- 1Mercator-Ocean International, Research and Development Department, Toulouse, France
- 2Ifremer, Plouzané, France
Observing System Simulation Experiments (OSSEs) with the Mercator Ocean/Copernicus Marine global 1/12° data assimilation system have been carried out to compare and quantify the expected performance of two high resolution altimetry mission concepts envisioned for the long-term evolution (post-2032) of the Copernicus Sentinel-3 topography mission. The two mission concepts are a constellation of two wide-swath altimeters and a constellation of 12 nadir altimeters. These two configurations greatly improve ocean forecasting and monitoring capabilities. Compared to a constellation of three nadir altimeters (the present configuration), analysis and forecast errors are reduced by a factor of 2. Our results also show that a constellation of two wide-swath altimeters has better performance than a constellation of 12 nadirs. Compared to a constellation of 12 nadirs, the error of the Sea Surface Height (SSH) forecast of a two wide swath constellation is reduced by 14% overall. Improvements are also observed when analyzing surface currents and Lagrangian diagnostics. A constellation of two wide-swath altimeters thus seems to be a very promising concept for the long-term evolution of the Sentinel-3 topography mission.
1 Introduction
The Copernicus Marine Service implemented by Mercator Ocean International (MOi) provides operational, regular, and systematic reference information on physical, biogeochemical ocean and sea-ice states for the global ocean and European regional seas. More than 55,000 expert downstream services and users are connected to the service. The Copernicus Marine Service responds to public and private user needs and supports policies related to all marine and maritime sectors.
The Copernicus Marine Service depends on the upstream satellite and in-situ observation infrastructure. Sea Surface Height (SSH) measurements from satellite altimetry play a prominent role (see Le Traon et al., 2017) and the quality of Copernicus Marine analyses and forecasts are directly related to the status of the altimeter constellation. Copernicus Marine model present (e.g. 1/12° at the global scale and 1/36° at the regional scale) and future resolutions (e.g. 1/36° at the global scale and 1/108° at the regional scale) currently impose strong constraints on the altimeter constellation. The observation capabilities lag behind model resolutions. This calls for a much-improved space/time sampling of the ocean by satellite altimetry.
Wide-swath altimetry (WiSA) that will be demonstrated with the Surface Water Ocean Topography (SWOT) mission (Morrow et al., 2019; Benkiran et al., 2021; Tchonang et al., 2021) can address these needs. Benkiran et al. (2022) demonstrated, in particular, that a constellation of two wide-swath altimeters could dramatically improve the quality of analyses and forecasts. An alternative approach is to fly an optimized constellation of a large number (e.g. at least 10) of nadir altimeters. The goal of this paper is to evaluate the relative merits of those alternative that are considered for the Sentinel-3 Next Generation constellation. Updated observation error specification is used for the Wide Swath altimeter compared to the previous paper (Benkiran et al., 2022).
In this paper, we address the relative merits of these two approaches: a constellation of two wide-swath altimeters and a constellation of 12 nadir altimeters by running OSSEs with a global ocean 1/12° system. Results for this global study are presented and discussed in this paper, which is organized as follows: the WiSA concept is presented in Section 2; Section 3 details the OSSE design; results are discussed in Section 4, and Section 5 provides the main conclusions and recommendations of the study.
2 OSSE approach
2.1 The WiSA concept
The WiSA concept was developed as part of a Phase A study conducted by CNES (Centre National d’études Spatiales) and industry as an interim follow-up to the SWOT mission. The objective of the WiSA concept was to leverage the major improvement of the SWOT swath altimeter with significant modifications to better meet the needs of operational oceanography and hydrology, while making the satellite simpler, smaller, and more affordable than the SWOT precursor mission. The WiSA concept is well described in Benkiran et al. (2022).
The so-called WISA #A orbit was selected by CNES using the methodology of Dibarboure et al. (2018) to maximize the sampling for 1 to 3 swath altimeter satellites (or swath/nadir hybrid constellations). This sun-synchronous orbit has an altitude of approximately 750 km (14 + 7/17 revolutions per day) and the altimeter swath covers latitudes up to 82°, with an exact repeat cycle of 17 days.
2.2 Ocean model
In our study, we use the NEMO ocean model (Nucleus for European Modelling of the Ocean; Madec and NEMO System Team, 2015) for the Nature Run and the OSSEs but with different model configurations and atmospheric forcings. A free NEMO3.6 ocean model simulation at 1/12°forced by ERA-Interim reanalysis (Dee et al., 2011) with 3-h resolution is used as a so-called Nature Run, hereafter referred to as NatRun, to represent the real ocean and generate all the synthetic observations for the study. A detailed description and validation of the NatRun is presented in Benkiran et al. (2021). A second model configuration, which is embedded in the assimilation system used to assimilate synthetic observations from the NatRun, is based on a NEMO3.1 model configuration with different model parametrizations and atmospheric forcing. The major difference between the Nature Run and the OSSE model is the level of surface kinetic energy mainly due to the different wind/surface current coupling coefficient forcing parametrization used for each of the simulations, as mentioned in Benkiran et al. (2021). The NatRun uses absolute wind to force the surface current in the bulk parameterization of the turbulent momentum flux and a less diffusive scheme compared to the OSSE runs, which uses relative wind (50%). Those differences ensure a sufficient discrepancy between the simulated observations from the Nature Run and their forecasted values in the OSSEs, similar to the ones found in the system assimilating real data. This calibration phase (val/cal) of the OSSE, that follow the best practices for the OSSE design (Errico et al., 2013), is a necessary condition to ensure realistic results for the OSSEs.
2.3 Simulation of observations and noise
All pseudo-observations used in our OSSEs are simulated from the NatRun simulation over a period of 15 months (from October 1, 2014 to December 31, 2015). We simulated the high-resolution sea surface temperature (SST) maps at 1/10°, based on the ODYSSEA L3S SST (https://doi.org/10.48670/moi-00164) coverage, the temperature and salinity profiles based on the CORA database (https://doi.org/10.17882/46219) coverage, the ice concentration data and the Sea Surface Height (SSH). The aim is to simulate a realistic observation coverage compared to our present 1/12° operational system (see details in Benkiran et al., 2021). SSH data are distributed along the tracks of 3 nadirs, 12 nadirs and under the swath covered by 2 wide-swath altimeters (Swath1 and Swath2). SSH observations are directly simulated instead of SLA to avoid the definition of a Mean Dynamical Topography (MDT) that will have to be added to the pseudo-observations as it is done in the global 1/12° real time operational system.
The 3 nadir altimeters correspond to the existing baseline of the Sentinel operational altimeter constellation (Sentinel-6 (or Jason-3), Sentinel-3A, Sentinel-3B). A constellation of 12 nadir altimeters with SAR capabilities involves 12 altimeters in the same orbiting plane as the current Sentinel-3. The WiSA constellation consists of two wide-swath altimeters (S1 and S2) with their nadirs. The along-track nadir altimeter data were extracted from hourly mean SSH fields of the NatRun at 1 Hz frequency corresponding to a spatial resolution of 6-7 km. A random noise of 2 cm was added to the along-track data to take into account altimeter measurement noise. For the two-swath altimeters, the WiSA #A orbit (S1) selected by CNES (Dibarboure et al., 2018) was used together with a second (S2) on the same orbital plane, but separated by a 180° angle on the orbit circle. All SSH data were simulated from the NatRun using the Jet Propulsion Laboratory’s (JPL) SWOT Simulator (Gaultier et al., 2016). The simulator constructs a regular grid based on the baseline orbit parameters of the satellite. It produces random realizations of uncorrelated noise and correlated errors following the spectral descriptions of the SWOT error budget document (Esteban Fernandez et al., 2017). This simulation of observations is made from the hourly outputs of SSH from NatRun. We chose a resolution of 6km along and across swath. The simulator models the most significant errors that are expected to affect the data, i.e., the KaRIn (Ka-band Radar Interferometer) noise, roll errors, phase errors, baseline dilation errors, wet troposphere and timing errors.
Figure 1A shows the SSH from the NatRun at a given central date of our 7-day assimilation cycle (analysis window) over the Gulf Stream region. Data coverage along the tracks of the three nadir altimeters over the 7-day analysis window is shown in Figure 1B while Figure 1C shows the coverage of the combination 12 nadir altimeters and Sentinel-6, and Figure 1D of two Wide-Swath Altimeters and Sentinel-6. It can be observed that with two Wide-Swath Altimeters the ocean is almost totally covered by the measurements over a 7-day time period. While the number of SSH observations per analysis cycle (7 days) is very high for both experiments, the observations in the two Wide-Swath experiment are more than twice those of the 12nadir experiment. This indicates that a single swath altimeter can obtain more SSH observations (albeit correlated ones) than a constellation of the 12 nadir altimeters.
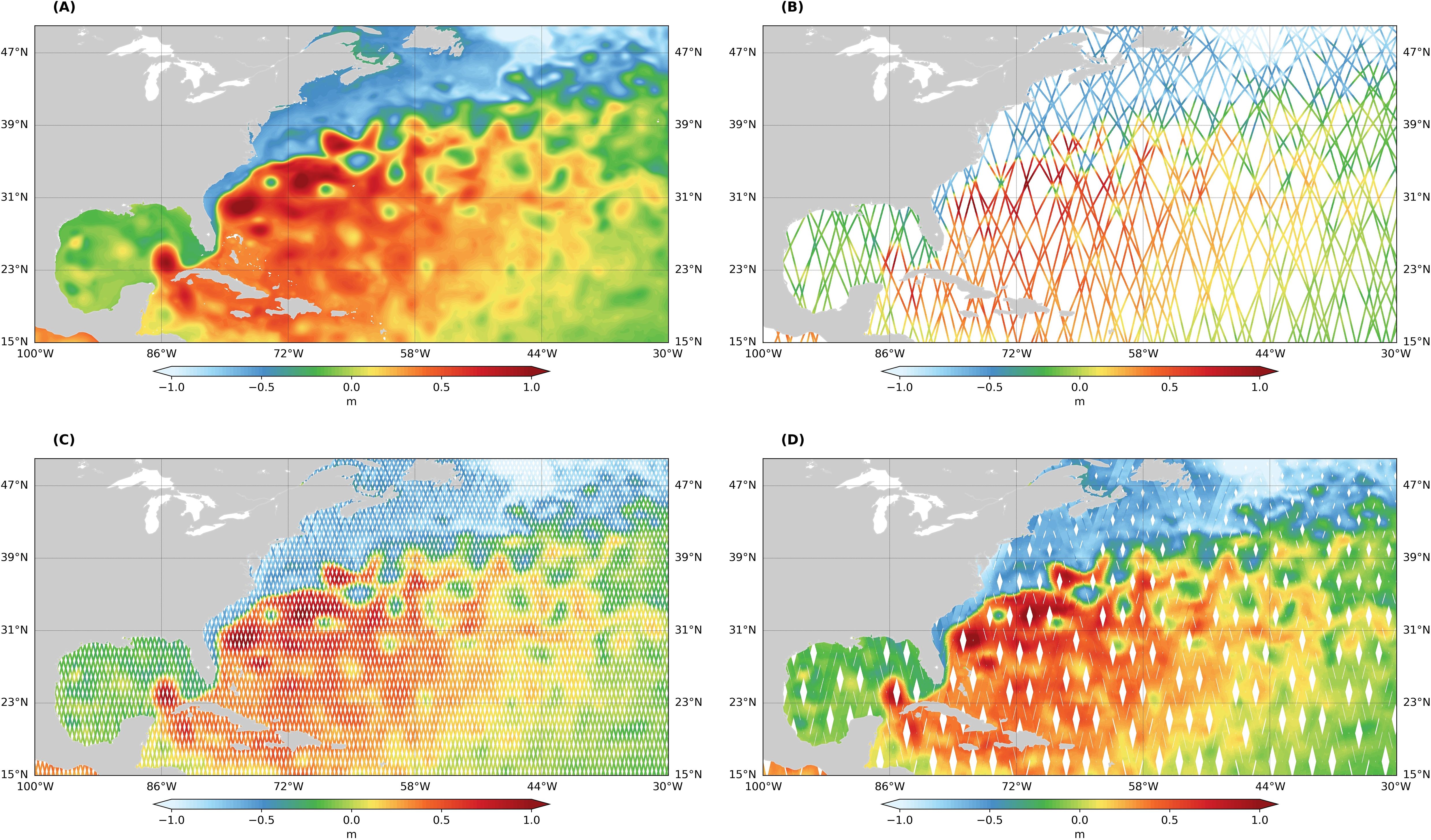
Figure 1. (A) SSH from NatRun on 4th January 2015, (B) simulated along-track data from Sentinel-6, Sentinel-3A and Sentinel-3B (3Nadirs) for seven-day assimilation cycle, (C) simulated along-track data from 12 nadir altimeters and Sentinel-6 (12Nadirs) in the same orbital plane as Sentinel-3 and (D) 2Wide-Swath and Sentinel-6 (2Swaths) data (01/01/2015-08/01/2015).
