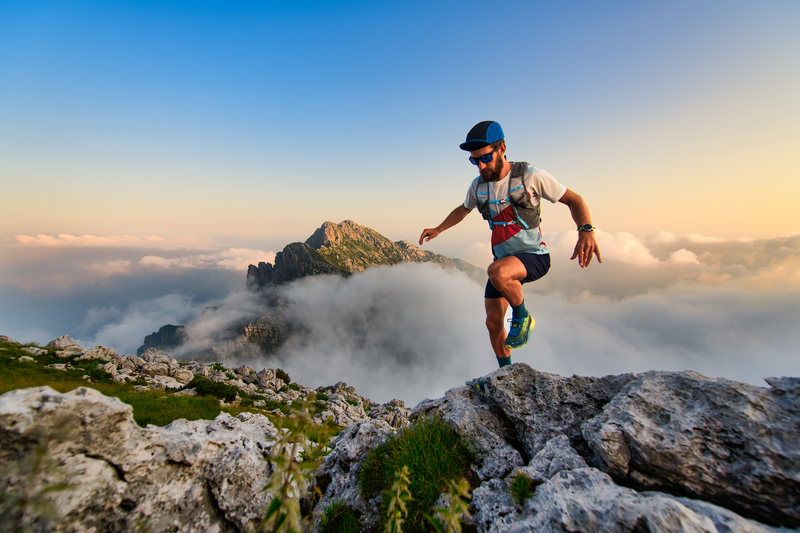
94% of researchers rate our articles as excellent or good
Learn more about the work of our research integrity team to safeguard the quality of each article we publish.
Find out more
METHODS article
Front. Mar. Sci. , 20 December 2024
Sec. Ocean Observation
Volume 11 - 2024 | https://doi.org/10.3389/fmars.2024.1467439
This article is part of the Research Topic Best Practices in Ocean Observing View all 85 articles
The collection of meteorological and oceanographic (met-ocean) data is essential to advance knowledge of the state of the oceans, leading to better-informed decisions. Despite the technological advances and the increase in data collection in recent years, met-ocean data collection is still not trivial as it requires a high effort and cost. In this context, data resulting from commercial activities increasingly complement existing scientific data collections in the vast ocean. Commercial fishing vessels (herein fishing vessels) are an example of observing platforms for met-ocean data collection, providing valuable additional temporal and spatial coverage, particularly in regions often not covered by scientific platforms. These data could contribute to the Global Ocean Observing System (GOOS) with Essential Ocean Variables (EOV) provided that the accessibility and manageability of the created datasets are guaranteed by adhering to the FAIR principles, and reproducible uncertainty is included in the datasets. Like other industrial activities, fisheries sometimes are reluctant to share their data, thus anonymization techniques, as well as data license and access restrictions could help foster collaboration between them and the oceanographic community. The main aim of this article is to guide, from a practical point of view, how to create highly FAIR datasets from fishing vessel met-ocean observations towards establishing fishing vessels as new met-ocean observing platforms. First, the FAIR principles are presented and comprehensively described, providing context for their later implementation. Then, the lifecycle of three datasets is showcased as case studies to illustrate the steps to be followed. It starts from data acquisition and follows with the quality control, processing and validation of the data, which shows good general performance and therefore further reassures the potential of fishing vessels as met-ocean data collection platforms. The next steps contribute to making the datasets as FAIR as possible, by richly documenting them with standardized and convention-based vocabularies, metadata and format. Subsequently, the datasets are submitted to widely used repositories while a persistent identifier is also assigned. Finally, take-home messages and lessons learned are provided in case they are useful for new dataset creators.
Observations of the state of the ocean have significantly increased in the last few years. According to the World Ocean Database, the amount of data transmitted in one year is comparable to that gathered in the past century (Tanhua et al., 2019). Technological advances have undoubtedly boosted such an increase by enabling the development, improvement and intensive use of sensors that can measure a wide range of data. Sensors are co-located on different observing platforms such as Argo floats (von Schuckmann et al., 2016), gliders (Rudnick, 2016; Testor et al., 2019), moorings (Venkatesan et al., 2018; Bailey et al., 2019), drifters (Lumpkin and Pazos, 2007; Lumpkin et al., 2017), satellites (Vignudelli et al., 2011; Groom et al., 2019; O’Carroll et al., 2019), HF radar systems (Paduan and Washburn, 2013; Rubio et al., 2017; Roarty et al., 2019), vessels (Patti et al., 2016; Uranga et al., 2017; Buck et al., 2019; Van Vranken et al., 2020; Gallo et al., 2022), marine animals (Fedak, 2013; March et al., 2020; Chung et al., 2021), etc. From a physical perspective, the combination of the data collected by these sensors informs about the state of the ocean and marine environment. Therefore, it improves the characterization of many oceanic processes providing essential information for different societal and environmental needs such as food, energy, transport, security, and human and environmental health.
Despite the increased spatiotemporal coverage of the current oceanographic observations, observational gaps remain in different periods and areas around the world or at certain spatiotemporal scales. Although ocean models provide more complete spatiotemporal information, observations are still key to assimilate, validate or assess the simulations (De Mey-Frémaux et al., 2019; Le Traon et al., 2019). Indeed, simulations can remarkably improve when/where observations are assimilated into them (Lamouroux et al., 2016; Turpin et al., 2016; Le Traon et al., 2019). Initial conditions and forcing could also be improved and models can better resolve previously unresolved processes by assimilating/integrating observations into them. There exist several programs such as SOOP (see all the acronyms listed in Table 1) (Goni et al., 2010), VOS (Kent et al., 2010) and GOOS (Moltmann et al., 2019) that coordinate different activities aimed at collecting and disseminating meteorological and oceanographic (herein met-ocean) observations from commercial vessels (e.g. cargo ships, fishing vessels, and ferries) to help to fill these observational gaps. Beyond the vessels involved in these programs, many other commercial vessels can still provide further observations. Particularly, commercial fishing vessels (herein only fishing vessels) have the potential to provide a high number of routinely made observations such as water temperature, salinity, currents, waves, atmospheric pressure and winds, measured by onboard mounted sensors or sensors located on their fishing gears (Martinelli et al., 2016; Patti et al., 2016; Van Vranken et al., 2020, 2023; Uriondo et al., 2024). These observations greatly interest the marine community and can significantly contribute to providing EOVs to the GOOS. There exist several programs that collect data from fishing vessels such as the FOOS and the subsequent AdriFOOS (Falco et al., 2007; Patti et al., 2016; Penna et al., 2023) in the Mediterranean Sea, the RECOPESCA project in the French fishing areas (Leblond et al., 2010; Duchêne et al., 2023), the Moana project in New Zealand (Jakoboski et al., 2024) and the fishery surveys run in the U.S. West coast (Gallo et al., 2022) among others (see Van Vranken et al. (2023) for a more detailed review). Some of these projects are linked to a recent initiative, the FVON, which is trying to build a global network of fishing vessels as additional platforms for ocean observations (Van Vranken et al., 2023). In fact, FVON has been recently endorsed as an emerging GOOS network (https://oceanexpert.org/document/34141). In addition, they also aim to establish community standards and best practices.
Fishing vessels have other priorities than managing met-ocean data and the cost of vessel digitalization needed for this could be a handicap (Bradley et al., 2019; Zhao et al., 2019). Moreover, although there are collaborative programs, there can also be a lack of trust in sharing their data (Yochum et al., 2011; Van Vranken et al., 2023). However, fisheries also depend on the met-ocean observations that inform about the state of the ocean and marine environment, to forecast fishing grounds and routes optimization (Granado et al., 2021; Goikoetxea et al., 2024) or to adapt to changing grounds due to climate change (Baudron et al., 2020; Rubio et al., 2022; Erauskin-Extramiana et al., 2023). Consequently, the collaboration between oceanographic and fisheries communities is essential for the benefit of the overall marine community (Yochum et al., 2011; Patti et al., 2016; Gawarkiewicz and Malek Mercer, 2019; Imzilen et al., 2019; Van Vranken et al., 2020, 2023; Gallo et al., 2022). To increase fisheries’ willingness to share their data, the conditions under which data can be accessed or published must be agreed with the data provider, hence, access restrictions, as well as data provider anonymization can be key (Smith et al., 2019).
