- 1Department of Fisheries, Ocean University of China, Qingdao, Shandong, China
- 2First Institute of Oceanography, Ministry of Natural Resources, Qingdao, Shandong, China
The Northwest Pacific Ocean is the most productive fishing ground in the Pacific Ocean, with a continuous rise in water temperature since 1990. We developed stacked species distribution models (SSDMs) to estimate the impacts of climate change on the distribution dynamics of economically significant species under three climate change scenarios for the periods 2040-2060 and 2080-2100. Overall, water temperature is the most important factor in shaping the distribution patterns of species, followed by water depth. The predictive results indicate that all the species show a northward migration in the future, and the migration distance varies greatly among species. Most pelagic species will expand their habitats under climate change, implying their stronger adaptability than benthic species. Tropical fishes are more adaptable to climate change than species in other climate zones. Though limitations existed, our study provided baseline information for designing a climate-adaptive, dynamic fishery management strategy for maintaining sustainable fisheries.
Introduction
According to the findings from the Sixth Assessment Report by the Intergovernmental Panel on Climate Change (IPCC), our planet is experiencing rapid global warming. The oceans, serving as vital carbon reservoirs within the global ecosystem, have absorbed a substantial quantity of heat from the climate system (Heinze, 2014). This oceanic warming is happening globally and is expected to continue throughout the 21st century. The average global sea surface temperature has risen by about 0.88°C from 2011 to 2020, compared to the baseline period of 1850-1900 (IPCC, 2021). Significantly, the thermal content in the Northwest Pacific (between 30°N and 62°N) has sharply increased since 1990, reaching unprecedented levels in 2021 (Cheng et al., 2022). Climate change is driving changes in ocean conditions, such as warming, acidification, deoxygenation, and declining primary productivity, which are expected to significantly alter species habitats and spawning areas (Hall and Lewandowska, 2022). These physical and biogeochemical shifts are also anticipated to transform the structure of biological communities (Doney et al., 2012), with particularly pronounced effects on the distribution of marine species.
The alterations in the structure and functionality of marine ecosystems induced by climate change may diminish the productivity of fisheries (Zeng et al., 2019; Do et al., 2022). It has been projected that ocean warming between 2041 and 2060 in global Exclusive Economic Zones (EEZs), under the high emissions scenario of RCP 8.5, could cause fish catch potential to decrease by 11% per decade, accompanied by an average biomass reduction of 3.6% relative to 1986-2005 (Cheung et al., 2021). Furthermore, the impact of climate change on marine life is expected to alter the composition of catch, simultaneously increasing bycatch rates and affecting the survival of bycatch species (Sabal et al., 2023). The Northwest Pacific Ocean is the most productive fishing region in the Pacific Ocean, with the highest catch proportion in Fishing Area 61, accounting for 41% of the total Northwest Pacific fishing yield (FAO, 2022). Climate change will undoubtedly alter the distribution of economically significant species within these fishing areas (Bell et al., 2021; Liu et al., 2023), thereby bringing a series of ecological and economic effects on the local communities. For instance, alterations in species distribution might change the harvested species and fishing operation of local fishermen (Cavalli et al., 2008). Such shifts in the economic value of captured species—specifically, a reduction in high-value species and an increment in low-value catches—could significantly impact fishermen’s earnings and pose challenges for the ongoing management strategy of fishery resources (Weatherdon et al., 2016; Spijkers and Boonstra, 2017; Andersen et al., 2024).
The imperative to comprehend shifts in the distribution of marine life amidst climate change is underscored by their critical ecological roles and their values as significant socio-economic and food resources (Smale et al., 2019). Species Distribution Models (SDMs) can effectively predict the spatio-temporal dynamics of species occurrence, so as to produce distribution maps of economically significant species, which are the essential data for designing climate-adaptive fishery management strategies. Currently, SDMs are the most commonly used tools for predicting distribution patterns and diversity on a large geographic range by integrating environmental predictors with species occurrence data (Guisan and Thuiller, 2005; Guisan et al., 2017; Phillips et al., 2017). The traditional SDMs were usually used to forecast the geographic distribution of individual species. However, probabilistic stacking (pS-SDM) or binary prediction overlay (bS-SDM) techniques can be used to create local species assemblages (Calabrese et al., 2014; D’Amen et al., 2015), which facilitate the identification of biodiversity conservation hotspots (Hu et al., 2022). While these predictions are not always precise, they provide a practical approach that complements traditional species surveys in assessing dynamic networks of species richness, offering crucial data for conservation management strategies (Ferrier and Guisan, 2006; Dubuis et al., 2011; Calabrese et al., 2014).
Conducting more comprehensive and systematic comparisons using SDMs for economically important species with diverse ecological preferences is crucial for advancing international fisheries cooperation and enhancing resource management strategies (Liu et al., 2018; Li et al., 2022; Zhu et al., 2024). This research employs the Stacked Species Distribution Models (SSDMs), integrating species occurrence data and extensive environmental variables, to investigate the distribution dynamics of economically significant species with diverse ecological preferences in the Northwest Pacific Ocean under three climate change scenarios. The objectives of this research are to: (1) investigate the current distribution patterns of these species under current climatic conditions; (2) identify the principal environmental factors that shape the distribution patterns of these species; (3) forecast the distribution of each species and its shift under three climate change scenarios; (4) compare the distributional changes of species with different ecological preferences in response to climate change. Our study can provide fundamental data for designing a climate-adaptive, dynamic fishery management strategy in favor of maintaining the sustainability of these ecologically significant species in the Northwest Pacific Ocean.
Materials and methods
Study area and species occurrence data
Our research focuses on the Food and Agriculture Organization (FAO, https://www.fao.org) Fishing Area 61 within the Northwest Pacific Ocean, spanning from 20°N to 65°N and from 110°E to 175°E. We selected nine highly productive representative species from this region based on FAO publications—Fishery and Aquaculture Statistics (FAO, 2021). Their ecological habitats were determined using information from FishBase (https://www.fishbase.org) and other relevant published sources (Liu and Ning, 2011). These species were distributed in different climatic zones: tropical, subtropical, temperate, and boreal. Furthermore, based on their living depths, they were categorized into pelagic and benthic species. Occurrence data for these species were collected from published literature and two online databases: the Global Biodiversity Information Facility (GBIF, https://www.gbif.org) and the Ocean Biodiversity Information System (OBIS, https://obis.org). To minimize sample bias, we retained one occurrence record per 5.5×5.5 km grid cell (Me et al., 2015). A total of 1552 occurrence records for the nine species were compiled for the modeling process. The ecological characteristics and the number of available occurrence points for each species are detailed in Table 1. The geographic scope of the study area, along with the distribution points of these species, is illustrated in Figure 1.
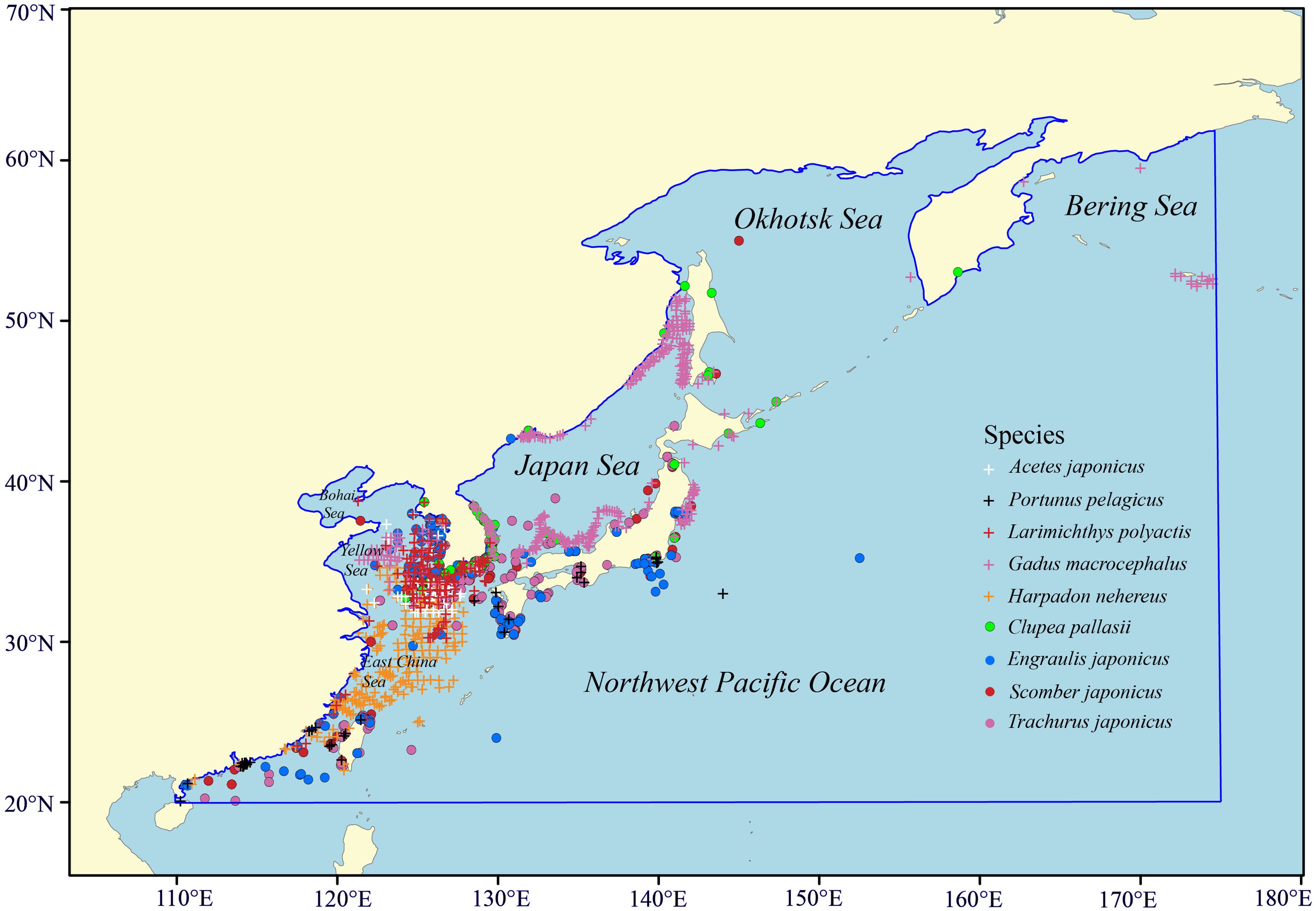
Figure 1. The distribution of available occurrence points for each species. Blue line indicates the boundary of study area.
