- 1Hakai Institute, Campbell River, BC, Canada
- 2Institute of Ocean Sciences, Fisheries and Oceans Canada, Sidney, BC, Canada
- 3LCJL Marine Ecological Services, Sidney, BC, Canada
Rapid environmental change is altering coastal phytoplankton dynamics and, thereby the productivity of coastal marine food webs. Unfortunately, a paucity of phytoplankton community data hinders the prediction of future conditions in ecologically productive regions such as the coastal northeast Pacific. To help fill this gap, this study characterized phytoplankton communities from 2018 to 2020 across a fjord, channel and shelf station transect on the central coast of British Columbia, Canada. Monthly samples were collected for microscopy-based taxonomy and pigment-based phytoplankton composition (i.e. CHEMTAX and size-fractionated chlorophyll). Correlation analysis was used to investigate drivers of phytoplankton biomass and hierarchical clustering and redundancy analysis highlighted drivers of compositional trends. Spring blooms formed the peak of annual biomass at each station and earlier blooms at the fjord station suggested a sheltering effect from winter wind conditions. Later spring blooms at the channel station coincided with seasonal wind reductions and increased sunlight. Of the six derived compositional clusters, three represented flagellate dominated conditions at all stations: two represented low biomass winter conditions and the third, moderate biomass spring and autumn blooms occurring under nutrient replete conditions. The remaining three clusters were diatom-dominated and spanned much of the growing season. The first diatom cluster represented Skeletonema marinoi dominated samples, many from 2020, observed under moderate nutrient and high stratification and freshwater discharge conditions. The second represented high diatom richness spring bloom conditions at all stations that were associated with nutrient depletion. Finally, the third included 2018 and 2019 summer shelf samples showing harmful Rhizosolenia setigera and Pseudo-nitzschia seriata blooms under high surface water salinity and temperature. These results highlight high spatial-temporal variability and sensitivity of coastal northeast Pacific phytoplankton communities to altered freshwater, temperature and wind dynamics with potential for profound ecosystem level implications.
1 Introduction
The interplay between terrestrial and marine processes creates strong environmental gradients across coastal systems which drive high variability in phytoplankton biomass, community composition and size structure (Strom et al., 2006; Carmack et al., 2015; Sejr et al., 2022; Cavalcanti-Lima et al., 2023; Olli et al., 2023). Understanding the complexity of these systems and their influence on phytoplankton communities is essential considering that phytoplankton form the base of marine food webs and play key roles in biogeochemical cycling and carbon sequestration (Arrigo, 2005; Winder and Sommer, 2012; Tréguer et al., 2018). The high turnover rates of phytoplankton species make them ideal sentinels of environmental change as they quickly respond to perturbations; however, a paucity of data, notably in terms of species composition, exists across coastal systems hindering the derivation of baseline conditions to assess change (Cloern and Jassby, 2008; Winder and Sommer, 2012; Henson et al., 2021; Horn et al., 2021; Longobardi et al., 2023). This knowledge gap is especially pertinent when considering that coastal regions are experiencing rapid climate-driven change including increased temperature and acidification, reduced oxygen and altered freshwater dynamics (Harley et al., 2006; Hare et al., 2020; Bidlack et al., 2021; Horn et al., 2021). The synergy of these influences has the potential to alter phytoplankton community and size structure having large downstream implications on ecosystem resiliency, food production and climate regulation (Marañón et al., 2012; Henson et al., 2021).
Phytoplankton include a complex array of species varying greatly in size, spanning nine orders of magnitude, and ecological roles. Broadly, diatoms are the primary bloom forming coastal species and are generally large and energy rich. These traits allow for grazing by large zooplankton species, shortening food web length and promoting efficient energy transfer to upper trophic levels supporting highly productive ecosystems (Carstensen et al., 2015; Lerner et al., 2022). In addition, their large size, high carbon content and silica shells enhance vertical export making them a key driver of carbon export and silica cycling (Tréguer et al., 2018; Taucher et al., 2022). In contrast, small flagellates and cyanobacteria are associated with less efficient food-web energy transfer and reduced carbon export (Mouw et al., 2016; Schmidt et al., 2020; Sejr et al., 2022). Furthermore, some phytoplankton species produce toxins resulting in notable economic loss and public health concerns (Hallegraeff, 2010; Trainer et al., 2020). High diversity within these broad classifications (e.g. diatoms and flagellates) necessitates intensive investigations using multiple approaches to fully characterize phytoplankton communities.
Traditionally, optical microscopy has been used to classify and enumerate cells to the lowest possible taxonomic level, but is biased towards larger species and provides outputs in abundance rather than biomass. Methods exist to convert abundance to biomass using geometric shape, either through direct measurement or published conversion values, but these are time consuming and prone to error from a variety of sources including fixative distortion, morphological complexity and analyst bias (Álvarez et al., 2014; Menden-Deuer et al., 2020). Despite these drawbacks, optical microscopy remains a cornerstone of phytoplankton research largely as a result of ongoing long-standing time series (Widdicombe et al., 2010; Longobardi et al., 2023). In an attempt to estimate biomass along with community composition, pigment-based approaches leverage differences in phytoplankton marker pigments, derived via high performance liquid chromatography (HPLC), to estimate total chlorophyll a (TChla) biomass of broad phytoplankton groups (e.g. chemotaxonomic analysis - CHEMTAX) (Mackey et al., 1996). This method is advantageous as it simultaneously characterizes the full-size spectrum of phytoplankton, provides a biomass estimate and, while not as taxonomically resolved as microscopy, output groupings generally represent species with comparable functional traits (Mutshinda et al., 2016; Del Bel Belluz et al., 2021). Finally, phytoplankton size-structure is often determined through the fractionation of chlorophyll a (Chla) into three size classes, pico (ChlaPICO, < 3um), nano (ChlaNANO > 3 and < 20 um) and micro-plankton (ChlaMICRO > 20 um), providing important biomass measures for food web efficiency and cell sinking rates (Brewin et al., 2014; Mouw et al., 2016; Lerner et al., 2022; McLaskey et al., 2022).