Apart from engaging fisheries in met-ocean data sharing, Van Vranken et al. (2023) identified other issues, including the processing and management of the increasing volume and diversity of data. These challenges are also observed in other disciplines due to advancements in technology, the proliferation of Big Data and the emergence of artificial intelligence. Hence, data management practices have been recognized as a critical part of research (Medina et al., 2022). Concerning met-ocean data, effectively making them accessible and manageable to current and future users is still a challenge (Tanhua et al., 2019). To address these difficulties, dataset creators should adhere as much as possible to the FAIR principles (Wilkinson et al., 2016; Dunning et al., 2017; Mons et al., 2017; Stall et al., 2019; Tanhua et al., 2019), which were established in a multi-stakeholder workshop (Wilkinson et al., 2016), and further revisited to clarify what is (and is not) considered as FAIR (Mons et al., 2017). Currently, met-ocean datasets have an increasingly high degree of FAIRness facilitating easier and better use of the data. At the same time, the increasing volume and diversity of data also present a challenge in enhancing the FAIRness of met-ocean data (Tanhua et al., 2019). Anyhow, adopting the FAIR principles during the data lifecycle should be considered in any data management practice (Tanhua et al., 2019; Jakoboski et al., 2024).
Considering the substantial unexploited met-ocean data collected by fishing vessels and the crucial importance of sharing highly FAIR datasets within the marine community, the main objective of this article is to foster fishing vessels as observing platforms by bringing guidance to future met-ocean dataset creators on adhering to the FAIR principles. The process from data acquisition to sharing a highly FAIR dataset is complex. This article provides guidance on this journey. In Section 1, the general topic and a list of acronyms are introduced. Section 2 explains the FAIR principles and provides information for their later practical implementation. Section 3 comprehensively describes the steps to be followed from the data acquisition to the final data sharing, illustrating the process for adhering to the FAIR principles and including techniques for anonymization. This is showcased through three case studies of three ECVs (two of them EOVs): seawater near-surface temperature, wind and near-surface current velocities, which are extensively collected by fishing vessels. These variables expand beyond those typically considered by FVON which mainly focuses on subsurface profiling data. Consequently, the datasets presented in this article further highlight the broader potential of fishing vessels for collecting a wide range of variables. Finally, Section 4 presents the final remarks. Although the guidelines presented in this article are oriented to met-ocean dataset creators from fishing vessel observations, they also can be useful for other kinds of dataset creators.
This section describes the FAIR principles (Wilkinson et al., 2016) and provides insights into their practical implementation. This will later help in understanding the steps followed during the fishing vessel data lifecycles, specifically described in Section 3. The next subsections present each of the principles (in italics) as defined by Tanhua et al. (2019) and each of them is further explained to enhance understanding and clarify their implications. Practical information about their application to fishing vessel-based met-ocean data is also provided. For more detailed information and general examples of each principle, the reader is referred to https://www.go-fair.org/fair-principles/ and to Tanhua et al. (2019).
Findable: each dataset should be identified by a unique persistent identifier and described by rich, standardized metadata that clearly include the persistent identifier. The metadata record should be indexed in a catalogue and carried with the data (from Tanhua et al., 2019).
The dataset created should be registered in a searchable online catalogue (i.e., a well-known or trusted data repository or aggregator. In the registry of research data repositories (http://www.re3data.org) the adequate ones can be found) and made discoverable by standardized and richly documented metadata and a unique persistent identifier. The metadata is a set of attributes that describe the dataset, which aids in making the dataset Findable by facilitating searchable keywords and information. Thus, even if data are not easily accessible or are restricted to specific uses, making metadata available is still important. Concerning the persistent identifiers, they provide an infrastructure for persistent unique identification of the dataset and should be also included in the metadata. Persistent identifiers are assigned to share objects with anyone who wants to find them easily, usually the interested user community, and can be also used as the register of the intellectual property of the object. They can be defined as names (with letters, numbers, dots and slashes) but they are often expressed as URLs, contributing to more Findable objects. Persistent identifiers improve the traceability of the original source, thus acknowledging the data provider and the dataset creator, and facilitating the exchange between the latter and the user if necessary (Tanhua et al., 2019). There are several persistent identifiers oriented to datasets such as the ARK, which identifies anything digital, physical or abstract; the Handle, which is a general-purpose global name service for digital contents, used by many high-level identification systems; and the DOI, which is the most used persistent identifier (there are approximately 300 million DOIs assigned to date, https://www.doi.org/the-identifier/resources/faqs) intended for digital objects such as data, documents or code.
There are two main ways of assigning a DOI to a dataset. One way is by becoming a member of one of the Registration Agencies managed and governed by the International DOI Foundation (https://www.doi.org/), which safeguards all intellectual property rights relating to the DOI system (i.e. owns or licenses on behalf of registrants). Many millions of DOI names have been assigned to date through a growing federation of Registration Agencies worldwide. On a local scale, the Chinese DOI or the Japan Link Center provide DOIs for research data among other objects (https://www.doi.org/the-community/existing-registration-agencies/), however, at a global scale, DataCite (https://datacite.org/) is the used one specifically for research data. Another way for assigning a DOI is by publishing the datasets in established repositories which facilitate assigning a DOI for the submitted datasets.
The DOI is a widely used persistent identifier for ocean datasets and can be perfectly used for fishing vessel-based met-ocean datasets (e.g. doi:10.17882/75396, doi:10.17882/91719). In general, DataCite is the proper Registration Agency for obtaining these DOIs. Concerning the repositories that facilitate assigning a DOI, these could be non-specific such as Zenodo (https://zenodo.org/) or more specific to shelter met-ocean data such for example, Pangaea (https://www.pangaea.de/), which addresses georeferenced data from Earth system research; SEANOE (https://www.seanoe.org/) and MDA (https://marinedataarchive.org/), which are focused on ocean data; NCEI (https://www.ncei.noaa.gov/products), which provides environmental data, products and services covering the ocean; GKH (https://gkhub.earthobservations.org/), which focus on Earth observation data; or the NERC Data Catalogue Service (https://data-search.nerc.ac.uk/geonetwork/srv/eng/catalog.search#/home), which shelters environmental data.
Accessible: the dataset and its metadata record should be retrievable by using the persistent identifier and a standardized communications protocol. In turn, that protocol should allow for authentication and authorization, where necessary. All metadata records should remain accessible even when the datasets they describe are not easily accessible (from Tanhua et al., 2019).
Data published by data repositories or aggregators shall be made available through their data access protocols based on universal or standardized implementations (e.g. http, ftp). Machine-to-machine interface is also encouraged. The data provider and dataset creators should be granted to decide the required level of authentication, i.e. to which degree, or under which conditions the data are available (Mons et al., 2017). For instance, specific regulations might be applied when data are used for non-scientific or commercial purposes, or separate fees may apply for the reproduction and delivery of data when the transfer of data does not cover reproduction costs. In these cases, the user should find the contact information of the dataset creator in the metadata, or it should be provided during the data access steps, allowing users to ask for authorization. Nevertheless, access to the metadata of each dataset should be open without any restrictions and should continue to exist even if the data are no longer available.
The selection of the most appropriate data publisher depends on the research topic and data characteristics. Dataset creators should find the ones that best adjust to their requirements. Nonetheless, publishers with universal data access protocols are recommended. Several non-specific general data repositories have emerged in recent years such as Harvard Dataverse (https://dataverse.harvard.edu/), DataHub (https://datahub.io/) and Zenodo, the latter developed under the European OpenAIRE program (https://www.openaire.eu/). Through OpenAIRE, Zenodo allows for easy connection with specific European Funding that must be acknowledged when datasets are used. Concerning specific repositories that can shelter met-ocean data, examples include Coriolis (https://www.coriolis.eu.org/), which provides in-situ data for operational oceanography, and the above-mentioned Pangaea, SEANOE, MDA, GKH and NERC Data catalogue service, to name just a few. Regarding data aggregators, these are organizations that collect data from different sources and provide useful datasets with value-added processing (Loshin, 2012). For ocean data, in addition to sheltering ocean observations, several data aggregators make value-added ocean data publicly available, such as CMEMS (https://marine.copernicus.eu/), NCEI, SeaDataNet (https://www.seadatanet.org/) and EMODnet (https://emodnet.ec.europa.eu/en) among others. A comprehensive registry to find and assess the most suitable data repositories and aggregators can be found at http://www.re3data.org.
General or specific data repositories and aggregators publish the data through data servers, nevertheless, individuals or companies have also the option to establish their own. Several web platforms facilitate the creation of personal data servers such as OPeNDAP (https://www.opendap.org/), WCS (https://www.ogc.org/standard/wcs/), SOS (https://www.sosinventory.com/), OBIS (https://obis.org/) and ERDDAP (https://www.ncei.noaa.gov/erddap/index.html), among others. Each of them is good on its own, however, ERDDAP is especially interesting because it enables the creation of data servers in a free and open-source way and facilitates the easy downloading of subsets of scientific datasets in common file formats. Moreover, it allows the addition of extensive metadata and can unify data from different data servers with different file formats and consistently provide the data in the required one (it can provide data in e.g. NetCDF (.nc), .csv, .json, .mat, and other formats).
