeavor. Thus, we are hopeful that this review will also be a call for action to funders to increase their support for marine social science and interdisciplinary research. We strongly believe that interdisciplinarity is the path forward for increasing trust in model outputs, uptake in decision-making processes, and using our capacity to move the dial towards more resilient marine ecosystems and just futures for those who depend on them for their livelihoods and wellbeing.
Conclusion
Ecocentric fisheries management (and ecosystem-based management) is crucial for ensuring healthy aquatic ecosystems. This also includes the humans that depend on the aquatic biota. Food web models are one of the main tools available for representing the consequences of environmental and policy changes on aquatic systems. Although research interest in these models is on the rise, as is their use in decision-making processes, models are still overlooking critical aspects of the human dimensions of systems they are seeking to represent. This systematic review provides an overview of how food web models have been used to date to characterize and simulate the socio-economic consequences of change in aquatic systems. There is a pressing need to improve our understanding of the interlinkages between the biophysical and anthropogenic components of aquatic systems. We hope this review is seen as a call for action and that the food web modeling community rises to the challenge of embracing interdisciplinarity to bridge existing knowledge silos and improve our ability to model aquatic systems across all their domains and components.
Data availability statement
The original contributions presented in the study are included in the article/Supplementary Material. Further inquiries can be directed to the corresponding author.
Author contributions
DC: Conceptualization, Data curation, Formal analysis, Investigation, Methodology, Writing – original draft, Writing – review & editing. EA: Conceptualization, Data curation, Formal analysis, Investigation, Methodology, Visualization, Writing – review & editing. SD: Conceptualization, Data curation, Formal analysis, Investigation, Methodology, Supervision, Visualization, Writing – original draft, Writing – review & editing.
Funding
The author(s) declare financial support was received for the research, authorship, and/or publication of this article. This article was written as part of the Ecocentric management for sustainable fisheries and healthy marine ecosystems project (EcoScope). EcoScope received funding from the EU’s research and innovation funding programme Horizon 2020 under Grant agreement no. 101000302.
Conflict of interest
The authors declare that the research was conducted in the absence of any commercial or financial relationships that could be construed as a potential conflict of interest.
Publisher’s note
All claims expressed in this article are solely those of the authors and do not necessarily represent those of their affiliated organizations, or those of the publisher, the editors and the reviewers. Any product that may be evaluated in this article, or claim that may be made by its manufacturer, is not guaranteed or endorsed by the publisher.
Supplementary material
The Supplementary Material for this article can be found online at: https://www.frontiersin.org/articles/10.3389/fmars.2024.1489984/full#supplementary-material
References
Abedin M. A., Collins A. E., Habiba U., Shaw R. (2019). Climate change, water scarcity, and health adaptation in southwestern coastal Bangladesh. Int. J. Disaster Risk Sci 10, 28–42. doi: 10.1007/s13753-018-0211-8
Agnetta D., Badalamenti F., Colloca F., Cossarini G., Fiorentino F., Garofalo G., et al. (2022). Interactive effects of fishing effort reduction and climate change in a central Mediterranean fishing area: Insights from bio-economic indices derived from a dynamic food-web model. Front. Mar. Sci 9. doi: 10.3389/fmars.2022.909164
Ainsworth C. H., Morzaria-Luna H. N., Kaplan I. C., Levin P. S., Fulton E. A. (2012). Full compliance with harvest regulations yields ecological benefits: Northern Gulf of California case study. J. OF Appl. Ecol 49, 63–72. doi: 10.1111/j.1365-2664.2011.02064.x
Alms V., Romagnoni G., Wolff M. (2022). Exploration of fisheries management policies in the Gulf of Nicoya (Costa Rica) using ecosystem modelling. OCEAN Coast. Manage 230, 106349. doi: 10.1016/j.ocecoaman.2022.106349
Apriesnig J. L., Warziniack T. W., Finnoff D. C., Zhang H. Y., Lee K. D., Mason D. M., et al. (2022). The consequences of misrepresenting feedbacks in coupled human and environmental models. Ecol. ECONOMICS 195, 107355. doi: 10.1016/j.ecolecon.2022.107355