In this study, we investigate trends in phytoplankton community composition on the central coast of British Columbia. This area is highly dynamic being located within the North American Pacific Fjordland marine ecoregion (Spalding et al., 2007), the Northeast Pacific Coastal Temperate Rainforest (NPCTR) and the transition zone between upwelling and downwelling dominant wind conditions (Foreman et al., 2011) (Figure 1). While seasonal trends in phytoplankton biomass on the central coast of British Columbia have been characterized, most observations are derived from large spatial-scale satellite surface chlorophyll analysis that lack compositional information. In British Columbia, the majority of phytoplankton compositional studies have been concentrated within the Salish Sea or the oceanic northeast Pacific (Harrison et al., 1983; Taylor et al., 1994; Hobson and McQuoid, 1997; Peña et al., 2019b; Del Bel Belluz et al., 2021; Nemcek et al., 2023) and in the coastal Gulf of Alaska, similar studies have been concentrated near Prince William Sound (Strom et al., 2006; Batten et al., 2018; Du et al., 2023). Comparatively few studies have been conducted over the British Columbia central coast, with this area representing a significant data gap (Peña et al., 2019a). The most detailed contemporary investigation of phytoplankton taxonomy and size was done by Peterson et al. (2007) in the coastal waters of Haida Gwaii, which are geographically distant and less influenced by continental glacierized watersheds when compared to the waters studied here. This paucity of data is troubling as this area is of high importance to a wide array of species whose survival have been linked to phytoplankton (e.g. Pacific Salmon and seabirds) and is experiencing considerable change as a result of marine heatwaves (Di Lorenzo and Mantua, 2016; Jackson et al., 2018; Amaya et al., 2020; Xu et al., 2022), altered freshwater inputs (Bidlack et al., 2021) and upwelling dynamics (Foreman et al., 2011).
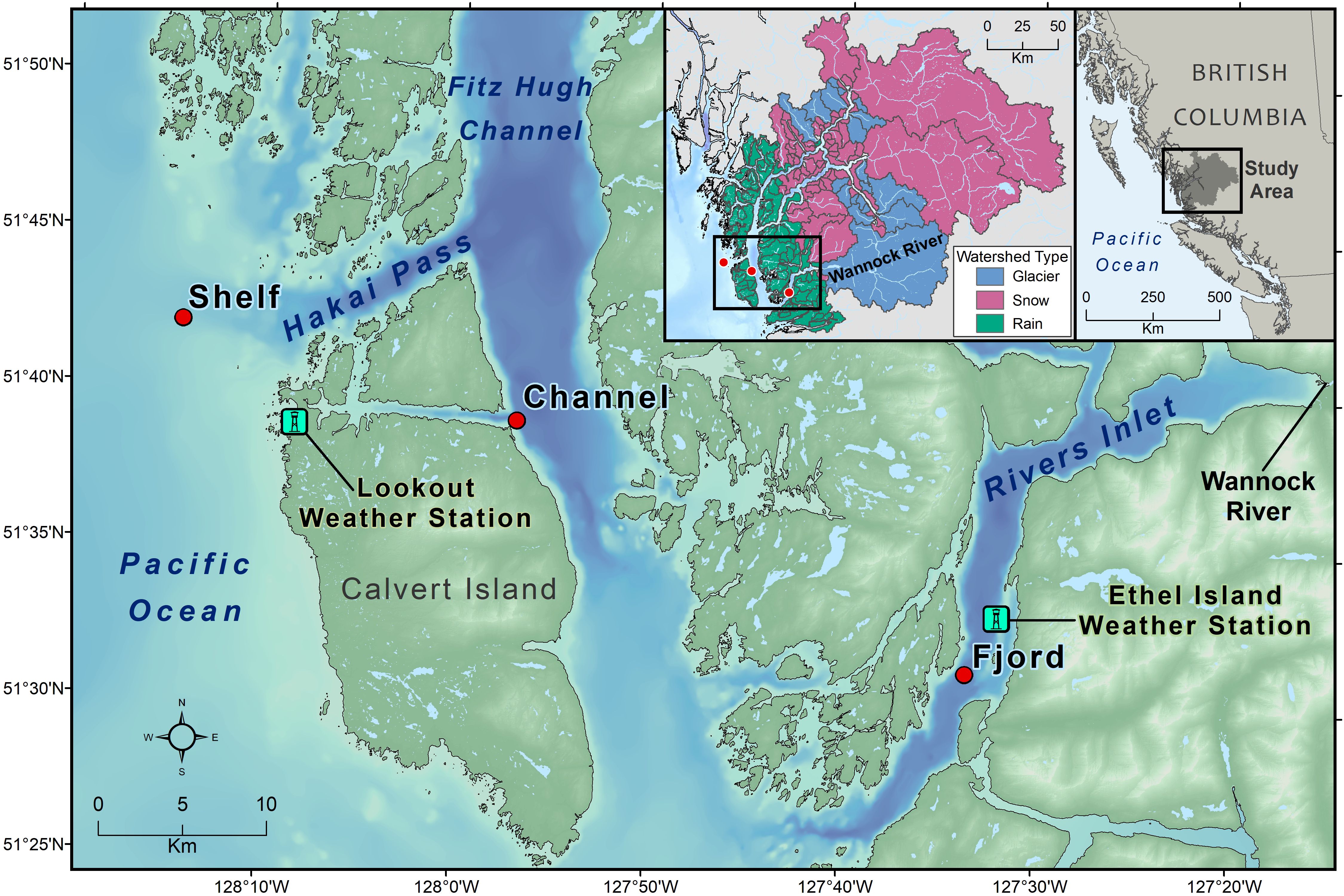
Figure 1. Study Area map showing the location of the three sampling stations along the fjord to shelf gradient starting with the fjord station within Rivers Inlet, channel station within Fitz Hugh Channel and the shelf station outside of Hakai Pass. The main map also shows the location of the two utilized weather stations: The Lookout on the west coast of Calvert Island and Ethel Island within Rivers Inlet. The insets show the watersheds types used for modelling discharge (rain-type = green, snow mountain-type = pink and glacierized mountain-type = blue) and the location of the study area on the central coast of British Columbia, Canada, situated in the northeast Pacific.
