- 1Department of Atmosphere, Ocean and Earth System Modeling Research, Meteorological Research Institute, Japan Meteorological Agency, Tsukuba, Japan
- 2Department of Interdisciplinary Statistical Mathematics,The Institute of Statistical Mathematics, Tachikawa, Japan
- 3Research and Development Department, Mercator Ocean International, Ramonville-Saint-Agne, France
- 4Research Department, European Centre for Medium-Range Weather Forecasts, Reading, United Kingdom
- 5Application Laboratory, Japan Agency for Marine-Earth Science and Technology, Yokohama, Japan
- 6Ocean Forecasting Research and Development, Met Office, Exeter, United Kingdom
- 7Environmental Numerical Research Division, Environment and Climate Change Canada, Dorval, QC, Canada
- 8Meteorological Service of Canada, Environment and Climate Change Canada, Dorval, QC, Canada
“Synergistic Observing Network for Ocean Prediction (SynObs)” was launched in 2022 as a project of the United Nations Decade of Ocean Science for Sustainable Development to evaluate the importance of ocean observation systems and co-design the future evolution of the ocean observing network. SynObs is currently leading the flagship OSEs/OSSEs, an internationally coordinated activity in which observing system experiments (OSEs) and observing system simulation experiments (OSSEs) are conducted using a variety of ocean and coupled atmosphere–ocean prediction systems to evaluate ocean observation impacts consistent across most prediction systems. The flagship OSEs/OSSEs comprises the ocean prediction (OP) OSEs for high-resolution ocean predictions, the subseasonal-to-seasonal (S2S) OSEs for long-term lead-time coupled ocean–atmosphere predictions, and the OP OSSEs for evaluating new and future observing systems. SynObs plans to use the results of the flagship OSEs to contribute to the reports on the ocean observing network design made by international organizations and projects. Here, we introduce this initiative, and we report on some initial results. Some observation impacts consistent across four ocean prediction systems are found by a preliminary analysis of the analysis runs for the OP OSEs. For example, impacts of the altimetry data on the assimilated sea surface height (SSH) field are generally large in the westerly boundary current regions and around Antarctic Circumpolar Currents where SSH has large variability but are small in the tropical regions, despite the relatively large SSH variability there. The analysis also indicates the possibility that there are some characteristic differences in the observation impacts between low-resolution and eddy-resolving ocean prediction systems. Although OSE outputs of only four ocean prediction systems are available now, we will make further investigation, adding OSE outputs of other prediction systems that will be submitted in the near future.
1 Introduction
Ocean observation data are essential for ocean predictions (e.g., Davidson et al., 2019) and also contribute to weather and climate predictions based on coupled atmosphere–ocean models, especially at subseasonal and longer time scales (e.g., Subramanian et al., 2019). However, developing and sustaining the ocean observation network requires a huge amount of human and financial resources. Therefore, the ocean observation network should be designed to efficiently acquire effective observation data, and their adequacy should be continually evaluated (e.g., Fujii et al., 2019). Especially nowadays, many ocean observing systems (e.g., the global Argo array, mooring arrays, ocean observing satellites, ocean gliders, and autonomous underwater vehicles) require scientific support for their usefulness in order to sustain them.
There are several strategies to demonstrate the importance of observation data and to scientifically support the maintenance and expansion of the ocean observation network. Among them, observing system experiments (OSEs) are widely used in order to evaluate the importance of the ocean observation data and the effectiveness of ocean observing systems for ocean predictions (e.g., Vidard et al., 2007; Balmaseda et al., 2009; Oke and Schiller, 2007; Lea et al., 2014; Fujii et al., 2015a; Turpin et al., 2016; Xue et al., 2017). OSEs are data assimilation and prediction experiments in which specific observation types are excluded from or added to the data being assimilated, and the impact of the observation types is assessed by comparing it with regular data assimilation and prediction. Observing system simulation experiments (OSSEs), which are the same as OSEs but use synthetic observation data generated from a reference simulation instead of real observation data, are also performed for evaluating the future observing system or proposing a new design of an observation network (e.g., Halliwell et al., 2017; Bonaduce et al., 2018; Gasparin et al., 2020; Gasparin et al., 2023; Waters et al., 2024). However, the results of OSEs and OSSEs severely depend on the property of the system, including systematic errors of the system (i.e., model biases), physical parameterizations, and data assimilation schemes applied in the system, as demonstrated in previous studies (e.g., Fujii et al., 2015b, 2019; Gasparin et al., 2019). Therefore, it is preferable to conduct the OSEs/OSSEs using multiple prediction systems in order to mitigate the differences in evaluations caused by the system dependency and to draw robust and reliable conclusions.
The United Nations (UN) Decade of Ocean Science for Sustainable Development, that is, the UN Ocean Decade (UNESCO-IOC, 2021; see also https://oceandecade.org/), provides a good opportunity to make a close collaboration between ocean observing and ocean and coupled prediction communities, as well as among various groups in the ocean prediction community, for evaluating the importance of ocean observation systems and co-designing the future evolution of the ocean observing network. “Synergistic Observing Network for Ocean Prediction (SynObs)” was launched as a UN Ocean Decade Project in 2022 to take advantage of this collaborative opportunity (see https://oceanpredict.org/un-decade-of-ocean-science/synobs-2/). SynObs is currently coordinating the flagship OSEs/OSSEs, a collaborative OSEs/OSSEs using multiple ocean and subseasonal-to-seasonal (S2S) prediction systems to make robust and reliable evaluations that are seen across a variety of substantially varying ocean data assimilation and prediction systems. The flagship OSEs/OSSEs has the following five goals. The first is to demonstrate the importance of major ocean observing systems [Argo array, tropical mooring arrays, satellite altimetry, Surface Water and Ocean Topography (SWOT) mission, etc.] for ocean and coupled predictions. The second is to evaluate the synergy among different observing systems, typically between satellite and in situ observing systems, in ocean predictions. The third is to contribute to the future design of the ocean observing network to improve its effectiveness and efficiency. The fourth is to build a coordinated framework to co-design the future ocean observing network among ocean observing and prediction communities. The fifth is, then, to establish the best practice to evaluate the current ocean observing network and to design its future evolution.
The SynObs flagship OSEs/OSSEs comprises ocean prediction (OP) OSEs, OP OSSEs, and S2S OSEs. The OP OSEs are designed to evaluate the impacts of existing observing systems on ocean predictions, particularly those made by relatively high-resolution ocean data assimilation and prediction systems. The period of data assimilation (analysis) runs for the OP OSEs is at least 1 year during 2020 and preferably extended to 3 years until 2022 (with the possibility of further extension in the future). It is also encouraged to perform 10-day forecast runs for the same period, with particular priority given to the period from June 2021 to May 2022. The OP OSSEs are designed to evaluate new or future observing systems and the observing network design on ocean predictions. For example, we aim to evaluate SWOT data and the new Tropical Pacific Observing System (TPOS) design (Kessler et al., 2019; Kessler and Cravatte, 2021) here. It is planned to perform assimilation runs for approximately 1 year and 10-day forecast runs from the assimilation results. For the OP OSSEs, the use of relatively high-resolution ocean data assimilation and prediction systems is also expected, and the GEOS/NASA high-resolution coupled atmosphere–ocean simulation of approximately 1 year (Menemenlis, 2023) will be used as the Nature Run from which virtual observation data will be synthesized. The S2S OSEs are designed to evaluate observation impacts on atmosphere and ocean predictions at S2S timescales. They cover a long analysis period (2003–2022, with possible extension to 2023), and execution of 1-month or 4-month forecasts with coupled ocean–atmosphere models is also encouraged. Output data from the analysis and forecast runs performed for the flagship OSEs/OSSEs will be collected and shared as the SynObs common database with volunteer groups responsible for the diagnostics and will be eventually publicly available to the broader scientific community.
This paper presents the outline of the OP OSE and the early results from analyzing the OSE output of analysis runs made by multiple systems. We then discuss future perspectives on SynObs and the flagship OSEs/OSSEs activities.
2 Outline of the OP OSEs included in the SynObs flagship OSEs/OSSEs
SynObs is currently coordinating the OP OSEs as part of the flagship OSEs/OSSEs in order to evaluate the impacts of the ocean observing network on ocean prediction systems using relatively high-resolution (typically finer than 0.1°) ocean models that resolve mesoscale eddies. For this purpose, prediction centers are requested to perform ocean data assimilation runs with control and observation denial settings listed in Table 1. It is also encouraged to perform ocean forecast runs from the oceanic initial condition reproduced by the assimilation runs using their ocean prediction systems.
As for the data assimilation runs, prediction centers are requested to provide outputs of ocean variables and diagnostics for at least 1 year from Jan. 1 to Dec. 31, 2020, and preferably for 3 years until Dec. 20, 2022. The assimilation runs are recommended to be started at least 3 months before the beginning of the output period mentioned above from the ocean state generated by regular data assimilation or free simulation in order to allow sufficient spin-up time.
Prediction centers are, then, requested to perform forecast runs from the end of every pentad in 2020–2022 from the oceanic initial condition generated by the above assimilation runs with each OSE setting. The requested forecast length is 10 days. It is recommended that the forecast runs in the period from Jun. 5, 2021, to May 31, 2022, be prioritized over the forecast runs in other periods, as mentioned in the previous section. Ideally, the ocean model should be forced by the results of atmospheric forecasts in the ocean forecast run if the model is not coupled to any atmospheric models. However, atmospheric forcing calculated from atmospheric reanalysis data can be used in the forecast run if the atmospheric forecast data are not available.