In this study, the KaRin error takes into account the calculated sea wave height (SWH), unlike the previous study (Benkiran et al., 2022) where the wave height was fixed at 2m. We use the error of the wet troposphere residual error after correction with 2 beams calculated by the simulator according to the predefined spectrum. For SSH observations, an error of 2cm (SAR mode) for nadir was prescribed. for WiSA the Karin noise is more important at the borders of the swath and increases with the SWH. Thus, it varies geographically and temporally. The wave model was also generated in conjunction with the main nature run which provided additional inputs to the SWOT Simulator (Gaultier et al., 2016). The SST error equal to 0.5°C was prescribed.
2.4 Data assimilation scheme
In this study, an updated version of MOi’s data assimilation scheme (SAM2: Système d’Assimilation Mercator V2) compared with the operational one descried in Lellouche et al. (2018) was used. This assimilation system is a hybrid between a 3D-Var bias correction for the slowly evolving large-scale biases in temperature and salinity, and a local version of a reduced-order Kalman filter based on the Singular Evolutive Extended Kalman Filter (SEEK)formulation introduced by Pham et al. (1998). It is the same system that is used done in the global 1/12° and regional 1/36° real time operational system and several previous OSSEs (Gasparin et al., 2018; Verrier et al., 2018; Hamon et al., 2019) including some related to Swath observations (Bonaduce et al., 2018), and for SWOT (Benkiran et al., 2021; Tchonang et al., 2021) used this system.
In this study, we use the same system as descried in previous studies (Benkiran et al., 2021). Our system uses 7 days for analysis cycle. The most important improvement is made on the adaptativity technique used in the analysis. This gives more weight to the assimilated data and helps to reduce more efficiently the observation residuals. In addition, a four-dimensional (4D) version of the assimilation scheme is used, in which the analysis uses a 4D subspace and produces a daily model correction of the SSH, temperature, salinity and velocity fields. These corrections are based on 7-day innovations (observations minus model forecasts), weighted by the distance in time from the analysis date. The analysis increment is inserted into the model using the Incremental Analysis Update (IAU) method (Bloom et al., 1996; Benkiran and Greiner, 2008). All these updates and their impact on system performance are described in Benkiran et al. (2021). These updates to the system allow us to better control the mesoscale structures and high temporal frequencies.
2.5 Experimental set-up
Three OSSEs were carried out assimilating the simulated observations with their associated errors. An additional experiment was performed, called the Free Run (FR), in which no observation is assimilated. This simulation is used to assess the performance of the assimilative experiments. The data assimilated in the different OSSEs are detailed in Table 1.
All those simulations start from a free model state on October 1, 2014. Then a three-month simulation (until the end of December 2014) is carried out with assimilation of SSH from the 3 nadir altimeters together with SST, IC and T&S data as in the 3Nadirs OSSE presented in Table 1. The final state of this simulation is used to initialize the different experiments presented in this paper. All experiments then start from the same state on January 1, 2015 and cover one year.
Analysis of the impact of assimilation of the SSH from the nadir altimeters (12Nadirs) and from the two wide-swath altimeters is detailed in this section. This analysis is based on comparison between each experiment and our real ocean (NatRun) data over a period of one year (2015).
We present the results as follows: first, the impact of the different altimeter constellations on the quality of the SSH analysis and prediction. We then show the impact on the space-time scales by analyzing the spectra (coherence, error spectrum) of the SSH in different regions (black boxes in Figure 2) with large variations. Scale separation has been done respecting the spectra of SSH error field to better define the cut-off of the scales (<200 and <500km here) on which the impact of the assimilation of swath data is greater compared to the Nadir data. We also show the impact on surface and interior velocities (model dynamics) and temperature and salinity profiles.
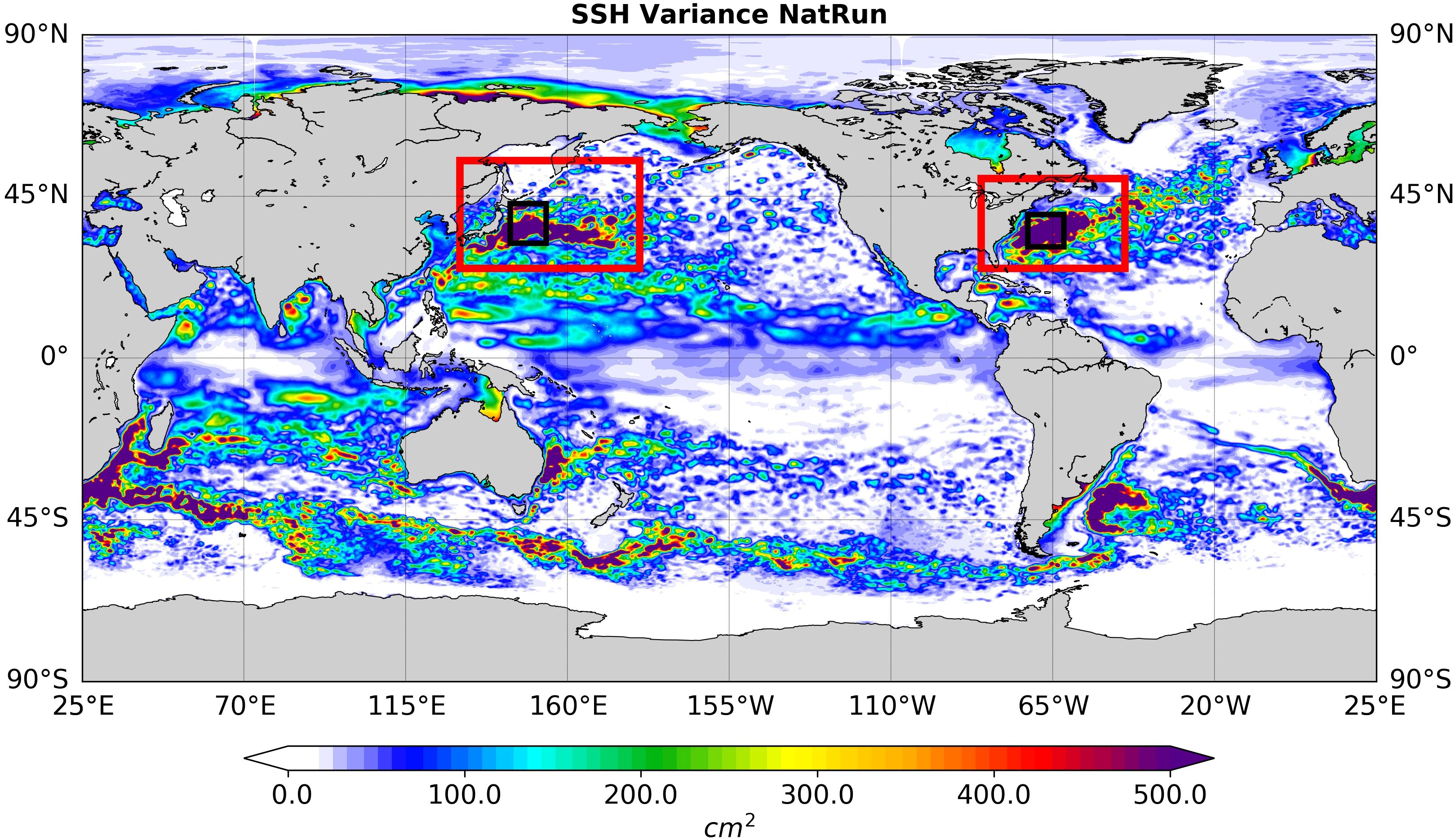
Figure 2. SSH variance (in cm2) in the NatRun over the period from February to December 2015. The red boxes denote the rectangular sub-regions for which statistics were calculated and the black boxes those for which wavenumber spectra and coherence analyses were performed.
3 Results
3.1 Impact on SSH analyses and forecasts
The aim of this study is to show the impact of assimilating SSH data from 2 wide-swath altimeters compared to a 12-nadir constellation in the same plane as Sentinel-3. The SSH variance in the NatRun computed over one year (2015) is shown in Figure 2. It shows a high variability in the more energetic regions such as the Gulf Stream (GS) and Kuroshio (KS) regions. The SSH variance in the NatRun compares very favorably with the estimation from real altimeter observations (as detailed in Benkiran et al., 2021).
The time series of SSH variance error over the global ocean for each experiment is shown in Figure 3. This variance of the error (VarError) is calculated by comparing each OSSE analysis or forecast fields with the NatRun as shown in Benkiran et al. (2022). The forecasts shown here are between 1 and 7 days, the initial state corresponds to the analysis which is done every 7 days (the duration of our assimilation cycle. This variance decreases over a few weeks (6 weeks) to reach a stable state for the analyses (continuous lines) and the forecasts (dotted lines). The 2Swaths experiment has an SSH analysis error variance of about 9.6 cm2 compared to the 12Nadirs experiment which has an error variance of 11.0 cm2. The gain is of about 14% for the analysis and 15% for the forecast (dotted lines). We summarize these overall statistics in Table 2. To highlight the impact on different ranges of spatial scales, we have calculated statistics on SSH errors with scale separation based on the spectra of these errors. We show a gain of about 13% for scales smaller than 200 km between the 12Nadirs and 2Swaths experiments.
