- 1Marine Mammal Institute, Oregon State University, Newport, OR, United States
- 2Department of Fisheries, Wildlife, and Conservation Sciences, Oregon State University, Newport, OR, United States
- 3HDR, Inc., San Diego, CA, United States
- 4Marine Ecology and Telemetry Research, Seabeck, WA, United States
Blue (Balaenoptera musculus) and fin (B. physalus) whales are prominent species in the California Current Ecosystem (CCE) using the area for migration and foraging. Blue whales migrate between tropical wintering grounds and higher latitude foraging grounds in the CCE, whereas fin whales may remain there year-round. We used Argos tracks of 182 blue (mean duration: 78 days) and 98 fin (mean duration: 35 days) whales tagged from 1994-2018 along the U.S. West Coast to examine residence time and occupancy in U.S. Navy training and testing areas. Bayesian hierarchical switching state-space model locations calculated from Argos tracks were used to provide residence time and proportion of tracking duration within each Navy area. We also calculated relative occupancy isopleths from the state space models, for both directed and non-directed movement, to assess spatial use of Navy areas. We then used generalized linear mixed models and beta regression to examine relationships between Navy area use and covariates, including six climate indices. Point Mugu Sea Range (PMSR) was the most heavily used Navy area by both species in terms of whale numbers, followed by the Southern California Range Complex (SOCAL). Residence time was longest in PMSR for blue whales and in the Northwest Training and Testing Study Area (NWTT) for fin whales. Blue whale use in PMSR was greater in summer versus autumn and for whales tagged in Southern versus Central California. Proportion of tracking duration decreased in all areas with increasing values of the Oceanic Niño Index (ONI), and in PMSR, with increasing values of the North Pacific Gyre Oscillation. Fin whale residence time was longer in PMSR during summer than winter or spring and decreased with increasing values of Habitat Compression Index and ONI. Proportion of tracking duration for fin whales was greatest in summer in PMSR and in winter in SOCAL and NWTT. Overlap of directed and non-directed movement was greatest in PMSR for both species. This assessment of blue and fin whale residence time and occupancy in Navy areas improves our understanding of potential threats these animals face, not only from military activities in Navy areas, but throughout the CCE.
Introduction
Blue (Balaenoptera musculus) and fin (B. physalus) whales are two of the most encountered whale species along the west coast of the U.S (Oldach et al., 2022), as their populations continue to recover from whaling. The California Current Ecosystem (CCE), a highly productive marine environment off the U.S. West Coast, serves as a migratory and foraging habitat for both species (Calambokidis et al., 2015; Scales et al., 2017; Palacios et al., 2019; Falcone et al., 2022). The U.S. Navy (Navy) also uses designated waters off this coast for at-sea training and testing activities (Department of the Navy, 2018). This results in spatio-temporal overlap between whales and Navy training and testing areas, although the extent of this overlap has not been explicitly quantified.
Blue whales in the eastern North Pacific (ENP) migrate between tropical wintering/breeding grounds and productive foraging grounds at higher latitudes in the CCE (Mate et al., 1999; Abrahms et al., 2019a, 2019b), occurring in the latter mainly in summer and autumn months. Fin whales are found year-round in the CCE, with a hotspot of habitat suitability in the Southern California Bight (Scales et al., 2017), the latter of which supports a small, year-round resident fin whale population, suggesting the possibility of two subgroups of fin whales in the CCE (Falcone et al., 2022). Abundance calculations for blue whales in the ENP, based on photo identifications of individual whales from 2015 to 2018, resulted in an estimated 1,898 animals (Calambokidis and Barlow, 2020). Using line-transect survey data, Nadeem et al. (2016) calculated fin whale abundance as 9,029 animals in the waters of California, Oregon, and Washington. The ENP blue whale population has shown no sign of increase, or possibly a slight increase, since the early 1990s (Calambokidis and Barlow, 2013; Barlow, 2016; Calambokidis and Barlow, 2020), in contrast to the population of fin whales in the CCE, which increased between 1991 and 2008 and remained stable between 2008 and 2014 (Nadeem et al., 2016). Blue whales are currently listed as Endangered on the IUCN Red List of Threatened Species (Cooke, 2018a), while fin whales are listed as Vulnerable (Cooke, 2018b), and both species are listed as Endangered under the U.S. Endangered Species Act (ESA) of 1973 (NOAA Fisheries, 2024).
Both blue and fin whales face a variety of potential threats while in the waters of the CCE, including ship strikes, entanglement in fishing gear, and exposure to underwater noise from anthropogenic activities (Oldach et al., 2022). Encounter theory models estimate an annual ship strike mortality of 18 blue whales (based on an assumption of a moderate level of ship avoidance) or 40 blue whales (under the assumption of no ship avoidance) along the U.S. West Coast, combining species distribution models of whale density, vessel traffic characteristics (size, speed, and spatial use), and satellite-tagged whale movement patterns (Rockwood et al., 2017). Forty blue whales represent approximately 2.4% of the estimated population size of blue whales in the ENP. Fin whales are the most frequently ship-struck large whale along the U.S. West Coast (Keen et al., 2019) and similar models for this species estimate an annual ship strike mortality of 43 whales (with ship avoidance), or 95 whales (without avoidance), along the U.S. West Coast, with the latter representing approximately 1% of the estimated population size (Rockwood et al., 2017). It is estimated that 1.54 blue whales and 0.64 fin whales are entangled in fishing gear annually, based on the sum of observed annual entanglements and probability assignments from unidentified entangled whales (Carretta et al., 2023).
Underwater noise is also of concern for baleen whales. In the CCE, key sources of underwater noise include vessel noise, "seal bombs" (explosive charges sometimes used by commercial fishers) (Simonis et al., 2020), and the use of military sonars and explosives (Hildebrand, 2009). Depending on the received levels and frequency of these sounds, their effects on marine mammals can range from behavioral avoidance to auditory masking, temporary or permanent hearing loss, bodily injury, and/or mortality (Southall et al., 2019a). Auditory masking occurs when noise interferes with an animal's ability to communicate with conspecifics, navigate, find prey, and avoid predators (Erbe et al., 2016). Baleen whales are thought to be sensitive primarily to sounds in the low-frequency range (10 to 500 Hz) and are therefore particularly vulnerable to auditory masking from low-frequency sources such as vessel noise (Clark et al., 2009; Southall et al., 2023a) or to behavioral disturbance from low-frequency active sonar or airgun noise (Tyack and Clark, 1998; Miller et al., 2000; Dunlop et al., 2020; Gailey et al., 2022) Baleen whales may also respond to sounds in the mid-frequency range (500 Hz to 25 kHz). Observed behavioral responses of baleen whales to simulated mid-frequency active sonar (MFAS), used by the Navy in military training and testing activities, included termination of deep dives, directed travel away from sound sources, cessation of feeding, and changes in body orientation or swim speed, and were influenced by behavioral state, environmental context, prior exposure to similar sound sources, and received noise levels (Goldbogen et al., 2013; Southall et al., 2019b; 2023b; Pirotta et al., 2022).
The Navy conducts at-sea military readiness activities, including the use of MFAS and high-frequency active sonar (but currently not low-frequency active sonar), in several training and testing areas located off the contiguous U.S. West Coast, including the Southern California Range Complex (SOCAL) portion of the Hawaii-Southern California Training and Testing (HSTT) Study Area; the Point Mugu Sea Range (PMSR); and the Northwest Training and Testing (NWTT) Study Area (Figure 1). The SOCAL Range Complex is located between Dana Point and Baja California, extends over 600 nautical miles (1,111 kilometers) southwest into the Pacific Ocean (Figure 1), and is the principal Naval training venue in the eastern Pacific (Department of the Navy, 2008). PMSR is located north of, and adjacent to, the SOCAL Range Complex and is a designated Major Range and Test Facility Base, home to a variety of weapon system development programs. NWTT is located in both the offshore and inland waters of Washington, as well as in the offshore waters of Oregon and Northern California (Figure 1). Both SOCAL and NWTT are in turn comprised of smaller subareas, including the Southern California Anti-Submarine Warfare Range (SOAR) subarea and the Warning Area-237 (W237), respectively. Military training activities conducted in these areas include the use of MFAS and the detonation of underwater explosives (Department of the Navy, 2018).
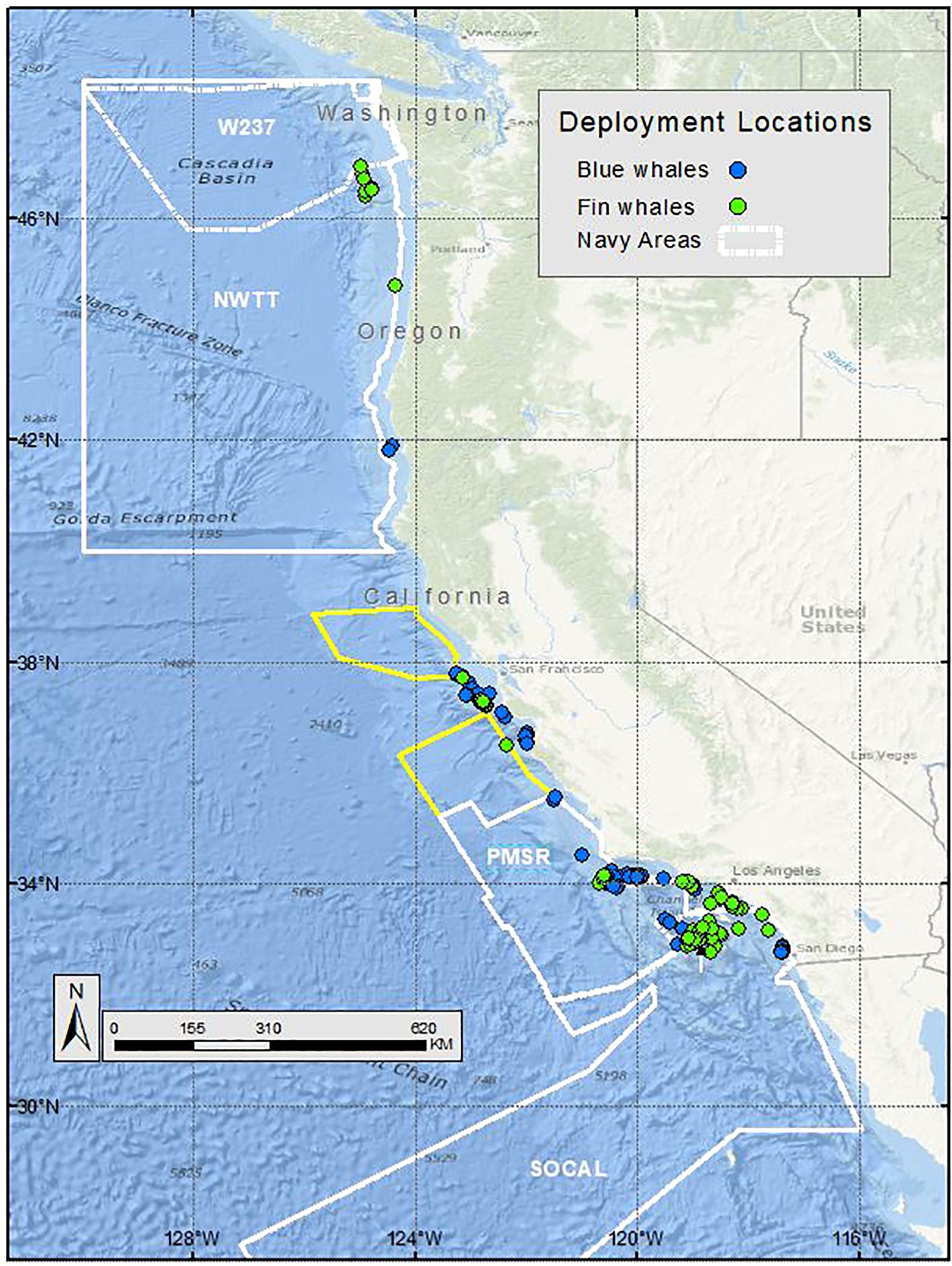
Figure 1. Deployment locations of blue (blue circles) and fin whales (green circles) satellite tagged by Oregon State University and Marine Ecology and Telemetry Research, off the U.S. West Coast, from 1994-2018. Navy training and testing areas used in analyses are shown as white polygons. Navy areas not included in analyses are shown as yellow polygons.
Several legal cases in the U.S. have challenged environmental assessments of Navy activities, federal permitting of these activities, and associated marine species mitigation measures imposed by regulatory agencies (e.g., Zirbel et al., 2011). The extent to which anthropogenic disturbance, including Navy activities, may result in population-level consequences for marine mammals is an obvious concern for endangered species such as blue and fin whales and is a field of ongoing research. For example, attempts to model the population consequences of disturbance consider species occurrence in affected areas, the degree of exposure to disturbance, behavioral and physiological states, vital rates, and the population dynamics of the species involved (Pirotta et al., 2018, 2022). Although military activities represent only a portion of the anthropogenic disturbances to which blue and fin whales in the CCE are routinely exposed, an assessment of how these animals use Navy training areas will contribute to our overall understanding of the regional threats these animals face. This work was funded by the Navy specifically to support monitoring requirements in range-specific Letters of Authorization, issued to the Navy by the National Marine Fisheries Service under the Marine Mammal Protection Act and Endangered Species Act.
Between 1994 and 2018, Oregon State University (OSU) and Marine Ecology and Telemetry Research (MarEcoTel; Seabeck, Washington) tagged blue and fin whales off the U.S. West Coast to improve our understanding of distribution and movements of these species in the CCE. The tracking data from all years and tagging areas combined provided an extensive data set from which to evaluate movement patterns, occurrence, and residence times for blue and fin whales within Navy training and testing areas. Here we examine the relationship between individual residence times and proportion of tracking duration in Navy Areas with season, year, deployment location, and sex, based on a large tagging dataset consisting of tracks from 182 blue whales and 98 fin whales, to further define and explain blue and fin whale occurrence in these areas. We hypothesize that blue whale occurrence will be greater in Navy areas in summer and autumn, due to their migration to southern wintering areas outside U.S. waters, whereas similar seasonal differences may not be seen for non-migratory fin whales. We also explore the potential influence of environmental conditions on blue and fin whale occurrence in Navy areas by including broad-scale ocean climate indices in our analyses. Behavioral state derived from the movement data is also described for the Navy areas to help inform the ways in which blue and fin whales are using these areas.
Materials and methods
Field efforts
Field efforts were conducted by OSU (1994–2018) and MarEcoTel (2008–2017). Blue and fin whales were tagged primarily off southern California (from the California/Mexico border to Point Conception), central California (from Point Conception to Cape Mendocino), and Washington, with tagging locations being determined by local whale distribution (Figure 1). A small number of blue whale tag deployments also took place off northern California (north of Cape Mendocino), in the Gulf of California (Mexico), and in the vicinity of the Costa Rica Dome in the offshore Eastern Tropical Pacific, to provide a final sample size of 183 and 98 blue and fin whale tracks, respectively. All tagging efforts were conducted from rigid-hulled inflatable boats (5.4 to 8.0 m in length). Identification photographs were taken of all tagged whales for comparison with existing photo-identification catalogs for blue whales (maintained by Cascadia Research Collective, Olympia, Washington) and fin whales (maintained by MarEcoTel). Skin and blubber tissue samples were attempted for all tagged whales, when feasible, using a remote biopsy system, for genetic identification of sex and individual. Candidate whales for tagging were selected based on visual observation of their body condition. No whales were tagged that appeared emaciated or that were extensively covered by external parasites.
Satellite tags
Four types of fully implantable, non-recoverable, Argos-based tags were used: Telonics ST15 tags (1998–2008) and Wildlife Computers Smart Positioning or Temperature Transmitting Tags (SPOT), versions 5 and 6 (SPOT5 and SPOT6 tags; 2014–2017), hereafter referred to as Location-Only or LO tags, and Telonics ST21 (2004) and RDW-665 (2016–2017), hereafter referred to as Dive-Monitoring or DM tags. All four tag types followed the same "consolidated" design (Andrews et al., 2019), which was composed of a main body, a penetrating tip, and an anchoring system. Complete details of implantable tag construction and design configuration are provided in Mate et al. (2007, 2015, 2018).
Four types of surface-mounted, non-recoverable, Argos-based tags were also used: Telonics ST6 tags (1993–1995), Telonics ST10 tags (1994–1995), and Wildlife Computers SPOT5 and SPLASH10-A tags (by MarEcoTel, 2008–2017). Each of these tag types followed the "anchored" design described by Andrews et al. (2019) and was externally mounted with two bladed attachment posts. Further details of anchored tag construction and configuration are provided in Mate et al. (1998); Schorr et al. (2014), and Keen et al. (2019).
The final type of tag used in this study was a partially implantable, recoverable, archival tag consisting of a Wildlife Computers MK-10 time-depth recorder platform (2014 and 2015). This tag provided depth, temperature, and tri-axial accelerometer and magnetometer data, as well as Fastloc® GPS and Argos locations. Complete details of the recoverable tag configuration are provided in Mate et al. (2017).
Satellite tags were deployed using a modified 68-kg Barnett compound crossbow or one of two air-powered applicator systems, the Air Rocket Transmitter System (Heide-Jørgensen et al., 2001) and the Dan-inject pneumatic projector (Scales et al., 2017), following the methods described in Mate et al. (2007). Tags were deployed from distances of 1 to 20 m.
LO tags were programmed with one of three duty cycles: 1) transmitting every day, 2) transmitting every other day, or 3) transmitting every day for the first 90 d, then transmitting every other day for the remainder of the tag life. On scheduled transmission days, the tags were programmed to transmit every 10 s (when "dry"; i.e., at the surface) during four 1-h periods, with the transmission periods scheduled to coincide with the most likely times a satellite was overhead. DM tags were programmed to transmit every 10 s (when dry) during six 1-h periods every day for approximately one month, after which they transmitted for six 1-h periods every other day to prolong battery life. As with LO tags, the transmission periods were chosen to coincide with times when satellites were most likely to be overhead.
SPOT5 tags deployed by MarEcoTel were programmed to transmit 24 h/d, daily for 50 d and then switch to every other day for 20 d, followed by every third day for 30 d, every fifth day for 50 d, and then every 10th day thereafter. The majority of SPLASH10-A tags (n = 10) transmitted daily before they stopped, while the remainder (n = 8) switched to every other day duty cycle after periods ranging from 20 to 28 d.
Tag transmissions were processed by Service Argos to calculate locations during polar-orbiting satellite passes (Collecte Localisation Satellites, 2015). Locations were calculated from the Doppler shift of the transmissions when three or more messages reached a satellite during a single pass overhead. Service Argos assigns a quality to each location, depending, among other things, on the number and temporal distribution of transmissions received for each satellite pass (of varying duration from horizon to horizon, Collecte Localisation Satellites, 2015). The accuracy associated with each Argos satellite location was reported as one of six possible location classes (LCs) ranging from less than 200 m (LC = 3) to greater than 5 km (LC = B) (Vincent et al., 2002).