Armada N. B., Bacalso R. T. M., Rosales R. M. P., Lazarte A. T. (2018). Right-sizing as a strategy for allocating fishing effort in a defined marine ecosystem: A Philippines case study. OCEAN Coast. Manage 165, 167–184. doi: 10.1016/j.ocecoaman.2018.08.018
Audzijonyte A., Pethybridge H., Porobic J., Gorton R., Kaplan I., Fulton E. A. (2019). Atlantis: A spatially explicit end-to-end marine ecosystem model with dynamically integrated physics, ecology and socio-economic modules. Methods Ecol. Evol 10, 1814–1819. doi: 10.1111/2041-210X.13272
Bacalso R. T. M., Wolff M., Rosales R. M., Armada N. B. (2016). Effort reallocation of illegal fishing operations: A profitable scenario for the municipal fisheries of Danajon Bank, Central Philippines. Ecol. Model 331, 5–16. doi: 10.1016/j.ecolmodel.2016.01.015
Barclay K. M., Bush S. R., Poos J. J., Richter A., van Zwieten P. A., Hamon K. G., et al. (2023). Social harvest control rules for sustainable fisheries. Fish Fisheries. 24, 896–905. doi: 10.1111/faf.12769
Barreto G. C., Di Domenico M., Medeiros R. P. (2020). Human dimensions of marine protected areas and small-scale fisheries management: A review of the interpretations. Mar. Policy 119, 104040. doi: 10.1016/j.marpol.2020.104040
Bauer B., Gustafsson B. G., Hyytiainen K., Meier H. E. M., Muller-Karulis B., Saraiva S., et al. (2019). Food web and fisheries in the future Baltic Sea. AMBIO 48, 1337–1349. doi: 10.1007/s13280-019-01229-3
Bellido J. M., Sumaila U. R., Sánchez-Lizaso J. L., Palomares M. L., Pauly D. (2020). Input versus output controls as instruments for fisheries management with a focus on Mediterranean fisheries. Mar. Policy 118, 103786. doi: 10.1016/j.marpol.2019.103786
Bennett N. J., Alava J. J., Ferguson C. E., Blythe J., Morgera E., Boyd D., et al. (2023). Environmental (in)justice in the anthropocene ocean. Mar. Policy 147, 105383. doi: 10.1016/j.marpol.2022.105383
Bennett N. J., Schuhbauer A., Skerritt D., Ebrahim N. (2021). Socio-economic monitoring and evaluation in fisheries. Fisheries Res 239, 105934. doi: 10.1016/j.fishres.2021.105934
Bennett N. J., Villasante S., Espinosa-Romero M. J., Lopes P. F. M., Selim S. A., Allison E. H. (2022). Social sustainability and equity in the blue economy. One Earth 5, 964–968. doi: 10.1016/j.oneear.2022.08.004
Bentley J. W., Chagaris D., Coll M., Heymans J. J., Serpetti N., Walters C. J., et al. (2024). Calibrating ecosystem models to support ecosystem-based management of marine systems. ICES J. Mar. Sci 81, 260–275. doi: 10.1093/icesjms/fsad213
Bentley J. W., Hines D. E., Borrett S. R., Serpetti N., Hernandez-Milian G., Fox C., et al. (2019a). Combining scientific and fishers’ knowledge to co-create indicators of food web structure and function. ICES J. Mar. Sci 76, 2218–2234. doi: 10.1093/icesjms/fsz121
Bentley J. W., Serpetti N., Fox C., Heymans J. J., Reid D. G. (2019b). Fishers’ knowledge improves the accuracy of food web model predictions. ICES J. Mar. Sci 76, 897–912. doi: 10.1093/icesjms/fsz003
Bevilacqua A. H. V., Angelini R., Steenbeek J., Christensen V., Carvalho A. R. (2019). Following the fish: the role of subsistence in a fish-based value chain. Ecol. ECONOMICS 159, 326–334. doi: 10.1016/j.ecolecon.2019.02.004
Bevilacqua A. H. V., Carvalho A. R., Angelini R., Christensen V. (2016). More than anecdotes: fishers’ Ecological knowledge can fill gaps for ecosystem modeling. PloS One 11, e0155655. doi: 10.1371/journal.pone.0155655
Blenckner T., Llope M., Möllmann C., Voss R., Quaas M. F., Casini M., et al. (2015). Climate and fishing steer ecosystem regeneration to uncertain economic futures. Proc. R. Soc. B: Biol. Sci 282, 20142809. doi: 10.1098/rspb.2014.2809
Bossier S., Palacz A. P., Nielsen J. R., Christensen A., Hoff A., Maar M., et al. (2018). The Baltic Sea Atlantis: An integrated end-to-end modelling framework evaluating ecosystem-wide effects of human-induced pressures. PloS One 13, e0199168. doi: 10.1371/journal.pone.0199168
Bracis C., Lehuta S., Savina-Rolland M., Travers-Trolet M., Girardin R. (2020). Improving confidence in complex ecosystem models: The sensitivity analysis of an Atlantis ecosystem model. Ecol. Model 431, 109133. doi: 10.1016/j.ecolmodel.2020.109133
Byron C. J., Jin D., Dalton T. M. (2015). An Integrated ecological-economic modeling framework for the sustainable management of oyster farming. Aquaculture 447, 15–22. doi: 10.1016/j.aquaculture.2014.08.030
Celic I., Libralato S., Scarcella G., Raicevich S., Marceta B., Solidoro C. (2018). Ecological and economic effects of the landing obligation evaluated using a quantitative ecosystem approach: a Mediterranean case study. ICES J. OF Mar. Sci 75, 1992–2003. doi: 10.1093/icesjms/fsy069
CERES. (2024). Climate change and European aquatic resources. Available online at: https://ceresproject.eu/ (Accessed July 10 2024).