Outputs of the data assimilation runs and forecasts run with the OSE settings conducted in prediction centers are converted to data in a common regular latitude–longitude coordinate grid. Some variables at the times and locations of observations by Argo floats and mooring buoys are also output. The variables and diagnostics to be output, their resolution, and time intervals have been discussed and agreed upon. The output data are, then, collected and compiled in the SynObs database, as well as S2S OSE and OP OSSE outputs, and will be eventually open to the public. SynObs plans to ask several volunteer diagnostic groups of experts in specific observing systems, target regions, phenomena, applications, etc., to examine the impacts of observation data on several targeted variables or diagnostics (typically, the difference in the variables/diagnostics among OSEs). The diagnostic groups will mainly use the database and conduct the diagnostics focusing on their own expertise.
Details of the OSE settings listed in Table 1 are given below. CNTL is the reference run in the set of OSEs. In CNTL, Sea Surface Temperature (SST) data, 80% of Argo data, mooring data, other in situ temperature and salinity profile data, and data from satellite nadir altimeters are assimilated. Other types of observation data (e.g., sea ice concentration ratio) can also be assimilated in some systems if they are used in the operation. Other settings of the model and assimilation system can be different from their operational settings. It is noted that 20% of the Argo data are withheld from assimilation in order to use as independent reference Argo data for validation. Which data are withheld is determined by the World Meteorological Organization (WMO) number of the floats, that is, the data for the floats whose last digit of the WMO number is 8 or 9 are withheld. With this method, the common reference Argo data are easily identified among prediction centers, and they are expected to distribute homogeneously in the global ocean as shown in Figure 1 (also see Fujii et al., 2015a). It is recommended that the World Ocean Database (WOD; Boyer et al., 2016; Locarnini et al., 2013; Zweng et al., 2013) be used to collect the in situ temperature and salinity (TS) profiles, which will be assimilated because Argo, mooring, and other profiles are already sorted in different files, and thus, it is easy to identify Argo and mooring profiles. However, it is approved to use other datasets if necessary.
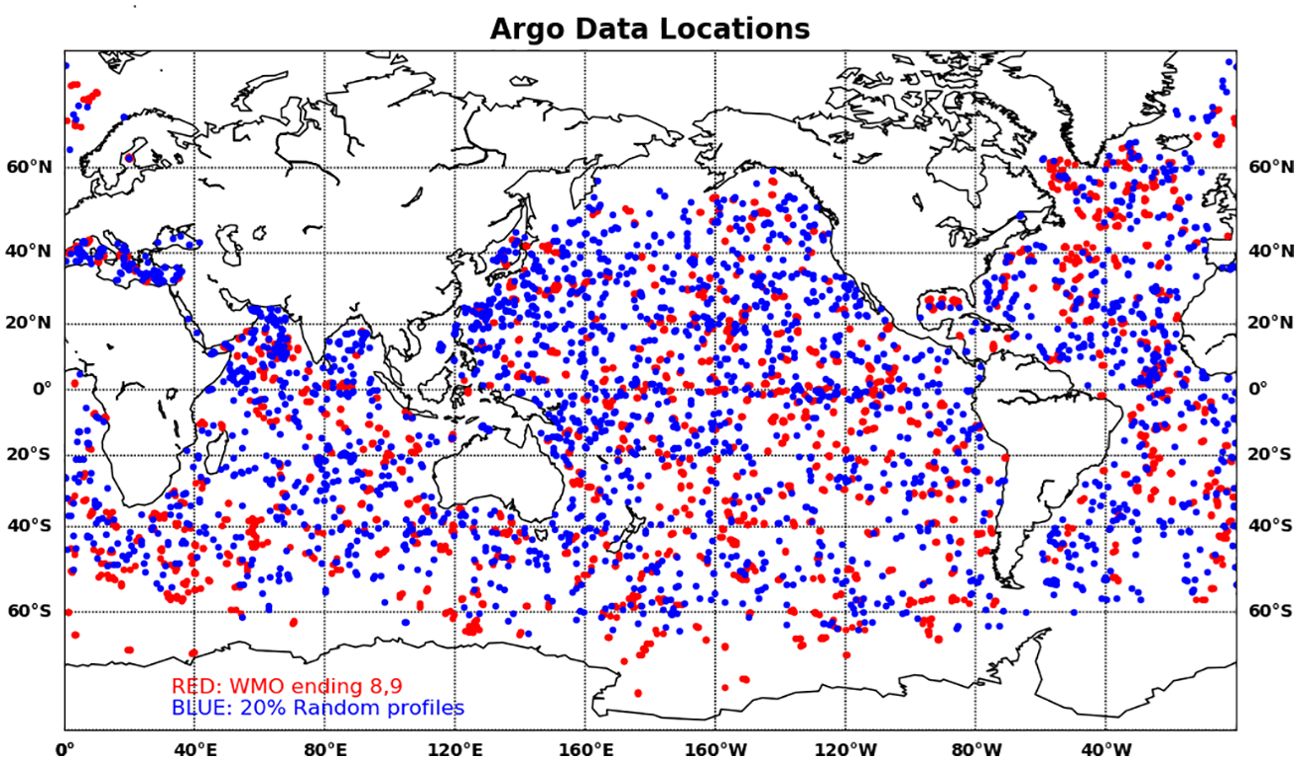
Figure 1. Distributions of the Argo floats whose last digits of WMO number are 8 or 9 (red) and 20% random profiles (blue). Example for January 2020. TS profiles observed by the Argo floats whose last digits of WMO number are 8 or 9 will be withheld in all OSEs and used for the validation as the reference data. WMO, World Meteorological Organization; TS, temperature and salinity; OSEs, observing system experiments.
In the following five OSEs, specific types of observations are excluded from the data to be assimilated. The impact of that observation type will be assessed by comparing the specific OSE with CNTL, where all observation types are assimilated. The impact of the satellite nadir altimeter data is assessed using NoAlt, in which the altimeter data are excluded. The impact of Argo floats is assessed using NoArgo, in which all TS profiles observed by Argo floats are excluded. The impact of moorings, which are mainly in the tropical ocean, is assessed using NoMoor, in which all TS profiles observed by moorings are excluded. The impact of SST data, which are mainly observed by satellites, is assessed using NoSST, in which SST data are excluded. Then, the impact of all in situ data is assessed using NoInsitu, in which all kinds of in situ TS profiles are excluded.
SSTonly is implemented for checking the performance of the system when only SST data are ingested. SSTonly is performed with the same setting as CNTL, but only SST data are assimilated. Free is implemented for checking the model performance without data assimilation and is performed with the same setting of the ocean model as CNTL and without any procedures related to data assimilation (including climatological nudging and bias correction). In Free, information related to the actual ocean condition only comes from atmospheric forcing. HalfArgo is implemented for evaluating the impact of changing the density of Argo floats and is performed with the same setting as CNTL, but the number of Argo data being assimilated is reduced from 80% to 40%. The data for floats whose last digit of the WMO number is 4, 5, 6, 7, 8, or 9 are withheld. Oper is the result of data assimilation runs with identical settings as used in the operational system and provided for checking the performance of the actual operational systems.
Table 1 lists OSEs following their general priority order. However, it is left up to each prediction center to decide the order of implementing the OSEs on their own according to their own priority. It should also be mentioned that the OSE settings listed in Table 1 are common with those for S2S OSEs. Therefore, some prediction centers are currently conducting ocean reanalyses with the OSE settings for 2003–2022.
3 Early results of the OP OSEs
In this section, we present early evaluation results of OP OSEs using the output of the data assimilation runs of the four systems (FOAM, GIOPS, MOVE-G3, and JCOPE-FGO) with the CNTL, NoAlt, and NoArgo settings. A brief summary of the configuration of the four prediction systems is presented in Table 2, and further descriptions of those systems are given in the Supplementary Material. Detailed descriptions of the diagnostics used for evaluating observation impacts in this section are also provided in the Supplementary Material.