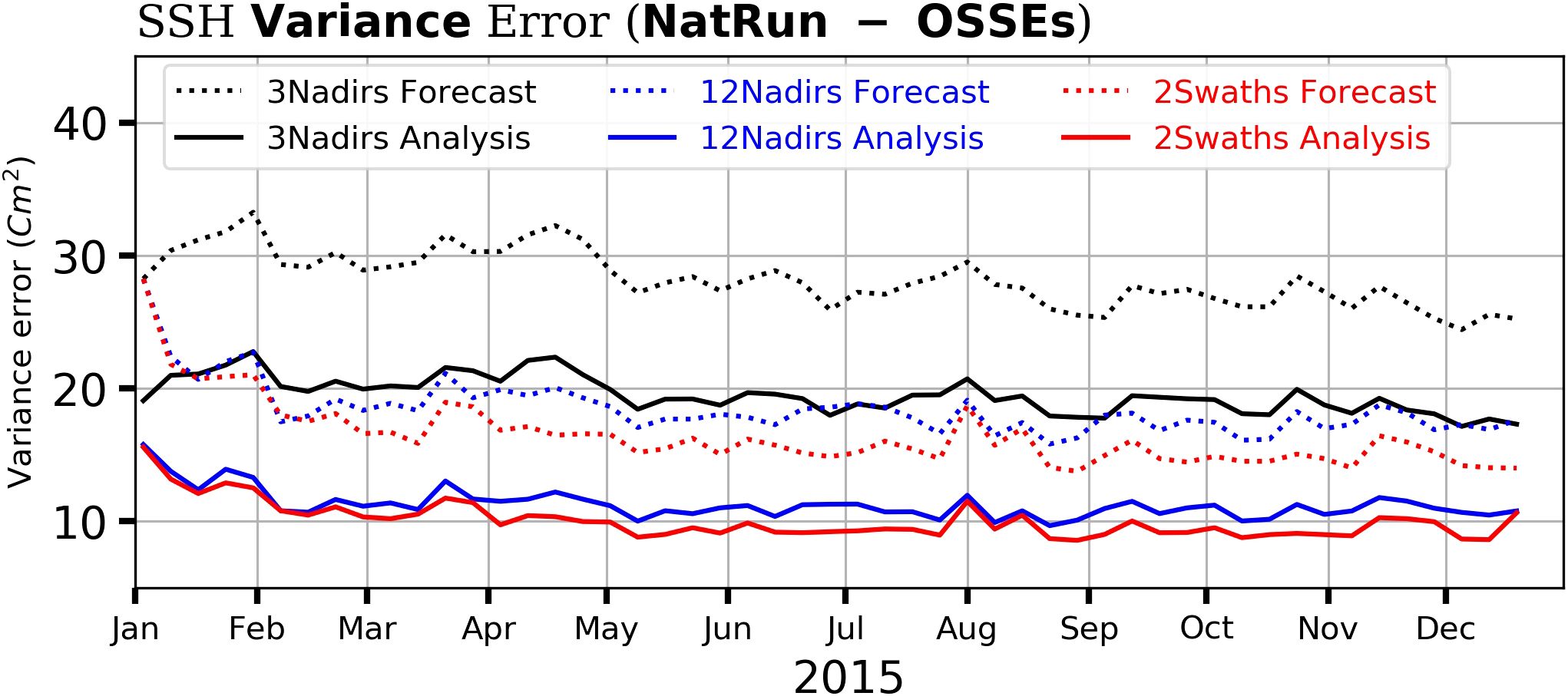
Figure 3. The temporal evolution of the SSH error variance in global ocean analysis and forecast over 2015. Results obtained by comparing the SSH ocean analysis (solid lines) and forecast (dash lines) with the SSH from the NatRun. Experiments 3Nadirs: black lines, 12Nadirs: blue lines and with 2Swaths: red lines (see Table 1 for descriptions of each experiment).
In Figure 4, we compare the SSH variance of each experiment with that of the 3Nadirs simulation. In the first figure, we have the global map of the error variance of the SSH analysis of the 3Nadirs run. In the middle figure, we have the difference between the SSH error variance of the 3Nadirs experiment and that of the 12Nadirs simulation. The red areas correspond to areas of improvement. We have a clear improvement with 12Nadirs in the western boundary currents and the Antarctic Circumpolar Current (ACC). Overall, 67% of the domain (calculated as a function of the improvement area) has been improved in the global ocean. Similarly, we have an additional improvement with the assimilation of wide swath data (2Swaths, bottom figure) compared with the 12Nadirs run (improvement for 75% of the global ocean).
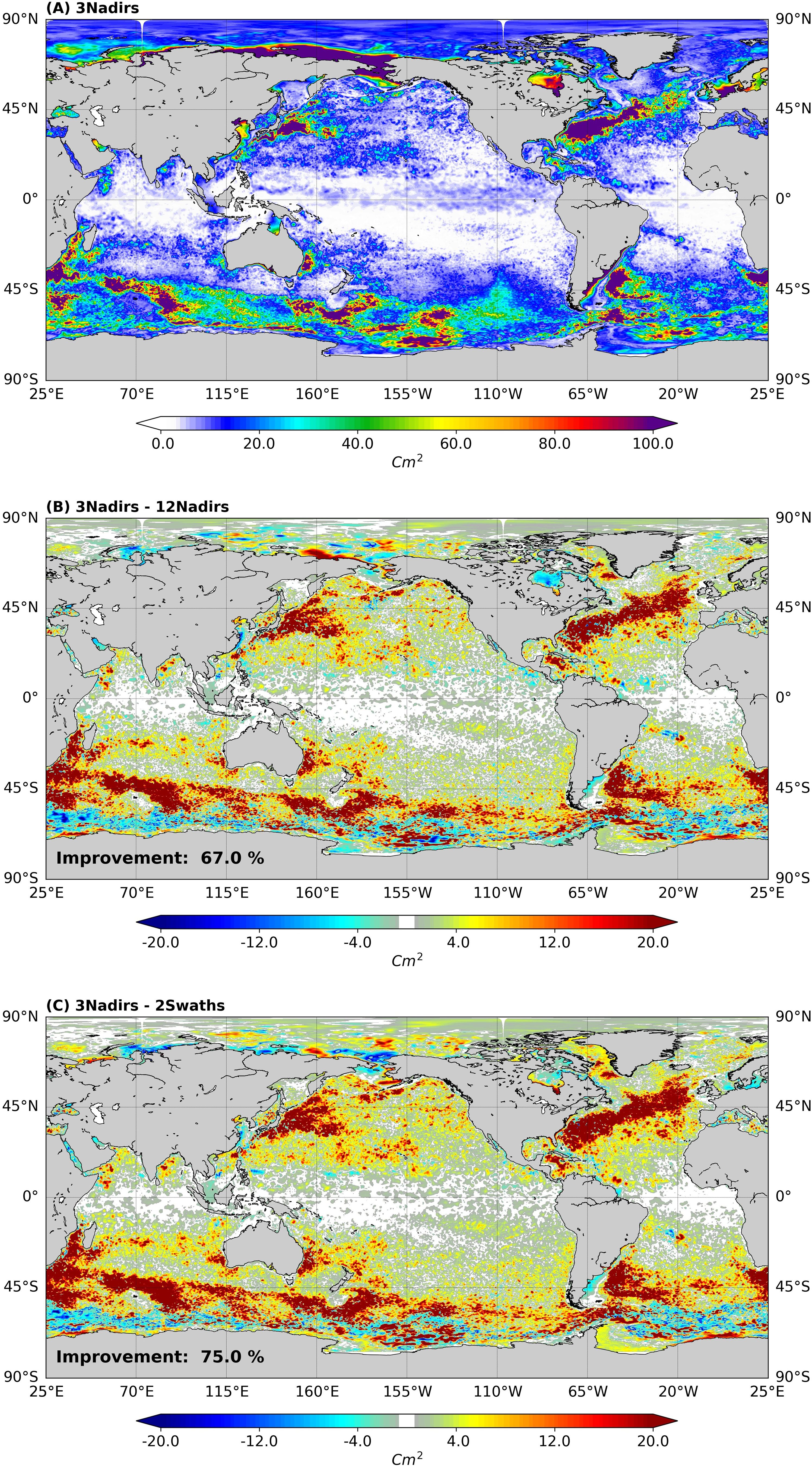
Figure 4. Global Maps of SSH analysis error (NatRun – Model Analysis) variance (in cm2, 2015). (A) 3Nadirs, (B) difference between analysis error variance of 3Nadirs and 12Nadirs, (C) difference between analysis error variance of 3Nadirs and 2Swaths.
Figure 5 summarizes the main results of these analyses. The three panels represent the mean analyzed SSH error variance as a function of latitude for the total error, the error for wavelengths smaller than 500 km and the error for wavelengths smaller than 200 km. Assimilation of data from the two wide-swath altimeters reduces the error at each latitude (red curves on the panels). This improvement is more pronounced at middle and high latitudes than at low latitudes. The impact on western boundary currents and Antarctic Circumpolar Current (ACC) is more evident at mesoscale (<200 km) as a function of the amplitude of the signal. This answers our question: data assimilation of SSH from two wide-swath altimeters provides a better estimate of the mesoscale than nadir altimeters.
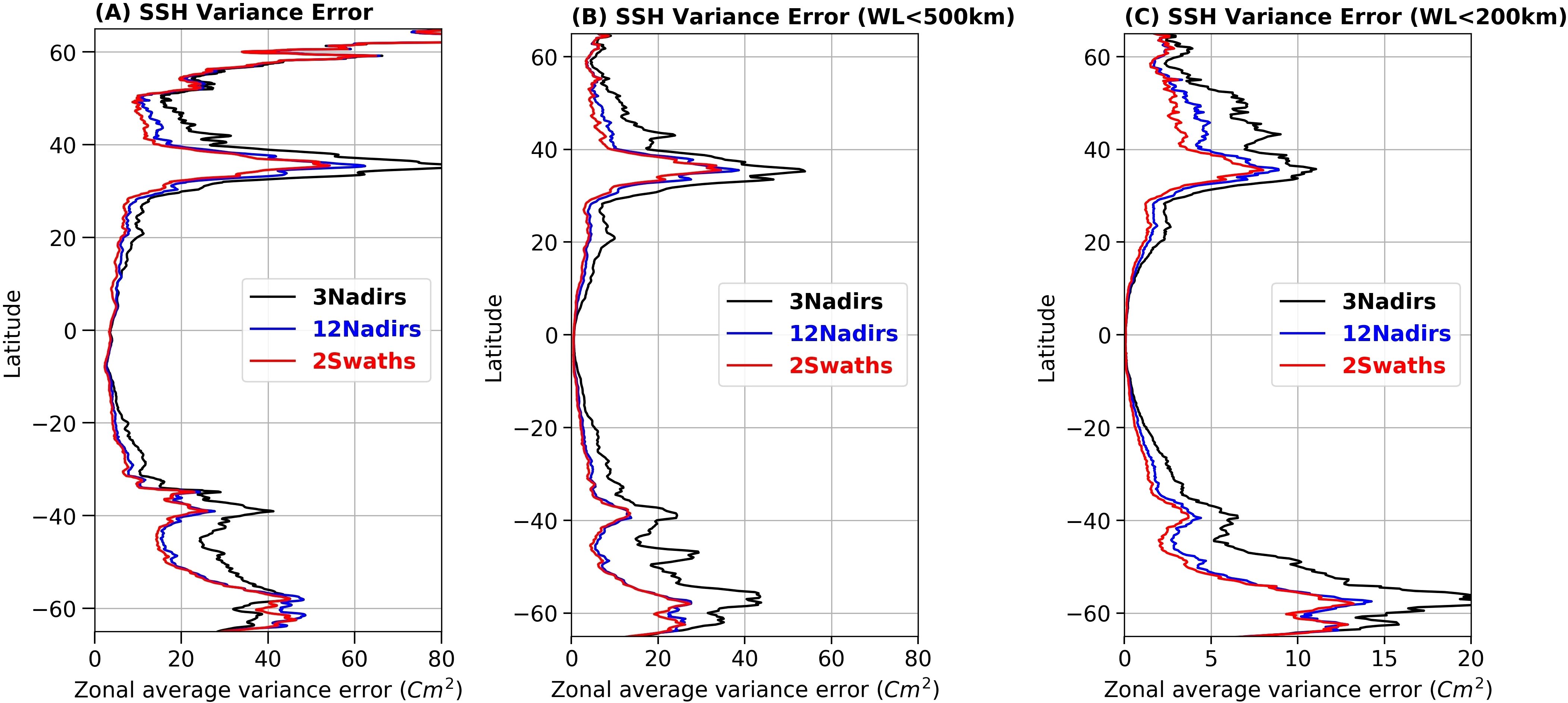
Figure 5. The zonal SSH averaged error variance: (A) for full scales, (B) for scales less than 500 km and (C) for scales less than 200 km; assimilation of 3Nadirs Run (black lines), 12Nadirs Run (blue lines) and 2Swaths Experiment (red lines). Units are cm2.