Cheung W. W., Sumaila U. R. (2015). Economic incentives and overfishing: a bioeconomic vulnerability index. Mar. Ecol. Prog. Ser 530, 223–232. doi: 10.3354/meps11135
Christensen V., de la Puente S., Sueiro J. C., Steenbeek J., Majluf P. (2014). Valuing seafood: The Peruvian fisheries sector. Mar. Policy 44, 302–311. doi: 10.1016/j.marpol.2013.09.022
Christensen V., Steenbeek J., Failler P. (2011). A combined ecosystem and value chain modeling approach for evaluating societal cost and benefit of fishing. Ecol. Model 222, 857–864. doi: 10.1016/j.ecolmodel.2010.09.030
Christensen V., Walters C. J. (2004). Ecopath with Ecosim: methods, capabilities and limitations. Ecol. Model 172, 109–139. doi: 10.1016/j.ecolmodel.2003.09.003
Coll M., Ortega-Cerda M., Mascarell-Rocher Y. (2021). Ecological and economic effects of COVID-19 in marine fisheries from the Northwestern Mediterranean Sea. Biol. Conserv 255, 108997. doi: 10.1016/j.biocon.2021.108997
Colléter M., Valls A., Guitton J., Gascuel D., Pauly D., Christensen V. (2015). Global overview of the applications of the Ecopath with Ecosim modeling approach using the EcoBase models repository. Ecol. Model 302, 42–53. doi: 10.1016/j.ecolmodel.2015.01.025
Costello C., Ovando D., Clavelle T., Strauss C. K., Hilborn R., Melnychuk M. C., et al. (2016). Global fishery prospects under contrasting management regimes. Proc. Natl. Acad. Sci 113, 5125–5129. doi: 10.1073/pnas.1520420113
Craig J. K., Link J. S. (2023). It is past time to use ecosystem models tactically to support ecosystem-based fisheries management: Case studies using Ecopath with Ecosim in an operational management context. Fish Fisheries 24, 381–406. doi: 10.1111/faf.12733
CSIRO. (2024). Usage to date. Available online at: https://www.frontiersin.org/guidelines/author-guidelines (Accessed July 10 2024).
Dichmont C. M., Ellis N., Bustamante R. H., Deng R., Tickell S., Pascual R., et al. (2013). Evaluating marine spatial closures with conflicting fisheries and conservation objectives. J. OF Appl. Ecol 50, 1060–1070. doi: 10.1111/1365-2664.12110
Dowd S., Chapman M., Koehn L. E., Hoagland P. (2022). The economic tradeoffs and ecological impacts associated with a potential mesopelagic fishery in the California Current. Ecol. Appl 32, e2578. doi: 10.1002/eap.2578
Dybas C. L. (2002). In the sea, no fish is an island. BioScience 52, 124–127. doi: 10.1641/0006-3568(2002)052[0124:Itsnfi]2.0.Co;2
Ecopath Research and Development Consortium. (2024). EcoBase: a database of Ecopath with Ecosim models. Available online at: https://ecobase.ecopath.org/ (Accessed July 10 2024).