Tracking analyses
Track regularization and behavioral annotation with state-space models
To produce tracks with locations spaced at regular intervals and associated behavioral annotation, the raw Argos locations of all tracks >3 d with >10 locations were used largely unedited (except for the removal of Z-class locations, locations >20 km on land, and extreme outliers with speeds >100 km/h) as input into a Bayesian hierarchical switching state-space model (hSSSM) (Jonsen, 2016) in the software package R v. 3.4.4 (R Core Team, 2018) using the bsam and rjags add-on packages (which interfaced with the software package JAGS v. 4.3 to run Markov chain Monte Carlo simulations using the Gibbs sampler). Prior to running hSSSM, track segments with no Argos locations for >10 d ("gaps") were removed. Most gaps occurred at the beginnings or ends of tracks. In cases where gaps occurred in the middle of a track, the track was split into two segments (on either side of the gap) and run separately through hSSSM. The model output provided a regularized track with three estimated locations per day after accounting for Argos satellite location errors (based on Irvine et al., 2020) and the movement dynamics of the animals (Figures 2, 3). The hSSSM model ran two Markov chain Monte Carlo simulations each for 350,000 iterations, with the first 50,000 iterations being discarded as burn-in and the remaining iterations being thinned by retaining every 31st sample to reduce autocorrelation, yielding a final 9,677 posterior samples to be used with location estimates calculated as the mean of the posterior distribution (Jonsen, 2016). Included in the model was the classification of locations into two behavioral modes based on mean turning angles and autocorrelation in speed and direction: "transiting" (mode 1; high autocorrelation and low turning angle) and "area-restricted searching" (ARS; mode 2; low autocorrelation and high turning angle). Here we refer to mode 1 behavior as "directed" movement and mode 2 behavior as "non-directed" movement. Although only two behavioral modes were modeled, the means of the Markov chain Monte Carlo samples provided a continuous behavioral state value from 1 to 2 (Jonsen, 2016). As in Bailey et al. (2009) and Irvine et al. (2014), we chose locations with behavioral state values greater than 1.75 to represent non-directed movement and values lower than 1.25 to represent directed movement. Locations with behavioral state values in between were considered "uncertain."
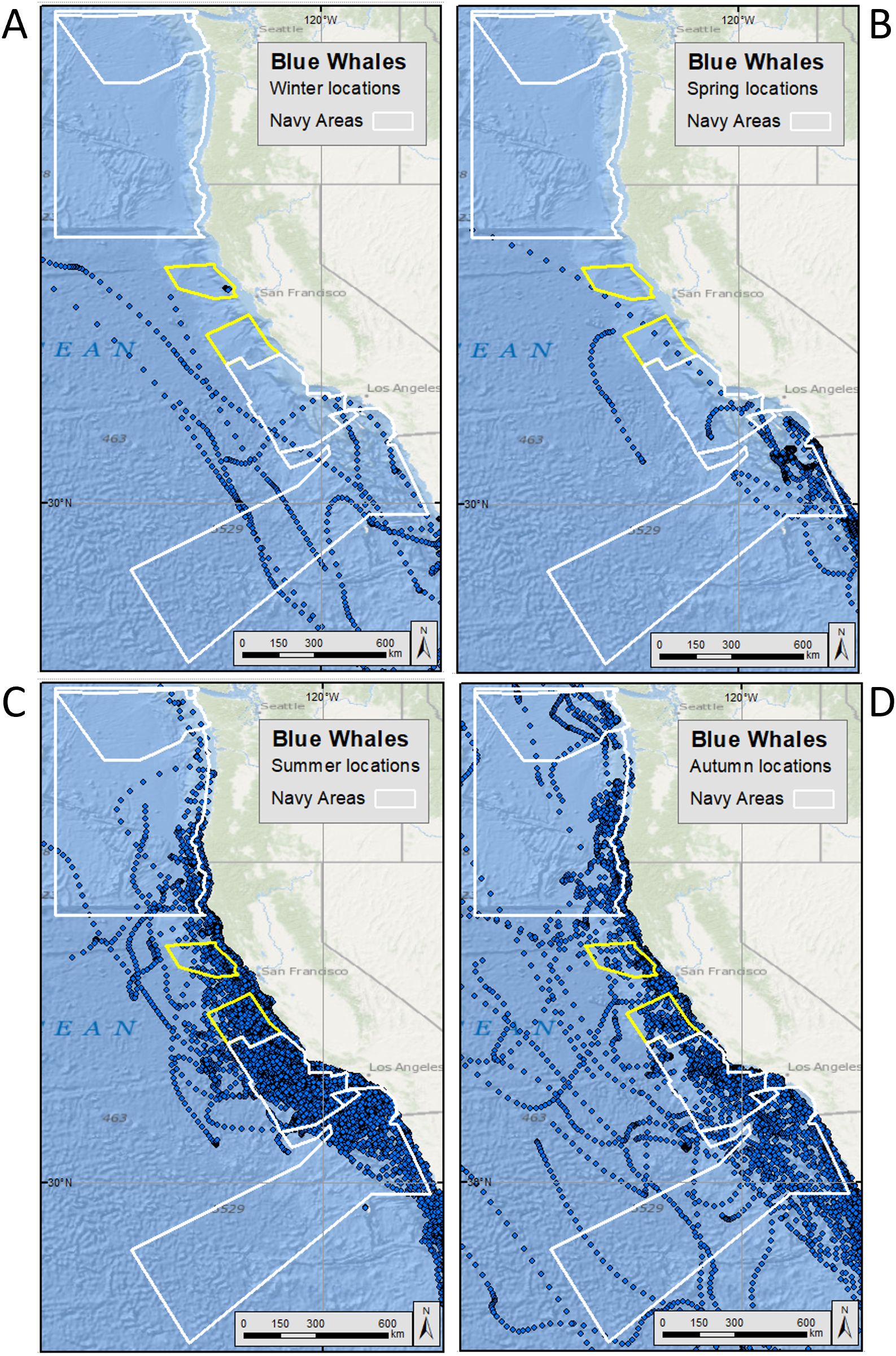
Figure 2. Hierarchical switching state space modeled (hSSSM) locations for blue whales [in (A) winter, (B) spring, (C) summer, and (D) autumn] satellite tagged by Oregon State University off the U.S. West Coast from 1994-2017. Navy training and testing areas used in analyses are shown as white polygons. Navy areas not included in analyses are shown as yellow polygons. For maps showing all blue whale hSSSM locations (beyond the boundaries of the maps shown here), see Supplementary Figure S7.
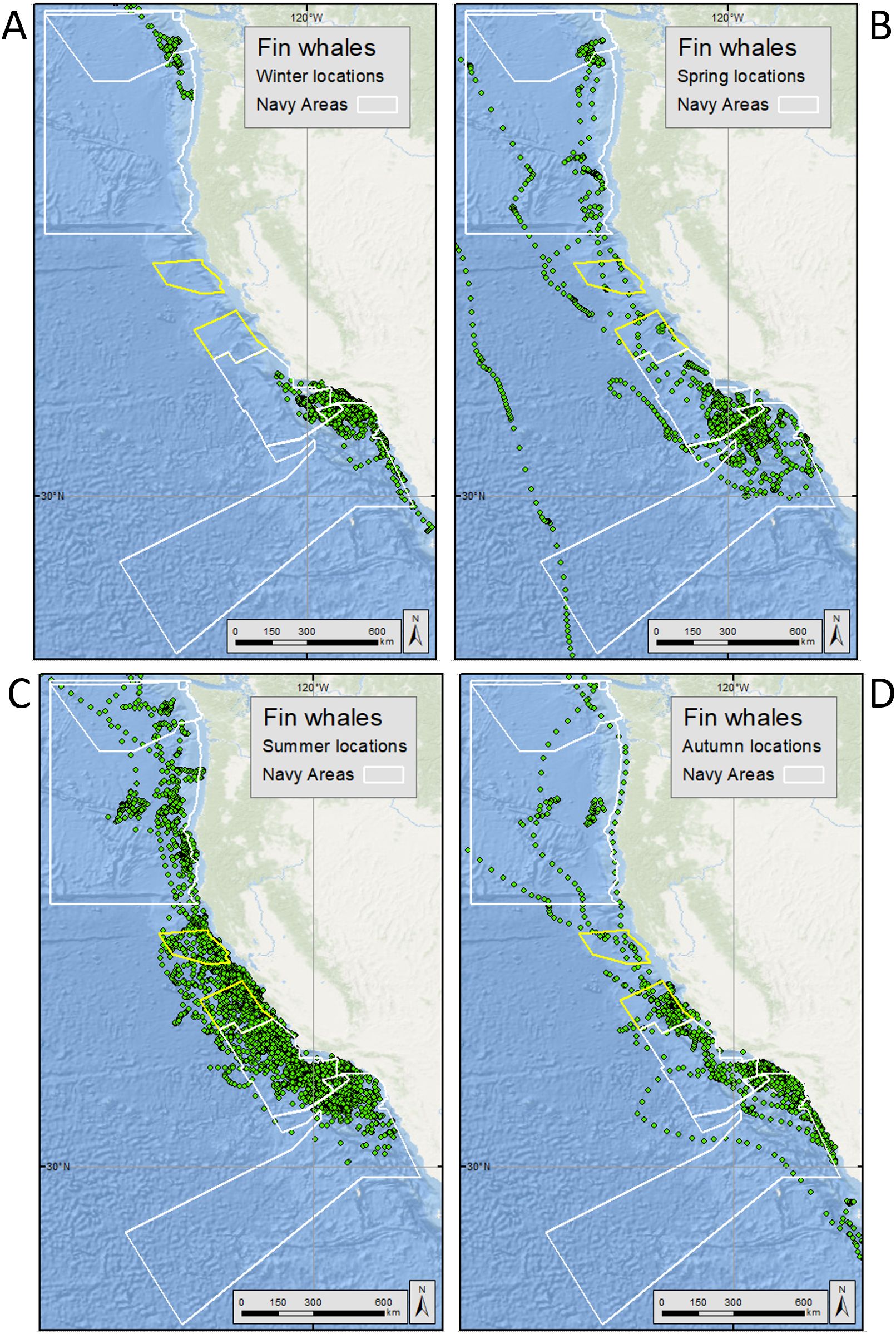
Figure 3. Hierarchical switching state space modeled (hSSSM) locations for fin whales [in (A) winter, (B) spring, (C) summer, and (D) autumn] satellite tagged by Oregon State University and Marine Ecology and Telemetry Research off the U.S. West Coast from 2004-2018. Navy training and testing areas used in analyses are shown as white polygons. Navy areas not included in analyses are shown as yellow polygons. For maps showing all fin whale hSSSM locations (beyond the boundaries of the maps shown here), see Supplementary Figure S8.
Diagnostic plots were visually assessed to ensure adequate mixing of hSSSM model chains and convergence to similar values. Convergence was further assessed using the Potential Scale Reduction Factor (also known as Rhat) (Gelman and Rubin, 1992), which compares the within-chain variance to the between-chain variance. If chains have converged, the value of this factor should be close to 1, so values of the 0.975 quantile <1.1 were taken as evidence of convergence.
hSSSM locations computed over gaps in Argos locations between 2 and 9 d were flagged and removed from the final hSSSM tracks prior to further analyses. A land polygon with a 3-km distance buffer was then used to identify hSSSM locations that occurred on land (366 of 44,416 total hSSSM locations). These locations were repositioned into the closest water based on the shortest path through a visibility graph network of the land polygon using the package pathroutr (London, 2021) in R.
Occurrence in Navy areas
The number of hSSSM locations occurring inside versus outside Navy areas was computed for each whale track, with the percentage of locations inside reported as a proportion of the total number of hSSSM locations obtained for each whale. The Navy areas considered were: (1) SOCAL, (2) PMSR, (3) NWTT, and (4) W237 within the NWTT. W237 is located within NWTT, so whale occurrence in W237 is also counted as occurrence in NWTT as the two areas were analyzed separately. Navy area SOAR, located within SOCAL, was not considered in analyses due to its small size compared to other areas (<3% of the size of the next smallest area, W237).
To compute estimates of residence time inside Navy areas, interpolated locations were derived at 10-min intervals between hSSSM locations, assuming a linear track and a constant speed. These interpolated locations provided evenly spaced time segments from which accurate estimates of residence times could be generated. Residence time was calculated as the sum of all 10-min segments from the interpolated tracks that were completely within each Navy area. The amount of time spent inside these areas was expressed as the number of days as well as the proportion (percentage) of the total track duration. The number of hSSSM locations inside these areas was also reported, as was the proportion (percentage) of the total number of hSSSM locations per track. Residence time and proportion of tracking duration were further classified within each Navy area to season (winter = January through March; spring = April through June; summer = July through September; and autumn = October through December), deployment year, and deployment region. For blue whales, deployment region consisted of four areas: 1) Northern California (NCA); from Cape Mendocino to the California/Oregon border; 2) Central California (CCA); from Point Conception to Cape Mendocino; 3) Southern California (SCA); from the Mexico/California border to Point Conception; and 4) the Gulf of California (GCMX). For fin whales, deployment region consisted of three areas: 1) Southern Washington (SWA; from Grays Harbor to the Oregon/Washington border), 2) CCA, and 3) SCA.
Relative occupancy
Posterior samples from the hSSSMs were used to generate spatially explicit relative occupancy grids for each field effort (typically one effort per year), using a method adapted from Pedersen et al. (2011) and Braun et al. (2018). The relative occupancy grid incorporates temporal variability, the number of individuals tracked, and the uncertainty associated with each model-estimated location into a gridded representation of where model-estimated locations most commonly fell. HSSSM model output was divided into one-day time periods per field effort to minimize the effect of variable tracking durations and tag deployment dates. Posterior samples (9,677; see Track Regularization methodology above) for locations of all tracks occurring within each daily time period were mapped onto a 0.25-degree hexagonal grid and relativized by the grid cell with the largest value to create a daily occupancy grid. Posterior samples falling on land were removed. Daily grids were then weighted by their respective number of posterior samples and summed across an entire field effort to form a single grid, which was again relativized by the cell with the largest value to produce the relative occupancy values on a scale from 0 to 1. Finally, these values were grouped into discrete intervals (isopleths), representing the smallest area encompassing a specified proportion of the relative occupancy values (e.g., 100%, 90%, 50%; Figure 4). We then calculated the proportion of each Navy area overlapped by the relative occupancy isopleths. A 0.25-degree grid was chosen for this analysis based on the median distance between hSSSM location pairs (8.6 km), thus ensuring that at least three locations occurred per grid cell. We also used this method to create relative occupancy grids and associated isopleths using only non-directed and only directed posterior locations (Figure 5).
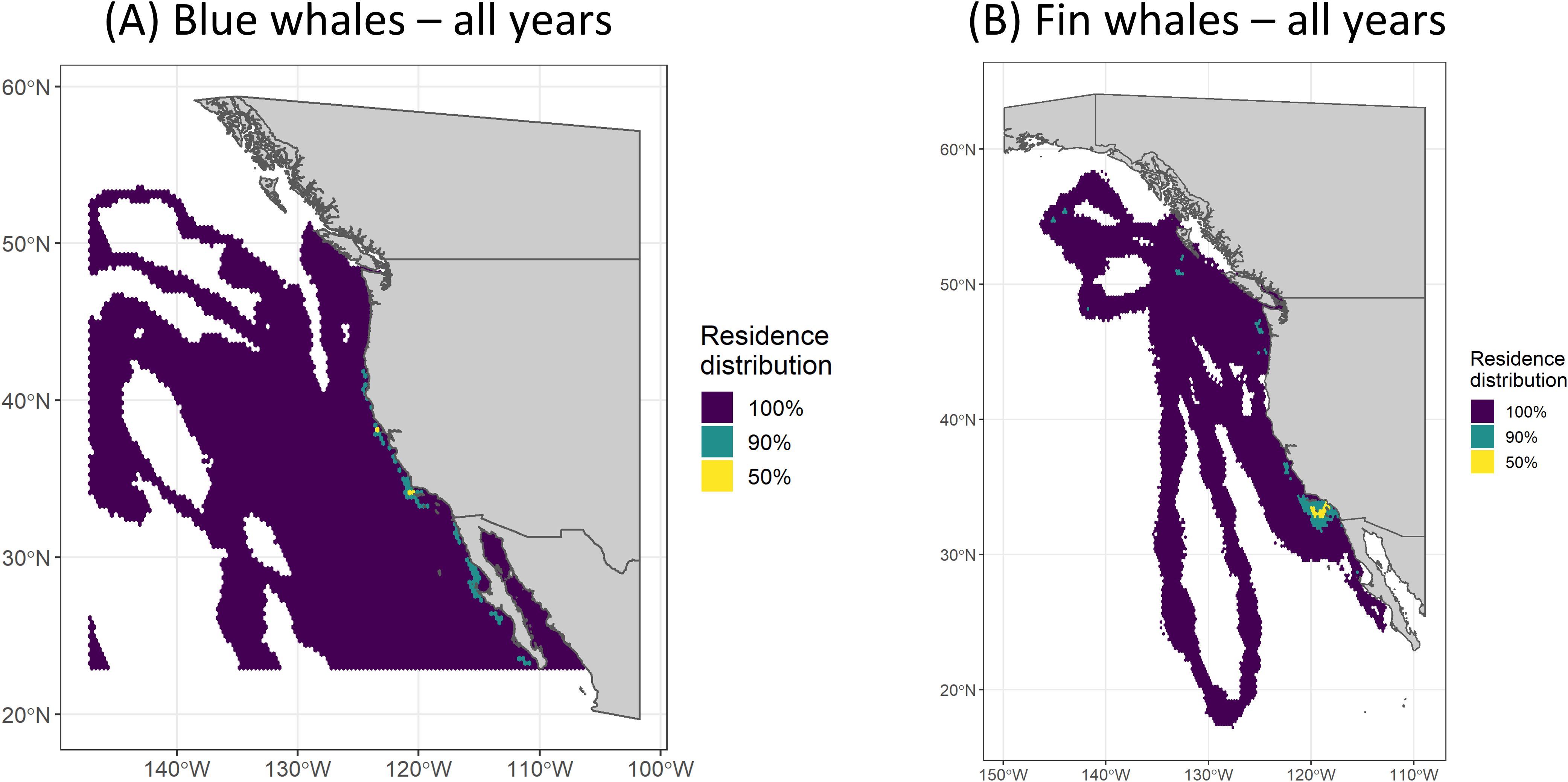
Figure 4. Residence distributions (all behavior modes) for (A) blue and (B) fin whales for all years combined, showing the three calculated isopleths (100%, 90%, and 50%).