The above-mentioned non-specific or specific (for met-ocean data) repositories, can be suitable for fishing vessel-based met-ocean datasets. For instance, in the AdriFOOS project data is available in SEANOE (Penna et al., 2023). The mentioned aggregators can also be adequate to ingest these datasets as long as the required requisites (by the aggregator) are fulfilled. In fact, there already exist fishing vessel-based met-ocean datasets in SeaDataNet (https://cdi.seadatanet.org/search), EMODnet (https://emodnet.ec.europa.eu/geonetwork/emodnet/eng/catalog.search#/search?facet.q=keyword%2Ffishing%2520vessel&resultType=details&sortBy=sortDate&from=1&to=20&fast=index&_content_type=json&any=fishing%20vessel) or CMEMS (https://marine.copernicus.eu/news/fishing-data-meet-vessels-helping-monitor-and-map-north-sea). Data published in personal data servers is another option and the ERDDAP is widely used by met-ocean dataset creators. Particularly for fishing vessel-based met-ocean datasets, examples include the AdriFOOS (Penna et al., 2023; https://data-nautilos-h2020.eu/erddap/info/index.html?page=1&itemsPerPage=1000) and the ODN Fisheries Ocean Data (https://erddap.oceandata.net/erddap/index.html) ERDDAPs. Data aggregators also provide ERDDAPs to shelter met-ocean data such as the EMODnet Physics ERDDAP (https://erddap.emodnet-physics.eu/erddap/index.html) where for example, the Moana Project (Jakoboski et al., 2024) publish fishing vessels-based met-ocean datasets (https://erddap.emodnet-physics.eu/erddap/info/moanaproject/index.html).
Interoperable: Both metadata and datasets use formal, accessible, shared, and broadly applicable vocabularies and/or ontologies to describe themselves. They should also use vocabularies that follow FAIR principles and provide qualified references to other relevant metadata and data. Importantly, the data and metadata should be machine accessible and parsable (from Tanhua et al., 2019).
Interoperability allows easy data exchange and reuse between researchers, institutions, organizations, countries and others. To that end, datasets should follow recognized standards and conventions, as much as possible, so that they are understandable for everyone. The use of standard vocabularies is key for avoiding ambiguities and achieving the consistency required for Interoperable datasets. This ensures that data from different sources can be harmonized and compared more easily. Moreover, it contributes to a better interpretation by computers (machine readability) for more automated management of the data and thereby also facilitates integration into larger data systems. Vocabularies on their own should also adhere to the FAIR principles so that they can be found, accessed, interoperated and reused. For the oceanographic community, there exist several standard vocabularies such as the ones of the NERC Vocabulary Server (https://vocab.nerc.ac.uk/, https://vocab.nerc.ac.uk/search_nvs/), which includes controlled vocabularies and standardized concepts from SeaDataNet, EMODnet, OSPAR, etc. If a dataset is linked to another one (because it is built on it or provides complementary information) it should be specified in the metadata to provide more context on the dataset.
In addition to standard vocabularies, the way data and metadata are structured within the files, as well as the file format, should follow international standards or conventions (Smith et al., 2019). Note that, preferably, file formats should be machine readable by common or free-to-use software. As in many other communities, the oceanographic community commonly uses the NetCDF machine-independent format, created by UNIDATA, to support the creation, access, and sharing of array-oriented (temporal and spatial) scientific data (https://www.unidata.ucar.edu/software/netcdf/). This format enables the adoption of the CF conventions that combine data and metadata in a single file (https://cfconventions.org/). There are several versions of the CF conventions that use different attributes, and the datasets can additionally contain non-standard attributes without representing a violation of the convention. Moreover, there are other complementary standards such as ISO 19115, related to geographical information and ISO 19139, related to XML implementation schema for the geographical information of the metadata facilitating data and metadata exchange by machines. All these standards and conventions emerged several years ago as the principal ones for the oceanographic community (Hankin et al., 2010; Pouliquen et al., 2010; Snowden et al., 2010) and have had increasing adoption in recent years. Note that standards and conventions should be documented in the metadata.
The mentioned vocabulary standards as well as the file structure and format standards or conventions are widely used within the oceanographic community and arise as the appropriate ones for fishing vessel-based met-ocean datasets. In fact, the file convention adopted currently by FVON is the CF convention as the basis (Van Vranken et al., 2023) and different projects have adopted the CF convention and the NetCDF format such as Moana (Jakoboski et al., 2024), OBSERVA.FISH (Santos et al., 2024) and AdriFOOS (Penna et al., 2023).
Reusable: To meet this principle, data must already be findable, accessible, and interoperable. Additionally, the data and metadata should be sufficiently richly described that it can be readily integrated with other data sources. Published data objects should contain enough information on their provenance to enable them to be properly cited and should meet domain-relevant community standards (from Tanhua et al., 2019).
Standards and conventions are needed to ensure Interoperability; however, they should also meet the ones of the targeted community or agree with the community’s best practices for being Reusable. In case the community’s best practices are undefined, the way to achieve the highest degree of Reusability is by using internationally agreed standards and conventions (Tanhua et al., 2019). For the oceanographic community, the standards and conventions defined in the previous section are the most used ones (Hankin et al., 2010; Pouliquen et al., 2010; Snowden et al., 2010). Additionally, it might be helpful to look at the standards, conventions and attributes used in similar datasets as done by the FVON-participating programs (Van Vranken et al., 2023).
Metadata is critical to making data Reusable. It should be carried together with the data and richly describe the dataset, also stating its provenance so that the user can make appropriate decisions on whether the data is Reusable in each case. In addition, the conditions for the use of the data (i.e. data usage license) should be explicitly specified (Margoni and Tsiavos, 2018). Nowadays, there is a wide range of licensing options available, ranging from more to less restrictive (Labastida and Margoni, 2020). Creative Commons licenses (https://creativecommons.org/), for example, facilitate a standardized way to grant the public permission to use creators’ (from individuals to large companies) work while recognizing the original creator under copyright law. There are also other legal tools called Open Data Commons (https://opendatacommons.org/), which are very specifically designed for open data rather than for different types of content. The license should be directly stated in the metadata as other previous information.
The adoption of the CF convention by FVON is in line with the trend of the oceanographic community (Hankin et al., 2010; Pouliquen et al., 2010; Snowden et al., 2010) and, thus should be the one used for fishing vessel-based met-ocean datasets. In addition to richly describing the provenance of the data, additional documentation can be added to the metadata thus increasing the Reusability. To this end, documents can be shared along with the data if the data repository or aggregator permits it (e.g. Zenodo, GKH, CMEMS). Concerning the conditions for the usage of the data, it is advisable to agree them with the data provider so that providers can contribute and have more control over what is done with their data (e.g. Jakoboski et al., 2024).
Once the datasets are created and published, the FAIRness can be assessed by introducing the DOI into online checkers such as https://fair-checker.france-bioinformatique.fr/check (Gaignard et al., 2023).
This section presents the lifecycle of fishing vessel-based met-ocean data through three specific case studies to showcase the steps followed for creating datasets that adhere to the FAIR principles as much as possible. The data used are (i) seawater near-surface temperature and (ii) wind measurements collected by fishing vessel onboard sensors (Figure 1A), and (iii) near-surface ocean currents derived from buoy trajectories collected by fishing vessels.
Figure 1. (A) The location of the sensors in the vessels (adapted from Uriondo et al., 2024). (B) The area covered by the vessels in orange color in the Indian Ocean.
Temperature data were obtained by external Pt100 temperature sensors placed on the vessels’ hulls at -7 m (Figure 1A), thus providing near-surface temperature data with accuracies of 0.3°C. Wind data were obtained by Furuno FI5001 anemometers, which measure the speed and direction of the apparent wind relative to the vessel with accuracies of 10 m/s and 10°, respectively. Therefore, it was necessary to compensate for the vessels’ speed and heading to establish the true wind speed and direction (relative to the Earth’s north). To reduce vessels’ disturbances in the measurements, anemometers were positioned on the foreside of the bow pole as far as possible from the vessel structure and at 7.5 m from the deck (Figure 1A). The buoys, typically used by tuna fisheries, are attached to a floating structure usually made of a bamboo raft, equipped with floats and a subsurface structure built of old fishing nets that covers the upper water column and that follows the water parcels’ movement, like oceanographic drifters and their drogues. These buoys transmit their position by satellite communication and therefore can provide information about oceanic currents with accuracies of around 1 cm/s (Niiler et al., 1995; Poulain et al., 2012) as they have a similar configuration as drifters.
The steps followed during the lifecycles are depicted in Figure 2 and they are further described in the following subsections. As the main aim of this section is to showcase the steps of the data lifecycle, from acquisition to sharing, several non-essential details were omitted. However, the lifecycle is still comprehensively described, and more specific information can be found in the PDDs (later introduced in Sections 3.3 and 3.4; https://zenodo.org/records/10677365) generated for each dataset. Moreover, details such as the amount of fishing vessels or the exact area covered by the vessels were also omitted in order to anonymize the data provider and its activity, as agreed with them.