Failler P., Pan H., Akbari N. (2022). Integrated social-economic-ecological modeling for fisheries: the ECOST model. Front. Mar. Sci 8, 704371. doi: 10.3389/fmars.2021.704371
FAO. (2022). The state of world fisheries and aquaculture 2022. Towards Blue Transformation. 266. doi: 10.4060/cc0461en
Fay G., DePiper G., Steinback S., Gamble R. J., Link J. S. (2019). Economic and ecosystem effects of fishing on the northeast US shelf. Front. IN Mar. Sci 6, 133. doi: 10.3389/fmars.2019.00133
Forrest R. E., Savina M., Fulton E. A., Pitcher T. J. (2015). Do marine ecosystem models give consistent policy evaluations? A comparison of Atlantis and Ecosim. FISHERIES Res 167, 293–312. doi: 10.1016/j.fishres.2015.03.010
Frazão Santos C., Agardy T., Aheto D., Allison E. H., Bennett N. J., Blythe J. L., et al. (2023). Advancing interdisciplinary knowledge for ocean sustainability. NPJ Ocean Sustainability 2, 18. doi: 10.1038/s44183-023-00026-6
Fulton E. A. (2021). Opportunities to improve ecosystem-based fisheries management by recognizing and overcoming path dependency and cognitive bias. Fish Fisheries 22, 428–448. doi: 10.1111/faf.12537
Fulton E. A., Link J. S., Kaplan I. C., Savina-Rolland M., Johnson P., Ainsworth C., et al. (2011). Lessons in modelling and management of marine ecosystems: the Atlantis experience. Fish Fisheries 12, 171–188. doi: 10.1111/j.1467-2979.2011.00412.x
FutureMARES. (2024). Nature-based solutions and nature-inclusive harvesting for climate change adaptation. Available online at: https://www.futuremares.eu/ (Accessed 2024 July 10).
Galland G., Nickson A., Hopkins R., Miller S. (2018). On the importance of clarity in scientific advice for fisheries management. Mar. Policy 87, 250–254. doi: 10.1016/j.marpol.2017.10.029
Garcia S. M., Charles A. T. (2008). Fishery systems and linkages: Implications for science and governance. Ocean Coast. Manage 51, 505–527. doi: 10.1016/j.ocecoaman.2008.05.001
Girardin R., Hamon K. G., Pinnegar J., Poos J. J., Thébaud O., Tidd A., et al. (2017). Thirty years of fleet dynamics modelling using discrete-choice models: What have we learned? Fish Fisheries 18, 638–655. doi: 10.1111/faf.12194
Hamon K. G., Kreiss C. M., Pinnegar J. K., Bartelings H., Batsleer J., Catalán I. A., et al. (2021). Future socio-political scenarios for aquatic resources in europe: an operationalized framework for marine fisheries projections. Front. Mar. Sci 8. doi: 10.3389/fmars.2021.578516
Haugen J. B., Link J. S., Cribari K., Bundy A., Dickey-Collas M., Leslie H. M., et al. (2024). Marine ecosystem-based management: challenges remain, yet solutions exist, and progress is occurring. NPJ Ocean Sustainability 3, 7. doi: 10.1038/s44183-024-00041-1
Heijboer A., Ruess L., Traugott M., Jousset A., Ruiter P. (2018). Empirical methods of identifying and quantifying trophic interactions for constructing soil food-web models. Adaptive Food Webs: Stability and Transitions of Real and Model Ecosystems. (Cambridge University Press, Cambridge UK), 257–285. doi: 10.1017/9781316871867
Heymans J. J., Mackinson S., Sumaila U. R., Dyck A., Little A. (2011). The impact of subsidies on the ecological sustainability and future profits from north sea fisheries. PloS One 6, e20239. doi: 10.1371/journal.pone.0020239
Hodgson E. E., Kaplan I. C., Marshall K. N., Leonard J., Essington T. E., Busch D. S., et al. (2018). Consequences of spatially variable ocean acidification in the California Current: Lower pH drives strongest declines in benthic species in southern regions while greatest economic impacts occur in northern regions. Ecol. Model 383, 106–117. doi: 10.1016/j.ecolmodel.2018.05.018
Howell D., Schueller A. M., Bentley J. W., Buchheister A., Chagaris D., Cieri M., et al. (2021). Combining ecosystem and single-species modeling to provide ecosystem-based fisheries management advice within current management systems. Front. Mar. Sci 7. doi: 10.3389/fmars.2020.607831
Hyytiainen K., Bauer B., Joyce K. B., Ehrnsten E., Eilola K., Gustafsson B. G., et al. (2021). Provision of aquatic ecosystem services as a consequence of societal changes: The case of the Baltic Sea. POPULATION Ecol 63, 61–74. doi: 10.1002/1438-390X.12033
Izquierdo-Gomez D., Bayle-Sempere J. T., Arreguin-Sanchez F., Sanchez-Jerez P. (2016). Modeling population dynamics and small-scale fisheries yields of fish farming escapes in Mediterranean coastal areas. Ecol. Model 331, 56–67. doi: 10.1016/j.ecolmodel.2016.01.012