Predictive variables
Considering the distribution environments of these species (Table 1) and data availability, we selected ten and twelve predictor variables related to marine surface and benthic environments for pelagic and benthic species, respectively (Table 2). We obtained the environmental data from the online database of Bio-ORACLE v3.0 (https://www.bio-oracle.org). All environmental variables have a spatial resolution of 0.05° (approximately 5.5 × 5.5 km at the equator). These variables were then clipped to the study area using the “crop” function in the R package “raster” for subsequent analyses. Research indicated that ocean temperature, dissolved oxygen concentration, pH, and primary productivity are fundamental controlling factors in marine ecosystems (Fischlin et al., 2007). Sea water velocity, depth, salinity, and slope are commonly utilized as predictor variables in marine biogeography studies (Bradie and Leung, 2017; Assis et al., 2018; Melo-Merino et al., 2020). The mixed layer depth is vital in regulating the exchange of heat and carbon between the atmosphere and the ocean. It supports the majority of primary production within the marine ecosystem, thereby playing a crucial role in sustaining marine life (Sallée et al., 2021). Hence, the mixed layer depth was selected as a predictor variable for pelagic marine species. For benthic species with habitats intrinsically linked to seabed substrates, variables including the topographic position index, terrain ruggedness index, and topographic aspect are deemed significant for their habitats (Pittman and Brown, 2011). To avoid multicollinearity among environmental factors, the two variables with a Pearson correlation coefficient greater than |0.7| are collinear and one of them was removed (Supplementary Figure 1; Dormann et al., 2013; Schickele et al., 2020; Sun et al., 2022). Additionally, we analyzed the collinearity of predictor variables using the Variance Inflation Factor (VIF). The VIF values of the retained variables were all below 3 (Supplementary Tables 1, 2), indicating the low collinearity among them (Kim, 2019; Sioni et al., 2019; Panzeri et al., 2024). For pelagic species, the dynamic variables of mean ocean temperature (Temp), mean mixed layer depth (Mlay), mean pH (pH), mean salinity (Salt), mean sea water velocity (Cv), mean primary productivity (Phy) in the surface, and the static topographic factors of mean bathymetry (Depth) and topographic slope (Slope) were retained for modeling (Supplementary Figure 1). For benthic species, the dynamic variables of mean ocean temperature (Temp), mean sea water velocity (Cv), mean dissolved oxygen concentration (Do), mean primary productivity (Phy) in the bottom, and the static topographic factors of mean bathymetry (Depth), topographic slope (Slope), topographic position index (Position), and geomorphological features (Aspect) were retained for modeling. To match the temporal scale of the available occurrence data, the environmental data representing current climatic conditions in this study are based on the average environmental conditions of the years from 2000 to 2020.
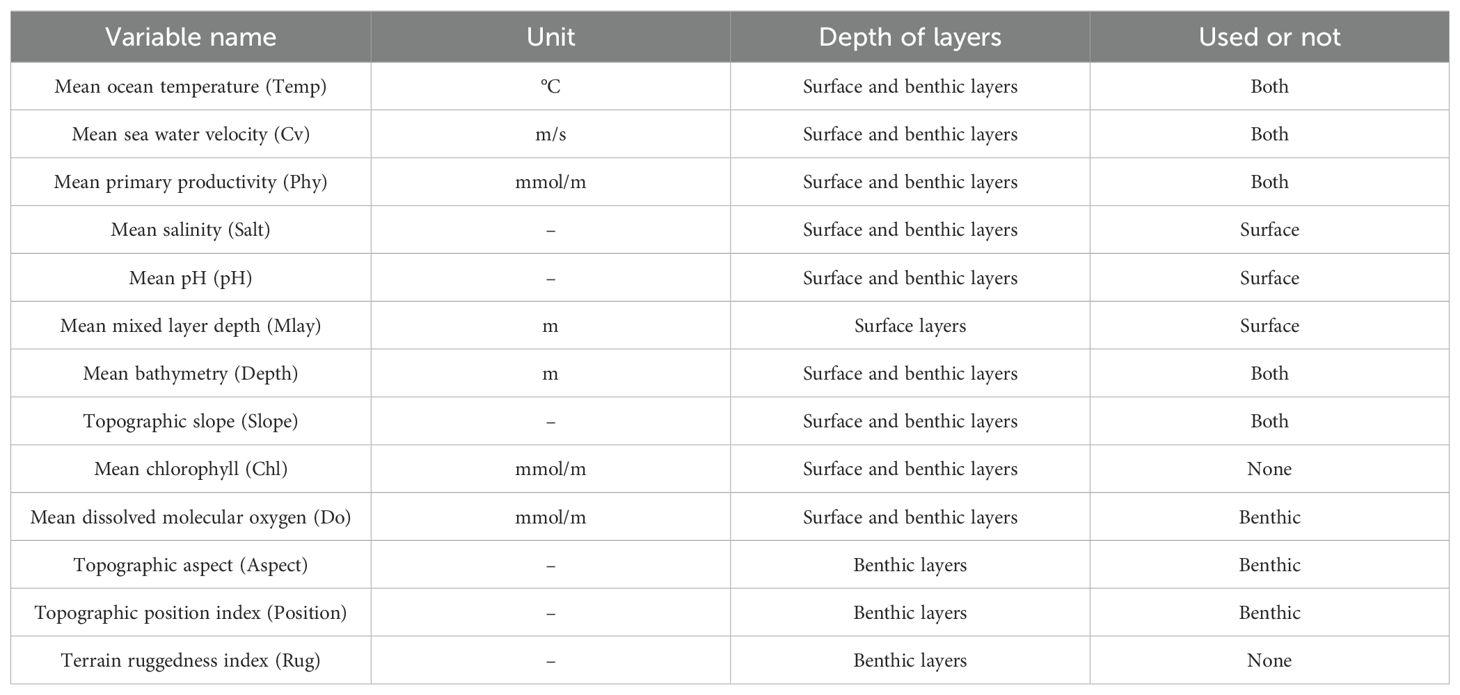
Table 2. The environmental variables used at different depth layers, their units, and final selection in the modeling process.
We categorized the environmental variables into three groups for distinguishing the contributions of different types of variables on species distribution: the physical oceanographic group (POG), the physiology-based group (PBG), and the substrate group (SG) (Pickens et al., 2021). The POG included the predictors of mean bathymetry, mean sea water velocity, and mean mixed layer depth. The PBG included the predictors of mean ocean temperature, mean dissolved oxygen concentration, mean salinity, and mean pH. Mean primary productivity is usually calculated based on phytoplankton abundance, which affects the prey distribution of these important economical species (Roxy et al., 2016; Lewis et al., 2020). Thus, we added this factor to the physiology-based group. The SG included the predictors of topographic slope, topographic position index, and topographic aspect.
To predict and compare the distribution changes of species under future climate scenarios, we accessed future climate data also from the online database Bio-ORACLE v3.0. This database provides free downloads of future environmental conditions under various emission scenarios based on the Shared Socioeconomic Pathways (SSPs) outlined in the Intergovernmental Panel on Climate Change’s (IPCC) Sixth Assessment Report (AR6) (Assis et al., 2024). For our study, we selected two future time periods: 2040-2060 and 2080-2100, and employed three SSP scenarios to represent varying challenges of climate change: SSP1-2.6 (sustainability), SSP2-4.5 (middle of the road), and SSP5-8.5 (fossil-fueled development) (O’Neill et al., 2017). The topographic factors were assumed to be unchanged over time.
Building prediction model
We used stacked species distribution models supported by the R package SSDM to model the distribution of nine ecologically significant species in the Northwest Pacific Ocean and their assemblages (Schmitt et al., 2017). Compared to other SDM packages such as ‘sdm’ and ‘biomod2’, the SSDM package enables the concurrent prediction of distributions for multiple species and facilitates assessing their collective assemblage scenarios (Thuiller et al., 2009; Naimi and Araújo, 2016). This package includes nine distinct modeling algorithms: generalized additive models (GAM), generalized linear models (GLM), multivariate adaptive regression splines (MARS), classification tree analysis (CTA), generalized boosted models (GBM), maximum entropy (MAXENT), artificial neural networks (ANN), random forests (RF), and support vector machines (SVM). Due to lack of actual absence data, we used the method based on Barbet-Massin et al. (2012) embedded in the SSDM package to compute and generate pseudo-absence points for each algorithm. This method has been widely used in the SDM researches (e.g., Mainali et al., 2015; Jarnevich et al., 2015; Naimi and Araújo, 2016). Following the method, the regression techniques such as GAM, GLM, and MARS, as well as for MAXENT achieved an optimal model performance when a large number of pseudo-absence points through random selection were generated. However, classification and machine learning techniques, including GBM, CTA, ANN, RF, and SVM, showed a better model performance when a moderate number of pseudo-absence points were generated by constructing geographical exclusion with a 2° buffer. The number of pseudo-absence points for each model per species was showed in the Supplementary Table 3. The performance of each model was evaluated using a five-fold cross-validation, which was repeated ten times for robustness. This approach randomly partitions the data into a 4:1 ratio, allocating 80% for training and 20% for validation (Guisan et al., 2017). Model transferability and predictive performance were evaluated based on two commonly used evaluation indices: area under the receiver operating characteristic curve (AUC) and true skill statistic (TSS). AUC is calculated based on the retained test data during cross-validation and signals robust model performance if its value exceeds 0.8 (Luo et al., 2017; Castellanos et al., 2019). TSS, calculated as sensitivity (correctly predicted presence points) plus specificity (correctly predicted absence or background points) minus one, indicates the prediction is reliable if its values exceeds 0.7. To reduce uncertainty associated with individual models, a weighted average ensemble model was created using only those models with an AUC value greater than 0.9, implying good predictive accuracy for final prediction (Marmion et al., 2009).
Analysis of predictor contribution
We first calculated the percent contribution of each predictor for the distribution of each species using the permutation method provided by the SSDM package. We then categorized the predictor variables into three groups as previously mentioned and assessed the contribution of each group to the distributions of pelagic and benthic species separately. Because the substrate variables were assumed to be temporally static, we did not analyze the contribution of these variables to the distribution of species. We employed Variation Partitioning Analysis (VPA) to partition the contributions from the physical oceanographic and physiology-based groups into individual and common contributions to the distribution patterns of species (Yang et al., 2023b). For the most important variable, water temperature, we fitted a response curve for each species using the GAM.