All the data herein presented were collected by fishing vessels in the Indian Ocean (main sampling areas in Figure 1B). Temperature and wind data were collected for two years, whereas the buoy positions covered a period of a decade. Temperature and wind measurements were continuously made at 1 Hz and were also collected at an onboard server, while buoy positions were directly sent through a satellite connection. Then, all the data were automatically daily sent to land where they were stored at the data manager’s local data servers. For a detailed description of the onboard system and the data flow, the reader is referred to Uriondo et al. (2024).
Once all the data were obtained, they were QC, processed and manually validated (in delayed mode). These steps are briefly shown herein, however, their complete description as well as a brief discussion of the results can be found in the PDDs (https://zenodo.org/records/10677365). For the validation, comparisons with datasets that do not contain in-situ data (i.e. remote sensing or even model data) and have a lower spatiotemporal resolution were performed because there was no other data available. Therefore, the observations were grossly validated. Specific validation with in-situ high-resolution reference observations along the vast ocean is a difficult task that would require the use of more means and planning (e.g. Santos et al., 2024). Given the complexity of this type of validation, it can be considered an optional exercise. In addition, note that QC, processing, and validation steps are not necessary for adhering to the FAIR principles (https://www.go-fair.org/fair-principles/r1-metadata-richly-described-plurality-accurate-relevant-attributes/), provided that data provenance is well documented in the global attributes of the metadata, such as ‘comment’, ‘qc_manual’, ‘references’ and ‘summary’ (see Section 3.3). However, QC is a globally recommended step by the Ocean Best Practices Community (https://repository.oceanbestpractices.org/; see examples in Penna et al., 2023 and Jakoboski et al., 2024) for having a known data quality, hence making the dataset more appealing to a wider range of users and more likely ingested into global data repositories or aggregators. Conversely, QC and the subsequent uncertainty estimations are needed for transforming raw data into EOVs/ECVs (Lindstrom et al., 2012).
Temperature data were QC based on the QARTOD manual of the IOOS (Bushnell and Worthington, 2020), by selecting the QC filters that best suited the data and adapting them if needed. After the QC, 20.63% of the data were identified as spurious and then removed.
Then, the remaining data were compared against the satellite SST SST_GLO_SST_L4_REP_OBSERVATIONS_010_011 product from CMEMS (Good et al., 2020; https://doi.org/10.48670/moi-00168). Although this daily product has a lower temporal resolution compared to the 1 Hz data measured at the vessel, the comparisons enabled to grossly assess the quality of the vessel data. In order to make the data comparable, the temperature and position data from two selected vessels were daily averaged, and subsequently, CMEMS data were interpolated to the vessels’ positions.
The correlations show a good agreement (over 0.91), the RMSDs are not higher than 0.7°C (Table 2) and the uncertainty is 0.69°C. The slopes of the linear adjustment in Figure 3 are also close to 1. There is a slight overestimation of CMEMS temperature probably because it corresponds to the surface and not to temperatures at -7 m. This might also affect having RMSD values higher than the sensor accuracy (0.3°C). In any case, the general agreement is good.
Table 2. Correlation, RMSD and slope of the linear regression (shown in Figure 2) values of the comparisons between the temperatures of vessels 1 and 2 versus CMEMS.
Figure 3. Temperature comparisons between the vessel and CMEMS. For vessel 1 (A) and vessel 2 (B). The red line indicates the major axis regression model and the black line indicates the 1:1 isoline.
Given that temperature sensors were located at -7 m under the water, this was the depth considered for the datasets. For the final product, a final step was made towards the anonymization of the data provider, and temperature measurements (along with position and time data) from each vessel were half-hourly averaged and then the data of all the vessels were merged within the dataset as agreed with the data provider.
Wind data were QC based on the IOOS QARTOD manual (Bushnell and Worthington, 2017), selecting the QC filters that best suited the data and adapting them if needed. In addition, data affected by the pole where the anemometers were located were also removed (this analysis can be found in the wind PDD: https://zenodo.org/records/10677365). On the whole, 22.58% of the data were identified as spurious and then removed.
Then, the remaining data were compared against two wind datasets. These datasets were the hourly WIND_GLO_PHY_L4_NRT_012_004 product of CMEMS (https://doi.org/10.48670/moi-00305) and the ERA5 dataset (Hersbach et al., 2023; https://doi.org/10.24381/cds.adbb2d47) that combine numerical model and satellite observations. To make the data comparable, data from two selected vessels were adapted by hourly averaging wind and position values, while CMEMS and ERA5 data were interpolated to the vessels’ positions. The correlations show a fair agreement (between 0.47 and 0.65), the RMSDs are not higher than 4 m/s (see Table 3) and the uncertainty is 3.66 (4.08) m/s for U (V), thus indicating that anemometer data fairly represents the wind as the accuracy of the anemometer is 10 m/s. Since the anemometers are located 10 m above the water, this was the height considered for the dataset.
Table 3. Correlation and RMSD values of the comparisons between anemometer of vessels 1 and 2 versus CMEMS and ERA5 for U and V wind components.
For the final product, as with the temperature dataset, an anonymization step agreed with the data provider was performed by half-hourly averaging each vessel’s data and merging the information from all the vessels within the dataset.
First, several QC filters were applied based on Baidai et al. (2017) and Hansen and Poulain (1996) for removing erroneous locations, mainly related to failures in satellite communication, location data acquisition and onboard positions. 12.68% of the raw data were identified as spurious and consequently removed. Additionally, trajectories containing onboard sequences in between, as well as those with significant gaps, were split into separate trajectories.
Then, interpolation was carried out to obtain position data every 6 hours by the Kriging technique (Hansen and Herman, 1989; Hansen and Poulain, 1996). Once the positions were interpolated, the velocities were estimated using a 12-hour centered scheme and then decomposed into zonal and meridional components. A few positions (0.04% of the data) provided unrealistic velocities higher than 3 m/s (peak speeds of 2.6 m/s were observed in the Agulhas current (Lutjeharms, 2006)); thus, those positions were removed.
Subsequently, the velocity data obtained were compared against drifter-derived velocities. The drifter dataset used was the NOAA Global Drifter Program data drogued at -15 m (Lumpkin and Centurioni, 2019; https://www.aoml.noaa.gov/phod/gdp/interpolated/data/all.php), processed by the Drifter Data Assembly Center at the Atlantic Oceanographic and Meteorological Laboratory. Following the same approach as Imzilen et al. (2019), data pairs of the same date and a maximum distance of 10 nm were compared. As in Imzilen et al. (2019), the correlations showed good agreement with values of 0.90 for U and 0.89 for V (see Table 4). Concerning the errors, the mean of the absolute value of the difference between both datasets was around 10 cm/s and the RMSD value around 18 cm/s, much higher than the measurement accuracy (around 1 cm/s). However, note that all these comparisons were made with data pairs that did not correspond to the same position (maximum distance of 10 nm) resulting in bigger errors than for pairs that were closer to each other. These errors also depend on the oceanic processes occurring around the buoys, such as frontal areas, that can enlarge them, or (sub)mesoscale eddies, that can favor retention conditions and decrease the errors. The RRMSD ranged between 0.42 and 0.48, and the uncertainty is 29.9 (26.9) cm/s for U (V).
Table 4. The mean of the absolute value of the difference between buoy and drifter-derived velocities and its STD, RMSD between both datasets, the RRMSD relative to the drifter dataset and the correlation values for U and V current components.
The depth of the buoys’ subsurface structure varies, reaching depths of -80 m; however, they usually reach depths of -50 m or less in the Indian Ocean (Murua et al., 2016). Given the good agreement between currents from both devices, the velocities contained within the created dataset were considered representative of the same depth as the drifters’, that is -15 m.
Finally, to anonymize the source of current velocities, the final spatiotemporal resolution was agreed with the data provider as with the temperature and wind datasets. Thus, currents inside a 4.5° x 4.5° grid cell were monthly averaged and data were provided at the center of each cell.
After the data acquisition, QC, processing and validation, the bulk of the datasets were almost ready to be shared. However, they had to be correctly documented and standardized to adhere to the FAIR principles, as much as possible. The tables in this section show the metadata describing the variables (Table 5) and the global attributes (Table 6) included within the three datasets, thus providing a rich description of the data.
The proposed global attributes followed the standards and conventions of the ‘NetCDF CF Metadata Convention Standard Name Table Version 1.6’ (https://cfconventions.org/cf-conventions/v1.6.0/cf-conventions.html). They also followed the ISO 19115 for geographical information and ISO 19139 for XML implementation. All these standards were indicated in the metadata in the ‘standard_name_vocabulary’ global attribute. Regarding the vocabulary for naming the variables, the SeaDataNet standard (https://www.seadatanet.org/Standards/Common-Vocabularies) was adopted, also specified in the ‘standard_name_vocabulary’ global attribute. By adhering to commonly used standards and conventions within the oceanographic community, the datasets achieved increased Interoperability and Reusability and they also enhanced Findability. Additionally, certain global attributes, including the ‘distribution_statement’ specifying data usage conditions and the ‘acknowledgement’, ‘comment’ and ‘summary’ attributes detailing data provenance, further contributed to the Reusability of the data. Note that looking at the standards and attributes used in already published similar datasets was also helpful for structuring the data and metadata.