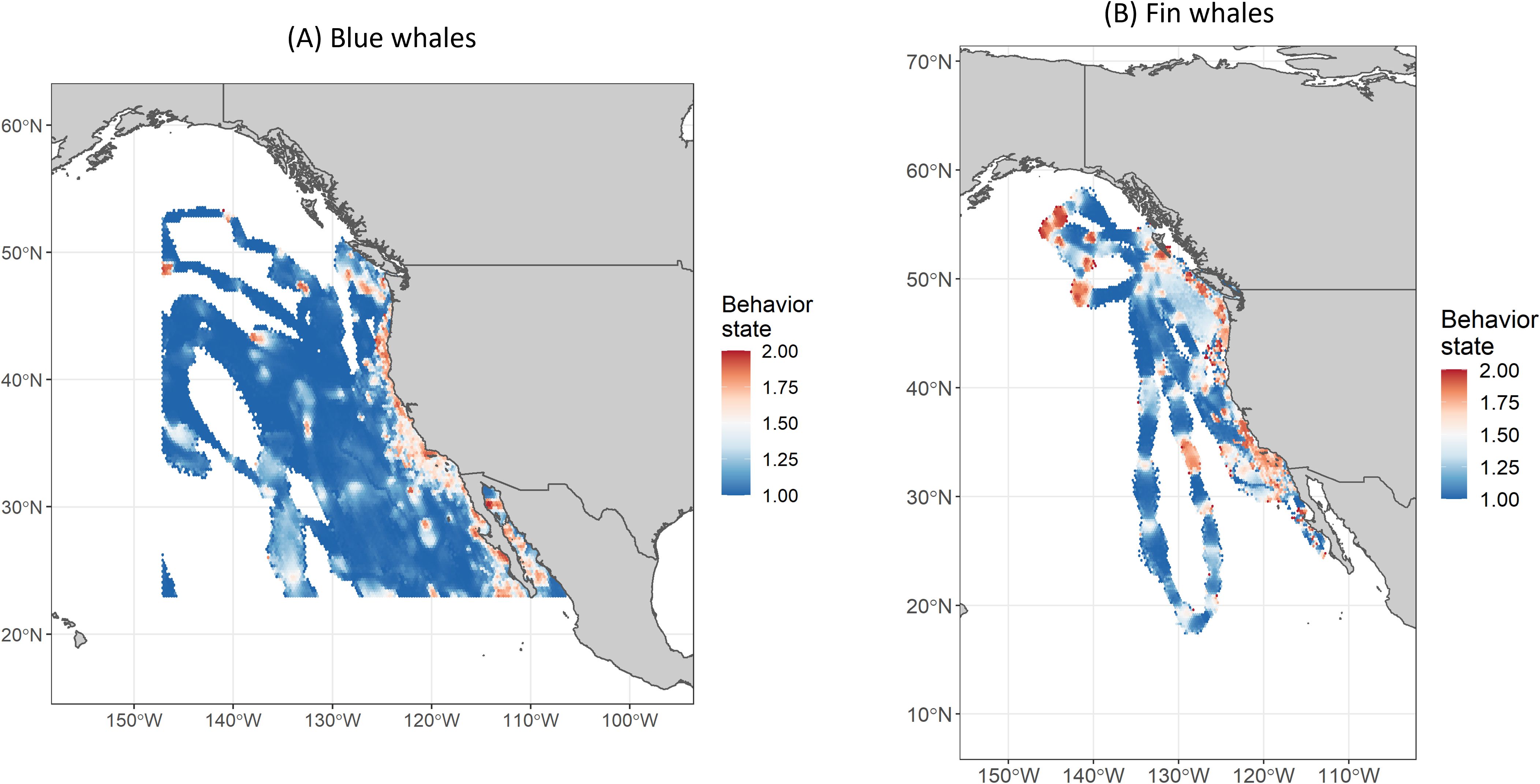
Figure 5. Residence distributions, by behavior mode, for (A) blue and (B) fin whales for all years combined. Behavior modes <1.25 are classified as "directed" movement and those >1.75 are classified as "non-directed" movement in analyses.
Genetic sex determination
Sex was identified from the skin biopsy samples by multiplex PCR using primers P1-5EZ and P2-3EZ to amplify a 443 to 445 bp region on the X chromosome (Aasen and Medrano, 1990) and primers Y53-3C and Y53-3D to amplify a 224 bp region on the Y chromosome (Gilson et al., 1998).
Climate indices
To explore the potential influence of environmental conditions on blue and fin whale occurrence in Navy areas, we used six different climate indices in this study. These indices describe distinct modes of climate-driven environmental variability and ecosystem-level effects in the North Pacific Ocean, spatially at regional to basin scales and temporally at interannual to decadal scales. The indices considered were: the Coastal Upwelling and Transport Index anomaly (CUTIa), the Habitat Compression Index (HCI), the Oceanic Niño Index (ONI), the North Pacific Gyre Oscillation (NPGO), the Pacific Decadal Oscillation (PDO), and the North Pacific High winter value (NPH). Climate indices were downloaded from their respective internet sources and trimmed to the common time period of this study (1994–2018). These indices are described in more detail below and are shown as time series plots in Supplementary Figures S1–S6 (Supplementary Figures and Tables hereafter referred to as "Sx").
Monthly CUTI values were available for the period 1988–2023 and were downloaded from https://oceanview.pfeg.noaa.gov/erddap/tabledap/cciea_OC_CUTI.html. CUTI provides estimates of the rate of vertical water volume transport (Ekman transport) at selected locations along the U.S. West Coast (33°N, 39°N, and 45°N) in response to upwelling- or downwelling-favorable winds, and it also accounts for cross-shore geostrophic flow associated with an alongshore sea surface height gradient (Jacox et al., 2018). As CUTI had a strong seasonal cycle, we implemented a seasonal decomposition on the monthly CUTI time series in R using the package seasonal (Sax and Eddelbuettel, 2018) to isolate the trend, seasonal, and irregular components and retained the irregular component (i.e., the anomaly; termed CUTIa here) as the index of interest in this study. For a given month, the CUTIa provides a measure of how much higher or lower the volume of upwelled water was compared to the long-term monthly average at that latitude.
Monthly HCI values were available for the period 1980–2023 and were downloaded from https://oceanview.pfeg.noaa.gov/erddap/tabledap/cciea_OC_HCI.html. Based on temperature output from a data-assimilative numerical model at 2-m depth, HCI measures the spatial footprint of cool upwelled coastal water that is regularly demarcated by the differential boundary of warmer oceanic water offshore over the domain of 35.5–40°N and extending out to 150 km from the coast (Schroeder et al., 2022). HCI values are provided for four contiguous biogeographical regions off the U.S. West Coast (30–35.5°N, 35.5–40°N, 40–43.5°N, and 43.5–48°N), with the minimum value (0) meaning that there is almost no cool-water habitat (it is compressed near shore) and the maximum value (1) meaning that cool-water habitat extends over the entire region (Schroeder et al., 2022).
ONI values were available for the period 1950-2023 and were downloaded from http://origin.cpc.ncep.noaa.gov/products/analysis_monitoring/ensostuff/ONI_v5.php. ONI represents the rolling three-month average temperature anomaly in the surface waters of the east-central tropical Pacific (Nino 3.4 region, 5°N-5° S, 120–170° W). Values of +0.5°C or higher indicate El Niño conditions and values of -0.5°C or lower indicate La Niña conditions when these thresholds are met for a minimum of five consecutive overlapping seasons (Trenberth and Stepaniak, 2002).
Monthly PDO values were available for the period 1854–2023 and were downloaded from https://www.ncei.noaa.gov/access/monitoring/pdo/. The PDO index is defined as the leading principal component of North Pacific monthly sea surface temperature anomalies, poleward of 20°N (Mantua et al., 1997). When sea surface temperatures are anomalously cool in the interior North Pacific and warm along the North American coast, and when sea level pressures are below average over the North Pacific, the PDO has a positive value. Conversely, a negative PDO phase is the result of warm sea surface temperature anomalies in the interior and cool anomalies along the North American coast, or above average sea level pressures over the North Pacific (Mantua et al., 1997).
Monthly NPGO values were available for the period 1950–2023 and were downloaded from http://www.o3d.org/npgo/. The NPGO is a climate pattern that emerges as the second dominant mode from an empirical orthogonal function decomposition of sea surface height variability in the Northeast Pacific (Di Lorenzo et al., 2008). NPGO fluctuations reflect changes in the intensity of the central and eastern branches of the North Pacific Gyre, which are driven by regional and basin-scale variations in wind-driven upwelling and horizontal advection, with impacts on salinity, nutrient concentrations, chlorophyll-a, and higher trophic levels (Di Lorenzo et al., 2008).
Annual, wintertime (January–February mean) values of the NPH were available for the period 1967–2023 and were downloaded from https://oceanview.pfeg.noaa.gov/erddap/tabledap/cciea_OC_NPH_JF.html. The NPH corresponds to the areal extent of the North Pacific High Pressure System, as indexed by the 1020-Pa isobar, which in turn is predictive of winter upwelling (Schroeder et al., 2013). Winter values of the NPH area can be used as an ecosystem pre-conditioning index, with demonstrated impacts on ecological processes in the CCE, including upper trophic levels (Schroeder et al., 2013).
For purposes of statistical modeling, rolling three-month averages were computed for the monthly climate indices (CUTIa, HCI, PDO, and NPGO) to generate seasonal (quarterly) values (ONI was originally obtained as a rolling three-month average). These seasonal values were matched to the seasonal values of individual residence time and the proportion of tracking duration (see next section). Since relative occupancy was computed on an annual basis, the only climate index suitable for consideration in models involving this response variable was NPH, which was obtained as a yearly value.
Statistical modeling
We implemented statistical models to explore the relationship between tag-derived metrics (individual residence time, proportion of tracking duration, and relative occupancy) and potential explanatory variables describing characteristics of the Navy areas as well as the climate indices mentioned above. Explanatory variables included Navy area, deployment region, deployment year, and season.
P-values resulting from statistical analyses were interpreted on a scale, following Ramsey and Schafer (2012) in which null hypotheses were rejected with: convincing evidence for values <0.001 - 0.01, moderate evidence for values between 0.01-0.05, suggestive, but inconclusive evidence for values between 0.05 - 0.10, and no evidence for values >0.10.
Individual residence time and proportion of tracking duration
To determine the relationship between individual residence time in Navy areas and potential explanatory variables, generalized linear mixed models (GLMM) were run using the package lme4 (Bates et al., 2014) in R, treating each individual whale (denoted as "tag ID") as a random effect. We used beta regression implemented in the R package betareg (Cribari-Neto and Zeileis, 2010) to analyze the proportion of tracking duration in Navy areas rather than GLMMs, as beta regression is better suited to proportion data (i.e., when the data distribution is bounded by 0 and 1; Geissinger et al., 2022). Prior to model selection, response variable distributions were examined and transformed where necessary to achieve normality. A precision effect was applied to Navy area in the beta regression model to account for over-dispersion in the model resulting from unequal variances in different Navy areas.
Models of individual residence time and proportion of tracking duration were weighted according to track duration to mitigate the potential bias that can occur in large-scale tagging studies if tags are deployed closely in time and space. This sampling strategy can artificially inflate the importance of the area surrounding a tagging location compared to distant areas that are visited by only the relatively few animals whose tags last for an extended period. Thus, we included a vector of prior weights to be used in our model-fitting process. For each individual whale, the weight was based on its total track duration, with shorter tracks being weighted more heavily than longer tracks. To derive these track-level weights, we implemented a modified version of the location-level weighting approach presented in Block et al. (2011), which corresponds to the number of individuals that have location estimates for the same relative day of track, with a threshold imposed on the weight such that locations from tags lasting beyond the 85th percentile of the number of tags transmitting on a given day receive the same weight as on the threshold day. For our approach, we combined all years of data and calculated the number of individuals transmitting on each relative day of track along a composite year. Thus, the vector of relative day along the composite year contained all possible track durations in the data and the corresponding weight vector was the total number of individuals for a given track duration up to the 85th percentile of the number of individuals. Weights were calculated separately for each species' dataset, resulting in weights being applied to track durations up to 48 d for blue whales and 49 d for fin whales (Supplementary Tables S1, S2).
Model selection followed a three-step process: first, a base model was evaluated using only the non-climate-related explanatory variables; second, an environmental model considered the additional effects of the relevant climate variability; and third, an expanded model considered interaction terms between Navy area and significant explanatory variables. In each step, explanatory variables explaining significant variation (p-values <0.05) in the response variable were kept, while insignificant variables (p-values >0.05) were removed following a backward stepwise model selection process. Insignificant variables were removed one at a time, beginning with the variable with the highest p-value. Akaike Information Criterion (AIC) values were then calculated at each step of the model selection process, and the model with the lowest AIC value and only significant variables was retained as the best model. Highly correlated significant climate variables (r >0.7) were iteratively removed from the models for comparison of AIC values and final model selection. Interaction terms between Navy area and season/deployment region were examined with subsets of data, when sample sizes for some seasons/deployment regions were too small for meaningful comparisons. For blue whales, interactions between Navy area and season were run with summer and fall only and between Navy area and deployment region with only SCA and CCA. For fin whales, the interaction between Navy area and season was run for only NWTT, PMSR, and SOCAL.
To evaluate the effect of sex on individual residence time, whales of unknown sex were removed from the analysis, and the final model was re-run, including sex as an additional explanatory variable. For blue whales, the diagnostics of the GLMM model including sex indicated that the random effect of individual whales did not improve the model, so we removed it and ran a fixed-effects generalized linear model (GLM) instead. We did not encounter a similar issue with the fin whale GLMM model that included a sex effect.
Final GLMM models were assessed visually by plots of residual versus fitted values, residual values versus leverage values, scale location plots, and Q-Q plots. Final beta regressions models were assessed by plots of standardized weighted residuals versus indices of observations, Cook's distance, generalized leverage versus predicted values, and standardized weighted residuals versus linear predictor values.
Relative occupancy
Beta regressions were also used to examine patterns in the proportion of Navy areas overlapped by yearly relative occupancy. Separate regression models were run for relative occupancy calculated from non-directed locations and those calculated from directed locations to determine whether behavior types differed between Navy areas. Hereafter, when referring to these two relative occupancies separately, we use non-directed movement and directed movement, respectively, without the words "relative occupancy" for simplicity. Only the 90 percent relative occupancy isopleths were considered for this analysis to ensure there was enough meaningful data to make comparisons. Using only the non-directed and directed locations, we focused on a subset of the data (excluding locations of uncertain behavioral mode). Thus, the 50% percent isopleths, while representing areas of highest occupancy (i.e., hotspots), were too small to be of value, and the 100% isopleths lent undue weight to locations in the periphery of the distributions.
Because relative occupancy was created from hSSSM locations for an entire year, the response variable had only one value per year. Thus, comparisons could not be performed between deployment year or deployment region, and Navy area was the only non-climate explanatory variable considered in the models. The only climate index considered was NPH, which, as an annual value, was a better match for the yearly relative occupancy than the seasonal climate indices used in the previous analyses. As with the beta regression for the proportion of tracking duration, a precision effect was applied to Navy area. Final models were assessed in the same way as described above for beta regression of proportion of tracking duration.
Results
Argos satellite tags were deployed on 275 blue whales and 108 fin whales in the ENP between 1994 and 2018. Of these, 102 (27%) transmitted <3 d and/or provided <10 locations, which were removed from further analyses. Overall tracking durations for the remaining tags ranged from 3.2 to 304.2 d for blue whales (n = 183, mean = 78.5 d, SD = 64.78 d) and from 3.0 to 301.0 d for fin whales (n = 98, mean = 35.1 d, SD = 42.49), with the majority of tag deployments for both species taking place in southern California waters (Table 1). Biopsy samples were collected from 91 blue whales (39 genetically sexed as female and 52 as male) and from 32 fin whales (16 genetically sexed as female and 16 as male).
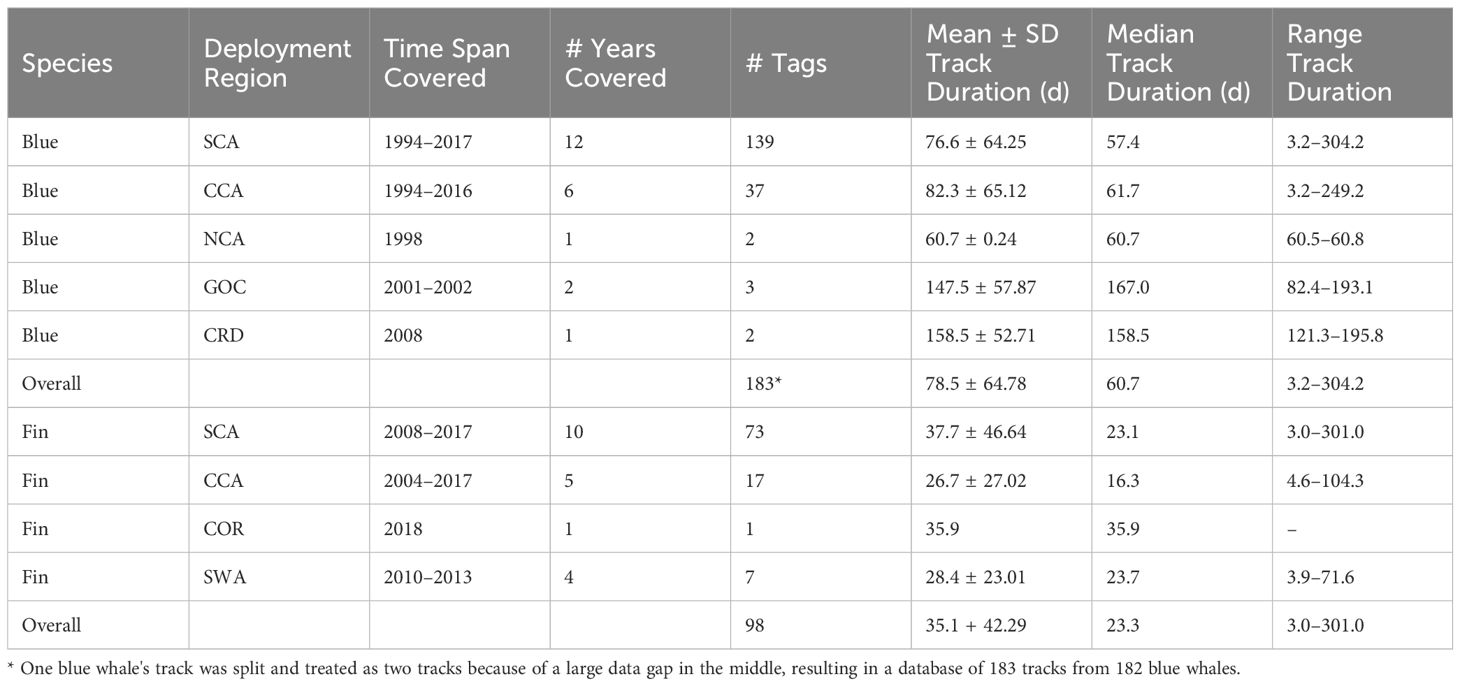
Table 1. Mean (± SD), median, and range of tracking duration (d) for satellite tags deployed on blue and fin whales off the U.S. West Coast between 1994 and 2018 by Oregon State University and Marine Ecology and Telemetry Research.
Blue whales
Individual residence time
Blue whales spent the most time in PMSR (mean = 17.3 d, SD = 16.5 d, range = 0.2–74.8 d, n = 149, Supplementary Table S3; Figure 6), followed by NWTT (mean = 10.2 d, SD = 10.5 d, range = 0.04–39.2 d, n = 26), and the least time in SOCAL (mean = 6.1 d, SD = 5.6 d, range = 0.2–29.6 d, n = 111). PMSR also had the most use in terms of the number of blue whales, with 81% of tagged whales (149 of 182) having locations there, followed by SOCAL (61% of whales), NWTT (14%), and finally W237 (2%).