The aim of our study is also to show the impact of wide swath data assimilation on the forecast skill for different lead times. A comparison of the SSH forecast error is made on the three experiments (3Nadirs, 12Nadirs and 2Swaths) at different timescales between 1 and 7 days. A large impact is observed in the forecast (Figure 6), where the SSH forecast variance error over the global ocean increased by about twice between the 1st and the last (seventh) day. The assimilation of 12Nadirs (blue line) reduces the SSH forecast error by almost 80% compared to the 3Nadirs experiment (with 3 nadirs, black line). With the 2Swaths experiment (red line) we have an additional gain of 10% for the 1-day forecast and 14% for the 7-day forecast. The 7-day forecast error for 2Swaths experiment is of the same order as the 2-day forecast error for the 3Nadirs experiment. This gain in the 7-day forecast shows us that the model is able to retain the mesoscale structures introduced by the analysis even better than the 12Nadirs experiment.
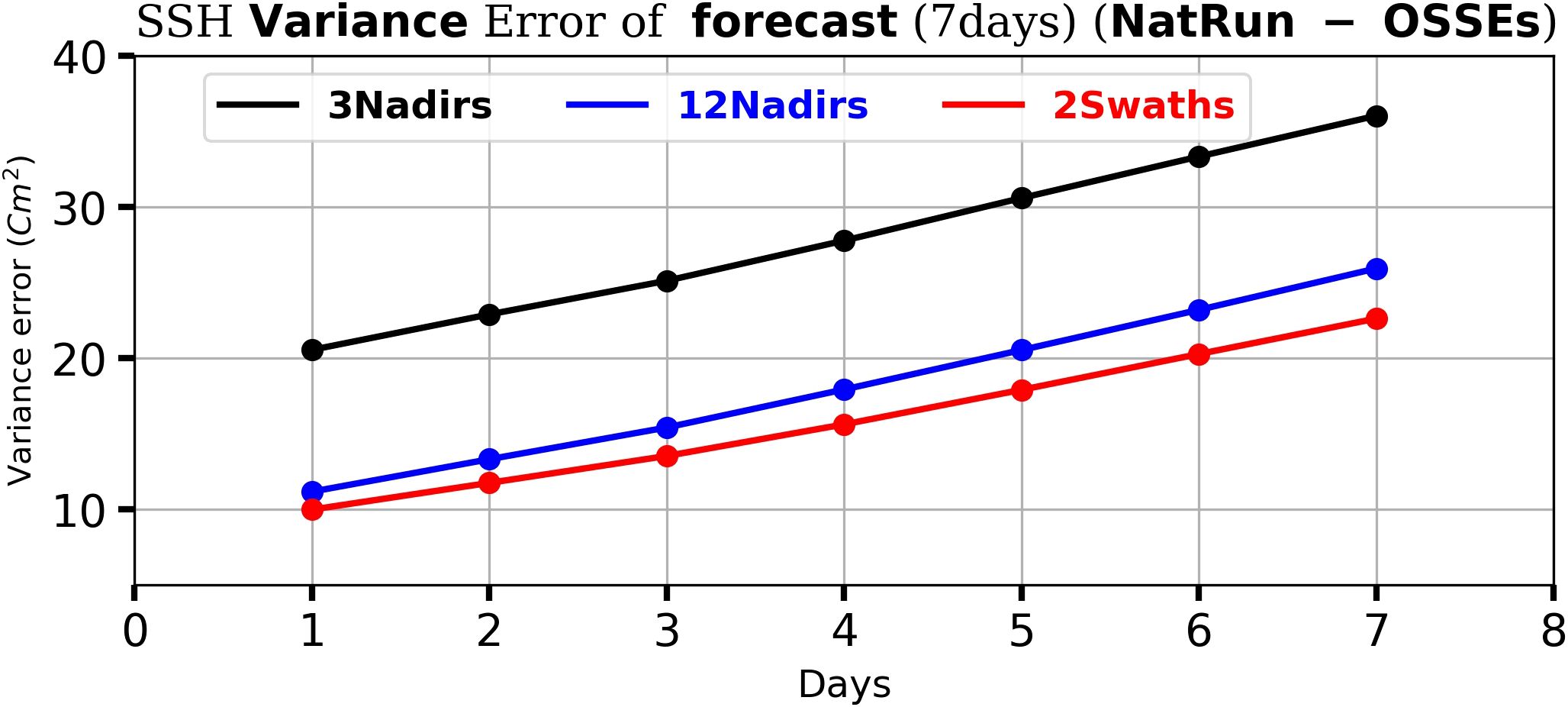
Figure 6. Variance of the error for each day of forecast (7 days, cm2) considering the SSH on the Global Ocean, over the period from February to December 2015. Results obtained by comparing the SSH of the ocean forecast in the 3Nadirs (black line), 12Nadirs (blue line) and 2Swaths (red line) experiments with the data from the NatRun.
3.2 Spectral analysis and coherence
Regional statistical and spectral analysis are performed and discussed in this section for two regions, the Gulf Stream and Kuroshio extension areas.
Figure 7 shows the maps of the SSH variance error of the 3Nadirs and the differences of the 12Nadirs and 2Swaths experiment compared to the 3Nadirs simulation with the temporal evolution of SSH RMS errors in the Gulf Stream area (red box in Figure 2). In Figure 7B (2Swaths-3Nadirs), we have an important difference (negative) compared to the difference between 12Nadirs and 3Nadirs (Figure 7C). With 2Swaths, the error is smaller than with 12Nadir. This is also seen on the time mean evolution of SSH RMS error in Figure 7D. The evolution of the RMS error in SSH of the 2Swaths experiment (red curve) is lower than that of 12Nadirs except at the beginning of the year, with an annual average of 0.080m instead of 0.086m over this region of the Gulf Stream area.
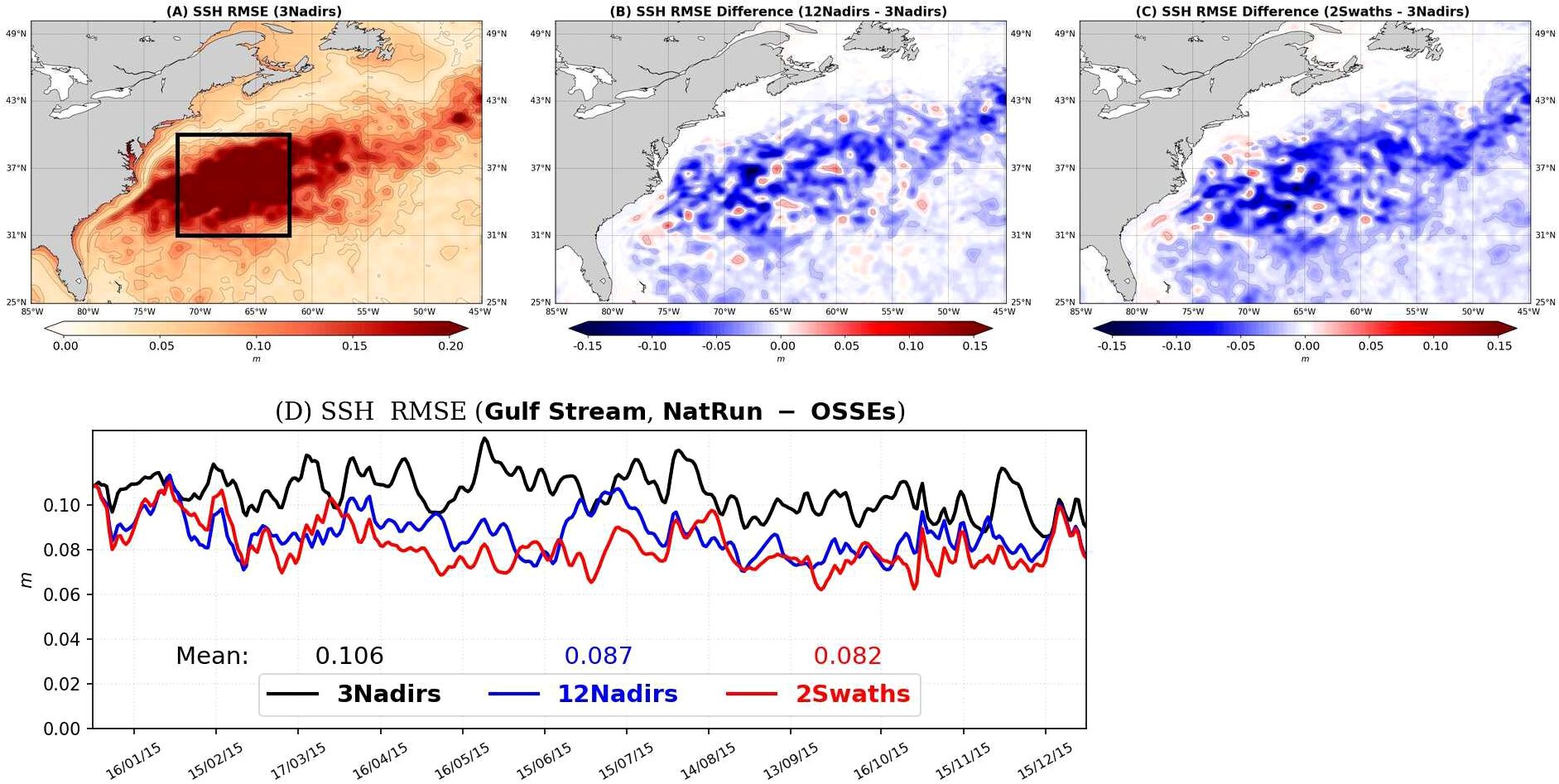
Figure 7. The SSH RMS error over 2015 for the 3Nadirs run [(A), top] and the difference in RMS error compared to the 3Nadirs for the 12Nadirs [(B), top] and 2Swaths [(C), top] for Gulf Stream area (red box Figure 2). The temporal evolution of the SSH RMS error analysis for this area; experiments 3Nadirs: black line, 12Nadirs: blue line and with 2Swaths: red line.
Figure 8 shows the power spectra of the SSH error in a variance preserving form (Thomson and Emery, 2014) in the Gulf Stream area. The assimilation of nadir altimeter data from 12 nadirs (blue curves) reduces the error compared to the 3Nadirs experiment (black line) for wavelengths greater than180km. On the other hand, with the 2Swaths experiment (red curve), this error is much reduced compared to the 12Nadirs (blue curve) for wavelength higher than 150km. A temporal spectral analysis (Figure 8B) is also carried out to highlight the impact of assimilating swath data on different temporal scales. Coherence is defined as the correlation between two signals as a function of wavelength. The effective temporal resolution for the 12Nadirs (blue curve) is 30 days, for the 2Swaths experiment is 28 days (red curves) compared with the 3Nadirs (48 days, black curve) with a coherence of 50%. There is better resolution with 2Swaths (red curve) compared to 12Nadirs at all timescale.