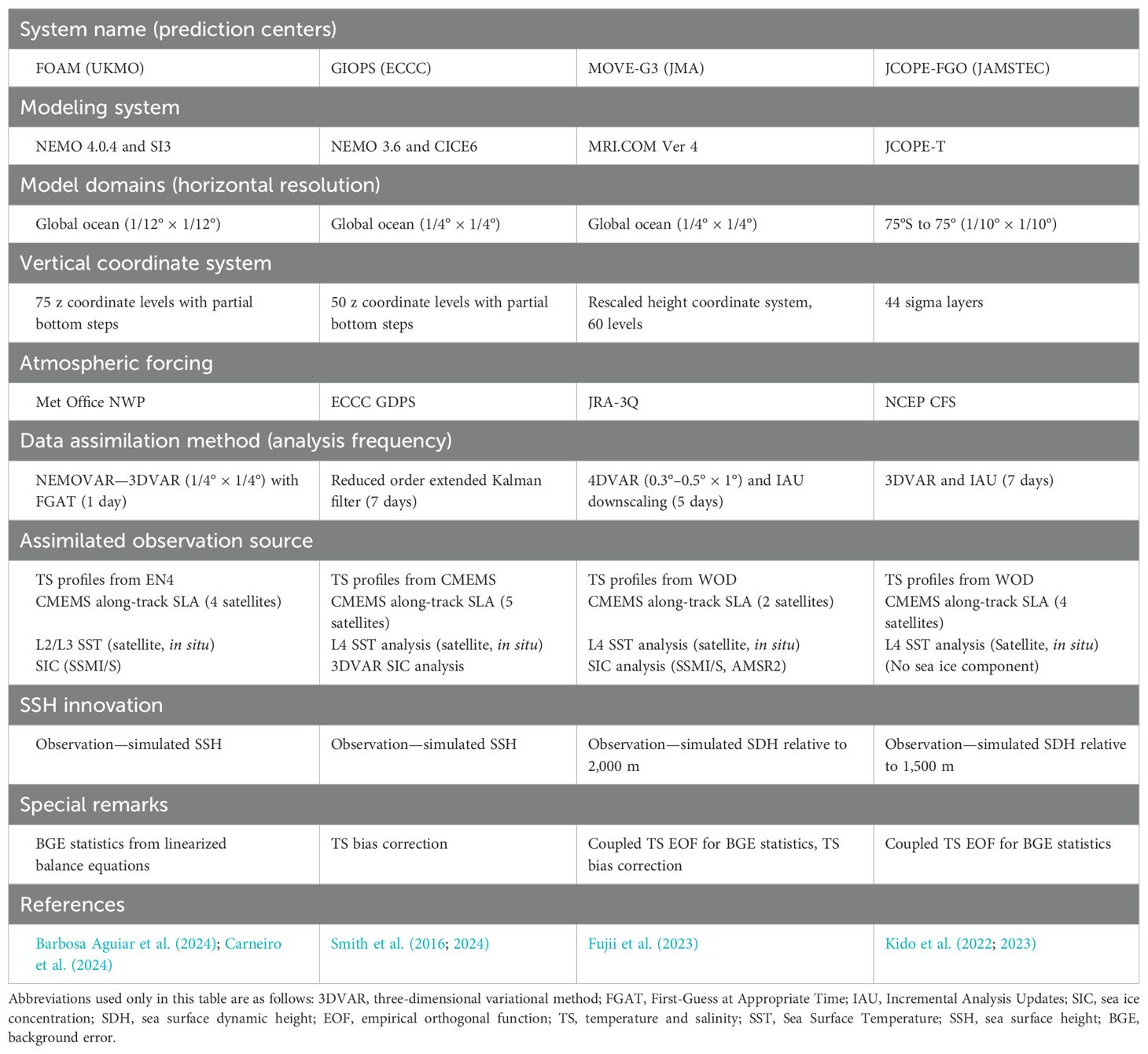
Table 2. Brief summary of the configurations of the prediction systems used in the observation impact evaluations in Section 3.
3.1 Evaluation using SSH outputs
First, we compare how sea surface height (SSH) changes when the altimetry or the Argo data are withheld in the four systems. Figure 2B shows that the root mean square difference (RMSD) of SSH between NoAlt and CNTL is relatively large in the western boundary current regions, including areas around the Kuroshio, its extension, the Gulf Stream, the Agulhas Current, the seas east of Australia and Argentina, and the area around the Antarctic Circumpolar Current (ACC). In these areas, SSH has large variability as shown in Figure 2A, and the variability often causes large deviations of simulated SSH from the altimetry data. Therefore, assimilating the altimetry data tends to make relatively large SSH changes in these areas. In contrast, the band along 15°N in the eastern subtropical North Pacific and the band near the equator in the Indian Ocean show relatively large SSH variability but are not significantly affected by the altimetry data in all systems. One possible reason for this is that the large variability in these bands is generated primarily by wind forcing and can be mostly reproduced by the model without assimilating the altimetry data, although the SSH variations are still likely subject to uncertainties in the atmospheric forcing. Another explanation is that this large variability is relatively well constrained by the in situ observing system, which will be discussed further later.
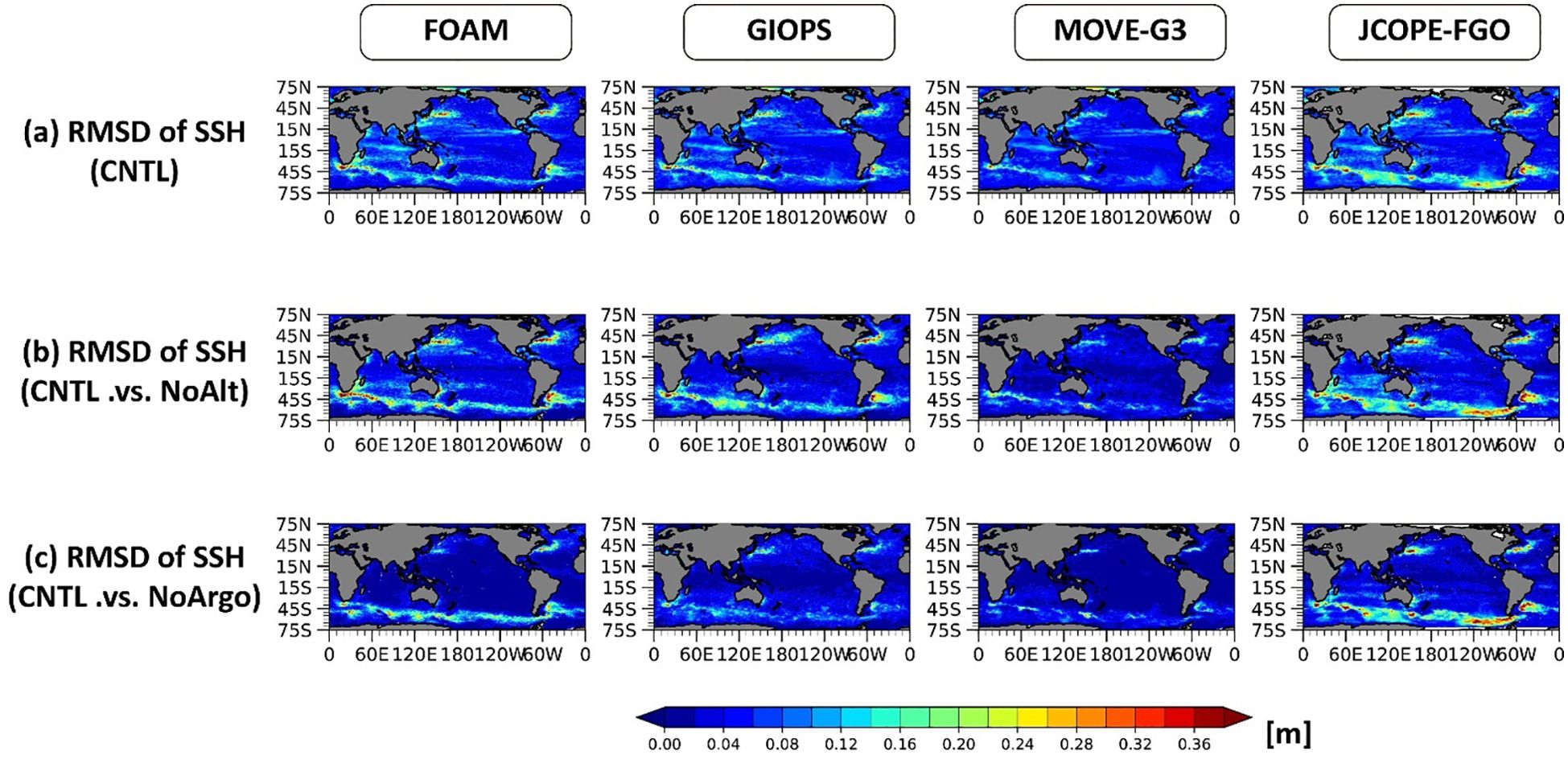
Figure 2. (A) Global maps of RMSD of daily mean SSH from the annual mean for 2020 in CNTL, (B) RMSD in daily mean SSH between NoAlt and CNTL (showing the impact of the altimetry data on the SSH fields), and (C) RMSD in SSH between NoArgo and CNTL (showing the impact of the Argo data on the SSH fields) for FOAM, GIOPS, MOVE-G3, and JCOPE-FGO (from left to right). Units in m. RMSD, root mean square difference; SSH, sea surface height.
Figure 2B also demonstrates the diversity of the altimetry data impact on the SSH field among four systems. In particular, the impact is generally small in MOVE-G3 compared with the impact in the other systems. This may be affected by the fact that, inadvertently, the sea level anomaly (SLA) data of only two satellites are assimilated in the MOVE-G3 OSEs, even though the data from six satellites are available. However, it may rather be due to the low resolution of the system. In MOVE-G3, information on small-scale features is filtered out by the 4DVAR scheme with the low resolution (0.3°–0.5° × 1°) model especially in areas of high-eddy activities due to the inability of the model to fit the information, and analysis increments are reduced consequently. The OSEs for MOVE-G3 will be rerun by assimilating more altimetry data, and the reason will be investigated again.
In contrast, the impact in GIOPS (resolution, 1/4°) is much larger than that in MOVE-G3 and similar, but still generally smaller in amplitude, to that in FOAM (resolution, 1/12°). JCOPE-FGO (1/10°) has a similar distribution of the altimetry data impact to FOAM in the northern hemisphere, but the area with large SSH variability and consequently larger altimetry data impact is more extended around the ACC. It has also a broader area with large altimetry data impact in the South Indian subtropics in comparison with the other systems.