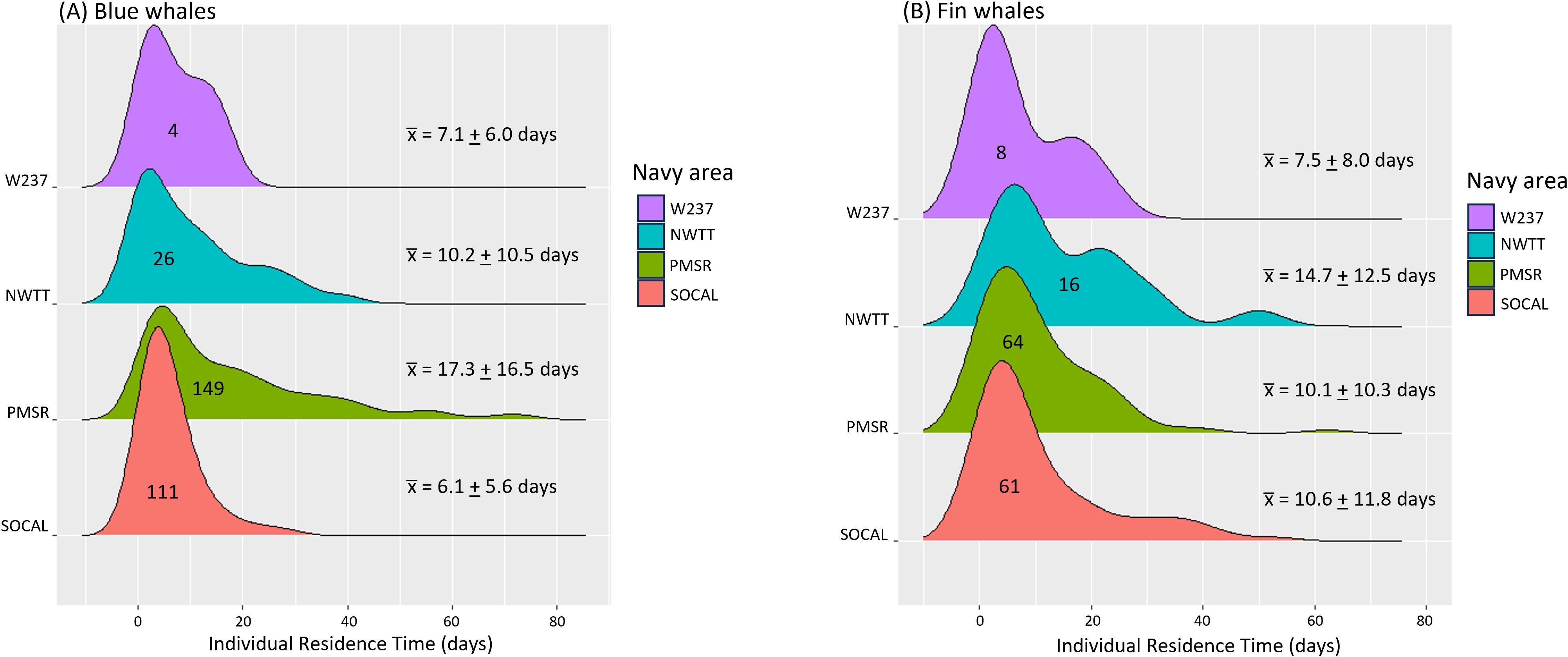
Figure 6. Individual residence time in Navy areas for (A) blue and (B) fin whales. Navy areas are depicted from south (bottom) to north (top) on the y-axis. Numbers inside distributions refer to the number of whales having locations within that Navy area. Mean (+ SD) residence times per Navy area are shown in the numbers on the right.
The final model for the blue whale GLMM (R-squared conditional [R2c] = 0.166; Table 2 and Supplementary Table S4) was:
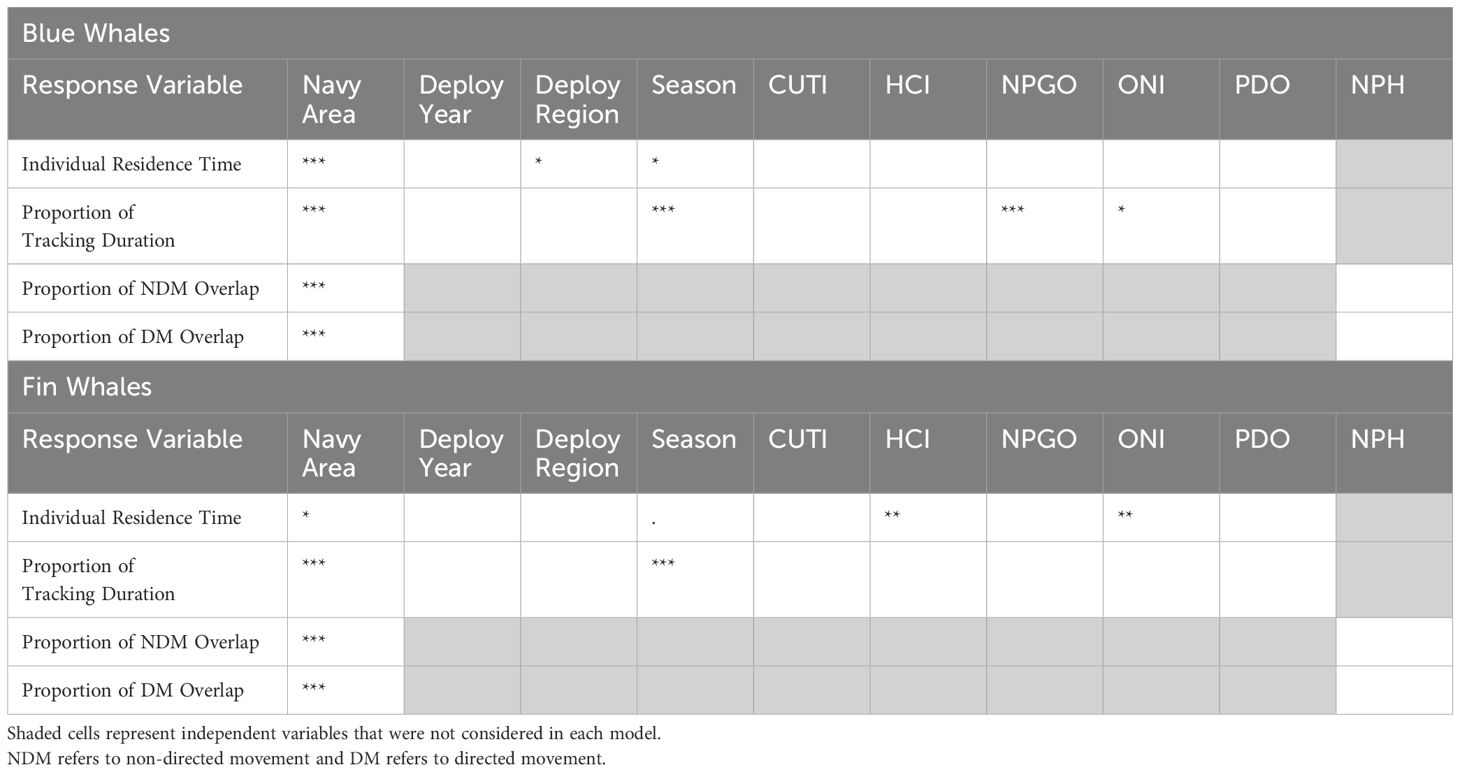
Table 2. Model results for complete datasets for blue and fin whales, showing significant independent variables in the final models, with their level of significance (. = p-values between 0.05-0.06; * = p-values between 0.01-0.05; ** = p-values between 0.001-0.01; *** = p-values < 0.001).
Residence times for blue whales were significantly longer in PMSR than in NWTT (1.9 times longer) or SOCAL (2.2 times longer; p-values <0.003; Supplementary Figure S9). Effect sizes of model covariates are presented here and elsewhere as mean values without error estimates, for the sake of brevity. Blue whales tagged in SCA had significantly longer residence times than whales tagged in NCA (4.0 times longer; p-value = 0.009), with moderate evidence for longer times than whales tagged in CCA (1.4 times longer; p-value = 0.028; Supplementary Figure S9). Residence times were also significantly longer in summer than during autumn (1.4 times longer; p-value = 0.018; Supplementary Figure S9).
There was moderate evidence of a significant interaction between Navy area and deployment region, when only SCA and CCA were considered (p-value = 0.024), such that individual residence time in PMSR was 2.1 times greater for whales tagged in SCA than for those tagged in CCA (p-value < 0.001; Figure 7). Deployment region did not significantly affect residence time in other Navy areas.
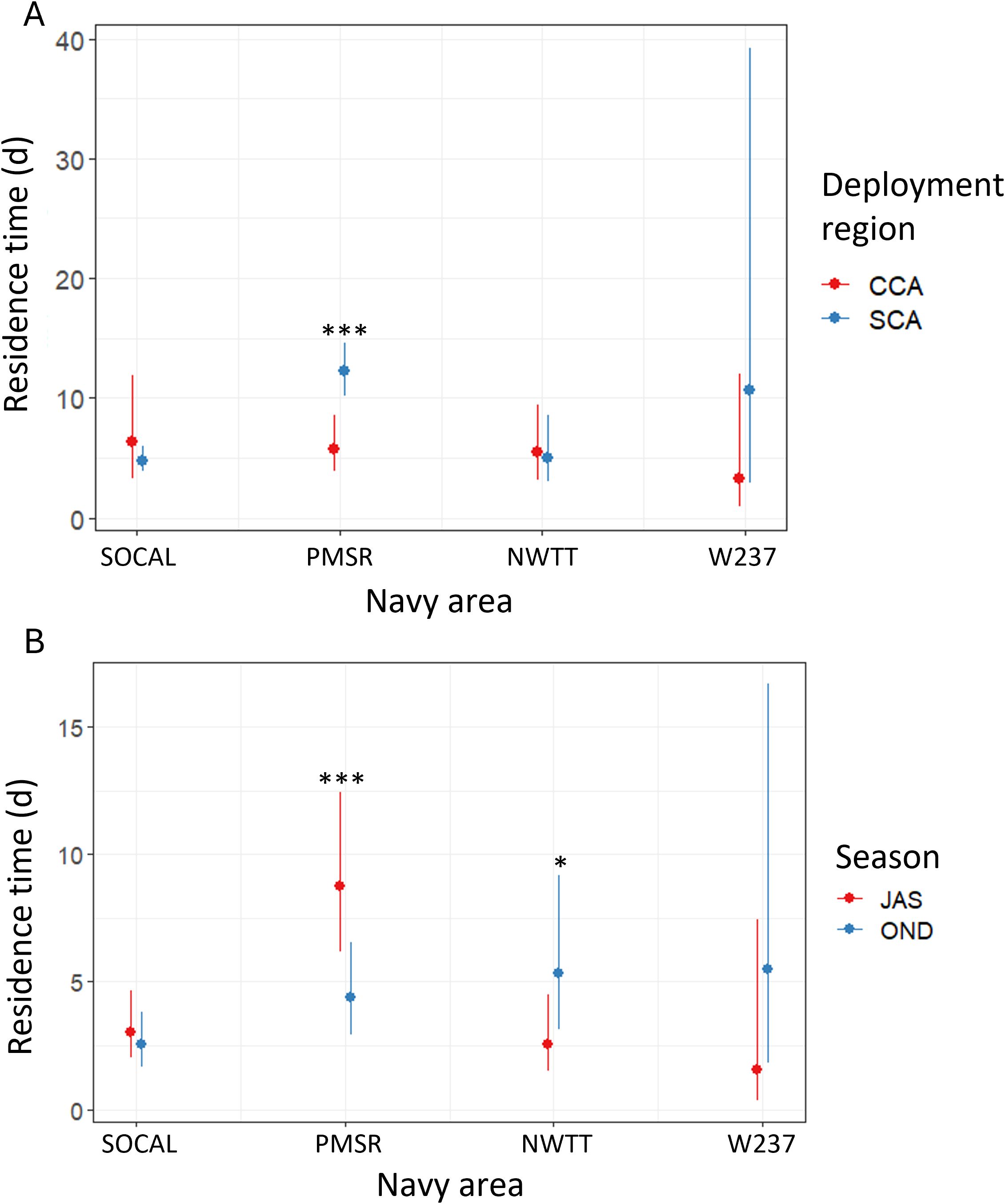
Figure 7. Predicted values of individual residence time for blue whales by Navy area in each of (A) two deployment regions (SCA, Southern California; CCA, Central California), and (B) two seasons (JAS, summer; OND, autumn). Asterisks denote significant differences between regions or seasons, at the following levels: * = p-values between 0.01-0.05; *** = p-values < 0.001.
The interaction of Navy area with season was significant (p-value = 0.001) and showed that in PMSR, residence time was significantly higher in summer than autumn (2.0 times higher; p-value < 0.001; Figure 7). There was suggestive evidence that residence time in NWTT was higher in autumn than in summer (2.1 times higher; p-value = 0.047; Figure 7).
There was suggestive but inconclusive evidence that male blue whales (n = 52) had longer residence times in Navy areas than female blue whales (n = 39; 1.4 times longer; p-value = 0.059).
Proportion of tracking duration
Proportion of tracking durations in Navy areas for blue whales was highest in PMSR (mean = 0.30, SD = 0.29, range = <0.01–1.0) and lowest in W237 (mean = 0.07, SD = 0.06, range = 0.02–0.17), with values for SOCAL (mean = 0.11, SD = 0.16; range = <0.01-0.99) and NWTT (mean = 0.09, SD = 0.08; range = <0.01-0.26) being intermediate and fairly similar to W237 (Supplementary Table S5; Figure 8).
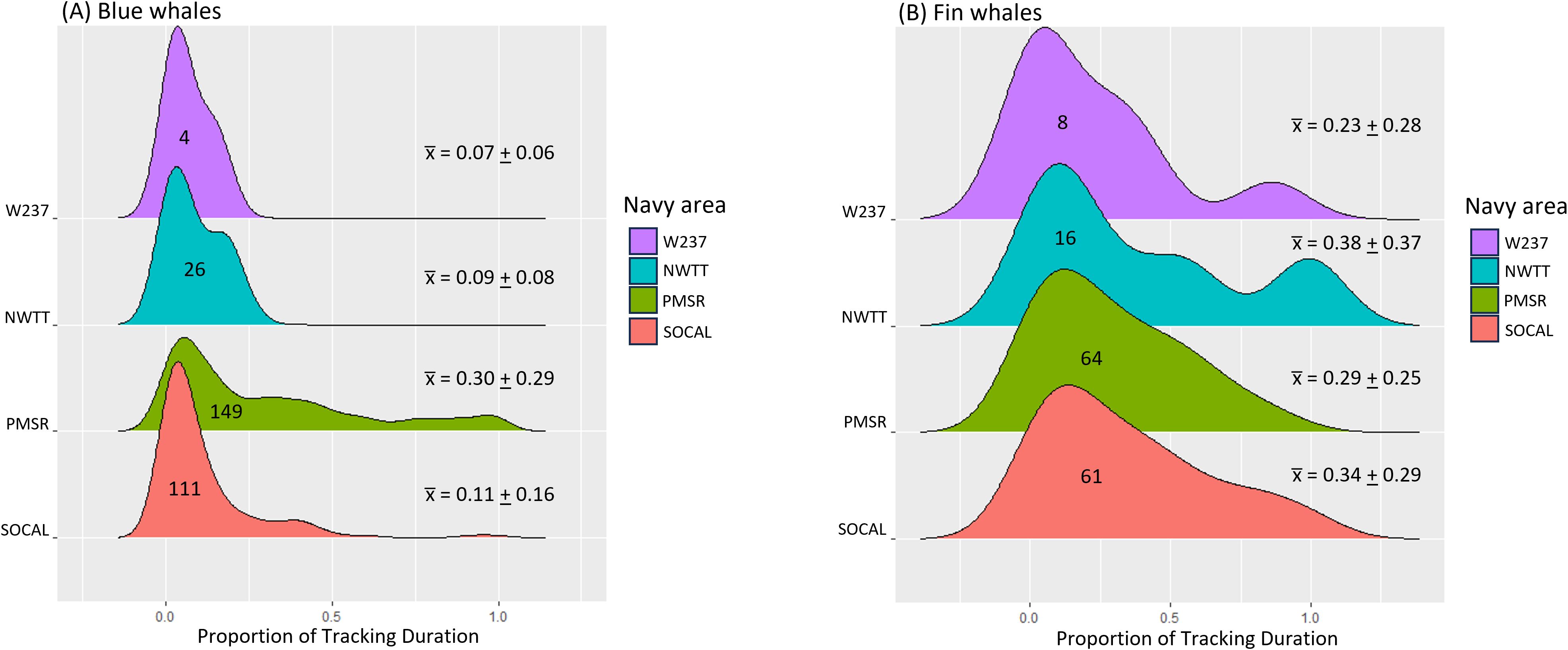
Figure 8. Proportion of tracking duration in Navy areas for (A) blue and (B) fin whales. Navy areas are depicted from south(bottom) to north (top) on the y-axis. Numbers inside distributions refer to the number of whales having locations within that Navy area. Mean (+ SD) proportions per Navy area are shown in the numbers on the right.
The best beta regression model for blue whales (pseudo R-squared [pR2] = 0.287; Table 2 and Supplementary Table S4) was:
The Navy area|Navy area notation indicates that an effect of Navy area was applied to the beta regression precision effect so that each Navy area was associated with a separate variance parameter.
The proportion of tracking duration in Navy areas for blue whales was significantly higher in PMSR than in all other Navy areas (p-values <0.001), with the proportion being 4.9 times higher in PMSR than in W237 in the most extreme case (Supplementary Figure S10). There was moderate evidence that proportion of tracking duration was higher in SOCAL compared to NWTT (1.6 times higher; p-value = 0.03). Whales tagged in SCA had a significantly higher proportion of tracking duration in Navy areas than whales tagged in CCA (1.8 times higher; p-value < 0.001; Supplementary Figure S10). Proportion of tracking duration in Navy areas was also significantly higher during summer than in autumn (1.8 times higher; p-value <0.001) or in spring (2.6 times higher; p-value = 0.007; Supplementary Figure S10). There was a negative relationship between proportion of tracking duration and ONI (p-value = 0.02), such that with each unit increase in ONI, proportion decreased by 14% (Figure 9). The significant interaction between Navy area and NPGO was driven almost entirely by a negative relationship between proportion of tracking duration and NPGO in PMSR (p-value < 0.001). For each unit increase in NPGO there was a 30% decrease in proportion in PMSR (Figure 9). The interaction between Navy area and NPGO was significantly different between PMSR and SOCAL (p-value < 0.001). There was suggestive but inconclusive evidence of a difference in the interaction term between PMSR and W237 (p-value = 0.061).