Freshwater sources to the studied area span coastal low elevation rain-driven systems to high elevation glacierized watersheds (Giesbrecht et al., 2022) with this diversity resulting in near continuous coastal freshwater introduction and a riverine coastal domain (Carmack et al., 2015). Seasonally, winter is characterized by high rainfall (annual rainfall > 3000 mm) coupled with strong downwelling favorable southeasterly winds driving mixing and the retention of coastal surface waters (Whitney et al., 2005). The spring transition to lower velocity upwelling favorable northwest winds occurs between March and May (Whitney et al., 2005). At this time, rainfall decreases and freshwater contributions are driven by high discharge from glacierized mountain-type watersheds from late spring through summer (see review within St. Pierre et al., 2022). In autumn, high winds return and intense autumn rain events associated with atmospheric rivers can produce synchronous high discharge across the diverse watershed types (Korver et al., 2022). Both wind and freshwater conditions strongly influence phytoplankton biomass and seasonality on the central coast of British Columbia.
Overall, phytoplankton production on the central coast is thought to be moderate when compared to other coastal British Columbia waters such as the Vancouver Island shelf and the Salish Sea (Jackson et al., 2015; Peña et al., 2019a). Broadly, winter biomass is low due to light limitation and strong mixing with annual spring blooms thought to coincide with the spring wind transition and increased freshwater driven buoyancy from the annual freshet (Jackson et al., 2015; Marchese et al., 2022; St. Pierre et al., 2022; Pramlall et al., 2023). In summer, freshwater driven stratification and low wind energy can result in surface nutrient limitation and reduced phytoplankton biomass (Chla ~2 mg m-3); however, localized mixing from tidal energy and/or wind events can resupply nutrients to the surface layer spurring large diatom blooms (Whitney et al., 2005; Jackson et al., 2015; Marchese et al., 2022). In autumn, the return of strong winds breaks stratification and during this time, autumn blooms periodically develop prior to the return to low biomass winter conditions (Jackson et al., 2015; St. Pierre et al., 2022).
In this work, we sought to improve regional understanding of phytoplankton community dynamics traversing a fjord to shelf gradient characteristic of the central coast of British Columbia. While analysis was largely centered on genus-species level abundances derived from optical microscopy, the results were complemented by functional group TChla biomass derived via CHEMTAX and sized fractionated Chla concentrations. Our objectives were to: 1) characterize the observed phytoplankton communities over a multiyear time series (2018-2020); 2) explain spatial-temporal variability of phytoplankton biomass and dominant species and; 3) leverage environmental data to investigate potential drivers of phytoplankton biomass and species composition. This work provides a baseline for continued phytoplankton studies and adds valuable knowledge to a data scarce, but biologically and biogeochemically important, area.
2 Methods
2.1 Study area
The three stations investigated in this study have strong freshwater influences from a variety of watershed types (St. Pierre et al., 2022). First, the shelf station (51.70°N and 128.24°W) was located approximately 150 km eastward of the shelf break at the head of Hakai pass connecting it to the inner coastal waters of Fitz Hugh Sound. Second, the channel station (51.65°N and 127.95°W) was located within Fitz-Hugh sound, which is a deep channel spanning the outlet of Rivers Inlet to the south and the outlets of the large Dean, Burke and Fisher channels to the north. Third, the fjord station (51.52°N and 127.56°W) was located within Rivers Inlet, which is a 45 km long and 340 m deep fjord separated from Fitz-Hugh sound by a 140 m sill. Discharge and estuarine circulation within this fjord are driven by glacial and snow-melt entering from the Wannock River (Hodal, 2011) and other watersheds in combination with runoff from fall and winter rain events across many smaller watersheds at lower elevations.
2.2 Sampling regime
From 2018 through 2020, monthly field sampling was conducted at each station; however, due to the remote nature of the study area and dynamic weather conditions, sampling was often limited, notably during winter and early spring at the exposed shelf station. Furthermore, the 2020 COVID-19 pandemic limited sampling at all stations for two months during the spring 2020 season. Sampling dates for each location and the available data for each sampling event are shown in Supplementary Tables S1-S3.
During each sampling event, water samples for size-fractionated chlorophyll, HPLC derived phytoplankton pigments, protist microscopy, and nutrients were collected with Niskin bottles in conjunction with CTD profiles. All water samples were single replicates and were collected and matched with CTD temperature and salinity at 5m depth.