Distribution predictions under current and future climatic conditions
We predicted the present-day distribution of each species and its shift in the face of future climate change under three climate change scenarios in the 2040-2060 and 2080-2100. The prediction from the ensemble model is the occurrence probability of species with the value between 0 and 1, indicating the least and best habitat suitability. To better show the habitat distribution of each species, we converted continuous habitat suitability maps into binary format by maximizing sensitivity and specificity equality using the SSDM package (Liu et al., 2013). Species richness per grid cell was calculated by aggregating presence-absence maps of multiple species. Additionally, binary distribution maps for benthic and pelagic species were summed separately to assess species richness across different marine strata (Fitzpatrick et al., 2008). The standard deviation ellipse (SDE) is an effective spatial statistical method for accurately revealing the spatial distribution characteristics of geographic features. The center of the ellipse represents the relative position of distribution center, while the major axis direction indicates the expansion direction of distribution (Ren et al., 2022; Duman et al., 2023). We used SDE to characterize the distribution center and range of species under different climate change scenarios. To compare the distribution shifts among species with diverse ecological preferences, we calculated the proportional changes in their distribution areas across different climate scenarios. Additionally, we estimated the future spatial movement direction and distance of distribution center for each species using the SDMToolbox 2.0 package (Brown, 2014).
Result
Model performance
The model performances evaluated based on AUC and TSS for each individual model revealed variability among species (Supplementary Tables 5, 6). After filtering and weighting, the ensemble models for all the species exhibited improved performances compared with individual models, indicating by the overall increase of AUC and TSS values. The high AUC (above 0.9) and TSS (above 0.8) values implied the good predictive performance of the ensemble models for all the species (Supplementary Table 4).
Contribution of predictor variables
The percentage contributions of predictor variables provided by the SSDM model indicate that mean ocean temperature is the most significant factor in shaping the distribution patterns of benthic species, except for Pacific cod (Figure 2). Depth makes the largest contribution to the distribution of pacific cod, followed by mean dissolved oxygen concentration and mean ocean temperature. Depth contributes the most to the potential distribution of pelagic species, followed by the mean ocean temperature. Altogether, mean ocean temperature plays a substantial role in the distributions of both benthic and pelagic species.
The results of the VPA indicate that 75% of the variation in the distribution of benthic species can be explained by the predictors from the physical oceanographic and physiology-based groups. The physiology-based group contributes the most, explaining 65% of the variation, while the physical oceanographic group explains 30%, and 20% of the contribution is shared by these two groups (Figure 3A). For pelagic species, 72% of the variation in their distribution can be explained by these two groups of predictors, with the physiology-based group again accounting for the majority, explaining 60% of the variation, while the physical oceanographic group accounts for 36%, and 24% of the contribution is shared by these two groups (Figure 3B).
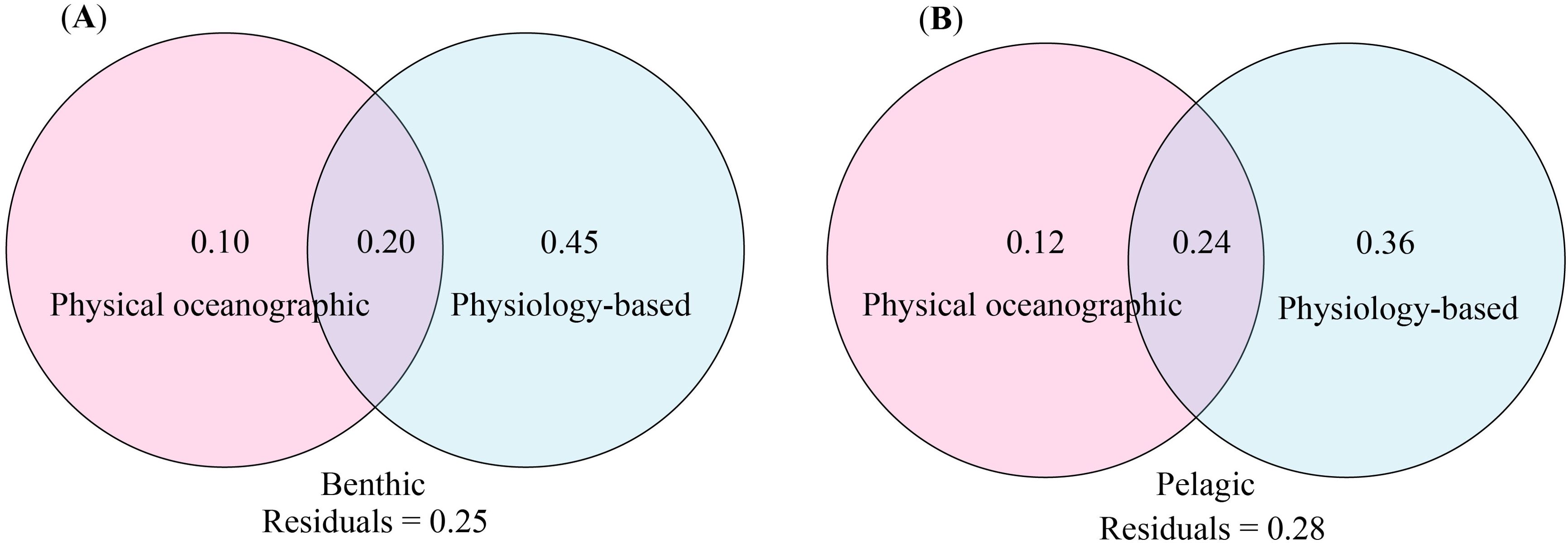
Figure 3. Contributions of different predictor groups to the distributions of benthic (A) and pelagic (B) species calculated by the VPA.
Predicted distribution under current and future climatic conditions
The continuous and binary distribution of habitat suitability for each species under current climatic conditions is shown in Supplementary Figure 2. The overlay of binary distributions over all species shows the distribution of species richness (Figure 4). Areas with high species richness are concentrated along the coast of the Northwest Pacific Ocean, particularly in the shallow waters of the Japan Sea, the Bohai Sea, the Yellow Sea, and the East China Sea (Figure 4).
Compared to the potential distribution areas at present day, areas of high numbers of species will continue to be located along the coast of the Northwest Pacific Ocean in the future. With the increase of greenhouse gas emission concentration, species richness per grid cell exhibits an expected trend of decrease at lower latitudes and increase at higher latitudes. The nearshore areas of the Yellow Sea, the East China Sea, and the Japan Sea will lose many species in the future. However, the coasts of the Okhotsk Sea and the Bohai Sea, as well as some offshore areas at higher latitudes, will attract more species to live in the future. Additionally, the spatial range of change in species richness will expand with the intensification of climate change (Figure 5).
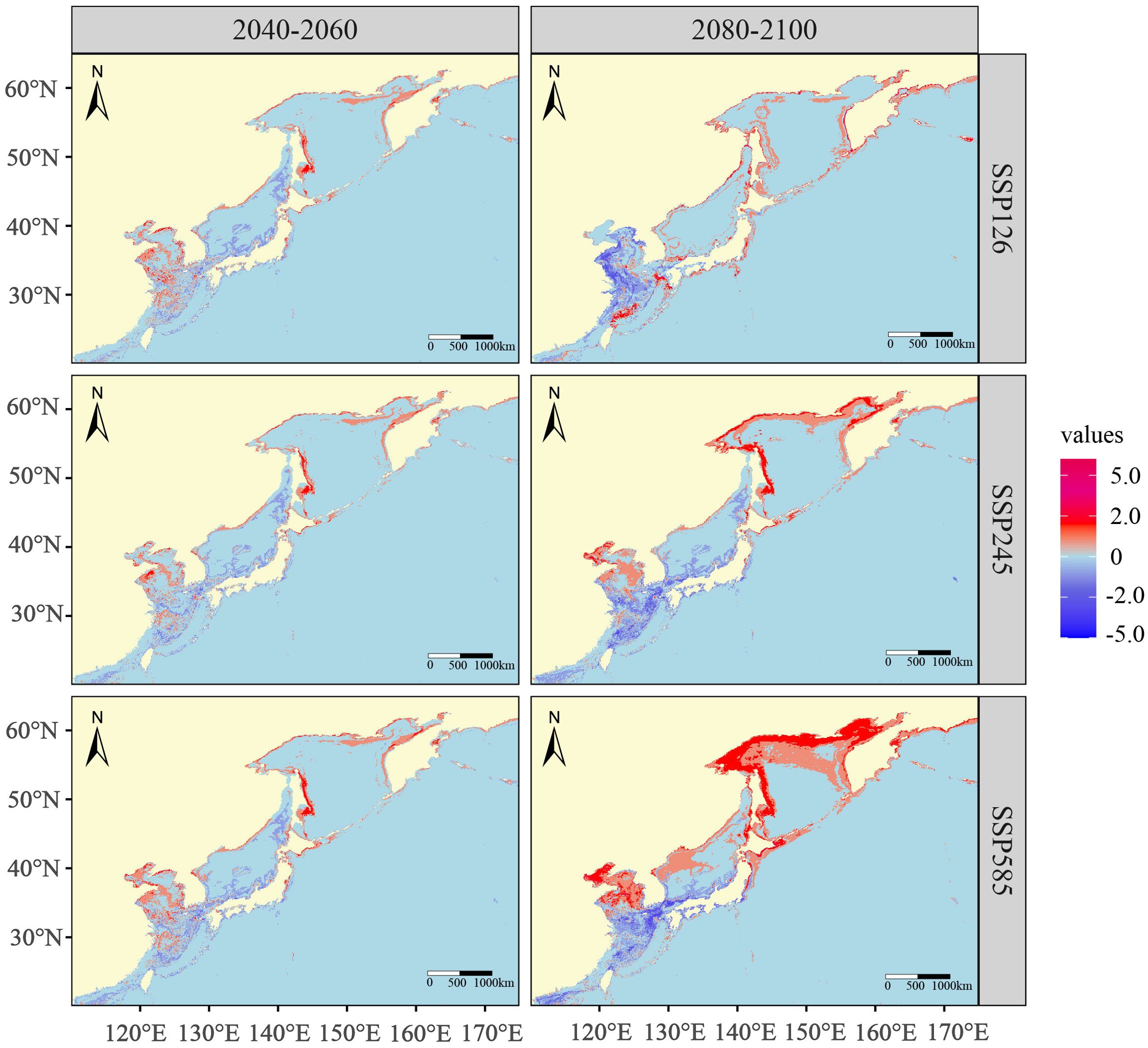
Figure 5. The change of future species richness in the periods 2040-2060 and 2080-2100 under three climate change scenarios.
The results from the standard deviation ellipse analysis show that the main distribution areas of high species richness are located along the Northwestern Pacific coast, centered in the southern part of the Japan Sea, close to the Korea Strait. As climate change progresses, the distribution range gradually expands and the center moves northeastward (Figure 6). The movement distance of the distribution center increases with time and intensity of climate change. It moves northeast by 104.06 km to the southeast area of Busan in the period 2040-2060, and then continues to move northeast by 168.48 km to the southern part of the Sea of Japan under the SSP5-8.5 scenario (Supplementary Table 7).