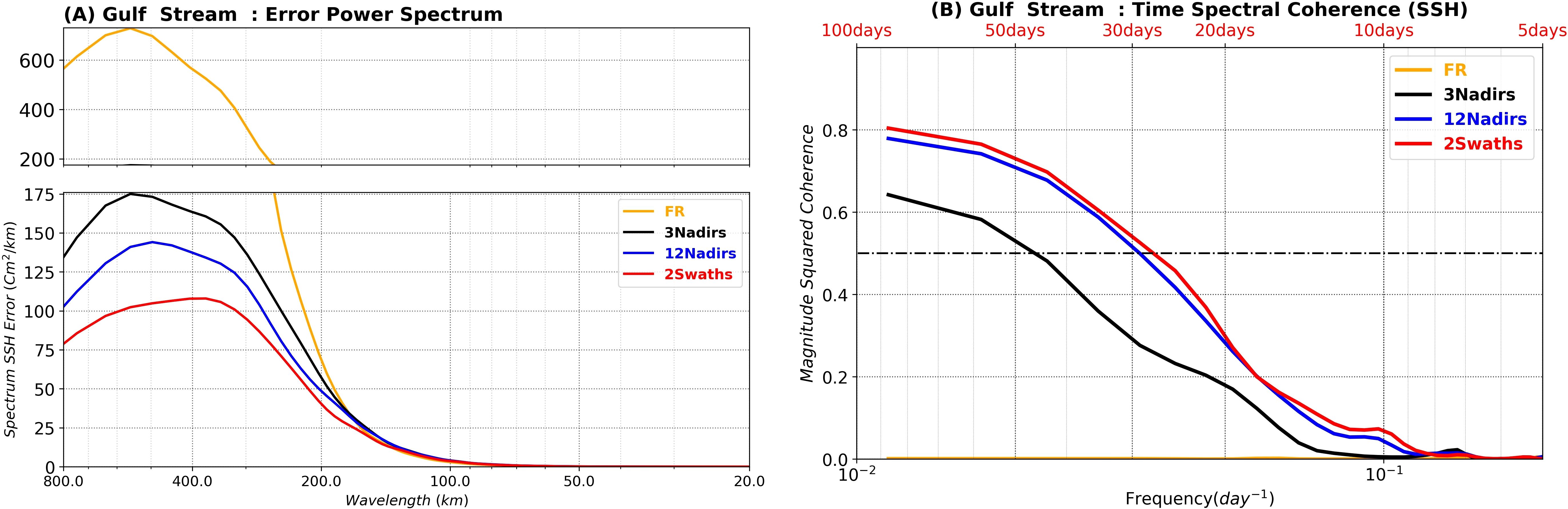
Figure 8. Power spectra SSH error with respect to the NatRun; the spectra are shown in a variance-preserving form (cm2), (A) Gulf Stream area (black box in Figure 7), (B) Time spectral coherence with respect to the NatRun (same area), 3Nadirs experiment: black line, 12Nadirs experiment: blue line and 2Swaths experiment: red line.
Looking at a different region, at the top of Figure 9 we show the SSH variance error maps in the Kuroshio extension area of the 3Nadirs and the differences of the 12Nadirs and 2Swaths experiments compared to the 3Nadirs simulation. On the right-hand figure, we have the difference between 12Nadirs and 3Nadirs. This confirms that even in high energy regions, the analyzed SSH in the 2Swaths experiment is better estimated than in the 12Nadirs experiment. We confirm this by the figure below, which represents the evolution of the SSH RMS error as a function of time over the year 2015. The red line, corresponding to the 2Swaths experiment, has on average over the whole period a lower mean compared to the blue line, corresponding to the 12Nadirs experiment. Figure 10 shows on the left the Power spectra of the SSH error with respect to the NatRun, while on the right we have time spectral coherence with respect to the NatRun. These spectra are calculated on the black box defined on Figure 9. Over this region, the impact is negligible, with an improvement for scales below 200km and a slight degradation compared to the 12Nadirs for scales above 200km on this region. Similarly, a very small difference regarding the temporal coherence is found between the 12Nadirs and 2Swaths experiments.
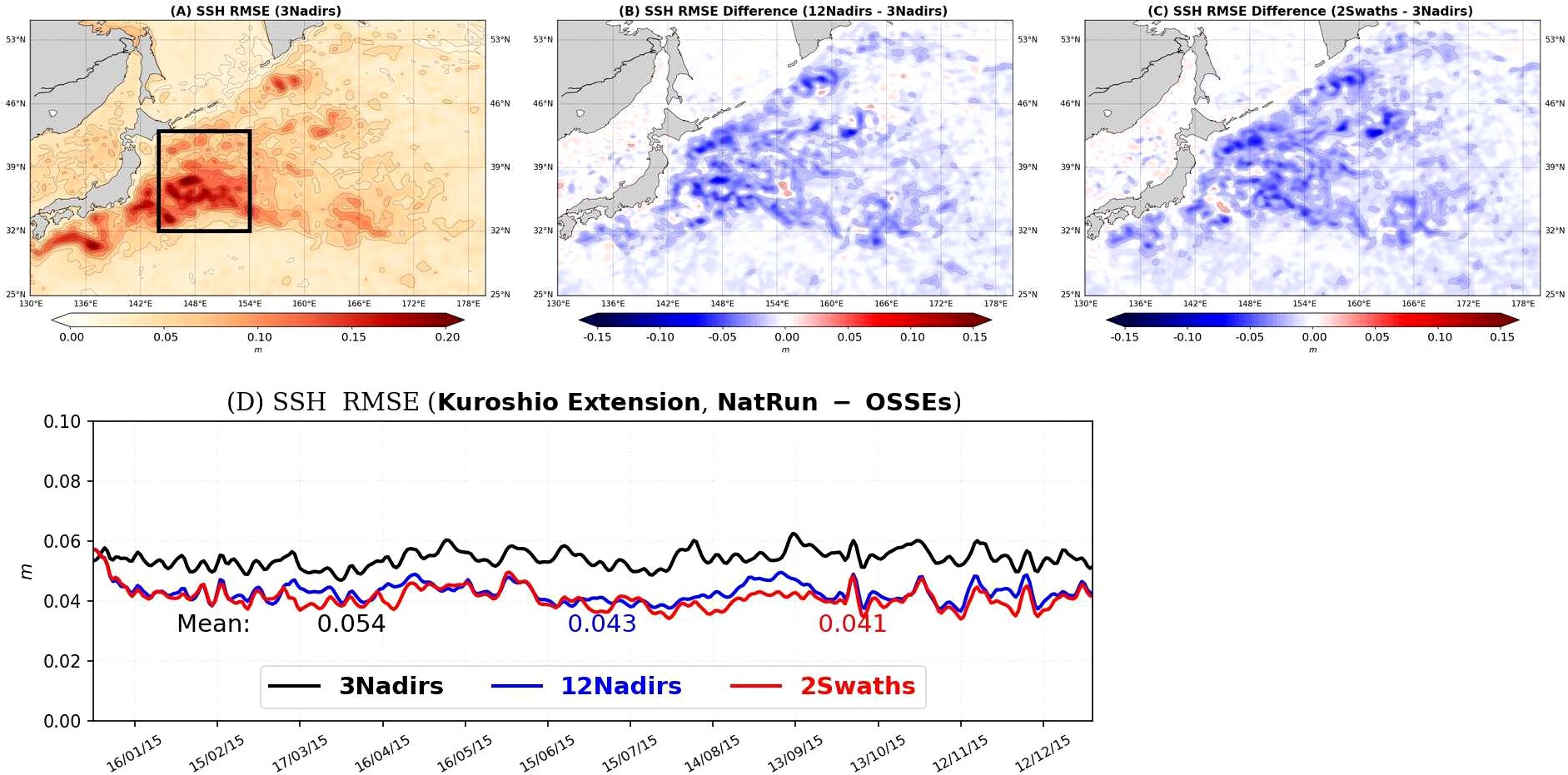
Figure 9. The SSH RMS error over 2015 for the 3Nadirs run [(A), top] and the difference in RMS error compared to the 3Nadirs for the 12Nadirs [(B), top] and 2Swaths [(C), top] for Kuroshio area (red box Figure 2). The temporal evolution of the SSH RMS error analysis for this area; experiments 3Nadirs: black line, 12Nadirs: blue line and with 2Swaths: red line.
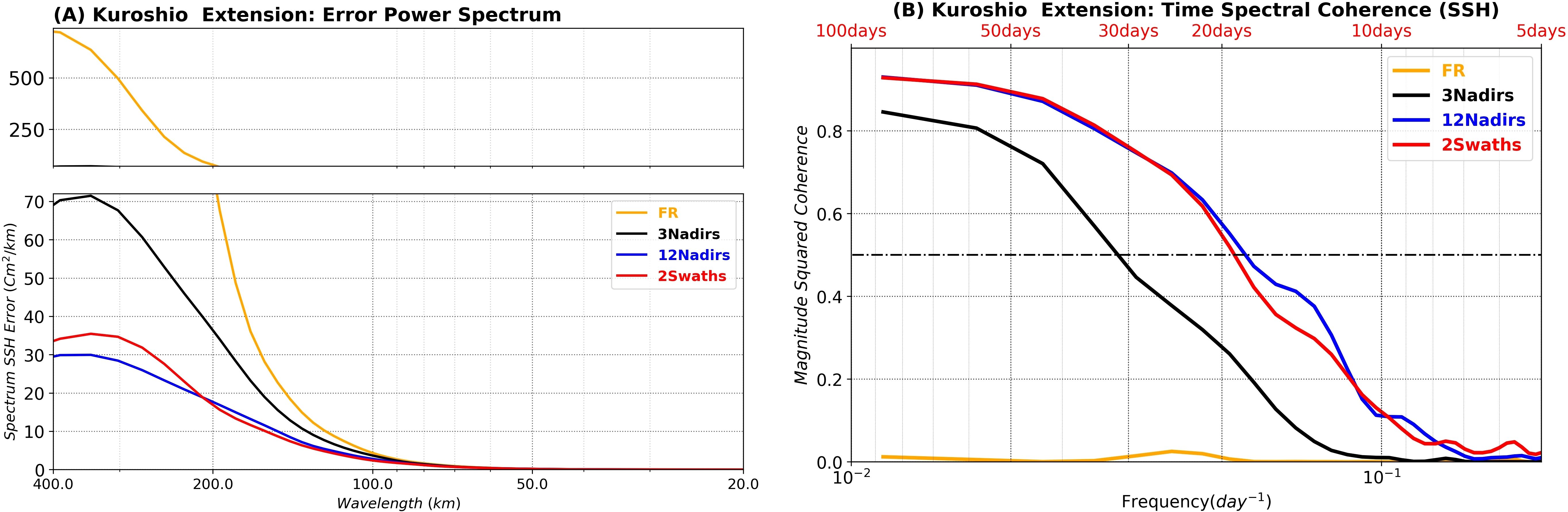
Figure 10. Power spectra SSH error with respect to the NatRun; the spectra are shown in a variance-preserving form (cm2), (A) Kuroshio Extension (black box in Figure 9), (B) Time spectral coherence with respect to the NatRun (same area), 3Nadirs experiment: black line, 12Nadirs experiment: blue line and 2Swaths experiment: red line.
The Supplementary Figure 1 shows a comparison of the wavenumber-frequency energy spectra of the NatRun SSH over the two regions. In the Kuroshio region (figure on the right), the NatRun has a relatively lower energy at all spatial scales (especially between 10 and 150 days) compared with the Gulf Stream region (figure on the left). The less energetic Kuroshio region, is fairly well controlled by the assimilation of Nadirs data (12Nadirs), with lower SSH errors for the different OSSEs than the Gulf Stream region that needs the assimilation of data from 2Swaths to be well constrained. This confirms the impact of 2Swaths data assimilation over these two regions.
3.3 Impact on temperature, salinity and zonal velocities
Through the multivariate background covariance specified in the assimilation scheme, the analysis increment for the 3D temperature, salinity and velocity fields will be different in the three OSSEs experiments. Analyzing the impact of assimilating SSH data from different altimeter constellation design on the other variables than the SSH itself allows to check if improvements seen in the SSH also leads to improved 3D T, S and velocity field or at least did not degrade them. The Figure 11, shows the variance and mean of the temperature and salinity error as a function of depth for the global ocean. This error is calculated between the temperature and salinity from the NatRun and each OSSE. The temperature error profile shows a maximum value at about 100m depth, which corresponds to the thermocline depth. This error is significantly reduced by the assimilation of 12 Nadirs (blue profile) compared to 3Nadirs (black profile). The assimilation of wide-swath altimetry data does not degrade this score and we even have a slight improvement between 100 and 750m depth. For salinity (right figure), the improvement is less clear, but no degradation is observed regardless of the depth.