Figure 2C indicates that, in all systems, Argo data assimilation changes the SSH field, particularly in the western boundary current regions and the area around the ACC, even if the SSH field is constrained by the altimetry data. The impact is the smallest in MOVE-G3, as is the impact of the altimetry data. The impact of the Argo data in GIOPS is also significantly smaller compared with the two eddy-resolving systems. Among the two eddy-resolving systems, the impact in JCOPE-FGO is generally larger than that in FOAM. In JCOPE-FGO, Argo data have a large impact as well as the altimetry data around the ACC, while in FOAM, the Argo impact is considerably smaller except in the western boundary current regions and around the ACC.
Although the SSH RMSD between CNTL and NoArgo is generally small in the tropical band between 15S and 15N in all systems, it does not necessarily mean that Argo data have only a small impact in this region. We suggested above that the small SSH RMSD between CNTL and NoAlt in this region (Figure 2B) may be due to the SSH field being sufficiently constrained by TS observations. Similarly, the altimetry data are likely sufficient to constrain the SSH field. In other words, although both the altimetry and Argo data may be effective in constraining the SSH field, the information they contained may be redundant, and complementary effects may barely appear in the tropical region. This possibility will be investigated later when outputs of NoSST and/or Free experiments become available.
We next show the root mean square error (RMSE) of SSH in CNTL evaluated against the Copernicus Marine Environment Monitoring Service (CMEMS) gridded altimetry maps from objective analysis (CMEMS, 2023), and its reduction compared to the RMSE in NoAlt and NoArgo in Figure 3. Here, the mean difference of SSH output from the CMEMS analysis, that is, the bias, is not removed before calculating the RMSE. Altimetry data assimilation generally reduces the RMSE in all four systems, as shown in Figure 3B. Here, it should be noted that a smaller value of the RMSE does not necessarily mean that the OSE run is more accurate, as the objective analysis also has errors, which include horizontal sampling errors and errors in the mean dynamic topography (MDT). However, we refer to the value as the RMSE in order to distinguish the value with the RMSD between different OSEs and because smaller values of the RMSE are generally more likely to be accurate. It is also important to point out that the finer-resolution models will have a much higher value of unconstrained error, whereby the model will resolve variability that the observations cannot constrain (Jacobs et al., 2021).
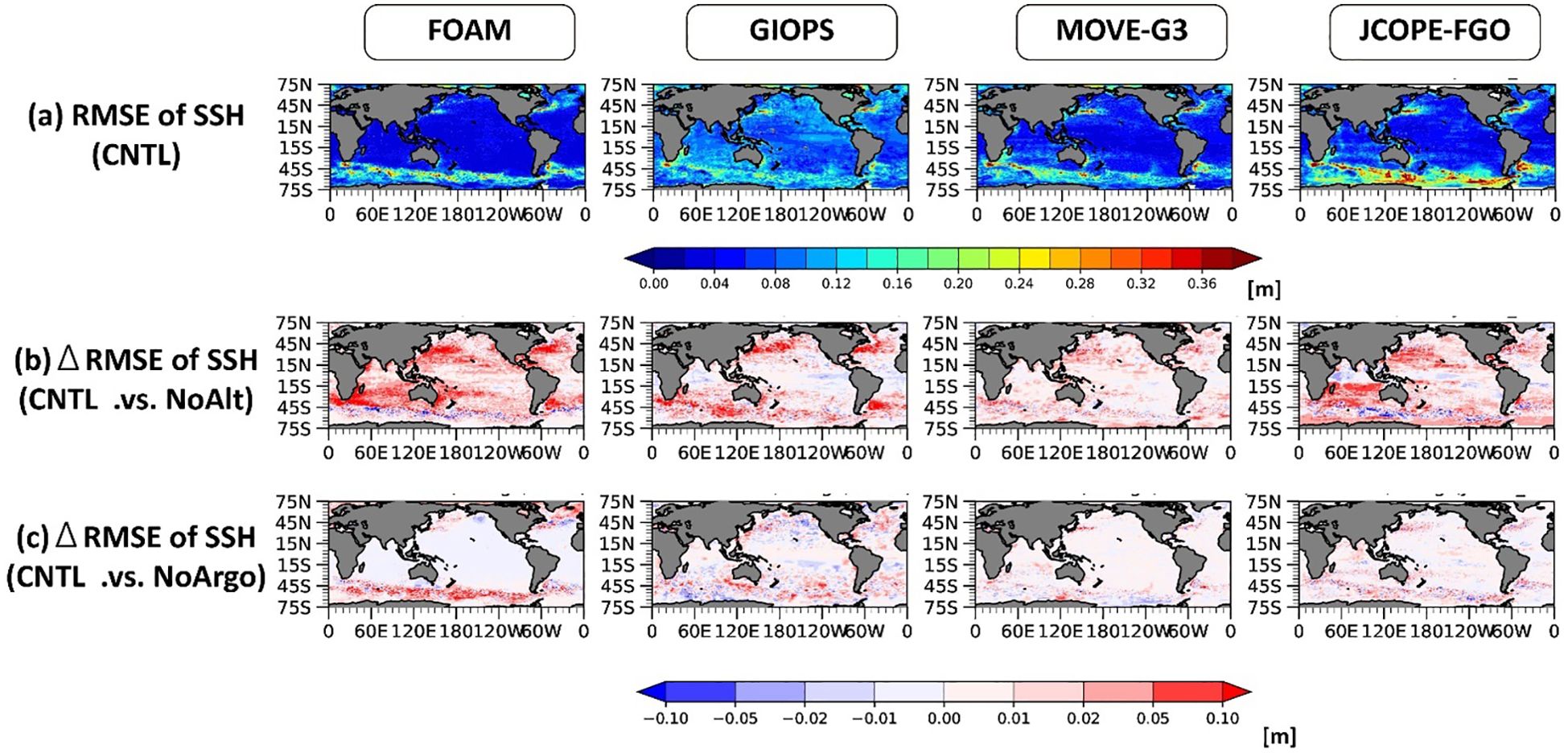
Figure 3. (A) Global maps of SSH RMSE in CNTL, (B) SSH RMSE difference between NoAlt and CNTL, and (C) SSH RMSE difference between NoArgo and CNTL for FOAM, GIOPS, MOVE-G3, and JCOPE-FGO (from left to right). A positive difference (red) means that the RMSE is smaller for CNTL; i.e., the altimeter or Argo data have a positive impact. RMSEs are evaluated against the CMEMS altimetry objective analysis. Units in m. SSH, sea surface height; RMSE, root mean square error.
The reduction of the RMSE by assimilating altimetry data is relatively large in the western boundary current regions and the areas around the ACC, in which the RMSE is relatively large in CNTL (Figure 3A). However, the areas of large reduction appear to be generally wider than the areas where the RMSE is large in CNTL. Particularly for the two eddy-resolving systems (FOAM and JCOPE-FGO), a significant impact can be seen even in the low-latitude regions where the RMSE is relatively small. In these areas, the representativeness error included in the prescribed observation error of the altimetry data is presumably set to a relatively small ratio relative to the background error (BGE), and the analysis increment of SSH effectively cancels the actual difference between the SSH first-guess and the observation in those systems. Eddy-resolving systems tend to set a smaller ratio of representativeness error relative to BGE than lower-resolution systems, given their higher capacity of representing small-scale phenomena, although common statistics are used in the 1/4°- and 1/12°-resolution versions of FOAM. Relatively frequent analysis (daily assimilation cycles) may also be a factor for FOAM. In contrast, in the two lower-resolution systems (GIOPS and MOVE-G3), areas with a large reduction of RMSE by the altimetry data assimilation appear to be closer to the areas where the RMSE in CNTL is relatively large. The representativeness error is likely set relative to the expected error or the extent of the variability in those systems.
It should be also noted there are a few areas with a slight increase of RMSE in the tropical band (15S–15N) in GIOPS, MOVE-G3, and JCOPE-FGO, which suggests difficulty in assimilating the altimetry data in the tropical regions. In the two eddy-resolving systems (FOAM and JCOPE-FGO), the RMSE is increased by the altimetry data assimilation in some places around the southern boundary of the ACC. One possible reason for this is that enhanced activities of the ACC and eddies around it stimulated by assimilating the altimetry data cause larger model errors there. Another reason may be the Dynamic Atmosphere Correction (DAC), which is often applied to altimetry data. In addition to the inverse barometer correction, DAC removes high-frequency barotropic processes associated with wind and pressure forcings from the altimetry observations and has the largest impact at high latitudes. The model SSH does represent some of the high-frequency processes associated with the wind forcing, and this leads to a discrepancy in resolved processes between the model and altimetry SSH and makes altimetry assimilation at high latitudes more difficult (Barbosa Aguiar et al., 2024). Furthermore, negative impacts may also be caused by the discrepancy between MDTs used in ocean prediction systems and the CMEMS analysis. The broader correction of SSH around the ACC by assimilating the altimetry data in JCOPE-FGO (Figure 2B) does not reduce the RMSE in the region effectively, suggesting a problem that can be improved in the future. The reduction of RMSE is generally the smallest in MOVE-G3, which is not surprising considering that the SSH change by the assimilation in MOVE-G3 is the smallest among the four systems.