2.3 Discrete sampling
2.3.1 Nutrients
Dissolved nitrogen (nitrate + nitrite (NO3- + NO2-, DIN), phosphate (PO4-3, DIP) and silicate (Si(OH)4, DSi) were collected and stored frozen at -20°C. Samples were analyzed at the University of British Columbia using a Lachat QuikChem 8500 Series 2 Flow Injection Analysis system with concentrations reported as μmol L-1. Sample thawing, which is important for the depolymerization of reactive silica, was performed following the method recommended in the GO-SHIP nutrient manual (30 min water bath at 50°C) (Becker et al., 2020). Limits of detection for DIN, DIP and DSi were 0.036, 0.032 and 0.100 μmol L-1, respectively, and accuracy was < 1%RSD as defined through comparison with analytical standards. Accuracy and precision statistics were maintained to those defined for the analysis system provided in Smith and Bogren (2003); Knepel and Bogren (2008) and Tucker (2010), for DIN, DIP and DSi, respectively.
2.3.2 Size-fractionated chlorophyll data
Water samples for fluorometric determination of size-fractionated chlorophyll (Chla) concentrations were transferred to opaque Nalgene bottles and stored in a dark cooler until filtration under subdued light within 1-2 hours of collection. Samples (250 mL) were consecutively filtered through 47mm filters starting with a 20-μm polycarbonate filter, followed by a 3 μm polycarbonate filter and ending with a GF/F filter for the determination of ChlaMICRO (> 20 μm Chla), ChlaNANO (3 – 20 μm Chla) and ChlaPICO (< 3 μm Chla), respectively. Filters were individually placed into Falcon tubes and stored at -80˚C until analysis using an annually calibrated Turner Trilogy fluorometer. Pigment extraction was done with HPLC grade 90% acetone (24 hours) and analysis was performed following the acidification method (Holm-Hansen et al., 1965). Bulk Chla (ChlaBULK) samples (single 47mm GF/F) were also collected and analyzed following the same method. Comparison of ChlaBULK and size-fractionated sum Chla (ChlaSUM) showed good correspondence with a slight underestimation by the size-fractionated estimate (ChlaBULK = 0.85ChlaSUM - 0.15, R2 = 0.82, p <0.001).
2.3.3 HPLC and CHEMTAX
Phytoplankton pigment samples for HPLC analysis were only available for 2019 and 2020. After collection via Niskin bottle, 1L of water was vacuum filtered under subdued light through a 47mm GF/F. The filter was then folded inwards, blotted to remove excess water, wrapped within aluminum foil and then stored at -80°C until analysis. Analysis was performed at the University of South Carolina Baruch Institute using the USC method (Hooker et al., 2010). Phytoplankton pigment concentrations were then input into CHEMTAX (v1.95) which applies matrix factorization and a steepest descent algorithm to estimate the biomass contribution of the main phytoplankton groups in terms of total chlorophyll a (TChla) (Mackey et al., 1996). Input pigments, pigment ratios, and phytoplankton groups were the same as Del Bel Belluz et al. (2021) in the northern Salish Sea with the exception of the inclusion of a raphidophyte group and are shown in Table 1. This group was included based on observations of raphidophyte species and ratios were derived from Lewitus et al. (2005). Similar to Del Bel Belluz et al. (2021) an initial run was done on the full dataset to optimize the pigment ratios to local conditions. Data were then clustered following Swan et al. (2016) to group samples with similar pigment ratios to help standardize for differences as a result of environmental conditions (Supplementary Figure S1). Clustering resulted in 3 groupings; however, one grouping consisted of a single outlier sample and it was necessary to place this sample with cluster-2 for analysis. CHEMTAX analysis was then performed on each cluster independently using the output ratios of the initial run performed on the full dataset.
In addition to derivation of group compositions, HPLC-derived TChla was used as the primary measure of phytoplankton biomass throughout the study. HPLC TChla was not available in 2018, but otherwise had greater data coverage than ChlaBULK in the following years. As a result, TChla was compared against ChlaBulk for 2019 and 2020 (Supplementary Figure S2) and the derived relationship (Chla = 1.24TChla – 0.04, R2 = 0.86, p <0.001) was then used to convert the 2018 ChlaBulk concentrations into TChla approximations. This approach resulted in the most comprehensive and consistent time series for comparison with the other variables.
2.3.4 Phytoplankton microscopy
Samples for the enumeration of planktonic protist (included phytoplankton and heterotrophic microzooplankton) abundance were collected in 250 mL amber glass bottles, immediately fixed using Lugol’s solution (1% concentration) and then stored at 4˚C in a dark fridge until analysis. Analysis was performed at LCJL Marine Ecological Services following the Utermöhl method using 50 mL settling chambers and an inverted light microscope (Utermöhl, 1931). Cells were identified to the lowest possible level and reported in cells L-1. Species identifications were based on Hasle and Syvertsen (1996) for diatoms, Steidinger and Tangen (1996) for dinoflagellates and Throndsen (1993) and Smith (1991) for other flagellates. Analyst taxa names were matched to the World Register of Marine Species (WORMs) database, and the most recent accepted taxonomic designations on this database were reported. For the observed species, Pseudo-nitzschia spp. enumerations were separated into three species complexes (P.multiseries, P.delicatissima and P.seriata) according to a regional library (Hobson, 2009); however, it is not possible to accurately separate these morphologically similar species with light microscopy (Peterson et al., 2007; Dodrill et al., 2023; Perry et al., 2023). As a result, species identified as P.multiseries and P.delicatissima, which were similar in size (girth = 1 and 2 μm, respectively and length = 30 – 40 and 20 – 30 μm, respectively) and may have been morphological variants of the same species were summed and included as Pseudo-nitzschia spp. Distinctively larger cells (girth = 10-15 μm and length = 150-160 μm) were classified as being within the Pseudo-nitzschia seriata complex and counts for this species were kept separate. Similar size differentiation has been done by other authors providing useful knowledge of Pseudo-nitzschia ecology (Houliez et al., 2023).