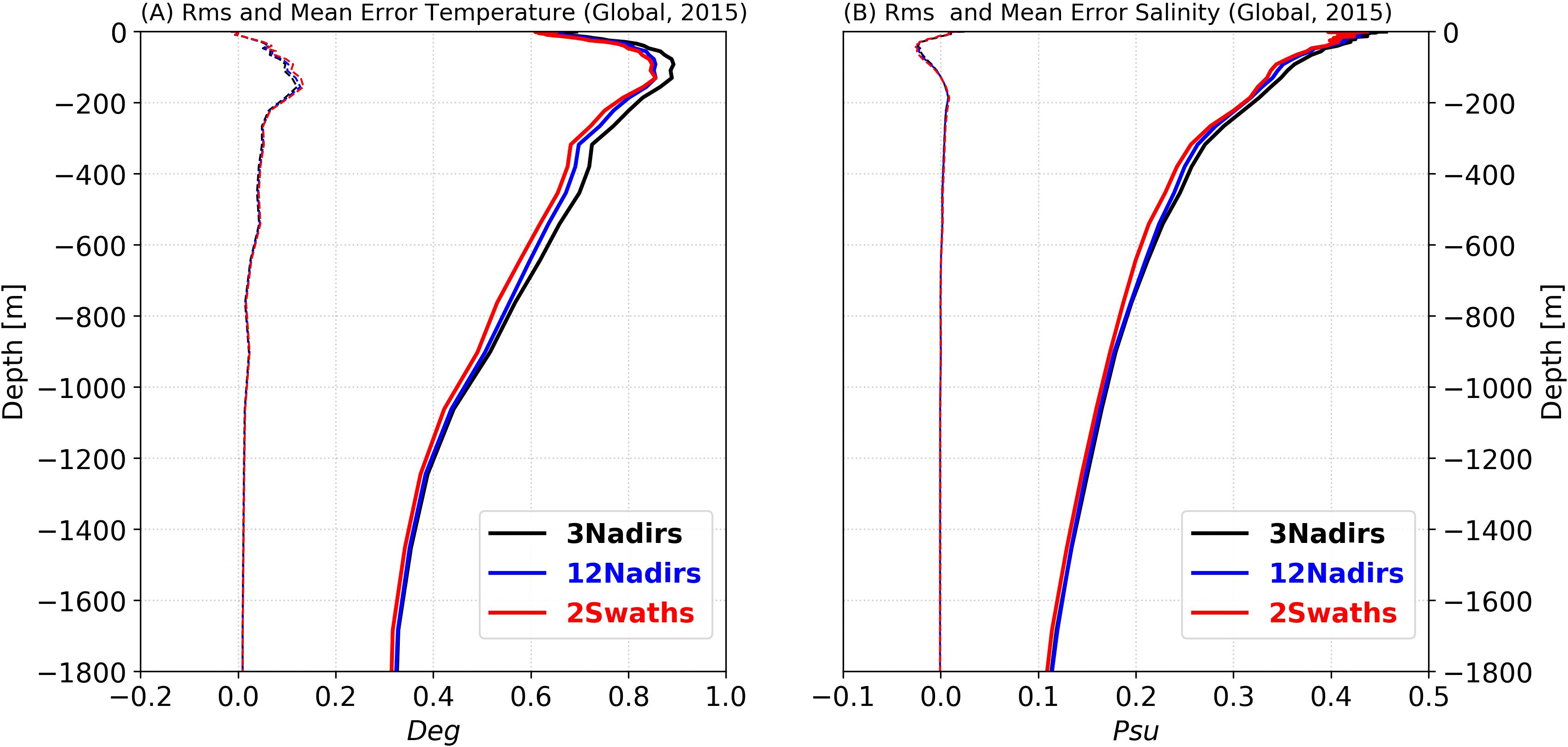
Figure 11. Global averaged RMS error (solid lines) and mean (dashed lines): (A) temperature (in °C) and (B) salinity (Psu) over the period 2015. The results were obtained by comparing temperature and salinity profiles of OSSEs with the profiles simulated from NatRun; 3Nadirs (black lines), 12Nadirs (blue lines) and 2Swaths (red lines).
In Figure 12, we compare the evolution of the variance of the velocity analysis error (NatRun - OSSEs) as a function of time over the year 2015 at the surface for the zonal (U, panel A) and meridional (V, panel B) components. We observe a reduction that sets in after one month and remains constant over the year. As shown in Table 3, we have a reduction of these errors for the global ocean (in variance) of about 10% for the 2Swaths experiment compared to the 12Nadirs experiment.
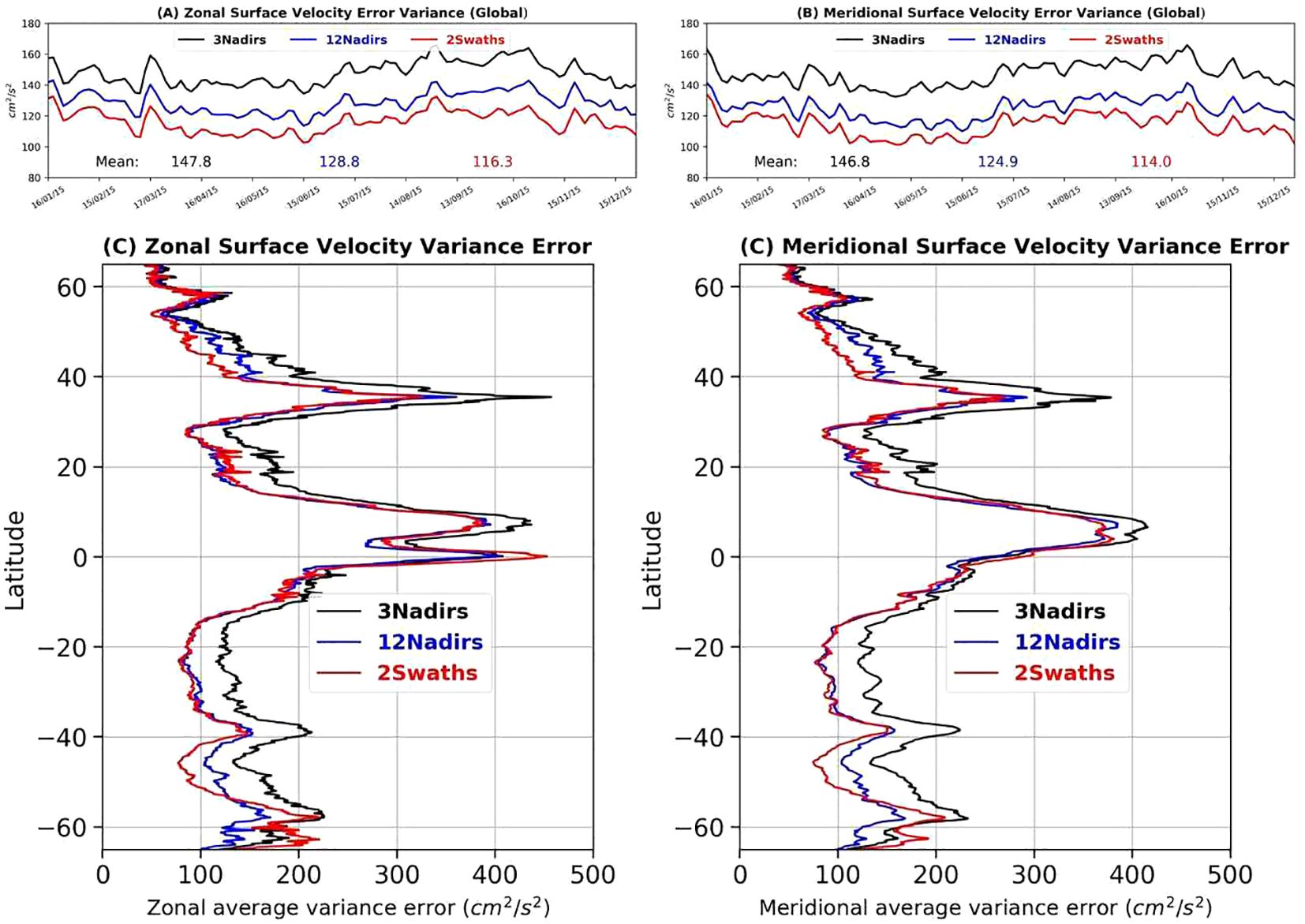
Figure 12. Temporal evolution of zonal (A) and meridional surface velocity (B) error variance (cm2/s2) for 7-day ocean analysis over the period from January to December 2015. the bottom panels are zonal average error variance zonal (C) and meridional surface velocity (D): assimilation of 3Nadirs (black lines) and assimilation of 2Swaths (red lines). Units are cm2/s2. These results were obtained by comparing both components of the velocity (U; V) to that of the NatRun. Black curves with assimilation of 3 Nadirs (3Nadirs); blue curves for 12Nadirs experiment and red curves with assimilation of 2Swaths experiment (2 Wide-Swath).
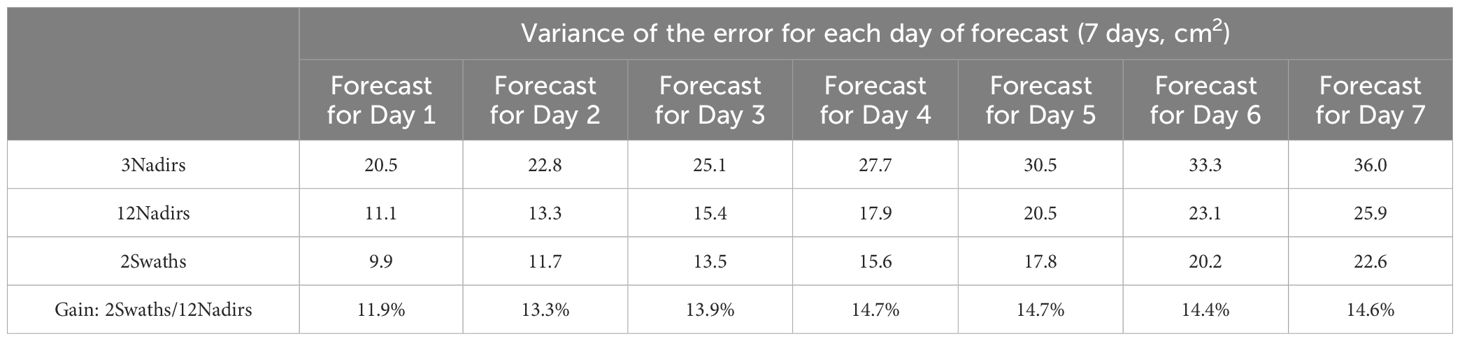
Table 3. Variance of the error for each day of forecast (7 days) considering the SSH on the Global Ocean, over the period from February to December 2015.
Figure 12 (bottom) summarize the impact of the 2Wide Swath experiment in relation to the 12nadirs on surface currents as a function of latitude. Both on the zonal component (figure C) and on the meridional component (figure D), the 2swaths experiment (red curves) presents less error (error variance) than the 12nadirs experiment, especially in western boundary currents (Gulf Stream, Kuroshio region, Agulhas…). These results show that the 2Wide Swath experiment allows a better representation of mesoscale variability there.
The Figure 13 shows the average variance and mean error both for the zonal (U) and meridional (V) velocity as a function of depth for the global ocean for each of the experiments. The SSH assimilation from 12Nadirs (blue profiles) shows a good reduction of the error on the two velocity components on the global ocean mean and on the whole water column compared to the 3Nadirs simulation. The 2Swaths (2Wide-Swaths) experiment (red profiles) brings a reduction of this error compared to 12Nadirs (blue profiles) on the water column.