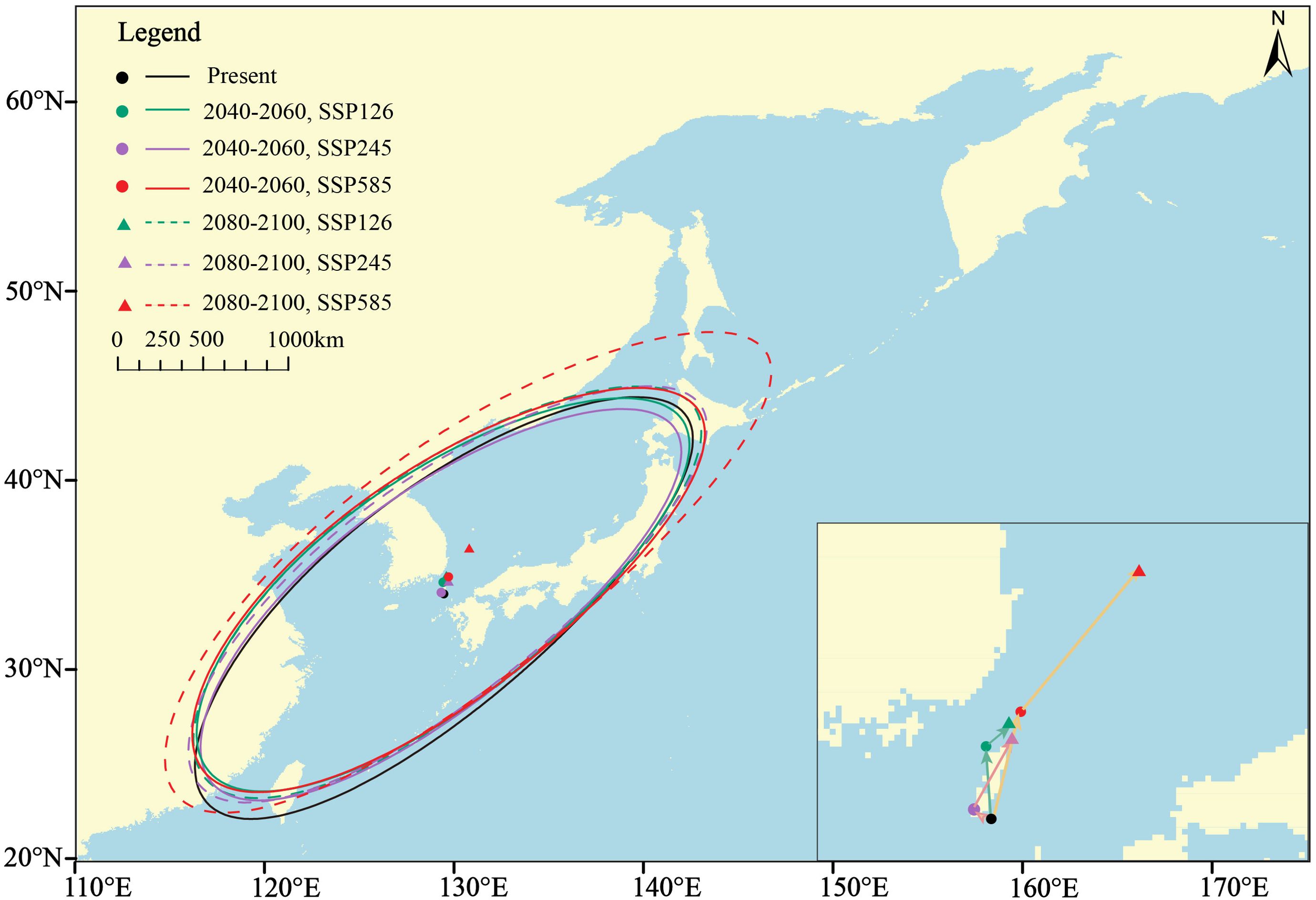
Figure 6. The standard deviation ellipses and distribution centers of species richness under current and future climatic conditions.
Distribution shift of species with different ecological preference
From the species category perspective, our projections indicate a general decline in suitable habitat areas for crustaceans, including Acetes japonicus and Portunus pelagicus. This decline is more pronounced under higher emission scenarios (SSP5-8.5), where both species exhibit substantial contractions in their habitats by 2080-2100. In contrast, changes in fish habitat areas under future climate change depend on their ecological preferences. All the tropical and temperate fishes in our study, including Harpadon nehereus, Trachurus japonicus, and Engraulis japonicus will experience habitat expansion compared to their current habitat. Two subtropical species show opposite trends in habitat change, with an expansion of Scomber japonicus and a contraction of Larimichthys polyactis in the face of future climate change. Two boreal species also show opposite change trends of habitat, with a great expansion of suitable habitats for Clupea pallasii and a slight contraction of suitable habitats for Gadus macrocephalus. Considering the living water strata of species, all four pelagic species are projected to expand their habitats under three climate change scenarios for both periods in the future. In contrast, all five benthic species, except for Harpadon nehereus, will contract their habitats under three climate change scenarios for period 2080-2100 (Table 1; Supplementary Table 8).
The distribution centers of species are linked to their respective climatic zones and typically shift towards higher latitudes as climate change progresses, with migration distance increasing with the intensity of climate change. Under present climatic conditions, Gadus macrocephalus, adapted to boreal climates, exhibits the northernmost distribution, followed by Clupea pallasii. In contrast, the tropical species Harpadon nehereus shows the southernmost distribution. The calculated results of movement distances of the distribution center for each species indicate that crustaceans typically migrate shorter distances than most fish species in response to climate change. Among fish, pelagic species typically exhibit greater migration distances than benthic species. Clupea pallasii shows the longest average migration distance, approximately 431.52 km, followed by Scomber japonicus with an average of 326.37 km. In contrast, the benthic species Harpadon nehereus and Gadus macrocephalus demonstrate shorter average movement distances, at 82.84 km and 287.47 km, respectively. Considering thermal preferences, fish inhabiting boreal and temperate climate zones with higher latitudes demonstrate greater migration distances than those inhabiting at tropical and subtropical zones with lower latitudes, implying that their habitats are more inclined to be impacted by climate change. Notably, the subtropical species Scomber japonicus exhibits a migration distance of 1115.95 km in the periods 2080-2100 under the SSP 5-8.5 scenario that far exceeds other tropical and subtropical fishes (Figure 7; Table 3).
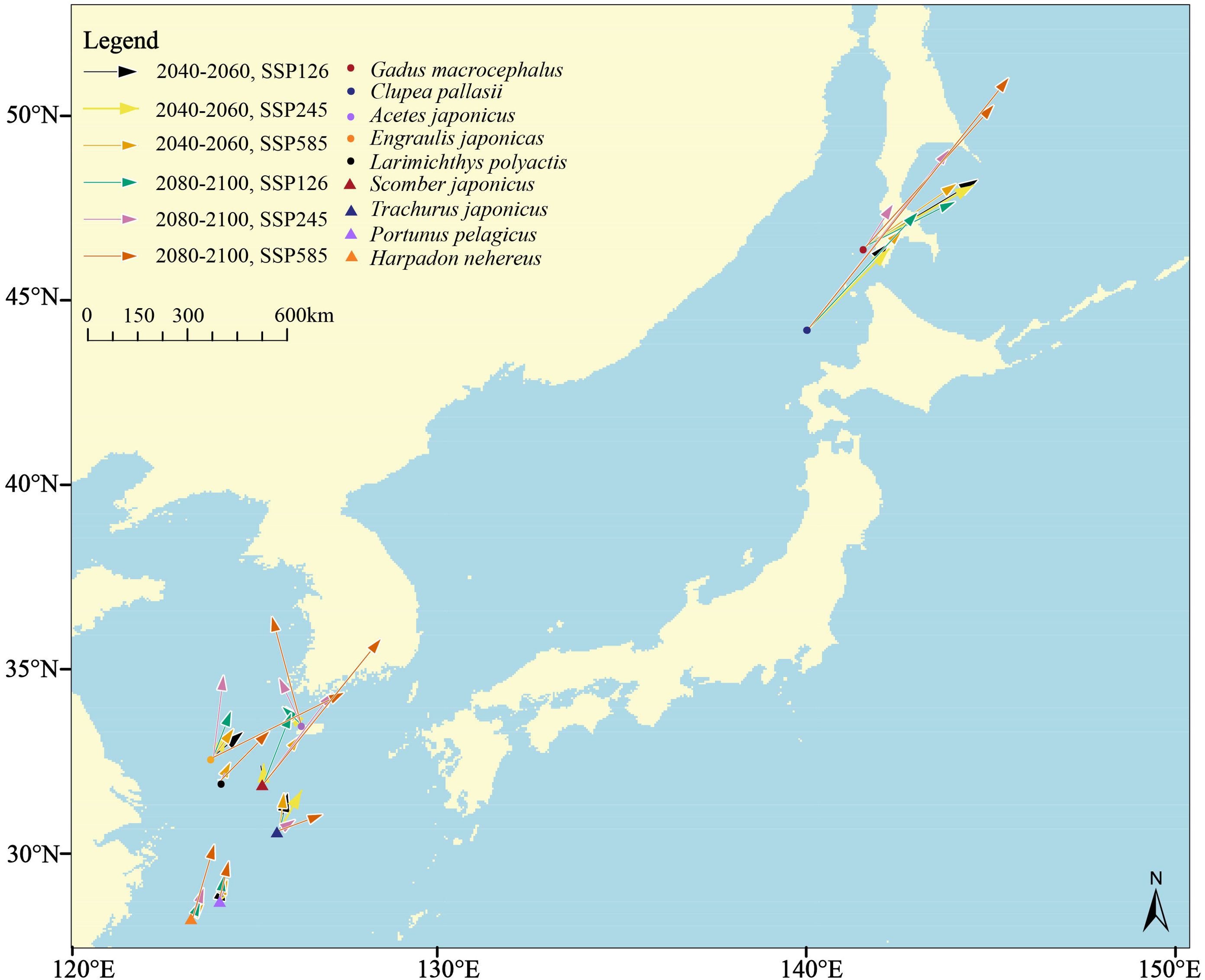
Figure 7. Movement distance and direction of the distribution center of each species indicated by the pointed direction and length of the arrow line, respectively, in the periods 2040-2060 and 2080-2100 under three climate change scenarios.