In addition to the information provided in the metadata, specific PDDs were generated for each dataset comprehensively describing the data lifecycle. These documents thoroughly outline QC, processing and validation steps, providing further description of the dataset provenance and thereby contributing to the Interoperability and Reusability while facilitating the reproducibility of the FAIR dataset generation.
Upon completing the documentation step, both data and metadata were saved as a unique NetCDF (.nc) file in each case. The NetCDF format and version were already specified in the ‘NetCDF_format’ and ‘NetCDF_version’ global attribute metadata. Then, the datasets were ready to be shared within the marine community and they were published in an ERDDAP server (https://erddap.sustuntech.eu:3030/erddap/info/index.html?page=1&itemsPerPage=1000). Although a personal ERDDAP server may not be recognized as a well-known data publisher for the community, it might be a convenient place for easily maintaining the data. From this server, well-known data repositories or aggregators can retrieve and subsequently publish the data. At this stage, the datasets were Accessible by the data access protocol of the ERDDAP. This server also contributes to the Interoperability by allowing the conversion of datasets into various interoperable file formats. Furthermore, the ERDDAP offers the capability to visualize the global attributes metadata of each dataset, thereby enhancing both the Interoperability and Reusability. Leveraging the easy retrieval from personal ERDDAPs into well-known data repositories or aggregators, the three datasets were ingested into the ERDDAP of the EMODnet Physics data aggregator (https://erddap.emodnet-physics.eu/erddap/search/index.html?page=1&itemsPerPage=1000&searchFor=Sustuntech).
Before the ingestion in the EMODnet Physics ERDDAP, the datasets, along with their associated PDDs, were also published through Zenodo, where a DOI was assigned (https://zenodo.org/records/10677365), contributing to the Findability. The location of the datasets in the mentioned ERDDAP servers was also displayed in Zenodo. Concerning the licensing of the datasets, the ‘Creative Commons Attribution-NonCommercial-NoDerivs 4.0 International License’ was applied, allowing their usage and sharing for non-commercial purposes as agreed with the data provider. This license protects the data and the provider while making the datasets open to the oceanographic community. Note that in this article the links to the PPDs are provided instead of using Supplementary Material, as their publication in Zenodo is part of the data lifecycle of the presented case studies.
Finally, the FAIRness of the datasets taken from the ERDDAPs was assessed through the checker mentioned in Section 2.4 (https://fair-checker.france-bioinformatique.fr/check) obtaining high FAIRness percentages of 91.67% for the three datasets.
This article aims to provide comprehensive guidance for creating highly FAIR datasets from fishing vessel met-ocean observations encouraging, supporting, and facilitating met-ocean data sharing within the marine community. First, the FAIR principles are defined and contextualized and then the necessary steps are illustrated through the use of three case studies. Despite the proposed steps, alternative approaches that best suit each case can be followed. Regardless of how the data is shared with the community, in near real-time (for instance by the GTS system) or in delayed mode (as in the cases shown in this article), it is crucial to thoroughly document the data provenance in the metadata along with the highest amount of information related to the dataset adhering, as much as possible, to the community standards and conventions (Van Vranken et al., 2023). The provided guidelines could also serve for data collected by other observing platforms or even for dataset creators within other communities.
Even if a dataset is no longer available, it is essential to retain the metadata as they need much lower maintenance costs and continue to serve as a reference for the dataset. This practice prevents users from perpetually searching for a dataset that no longer exists. The use of persistent identifiers is also key for the long-term maintenance of the dataset. Therefore, the created datasets should be published in data aggregators or repositories that ensure the highest degree of permanence possible. As long as a high degree of FAIRness is achieved, the submission of data into trustworthy digital repositories is preferable. These repositories should align with the TRUST principles (Lin et al., 2020), which guide digital data repositories towards creating and maintaining infrastructures that ensure continuous and long-term data management (L’Hours et al., 2019). In this context, the CoreTrustSeal certificate (https://www.coretrustseal.org/), launched in 2017, stands as the most recognized certificate to guarantee the quality and trustworthiness of repositories in the long term (L’Hours et al., 2019). In the same line, ISO/DIS 16363 (under development; previously: ISO 16363:2012) is presented as an auditor and certifier of trustworthy digital repositories. Although not all the widely used data repositories or aggregators possess this certificate within the oceanographic community, their use is encouraged whenever possible.
If QC, processing and validation of the raw data are performed, the dataset will probably be more appealing and reach more users. Moreover, the uncertainty estimates derived from these steps are needed to define the variables as EOV/ECVs (Lindstrom et al., 2012). However, although these steps are recommended (as performed in the three case studies shown in this article), they are not mandatory to adhere to the FAIR principles as long as the data provenance is comprehensively documented in the metadata and the pertinent standards and the DOI are used (https://www.go-fair.org/fair-principles/r1-metadata-richly-described-plurality-accurate-relevant-attributes/). The user needs to have enough information about the dataset to decide whether it is useful or not regardless of the state of the data. More information implies a better decision about its utility, thus enhancing proper Reusability. In case the data undergo QC and/or processing, widely used standards and procedures are also encouraged to increase the Reusability. Even if it is performed by the dataset creator or the user, the validation of the data also provides insights into the quality of the dataset, which should be considered for scientific studies (e.g. Bocca et al., 2011; Olofsson et al., 2013; Martinelli et al., 2016; Diky et al., 2019). The fair performance shown during the gross validations of the presented three datasets further indicates the potential of met-ocean datasets collected by fishing vessels for complementing observational gaps within the vast ocean (Van Vranken et al., 2020, 2023). Although not done in the examples provided in this article due to a lack of anticipation and means, and considered an optional exercise because of its complexity, planning and setting up optimal validation configurations against high-resolution datasets is preferable. This approach yields more accurate information about the quality of the final datasets (e.g. Santos et al., 2024).
While prioritizing open data is desirable, it may not always be possible, especially when dealing with sensitive or commercial data from companies or industry. In such cases, finding an intermediate solution agreed with the data provider, such as anonymizing the data, becomes preferable to secure their consent while maintaining the significance of the created dataset (Smith et al., 2019). As stated in the Introduction, the reluctance of fisheries to publicly share their data for commercial reasons remains a significant barrier to fully exploiting the met-ocean data they collect (Yochum et al., 2011; Van Vranken et al., 2023). During the data lifecycle presented in this article and within the documentation of the created datasets the data provider has been anonymized as much as possible. In addition, the information linked to vessel positions has been removed by creating averaged, merged and gridded datasets. Furthermore, despite the open availability of the datasets, they are only licensed for non-commercial purposes, further protecting the data provider while keeping data open to the oceanographic community. Implementing anonymization techniques and restrictive data access or licensing can encourage and promote the data sharing of fisheries. Consequently, this could foster closer collaboration between oceanographic and fisheries communities, which in the end leads to a better understanding of the marine environment and better decision-making in favor of both communities. Although they are out of the scope of this article, note that CARE principles (https://www.gida-global.org/care; Carroll et al., 2020), which aim to preserve the right to create value from Indigenous data for collective benefit, should be considered whenever they apply.
Despite the three datasets presented in this article being successfully published, the process posed several challenges and also provided valuable lessons. Firstly, when the met-ocean data were selected, a fourth dataset was discarded. These data comprised subsurface currents measured by onboard ADCPs, which are widely used sensors by fisheries. Along with the other three variables presented in this article, the data collected by ADCPs can greatly contribute to the oceanographic community as it comprises an EOV which is scarcely collected in the vast ocean. The potential addition of the ADCPs further showcases the potential of fishing vessels for the collection of relevant met-ocean data. However, the data from the ADCPs were excluded due to the limited information about the sensor and its configuration at the time of installation on the vessel, as well as the lack of attributes of the collected data. Therefore, the information obtained from this sensor was insufficient for extracting any dataset, even at its lowest raw quality level. This fact emphasized the importance of planning and documenting from the beginning the sensor data flow configuration so that all the information needed for extracting minimally valuable data and metadata is available.
After acquisition, QC, processing and validation steps, data had to be properly documented to adhere to the FAIR principles, as much as possible. At that point, the next challenge was to comprehensively understand FAIR principles and how to implement them, requiring a thorough review and consultation with experts. During the documentation of the datasets, the identification and search of the most appropriate standards and conventions for both format and vocabulary were another struggle. Concerning data sharing, it is worth highlighting that the setup of a personal ERDDAP server might be complicated depending on the computing capacity of the work team, available facilities and the available resources to maintain it. Nevertheless, this kind of data server can be beneficial as it enables direct management of the datasets and the subsequent ingestion into other ERDDAPs or data publishers. In case the setting up of an ERDDAP is not feasible, existing ones could shelter met-ocean data freely (e.g. EMODnet Physics ERDDAP). All in all, given the substantial volume of data daily collected by fishing vessels and its potential for the marine community, this article intends to fill the absence (to the author’s knowledge) of clear step-by-step guidance on creating fishing vessel-based met-ocean FAIR datasets as this might be a complex task.