2.4 CTD data
CTD profiles were collected with either a Sea-Bird 19+ or RBR Maestro system and over the course of the study 83 profiles were examined. CTD data were processed and 1-m binned using the Sea-Bird Scientific Seasoft software and the RBR processing steps outlined in Halverson et al. (2017), respectively. All utilized data are available at www.cioospacific.ca. Water Density was calculated using the R GSW package. Water stratification was assessed using the difference in density between 30 and 2 m depths (Δp, kg m-3) guided by use for phytoplankton studies within the Salish Sea and across coastal-shelf gradients in Prince William Sound, Alaska (Del Bel Belluz et al., 2021; Du et al., 2023). Visualization of CTD chlorophyll fluorescence profiles showed that the bulk of phytoplankton biomass was above 30m at each station regardless of season (Supplementary Figure S3).
2.5 Ancillary weather data
Wind speed (m s-1), direction and incoming photosynthetically active radiation (PAR mol m-2) data were collected at the Hakai Sensor Network “Lookout” station (51.65°N and 128.14°W, Figure 1), which was located at 43m elevation on the western side of Calvert Island. This station was selected to provide a general representation of local incoming wind conditions less influenced by terrain effects when compared to weather stations closer to the channel and fjord sampling stations (Hodal, 2011). Wind data from the “Ethel Island” station (51.55°N and 127.53°W, Figure 1) within Rivers Inlet near the fjord station, were also assessed with wind speeds correlated to the Lookout station (R2 = 0.28, windETHEL = 0.28windLOOKOUT + 1.6, p <0.001), but with lower magnitudes (Supplementary Figure S4). These data were not included in multi-variate statistical analysis due to their relevance to only the fjord station and their correlation with the Lookout station wind data.
When compared to field samples, wind, PAR and upwelling index data from 51°N and 131°W (https://coastwatch.pfeg.noaa.gov/erddap/tabledap/erdUI516hr.html were averaged over the three days prior to each sample collection event (Del Bel Belluz et al., 2021; Du et al., 2023). In turn, discharge was averaged over the prior 10 days to sample collection: surface residence times within Rivers Inlet have been found to be discharge dependent and generally fall between 5-10 days over the spring-summer period (Hodal, 2011). Here, significant correlations where found between 10-day backward average discharge and salinity/stratification at each station suggesting that this averaging period captured dominant trends in the data (Supplementary Figure S5). Comparable backwards averages for discharge have been used by other authors (Dodrill et al., 2023; Goñi et al., 2023).
2.6 Freshwater discharge estimates
The study area (~28,000 km2 of watershed area) has a sparse network of stream gauges available to characterize discharge from the diverse range of watersheds and climatic conditions. To generate a daily estimate of total freshwater discharge including ungauged areas, we developed a set of simple discharge models based on watershed characteristics (Supplementary Table S4). Gauged discharge data were acquired from the Water Survey of Canada database (tidyhydat in R) and from the Hakai Institute’s observatory watersheds in Kwakshua Channel (Giesbrecht et al., 2021; Korver et al., 2022). A large data gap in 2018 at gauge 08FB011 in the Bella Coola watershed was filled using a linear relationship with daily discharge at the Kliniklini River gauge (08GE002) (R2 = 0.90, p <0.001).
Earlier research across the NPCTR region showed that streamflow regimes and mean annual discharge vary with watershed characteristics including watershed type (Giesbrecht et al., 2022). In this study, we used these watershed-discharge relationships to assign donor gauges to all ungauged zones (groups of watersheds) with similar watershed characteristics and geographic location (Supplementary Table S4). Watershed characteristics – such as mean annual precipitation, precipitation as snow, maximum elevation, area, and % glacier cover – were taken from Giesbrecht et al. (in review, Ecosystems). After generating modelled discharge estimates for all 9 zones, we summarized discharge from three broad watershed types: rain, snow mountain, and glacierized mountain-type watersheds.
2.7 Statistical analysis
Hierarchical clustering was performed using the R “vegan” package on the microscopy-based planktonic protist abundance data to investigate taxonomic groupings and underlying structures (Oksanen et al., 2019). Prior to clustering, rare species (not present in at least 10% of samples) were removed to minimize the influence of double-zeros on the statistical analysis and the remaining abundance counts were then square root transformed (Legendre and Legendre, 2012; Krawczyk et al., 2015; Dodrill et al., 2023; Du et al., 2023). This filtering removed Chatonella/Heterosigma species, which formed a single bloom, and 49 other species that were low in prevalence (present in, on average, only 4% of the samples) and low in abundance, never exceeding 0.35 x 104 cells L-1. A Bray-Curtis distance matrix was then derived from the transformed abundance counts, clustering was performed with the complete linkage method and a > 33% similarity (67% dissimilarity) distance was utilized to define clusters. The complete linkage method was chosen as it provided well defined clusters that weren’t achievable with other approaches while the similarity threshold was selected based on visualization of the dendrogram, significant indicator values and groupings that made ecological sense (McLaskey et al., 2022). Similar thresholds have been used on phytoplankton and protist data along the coastal northeast Pacific (Peterson et al., 2007; Dodrill et al., 2023; Du et al., 2023) and confidence in our method was provided by good cluster separation in ordination space (via both non-metric multidimensional scaling and redundancy analysis) (Clarke and Warwick, 2001). Significance of the resulting taxonomic clusters was assessed using ANOSIM which provides a rank coefficient (R) ranging from 0 to 1 with values closer to 1 indicating greater differences and better separation between clusters (Du et al., 2023).