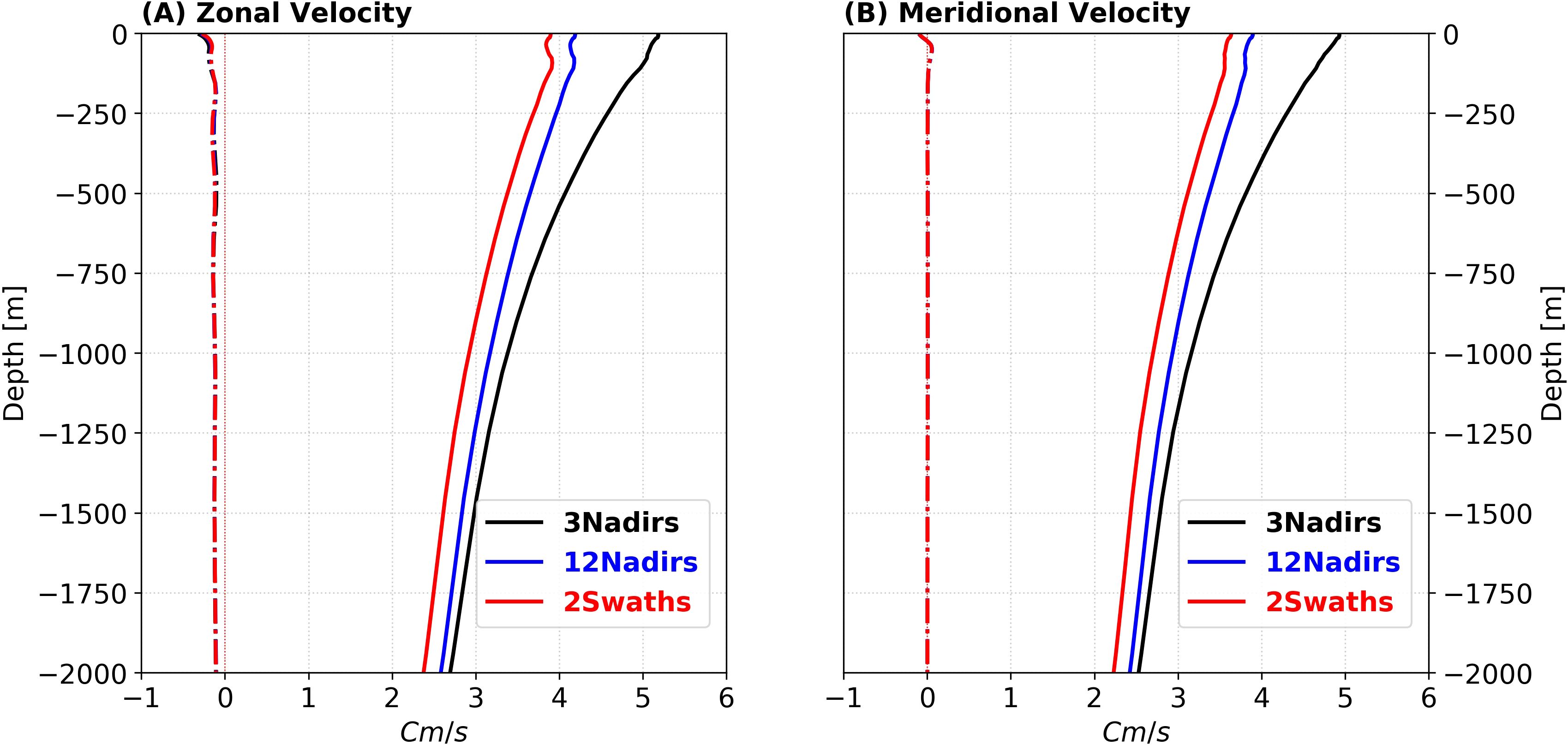
Figure 13. The average error variance of zonal (left figure) and meridional velocity (right figure): assimilation of 3Nadirs (black lines) and assimilation of 2Swaths (red lines). Units are cm2/s2.
3.4 Lagrangian diagnostics
The aim of this study is to quantify the contribution of assimilating swath data compared with 12Nadirs data on the quality of the model’s surface currents (analysis and forecast). With this new generation of swath altimeters, we should have a better representation of mesoscale phenomena. To quantify this impact, we carried out the same analysis as that presented in the paper by Tchonang et al. (2021). We evaluate the ability of OSSEs to reproduce the particle drift observed with the NatRun compared to our OSSEs. Lagrangian particle positions are initialized uniformly at 1/12° (between latitudes 66°S and 66°N, the maximum latitude of the Jason/S6 orbit). These particles are advected by the surface currents in each simulation (NatRun and OSSEs) over a period of 7 days. We used 6,130,410 particles in each experiment. We used the Ariane software (Blanke and Raynaud, 1997) to carry out these experiments.
To highlight the impact of the different missions, we compared the arrival distance of these particles after 7 days drift with their positions in the NatRun at the same date. The separation distance for the 3Nadirs run is shown in Figure 14A. In this figure, we can see that after 7 days, particles in areas of high activity (western boundary currents, tropics, etc.) are more than 100km from their position in the NatRun. The figures show the change in separation distance compared with 3Nadirs. Figures 14B, C show respectively the change in direction of particles in the 12Nadirs and 2Swaths experiments compared with the 3Nadirs run. The 2Swaths experiment (Figure C) improves particle trajectory compared with the 12Nadirs run (Figure B) over almost the entire globe. On average, 2Swaths shows a gain of 17.5% compared with 3Nadirs, while 12Nadirs shows a gain of 14.46% (Table 4). This table also shows (first column) the percentage of particles that remain at a distance of less than 50km from their position in NatRun (our reality). In the 2Swaths experiment, 73.9% of particles are 50km from reality (NatRun), compared with 64.5% for 12Nadirs and 40.6% for the 3Nadirs run.

Figure 14. (A) Separation distance between particles deployed in 3Nadirs (three nadirs) and their equivalent in the NatRun after 7 days of advection by their respective surface current velocities. The results were averages in 2x2 degree bins. (B, C) Changes (in %) of the separation distance when using 12Nadirs or 2Swaths (2Wide-Swaths) surface velocities relative to results with 3Nadirs.
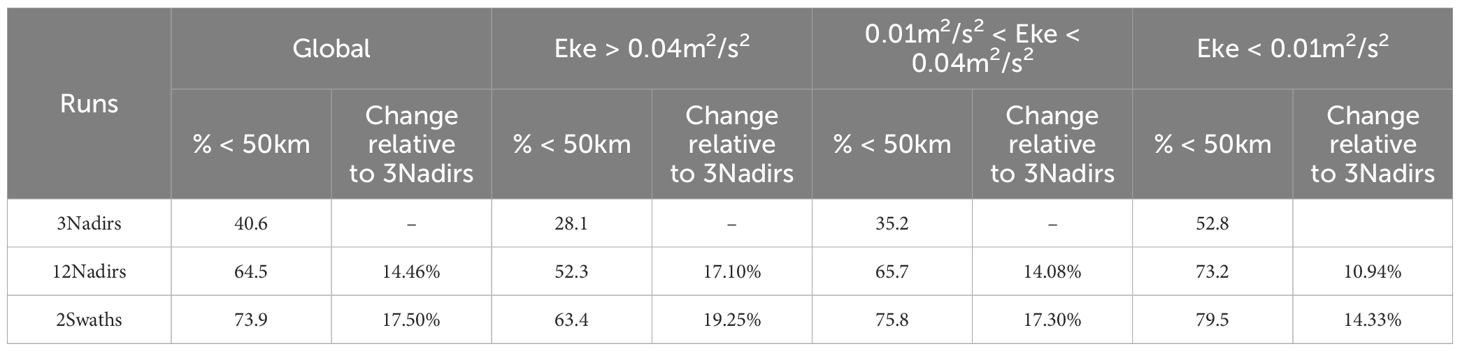
Table 4. Summary of statistics for the separation distance of OSSE Lagrangian particles with their NatRun equivalent after 7 days of advection, for the global ocean and three regions with a range of NatRun Eke (see Figure 15).
An analysis by different energy zones was carried out to better show the impact of swath data compared to nadirs data on the separation distance error. We chose to carry out an analysis in three bands of EKE (Eddy kinetic energy) to distinguish regions with different regimes. Figure 15 shows the result of this analysis. Regions with EKE greater than 0.04m2/s2 (Figure 15A) show a gain of 11% with 2Swaths compared to 12Nadirs (63.4% for 2Swaths instead of 52.3% for 12Nadirs). In the second energy range (0.01 m2/s2 < Eke < 0.04 m2/s2, panel B) the gain remains fairly significant at around 10%. On the other hand, in the low-energy zones (< 0.01m2/s2, weaker currents) the gain is less marked, but remains significant at around 6%. Figure 15 summarizes these percentage gains for the 2Swaths experiment compared to the 12Nadirs for each region with a different energy range. The assimilation of swath altimeter data has a positive impact on surface currents in our analysis and forecasts compared with nadir data.
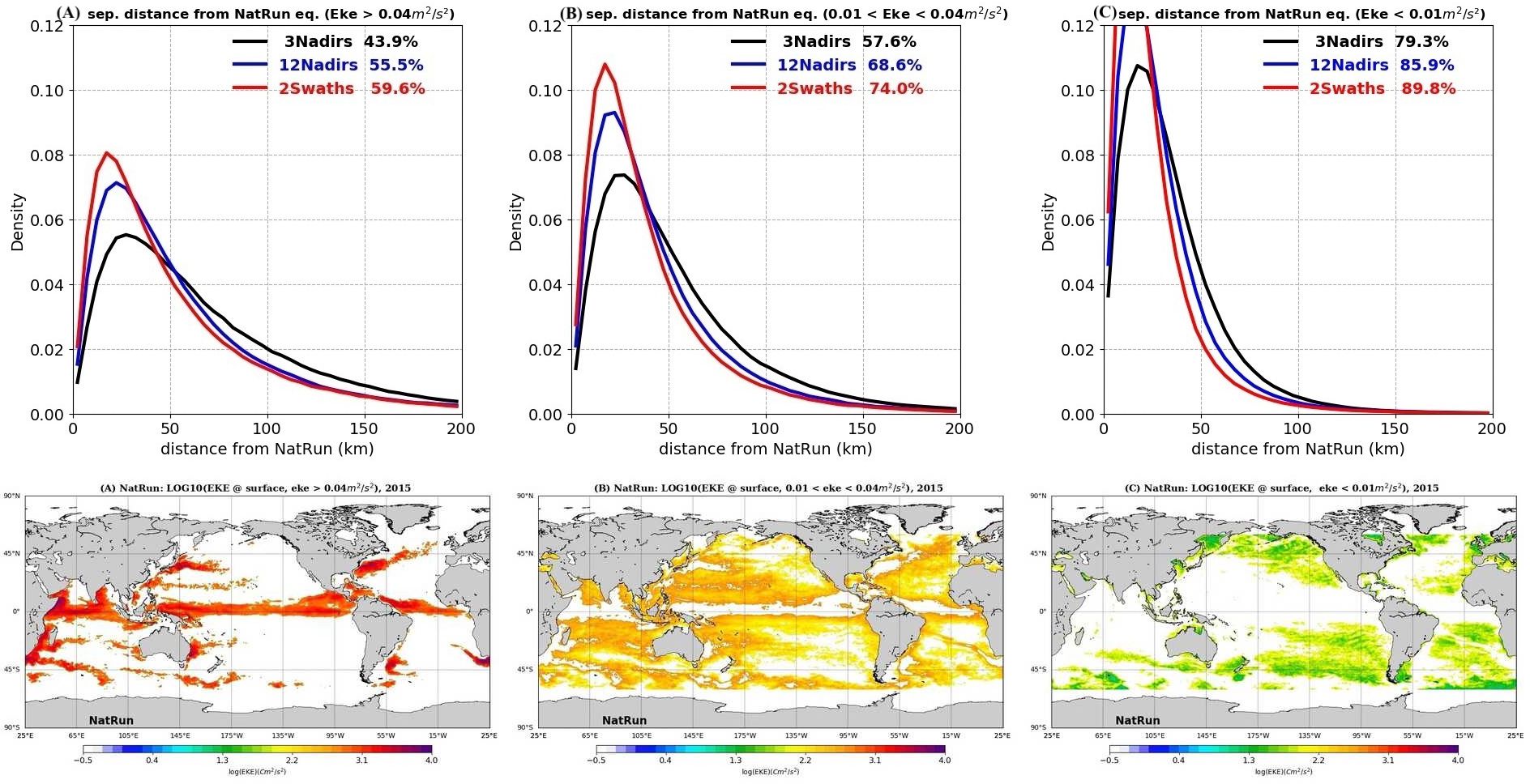
Figure 15. Binomial histogram of separation distance for all OSSEs: Each graph represents a region with a range of NatRun Eke (mean annual Eke): (A) region with high Eke (>0.04 m2/s2), (B) region with medium Eke (0.01 m2/s2 < Eke > 0.04 m2/s2) and (C) region with low Eke (<0.01 m2/s2). The figure noted for each experiment represents the percentage of the number of particles for which the distance between the OSSE particles and their NatRun equivalent remains less than 50Km.