The references and links to the used and created datasets are shown throughout Section 3. However, they are again listed here. The created datasets: Own ERDDAP: https://erddap.sustuntech.eu:3030/erddap/info/index.html?page=1&itemsPerPage=1000; EMODnet ERDDAP: https://erddap.emodnet-physics.eu/erddap/search/index.html?page=1&itemsPerPage=1000&searchFor=Sustuntech; Zenodo (also contains the PDDs): https://zenodo.org/records/10677365. The datasets used in the validations: SST data: SST_GLO_SST_L4_REP_OBSERVATIONS_010_011 product from CMEMS (Good et al., 2020; https://doi.org/10.48670/moi-00168); Wind data: WIND_GLO_PHY_L4_NRT_012_004 product of CMEMS (https://doi.org/10.48670/moi-00305) and the ERA5 dataset (Hersbach et al., 2023; https://doi.org/10.24381/cds.adbb2d47); Currents: NOAA Global Drifter Program data drogued at -15 m (Lumpkin and Centurioni, 2019; https://www.aoml.noaa.gov/phod/gdp/interpolated/data/all.php).
IM-N: Conceptualization, Data curation, Formal analysis, Investigation, Writing – original draft, Writing – review & editing. LS: Conceptualization, Data curation, Investigation, Writing – original draft, Writing – review & editing. AC: Conceptualization, Data curation, Investigation, Project administration, Writing – original draft, Writing – review & editing. AA: Data curation, Writing – review & editing. CK: Writing – review & editing. CD: Writing – review & editing. JF: Conceptualization, Funding acquisition, Investigation, Project administration, Writing – original draft, Writing – review & editing.
The author(s) declare financial support was received for the research, authorship, and/or publication of this article. This work has received funding from the European Union’s Horizon 2020 research and innovation program under grant agreement No. 869342 (SusTunTech). Funding was also provided by #ebegi project, funded by the Fisheries and Aquaculture Direction of the Economic Development, Sustainability, and Environment Department of the Basque Government.
We would like to thank the collaborating fishing vessels that contributed to the collection and sharing of data. We also want to thank the Marine Instruments (https://www.marineinstruments.es/) team that set up the ERDDAP server and facilitated the subsequent ingestion of the datasets. This research was partially carried out under the framework of e-begi project. This study has been conducted using EU Copernicus Marine Service information. ERA5 data was also downloaded from the Copernicus Climate Change Service. Data presented in this publication were made available by the EMODnet Ingestion project, https://www.emodnet-ingestion.eu/, funded by the European Commission Directorate General for Maritime Affairs and Fisheries. We would also like to thank the Atlantic Oceanographic and Meteorological Laboratory’s drifting buoy group for making their drifter data available. This is manuscript number 1248 from AZTI’s Marine Research Division, Basque Research and Technology Alliance (BRTA).
The authors declare that the research was conducted in the absence of any commercial or financial relationships that could be construed as a potential conflict of interest.
All claims expressed in this article are solely those of the authors and do not necessarily represent those of their affiliated organizations, or those of the publisher, the editors and the reviewers. Any product that may be evaluated in this article, or claim that may be made by its manufacturer, is not guaranteed or endorsed by the publisher.
Baidai Y., Capello M., Billet N., Floch L., Simier M., Sabarros P., et al. (2017). Towards the derivation of fisheries-independent abundance indices for tropical tunas: Progress in the echosounders buoys data analysis. IOTC-2017-WPTT19-22 Rev 1. (accessed November 26, 2024).
Bailey K., Steinberg C., Davies C., Galibert G., Hidas M., McManus M. A., et al. (2019). Coastal mooring observing networks and their data products: recommendations for the next decade. Front. Mar. Sci. 6. doi: 10.3389/fmars.2019.00180
Baudron A. R., Brunel T., Blanchet M., Hidalgo M., Chust G., Brown E. J., et al. (2020). Changing fish distributions challenge the effective management of European fisheries. Ecogr. (Cop.). 43, 494–505. doi: 10.1111/ecog.04864
Bocca B., Mattei D., Pino A., Alimonti A. (2011). Monitoring of environmental metals in human blood: The need for data validation. Curr. Anal. Chem. 7, 269–276. doi: 10.2174/157341111797183119
Bradley D., Merrifield M., Miller K. M., Lomonico S., Wilson J. R., Gleason G. (2019). Opportunities to improve fisheries management through innovative technology and advanced data systems. Fish Fish. 20, 564–583. doi: 10.1111/faf.12361
Buck J. J. H., Bainbridge S. J., Burger E. F., Kraberg A. C., Casari M., Casey K. S., et al. (2019). Ocean data product integration through innovation-the next level of data interoperability. Front. Mar. Sci. 6, 32. doi: 10.3389/fmars.2019.00032
Bushnell M., Worthington H. (2017). Manual for real-time quality control of wind data : a guide to quality control and quality assurance for coastal and oceanic wind observations. doi: 10.7289/V5FX77NH
Bushnell M., Worthington H. (2020). Manual for real-time quality control of in-situ temperature and salinity data : a guide to quality control and quality assurance for in-situ temperature and salinity observations. doi: 10.25923/x02m-m555
Carroll S., Garba I., Figueroa-Rodríguez O., Holbrook J., Lovett R., Materechera S., et al. (2020). The CARE principles for indigenous data governance. Data Sci. J. 19, 1–12. doi: 10.5334/dsj-2020-043
Chung H., Lee J., Lee W. Y. (2021). A review: Marine bio-logging of animal behaviour and ocean environments. Ocean Sci. J. 56, 117–131. doi: 10.1007/s12601-021-00015-1
De Mey-Frémaux P., Ayoub N., Barth A., Brewin R., Charria G., Campuzano F., et al. (2019). Model-observations synergy in the coastal ocean. Front. Mar. Sci. 6. doi: 10.3389/fmars.2019.00436
Diky V., Bazyleva A., Paulechka E., Magee J. W., Martinez V., Riccardi D., et al. (2019). Validation of thermophysical data for scientific and engineering applications. J. Chem. Thermodyn. 133, 208–222. doi: 10.1016/j.jct.2019.01.029
Duchêne J., Leblond E., Quéméner L., Charria G. (2023). Bilan du projet RECOPESCA. Available online at: https://archimer.ifremer.fr/doc/00858/97035/ (accessed November 25, 2024).
Dunning A., De Smaele M., Böhmer J. (2017). Are the FAIR data principles fair? Int. J. Digit. Curation 12, 177–195. doi: 10.2218/ijdc.v12i2.567
Erauskin-Extramiana M., Chust G., Arrizabalaga H., Cheung W. W. L., Santiago J., Merino G., et al. (2023). Implications for the global tuna fishing industry of climate change-driven alterations in productivity and body sizes. Glob. Planet. Change 222, 104055. doi: 10.1016/j.gloplacha.2023.104055
Falco P., Belardinelli A., Santojanni A., Cingolani N., Russo A., Arneri E. (2007). An observing system for the collection of fishery and oceanographic data. Ocean Sci. 3, 189–203. doi: 10.5194/os-3-189-2007
Fedak M. A. (2013). The impact of animal platforms on polar ocean observation. Deep Sea Res. Part II Top. Stud. Oceanogr. 88, 7–13. doi: 10.1016/j.dsr2.2012.07.007
Gaignard A., Rosnet T., De Lamotte F., Lefort V., Devignes M.-D. (2023). FAIR-Checker: supporting digital resource findability and reuse with Knowledge Graphs and Semantic Web standards. J. Biomed. Semantics 14, 7. doi: 10.1186/s13326-023-00289-5
Gallo N. D., Bowlin N. M., Thompson A. R., Satterthwaite E. V., Brady B., Semmens B. X. (2022). Fisheries surveys are essential ocean observing programs in a time of global change: a synthesis of oceanographic and ecological data from US West Coast Fisheries Surveys. Front. Mar. Sci. 9. doi: 10.3389/fmars.2022.757124
Gawarkiewicz G., Malek Mercer A. (2019). Partnering with fishing fleets to monitor ocean conditions. Ann. Rev. Mar. Sci. 11, 391–411. doi: 10.1146/annurev-marine-010318-095201
Goikoetxea N., Goienetxea I., Fernandes-Salvador J. A., Goñi N., Granado I., Quincoces I., et al. (2024). Machine-learning aiding sustainable Indian Ocean tuna purse seine fishery. Ecol. Inform. 81, 102577. doi: 10.1016/j.ecoinf.2024.102577
Goni G., Roemmich D., Molinari R., Meyers G., Sun C., Boyer T., et al. (2010). “The ship of opportunity program,” in Proceedings of oceanObs: Sustained Ocean Observations and Information for Society. Eds. Hall J., Harrison D. E., Stammer D. (ESA Publications, Auckland), 366–383. Available at: http://www.oceanobs09.net/proceedings/cwp/Goni-OceanObs09.cwp.35.pdf.