Indicator species analysis using the R “ISA” function (“indicspecies” package) was performed on the output taxonomic clusters to identify taxa that were driving differences between clusters. This analysis considers both the abundance and frequency of species within each cluster and outputs an indicator species value (ISV) for each derived indicator species. The ISV ranges between 0 and 1 with values closer to 1 indicating that the species was more prominent and at a higher abundance within the cluster when compared to the other groupings. Only significant indicator taxa that had an ISV > 0.25 were included (Dufrene and Legendre, 1997; Tommasi et al., 2013).
Two approaches were utilized to investigate links between environmental drivers, phytoplankton biomass and species abundances across the time series. First, non-parametric Spearman’s rank correlations were used to investigate relationships between environmental parameters and TChla, size-fractionated Chla and CHEMTAX ouputs. This method was used as many of the observations failed to meet the assumptions required for Pearson correlations. Scatterplots were visually inspected for monocity and extreme outliers and p-values were corrected for multiple comparisons using the Benjamini and Hochberg (FDR) correction (Benjamini and Hochberg, 1995). Correlations (rs) were considered significant at padj <0.05. Second, redundancy analysis (RDA) was employed using the R package “vegan” to investigate environmental drivers of phytoplankton community composition (Oksanen et al., 2019). Redundancy analysis is a constrained ordination technique that is commonly used in ecological studies including several from coastal British Columbia (Peterson et al., 2007; Ramette, 2007; Del Bel Belluz et al., 2021; Esenkulova et al., 2021; Nemcek et al., 2023). This analysis was performed on the microscopy-based planktonic protist abundances and similar to the clustering analysis, only species observed in > 10% of all samples were used to limit the influence of double-zeroes in the analysis. Separate RDA were independently performed on the full remaining taxonomic suite and then, just on diatom species to highlight diatom-specific correlations partially obscured by the inclusion of other taxa. Input explanatory variables were temperature, salinity, stratification, nutrients, secchi depth, 3-day backwards mean windspeed, direction and upwelling index and 10-day backwards mean modelled discharge from the glacierized mountain, snow mountain and rain-type components. These variables were standardized prior to analysis to account for large differences in range as a result of differing units. Response variables (protist abundance counts) were Hellinger transformed prior to analysis to increase suitability for the linear-based RDA and to give groups with low or zero biomass reduced weights (Legendre and Gallagher, 2001; Ramette, 2007). Once transformed, explanatory variables with variance inflation factors > 8 were removed and RDAs were performed including all remaining explanatory variables to check for global significance (p <0.001 after 9999 permutations) which is required for further investigation using forward selection of explanatory variables (McLaskey et al., 2022). Once significance was determined, forward selection was performed utilizing the OrdiR2step function that determines significant explanatory variables (p <0.05) using Monte Carlo permutations (n = 9999) and correcting for overestimations of variances (Blanchet et al., 2008). Redundancy analysis was then performed on the significant variables.
3 Results
3.1 Environmental variability
Environmental parameters were highly variable but showed both seasonal and spatial patterns (Figure 2). Seasonally, 5m depth temperature increased from a minimum in January and February to maxima in August and September at all stations, with the fjord showing the lowest temperatures (median = 9.41, 6.74 – 10.82°C) and the shelf the highest (11.56, 6.50 – 16.12°C, median skewed high due to a lack of winter data, but temperature maxima were typically highest at shelf). In turn, each year showed 5m depth salinity decreases in spring and summer with lower minimum salinities observed in the fjord (29.48, 24.99 – 30.79) and channel (29.95, 26.97 – 31.35) and higher salinities at the shelf (31.09, 29.94 – 32.20). Opposite patterns were observed in stratification with the highest stratification observed in the fjord (Δρ, 7.35, 0.64 – 18.44 kg m-3) and decreasing values at the channel (2.19, 0.15 – 8.18 kg m-3) and shelf (1.30, 0.15 – 2.27 kg m-3). Furthermore, annual spring season nutrient drawdown occurred at each station and nutrients were always highest within the fjord where DIN was > 3.96 μmol L-1 (11.40, 3.96 – 19.52 μmol L-1, Supplementary Figure S6), DIP was > 0.43 μmol L-1 (1.06, 0.43 – 1.59 μmol L-1, Supplementary Figure S6) and DSi was > 15 μmol L-1 with exception to a single low concentration (25.00, 0.98 – 33.72 μmol L-1, Figure 2) that occurred during moderate DIN conditions (DSi:DIN = 0.21). All nutrient measures were highly correlated at each station (R2, DIN to DSi > 0.78 and DIP to DIN > 0.96) broadly showing DSi and DIP in excess of DIN (Redfield et al., 1963, 16:16:1 for DSi:DIN:DIP, respectively, Supplementary Figure S7).