Figure 3C demonstrates that the SSH RMSE is generally further reduced when the Argo data are assimilated in addition to the altimetry data. As for FOAM, the RMSE is effectively reduced in the western boundary current regions and around the ACC but slightly increased in the other areas. The system strongly constrains the SSH field to the altimetry data, but the difference is increased when the system tries to fit TS fields to the Argo data. As for the other three systems, the RMSE is generally reduced in the global ocean, but the reduction is smaller than that in FOAM. An increase of RMSE is found near the Antarctic coast in GIOPS and MOVE-G3, suggesting the difficulty of assimilation near the sea ice. The RMSE is increased by assimilating the Argo data in the Alaskan Gyre in FOAM and GIOPS. The RMSE reduction by the Argo data assimilation is also the smallest in the MOVE-G3.
The multi-system ensemble spread can be considered as a fair metric of uncertainty that does not depend on any particular system. The global map of time-averaged multi-system ensemble spread of SSH for CNTL, NoAlt, and NoArgo (Figures 4A–C) shows a good correspondence in the spatial patterns with the SSH RMSE in the four systems (Figure 3A). Figure 4D demonstrates that the ensemble spread of SSH is effectively reduced in most areas of the global ocean by assimilating the altimetry data in addition to the Argo data and other regular observation data, which implies a reduction of the SSH uncertainty by the altimetry data assimilation. The reduction is relatively large in the western boundary current regions and around the ACC but less noticeable than the reduction of the SSH RMSEs by the altimetry data assimilation. Areas of the spread increase can be seen in the southern boundary of the ACC, where the negative impact of assimilating the altimetry data on the RMSE is observed in Figure 3B. It should also be noted that assimilating the Argo data in addition to the altimetry data and other regular data reduces the SSH uncertainty in the western boundary regions and around the ACC (Figure 4E). In particular, Argo data decrease spread (red) in the southern portions of the ACC where assimilation of the altimetry data is increasing spread (blue)—this also seems to apply in the Greenland and Barents Seas. This fact suggests that Argo data have information of SSH complementary to the altimetry data and that the information is effectively used in the ocean prediction systems, particularly in regions like the areas near the sea ice, where errors in the assimilation of the altimetry data could be larger. These reductions of the multi-system ensemble spreads can be considered as a fair metric of the impact of assimilating the altimetry and Argo data, which are not affected by systematic errors of any particular system.
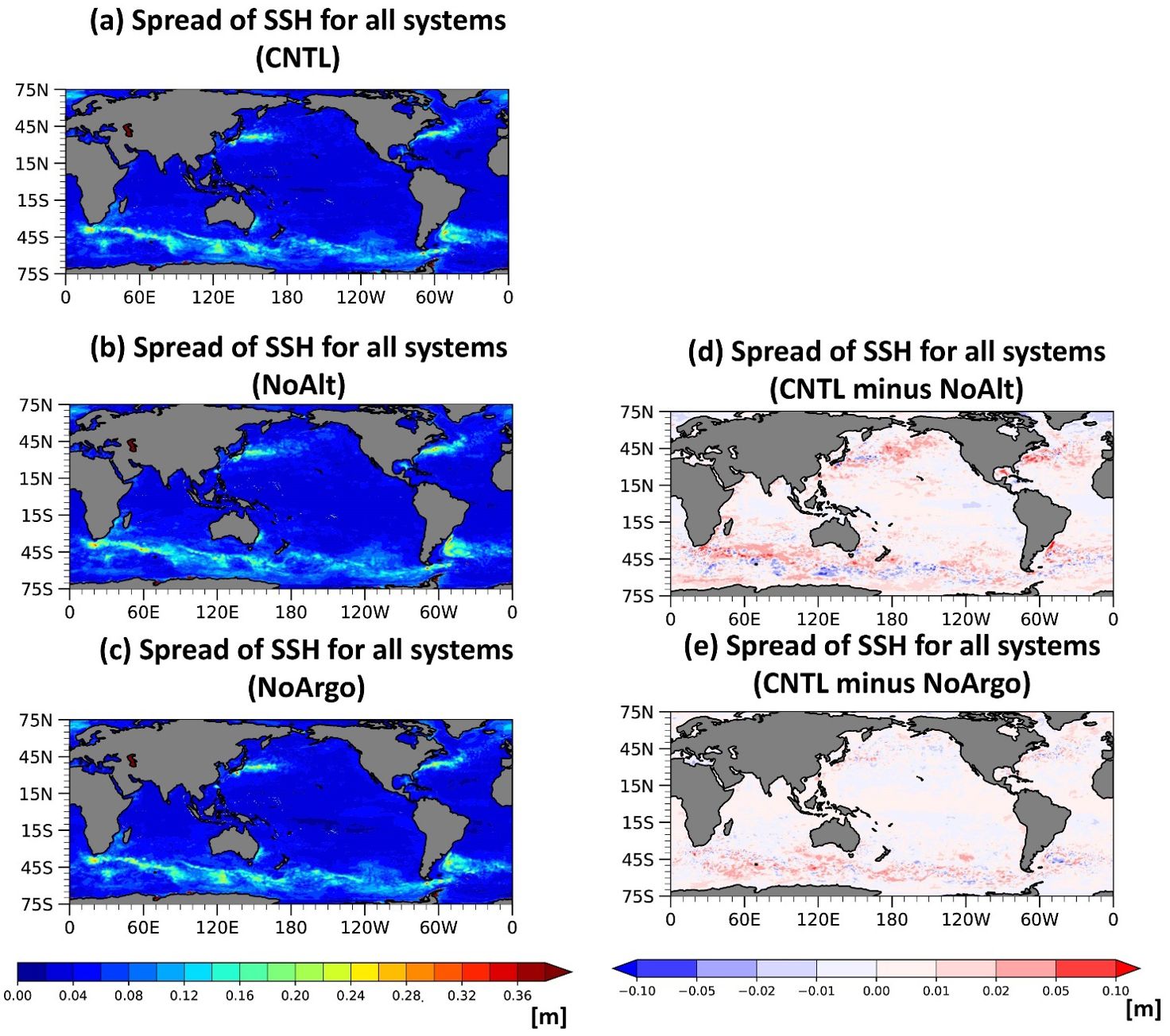
Figure 4. Left panels: global maps of the ensemble spreads of SSH among the four systems for (A) CNTL, (B) NoAlt, and (C) NoArgo, averaged over the whole period of 2020. Right panels: differences of the ensemble spreads (D) between NoAlt and CNTL and (E) between NoArgo and CNTL. A positive difference (red) means that the spread is smaller for CNTL; i.e., the altimeter or Argo data reduce the ensemble spread. Units in m. SSH, sea surface height.
Figure 5 shows the time variation of the SSH multi-system ensemble spread averaged over the global ocean and the tropical Pacific (120E–80W, 20S–20N) for the three data denial settings. As for the global averages (Figure 5A), the multi-system ensemble spread for CNTL is consistently smaller than that for NoAlt, indicating the substantial impact of the altimetry data assimilation. However, the difference tends to narrow toward the end of 2020 as the spread for NoAlt becomes smaller. The relatively large spread for NoAlt in the early part of the year may be related to the shock of halting the altimetry data assimilation at the beginning of the year for JCOPE-FGO and more than 3 months earlier for other systems. In contrast, the ensemble spread for NoArgo is just slightly larger than that for CNTL until the end of May but becomes distinctly larger than that for CNTL and almost comparable to that for NoAlt later. It is even larger than that for NoAlt after November. The Argo data impact on the global SSH field, thus, becomes increasingly evident, which may be due to the slow deterioration of the oceanic density structure without sufficient constraint of temperature and salinity observations in NoArgo OSEs. This possibility will be explored when OSE outputs of more systems become available.