Good S., Fiedler E., Mao C., Martin M. J., Maycock A., Reid R., et al. (2020). The current configuration of the OSTIA system for operational production of foundation sea surface temperature and ice concentration analyses. Remote Sens. 12, 720. doi: 10.3390/rs12040720
Granado I., Hernando L., Galparsoro I., Gabina G., Groba C., Prellezo R., et al. (2021). Towards a framework for fishing route optimization decision support systems: Review of the state-of-the-art and challenges. J. Clean. Prod. 320, 128661. doi: 10.1016/j.jclepro.2021.128661
Groom S., Sathyendranath S., Ban Y., Bernard S., Brewin R., Brotas V., et al. (2019). Satellite ocean colour: current status and future perspective. Front. Mar. Sci. 6. doi: 10.3389/fmars.2019.00485
Hankin S., Bermudez L., Blower J. D., Blumenthal B., Casey K. S., Fornwall M., et al. (2010). Data management for the ocean sciences—perspectives for the next decade. Proceedings Ocean. 9. Available at: https://www.researchgate.net/publication/228552730_Data_Management_for_the_Ocean_Sciences_-_Perspectives_for_the_Next_Decade.
Hansen D. V., Herman A. (1989). Temporal sampling requirements for surface drifting buoys in the tropical Pacific. J. Atmos. Ocean. Technol. 6, 599–607. doi: 10.1175/1520-0426(1989)006%3C0599:TSRFSD%3E2.0.CO;2
Hansen D. V., Poulain P.-M. (1996). Quality control and interpolations of WOCE-TOGA drifter data. J. Atmos. Ocean. Technol. 13, 900–909. doi: 10.1175/1520-0426(1996)013%3C0900:QCAIOW%3E2.0.CO;2
Hersbach H., Bell B., Berrisford P., Biavati G., Horányi A., Muñoz Sabater J., et al. (2023). ERA5 monthly averaged data on single levels from 1940 to present. doi: 10.24381/cds.adbb2d47. (accessed November 26, 2024)
Imzilen T., Chassot E., Barde J., Demarcq H., Maufroy A., Roa-Pascuali L., et al. (2019). Fish aggregating devices drift like oceanographic drifters in the near-surface currents of the Atlantic and Indian Oceans. Prog. Oceanogr. 171, 108–127. doi: 10.1016/j.pocean.2018.11.007
Jakoboski J., Roughan M., Radford J., de Souza J. M. A. C., Felsing M., Smith R., et al. (2024). Partnering with the commercial fishing sector and Aotearoa New Zealand’s ocean community to develop a nationwide subsurface temperature monitoring program. Prog. Oceanogr. 225, 103278. doi: 10.1016/j.pocean.2024.103278
Kent E., Ball G., Berry I. D., Fletcher J., Hall A., North S., et al. (2010). “The Voluntary Observing Ship(VOS) Scheme,” in Proceedings of the “OceanObs’09: Sustained ocean observations and information for society”. Eds. Hall J., Harrison D. E., Stammer D. (ESA Publication, Venice), 551–561. Available at: http://www.oceanobs09.net/proceedings/cwp/Kent-OceanObs09.cwp.48.pdf.
L’Hours H., Kleemola M., de Leeuw L. (2019). CoreTrustSeal: From academic collaboration to sustainable services. IASSIST Q. 43, 1–17. doi: 10.29173/iq936
Labastida I., Margoni T. (2020). Licensing FAIR data for reuse. Data Intell. 2, 199–207. doi: 10.1162/dint_a_00042
Lamouroux J., Charria G., De Mey P., Raynaud S., Heyraud C., Craneguy P., et al. (2016). Objective assessment of the contribution of the RECOPESCA network to the monitoring of 3D coastal ocean variables in the Bay of Biscay and the English Channel. Ocean Dyn. 66, 567–588. doi: 10.1007/s10236-016-0938-y
Leblond E., Lazure P., Laurans M., Rioual C., Woerther P., Quemener L., et al. (2010). The Recopesca Project: a new example of participative approach to collect fisheries and in situ environmental data. Mercat. Ocean. Newsl. 37, 40–48. Available at: https://archimer.ifremer.fr/doc/00024/13500/.
Le Traon P. Y., Reppucci A., Alvarez Fanjul E., Aouf L., Behrens A., Belmonte M., et al. (2019). From observation to information and users: The Copernicus Marine Service perspective. Front. Mar. Sci. 6. doi: 10.3389/fmars.2019.00234
Lin D., Crabtree J., Dillo I., Downs R. R., Edmunds R., Giaretta D., et al. (2020). The TRUST Principles for digital repositories. Sci. Data 7, 1–5. doi: 10.1038/s41597-020-0486-7
Lindstrom E., Gunn J., Fischer A., McCurdy A., Glover L. K. (2012). A Framework for Ocean Observing. By the Task Team for an Integrated Framework for Sustained Ocean Observing (Paris: UNESCO). doi: 10.5270/OceanObs09-FOO
Loshin D. (2012). Business intelligence: the savvy manager’s guide. Ed. Loshin D. (San Francisco: Morgan Kaufmann). doi: 10.1016/C2010-0-67240-3
Lumpkin R., Centurioni L. (2019). Global Drifter Program quality-controlled 6-hour interpolated data from ocean surface drifting buoys. doi: 10.25921/7ntx-z961. (accessed November 26, 2024)
Lumpkin R., Özgökmen T., Centurioni L. (2017). Advances in the application of surface drifters. Ann. Rev. Mar. Sci. 9, 59–81. doi: 10.1146/annurev-marine-010816-060641
Lumpkin R., Pazos M. (2007). Measuring surface currents with Surface Velocity Program drifters: the instrument, its data, and some recent results. Lagrangian Anal. Predict. Coast. Ocean Dyn. 39, 67. doi: 10.1017/CBO9780511535901
Lutjeharms J. R. E. (2006). The Agulhas Current (Berlin: Springer Science & Business Media). Available at: https://books.google.es/books?id=BRVGAAAAQBAJ.
March D., Boehme L., Tintoré J., Vélez-Belchi P. J., Godley B. J. (2020). Towards the integration of animal-borne instruments into global ocean observing systems. Glob. Change Biol. 26, 586–596. doi: 10.1111/gcb.14902
Margoni T., Tsiavos P. (2018). Toolkit for Researchers on Legal Issues. Zenodo. doi: 10.5281/zenodo.2574619
Martinelli M., Guicciardi S., Penna P., Belardinelli A., Croci C., Domenichetti F., et al. (2016). Evaluation of the oceanographic measurement accuracy of different commercial sensors to be used on fishing gears. Ocean Eng. 111, 22–33. doi: 10.1016/j.oceaneng.2015.10.037
Medina J., Ziaullah A. W., Park H., Castelli I. E., Shaon A., Bensmail H., et al. (2022). Accelerating the adoption of research data management strategies. Matter 5, 3614–3642. doi: 10.1016/j.matt.2022.10.007
Moltmann T., Turton J., Zhang H.-M., Nolan G., Gouldman C., Griesbauer L., et al. (2019). A global ocean observing system (GOOS), delivered through enhanced collaboration across regions, communities, and new technologies. Front. Mar. Sci. 6. doi: 10.3389/fmars.2019.00291
Mons B., Neylon C., Velterop J., Dumontier M., da Silva Santos L. O. B., Wilkinson M. D. (2017). Cloudy, increasingly FAIR; revisiting the FAIR Data guiding principles for the European Open Science Cloud. Inf. Serv. Use 37, 49–56. doi: 10.3233/ISU-170824
Murua J., Itano D., Hall M., Dagorn L., Moreno G., Restrepo V. (2016). Advances in the use of entanglement-reducing Drifting Fish Aggregating Devices (DFADs) in tuna purse seine fleets (Washington, D.C., USA: International Seafood Sustainability Foundation). Available at: https://www.bmis-bycatch.org/system/files/zotero_attachments/library_1/QJ3GAD9E-ISSF-2016-08-Advances-in-the-Use-of-Entanglement-Reducing-Drifting-Fish-Aggregating-Devices-in-Tuna-Purse-Seiners.pdf. ISSF Technical Report 2016-08.