Jin D., Hoagland P., Dalton T. M., Thunberg E. M. (2012). Development of an integrated economic and ecological framework for ecosystem-based fisheries management in New England. Prog. Oceanography 102, 93–101. doi: 10.1016/j.pocean.2012.03.007
Jouffray J.-B., Blasiak R., Norström A. V., Österblom H., Nyström M. (2020). The blue acceleration: the trajectory of human expansion into the ocean. One Earth 2, 43–54. doi: 10.1016/j.oneear.2019.12.016
Kaplan I. C., Horne P. J., Levin P. S. (2012). Screening California Current fishery management scenarios using the Atlantis end-to-end ecosystem model. Prog. Oceanography 102, 5–18. doi: 10.1016/j.pocean.2012.03.009
Kaplan I. C., Leonard J. (2012). From krill to convenience stores: Forecasting the economic and ecological effects of fisheries management on the US West Coast. Mar. Policy 36, 947–954. doi: 10.1016/j.marpol.2012.02.005
Keramidas I., Dimarchopoulou D., Ofir E., Scotti M., Tsikliras A. C., Gal G. (2023). Ecotrophic perspective in fisheries management: a review of Ecopath with Ecosim models in European marine ecosystems. Front. Mar. Sci 10. doi: 10.3389/fmars.2023.1182921
Koehn L. E., Essington T. E., Marshall K. N., Sydeman W. J., Szoboszlai A. I., Thayer J. A. (2017). Trade-offs between forage fish fisheries and their predators in the California Current. ICES J. Mar. Sci 74, 2448–2458. doi: 10.1093/icesjms/fsx072
Kreiss C. M., Papathanasopoulou E., Hamon K. G., Pinnegar J. K., Rybicki S., Micallef G., et al. (2020). Future socio-political scenarios for aquatic resources in europe: an operationalized framework for aquaculture projections. Front. Mar. Sci 7. doi: 10.3389/fmars.2020.568159
Lee K., Apriesnig J., Zhang H. Y. (2021). Socio-ecological outcomes of single-species fisheries management: the case of yellow perch in lake erie. Front. IN Ecol. AND Evol 9, 703813. doi: 10.3389/fevo.2021.703813
Link J. S., Ihde T. F., Harvey C. J., Gaichas S. K., Field J. C., Brodziak J. K. T., et al. (2012). Dealing with uncertainty in ecosystem models: The paradox of use for living marine resource management. Prog. Oceanography 102, 102–114. doi: 10.1016/j.pocean.2012.03.008
Lofgren H., Harris R. L., Robinson S. (2002). A standard computable general equilibrium (CGE) model in GAMS. Microcomputers in Policy Research. (Washington, D.C., USA: International Food Policy Research Institute), 5, 69. Available online at: https://www.un.org/en/development/desa/policy/mdg_workshops/training_material/lofgren_lee_and_robinson_2002.pdf.
Mainar-Causapé A. J., Ferrari E., McDonald S. (2018). Social accounting matrices: basic aspects and main steps for estimation. (Luxembourg: Publications Office Eur. Union). doi: 10.2760/010600
Marshall K. N., Levin P. S., Essington T. E., Koehn L. E., Anderson L. G., Bundy A., et al. (2018). Ecosystem-based fisheries management for social–ecological systems: renewing the focus in the United States with next generation fishery ecosystem plans. Conserv. Lett 11, e12367. doi: 10.1111/conl.12367
Melbourne-Thomas J., Tommasi D., Gehlen M., Murphy E. J., Beckensteiner J., Bravo F., et al. (2022). Integrating human dimensions in decadal-scale prediction for marine social–ecological systems: lighting the grey zone. ICES J. Mar. Sci 80, 16–30. doi: 10.1093/icesjms/fsac228
Memmott J. (2009). Food webs: a ladder for picking strawberries or a practical tool for practical problems? Philos. Trans. R. Soc. B: Biol. Sci 364, 1693–1699. doi: 10.1098/rstb.2008.0255
Michael-Bitton G., Gal G., Corrales X., Ofir E., Shechter M., Zemah-Shamir S. (2022). Economic aspects of fish stock accounting as a renewable marine natural capital: The Eastern Mediterranean continental shelf ecosystem as a case study. Ecol. ECONOMICS 200, 107539. doi: 10.1016/j.ecolecon.2022.107539
Moher D., Shamseer L., Clarke M., Ghersi D., Liberati A., Petticrew M., et al. (2015). Preferred reporting items for systematic review and meta-analysis protocols (PRISMA-P) 2015 statement. Systematic Rev 4, 1–9. doi: 10.1186/2046-4053-4-1
Morzaria-Luna H. N., Ainsworth C. H., Kaplan I. C., Levin P. S., Fulton E. A. (2013). Indirect effects of conservation policies on the coupled human-natural ecosystem of the upper gulf of california. PloS One 8, e64085. doi: 10.1371/journal.pone.0064085
Natugonza V., Ainsworth C., Sturludottir E., Musinguzi L., Ogutu-Ohwayo R., Tomasson T., et al. (2020). Simulating trade-offs between socio-economic and conservation objectives for Lake Victoria (East Africa) using multispecies, multifleet ecosystem models. FISHERIES Res 229, 105593. doi: 10.1016/j.fishres.2020.105593