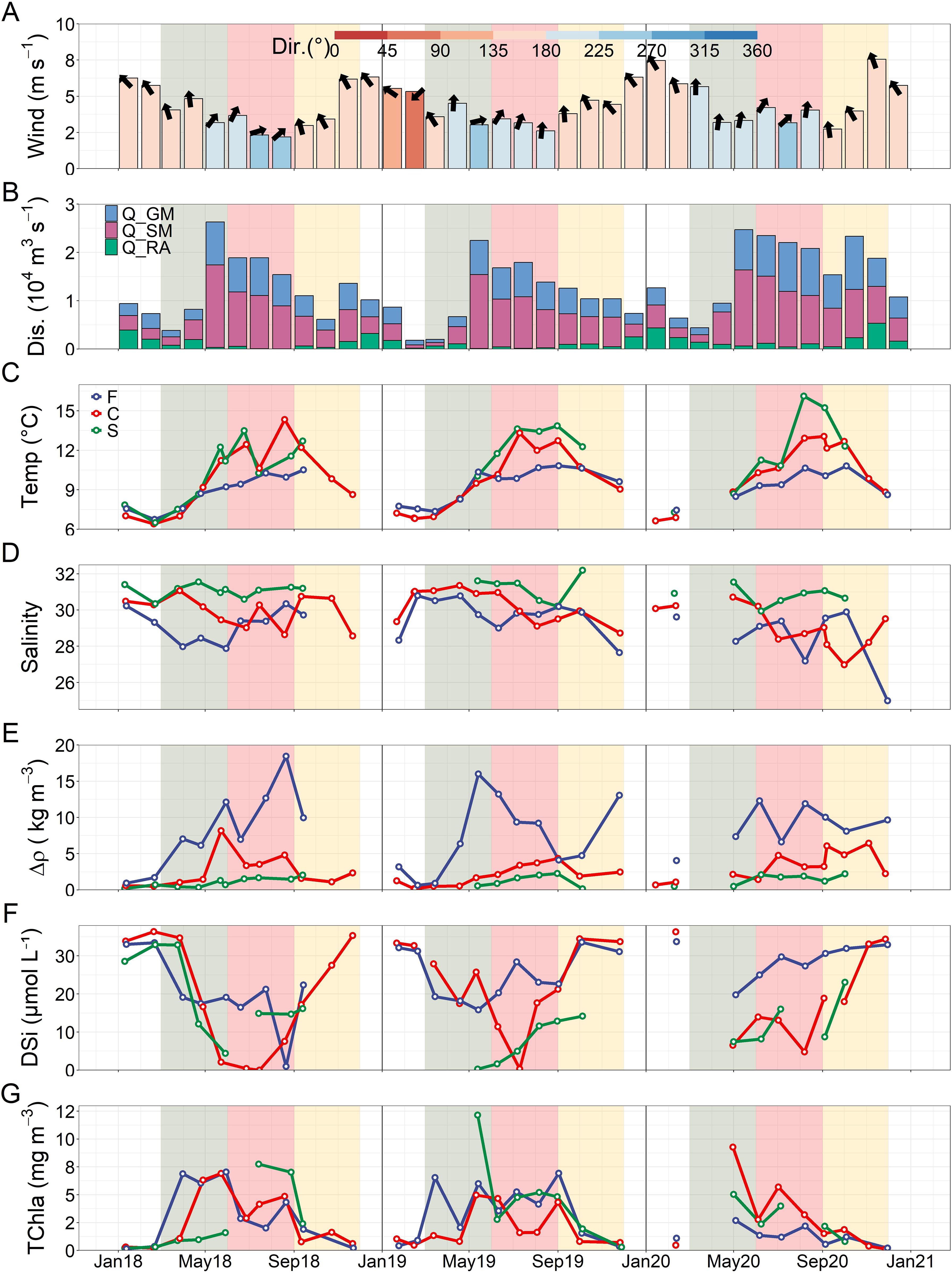
Figure 2. Time series (2018 – 2020) of monthly median (A) windspeed from the Lookout station with the colors of the bars and arrows representing the wind direction; (B) total discharge (Dis.) with stacked bars representing contributions by the different watershed types: Glacierized Mountain (Q_GM), Snow Mountain (Q_SM) and Rain-types (Q_RA). Median values were displayed for discharge data to be consistent with the wind data and due to the presence of short-duration high discharge events (Supplementary Figure S8). In C through G, monthly point measurements, separated by station (Fjord, F = blue, Channel, C = red and Shelf, S = green), are plotted for (C) 5m depth temperature (°C); (D) 5m depth salinity; (E) stratification as Δρ (kg m-3); (F) 5m depth dissolved silicate (DSi, μmol L-1) and; (G) 5m depth total Chlorophyll-a (TChla, mg m-3). The shaded background represents meterological seasons with spring (green = March, April , May), summer (red = June, July, August) and autumn (yellow = September, October, November).
Freshwater discharge had large influences on oceanographic properties showing significant correlations with stratification, but with different relationships at each station. Specifically, the fjord showed a significant positive correlation between 10-day backwards averaged total discharge and stratification (R2 = 0.46, Δρ = 0041discharge10-Day + 1.2, p <0.001), with a higher slope than those at the other stations (i.e. higher stratification per discharge amount). At the channel, a lower slope (R2 = 0.56, Δρ = 0014discharge10-Day + 0.13, p <0.001) was found, whereas the weakest correlation and lowest slope was observed at the shelf (R2 = 0.32, Δρ = 0005discharge10-Day + 0.22, p = 0.005). When broken into individual watershed components, discharge from rain-type watersheds, which often peaked in autumn and winter when rainfall and wind mixing was high, was not correlated with stratification at any station (Supplementary Figure S5).
Notable differences in freshwater and environmental data were observed between stations both seasonally and interannually. In 2018, annual and seasonal discharge was moderate when compared to the other years, but a high median May value was observed representing a strong freshest (2656 m3 s-1, Table 2, Figure 2). This high May discharge value corresponded with decreased salinity and increased stratification at the channel and shelf (the fjord showed earlier freshwater signals) and the time series maxima stratification for the channel station (8.18 kg m-3). In summer 2018, discharge remained moderate and lacked the episodic high discharge events seen in other years (Supplementary Figure S8), with the fjord and channel showing variable, but generally low salinity and high stratification and the shelf showing comparatively less freshwater influence. The lack of high episodic discharge events in summer 2018 corresponded with a period of sunny and calm wind conditions in July and August resulting in the lowest summer season median wind speed (2.55, 1.11 – 8.76 m s-1) and highest PAR (1.62, 0.27 – 2.55 mol m-2) and upwelling index (30.17, -247.85 – 180.67 m3 s-1) (Table 2).