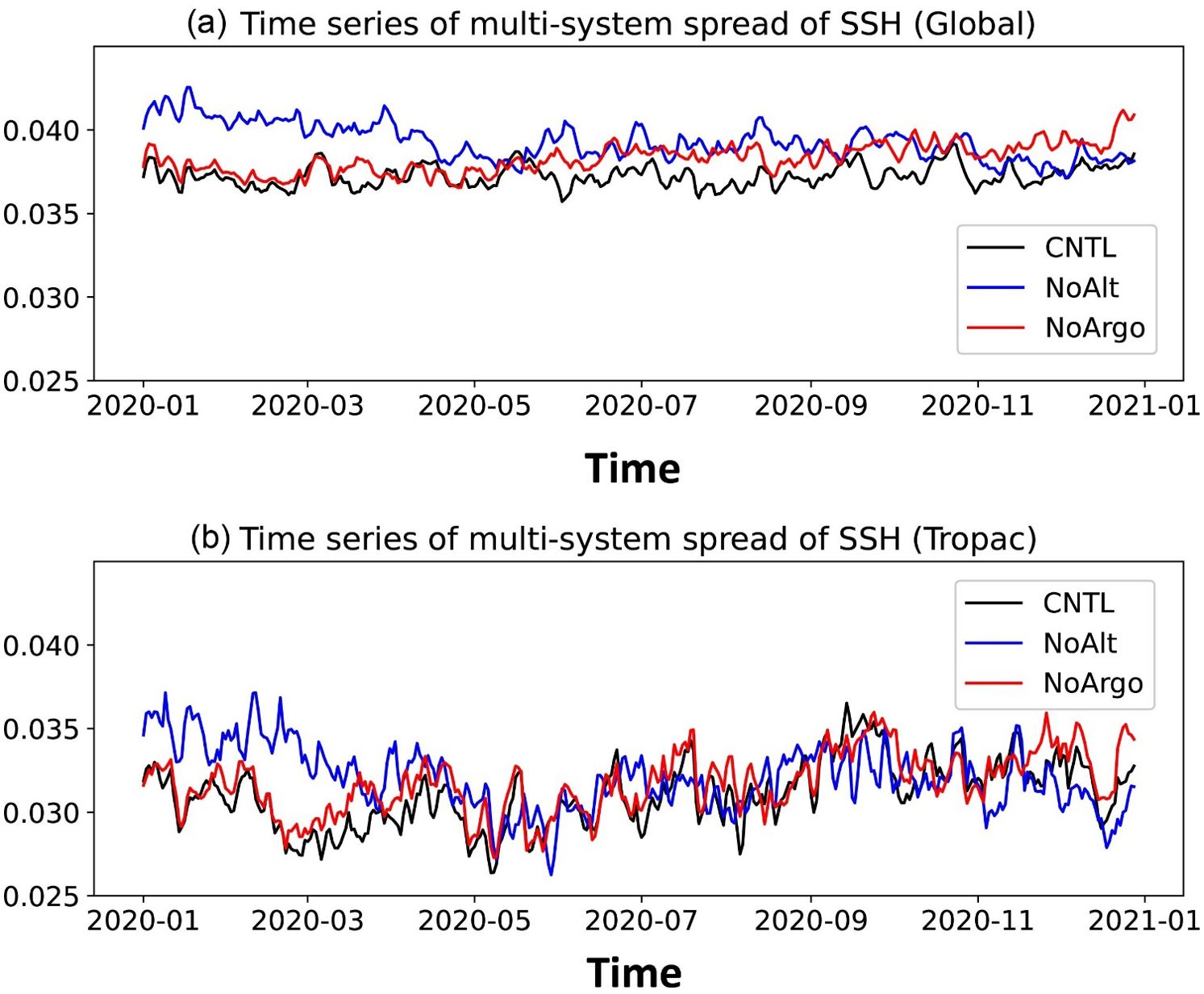
Figure 5. Time series of the ensemble spread of SSH among the four systems averaged over (A) the global ocean and (B) the tropical Pacific (20S–20N, 120E–80W) for CNTL (black), NoAlt (blue), and NoArgo (red). Units in m. SSH, sea surface height.
In the tropical Pacific (Figure 5B), the ensemble spread for NoAlt is distinctively larger than that for CNTL at the start of the analysis period but decreases until May. The spread is then comparable to that for CNTL from June to October and slightly smaller than CNTL in December. The transition in the first 4 months is caused by the experimental setting of the NoAlt run using JCOPE-FGO, which started from the same initial state as CNTL on Jan. 1, 2020. The tropical Pacific SSH fields in the NoAlt run of JCOPE-FGO increasingly deviate from that in CNTL and the CMEMS objective analysis; however, it gets closer to the NoAlt run in the other three systems, which means that those systems have similar SSH errors in the tropical Pacific when the altimetry data are not assimilated. The ensemble spread for NoArgo is generally comparable to that for CNTL but slightly larger than CNTL on average, indicating a weak impact of the Argo data on the SSH field in the tropical Pacific.
3.2 Evaluation using reference Argo data
We also evaluate the impact of the altimetry and Argo data on the global TS fields using RMSEs evaluated against the reference Argo data, which were collected from the snapshot of the Argo Global Data Assembly Center (GDAC) in Mar. 2023. Here, it should be noted again that a smaller value of the RMSE does not necessarily mean that the OSE run is more accurate because of observation errors. Figure 6A shows that FOAM effectively reduces the TS RMSEs at almost all levels by assimilating the Argo data and also by assimilating the altimetry data. It should particularly be noted that the impact of the altimetry data is greater than that of the Argo data between 100- and 700-m depths. This system seems to use the altimetry data efficiently to improve not only SSH but also TS fields.
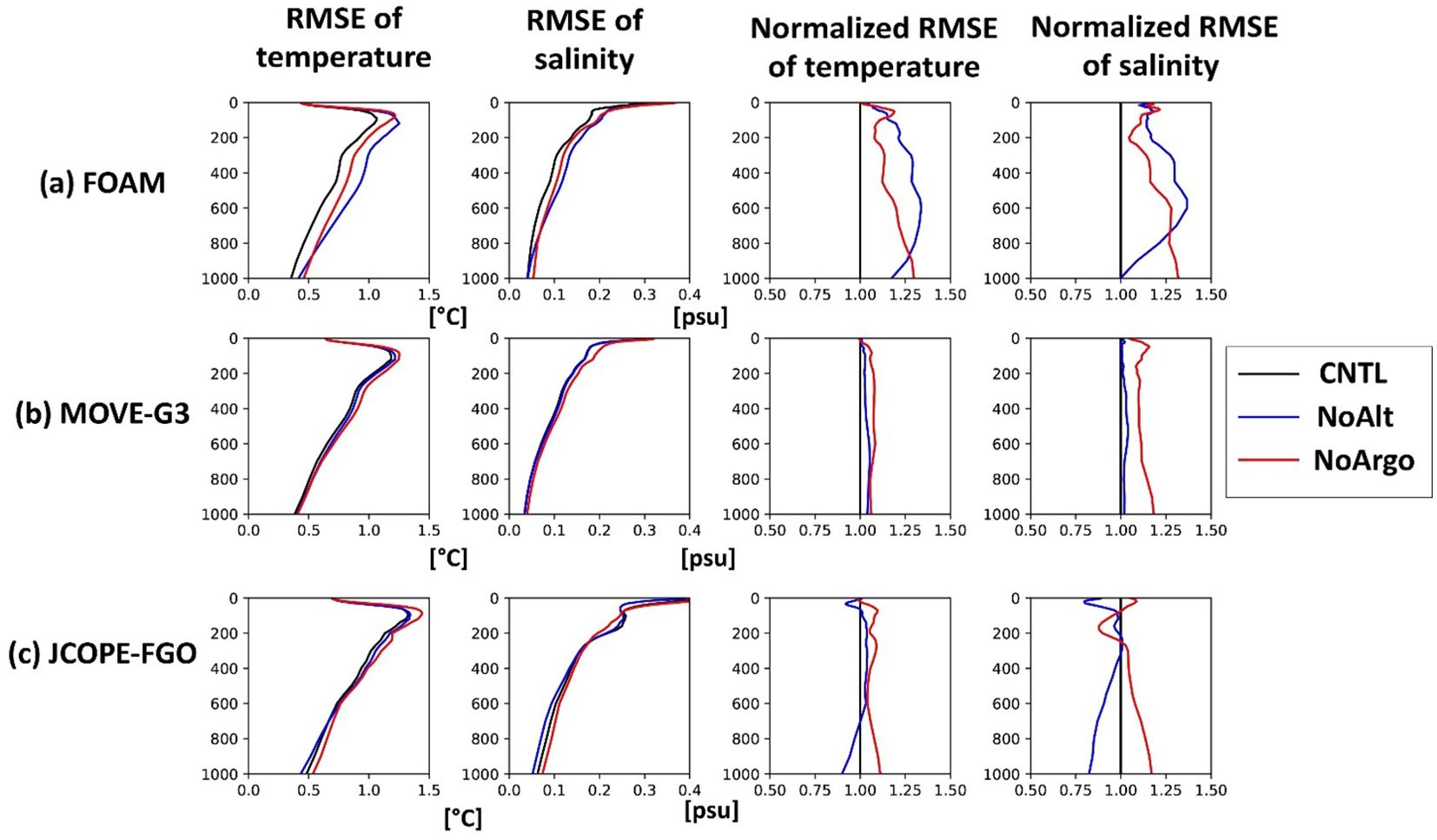
Figure 6. Vertical profiles of global mean RMSEs of temperature (leftmost, units in degree C) and salinity (2nd from left, units in PSU) for CNTL (black), NoAlt (red), and NoArgo (blue), and the global mean RMSEs of temperature (3rd from left) and salinity (rightmost) for NoAlt (red) and NoArgo (blue) normalized by the global mean RMSE for CNTL. Plots are depicted for depths of 0–1,000 m and (A) FOAM, (B) MOVE-G3, and (C) JCOPE-FGO. The RMSEs are evaluated against the independent Argo data, which are not assimilated in all OSEs. Note that only delayed-mode Argo profiles where the maximum depth with valid temperature values exceeds 100 m are used for calculation of RMSEs. RMSEs, root mean square errors; OSEs, observing system experiments. .
Both the Argo and altimetry data also have positive impacts on the global TS fields at all levels for MOVE-G3 (Figure 6B). However, when these impacts are compared to the impacts for FOAM, the Argo data impacts in MOVE-G3 are significantly smaller, and the altimetry data impacts are even smaller. As can be seen in the normalized RMSE plots, the Argo data have a larger impact on salinity than on temperature in MOVE-G3. In contrast, the altimetry data have a larger impact on temperature, while the impact on salinity is considerably small.
The impact of the Argo data on TS for JCOPE-FGO is comparable to that for MOVE-G3 at most levels (Figure 6C). However, the impact on salinity at approximately 200-m depth is negative. As for the altimetry data, the impact is negative for the temperature at near-surface levels, the levels below 800 m, and salinity for all depths. The negative impact may be due to not considering the changes in the global water mass and the global mean steric height when assimilating the altimetry data, or inaccurate error statistics used in the data assimilation scheme, and there is still room for improvement.