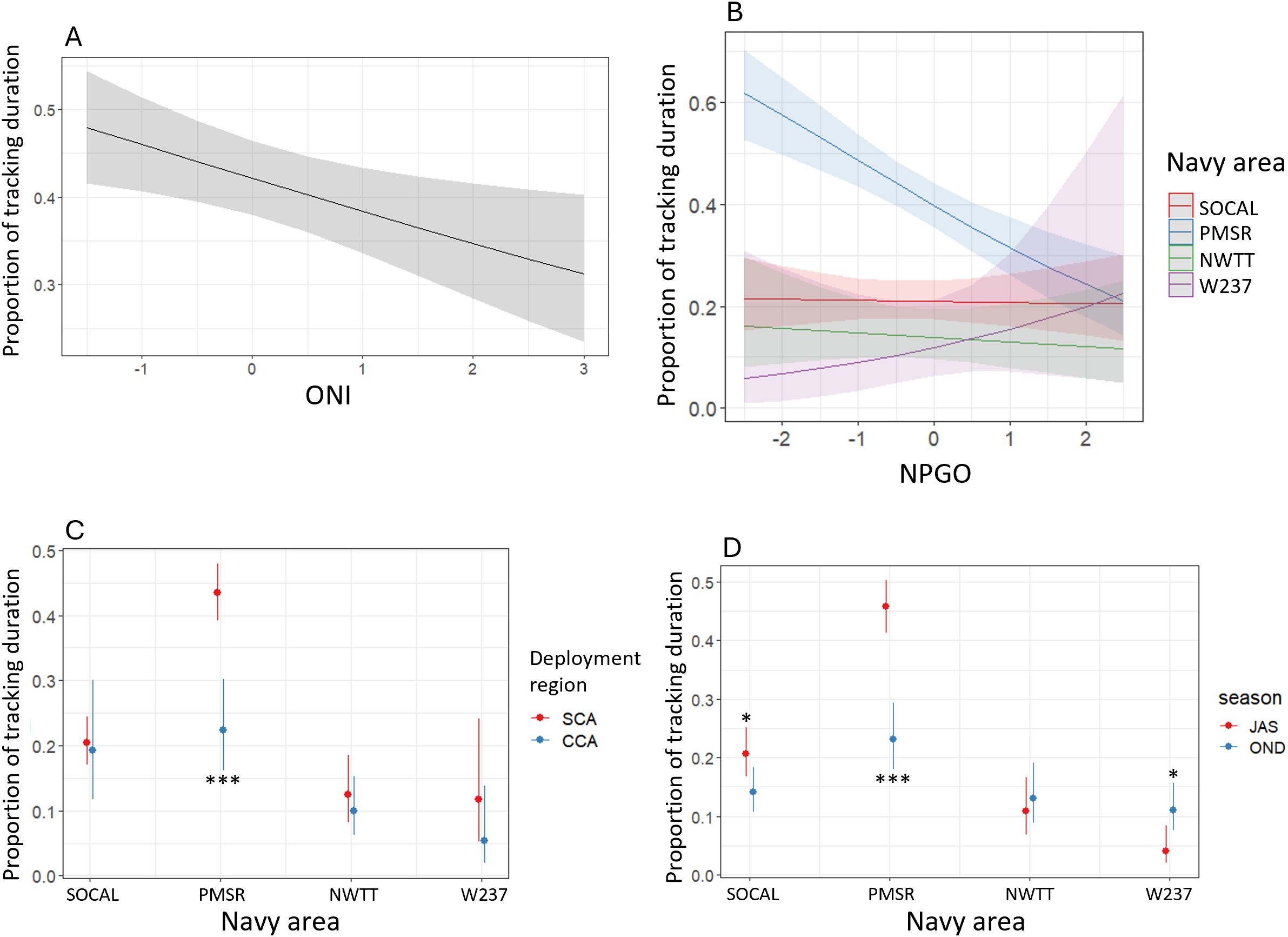
Figure 9. Predicted proportion of tracking duration for blue whales by (A) ONI, (B) NPGO in each Navy area, (C) Navy area in each of two deployment regions (SCA, Southern California; CCA, Central California), and (D) Navy area in each of two seasons (JAS, summer; OND, autumn). Asterisks denote significant differences between regions or seasons, at the following levels: * = p-values between 0.01-0.05; *** = p-values < 0.001.
There was a significant interaction between Navy area and deployment region, when only SCA and CCA were considered, such that in PMSR proportion of tracking duration time was 2.7 times higher for whales tagged in SCA than for those tagged in CCA (p-value < 0.001; Figure 9). Deployment region did not significantly affect proportion of tracking duration in other Navy areas.
The interaction of Navy area with season was also significant and showed that in PMSR proportion of tracking duration was significantly higher in summer than autumn (2.8 times higher; p-value < 0.001 in PMSR) and in SOCAL there was moderate evidence that proportion was also higher in summer than autumn (1.6 times higher; p-value = 0.014; Figure 9). There was also moderate evidence that proportion of tracking duration was higher in autumn than summer in W237 (2.9 times higher; p-value = 0.011; Figure 9).
There was no evidence that proportion of tracking duration in Navy areas differed between male (n = 52) and female blue whales (n = 39).
Relative occupancy – non-directed movement
The proportion of overlap of non-directed movement in Navy areas for blue whales was highest in PMSR (mean = 8%, SD = 5.8%, range = 1.0–19.8%; Supplementary Table S6). Mean proportion of overlap was less than 2% in each of the other Navy areas.
NPH did not have a significant effect on the proportion of Navy areas overlapped by non-directed movement and was removed from the model. W237 also had to be removed from the model as there was very little overlap in that area (with only one year represented), leading to a very skewed Cook's distance plot. The final model for non-directed movement, without the precision term, (pR2 = 0.4364; Table 2 and Supplementary Table S4) was:
According to the final model, the proportion of overlap of non-directed movement with Navy areas was significantly greater for PMSR than for NWTT (4.7 times greater) or SOCAL (5.4 times greater; p-values <0.001; Figure 10).
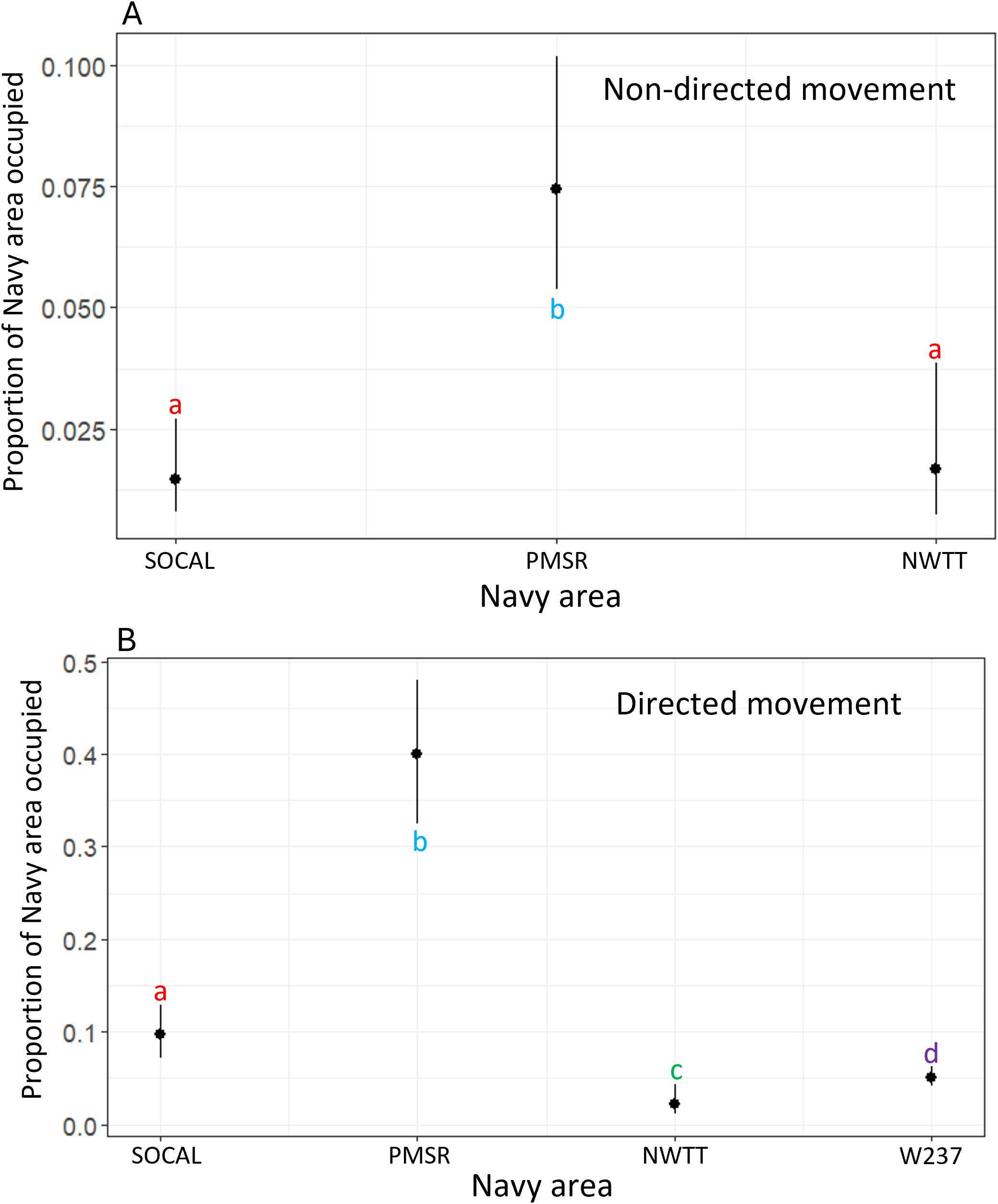
Figure 10. Predicted values for proportion of overlap of Navy areas by (A) non-directed and (B) directed movement for blue whales. Different letters/colors illustrate levels that are significantly different from one another (i.e., red "a's" are the same as one another, but significantly different than blue "b's" or green "c's").
Relative occupancy – directed movement
The proportion of overlap of directed movement in Navy areas for blue whales was also highest in PMSR (mean = 41%, SD = 13.3%, range = 7.2–55.7%; Supplementary Table S7). Mean proportion of directed movement was next highest in SOCAL (10%, SD = 6.2%, range = 2.3–27.1%), followed by W237 (5%, SD = 1.1%, range = 3.9–6.1%) and lastly NWTT (2%, SD = 1.7%, range = <1–5.6%; Supplementary Table S7).
As with the non-directed movement model, NPH was removed, as it was not significant. The final model for directed movement (pR2 = 0.631; Table 2 and Supplementary Table S4) was the same as the one for non-directed movement, with Navy area as the only significant covariate:
According to this model, the proportion of overlap of directed movement with Navy areas was significantly different between most Navy areas (p-values <0.001; Figure 10), with the largest difference occurring between PMSR and NWTT (29.8 times higher in PMSR than in NWTT). The exception was between NWTT and W237, for which there was only moderate evidence of a difference (2.4 times higher overlap in W237 than in NWTT; p-value = 0.02; Figure 10).
Fin whales
Individual residence time
Fin whales spent the most time in NWTT (mean = 14.7 d, SD = 12,5 d, range = 1.0–49.9 d; Supplementary Table S8; Figure 6), followed by SOCAL (mean = 10.6 d, SD = 11.8 d, range = 0.01–52.6 d) and PMSR (mean = 10.1 d, SD = 10.3 d, range = 0.01–62.2 d). Residence time was shortest in W237 (mean = 7.5 d, SD = 8.0 d, range = 0.03-22.0 d). PMSR had the most use by fin whales, with 64 whales spending time there, followed by SOCAL (61 whales), NWTT (16 whales), and W237 (8 whales).
The final model for the fin whale GLMM (pR2 = 0.215; Table 2 and Supplementary Table S4), was:
Individual residence times were 4.9 times longer in NWTT than in W237 (p-value = 0.006) and there was moderate evidence they were also longer in NWTT compared to PMSR (2.7 times longer; p-value = 0.014; Supplementary Figure S11). There was suggestive evidence that residence times were longer in summer than in autumn (2.2 times longer; p-value = 0.050), or in spring (1.8 times longer; p-value = 0.056; Supplementary Figure S11). HCI (p-value = 0.003) and ONI (p-value = 0.008) had significant negative effects on residence time, such that with a unit increase in each climate index, residence time in Navy areas decreased by 92% for HCI, and 43% for ONI (Figure 11).
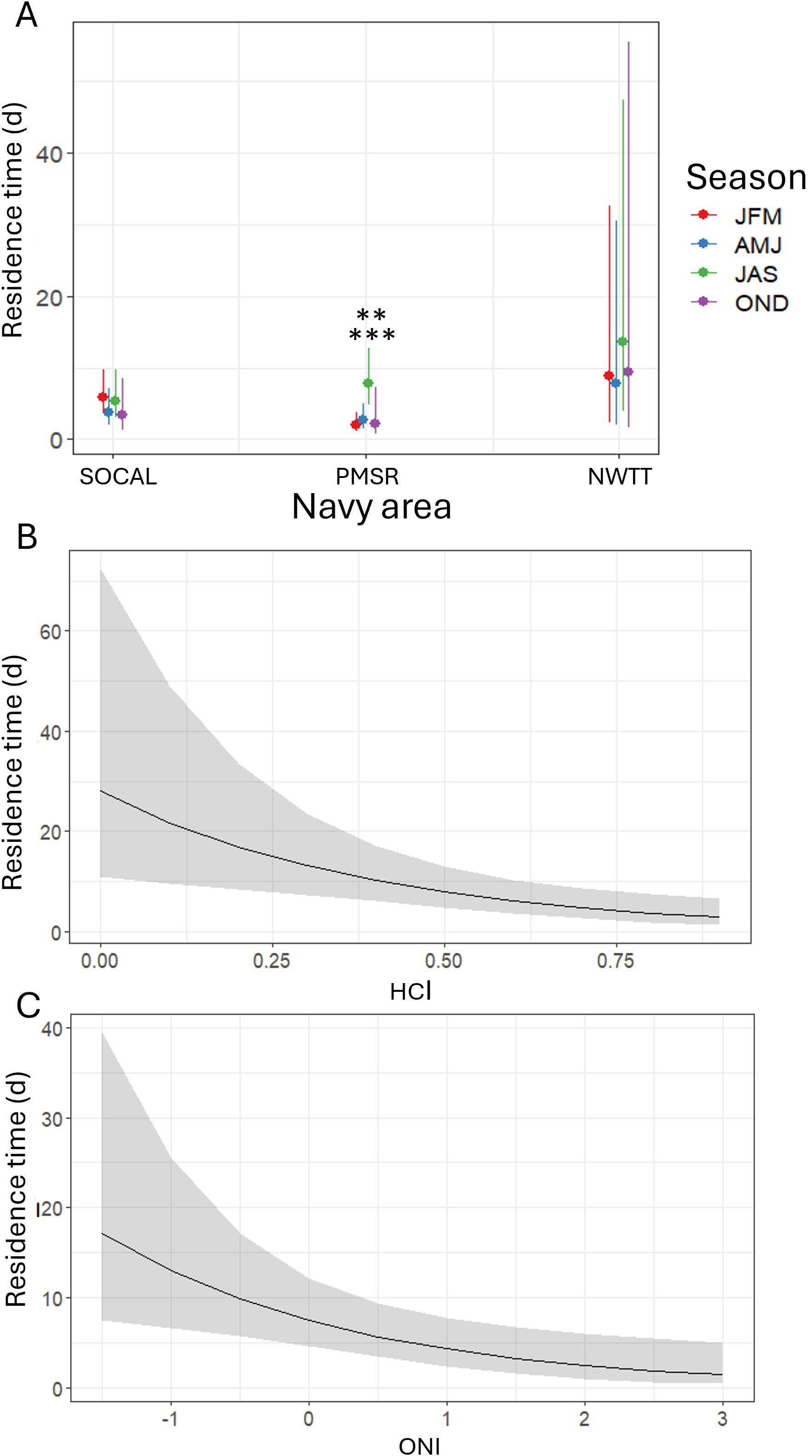
Figure 11. Predicted values of individual residence time for fin whales by (A) season within three Navy areas (SOCAL, PMSR, and NWTT), (B) HCI, and (C) ONI in all Navy areas. Asterisks in (A) denote a significant difference in PMSR between summer and winter (p-value < 0.001) and between summer and autumn (p-value = 0.008) at the following levels: ** = p-values between 0.001-0.01; *** = p-values < 0.001.
Residence times were significantly longer in summer than in winter (3.7 times longer; p-value = 0.001; Figure 11) and spring (2.8 times longer; p-value = 0.008) in PMSR. There was suggestive yet inconclusive evidence of residence times being longer in summer than in autumn (3.4 times longer; p-value = 0.050). There were no seasonal differences in residence times in NWTT or SOCAL.
The GLMM comparing residence time and sex showed no statistical difference in residence time between male and female fin whales, though the sample size of sexed whales was low (16 males, 16 females).
Proportion of tracking duration
Proportion of tracking durations in Navy areas for fin whales was highest in NWTT (mean = 0.38, SD = 0.37, range = <0.01–1.0) and lowest in W237 (mean = 0.23, SD = 0.28, range = <0.01–0.86; Supplementary Table S9; Figure 8). Values for SOCAL (mean = 0.34, SD = 0.29) and PMSR (mean = 0.29, SD = 0.25) were intermediate (Figure 8).
The best beta regression model for fin whales, excluding the one fin whale tagged in COR (removed because of insufficient sample size [n = 1] in this region) (pR2 = 0.083; Table 2 and Supplementary Table S4) was:
Fin whales had significantly higher proportions of tracking duration in NWTT than in PMSR (3.3 times higher; p-value < 0.001) and in W237 (3.3 time higher; p-values = 0.005; Supplementary Figure S12), with moderate evidence for higher proportion in NWTT than in SOCAL (1.9 times higher; p-value = 0.013). Proportion was 1.7 times higher in SOCAL than in PMSR (p-value < 0.001). Proportions of tracking duration were also significantly lower during autumn than during all other seasons (p-values < 0.004), with proportions being 2.5 times higher in winter than in autumn in the most extreme case (Supplementary Figure S12).
The interaction of Navy area with season was significant and showed that in PMSR, proportion of tracking duration was higher in summer than autumn (3.5 times higher; p-value < 0.001; Figure 12) or winter (2.4 times higher; p-value < 0.001). There was also moderate evidence for higher proportions in spring than in autumn (2.4 times higher; p-value = 0.03) or winter (1.6 times higher; p-value = 0.04). In SOCAL, proportion of tracking duration was significantly higher in winter than in spring (2.0 times higher; p-value = 0.003), summer (2.5 times higher; p-value < 0.001), or autumn (3.3 times higher; p-value < 0.001; Figure 12). In NWTT, proportion was significantly higher in winter than in autumn (11.8 times higher; p-value = 0.001) and there was moderate evidence that it was also higher in winter than in spring (3.7 times higher; p-value = 0.038; Figure 12). There was also suggestive evidence that proportion of tracking duration in NWTT was higher in summer than in autumn (4.9 times higher; p-value = 0.045; Figure 12).