Niiler P. P., Sybrandy A. S., Bi K., Poulain P. M., Bitterman D. (1995). Measurements of the water-following capability of holey-sock and TRISTAR drifters. Deep Sea Res. Part I Oceanogr. Res. Pap. 42, 1951–1964. doi: 10.1016/0967-0637(95)00076-3
O’Carroll A. G., Armstrong E. M., Beggs H. M., Bouali M., Casey K. S., Corlett G. K., et al. (2019). Observational needs of sea surface temperature. Front. Mar. Sci. 6. doi: 10.3389/fmars.2019.00420
Olofsson P., Foody G. M., Stehman S. V., Woodcock C. E. (2013). Making better use of accuracy data in land change studies: Estimating accuracy and area and quantifying uncertainty using stratified estimation. Remote Sens. Environ. 129, 122–131. doi: 10.1016/j.rse.2012.10.031
Paduan J. D., Washburn L. (2013). High-frequency radar observations of ocean surface currents. Ann. Rev. Mar. Sci. 5, 115–136. doi: 10.1146/annurev-marine-121211-172315
Patti B., Martinelli M., Aronica S., Belardinelli A., Penna P., Bonanno A., et al. (2016). The Fishery and Oceanography Observing System (FOOS): a tool for oceanography and fisheries science. J. Oper. Oceanogr. 9, s99–s118. doi: 10.1080/1755876X.2015.1120961
Penna P., Domenichetti F., Belardinelli A., Martinelli M. (2023). Dataset of depth and temperature profiles obtained from 2012 to 2020 using commercial fishing vessels of the AdriFOOS fleet in the Adriatic Sea. Earth Syst. Sci. Data 15, 3513–3527. doi: 10.5194/essd-15-3513-2023
Poulain P.-M., Menna M., Mauri E. (2012). Surface geostrophic circulation of the Mediterranean Sea derived from drifter and satellite altimeter data. J. Phys. Oceanogr. 42, 973–990. doi: 10.1175/JPO-D-11-0159.1
Pouliquen S., Hankin S., Keeley R., Blower J., Donlon C., Kozyr A., et al. (2010). “The development of the data system and growth in data sharing,” in OceanObs’ 09: Sustained Ocean Observations and Information for Society, vol. 2. (Venice, Italy: ESA Publications). Available at: https://archimer.ifremer.fr/doc/00029/14041/11234.pdf.
Roarty H., Cook T., Hazard L., George D., Harlan J., Cosoli S., et al. (2019). The global high frequency radar network. Front. Mar. Sci. 6. doi: 10.3389/fmars.2019.00164
Rubio I., Hobday A. J., Ojea E. (2022). Skippers’ preferred adaptation and transformation responses to catch declines in a large-scale tuna fishery. ICES J. Mar. Sci. 79, 532–539. doi: 10.1093/icesjms/fsab065
Rubio A., Mader J., Corgnati L., Mantovani C., Griffa A., Novellino A., et al. (2017). HF radar activity in European coastal seas: next steps toward a pan-European HF radar network. Front. Mar. Sci. 4. doi: 10.3389/fmars.2017.00008
Rudnick D. L. (2016). Ocean research enabled by underwater gliders. Ann. Rev. Mar. Sci. 8, 519–541. doi: 10.1146/annurev-marine-122414-033913
Santos F. P., Rosa T. L., Hinostroza M. A., Vettor R., Piecho-Santos A. M., Guedes Soares C. (2024). Field test of an autonomous observing system prototype for measuring oceanographic parameters from ships. Oceans 5 (1), 127–149. doi: 10.3390/oceans5010008
Smith S. R., Alory G., Andersson A., Asher W., Baker A., Berry D. I., et al. (2019). Ship-based contributions to global ocean, weather, and climate observing systems. Front. Mar. Sci. 6. doi: 10.3389/fmars.2019.00434
Snowden D., Belbeoch M., Burnett B., Carval T., Graybeal J., Habermann T., et al. (2010). “Metadata Management in global distributed ocean observing networks,” in Proceedings of OceanObs’09: Sustained Ocean Observations and Information for Society. Eds. Hall J., Harrison D. E., Stammer D. (Venice: ESA Publications), 969–978.
Stall S., Yarmey L., Cutcher-Gershenfeld J., Hanson B., Lehnert K., Nosek B., et al. (2019). Make scientific data FAIR. Nature 570, 27–29. doi: 10.1038/d41586-019-01720-7
Tanhua T., Pouliquen S., Hausman J., O’brien K., Bricher P., De Bruin T., et al. (2019). Ocean FAIR data services. Front. Mar. Sci. 6. doi: 10.3389/fmars.2019.00440
Testor P., De Young B., Rudnick D. L., Glenn S., Hayes D., Lee C. M., et al. (2019). OceanGliders: a component of the integrated GOOS. Front. Mar. Sci. 6. doi: 10.3389/fmars.2019.00422
Turpin V., Remy E., Le Traon P.-Y. (2016). How essential are Argo observations to constrain a global ocean data assimilation system? Ocean Sci. 12, 257–274. doi: 10.5194/os-12-257-2016
Uranga J., Arrizabalaga H., Boyra G., Hernandez M. C., Goni N., Arregui I., et al. (2017). Detecting the presence-absence of bluefin tuna by automated analysis of medium-range sonars on fishing vessels. PloS One 12, e0171382. doi: 10.1371/journal.pone.0171382
Uriondo Z., Fernandes-Salvador J. A., Reite K.-J., Quincoces I., Pazouki K. (2024). Toward digitalization of fishing vessels to achieve higher environmental and economic sustainability. ACS Environ. Au 4, 142–151. doi: 10.1021/acsenvironau.3c00013
Van Vranken C., Jakoboski J., Carroll J. W., Cusack C., Gorringe P., Hirose N., et al. (2023). Towards a global Fishing Vessel Ocean Observing Network (FVON): state of the art and future directions. Front. Mar. Sci. 10. doi: 10.3389/fmars.2023.1176814
Van Vranken C., Vastenhoud B. M. J., Manning J. P., Plet-Hansen K. S., Jakoboski J., Gorringe P., et al. (2020). Fishing gear as a data collection platform: opportunities to fill spatial and temporal gaps in operational Sub-surface observation networks. Front. Mar. Sci. 7. doi: 10.3389/fmars.2023.1176814
Venkatesan R., Ramesh K., Kishor A., Vedachalam N., Atmanand M. A. (2018). Best practices for the ocean moored observatories. Front. Mar. Sci. 5. doi: 10.3389/fmars.2018.00469
Vignudelli S., Kostianoy A. G., Cipollini P., Benveniste J. (2011). Coastal altimetry (Berlin: Springer Science & Business Media).
von Schuckmann K., Palmer M. D., Trenberth K. E., Cazenave A., Chambers D., Champollion N., et al. (2016). An imperative to monitor Earth’s energy imbalance. Nat. Clim. Change 6, 138–144. doi: 10.1038/nclimate2876
Wilkinson M. D., Dumontier M., Aalbersberg I., Appleton G., Axton M., Baak A., et al. (2016). The FAIR Guiding Principles for scientific data management and stewardship. Sci. Data 3, 1–9. doi: 10.1038/sdata.2016.18
Yochum N., Starr R. M., Wendt D. E. (2011). Utilizing fishermen knowledge and expertise: keys to success for collaborative fisheries research. Fisheries 36, 593–605. doi: 10.1080/03632415.2011.633467
Keywords: FAIR, fishing vessel, data repositories, marine observing platforms, met-ocean data
Citation: Manso-Narvarte I, Solabarrieta L, Caballero A, Anabitarte A, Knockaert C, Dhondt CAL and Fernandes-Salvador JA (2024) Fishing vessels as met-ocean data collection platforms: data lifecycle from acquisition to sharing. Front. Mar. Sci. 11:1467439. doi: 10.3389/fmars.2024.1467439
Received: 19 July 2024; Accepted: 14 November 2024;
Published: 20 December 2024.
Edited by:
Johannes Karstensen, Helmholtz Association of German Research Centres (HZ), GermanyReviewed by:
Cooper Hoffman Van Vranken, Ocean Data Network, United StatesCopyright © 2024 Manso-Narvarte, Solabarrieta, Caballero, Anabitarte, Knockaert, Dhondt and Fernandes-Salvador. This is an open-access article distributed under the terms of the Creative Commons Attribution License (CC BY). The use, distribution or reproduction in other forums is permitted, provided the original author(s) and the copyright owner(s) are credited and that the original publication in this journal is cited, in accordance with accepted academic practice. No use, distribution or reproduction is permitted which does not comply with these terms.
*Correspondence: Ivan Manso-Narvarte, aW1hbnNvQGF6dGkuZXM=
Disclaimer: All claims expressed in this article are solely those of the authors and do not necessarily represent those of their affiliated organizations, or those of the publisher, the editors and the reviewers. Any product that may be evaluated in this article or claim that may be made by its manufacturer is not guaranteed or endorsed by the publisher.
Research integrity at Frontiers
Learn more about the work of our research integrity team to safeguard the quality of each article we publish.