In summary, assimilating the Argo data in addition to other regular data generally reduces the TS RMSE. The impact is significantly larger in FOAM than the impact in MOVE-G3 and JCOPE-FGO. The Argo data have a larger impact on salinity than on temperature in MOVE-G3. The extent of the altimetry data impact on TS depends on the system. The impact is comparable to the Argo data on TS in FOAM, but in MOVE-G, the impact is significantly smaller than that in FOAM. The altimetry data do not effectively reduce TS RMSEs in JCOPE-FGO.
4 Summary and future perspectives of SynObs
The UN Ocean Decade Project SynObs has been promoting various activities associated with the evaluation of the ocean observation network since its launch in 2022. In particular, SynObs has started the internationally coordinated multi-system OSEs/OSSEs, or the flagship OSEs/OSSEs, to make a fair evaluation of the ocean observing network, which does not depend on any particular ocean prediction system, and provide a scientifically justified rationale for its maintenance and evolution. The flagship OSEs/OSSEs consists of three components: the OP OSEs for high-resolution ocean predictions, the S2S OSEs for long lead-time coupled ocean–atmosphere predictions, and the OP OSSEs for evaluating new and future observing systems.
We have begun to collect the outputs of the OP OSEs, and, as shown in the previous section, early analysis of those outputs indicates that there are observation impacts consistent across the four ocean prediction systems. For example, the impacts of the altimetry data on the assimilated SSH field are generally large in the western boundary current regions and around the ACC where SSH has large variability but are small in the tropical regions despite the relatively large SSH variability there. We also found characteristics of observation impacts that are specific to some ocean prediction systems. Our analysis indicates the possibility that there are some characteristic differences in the observation impacts between low-resolution and eddy-resolving ocean prediction systems. In addition, the OSEs have shown synergies between the observing systems, with profile observations improving fits to SSH observations in regions where the altimeter observations alone cannot improve the simulation. Similarly, altimeter observations universally demonstrate improvements in fits to non-assimilated Argo profiles, to the same extent the remaining assimilated Argo profiles do. These results generally demonstrate the importance of satellite altimetry data and the global Argo array and provide scientific support for maintaining and further developing these observation systems.
However, the results here are just an illustration of the potential of the SynObs project. Since outputs of the OP OSEs for only four ocean prediction systems are currently available, it is not sufficient to conclude what the observation impacts are consistent across most ocean prediction systems and what are impacts specific to particular prediction systems. It is also difficult to analyze what characteristics cause observation impacts specific to some prediction systems, for example, how observation impacts are affected by the resolution of the prediction system. In addition, it should be noted that we have analyzed only outputs of data assimilation runs so far, and the observation impacts on forecast runs have not been assessed yet. The impacts depend on the limits of the model’s forecast ability and are likely to be reduced compared to the impacts on data assimilation runs because the forecast errors deteriorate information from observations.
Currently, some other ocean prediction centers will conduct or are conducting the analysis runs for the OP OSEs and plan to provide outputs. Some of the submitted OSE outputs are planned to be replaced by a new version that fixes inadvertent defects. We will conduct further investigation on the consistency of observation impacts, the characteristics of observation impacts specific to some ocean prediction systems, and their contributing factors using the original and newly submitted OSE outputs in the near future. In addition, forecast runs from the analysis fields of the assimilation runs for each OSE are planned to be performed, and observation impacts on the forecast accuracy will be also investigated.
Meanwhile, some ocean/climate prediction centers are currently running ocean reanalysis runs for S2S OSEs and plan to perform the coupled atmosphere–ocean model forecasts using those reanalysis fields as oceanic initial conditions. The results of these coordinated OSEs are expected to provide a clear indication of ocean observation impacts on the atmospheric fields in the coupled forecasts, which have only been identified for a few forecasting systems following different experimental protocols (Balan-Sarojini et al., 2024; Balmaseda et al., 2024). We are currently preparing virtual ocean observations for OP OSSEs and discussing the setting of the OSSE runs. The evaluation of the OSSE integrations will target the suitability of the new TPOS design and demonstrate the effectiveness of SWOT SSH data and some other new ocean observations. All outputs of the flagship OSEs/OSSEs will be compiled as a database and shared with several expert groups in order to carry out SynObs multi-system evaluation according to the area of expertise: specific observing systems, target regions, phenomena, applications, and so forth. Finally, the database will eventually be made available to the public. We expect that sharing the flagship OSE/OSSE outputs with various expert groups will boost the impact of SynObs by providing a broader and more rounded evaluation of the observing system evaluation and design, enhancing the communication between the producers and users of data, and increasing the understanding and awareness of the impact of the ocean observations on predictions of the ocean and atmosphere at a range of time scales.
SynObs will promote the sharing of the achievements of research activities and community efforts on the ocean observing system design and evaluation, including the results of the flagship OSEs/OSSEs, with the broader oceanic and earth system research communities through academic papers, scientific meetings, web pages, etc. SynObs also aims to provide significant inputs to the reports on the ocean observation impacts and the design of the ocean observing network made by international organizations, such as the WMO and Global Ocean Observing System (GOOS), and internationally coordinated projects, such as the UN Ocean Decade Programme “Ocean Observing Co-Design” (https://goosocean.org/what-we-do/goos-at-the-heart-of-the-ocean-decade/ocean-observing-co-design), and to contribute to the international designing of the ocean observing network from the perspectives of ocean and coupled predictions. We believe that these SynObs activities will enhance the communications between ocean observing and ocean and coupled prediction communities and lead to a transformative change in the ocean observing network that will generate substantial societal benefits.
Data availability statement
The datasets presented in this study can be found in online repositories. The names of the repository/repositories and accession number(s) can be found below: https://www.jamstec.go.jp/jcope/data/synobs_frontiers/OP-AN/.
Author contributions
YF: Conceptualization, Data curation, Formal analysis, Funding acquisition, Methodology, Project administration, Validation, Writing – original draft, Writing – review & editing. ER: Conceptualization, Funding acquisition, Methodology, Project administration, Writing – review & editing. MB: Conceptualization, Funding acquisition, Methodology, Project administration, Writing – review & editing. SK: Conceptualization, Data curation, Formal analysis, Funding acquisition, Methodology, Resources, Visualization, Writing – review & editing. JW: Funding acquisition, Resources, Validation, Writing – review & editing. KP: Funding acquisition, Resources, Validation, Writing – review & editing. GS: Funding acquisition, Resources, Writing – review & editing. II: Data curation, Resources, Validation, Writing – review & editing. KC: Resources, Writing – review & editing.
Funding
The author(s) declare financial support was received for the research, authorship, and/or publication of this article. For this work, YF was supported by the Grant-in-Aid for Transformative Research Areas under grant No. JP24H02226 from the Ministry of Education, Culture, Sports, Science and Technology, Japan, and ROIS-DS-JOINT (027RP2024). SK was supported by JSPS KAKENHI JP21K13997, 24H02221, and 23K2593. The publication fee is paid by JMA/MRI.
Acknowledgments
We thank all who participated in the SynObs meetings and contributed to the discussion for determining the configuration of the flagship OSEs/OSSEs. We thank Li Ren at NASA/GMAO for providing Figure 1. We also thank the reviewers for their fruitful comments.
Conflict of interest
The authors declare that the research was conducted in the absence of any commercial or financial relationships that could be construed as a potential conflict of interest.
Publisher’s note
All claims expressed in this article are solely those of the authors and do not necessarily represent those of their affiliated organizations, or those of the publisher, the editors and the reviewers. Any product that may be evaluated in this article, or claim that may be made by its manufacturer, is not guaranteed or endorsed by the publisher.
Supplementary material
The Supplementary Material for this article can be found online at: https://www.frontiersin.org/articles/10.3389/fmars.2024.1476131/full#supplementary-material
References
Balan-Sarojini B., Balmaseda B. M., Vitart F., Roberts C., Zuo H., Tietsche S., et al. (2024). Impact of ocean in-situ observations in subseasonal forecasts. Front. Mar. Sci. 11. doi: 10.3389/fmars.2024.1396491
Balmaseda M. A., Anderson D., Vidard A. (2009). Impact of Argo on analyses of the global ocean. Geophys. Res. Lett. 34, L16605. doi: 10.1029/2007GL030452
Balmaseda M. A., Balan-Sarojini B., Mayer M., Tietsche S., Zuo H., Vitart F., et al. (2024). Impact of ocean in-situ observations on the ECMWF seasonal forecasting system. Frot. Mar. Sci. 11. doi: 10.3389/fmars.2024.1456013
Barbosa Aguiar A., Bell M. J., Blockley E., Calvert D., Crocker R., Inverarity G., et al. (2024). The Met Office Forecast Ocean Assimilation Model (FOAM) using a 1/12 degree grid for global forecasts. Q.J.R. Meteorol. Soc. 150, 3827–3852. doi: 10.1002/qj.4798
Bonaduce A., Benkiran M., Rémy E., Le Traon P. Y., Garric G. (2018). Contribution of future wide swath altimetry missions to ocean analysis and forecasting. Ocean Sci. 14, 1405–1421. doi: 10.5194/os-14-1405-2018
Boyer T. P., Antonov J. I., Baranova O. K., Coleman C., García H. E., Grodsky A., et al. (2016). NCEI Standard Product: World Ocean Database (WOD) (NOAA National Centers for Environmental Information). Available online at: https://www.ncei.noaa.gov/archive/accession/NCEI-WOD (Accessed 22-Nov-2023).