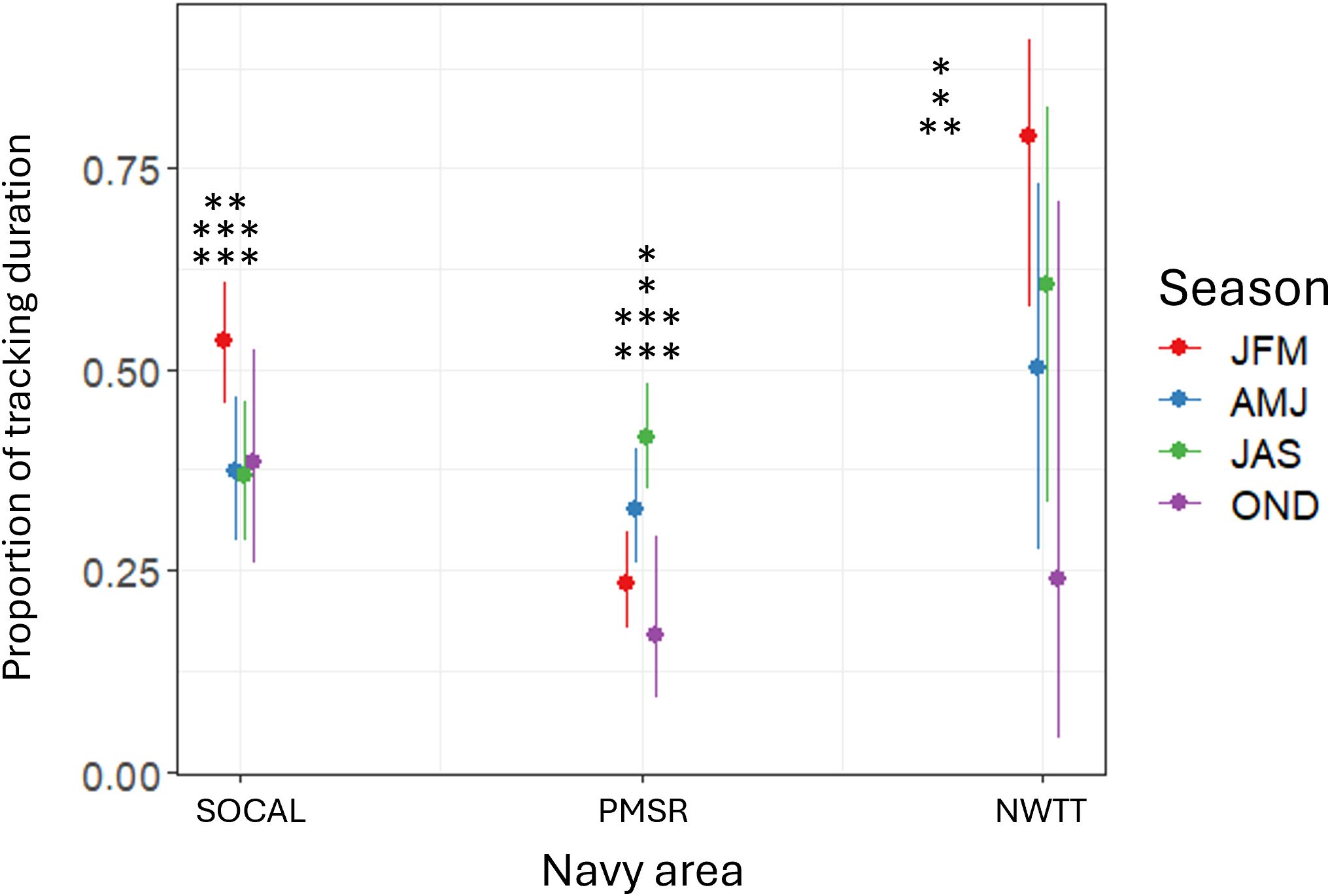
Figure 12. Predicted proportion of tracking duration for fin whales by season within three Navy areas (SOCAL, PMSR, and NWTT). Asterisks in denote significant differences in Navy areas between seasons at the following levels: * = p-values between 0.01-0.05; ** = p-values between 0.001-0.01; *** = p-values <0.001. In SOCAL, proportion of tracking duration was significantly higher in winter than in spring (p-value = 0.003), summer (p-value <0.001), or autumn (p-value <0.001). In PMSR, proportion was significantly higher in summer than in autumn (p-value <0.001) or winter (p-value <0.001) and higher in spring than in autumn (p-value = 0.03) or winter (p-value = 0.04). In NWTT, proportion was significantly higher in winter than in autumn (p-value = 0.001), with moderate evidence that it was also higher in winter than in spring (p-value = 0.038). In NWTT, there was also suggestive evidence that proportion was higher in summer than in autumn (p-value = 0.045).
There was no difference in proportion of tracking duration in Navy areas between male and female fin whales, though, as mentioned above, the sample size of sexed whales was low (16 males, 16 females).
Relative occupancy – non-directed movement
The proportion of overlap of non-directed movement in Navy areas for fin whales was highest in PMSR (mean = 11%, SD = 6.7%, range = 2.6–22.5%; Supplementary Table S10). Mean proportion of non-directed movement overlap was less than 3% in each of the other three Navy areas.
The final model for non-directed movement for fin whales (pR2 = 0.459; Table 2 and Supplementary Table S4), including the precision effect but not NPH, was:
According to the final model, the proportion of overlap of non-directed movement with Navy areas was significantly higher for PMSR than for SOCAL (6 times higher), NWTT (5.2 times higher), or W237 (4.4 times higher; p-values <0.001; Figure 13).
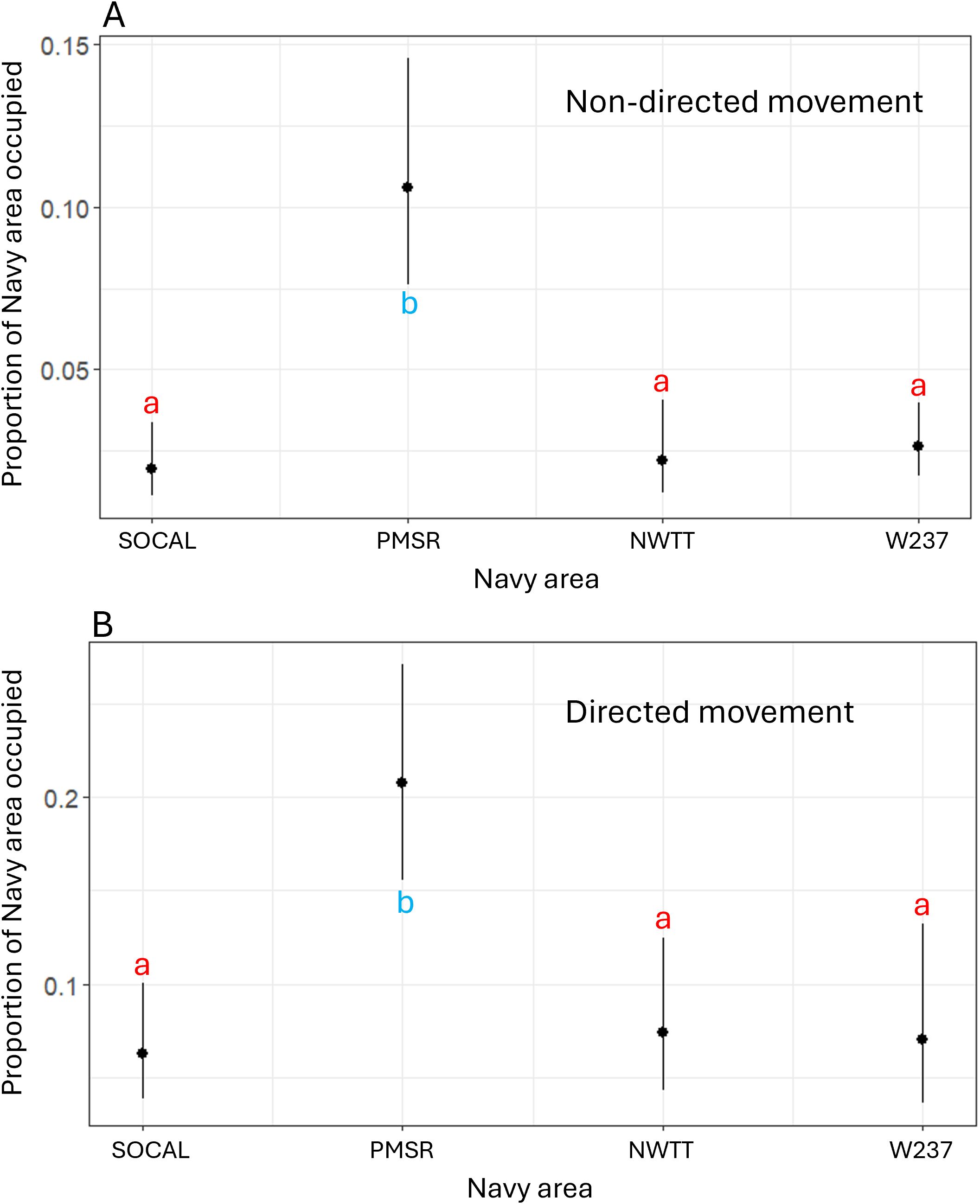
Figure 13. Predicted values for proportion of overlap of Navy areas by (A) non-directed and (B) directed movement for fin whales. Different letters/colors illustrate levels that are significantly different from one another (i.e., red "a's" are the same as one another, but significantly different than blue "b's").
Relative occupancy – directed movement
The proportion of overlap of directed movement in Navy areas for fin whales was highest in PMSR (mean = 22%, SD = 15.8%, range = 7.3–54.5%; Supplementary Table S11). Mean proportion of overlap was less than 8% in each of the other three Navy areas.
The final model for directed movement (pR2 = 0.374; Table 2 and Supplementary Table S4) included neither the precision effect nor NPH:
According to this model, PMSR had a significantly higher proportion of overlap of directed movement than did SOCAL (3.9 times higher), W237 (3.5 times higher), or NWTT (3.3 times higher; p-values <0.002; Figure 13).
Discussion
This study presents information from the largest blue and fin whale tracking dataset to date, spanning 23 years for blue whales (n = 182) and 14 years for fin whales (n = 98), with maximum tracking durations over 300 d for both species. Both species were widely distributed throughout the CCE and beyond, with locations for blue whales ranging over 52 degrees of latitude (from <1° N to 53°N) and offshore to a maximum distance of over 2,800 km and ranging over 38 degrees of longitude (from 18° N to 57° N) and over 1,800 km offshore for fin whales. Overlap with the Navy areas was extensive, with both species being found in all four Navy areas considered in this study. Differences in Navy area use were observed between the two species as well as within species, in relation to time of year, deployment region, and climatological forcing. PMSR saw the largest use by both blue (81% of all tagged whales) and fin (65% of all tagged whales) whales in terms of number of whales having locations within its boundary. SOCAL was the second most heavily used Navy area for blue (61%) and fin (62%) whales in terms of number of whales.
Blue whales
Navy area, deployment region, and season all had a significant effect on use of Navy areas by blue whales. PMSR had significantly longer residence times (mean of 17.3 d) and significantly higher proportions of tracking durations (mean of 30%) than most other Navy areas, with W237 being the one exception where residence time was not significantly different than PMSR. Most blue whales (62%) were tagged within PMSR, so high use of the area is not surprising. However, PMSR is also located in a highly productive region where southward wind-driven upwelling from Point Conception, shelf breaks, island slopes, and nearby seamounts at the west end of the Channel Islands support dense aggregations of euphausiids, blue whales' primary prey, thus contributing to the importance of this area for blue whales (Fiedler et al., 1998; Burtenshaw et al., 2004; Calambokidis et al., 2024). Together with whales' capacity for rapid dispersal (Palacios et al., in review1), this indicates the high use of PMSR is more likely due to the selection of habitat features contained in the area, rather than bias inherent to the data collection method.
Residence time in W237 was not significantly different than in PMSR, despite a mean value less than half that of PMSR (7 d vs. 17 d). This is likely due to the small sample size (4 blue whales) and comparatively high variability in individual residence times (range of 1-16 d) of whales in W237. NWTT was used by 26 blue whales and, although residence times were significantly shorter than those in PMSR, they were still relatively long (mean of 10 d, maximum of 39 d). Thus, while the number of blue whales occurring in the northernmost Navy areas (NWTT and W237) was not high compared to southern areas, the residence times in these areas highlight their importance as northern feeding habitat for some blue whales.
Residence time of blue whales in SOCAL was limited (mean = 6 d) despite its comparatively large size in relation to the other Navy areas in this study. This may have been due to the focus on deploying tags during mid-summer to early fall. Blue whales arrive off the U.S. West Coast seasonally in the late spring following the average timing of the spring phytoplankton bloom (Abrahms et al., 2019b). The whales in this study may have already moved north of SOCAL, the southernmost Navy area, by the time tags were deployed, and only a limited number of tags functioned long enough to record the return migration the following spring, when SOCAL occupancy might be more likely. For instance, one of these tags recorded the whale spending the month of May 2005 in the nearshore waters off Ensenada, Mexico, during its northbound migration (Irvine et al., 2014), with some of this time within the SOCAL boundary. Thus, additional tagging effort may be needed to characterize blue whale occurrence in SOCAL with respect to this potential bias toward early-season occurrence.
The greater use of Navy areas by blue whales tagged in SCA compared to those tagged in CCA or NCA is unsurprising as almost all SCA waters are within Navy area boundaries. Further, the newer CCA portions of PMSR were not considered in our analyses, limiting the extent of Navy areas near whales tagged in that area. High Navy area use by blue whales tagged in GCMX is also unsurprising as SOCAL extends almost halfway down the Baja California Peninsula, requiring migrating blue whales to spend extended periods of time traveling through it or perhaps stopping in it enroute to/from breeding areas, as reported above. Sample sizes for NCA and GCMX deployment regions were quite small, however, so perhaps even more informative is the difference noted when only SCA and CCA were considered in the models. In the latter case, residence time and proportion of tracking duration was greater for whales tagged in SCA than those tagged in CCA but only for PMSR and not the other Navy areas.
Residence time and proportion of tracking duration in Navy areas were also significantly greater during summer than in autumn, and, in the case of proportion of tracking duration, also greater during summer than in spring. The latter, especially, reflects the seasonality of blue whales in the CCE and their annual migration to wintering areas south of U.S. waters (Bailey et al., 2009). Sample sizes were small in winter and spring and when only summer and autumn were considered seasonal differences were apparent in only some Navy areas. In PMSR, residence time was greater in summer than autumn and in NWTT there was suggestive evidence that the reverse was true. Proportion of tracking duration was greater in summer than autumn for PMSR and SOCAL and greater in autumn than summer in W237. A northward movement of blue whales in the CCE as the feeding season progresses (Abrahms et al., 2019b) could explain these differences. Bograd et al. (2009) described a northward trend towards later and shorter upwelling seasons in the CCE, which could result in a northward progression of blue whales as they follow productivity throughout the feeding season, as has been documented by Burtenshaw et al. (2004); Irvine et al. (2014), and Abrahms et al. (2019b). This would, in turn, result in reduced residency of southern Navy areas and increased residency in northern areas.
PMSR had a significantly higher proportion of overlap of non-directed movement than NWTT or SOCAL, and while size of Navy area may play a role here, with PMSR being much smaller than the other two areas, PMSR also had many more years of overlapping non-directed movement (13 years) than NWTT (5 years), highlighting the importance of PMSR to foraging blue whales. While overlap of SOCAL was quite low, the number of years for which non-directed movement by blue whales occurred in SOCAL (12 years) was roughly the same as for PMSR, suggesting this area is also important for foraging individuals. The proportion of overlap of directed movement in Navy areas by blue whales was much higher than that of non-directed movement, while at the same time, having very similar differences between areas (highest in PMSR, for which there was 41% and 8% overlap for directed and non-directed movement, respectively). This suggests that larger portions of Navy areas are used for transiting than for foraging, as it is generally assumed that non-directed movement in feeding areas is indicative of foraging in prey patches, whereas directed movement indicates moving between prey patches (Palacios et al., 2019). Irvine et al. (2019), using a small subset of the same tagging data used in this study, showed that feeding bouts for both blue and fin whales occurred farther apart as the distance from shore increased but that the number of lunges per dive remained the same. The authors suggested two feeding strategies, with more spatially aggregated foraging nearshore around localized upwelling centers and more dispersed foraging in larger areas of elevated, but patchy, productivity offshore (Irvine et al., 2019). A more dispersed foraging strategy could result in locations being categorized as directed movement and contribute to the higher proportions of overlap of this type of movement seen here.
Climate indices did not explain a significant amount of variability in our residence time or relative occupancy models for blue whales. This is not to say that climate variability is not an important factor influencing blue whale movements (e.g., Calambokidis et al., 2009; Ryan et al., 2022). Rather, it is possible that blue whales respond to climate variability on a smaller scale than was examined here. Previous studies have shown linkages between environmental conditions and blue whale density and movements (Becker et al., 2016; 2022; Hazen et al., 2017; Abrahms et al., 2019a; Palacios et al., 2019; Becker et al., 2022), but most of these considered oceanographic factors of finer scale, both spatially and temporally (such as chlorophyll-a concentrations, sea surface temperature, sea surface height, seafloor aspect, depth), than the more regional and basin-scale climate indices we used. It should be noted that both Abrahms et al. (2019a) and Palacios et al. (2019) used subsets of the same telemetry data used in this study, including blue whale tracks from 1993 through 2008, whereas here we included 82 additional blue whale tracks obtained from 2014 through 2017. Despite these linkages, Abrahms et al. (2019b) demonstrate that memory plays an important role in the movement decisions of migrating blue whales in the CCE and not resource tracking alone, showing that blue whales selected foraging areas characterized by low year-to-year variability and high long-term productivity compared with contemporaneous measurements. For instance, in addition to responding to finer scale oceanographic conditions, blue whales may also visit areas where they previously experienced foraging success, such as in the lee of capes along the coast which generate increased upwelling and, where local ocean currents are conducive to on-shelf retention of upwelled nutrients and increased primary productivity (Fiechter et al., 2018). Within the shelf break/slope region of the CCE, dense concentrations of krill, or krill hotspots, are found primarily within or in proximity to submarine canyons and in upwelling shadows or areas where there is a balance between Ekman transport and food availability from upwelling centers (Santora et al., 2011, 2018). Such areas may represent a foraging network, where encountering food patches may be predictable in time and space (Santora et al., 2018). Riotte-Lambert and Matthiopoulos (2020) also highlight this concept in describing how animals adopt multimode movement strategies, such as using spatial memory to relocate predictable habitat features and developing home ranges in predictable landscapes, while also using area-restricted searching behavior (or non-directed movement) to search for prey items within resource patches in these home ranges.
Climate indices did have significant effects on proportion of tracking duration in Navy areas for blue whales. Both ONI and NPGO had negative relationships with proportion of tracking duration, but only in PMSR for the latter. Increasing values of ONI (+ 0.5 or higher) indicate El Niño conditions, which can influence the CCE by depressing the thermocline/nutricline, reducing the strength of upwelling (and conversely, increasing the strength of downwelling), and advecting warm, saline subtropical water into the system from the south (Jacox et al., 2016). The two main krill prey species for blue whales in the CCE, Euphausia pacifica and Thysanoessa spinifera, tend to shift their populations north (and inshore in the case of E. pacifica) during El Niño conditions, toward cooler, upwelling areas (Lilly and Ohman, 2021). During the strong El Niño of 1997/98, changes in species assemblages were noted for Monterey Bay, California, with a temporary influx of warm-water odontocetes and a decline in baleen whale densities (Benson et al., 2002). The decreased proportion of tracking durations in Navy areas seen here by blue whales with El Niño conditions could potentially be a reflection of a northward shift in whales out of U.S. West Coast waters, as shown by Calambokidis et al. (2009) for blue whales, or more wide-ranging movements if whales have to travel farther to find productive prey patches. Conversely, blue whales may have increased site fidelity to areas, including Navy areas, in U.S. West Coast waters during cool, La Niña events when primary productivity and zooplankton production is increased.
In PMSR, proportion of tracking duration for blue whales was higher in times of negative phases of NPGO. NPGO is a climate pattern that represents the intensity of the North Pacific Gyre circulations and is correlated with fluctuations of salinity, nutrients, and chlorophyll-a (Di Lorenzo et al., 2008). As such, it provides a strong indicator of fluctuations in the mechanisms driving planktonic ecosystem dynamics at higher latitudes (Di Lorenzo et al., 2008). Positive phases of NPGO are associated with enhanced equatorward winds (and therefore offshore Ekman transport) and are upwelling-favorable (Jacox et al., 2018), and vice versa during negative phases of NPGO. During times of lower primary productivity, such as in negative phases of NPGO, movements of blue whales may be constrained to those areas that remain predictably productive, such as submarine canyon areas and upwelling shadows. Santora et al. (2018) noted that, in the CCE, dense patches of krill were most associated with large shelf-incising canyons (Santora et al., 2018). One such shelf-incising canyon occurs off Point Conception, within PMSR and may provide increased foraging habitat to krill predators during times of reduced upwelling. The largest shelf-incising canyon in the CCE is the Juan de Fuca Canyon off northern Washington (Santora et al., 2018), within NWTT, so we might also expect a similar relationship with NPGO in this Navy area, were it not for smaller sample sizes in NWTT.
Fin whales
Both Navy area and season had significant effects on Navy area use (individual residence time and proportion of tracking duration) by fin whales, with use being highest in NWTT (15 d and 38% of tracking duration) and lowest in W237 (7.5 d and 23% of tracking duration). High use of NWTT by fin whales was driven by a relatively small number of individuals (16; 7 of which were tagged within NWTT) when compared to use of PMSR (64 individuals) or SOCAL (61 individuals), both of which had slightly lower use than NWTT. The large size of NWTT (over four times the size of PMSR) and the tag deployment locations within the area likely contribute to increased residency in this area. At approximately 410,000 km2, NWTT covers waters from northern California to the Canada/U.S. border, and fin whales traveling through the area to and from feeding habitat to the north would end up spending quite a bit of time there. The average residency in NWTT for fin whales in this study (15 d with a maximum of 50 d), however, suggests that NWTT may be more than just an area to transit through.