Nielsen J. R., Thunberg E., Holland D. S., Schmidt J. O., Fulton E. A., Bastardie F., et al. (2018). Integrated ecological–economic fisheries models—Evaluation, review and challenges for implementation. Fish Fisheries 19, 1–29. doi: 10.1111/faf.12232
Ouréns R., Melnychuk M. C., Crowder L. B., Gutierrez N. L., Hilborn R., Pita C., et al. (2022). Linking small-scale fisheries performance to governance attributes: A quantitative assessment from stakeholders' perceptions in the Americas and Europe. Mar. Policy 136, 104876. doi: 10.1016/j.marpol.2021.104876
Partelow S., Schlüter A., Ban N. C., Batterbury S., Bavinck M., Bennett N. J., et al. (2023). Five social science intervention areas for ocean sustainability initiatives. NPJ Ocean Sustainability 2, 24. doi: 10.1038/s44183-023-00032-8
Patrick W. S., Link J. S. (2015). Myths that continue to impede progress in ecosystem-based fisheries management. Fisheries 40, 155–160. doi: 10.1080/03632415.2015.1024308
Pimm S. L., Lawton J. H., Cohen J. E. (1991). Food web patterns and their consequences. Nature 350, 669–674. doi: 10.1038/350669a0
Pinnegar J. K., Blanchard J. L., Mackinson S., Scott R. D., Duplisea D. E. (2005). Aggregation and removal of weak-links in food-web models: system stability and recovery from disturbance. Ecol. Model 184, 229–248. doi: 10.1016/j.ecolmodel.2004.09.003
Plagányi É. E. (2007). Models for an ecosystem approach to fisheries. FAO Fisheries Technical Paper. (Rome, Italy) 477, 108. Available online at: https://www.fao.org/4/a1149e/a1149e.pdf.
Plummer M. L., Harvey C. J., Anderson L. E., Guerry A. D., Ruckelshaus M. H. (2013). The role of eelgrass in marine community interactions and ecosystem services: results from ecosystem-scale food web models. ECOSYSTEMS 16, 237–251. doi: 10.1007/s10021-012-9609-0
Proops J. L., Safonov P. (2004). Modelling in ecological economics (Cheltenham, Gloucestershire, UK: Edward Elgar Publishing Limited), 224.
Ramirez A., Ortiz M., Steenbeek J., Christensen V. (2015). Evaluation of the effects on rockfish and kelp artisanal fisheries of the proposed Mejillones Peninsula marine protected area (northern Chile, SE Pacific coast). Ecol. Model 297, 141–153. doi: 10.1016/j.ecolmodel.2014.11.012
Ramírez-Monsalve P., Nielsen K. N., Ballesteros M., Kirkfeldt T. S., Dickey-Collas M., Delaney A., et al. (2021). Pulling mechanisms and pushing strategies: How to improve Ecosystem Approach Fisheries Management advice within the European Union’s Common Fisheries Policy. Fisheries Res 233, 105751. doi: 10.1016/j.fishres.2020.105751
Ramírez-Monsalve P., Raakjær J., Nielsen K. N., Santiago J. L., Ballesteros M., Laksá U., et al. (2016). Ecosystem Approach to Fisheries Management (EAFM) in the EU – Current science–policy–society interfaces and emerging requirements. Mar. Policy 66, 83–92. doi: 10.1016/j.marpol.2015.12.030
Rehren J., Wolff M., Jiddawi N. (2018). Holistic assessment of Chwaka Bay's multi-gear fishery - Using a trophic modeling approach. J. OF Mar. Syst 180, 265–278. doi: 10.1016/j.jmarsys.2018.01.002
Rodriguez-Perez A., Tsikliras A. C., Gal G., Steenbeek J., Falk-Andersson J., Heymans J. J. (2023). Using ecosystem models to inform ecosystem-based fisheries management in Europe: a review of the policy landscape and related stakeholder needs. Front. Mar. Sci 10. doi: 10.3389/fmars.2023.1196329
Rovellini A., Punt A. E., Bryan M. D., Kaplan I. C., Dorn M. W., Aydin K., et al. (2024). Linking climate stressors to ecological processes in ecosystem models, with a case study from the Gulf of Alaska. ICES J. Mar. Sci. 1–13. doi: 10.1093/icesjms/fsae002
Russo T., Pulcinella J., Parisi A., Martinelli M., Belardinelli A., Santojanni A., et al. (2015). Modelling the strategy of mid-water trawlers targeting small pelagic fish in the Adriatic Sea and its drivers. Ecol. Model 300, 102–113. doi: 10.1016/j.ecolmodel.2014.12.001
Sanchirico J. N., Essington T. E. (2021). Direct and ancillary benefits of ecosystem-based fisheries management in forage fish fisheries. Ecol. Appl 31, e02421. doi: 10.1002/eap.2421
Schadeberg A., Kraan M., Hamon K. G. (2021). Beyond métiers: social factors influence fisher behaviour. ICES J. Mar. Sci 78, 1530–1541. doi: 10.1093/icesjms/fsab050
Schindler D. E., Hilborn R. (2015). Prediction, precaution, and policy under global change. Science 347, 953–954. doi: 10.1126/science.1261824
Schwermer H., Barz F., Zablotski Y. (2020). “A literature review on stakeholder participation in coastal and marine fisheries,” in YOUMARES 9-the oceans: our research, our future: proceedings of the 2018 conference for YOUng MArine RESearcher in oldenburg (Springer International Publishing, Germany), 21–43.