Carneiro D. M., Waters J., Lea D., Martin M., While J., Weaver A., et al. (2024). Updates to the global FOAM system including model and data assimilation changes Vol. 2024 (Exeter, UK: Met Office technical report, 659, Met Office). Available at: https://digital.nmla.metoffice.gov.uk/IO_cb2028c5-0dd9-46d3-9910-bdd125f7f3ad/.
CMEMS (2023). Global Ocean Gridded L 4 Sea Surface Heights And Derived Variables Reprocessed 1993 Ongoing (Copernicus Marine Environment Monitoring Service (CMEMS) (Accessed 16-Jul-2024).
Davidson F., Alvera-Azcárate A., Barth A., Brassington G. B., Chassignet E. P., Clementi E., et al. (2019). Synergies in operational oceanography: the intrinsic need for sustained ocean observations. Front. Mar. Sci. 6. doi: 10.3389/fmars.2019.00450
Fujii Y., Cummings J., Xue Y., Schiller A., Lee T., Balmaseda M. A., et al. (2015b). Evaluation of the tropical pacific observing system from the ocean data assimilation perspective. Q. J. R. Meteorol. Soc. 141, 2481–2496. doi: 10.1002/qj.2579
Fujii Y., Ogawa K., Brassington G. B., Ando K., Yasuda T., Kuragano T. (2015a). Evaluating the impacts of the tropical Pacific observing system on the ocean analysis fields in the global ocean data assimilation system for operational seasonal forecasts in JMA. J. Oper. Oceanogr 8, 25–39. doi: 10.1080/1755876X.2015.1014640
Fujii Y., Rémy E., Zuo H., Oke P., Halliwell G., Gasparin F., et al. (2019). Observing system evaluation based on ocean data assimilation and prediction systems: on-going challenges and a future vision for designing and supporting ocean observational networks. Front. Mar. Sci. 6. doi: 10.3389/fmars.2019.00417
Fujii Y., Yoshida T., Sugimoto H., Ishikawa I., Urakawa S. (2023). Evaluation of a global ocean reanalysis generated by a global ocean data assimilation system based on a four-dimensional variational (4DVAR) method. Front. Clim 4. doi: 10.3389/fclim.2022.1019673
Gasparin F., Guinehut S., Mao C., Mirouse I., Rémy E., King R., et al. (2019). Requirements for an integrated in situ atlantic ocean observing system from coordinated observing system simulation experiments. Front. Mar. Sci. 6. doi: 10.3389/fmars.2019.00083
Gasparin F., Hamon M., Rémy E., Le Traon P. Y. (2020). How deep Argo will improve the deep ocean in an ocean reanalysis. J. Clim. 33 (1), 77–94.
Gasparin F., Lellouche J. M., Cravatte S. E., Ruggiero G., Rohith B., Le Traon P. Y, et al. (2023). On the control of spatial and temporal oceanic scales by existing and future observing systems: An observing system simulation experiment approach. Front. Marine Sci. 10, 1021650.
Halliwell G. R., Mehari M. F., Le Henaff M., Kourafalou V. H., Androulidakis I. S., Kang H. S., et al. (2017). North Atlantic Ocean OSSE system: Evaluation of operational ocean observing system components and supplemental seasonal observations for potentially improving tropical cyclone prediction in coupled systems. J. Oper. Oceanogr 10, 154–175. doi: 10.1080/1755876X.2017.1322770
Jacobs G., D’Addezio J. M., Ngodock H., Souopgui I. (2021). Observation and model resolution implications to ocean prediction. Ocean Model. 159, 101760. doi: 10.1016/j.ocemod.2021.101760
Kessler W., Cravatte S. (2021). Final Report TPOS 2020. GOOS-268. Available online at: https://tropicalpacific.org/tpos2020-project-archive/reports/ (Accessed 03-Jul-2024).
Kessler W. S., Wijffels S. E., Cravatte S., Smith N. (2019). Second Report of TPOS 2020. GOOS-234. Available online at: https://tropicalpacific.org/tpos2020-project-archive/reports/ (Accessed 03-Jul-2024).
Kido S., Nonaka M., Miyazawa Y. (2022). JCOPE-FGO: An eddy-resolving quasi-global ocean reanalysis product. Ocean Dyn. 72 (8), 599–619. doi: 10.1007/s10236-022-01521-z
Kido S., Nonaka M., Miyazawa Y. (2023). Skillful multiyear prediction of the Kuroshio and Gulf Stream jets and eddy activity. Geophy. Res. Lett. 50, e2023GL103705. doi: 10.1029/2023GL103705
Lea D. J., Martin M. J., Oke P. R. (2014). Demonstrating the complementarity of observations in an operational ocean forecasting system. Q.J.R. Meteorol. Soc. 140, 2037–2049. doi: 10.1002/qj.2281
Locarnini R. A., Mishonov A. V., Antonov J. I., Boyer T. P., García H. E., Baranova O. K., et al. (2013). World Ocean Atlas 2013, Volume 1: Temperature, Vol. 73. Eds. Levitus S., Mishonov A. Technical NOAA Atlas NESDIS 73, National Oceanographic Data Center, Silver Spring, MD, 40pp.
Menemenlis D. (2023). GEOS/ECCO coupled nature run data portal. Available online at: https://data.nas.nasa.gov/geosecco (Accessed 05-Jul-2024).
Oke P. R., Schiller A. (2007). Impact of Argo, SST, and altimeter data on an eddy-resolving ocean reanalysis. Geophys. Res. Lett. 34, L19601pp. doi: 10.1029/2007GL031549
Smith G. C., Liu Y., Lemieux J.-F., Chikhar K., Colan D. S., Gauthier A.-A., et al. (2024). Global ice ocean prediction system (GIOPS): update from version 3.4.1 to version 3.5.0. Meteorological Service of Canada, Environment and Climate Change Canada, Environment Canada, Centre Météorologique Canadien, division du développement. Tech. Rep. Available at: https://collaboration.cmc.ec.gc.ca/cmc/cmoi/product_guide/docs/tech_notes/technote_giops-350_e.pdf.
Smith G. C., Roy F., Reszka M., Surcel Colan D., He Z., Deacu D., et al. (2016). Sea ice forecast verification in the Canadian Global Ice Ocean Prediction System. Q. J. R. Meteorol. Soc 142, 659–671. doi: 10.1002/qj.2555
Subramanian A. C., Balmaseda M. A., Centurioni L., Chattopadhyay R., Cornuelle B. D., DeMott C., et al. (2019). Ocean observations to improve our understanding, modeling, and forecasting of subseasonal-to-seasonal variability. Front. Mar. Sci. 6. doi: 10.3389/fmars.2019.00427
Turpin V., Rémy E., Le Traon P. Y. (2016). How essential are Argo observations to constrain a global ocean data assimilation system. Ocean Sci. 12, 257–274. doi: 10.5194/os-12-257-2016
UNESCO-IOC (2021). The United Nations Decade of Ocean Science for Sustainable Development, (2021–2030) Implementation Plan. Ocean Decade series, 20, Paris, France: UNESCO Digital Library, 54pp. Available at: https://unesdoc.unesco.org/ark:/48223/pf0000377082.
Vidard A., Anderson D. L. T., Balmaseda M. (2007). Impact of ocean observation systems on ocean analysis and seasonal forecasts. Mon. Wea. Rev. 135, 409–429. doi: 10.1175/MWR3310.1
Waters J., Martin M. J., Mirouze I., Rémy E., King R. R., Gautier L., et al. (2024). The impact of simulated Total Surface Current Velocity observations on operational ocean forecasting and requirements for future satellite missions. Front. Mar. Sci. 11. doi: 10.3389/fmars.2024.1408495
Xue Y., Wen C., Yang X., Behringer D., Kumar A., Vecchi G., et al. (2017). Evaluation of tropical Pacific observing system using NCEP and GFDL ocean data assimilation systems. Clim. Dyn 49, 843–868. doi: 10.1007/s00382-015-2743-6
Keywords: ocean prediction, OSE, ocean observing network, Argo, satellite altimetry, data assimilation, UN Ocean Decade
Citation: Fujii Y, Remy E, Balmaseda MA, Kido S, Waters J, Peterson KA, Smith GC, Ishikawa I and Chikhar K (2024) The international multi-system OSEs/OSSEs by the UN Ocean Decade Project SynObs and its early results. Front. Mar. Sci. 11:1476131. doi: 10.3389/fmars.2024.1476131
Received: 05 August 2024; Accepted: 30 September 2024;
Published: 30 October 2024.
Edited by:
John Roland Moisan, National Aeronautics and Space Administration (NASA), United StatesReviewed by:
Han Zhang, Ministry of Natural Resources, ChinaAndrea Storto, National Research Council (CNR), Italy
Copyright © 2024 Fujii, Remy, Balmaseda, Kido, Waters, Peterson, Smith, Ishikawa and Chikhar. This is an open-access article distributed under the terms of the Creative Commons Attribution License (CC BY). The use, distribution or reproduction in other forums is permitted, provided the original author(s) and the copyright owner(s) are credited and that the original publication in this journal is cited, in accordance with accepted academic practice. No use, distribution or reproduction is permitted which does not comply with these terms.
*Correspondence: Yosuke Fujii, eWZ1amlpQG1yaS1qbWEuZ28uanA=