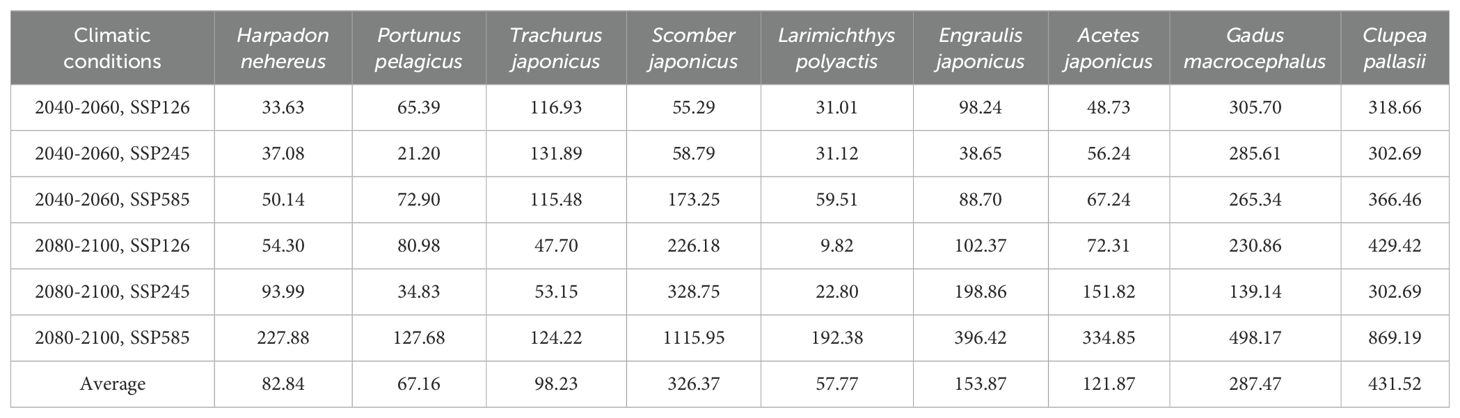
Table 3. Movement distance (km) of the distribution center of each species in the periods 2040-2060 and 2080-2100 under three climate change scenarios.
Discussion
A great deal of research has verified that marine species will generally migrate to higher latitudes or deeper waters to adapt to future climate change, though the adaptability of these species depends on their ecological preferences (e.g., Cheung et al., 2015; Schickele et al., 2021; Weinert et al., 2021; Hu et al., 2022). These climate-induced relocations of species will change the community structure of the global marine ecosystem, resulting in a series of rearrangements in social, economic, and administrative resources (Gaines et al., 2018; Holsman et al., 2019; Sullaway et al., 2021). It is hard to monitor or project future distribution changes of all marine species at a global scale because of the limitations in data acquisition. However, it is practicable and valuable to predict future habitat distribution of ecologically significant species with differential ecological preferences to inform climate-smart management strategies. We selected nine representative economic species in the Northwest Pacific with enough data available to conduct this study to provide knowledge support for the sustainable management of these species.
The relationship of habitat change with ecological preference
Pelagic species seem to be more resilient to changing climate than benthic species, as evidenced by their better ability to colonize new areas in the future. The stronger swimming or dispersal ability of pelagic species facilitates these species to occupy more regions, resulting in a broader range of spatial distribution and environmental niche. Studies have found that climate change facilitates habitat expansions of environmentally tolerant species (Thomas et al., 2016). For instance, our study showed that Scomber japonicus can live within a temperature range of 5-25°C (Supplementary Figure 4), and this broader adaptive range facilitates this species to expand its habitat in the future (Han et al., 2023).
Benthic crustacean species commonly have limited mobility and do not move or move only a short distance in a lifetime. The narrow ranges of spatial distribution and environmental niche may result in vulnerability to future climate change (Green et al., 2014; Cloyed et al., 2021; Dubos et al., 2022). Our study indicates a decrease in suitable habitat for Acetes japonicus and Portunus pelagicus and the reduced extent increases with environmental change intensity. The largest habitat reduction was found in the period 2080-2100 under the SSP5-8.5 scenario. Compared to benthic crustaceans, benthic fish usually possess superior swimming abilities, facilitating them to adapt to a wider range of environmental conditions. However, we found a habitat contraction for Larimichthys polyactis and Gadus macrocephalus, consistent with published studies (Li et al., 2022; Yang et al., 2023a), and a habitat expansion for Harpadon nehereus. This contrasting result implies that climate-induced changes in the benthic environment might mostly compress the distribution ranges of species, especially for cold water species, but can also generate more suitable habitats, especially for warm water species. Wang et al. (2021) predicted a habitat reduction of Harpadon nehereus in the coast of China due to climate change. We used a greater number of predictor factors with updated environmental data to predict a habitat expansion at a larger spatial scale. The differences in data and spatial scale may be the reasons for generating this inconsistent result. Our results indicate that for the cold-water species, Pacific cod (Gadus macrocephalus), habitat area may experience a slight increase under the SSP2-4.5 scenario during the 2040-2060 period compared to other climate scenarios. This increase is likely driven by moderate warming in high-latitude regions, where previously colder areas become more conducive to species distribution (Supplementary Figure 3). Moderate warming extends the range of favorable environmental conditions in these regions, thereby enhancing habitat suitability. Conversely, under SSP1-2.6, which represents lower warming, the temperature increase may be insufficient to significantly expand suitable habitats in colder regions, resulting in a more limited range expansion. For Portunus pelagicus, our results indicate that the SSP2-4.5 and SSP5-8.5 scenarios, compared to SSP1-2.6, may result in the acquisition of more suitable habitats at higher latitudes (Supplementary Figure 3). This is because the Portunus pelagicus, a demersal species with an optimal temperature of 26°C (Supplementary Figure 4; Liao and Li, 2002), benefits from slower rates of environmental change in the demersal layer, which is less immediately affected by climate change (Heuzé et al., 2015). Climate change could gradually shift the species’ suitable habitats to higher latitudes as it seeks optimal environmental conditions.
In terms of climatic zone, we expect that tropical fishes may be more resilient to climate change than fishes in other climate zones because large amounts of suitable habitats may arise in temperate or boreal zones (Nakamura et al., 2013; Osland et al., 2021; Haslam et al., 2023). We predicted habitat expansions and northward movements of the distribution center of tropical species, Trachurus japonicus and Scomber japonicus, in the face of climate change. However, this result may change when the spatial extent of the study area changes. For instance, Xiong et al. (2024) estimated the impact of climate change on the distribution of Trachurus japonicus in the Northern South China Sea and found the distribution of this species expanded northward and contracted southward. The rate of habitat contraction surpassed that of expansion, with an estimated decrease in suitable habitats by the end of the 21st century. When we extended the study area, especially to the north, it is possible to find that the rate of habitat expansion surpasses that of habitat contraction.
In terms of fish size, studies indicated that small pelagic fishes exhibit stronger adaptability to climate change than big fishes (Lefort et al., 2015). Similar to previous findings (Montero-Serra et al., 2015), our results indicate that climate change will drive the small pelagic fish Engraulis japonicus to expand its distribution in the period 2080-2100 under the SSP2-4.5 and SSP5-8.5 scenarios. A long-term change of environmental conditions can gradually motivate genetic variation to adapt to environmental change stresses (Geerts et al., 2015; Scheffers et al., 2016; Krajmerová et al., 2017). Small fishes usually have a short lifespan and reproductive cycle and thus a short time interval in the alternation of generations, which may speed up the genetic variation to adapt to climate change (Munday et al., 2013). Furthermore, Benedetti et al. (2021) predicted that the abundance of diatoms, dinoflagellates, haptophytes, and other plankton will increase in temperate zones, leading to a rise in the biomass of small fish species feeding on them. Climate change is also expected to drive changes in plankton community composition, with a shift towards smaller species at higher latitudes (Henson et al., 2021). This shift could significantly impact the nutritional value and availability of specific prey species, potentially affecting the long-term sustainability of species reliant on them. The strong environmental tolerance and richer food sources induced by climate change are among the reasons for the habitat expansion of small fishes, such as Engraulis japonicas.
We separated predictor variables into three groups, and physiology-based group showed dominant importance in affecting the distribution patterns of species in the Northwest Pacific Ocean. This implies that climate-induced changes in the physiology-based factors may result in physiological maladjustment and then prompt adaptive evolution of species, such as alterations in metabolism, growth patterns, and reproductive strategies (Bates et al., 2014). Though this adaptive evolution differs among species, it may alleviate climate change impact on species.
The implication of species distribution shifts for spatial fisheries management
Our study demonstrated that the distribution centers of all nine species, whether expanding or contracting, will shift northward as a result of climate change. This redistribution may diminish the effectiveness of existing spatial fisheries management strategies. For example, our predictions suggested that Larimichthys polyactis, an economically important species in China, will lose substantial suitable habitats in the East China Sea by 2080-2100 under the SSP5-8.5 scenario. This suggests that current management practices, such as the establishment of national aquatic germplasm resource conservation areas in 2008 to protect spawning grounds for hairtail (Trichiurus haumela) and migratory routes of small yellow croaker (Larimichthys polyactis), will become less effective by the end of the 21st century.
Furthermore, these distribution shifts of economically significant species may make transboundary fisheries face more serious challenges because these fisheries are prone to over exploitation even if climate change effects on stocks were taken into account (Liu and Molina, 2021). The inconsistent management policies between nations may exacerbate these challenges. Our predictions suggested that Pacific cod (Gadus macrocephalus) habitats will shrink in the China Seas and Sea of Japan while increasing in the Sea of Okhotsk. This distribution shift is unfavorable to China, Japan, and Korea, while benefiting Russia. Similar scenarios will occur for other species in various regions (Astthorsson et al., 2012; Ojea et al., 2020). These new distribution patterns induced by climate change underscore the necessity for strengthening international cooperation to manage transboundary stocks. Regional Fisheries Management Organizations (RFMOs), such as the South Pacific Regional Fisheries Management Organization (for jack mackerel), will face growing pressure to adapt their frameworks to prevent overexploitation and balance stakeholder interests on shared stocks.
Spatial fisheries management tools are critical for the sustainability of stocks. For instance, Liu et al. (2018) applied these tools to address stock migration on groundfish fisheries, offering a potential model for adapting to climate-induced distribution shifts. While long-term fishery resource surveys are critical for designing fishery management strategies, predictive models can predict future distribution of species under different climate scenarios, allowing managers to explore and plan for possible changes in biomass or distribution, rather than being caught on the back foot once it has already happened. Therefore, our models can be as part of a toolkit that fisheries managers can use to ensure sustainability of fish stocks into the future.