Scotti M., Opitz S., MacNeil L., Kreutle A., Pusch C., Froese R. (2022). Ecosystem-based fisheries management increases catch and carbon sequestration through recovery of exploited stocks: The western Baltic Sea case study. Front. Mar. Sci 9. doi: 10.3389/fmars.2022.879998
Simons S. L., Döring R., Temming A. (2014). Modelling the spatio-temporal interplay between North Sea saithe (Pollachius virens) and multiple fleet segments for management evaluation. Aquat. Living Resour 27, 1–16. doi: 10.1051/alr/2014003
Steel P., Fariborzi H., Hendijani R. (2023). An application of modern literature review methodology: finding needles in ever-growing haystacks. (London, UK: SAGE Publications Ltd), 18. doi: 10.4135/9781529667417
Stephenson R. L., Benson A. J., Brooks K., Charles A., Degnbol P., Dichmont C. M., et al. (2017). Practical steps toward integrating economic, social and institutional elements in fisheries policy and management. ICES J. Mar. Sci 74, 1981–1989. doi: 10.1093/icesjms/fsx057
Thébaud O., Nielsen J. R., Motova A., Curtis H., Bastardie F., Blomqvist G. E., et al. (2023). Integrating economics into fisheries science and advice: progress, needs, and future opportunities. ICES J. Mar. Sci 80, 647–663. doi: 10.1093/icesjms/fsad005
Thorson J. T., Adams G., Holsman K. (2019). Spatio-temporal models of intermediate complexity for ecosystem assessments: A new tool for spatial fisheries management. Fish Fisheries 20, 1083–1099. doi: 10.1111/faf.12398
Tittensor D. P., Novaglio C., Harrison C. S., Heneghan R. F., Barrier N., Bianchi D., et al. (2021). Next-generation ensemble projections reveal higher climate risks for marine ecosystems. Nat. Climate Change 11, 973–981. doi: 10.1038/s41558-021-01173-9
Tunca S., Lindegren M., Ravn-Jonsen L., Lindroos M. (2019). Cooperative fisheries outperform non-cooperative ones in the baltic sea under different climate scenarios. Front. Mar. Sci 6. doi: 10.3389/fmars.2019.00622
Ulrich C., Wilson D. C. K., Nielsen J. R., Bastardie F., Reeves S. A., Andersen B. S., et al. (2012). Challenges and opportunities for fleet- and métier-based approaches for fisheries management under the European Common Fishery Policy. Ocean Coast. Manage 70, 38–47. doi: 10.1016/j.ocecoaman.2012.06.002
Uusitalo L., Blenckner T., Puntila-Dodd R., Skytta A., Jernberg S., Voss R., et al. (2022). Integrating diverse model results into decision support for good environmental status and blue growth. Sci. OF THE TOTAL Environ 806, 150450. doi: 10.1016/j.scitotenv.2021.150450
Viet Anh P., De Laender F., Everaert G., Tien Vinh C., Goethals P. (2014). An integrated food web model to test the impact of fisheries management scenarios on the coastal ecosystem of Vietnam. Ocean Coast. Manage 92, 74–86. doi: 10.1016/j.ocecoaman.2014.02.003
Villasante S., Gianelli I., Castrejón M., Nahuelhual L., Ortega L., Sumaila U. R., et al. (2022). Social-ecological shifts, traps and collapses in small-scale fisheries: Envisioning a way forward to transformative changes. Mar. Policy 136, 104933. doi: 10.1016/j.marpol.2021.104933
Voss R., Quaas M., Neuenfeldt S. (2022). Robust, ecological-economic multispecies management of Central Baltic fishery resources. ICES J. Mar. Sci 79, 169–181. doi: 10.1093/icesjms/fsab251
Walters C., Martell J. (2004). Temporal and spatial dynamics of fishing effort. Fisheries Ecol. Management. (Princeton USA: Princeton Univ. Press), 200–227. Available online at: https://api.pageplace.de/preview/DT0400.9780691214634_A39698941/preview-9780691214634_A39698941.pdf.