4 Summary and conclusions
The SWOT mission, which was launched in December 2022, will likely demonstrate the major contribution of swath altimeters to ocean monitoring and forecasting. OSSEs (Benkiran et al., 2021; Tchonang et al., 2021) carried out with a global 1/12° data assimilation system have shown that SWOT will provide a large improvement in ocean analyses and forecasts but with a limitation due to its 21-day time revisit. A complementary study (Benkiran et al., 2022) showed, however, that the constellation of two wide-swath altimeters should provide a much larger improvement.
New OSSEs with the same system setup as the previously mentioned ones but with refined observation error characteristics were carried out in this study to compare the relative merits of a constellation of two wide-swath altimeters and a constellation of 12 nadir altimeters (in the same orbital plane as Sentinel-3). Such configurations are envisioned by ESA for the long-term evolution (post-2032) of the Copernicus Sentinel-3 topography mission to meet the requirements expressed by the Copernicus Marine Service and its applications (CMEMS, 2017). These two configurations greatly improve ocean forecasting and monitoring capabilities. Compared to a constellation of three nadir altimeters (the present configuration), analysis and forecast errors are reduced by a factor of 2. Our results also show that a constellation of two wide-swath altimeters performs better than a constellation of 12 nadirs. Compared to a constellation of 12 nadirs, the error of the SSH forecast of a two wide-swath constellation will be reduced by 14% overall. Improvements are also observed for surface currents (~10%) and Lagrangian diagnostics. We would point out that these results depend on the forecasting system used and the study period (2015 in our study).
Flying a constellation of two wide-swath altimeters thus looks to be a very promising solution for the long-term evolution of the Sentinel-3 constellation and the Copernicus Marine Service. End-to-end simulations taking into account a full error budget and all processing steps (e.g. reduction of large scale errors, intercalibration) before data are assimilated would be needed to fine tune these results. It will be useful, in particular, to assess how along-track long wavelength errors (e.g. due to orbit error, tidal or inverse barometer correction errors) by inducing spurious cross-track errors impact the ability of a constellation of multiple altimeters to map the mesoscale signals.
With respect to swath techniques, the comparison of these results with real data from SWOT will also allow us to verify the degree of realism of our simulations.
Data availability statement
The raw data supporting the conclusions of this article will be made available by the authors, without undue reservation.
Author contributions
MB: Methodology, Validation, Visualization, Writing – original draft, Writing – review & editing. P-YL: Funding acquisition, Writing – review & editing. ER: Writing – review & editing. YD: Writing – review & editing.
Funding
The author(s) declare financial support was received for the research, authorship, and/or publication of this article. The study was funded by ESA and was also carried out as part of a partnership agreement between Mercator Ocean International and CNES.
Acknowledgments
The authors would like to acknowledge the reviewers for their valuable comments that helped improve the manuscript’s clarity.
Conflict of interest
The authors declare that the research was conducted in the absence of any commercial or financial relationships that could be construed as a potential conflict of interest.
Publisher’s note
All claims expressed in this article are solely those of the authors and do not necessarily represent those of their affiliated organizations, or those of the publisher, the editors and the reviewers. Any product that may be evaluated in this article, or claim that may be made by its manufacturer, is not guaranteed or endorsed by the publisher.
Supplementary material
The Supplementary Material for this article can be found online at: https://www.frontiersin.org/articles/10.3389/fmars.2024.1465065/full#supplementary-material
Supplementary Figure 1 | SSH NatRun wavenumber-frequency energy spectra in Gulf Stream box (black box in Figure 8A, left panel) and Kuroshio (black box in Figure 9A, panel right).
References
Benkiran M., Greiner E. (2008). Impact of the incremental analysis updates on a real-time system of the North Atlantic Ocean. J. Atmospher. Ocean. Technol. 25, 2055–2073. doi: 10.1175/2008jtecho537.1
Benkiran M., Le Traon P.-Y., Dibarboure G. (2022). Contribution of a constellation of two wide-swath altimetry missions to global ocean analysis and forecasting. Ocean Sci. 18, 609–625. doi: 10.5194/os-18-609-2022
Benkiran M., Ruggiero G., Greiner E., Le Traon P. Y., Rémy E., Lellouche J. M., et al. (2021). Assessing the impact of the assimilation of SWOT observations in a global high-resolution analysis and forecasting system. Part 1: method. Front. Mar. Sci. 8. doi: 10.3389/fmars.2021.691955
Blanke B., Raynaud S. (1997). Kinematics of the Pacific equatorial undercurrent: an Eulerian and Lagrangian approach from GCM results. J. Phys. Oceanogr. 27, 1038–1053.
Bloom S. C., Takas L. L., Da Silva A. M., Ledvina D. (1996). Data assimilation using incremental analysis updates. Mon. Weather Rev. 124, 1256–1271. doi: 10.1175/1520-0493(1996)124<1256:dauiau>2.0.co;2
Bonaduce A., Benkiran M., Remy E., Le Traon P. Y., Garric G. (2018). Contribution of future wide-swath altimetry missions to ocean analysis and forecasting. Ocean Sci. 14, 1405–1421. doi: 10.5194/os-14-1405-2018
CMEMS (2017). CMEMS requirements for the evolution of the Copernicus Satellite Component. Available online at: https://marine.copernicus.eu/sites/default/files/media/pdf/2020-10/CMEMS-requirements-satellites.pdf (accessed February 21, 2017).
Dee D. P., Uppala S. M., Simmons A. J., Berrisford P., Poli P., Kobayashi S., et al. (2011). The ERA-interim reanalysis: configuration and performance of the data assimilation system. Q. J. R. Meteorol. Soc 137, 553–597. doi: 10.1002/qj.828
Dibarboure G., Lamy A., Pujol M. I., Jettou G. (2018). The drifting phase of SARAL: Securing stable ocean mesoscale sampling with an unmaintained decaying altitude. Remote Sens. 10, 1051.
Errico R. M., Yang R., Privé N. C., Tai K.-S., Todling R., Sienkiewicz M. E., et al. (2013). Development and validation of observing-system simulation experiments at NASA’s Global Modeling and Assimilation Office. Q. J. R. Meteorol. Soc 139, 1162–1178. doi: 10.1002/qj.2027
Esteban Fernandez D., Fu L.-L., Pollard B., Vaze P. (2017). SWOT project mission performance and error budget. Tech. Rep., 117pp.
Gasparin F., Greiner E., Lellouche J.-M., Legalloudec O., Garric G., Drillet Y., et al. (2018). A large-scale view of oceanic variability from 2007 to 2015 in theglobal high-resolution monitoring and forecasting system at Mercator-Ocean. J. Mar. Syst. 187, 260–267. doi: 10.1016/j.jmarsys.2018.06.015
Gaultier L., Ubelmann C., Fu L. L. (2016). The challenge of using future SWOT data for oceanic field reconstruction. J. Atmospheric Oceanic Technol. 33, 119–126. doi: 10.1175/JTECH-D-15-0160.1
Hamon M., Greiner E., Le Traon P. Y., Remy E. (2019). Impact of multiple altimeter data and mean dynamic topography in a global analysis and forecasting system. J. Atmospheric Oceanic Technol. 36, 1255–1266. doi: 10.1175/JTECH-D-18-0236.1
Lellouche J. M., Greiner E., Le Galloudec O., Garric G., Regnier C., Drevillon M., et al. (2018). Recent updates to the Copernicus marine service global ocean monitoring and forecasting real-time 1/12° high-resolution system. Ocean Sci. 14, 1093–1126. doi: 10.5194/os-14-1093-2018
Le Traon P. Y., Dibarboure G., Jacobs G., Martin M., Remy E., Schiller A. (2017). “Use of satellite altimetry for operational oceanography,” in Satellite Altimetry Over Oceans and Land Surfaces. Eds. Stammer, Cazenave (CRC Press, Boca Raton, FL).
Morrow R., Fu L. L., Ardhuin F., Benkiran M., Chapron B., Cosme E., et al. (2019). Global observations of fine-scale ocean surface topography with the surface water and ocean topography (SWOT) mission. Front. Mar. Sci. 6. doi: 10.3389/fmars.2019.00232
Madec, G. and NEMO System Team (2015). NEMO ocean engine. Note du Pôle de modélisation de l’Institut Pierre-Simon Laplace No 27. Guyancourt: Institut Pierre-Simon Laplace (IPSL). Available: https://epic.awi.de/id/eprint/39698/1/NEMO_book_v6039.pdf (accessed accessed 6 Dec 2024).
Pham D., Verron J., Roubaud M. (1998). A Singular Evolutive Extended Kalman filter for data assimilation in oceanography. J. Mar. Syst. 16, 323–340. doi: 10.1016/s0924-7963(97)00109-7
Tchonang C. B., Benkiran M., Le Traon P. Y., Van Gennip S., Lellouche J. M., Ruggiero G. (2021). Assessing the impact of the assimilation of SWOT observations in a global high-resolution analysis and forecasting system. Part 2: Results. Front. Mar. Sci. 8. doi: 10.3389/fmars.2021.687414
Thomson R. E., Emery W. J. (2014). “Chapter 5 - Time Series Analysis Methods,” in Data Analysis Methods in Physical Oceanography, 3rd Edn. Eds. Thomson R. E., Emery W. J. (Elsevier, Boston), 425–591. doi: 10.1016/B978-0-12-387782-6.00005-3
Keywords: data assimilation, ocean forecasting, surface water ocean topography (SWOT) mission, satellite altimetry, observing system simulation experiment
Citation: Benkiran M, Le Traon P-Y, Rémy E and Drillet Y (2024) Impact of two high resolution altimetry mission concepts on ocean forecasting. Front. Mar. Sci. 11:1465065. doi: 10.3389/fmars.2024.1465065
Received: 15 July 2024; Accepted: 11 November 2024;
Published: 12 December 2024.
Edited by:
Virginie Van Dongen-vogels, Australian Institute of Marine Science (AIMS), AustraliaReviewed by:
Shuhei Masuda, Japan Agency for Marine-Earth Science and Technology (JAMSTEC), JapanMatthew Carrier, Naval Research Laboratory, United States
Copyright © 2024 Benkiran, Le Traon, Rémy and Drillet. This is an open-access article distributed under the terms of the Creative Commons Attribution License (CC BY). The use, distribution or reproduction in other forums is permitted, provided the original author(s) and the copyright owner(s) are credited and that the original publication in this journal is cited, in accordance with accepted academic practice. No use, distribution or reproduction is permitted which does not comply with these terms.
*Correspondence: Mounir Benkiran, bWJlbmtpcmFuQG1lcmNhdG9yLW9jZWFuLmZy