Limitations of the study
Though our study is significant in informing climate-smart fishery management strategies in the Northwest Pacific, there are several limitations that need to be addressed in the future work. First, although our modeling results indicate that species with different ecological preferences exhibit varying distributional responses to climate change, the intrinsic assumption of predictive models is that the niche space of each species does not change over time (Guisan et al., 2017; Smith et al., 2019). Ignoring intraspecific climate adaptation and population response variability to climate change may diminish prediction accuracy (Hällfors et al., 2016). Mechanistic models that incorporate population genomics are suggested to offer more reliable estimates than conventional statistical models. Overlooking adaptive evolution may lead to an overestimation of climate impacts on species’ habitats (Robinson et al., 2011; Razgour et al., 2019). Second, our predictions assumed that all species have unrestricted dispersal capabilities. This over-optimistic assumption implied that each species can migrate to all suitable areas under climate change. However, ecological and man-made barriers and species mobility may limit some species from reaching suitable habitats (Tamario et al., 2019; Liang et al., 2021). Therefore, this assumption undoubtedly overestimated species’ suitability to climate change. Third, due to data limitations, our study considered only environmental influences, excluding biological factors and interactions among them such as predation, competition, parasitism, mutualism, and facilitation. These relationships can interact in complex ways with climate change, influencing species’ spatial distribution patterns (Tylianakis et al., 2008; Braschler and Hill, 2007). For example, Estes et al. (2011) suggest that large apex predators can significantly limit the distribution and range of prey species in marine ecosystems. The exclusion of such interactions from our models may lead to an overestimation of available habitats for prey species, as predatory pressures are not considered. Fourth, most economically important species inhabit coastal continental shelf waters, where commonly face heavy fishing pressure and pollutions from human activities (Eigaard et al., 2017; Palummo et al., 2023). Ignoring fishing pressure and other disturbances by human activities will undoubtly overestimate the distribution range of species.
Conclusion
The Northwest Pacific Ocean is an important fishing ground in the world, and the targeted species are undergoing strong climate change stresses. We developed stacked species distribution models (SSDMs) to predict distribution shifts of nine ecologically significant species with diverse ecological preferences in the Northwest Pacific Ocean under climate change. Our predictions indicate that all species will migrate towards higher latitudes, resulting in high species richness in these areas, and the migration distance depends on ecological preference. Specifically, crustaceans and demersal fishes are projected to experience habitat contraction, whereas most pelagic fishes are projected to expand their habitats. Additionally, pelagic species, particularly highly migratory species, tend to migrate further distance than demersal species. The differential response of species to climate change highlights the importance of formulating species-specific climate-adaptive fishery management strategies. Our study can provide fundamental data for designing these strategies for maintaining fishery sustainability in the Northwest Pacific Ocean under the continuous impact of climate change.
Data availability statement
The original contributions presented in the study are included in the article/Supplementary Material. Further inquiries can be directed to the corresponding author.
Ethics statement
Ethical approval was not required for the study involving animals in accordance with the local legislation and institutional requirements because we used the data downloaded from the online datasets and published papers to conduct our study and did not carry out the field work.
Author contributions
WD: Data curation, Formal analysis, Software, Writing – original draft. XB: Supervision, Validation, Visualization, Writing – review & editing. LZ: Conceptualization, Methodology, Validation, Writing – review & editing. HD: Methodology, Software, Supervision, Visualization, Writing – review & editing. CL: Conceptualization, Methodology, Writing – review & editing.
Funding
The author(s) declare that no financial support was received for the research, authorship, and/or publication of this article.
Conflict of interest
The authors declare that the research was conducted in the absence of any commercial or financial relationships that could be construed as a potential conflict of interest.
Publisher’s note
All claims expressed in this article are solely those of the authors and do not necessarily represent those of their affiliated organizations, or those of the publisher, the editors and the reviewers. Any product that may be evaluated in this article, or claim that may be made by its manufacturer, is not guaranteed or endorsed by the publisher.
Supplementary material
The Supplementary Material for this article can be found online at: https://www.frontiersin.org/articles/10.3389/fmars.2024.1476097/full#supplementary-material
References
Andersen N. F., Cavan E. L., Cheung W. W., Martin A. H., Saba G. K., Sumaila U.R.J.N.O.S. (2024). Good fisheries management is good carbon management. NPJ Ocean Sustain. 3, 17. doi: 10.1038/s44183-024-00053-x
Assis J., Fernández Bejarano S. J., Salazar V. W., Schepers L., Gouvêa L., Fragkopoulou E., et al. (2024). Bio-ORACLE v3. 0. Pushing marine data layers to the CMIP6 Earth System Models of climate change research. Global Ecol. Biogeogr. 33, e13813. doi: 10.1111/geb.13813
Assis J., Tyberghein L., Bosch S., Verbruggen H., Serrão E. A., De Clerck O. J. G. E., et al. (2018). Bio-ORACLE v2. 0: Extending marine data layers for bioclimatic modelling. Global Ecol. Biogeogr. 27, 277–284. doi: 10.1111/geb.12693
Astthorsson O. S., Valdimarsson H., Gudmundsdottir A., Oskarsson G. J. (2012). Climate-related variations in the occurrence and distribution of mackerel (Scomber scombrus) in Icelandic waters. Ices J. Mar. Sci. 69, 1289–1297. doi: 10.1093/icesjms/fss084
Barbet-Massin M., Jiguet F., Albert C. H., Thuiller W.J.M.I.E. (2012). Selecting pseudo-absences for species distribution models: How, where and how many. Methods Ecol. Evol. 3, 327–338. doi: 10.1111/j.2041-210X.2011.00172.x
Bates A. E., Pecl G. T., Frusher S., Hobday A. J., Wernberg T., Smale D. A., et al. (2014). Defining and observing stages of climate-mediated range shifts in marine systems. Global Environ. Change 26, 27–38. doi: 10.1016/j.gloenvcha.2014.03.009
Bell J. D., Senina I., Adams T., Aumont O., Calmettes B., Clark S., et al. (2021). Pathways to sustaining tuna-dependent Pacific Island economies during climate change. Nat. Sustain. 4, 900–910. doi: 10.1038/s41893-021-00745-z
Benedetti F., Vogt M., Elizondo U. H., Righetti D., Zimmermann N. E., Gruber N. (2021). Major restructuring of marine plankton assemblages under global warming. Nat. Commun. 12, 5226. doi: 10.1038/s41467-021-25385-x
Bradie J., Leung B. (2017). A quantitative synthesis of the importance of variables used in MaxEnt species distribution models. J. Biogeogr. 44, 1344–1361. doi: 10.1111/jbi.12894
Braschler B., Hill J. K. (2007). Role of larval host plants in the climate-driven range expansion of the butterfly Polygonia c-album. J. Anim. Ecol. 76, 415–423. doi: 10.1111/j.1365-2656.2007.01217.x
Brown J. L. (2014). SDM toolbox: a python-based GIS toolkit for landscape genetic, biogeographic and species distribution model analyses. Methods Ecol. Evol. 5, 694–700. doi: 10.1111/2041-210X.12200
Calabrese J. M., Certain G., Kraan C., Dormann C. F. (2014). Stacking species distribution models and adjusting bias by linking them to macroecological models. Global Ecol. Biogeogr. 23, 99–112. doi: 10.1111/geb.12102
Castellanos A. A., Huntley J. W., Voelker G., Lawing A. M. (2019). Environmental filtering improves ecological niche models across multiple scales. Methods Ecol. Evol. 10, 481–492. doi: 10.1111/2041-210X.13142
Cavalli R. O., Wasielesky W., Peixoto S., Poersch L. H. D., Santos M. H. S., Soares R. B. (2008). Shrimp farming as an alternative to artisanal fishermen communities: the case of patos lagoon, Brazil. Arch. Biol. Technol. 51, 991–1001. doi: 10.1590/S1516-89132008000500016
Cheng L. J., Abraham J., Trenberth K. E., Fasullo J., Boyer T., Mann M. E., et al. (2022). Another Record: Ocean Warming Continues through 2021 despite La Nina Conditions. Adv. Atmos. Sci. 39, 373–385. doi: 10.1007/s00376-022-1461-3
Cheung W. W. L., Brodeur R. D., Okey T. A., Pauly D. (2015). Projecting future changes in distributions of pelagic fish species of Northeast Pacific shelf seas. Prog. Oceanogr. 130, 19–31. doi: 10.1016/j.pocean.2014.09.003
Cheung W. W. L., Frolicher T. L., Lam V. W. Y., Oyinlola M. A., Reygondeau G., Sumaila U. R., et al. (2021). Marine high temperature extremes amplify the impacts of climate change on fish and fisheries. Sci. Adv. 7, eabh0895. doi: 10.1126/sciadv.abh0895
Cloyed C. S., Balmer B. C., Schwacke L. H., Wells R. S., McCabe E. B. J., Barleycorn A. A., et al. (2021). Interaction between dietary and habitat niche breadth influences cetacean vulnerability to environmental disturbance. Ecosphere. 12, e03759. doi: 10.1002/ecs2.3759
D’Amen M., Pradervand J. N., Guisan A. (2015). Predicting richness and composition in mountain insect communities at high resolution: a new test of the SESAM framework. J. Biogeogr. 42, 1255–1266. doi: 10.1111/geb.12357
Do A. N. T., Tran H. D., Ashley M. (2022). Employing a novel hybrid of GA-ANFIS model to predict distribution of whiting fish larvae and juveniles from tropical estuaries in the context of climate change. Ecol. Inform. 71, 101780. doi: 10.1016/j.ecoinf.2022.101780
Doney S. C., Ruckelshaus M., Duffy J. E., Barry J. P., Chan F., English C. A., et al. (2012). Climate change impacts on marine ecosystems. Ann. Rev. Mar. Sci. 4, 11–37. doi: 10.1146/annurev-marine-041911-111611
Dormann C. F., Elith J., Bacher S., Buchmann C., Carl G., Carré G., et al. (2013). Collinearity: a review of methods to deal with it and a simulation study evaluating their performance. Ecography. 36, 27–46. doi: 10.1111/j.1600-0587.2012.07348.x
Dubos N., Montfort F., Grinand C., Nourtier M., Deso G., Probst J.-M., et al. (2022). Are narrow-ranging species doomed to extinction? Projected dramatic decline in future climate suitability of two highly threatened species. Perspect. Ecol. Conser. 20, 18–28. doi: 10.1016/j.pecon.2021.10.002
Dubuis A., Pottier J., Rion V., Pellissier L., Theurillat J. P., Guisan A. (2011). Predicting spatial patterns of plant species richness: a comparison of direct macroecological and species stacking modelling approaches. Divers. Distrib. 17, 1122–1131. doi: 10.1111/j.1472-4642.2011.00792.x
Duman Z., Mao X., Cai B., Zhang Q., Chen Y., Gao Y., et al. (2023). Exploring the spatiotemporal pattern evolution of carbon emissions and air pollution in Chinese cities. J. Environ. Manage. 345, 118870. doi: 10.1016/j.jenvman.2023.118870
Eigaard O. R., Bastardie F., Hintzen N. T., Buhl-Mortensen L., Buhl-Mortensen P., Catarino R., et al. (2017). The footprint of bottom trawling in European waters: distribution, intensity, and seabed integrity. ICES J. Mar. Sci. 74, 847–865. doi: 10.1093/icesjms/fsw194
Estes J. A., Terborgh J., Brashares J. S., Power M. E., Berger J., Bond W. J. (2011). Trophic downgrading of planet Earth. Science 333, 301–306. doi: 10.1126/science.1205106
FAO. (2021). FAO yearbook. Fishery and aquaculture statistics 2019/FAO annuaire (Rome: FAO). doi: 10.4060/cb7874t
FAO. (2022). “The state of world fisheries and aquaculture 2022,” in Towards blue transformation (FAO, Rome). doi: 10.4060/cc0461en
Ferrier S., Guisan A. (2006). Spatial modelling of biodiversity at the community level. J. Appl. Ecol. 43, 393–404. doi: 10.1111/j.1365-2664.2006.01149.x
Fischlin A., Midgley G. F., Price J., Leemans R., Gopal B., Turley C., et al. (2007). “Ecosystems, their properties, goods and services,” in Climate change 2007: impacts, adaptation and vulnerability. Contribution of Working Group II to the Fourth Assessment Report of the Intergovernmental Panel on Climate Change (Cambridge University Press, Cambridge), 211–272.