Wang Y., Hu J. F., Pan H. R., Li S. Y., Failler P. (2016). An integrated model for marine fishery management in the Pearl River Estuary: Linking socio-economic systems and ecosystems. Mar. Policy 64, 135–147. doi: 10.1016/j.marpol.2015.11.014
Wang Y., Hu J. F., Pan H. R., Pallier P. (2020). Ecosystem-based fisheries management in the Pearl River Delta: Applying a computable general equilibrium model. Mar. Policy 112, 103784. doi: 10.1016/j.marpol.2019.103784
Wang Y., Li S. Y., Duan L. J., Liu Y. (2012). Fishery policy exploration in the Pearl River Estuary based on an Ecosim model. Ecol. Model 230, 34–43. doi: 10.1016/j.ecolmodel.2012.01.017
Wang Y., Pan H., Li S., Failler P. (2015). An integrated socio-economic and ecological framework for evaluating the societal costs and benefits of fishing activities in the Pearl River delta. Open J. Mar. Sci 5, 477–497. doi: 10.4236/ojms.2015.54038
Wang T., Szuwalski C. S., Punt A. E., Hilborn R. (2024). Diversity of fishing strategies and high spatial adaptivity in the Alaskan snow crab fishery. ICES J. Mar. Sci 81, 929–943. doi: 10.1093/icesjms/fsae052
Weber C. T., Borit M., Aschan M. (2019). An interdisciplinary insight into the human dimension in fisheries models. A systematic literature review in a european union context. Front. Mar. Sci 6, 369. doi: 10.3389/fmars.2019.00369
Weijerman M., Gove J. M., Williams I. D., Walsh W. J., Minton D., Polovina J. J. (2018). Evaluating management strategies to optimise coral reef ecosystem services. J. OF Appl. Ecol 55, 1823–1833. doi: 10.1111/1365-2664.13105
Weijerman M., Grace-McCaskey C., Grafeld S. L., Kotowicz D. M., Oleson K. L. L., van Putten I. E. (2016). Towards an ecosystem-based approach of Guam's coral reefs: The human dimension. Mar. Policy 63, 8–17. doi: 10.1016/j.marpol.2015.09.028
Weijerman M., Oyafuso Z. S., Leong K. M., Oleson K. L. L., Winston M. (2021). Supporting Ecosystem-based Fisheries Management in meeting multiple objectives for sustainable use of coral reef ecosystems. ICES J. OF Mar. Sci 78, 2999–3011. doi: 10.1093/icesjms/fsaa194
Weiskopf S. R., Harmáčková Z. V., Johnson C. G., Londoño-Murcia M. C., Miller B. W., Myers B. J. E., et al. (2022). Increasing the uptake of ecological model results in policy decisions to improve biodiversity outcomes. Environ. Model. Software 149, 105318. doi: 10.1016/j.envsoft.2022.105318
Keywords: food web models, fisheries bioeconomic models, integrated ecological-economic fisheries models, comparative model evaluation, trade-off analyses, model uncertainty
Citation: Chakravorty D, Armelloni EN and de la Puente S (2024) A systematic review on the use of food web models for addressing the social and economic consequences of fisheries policies and environmental change. Front. Mar. Sci. 11:1489984. doi: 10.3389/fmars.2024.1489984
Received: 02 September 2024; Accepted: 09 December 2024;
Published: 20 December 2024.
Edited by:
Jeroen Gerhard Steenbeek, Ecopath International Initiative Research Association, SpainReviewed by:
Sebastian Villasante, University of Santiago de Compostela, SpainDiana L. Stram, North Pacific Fishery Management Council, United States
Copyright © 2024 Chakravorty, Armelloni and de la Puente. This is an open-access article distributed under the terms of the Creative Commons Attribution License (CC BY). The use, distribution or reproduction in other forums is permitted, provided the original author(s) and the copyright owner(s) are credited and that the original publication in this journal is cited, in accordance with accepted academic practice. No use, distribution or reproduction is permitted which does not comply with these terms.
*Correspondence: Diya Chakravorty, ZGl5YS5jaGFrcmF2b3J0eUBuaXZhLm5v