The number of years with fin whale relative occupancy overlapping Navy areas ranged from 5 (in W237) to 12 (in both PMSR and SOCAL), with NWTT falling between at 7 years. As with blue whales, proportion of overlap of relative occupancy with Navy areas was significantly higher in PMSR than in other Navy areas, both for non-directed and directed movement. While Navy area size likely plays a big role in these differences, there is more to it than that, as overlap of non-directed movement was four times higher (and three times higher for directed movement) in PMSR than in W237, areas of roughly equal size. There is increasing evidence of a smaller, year-round, resident subpopulation of fin whales in the Southern California Bight (SCB) that increasingly (since 2009) uses nearshore waters (Falcone et al., 2022). Much of the SCB is within PMSR and inclusion of these fin whales in our tagging data set could contribute to the high proportions of relative occupancy overlap seen in PMSR.
Fin whale use of Navy areas in summer months was similar to use during winter, and significantly higher than use in spring or autumn. Seasonality of tag deployments as well as tracking duration plays a role here, as the majority of fin whales were tagged in summer (43%) and winter (35%), compared to 11% in each of spring and autumn. In addition, mean tracking duration for fin whales was 23 d, with 75% of tags lasting less than 42 d, truncating use of Navy areas in seasons other than when tags were deployed. Seasonal differences in use of Navy areas were driven primarily by fin whale use in PMSR and SOCAL, In PMSR, residence time was significantly longer in summer than in winter and spring and proportion of tracking duration was significantly higher in summer than winter and autumn. In SOCAL, proportion of tracking duration was significantly higher in winter than in spring or summer. These seasonal differences align with southward distributional shifts in the winter described for both the wider-ranging California-Oregon-Washington portion of the population as well as a smaller, year-round, resident population in the Southern California Bight (Falcone et al., 2022), as habitat suitability in the CCE contracts and shifts south (Scales et al., 2017).
Two climate indices had significant effects on individual residence time in Navy areas for fin whales: HCI and ONI. Fin whales spent less time in Navy areas with increasing values of both climate indices. For HCI, this translates to increased residence times during periods of compression of cool-water habitat nearshore (low HCI). This may seem counter-intuitive, as in the CCE, cool water is associated with upwelling-driven primary productivity and, as a result, increased prey for baleen whales (Croll et al., 2005; Ryan et al., 2022). However, while low HCI values may indicate reduced foraging opportunity for whales based on more limited cold-water habitat, our results indicate it may also lead to whales remaining longer in Navy areas due to more limited alternative foraging areas. Menge and Menge (2013) report that surface-dwelling particles, such as weakly swimming zooplankton and phytoplankton, are moved away from the coast during upwelling and toward the coast during relaxation or downwelling. Fin whale movements may be more concentrated nearshore and perhaps result in more time in Navy areas (especially PMSR, where the highest number of fin whales spent time) during times of compressed upwelling whereas they may move further offshore, potentially outside of Navy areas, during periods of expansion of cool-water habitat to follow their prey.
Fin whale residence time in Navy areas also decreased with increasing values of ONI. Such a decrease during El Niño conditions (ONI values of + 0.5 or higher) could, as with blue whales, be a reflection of a northward shift out of U.S. West Coast waters or more wide-ranging movements contributing to decreased site fidelity in smaller localized areas. And again, as with blue whales, increased primary productivity and zooplankton production associated with La Niña conditions (low ONI values) may contribute to less wide-ranging movement in search of prey and higher site fidelity in areas of increased foraging success, including those inside Navy areas.
Species differences
A number of factors may contribute to the differences in Navy area use seen here between blue and fin whales, and key among them may be the migratory differences between the two species, as well as the existence of a resident subpopulation of fin whales in the SCB. Emphasizing these differences is the southern extent of hSSSM locations in this study between the two species, extending as far south as <1°N for blue whales compared to 18°N for fin whales. This difference in migratory behavior and residency is a likely explanation for the observed differences between the two species in proportion of tracking duration in Navy areas. Proportion of overall tracking duration in Navy areas would undoubtedly be less for a species, like blue whales, that migrates out of U.S. waters for several months per year.
Differences in prey preferences between the two species may also play a role in the differences in Navy area use seen here. Blue whales are stenophagous, feeding almost exclusively on krill (Fiedler et al., 1998; Croll et al., 2005; Nickels et al., 2018) while fin whales feed on small fish, squid, and copepods in addition to krill (Nemoto, 1959; Clapham et al., 1997; Pauly et al., 1998; Flinn et al., 2002; Croll and Kudela, 2007). Savoca et al. (2021) estimated that an adult ENP blue whale is likely to consume 16 tonnes of krill per day, emphasizing their reliance on finding dense patches of krill. Blue whales may have to travel widely to find sufficient quantities of food when prey patches are not localized or compressed in one area. Fin whales, on the other hand, have the flexibility to switch prey when krill patches are depleted and may be able to remain in an area otherwise unsuitable to blue whales.
Other likely contributors to species differences are the details of tagging. Tracking durations were over twice as long for blue whales as for fin whales. A large driver of this is tag style, as almost 70% of fin whale tags were of the LIMPET dart-attached "anchored" style compared to the deep-implant "consolidated" style of most of the blue whale tags. The former typically has a shorter attachment duration than the latter (Palacios et al., 2022). Twice as many tags were deployed on blue whales compared to fins, which means reduced opportunities for long-lasting tag deployments on fin whales. Shorter tracking durations for fin whales could play a big role in their higher proportion of tracking durations in Navy areas, as we had less ability to observe them moving out of Navy areas as we did for blue whales. Seasonality of tagging also plays a role in species differences, with most blue whale tag deployments taking place during summer compared to almost equal numbers of deployments in summer and winter for fin whales.
Conclusions
Blue and fin whale occurrence in Navy areas on the U.S. West Coast was extensive, with PMSR and SOCAL being used by the largest number of tagged whales for both species. PMSR also saw the longest residence times, for blue whales, while NWTT had the longest residence times for fin whales. Higher proportion of tracking durations in Navy areas for fin whales than for blue whales reflects the migratory behavior of the latter which leaves the CCE to travel to breeding areas in autumn and winter. Differences in Navy area use existed both between and within Navy areas, related to season and climate indices and Navy areas were used for both foraging and transiting. Additional tagging in spring, autumn, and winter will further our understanding of seasonal differences in Navy area use noted in this study. Our findings may be considered by Navy and regulatory agencies when assessing impacts and potential mitigation measures related to military activities, but these determinations are beyond the scope of this manuscript.
Data availability statement
The datasets presented in this study can be found in online repositories. The names of the repository/repositories and accession number(s) can be found below: Movebank Data Repository Study ID 650188969 https://doi.org/10.5441/001/1.5ph88fk2 Animal Telemetry Network 10.24431/rw1k463 https://doi.org/10.24431/rw1k463.
Ethics statement
The animal study was approved by Oregon State University Institutional Animal Care and Use Committee. The study was conducted in accordance with the local legislation and institutional requirements.
Author contributions
BL: Conceptualization, Formal analysis, Investigation, Methodology, Project administration, Supervision, Validation, Visualization, Writing – original draft, Writing – review & editing. LI: Conceptualization, Formal analysis, Investigation, Methodology, Supervision, Validation, Visualization, Writing – review & editing. TF: Data curation, Formal analysis, Investigation, Methodology, Writing – review & editing. KA: Project administration, Writing – original draft, Writing – review & editing. EF: Data curation, Investigation, Resources, Writing – review & editing. GS: Data curation, Investigation, Resources, Writing – review & editing. BM: Conceptualization, Funding acquisition, Investigation, Methodology, Project administration, Resources, Supervision, Writing – review & editing. DP: Conceptualization, Formal analysis, Funding acquisition, Investigation, Methodology, Project administration, Resources, Supervision, Visualization, Writing – review & editing.
Funding
The author(s) declare financial support was received for the research, authorship, and/or publication of this article. The OSU portion of this project for tagging from 2014-2018 was funded by the U.S. Navy, Commander, Pacific Fleet, under the U.S. Navy's Marine Species Monitoring Program, through an agreement with HDR, Inc. (Contract No. N62470-15-D-8006-17F4016) under contract to Naval Facilities Engineering Command. Funding for OSU research from 1994-2008 was provided by the Office of Naval Research (Grants 9610608, 0010085, 0310862), Tagging of Pacific Pelagics program of the Census of Marine Life (http://www.topp.org/), the National Science Foundation (www.nsf.gov), NASA (Grant NNX11AP71G), the Oregon State University Marine Mammal Institute Endowment (mmi.oregonstate.edu), the Alfred P. Sloan Foundation (www.sloan.org), the Moore Foundation (www.moore.org), the Packard Foundation (www.packard.org), and National Geographic (www.nationalgeographic.com).The MarEcoTel portion of this project was funded by the U.S. Navy, including the N45 and Living Marine Resources programs, which provided funding for fieldwork and tags. Additional funding was provided by NOAA's Southwest Fisheries Science Center and Alaska Regional Science Center, Washington Department of Fish and Wildlife Section 6, and the US Navy Pacific Fleet.
Acknowledgments
We thank Chip Johnson at Commander, Pacific Fleet, and Jessica Curran, and Christiana Boerger at Naval Facilities Engineering Command Southwest. OSU research was conducted under the authorization of NMFS Marine Mammal Protection Act/Endangered Species Act Research/Enhancement Permit Nos. 841, 369-1440, 369-1757, and 14856 and Oregon State University Institutional Animal Care and Use Committee (IACUC) Permit Nos. 2284, 2715, 3158, 3657, 4495, and 4884. We thank C. Scott Baker and Debbie Steel at Oregon State University for the genetic sexing of the skin tissue samples. We thank pilots Steve and Roxanne Parker for their aerial survey efforts; Kristopher Bauer, Collin Gillin, Theresa Kirchner, Natalie Mastick, Kyle Milliken, Ken Serven, and Will Sheehy for field assistance; R/V Pacific Storm captains Ron Briggs, Donnie Hassler, and Ken Serven, cook David Marakeljovic; and crew Jeff Lawrence, Steve Pultz, and Ryan Reyes for field support. Kathy Minta, Mark Wilke, and Minda Stiles at the OSU Marine Mammal Institute office provided invaluable logistical and administrative support for this project. We thank our collaborators from the Naval Undersea Warfare Center, in particular David J. Moretti and the Marine Mammal Monitoring on Naval Ranges group. Without the extensive logistic support of the Southern California Offshore Range staff and San Clemente Island personnel, this work would not have been possible. We also wish to acknowledge the support of the Office of Naval Research and Brad Hanson of the NOAA Northwest Fisheries Science Center for tag development. All appropriate ethics permissions were obtained prior to the commencement of this work. Tagging was conducted under NMFS Marine Mammal Protection Act/Endangered Species Act Research/Enhancement Permit Nos. 540-1811, 14245, 15330,16111, and 20465 and conducted under the Cascadia Research Collective and NOAA Marine Mammal Lab IACUC. We thank Jeff Foster, John Calambokidis, and Cascadia Research Collective for their assistance with tag deployments. The Argos Data Collection and Location System used for this project (http://www.argos-system.org/) is operated by Collecte Localisation Satellites. Argos is an international program that relies on instruments provided by the French Space Agency flown on polar-orbiting satellites operated by the U.S. National Oceanic and Atmospheric Administration, the European Organisation for the Exploitation of Meteorological Satellites, and the Indian Space Research Organization.
Conflict of interest
Author KA was employed by HDR, Inc.
The remaining authors declare that the research was conducted in the absence of any commercial or financial relationships that could be construed as a potential conflict of interest.
Publisher’s note
All claims expressed in this article are solely those of the authors and do not necessarily represent those of their affiliated organizations, or those of the publisher, the editors and the reviewers. Any product that may be evaluated in this article, or claim that may be made by its manufacturer, is not guaranteed or endorsed by the publisher.
Supplementary material
The Supplementary Material for this article can be found online at: https://www.frontiersin.org/articles/10.3389/fmars.2024.1471310/full#supplementary-material
Footnotes
- ^ Palacios, D. M., Irvine, L. M., Machette, T., Lagerquist, B. A., Falcone, E. A., Schorr, G. S., et al. Dispersal, site fidelity, and residency of satellite-tagged blue and fin whales in the California Current Ecosystem. Anim. Biotelemetry.
References
Aasen E., Medrano J. F. (1990). Amplification of the ZFY and ZFX genes for sex identification in humans, cattle, sheep, and goats. Nat. Biotechnol. 12, 1279–1281. doi: 10.1038/nbt1290-1279
Abrahms B., Hazen E. L., Aikens E. O., Savoca M. S., Goldbogen J. A., Bograd S. J., et al. (2019b). Memory and resource tracking drive blue whale migrations. Proc. Natl. Acad. Sci. U.S.A. 116, 5582–5587. doi: 10.1073/pnas.1819031116
Abrahms B., Welch H., Brodie S., Jacox M. G., Becker E. A., Bograd S. J., et al. (2019a). Dynamic ensemble models to predict distributions and anthropogenic risk exposure for highly mobile species. Divers. Distrib 25, 1182–1193. doi: 10.1111/ddi.12940
Andrews R. D., Baird R. W., Calambokidis J., Goertz C. E. C., Gulland F. M. D., Heide-Jorgensen M. P., et al. (2019). Best practice guidelines for cetacean tagging. J. Cetacean Res. Manage 20, 27–66. doi: 10.47536/jcrm.v20i1.237
Bailey H., Mate B., Palacios D., Irvine L., Bograd S., Costa D. (2009). Behavioural estimation of blue whale movements in the Northeast Pacific from state-space model analysis of satellite tracks. Endang. Species. Res. 10, 93–106. doi: 10.3354/esr00239
Barlow J. (2016). Cetacean Abundance in the California Current estimated from Ship-Based Line-Transect Surveys in 1991-2014. NOAA Southwest Fisheries Science Center Administrative Report LJ-16-01. Available online at: https://repository.library.noaa.gov/view/noaa/3916/noaa_3916_DS1.pdf (accessed July 23, 2024).
Bates D., Maechler M., Bolker B., Walker S. (2014). Lme4: Linear Mixed-Effects Models Using Eigen and s4. R Package Version 1. Available online at: http://CRAN.R-project.org/package=lme4 (accessed July 23, 2024).
Becker E., Forney K., Fiedler P., Barlow J., Chivers S., Edwards C., et al. (2016). Moving towards dynamic ocean management: how well do modeled ocean products predict species distributions? Remote Sens. 8, 149. doi: 10.3390/rs8020149
Becker E. A., Forney K. A., Miller D. L., Barlow J., Rojas-Bracho L., Urbán R J., et al. (2022). Dynamic habitat models reflect interannual movement of cetaceans within the california current ecosystem. Front. Mar. Sci. 9. doi: 10.3389/fmars.2022.829523
Benson S. R., Croll D. A., Marinovic B. B., Chavez F. P., Harvey J. T. (2002). Changes in the cetacean assemblage of a coastal upwelling ecosystem during El Niño 1997–98 and La Niña 1999. Prog. Oceanography 54, 279–291. doi: 10.1016/S0079-6611(02)00054-X
Block B. A., Jonsen I. D., Jorgensen S. J., Winship A. J., Shaffer S. A., Bograd S. J., et al. (2011). Tracking apex marine predator movements in a dynamic ocean. Nature 475, 86–90. doi: 10.1038/nature10082
Bograd S. J., Schroeder I., Sarkar N., Qiu X., Sydeman W. J., Schwing F. B. (2009). Phenology of coastal upwelling in the California Current. Geophys. Res. Lett. 36, L01602. doi: 10.1029/2008GL035933
Braun C. D., Galuardi B., Thorrold S. R. (2018). HMMoce: an R package for improved geolocation of archival-tagged fishes using a hidden Markov method. Methods Ecol. Evol. 9, 1212–1220. doi: 10.1111/2041-210X.12959
Burtenshaw J., Oleson E., Hildebrand J., McDonald M., Andrew R., Howe B., et al. (2004). Acoustic and satellite remote sensing of blue whale seasonality and habitat in the Northeast Pacific. Deep Sea Res. Part II Top. Stud. Oceanogr. 51, 967–986. doi: 10.1016/S0967-0645(04)00095-5
Calambokidis J., Barlow J. (2020). Updated Abundance Estimates for Blue and Humpback Whales Along The U.S. West Coast Using Data Through 2018 Vol. 20 (U.S. Department of Commerce), NOAA Technical Memorandum NMFS-SWRSC-634, 20. San Diego, CA.
Calambokidis J., Barlow J., Ford J. K. B., Chandler T. E., Douglas A. B. (2009). Insights into the population structure of blue whales in the Eastern North Pacific from recent sightings and photographic identification. Mar. Mammal Sci. 25, 816–832. doi: 10.1111/j.1748-7692.2009.00298.x
Calambokidis J., Kratofil M. A., Palacios D. M., Lagerquist B. A., Schorr G. S., Hanson M. B., et al. (2024). Biologically Important Areas II for cetaceans within U.S. and adjacent waters - West Coast Region. Front. Mar. Sci. 11. doi: 10.3389/fmars.2024.1283231
Calambokidis J., Steiger G. H., Curtice C., Harrison J., Ferguson M. C., Becker E., et al. (2015). Biologically important areas for selected cetaceans within U.S. Waters – west coast region. Aquat Mamm 41, 39–53. doi: 10.1578/AM.41.1.2015.39
Calambokidis P. J., Barlow J. (2013). Updated Abundance Estimates of Blue and Humpback Whales Off the US West Coast Incorporating Photo-Identifications from 2010 and 2011. Final report for Contract AB133F-10-RP-0106. PSRG-2013-13R. Available online at: https://cascadiaresearch.org/files/Rep-Mn-Bm-2011-Rev.pdf (accessed July 23, 2024).
Carretta J. V., Greenman J., Wilkinson K., Saez L., Lawson D., Viezbicke J. (2023). Sources of Human-Related injury and Mortality for U.S. Pacific West Coast Marine Mammal Stock Assessments 2017-2021 (La Jolla, CA: U.S. Department of Commerce). doi: 10.25923/qwf2-9b9
Clapham P. J., Leatherwood S., Szczepaniak I., Brownell R. L. (1997). Catches of humpback and other whales from shore stations at Moss Landing and Trinidad, California 1919–1926. Mar. Mammal Sci. 13, 368–394. doi: 10.1111/j.1748-7692.1997.tb00646.x
Clark C., Ellison W., Southall B., Hatch L., Van Parijs S., Frankel A., et al. (2009). Acoustic masking in marine ecosystems: intuitions, analysis, and implication. Mar. Ecol. Prog. Ser. 395, 201–222. doi: 10.3354/meps08402
Collecte Localisation Satellites (2015). Argos Users Manual. Available online at: http://www.argos-system.org/files/pmedia/public/r363_9_argos_users_manual-v1.6.4.pdf (Accessed December 19, 2023).
Cooke J. G. (2018a). Balaenoptera Musculus (The IUCN Red List of Threatened Species) (Accessed December 19, 2023).
Cooke J. G. (2018b). Balaenoptera Physalus (The IUCN Red List of Threatened Species) (Accessed December 19, 2023).