Fitzpatrick M. C., Gove A. D., Sanders N. J., Dunn R. R. (2008). Climate change, plant migration, and range collapse in a global biodiversity hotspot: the Banksia (Proteaceae) of Western Australia. Global Change Biol. 14, 1337–1352. doi: 10.1111/j.1365-2486.2008.01559.x
Gaines S. D., Costello C., Owashi B., Mangin T., Bone J., Molinos J. G., et al. (2018). Improved fisheries management could offset many negative effects of climate change. Sci. Adv. 4, eaao1378. doi: 10.1126/sciadv.aao1378
Geerts A., Vanoverbeke J., Vanschoenwinkel B., Van Doorslaer W., Feuchtmayr H., Atkinson D., et al. (2015). Rapid evolution of thermal tolerance in the water flea Daphnia. Nat. Clim. Change. 5, 665–668. doi: 10.1038/NCLIMATE2628
Green B. S., Gardner C., Hochmuth J. D., Linnane A. (2014). Environmental effects on fished lobsters and crabs. Rev. Fish Biol. Fisher. 24, 613–638. doi: 10.1007/s11160-014-9350-1
Guisan A., Thuiller W. (2005). Predicting species distribution: offering more than simple habitat models. Ecol. Lett. 8, 993–1009. doi: 10.1111/j.1461-0248.2005.00792.x
Guisan A., Thuiller W., Zimmermann N. E. (2017). Habitat suitability and distribution models: with applications in R (Cambridge: Cambridge University Press). doi: 10.1017/9781139028271
Hall C. A. M., Lewandowska A. M. (2022). Zooplankton dominance shift in response to climate-driven salinity change: A mesocosm study. Front. Mar. Sci. 9. doi: 10.3389/fmars.2022.861297
Hällfors M. H., Liao J., Dzurisin J., Grundel R., Hyvärinen M., Towle K., et al. (2016). Addressing potential local adaptation in species distribution models: implications for conservation under climate change. Ecol. Appl. 26, 1154–1169. doi: 10.1890/15-0926
Han H., Yang C., Jiang B., Shang C., Sun Y., Zhao X., et al. (2023). Construction of chub mackerel (Scomber japonicus) fishing ground prediction model in the northwestern Pacific Ocean based on deep learning and marine environmental variables. Mar. pollut. Bull. 193, 115158. doi: 10.1016/j.marpolbul.2023.115158
Haslam V. M., Bessey C., Chaplin J. A., van Keulen M. (2023). Evidence of corallivorous Drupella cornus breeding on the higher latitude reefs of Rottnest Island (32° S), Western Australia. Mar. Biol. 171, 1–28. doi: 10.21203/rs.3.rs-2905663/v1
Heinze C. (2014). The role of the ocean carbon cycle in climate change. Eur. Rev. 22, 97–105. doi: 10.1017/S1062798713000665
Henson S. A., Cael B. B., Allen S. R., Dutkiewicz S. (2021). Future phytoplankton diversity in a changing climate. Nat. Commun. 12, 5372. doi: 10.1038/s41467-021-25699-w
Heuzé C., Heywood K. J., Stevens D. P., Ridley J. K. (2015). Changes in global ocean bottom properties and volume transports in CMIP5 models under climate change scenarios. J. Climate 28, 2917–2944. doi: 10.1175/JCLI-D-14-00381.1
Holsman K. K., Hazen E. L., Haynie A., Gourguet S., Hollowed A., Bograd S. J., et al. (2019). Towards climate resiliency in fisheries management. Ices J. Mar. Sci. 76, 1368–1378. doi: 10.1093/icesjms/fsz031
Hu W. J., Du J. G., Su S. K., Tan H. J., Yang W., Ding L. K., et al. (2022). Effects of climate change in the seas of China: Predicted changes in the distribution of fish species and diversity. Ecol. Indic. 134, 108489. doi: 10.1016/j.ecolind.2021.108489
Intergovernmental Panel on Climate Change (2021).Climate change. In: The physical science basis. Available online at: https://www.ipcc.ch/report/ar6/wg1/ (Accessed 3 December 2022).
Jarnevich C. S., Stohlgren T. J., Kumar S., Morisette J. T., Holcombe T. R. (2015). Caveats for correlative species distribution modeling. Ecol. Inform. 29, 6–15. doi: 10.1016/j.ecoinf.2015.06.007
Kim J. H. (2019). Multicollinearity and misleading statistical results. Korean J. Anesthesiol. 72, 558–569. doi: 10.4097/kja.19087
Krajmerová D., Hrivnák M., Ditmarová L., Jamnická G., Kmet’ J., Kurjak D., et al. (2017). Nucleotide polymorphisms associated with climate, phenology and physiological traits in European beech (Fagus sylvatica L.). New Forest. 48, 463–477. doi: 10.1007/s11056-017-9573-9
Lefort S., Aumont O., Bopp L., Arsouze T., Gehlen M., Maury O. (2015). Spatial and body-size dependent response of marine pelagic communities to projected global climate change. Global Change Biol. 21, 154–164. doi: 10.1111/gcb.12679
Lewis K. M., van Dijken G. L., Arrigo K. R. (2020). Changes in phytoplankton concentration now drive increased Arctic Ocean primary production. Science. 369, 198–202. doi: 10.1126/science.aay8380
Li L. J., Zhao L. L., Fu J. B., Sun B., Liu C. D. (2022). Predicting the habitat suitability for populations of Pacific cod under different climate change scenarios considering intraspecific genetic variation. Ecol. Indic. 142, 109248. doi: 10.1016/j.ecolind.2022.109248
Liang J., Ding Z., Jiang Z., Yang X., Xiao R., Singh P. B., et al. (2021). Climate change, habitat connectivity, and conservation gaps: a case study of four ungulate species endemic to the Tibetan Plateau. Landscape Eco. 36, 1071–1087. doi: 10.1007/s10980-021-01202-0
Liao Y. Y., Li X. M. (2002). Demands of Portunus pelagicus for environmental conditions. Acta Oceanologica Sin. 24, 140–145.
Liu O. R., Kleisner K. M., Smith S. L., Kritzer J. P. (2018). The use of spatial management tools in rights-based groundfish fisheries. Fish Fish. 19, 821–838. doi: 10.1111/faf.12294
Liu O. R., Molina R. (2021). The persistent transboundary problem in marine natural resource management. Front. Mar. Sci. 8. doi: 10.3389/fmars.2021.656023
Liu J., Ning P. (2011). Species composition and faunal characteristics of fishes in the Yellow Sea. Biodiv. Sci. 19, 764. doi: 10.1111/jbi.12058
Liu O. R., Ward E. J., Anderson S. C., Andrews K. S., Barnett L. A. K., Brodie S., et al. (2023). Species redistribution creates unequal outcomes for multispecies fisheries under projected climate change. Sci. Adv. 9, eadg5468. doi: 10.1126/sciadv.adg5468
Liu C., White M., Newell G. (2013). Selecting thresholds for the prediction of species occurrence with presence-only data. J. Biogeogr. 40, 778–789. doi: 10.1111/jbi.12058
Luo M., Wang H., Lyu Z. (2017). Evaluating the performance of species distribution models Biomod2 and MaxEnt using the giant panda distribution data. Chin. J. Appl. Ecol. 28, 4001–4006. doi: 10.13287/j.1001-9332.201712.011
Mainali K. P., Warren D. L., Dhileepan K., McConnachie A., Strathie L., Hassan G., et al. (2015). Projecting future expansion of invasive species: comparing and improving methodologies for species distribution modeling. Global Change Biol. 21, 4464–4480. doi: 10.1111/gcb.13038
Marmion M., Parviainen M., Luoto M., Heikkinen R. K., Thuiller W. (2009). Evaluation of consensus methods in predictive species distribution modelling. Divers. Distrib. 15, 59–69. doi: 10.1111/j.1472-4642.2008.00491.x
Me A.-L., Ra B., Radosavljevic A., Vilela B., Rp A. J. E. (2015). An R package for spatial thinning of species occurrence records for use in ecological niche models. Ecography. 38, 541–545. doi: 10.1111/ecog.01132
Melo-Merino S. M., Reyes-Bonilla H., Lira-Noriega A. (2020). Ecological niche models and species distribution models in marine environments: A literature review and spatial analysis of evidence. Ecol. Model. 415, 108837. doi: 10.1016/j.ecolmodel.2019.108837
Montero-Serra I., Edwards M., Genner M. J. (2015). Warming shelf seas drive the subtropicalization of European pelagic fish communities. Glob. Change Biol. 21, 144–153. doi: 10.1111/gcb.12747
Munday P. L., Warner R. R., Monro K., Pandolfi J. M., Marshall D. J. (2013). Predicting evolutionary responses to climate change in the sea. Ecol. Lett. 16, 1488–1500. doi: 10.1111/ele.12185
Naimi B., Araújo M. B. (2016). sdm: a reproducible and extensible R platform for species distribution modelling. Ecography. 39, 368–375. doi: 10.1111/ecog.01881
Nakamura Y., Feary D. A., Kanda M., Yamaoka K. (2013). Tropical fishes dominate temperate reef fish communities within western Japan. PloS One 8, e81107. doi: 10.1371/journal.pone.0081107
O’Neill B. C., Kriegler E., Ebi K. L., Kemp-Benedict E., Riahi K., Rothman D. S., et al. (2017). The roads ahead: Narratives for shared socioeconomic pathways describing world futures in the 21st century. Global Environ. Change 42, 169–180. doi: 10.1016/j.gloenvcha.2015.01.004
Ojea E., Lester S. E., Salgueiro-Otero D. (2020). Adaptation of fishing communities to climate-driven shifts in target species. One Earth. 2, 544–556. doi: 10.1016/j.oneear.2020.05.012
Osland M. J., Stevens P. W., Lamont M. M., Brusca R. C., Hart K. M., Waddle J. H., et al. (2021). Tropicalization of temperate ecosystems in North America: The northward range expansion of tropical organisms in response to warming winter temperatures. Glob. Change Biol. 27, 3009–3034. doi: 10.1111/gcb.15563