Cribari-Neto F., Zeileis A. (2010). Beta regression in R. J. Stat. Softw 34, 1–24. doi: 10.18637/jss.v034.i02
Croll D., Kudela R. (2007). Ecosystem impact of the decline of large whales in the north pacific. Whales Whaling Ocean Ecosyst. 202–214. doi: 10.1525/california/9780520248847.003.0016
Croll D., Marinovic B., Benson S., Chavez F., Black N., Ternullo R., et al. (2005). From wind to whales: trophic links in a coastal upwelling system. Mar. Ecol. Prog. Ser. 289, 117–130. doi: 10.3354/meps289117
Department of the Navy (2008). Southern California Range Complex Coastal Consistency Determination. Available online at: https://documents.coastal.ca.gov/reports/2008/10/W8b-10-2008-a3.pdf (Accessed September 15, 2024).
Department of the Navy (2018). Hawaii-Southern California Training and Testing Activities Final Environmental Impact Statement/Overseas Environmental Impact Statement (Pearl Harbor, Hawaii: Naval Facilities Engineering Command, Pacific).
Di Lorenzo E., Schneider N., Cobb K. M., Franks P. J. S., Chhak K., Miller A. J., et al. (2008). North Pacific Gyre Oscillation links ocean climate and ecosystem change. Geophys. Res. Lett. 35, L08607. doi: 10.1029/2007GL032838
Dunlop R. A., McCauley R. D., Noad M. J. (2020). Ships and air guns reduce social interactions in humpback whales at greater ranges than other behavioral impacts. Mar. pollut. Bull. 154, 111072. doi: 10.1016/j.marpolbul.2020.111072
Erbe C., Reichmuth C., Cunningham K., Lucke K., Dooling R. (2016). Communication masking in marine mammals: a review and research strategy. Mar. pollut. Bull. 103, 15–38. doi: 10.1016/j.marpolbul.2015.12.007
Falcone E. A., Keene E. L., Keen E. M., Barlow J., Stewart J., Cheeseman T., et al. (2022). Movements and residency of fin whales (Balaenoptera physalus) in the California Current System. Mamm Biol. 102, 1445–1462. doi: 10.1007/s42991-022-00298-4
Fiechter J., Edwards C. A., Moore A. M. (2018). Wind, circulation, and topographic effects on alongshore phytoplankton variability in the california current. Geophysical Res. Lett. 45, 3238–3245. doi: 10.1002/2017GL076839
Fiedler P., Reilly S., Hewitt R., Demer D., Philbrick V., Smith S., et al. (1998). Blue whale habitat and prey in the California Channel Islands. Deep Sea Res. Part II Top. Stud. Oceanogr 45, 1781–1801. doi: 10.1016/S0967-0645(98)80017-9
Flinn R. D., Trites A. W., Gregr E. J., Perry R. I. (2002). Diets of fin, sei, and sperm whales in british columbia: an analysis of commercial whaling records 1963-1967. Mar. Mammal Sci. 18, 663–679. doi: 10.1111/j.1748-7692.2002.tb01065.x
Gailey G., Sychenko O., Zykov M., Rutenko A., Blanchard A., Melton R. (2022). Western gray whale behavioral response to seismic surveys during their foraging season. Environ. Monit. Assess. 194 (Suppl 1), 740. doi: 10.1007/s10661-022-10023-w
Geissinger E. A., Khoo C. L. L., Richmond I. C., Faulkner S. J. M., Schneider D. C. (2022). A case for beta regression in the natural sciences. Ecosphere 13, e3940. doi: 10.1002/ecs2.3940
Gelman A., Rubin D. B. (1992). Inference from iterative simulation using multiple sequences. Statist. Sci. 7, 457–472. doi: 10.1214/ss/1177011136
Gilson A., Syvanen M., Levine K., Banks J. (1998). Deer gender determination by polymerase chain reaction: validation study and application to tissues, bloodstains, and hair forensic samples in California. Calif. Fish Game 84, 159–169.
Goldbogen J. A., Southall B. L., DeRuiter S. L., Calambokidis J., Friedlaender A. S., Hazen E. L., et al. (2013). Blue whales respond to simulated mid-frequency military sonar. Proc. R. Soc B 280, 20130657. doi: 10.1098/rspb.2013.0657
Hazen E. L., Palacios D. M., Forney K. A., Howell E. A., Becker E., Hoover A. L., et al. (2017). WhaleWatch: a dynamic management tool for predicting blue whale density in the California Current. J. Appl. Ecol. 54, 1415–1428. doi: 10.1111/1365-2664.12820
Heide-Jørgensen M. P., Kleivane L., Øien N., Laidre K. L., Jensen M. V. (2001). A new technique for deploying satellite transmitters on baleen whales: tracking a blue whale (Balaenoptera musculus) in the North Atlantic. Mar. Mamm. Sci. 17, 949–954. doi: 10.1111/j.1748-7692.2001.tb01309.x
Hildebrand J. (2009). Anthropogenic and natural sources of ambient noise in the ocean. Mar. Ecol. Prog. Ser. 395, 5–20. doi: 10.3354/meps08353
Irvine L. M., Mate B. R., Winsor M. H., Palacios D. M., Bograd S. J., Costa D. P., et al. (2014). Spatial and temporal occurrence of blue whales off the U.S. West Coast, with implications for management. PloS One 9, e102959. doi: 10.1371/journal.pone.0102959
Irvine L. M., Palacios D. M., Lagerquist B. A., Mate B. R. (2019). Scales of blue and fin whale feeding behavior off California, USA, with implications for prey patchiness. Front. Ecol. Evol. 7. doi: 10.3389/fevo.2019.00338
Irvine L. M., Winsor M. H., Follett T. M., Mate B. R., Palacios D. M. (2020). An at-sea assessment of Argos location accuracy for three species of large whales, and the effect of deep-diving behavior on location error. Anim. Biotelemetry 8, 20. doi: 10.1186/s40317-020-00207-x
Jacox M. G., Edwards C. A., Hazen E. L., Bograd S. J. (2018). Coastal upwelling revisited: Ekman, Bakun, and improved upwelling indices for the U.S. West Coast. J. Geophysical Res. Oceans 123, 7332–7350. doi: 10.1029/2018JC014187
Jacox M. G., Hazen E. L., Zaba K. D., Rudnick D. L., Edwards C. A., Moore A. M., et al. (2016). Impacts of the 2015-2016 El Niño on the California Current System: Early assessment and comparison to past events: 2015-2016 El Niño Impact in the CCS. Geophys. Res. Lett. 43, 7072–7080. doi: 10.1002/2016GL069716
Jonsen I. (2016). Joint estimation over multiple individuals improves behavioural state inference from animal movement data. Sci. Rep. 6, 20625. doi: 10.1038/srep20625
Keen E. M., Falcone E. A., Andrews R. D., Schorr G. S. (2019). Diel dive behavior of fin whales (Balaenoptera physalus) in the southern california bight. Aquat Mamm 45, 233–243. doi: 10.1578/AM.45.2.2019.233
Lilly L. E., Ohman M. D. (2021). Euphausiid spatial displacements and habitat shifts in the southern California Current System in response to El Niño variability. Prog. Oceanography 193, 102544. doi: 10.1016/j.pocean.2021.102544
London J. M. (2021). jmlondon/pathroutr: pathroutr: An R Package for (Re-)Routing Paths Around Barriers (v0.2.1). Zenodo. doi: 10.5281/zenodo.5522909
Mantua N. J., Hare S. R., Zhang Y., Wallace J. M., Francis R. C. (1997). A pacific interdecadal climate oscillation with impacts on salmon production*. Bull. Am. Meteorol. Soc. 78, 1069–1080. doi: 10.1175/1520-0477(1997)078<1069:APICOW>2.0.CO;2
Mate B., Mesecar R., Lagerquist B. (2007). The evolution of satellite-monitored radio tags for large whales: One laboratory’s experience. Deep Sea Res. Part II Top. Stud. Oceanogr 54, 224–247. doi: 10.1016/j.dsr2.2006.11.021
Mate B. R., Gisiner R., Mobley J. (1998). Local and migratory movements of Hawaiian humpback whales tracked by satellite telemetry. Can. J. Zool 76, 863–868. doi: 10.1139/z98-008
Mate B. R., Ilyashenko V. Y., Bradford A. L., Vertyankin V. V., Tsidulko G. A., Rozhnov V. V., et al. (2015). Critically endangered western gray whales migrate to the eastern North Pacific. Biol. Lett. 11, 20150071. doi: 10.1098/rsbl.2015.0071
Mate B. R., Irvine L. M., Palacios D. M. (2017). The development of an intermediate-duration tag to characterize the diving behavior of large whales. Ecol. Evol. 7, 585–595. doi: 10.1002/ece3.2649
Mate B. R., Lagerquist B. A., Calambokidis J. (1999). Movements of North Pacific blue whales during the feeding season off Southern California and their southern fall migration. Mar. Mamm. Sci. 15, 1246–1257. doi: 10.1111/j.1748-7692.1999.tb00888.x
Mate B. R., Palacios D. M., Baker C. S., Lagerquist B. A., Irvine L. M., Follett T., et al. (2018). Baleen Whale Tagging in Support of Marine Mammal Monitoring Across Multiple Navy Training Areas Covering the Years 2014, 2015, 2016, and 2017. Final Report. Prepared for Commander, U.S. Pacific Fleet. Submitted to Naval Facilities Engineering Command Southwest under Contract No. N62470-15-8006-17F4016 issued to HDR, Inc (San Diego, California: US Navy Marine Species Monitoring).
Menge B. A., Menge D. N. L. (2013). Dynamics of coastal meta-ecosystems: the intermittent upwelling hypothesis and a test in rocky intertidal regions. Ecol. Monogr. 83, 283–310. doi: 10.1890/12-1706.1
Miller P. J. O., Biassoni N., Samuels A., Tyack P. L. (2000). Whale songs lengthen in response to sonar. Nature 405, 903–903. doi: 10.1038/35016148
Nadeem K., Moore J. E., Zhang Y., Chipman H. (2016). Integrating population dynamics models and distance sampling data: a spatial hierarchical state-space approach. Ecology 97, 1735–1745. doi: 10.1890/15-1406.1
Nemoto T. (1959). Food of baleen whales with reference to whale movements. Sci. Rep. Whales Res. Inst 14, 149–290.
Nickels C. F., Sala L. M., Ohman M. D. (2018). The morphology of euphausiid mandibles used to assess selective predation by blue whales in the southern sector of the California Current System. J. Crust. Biol. 38, 563–573. doi: 10.1093/jcbiol/ruy062
NOAA Fisheries (2024). NOAA Fisheries Species Directory. Available online at: https://www.fisheries.noaa.gov/species-directory (Accessed April 4, 2024).
Oldach E., Killeen H., Shukla P., Brauer E., Carter N., Fields J., et al. (2022). Managed and unmanaged whale mortality in the California Current Ecosystem. Mar. Policy 140, 105039. doi: 10.1016/j.marpol.2022.105039
Palacios D. M., Bailey H., Becker E. A., Bograd S. J., DeAngelis M. L., Forney K. A., et al. (2019). Ecological correlates of blue whale movement behavior and its predictability in the California Current Ecosystem during the summer-fall feeding season. Mov. Ecol. 7, 26. doi: 10.1186/s40462-019-0164-6
Palacios D. M., Irvine L. M., Lagerquist B. A., Fahlbusch J. A., Calambokidis J., Tomkiewicz S. M., et al. (2022). A satellite-linked tag for the long-term monitoring of diving behavior in large whales. Anim. Biotelemetry 10, 26. doi: 10.1186/s40317-022-00297-9
Pauly D., Trites A. W., Capuli E., Christensen V. (1998). Diet composition and trophic levels of marine mammals. ICES J. Mar. Sci. 55, 467–481. doi: 10.1006/jmsc.1997.0280
Pedersen M. W., Patterson T. A., Thygesen U. H., Madsen H. (2011). Estimating animal behavior and residency from movement data. Oikos 120, 1281–1290. doi: 10.1111/j.1600-0706.2011.19044.x
Pirotta E., Booth C. G., Calambokidis J., Costa D. P., Fahlbusch J. A., Friedlaender A. S., et al. (2022). From individual responses to population effects: integrating a decade of multidisciplinary research on blue whales and sonar. Anim. Conserv. 25, 796–810. doi: 10.1111/acv.12785
Pirotta E., Mangel M., Costa D. P., Mate B., Goldbogen J. A., Palacios D. M., et al. (2018). A dynamic state mmodel of migratory behavior and physiology to assess the consequences of environmental variation and anthropogenic disturbance on marine vertebrates. Am. Nat. 191, E40–E56. doi: 10.1086/695135
Ramsey F., Schafer D. (2012). The Statistical Sleuth: A Course in Methods of Data Analysis. Cengage Learning. Google Books ISBN 978-1-133-49067-8.
R Core Team (2018). R: a Language and Environment For Statistical Computing (Vienna, Austria: R Foundation for Statistical Computing). Available online at: https://cran.r-project.org/bin/windows/base/old/ (Accessed December 19, 2023).
Riotte-Lambert L., Matthiopoulos J. (2020). Environmental predictability as a cause and consequence of animal movement. Trends Ecol. Evol. 35, 163–174. doi: 10.1016/j.tree.2019.09.009
Rockwood R. C., Calambokidis J., Jahncke J. (2017). High mortality of blue, humpback and fin whales from modeling of vessel collisions on the U.S. West Coast suggests population impacts and insufficient protection. PloS One 12, e0183052. doi: 10.1371/journal.pone.0183052
Ryan J. P., Benoit-Bird K. J., Oestreich W. K., Leary P., Smith K. B., Waluk C. M., et al. (2022). Oceanic giants dance to atmospheric rhythms: Ephemeral wind-driven resource tracking by blue whales. Ecol. Lett. 25, 2435–2447. doi: 10.1111/ele.14116
Santora J. A., Sydeman W. J., Schroeder I. D., Wells B. K., Field J. C. (2011). Mesoscale structure and oceanographic determinants of krill hotspots in the California Current: Implications for trophic transfer and conservation. Prog. Oceanography 91, 397–409. doi: 10.1016/j.pocean.2011.04.002
Santora J. A., Zeno R., Dorman J. G., Sydeman W. J. (2018). Submarine canyons represent an essential habitat network for krill hotspots in a Large Marine Ecosystem. Sci. Rep. 8, 7579. doi: 10.1038/s41598-018-25742-9
Savoca M. S., Czapanskiy M. F., Kahane-Rapport S. R., Gough W. T., Fahlbusch J. A., Bierlich K. C., et al. (2021). Baleen whale prey consumption based on high-resolution foraging measurements. Nature 599, 85–90. doi: 10.1038/s41586-021-03991-5
Sax C., Eddelbuettel D. (2018). Seasonal adjustment by X-13ARIMA-SEATS in R. J. Stat. Softw 87, 1–17. doi: 10.18637/jss.v087.i11
Scales K. L., Schorr G. S., Hazen E. L., Bograd S. J., Miller P. I., Andrews R. D., et al. (2017). Should I stay or should I go? Modelling year-round habitat suitability and drivers of residency for fin whales in the California Current. Divers. Distrib 23, 1204–1215. doi: 10.1111/ddi.12611
Schorr G. S., Falcone E. A., Moretti D. J., Andrews R. D. (2014). First long-term behavioral records from cuvier’s beaked whales (Ziphius cavirostris) reveal record-breaking dives. PloS One 9, e92633. doi: 10.1371/journal.pone.0092633
Schroeder I. D., Black B. A., Sydeman W. J., Bograd S. J., Hazen E. L., Santora J. A., et al. (2013). The North Pacific high and wintertime pre-conditioning of California current productivity. Geophys. Res. Lett. 40, 541–546. doi: 10.1002/grl.50100
Schroeder I. D., Santora J. A., Mantua N., Field J. C., Wells B. K., Hazen E. L., et al. (2022). Habitat compression indices for monitoring ocean conditions and ecosystem impacts within coastal upwelling systems. Ecol. Indic 144, 109520. doi: 10.1016/j.ecolind.2022.109520
Simonis A. E., Forney K. A., Rankin S., Ryan J., Zhang Y., DeVogelaere A., et al. (2020). Seal bomb noise as a potential threat to monterey bay harbor porpoise. Front. Mar. Sci. 7. doi: 10.3389/fmars.2020.00142
Southall B. L., Allen A. N., Calambokidis J., Casey C., DeRuiter S. L., Fregosi S., et al. (2023b). Behavioural responses of fin whales to military mid-frequency active sonar. R. Soc. Open Sci. 10, 231775. doi: 10.1098/rsos.231775
Southall B. L., DeRuiter S. L., Friedlaender A., Stimpert A. K., Goldbogen J. A., Hazen E., et al. (2019b). Behavioral responses of individual blue whales (Balaenoptera musculus) to mid-frequency military sonar. J. Exp. Biol. 222, jeb190637. doi: 10.1242/jeb.190637
Southall B. L., Finneran J. J., Reichmuth C., Nachtigall P. E., Ketten D. R., Bowles A. E., et al. (2019a). Marine mammal noise exposure criteria: updated scientific recommendations for residual hearing effects. Aquat. Mamm 45, 125–232. doi: 10.1578/AM.45.2.2019.125
Southall B., Tollit D., Amaral J., Clark C., Ellison W. (2023a). Managing human activity and marine mammals: a biologically based, relativistic risk assessment framework. Front. Mar. Sci. 10. doi: 10.3389/fmars.2023.1090132
Trenberth K. E., Stepaniak D. P. (2002). Indices of el niño evolution. J. Clim 14, 1697–1701. doi: 10.1175/1520-0442(2001)014<1697:lioeno>2.0.co;2
Tyack P., Clark C. (1998). “Quick Look - Playback of low frequency sound to gray whales migrating past the central California coast - January 1998,” in Quick Look Report, 1–34.
Vincent C., Mcconnell B. J., Ridoux V., Fedak M. A. (2002). Assessment of Argos location accuracy from satellite tags deployed on captive gray seals. Mar. Mamm. Sci. 18, 156–166. doi: 10.1111/j.1748-7692.2002.tb01025.x
Keywords: baleen whales, occupancy, California current ecosystem, foraging, Navy areas, climate indices
Citation: Lagerquist BA, Irvine LM, Follett TM, Ampela K, Falcone EA, Schorr GS, Mate BR and Palacios DM (2024) Blue and fin whale residence time and occupancy in Navy training and testing areas off the U.S. West Coast. Front. Mar. Sci. 11:1471310. doi: 10.3389/fmars.2024.1471310
Received: 27 July 2024; Accepted: 06 November 2024;
Published: 27 November 2024.
Edited by:
James Scott Maki, Marquette University, United StatesReviewed by:
Dong Liang, University of Maryland, College Park, United StatesE. Christien Michael Parsons, National Science Foundation (NSF), United States
Artur Andriolo, Juiz de Fora Federal University, Brazil
Copyright © 2024 Lagerquist, Irvine, Follett, Ampela, Falcone, Schorr, Mate and Palacios. This is an open-access article distributed under the terms of the Creative Commons Attribution License (CC BY). The use, distribution or reproduction in other forums is permitted, provided the original author(s) and the copyright owner(s) are credited and that the original publication in this journal is cited, in accordance with accepted academic practice. No use, distribution or reproduction is permitted which does not comply with these terms.
*Correspondence: Barbara A. Lagerquist, YmFyYi5sYWdlcnF1aXN0QG9yZWdvbnN0YXRlLmVkdQ==
†Present address: Daniel M. Palacios, Center for Coastal Studies, Provincetown, MA, United States