Palummo V., Milisenda G., Canese S., Salvati E., Pica D., Passarelli A., et al. (2023). Effect of environmental and anthropogenic factors on the distribution and co-occurrence of cold-water corals. Front. Mar. Sci. 10. doi: 10.3389/fmars.2023.1272066
Panzeri D., Reale M., Cossarini G., Salon S., Carlucci R., Spedicato M. T., et al. (2024). Future distribution of demersal species in a warming Mediterranean sub-basin. Front. Mar. Sci. 11. doi: 10.3389/fmars.2024.1308325
Phillips S. J., Anderson R. P., Dudík M., Schapire R. E., Blair M. E. J. E. (2017). Opening the black box: An open-source release of Maxent. Ecography. 40, 887–893. doi: 10.1111/ecog.03049
Pickens B. A., Carroll R., Schirripa M. J., Forrestal F., Friedland K. D., Taylor J. C. (2021). A systematic review of spatial habitat associations and modeling of marine fish distribution: a guide to predictors, methods, and knowledge gaps. PloS One 16, e0251818. doi: 10.1371/journal.pone.0251818
Pittman S. J., Brown K. A. (2011). Multi-scale approach for predicting fish species distributions across coral reef seascapes. PloS One 6, e20583. doi: 10.1371/journal.pone.0020583
Razgour O., Forester B., Taggart J. B., Bekaert M., Juste J., Ibanez C., et al. (2019). Considering adaptive genetic variation in climate change vulnerability assessment reduces species range loss projections. PNAS. 116, 10418–10423. doi: 10.1073/pnas.1820663116
Ren S., Song C., Ye S., Cheng C., Gao P. (2022). The spatiotemporal variation in heavy metals in China’s farmland soil over the past 20 years: a meta-analysis. Sci. Total Environ. 806, 150322. doi: 10.1016/j.scitotenv.2021.150322
Robinson L. M., Elith J., Hobday A. J., Pearson R. G., Kendall B. E., Possingham H. P., et al. (2011). Pushing the limits in marine species distribution modelling: lessons from the land present challenges and opportunities. Glob. Ecol. Biogeogr. 20, 789–802. doi: 10.1111/j.1466-8238.2010.00636.x
Roxy M. K., Modi A., Murtugudde R., Valsala V., Panickal S., Kumar S. P., et al. (2016). A reduction in marine primary productivity driven by rapid warming over the tropical Indian Ocean. Geophys. Res. Lett. 43, 826–833. doi: 10.1002/2015gl066979
Sabal M. C., Richerson K., Moran P., Levi T., Tuttle V. J., Banks M. J. F., et al. (2023). Warm oceans exacerbate Chinook salmon bycatch in the Pacific hake fishery driven by thermal and diel depth-use behaviours. Fish Fish. 24, 910–923. doi: 10.1111/faf.12775
Sallée J.-B., Pellichero V., Akhoudas C., Pauthenet E., Vignes L., Schmidtko S., et al. (2021). Summertime increases in upper-ocean stratification and mixed-layer depth. Nature. 591, 592–598. doi: 10.1038/s41586-021-03303-x
Scheffers B. R., De Meester L., Bridge T. C., Hoffmann A. A., Pandolfi J. M., Corlett R. T., et al. (2016). The broad footprint of climate change from genes to biomes to people. Science 354, aaf7671. doi: 10.1126/science.aaf7671
Schickele A., Goberville E., Leroy B., Beaugrand G., Hattab T., Francour P., et al. (2021). European small pelagic fish distribution under global change scenarios. Fish Fish. 22, 212–225. doi: 10.1111/faf.12515
Schickele A., Leroy B., Beaugrand G., Goberville E., Hattab T., Francour P., et al. (2020). Modelling European small pelagic fish distribution: Methodological insights. Ecol. Model. 416, 108902. doi: 10.1016/j.ecolmodel.2019.108902
Schmitt S., Pouteau R., Justeau D., de Boissieu F., Birnbaum P. (2017). ssdm: An r package to predict distribution of species richness and composition based on stacked species distribution models. Methods Ecol. Evol. 8, 1795–1803. doi: 10.1111/2041-210x.12841
Sioni L., Zupa W., Calculli C., Garofalo G., Hidalgo M., Jadaud A., et al. (2019). Spatial distribution pattern of European hake, Merluccius merluccius (Pisces: Merlucciidae), in the Mediterranean Sea. Sci. Mar. 83, 21–32. doi: 10.3989/scimar.04988.12A
Smale D. A., Wernberg T., Oliver E. C. J., Thomsen M., Harvey B. P., Straub S. C., et al. (2019). Marine heatwaves threaten global biodiversity and the provision of ecosystem services. Nat. Clim. Change. 9, 306–312. doi: 10.1038/s41558-019-0412-1
Smith A. B., Godsoe W., Rodriguez-Sanchez F., Wang H. H., Warren D. (2019). Niche estimation above and below the species level. Trends Ecol. Evol. 34, 260–273. doi: 10.1016/j.tree.2018.10.012
Spijkers J., Boonstra W. J. (2017). Environmental change and social conflict: the northeast Atlantic mackerel dispute. Reg. Environ. Change. 17, 1835–1851. doi: 10.1007/s10113-017-1150-4
Sullaway G. H., Shelton A. O., Samhouri J. F. (2021). Synchrony erodes spatial portfolios of an anadromous fish and alters availability for resource users. J. Anim. Ecol. 90, 2692–2703. doi: 10.1111/1365-2656.13575
Sun B., Zhao L., Shao F., Lu Z., Tian J., Liu C. (2022). Estimating the impacts of climate change on the habitat suitability of common minke whales integrating local adaptation. Front. Mar. Sci. 9. doi: 10.3389/fmars.2022.923205
Tamario C., Sunde J., Petersson E., Tibblin P., Forsman A. (2019). Ecological and evolutionary consequences of environmental change and management actions for migrating fish. Front. Eco. Evol. 7. doi: 10.3389/fevo.2019.00271
Thomas Y., Pouvreau S., Alunno-Bruscia M., Barillé L., Gohin F., Bryère P., et al. (2016). Global change and climate-driven invasion of the Pacific oyster (Crassostrea gigas) along European coasts: a bioenergetics modelling approach. J. Biogeogr. 43, 568–579. doi: 10.1111/jbi.12665
Thuiller W., Lafourcade B., Engler R., Araújo M. B. J. E. (2009). BIOMOD–a platform for ensemble forecasting of species distributions. Ecography. 32, 369–373. doi: 10.1111/j.1600-0587.2008.05742.x
Tylianakis J. M., Didham R. K., Bascompte J., Wardle D. A. (2008). Global change and species interactions in terrestrial ecosystems. Ecol. Let. 11, 1351–1363. doi: 10.1111/j.1461-0248.2008.01250.x
Wang L. L., Zhang Z. X., Lin L. S., Peng X., Lin L., Kang B. (2021). Redistribution of the lizardfish in coastal waters of China due to climate change. Hydrobiologia. 848, 4919–4932. doi: 10.1007/s10750-021-04682-y
Weatherdon L. V., Magnan A. K., Rogers A. D., Sumaila U. R., Cheung W. W. L. (2016). Observed and projected impacts of climate change on marine fisheries, aquaculture, coastal tourism, and human health: an update. Front. Mar. Sci. 3. doi: 10.3389/fmars.2016.00048
Weinert M., Mathis M., Kroncke I., Pohlmann T., Reiss H. (2021). Climate change effects on marine protected areas: Projected decline of benthic species in the North Sea. Mar. Environ. Res. 163, 105230. doi: 10.1016/j.marenvres.2020.105230
Xiong P., Cai Y., Jiang P., Xu Y., Sun M., Fan J., et al. (2024). Impact of climate change on the distribution of Trachurus japonicus in the Northern South China Sea. Ecol. Indic. 160, 111758. doi: 10.1016/j.ecolind.2024.111758
Yang W., Hu W. J., Chen B., Tan H. J., Su S. K., Ding L. K., et al. (2023a). Impact of climate change on potential habitat distribution of Sciaenidae in the coastal waters of China. Acta Oceanol. Sin. 42, 59–71. doi: 10.1007/s13131-022-2053-x
Yang W., Sun S., Wang N., Fan P., You C., Wang R., et al. (2023b). Dynamics of the distribution of invasive alien plants (Asteraceae) in China under climate change. Sci. Total Environ. 903, 166260. doi: 10.1016/j.scitotenv.2023.166260
Zeng Z. Y., Cheung W. W. L., Li S. Y., Hu J. T., Wang Y. (2019). Effects of climate change and fishing on the Pearl River Estuary ecosystem and fisheries. Rev. Fish Biol. Fisher. 29, 861–875. doi: 10.1007/s11160-019-09574-y
Keywords: climate change, economic species, Stacked Species Distribution Models, habitat shift, Northwest Pacific Ocean
Citation: Dong W, Bai X, Zhao L, Dong H and Liu C (2024) Comparative analysis of climate-induced habitat shift of economically significant species with diverse ecological preferences in the Northwest Pacific. Front. Mar. Sci. 11:1476097. doi: 10.3389/fmars.2024.1476097
Received: 05 August 2024; Accepted: 21 October 2024;
Published: 06 November 2024.
Edited by:
Tomaso Fortibuoni, Istituto Superiore per la Protezione e la Ricerca Ambientale (ISPRA), ItalyReviewed by:
Diego Panzeri, National Institute of Oceanography and Applied Geophysics, ItalyElizabeth Talbot, Plymouth Marie Laboratory, United Kingdom
Copyright © 2024 Dong, Bai, Zhao, Dong and Liu. This is an open-access article distributed under the terms of the Creative Commons Attribution License (CC BY). The use, distribution or reproduction in other forums is permitted, provided the original author(s) and the copyright owner(s) are credited and that the original publication in this journal is cited, in accordance with accepted academic practice. No use, distribution or reproduction is permitted which does not comply with these terms.
*Correspondence: Changdong Liu, Y2hhbmdkb25nQG91Yy5lZHUuY24=