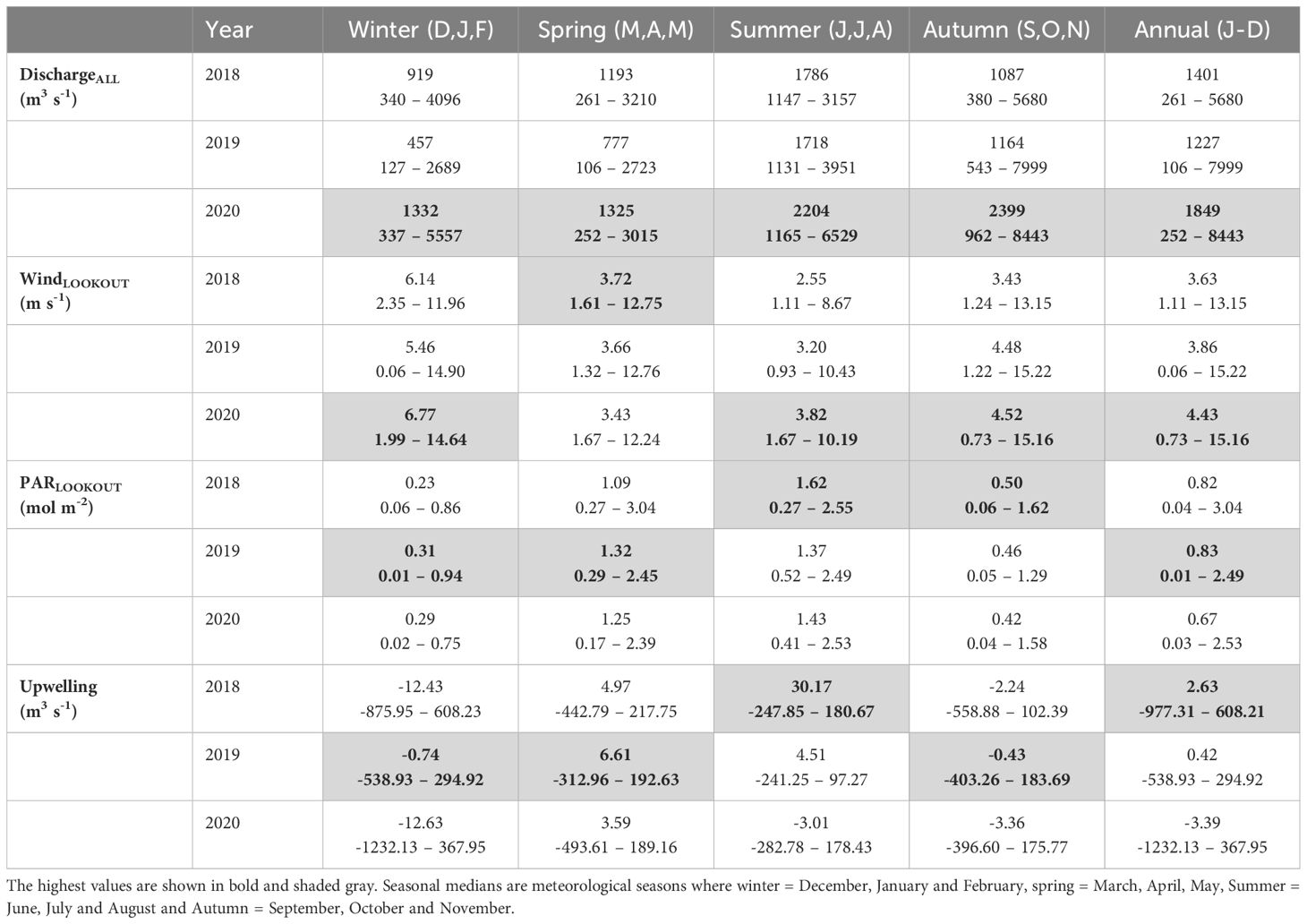
Table 2. Seasonal and annual median, minimum and maximum values for each year for modelled total freshwater discharge (summation of all discharge types), wind speed (Lookout station), incoming PAR (Lookout station) and upwelling index.
Of the three years, 2019 had the lowest early season freshwater influence with all stations showing high salinity through much of spring and early summer: freshwater arrival tended to follow the fjord to shelf gradient with reduced salinity first observed at the fjord in May (29.00), channel in July (29.84) and shelf in August (30.21) (Figure 2). This high early season salinity occurred in conjunction with low February and March monthly median discharge (195 and 478 m3 s-1, respectively) and seasonal winter and spring discharge being considerably lower than in the other years (Table 2). Interestingly, when compared to the other years, 2019 showed pronounced late summer season freshwater arrival at the channel (early August) and shelf (late August) following episodic discharge events not observed in 2018 (Figure 2; Supplementary Figure S8).
Early spring oceanographic data was largely missing in 2020, but following May, this year showed lower median annual incoming PAR and upwelling index magnitudes and higher wind and discharge conditions when compared to the prior years (Table 2). Seasonal discharge was noticeably high during summer and was driven by multiple large episodic discharge events which corresponded with time series minimum salinities at most stations, but most notably at the channel where values were < 30 throughout summer (Figure 2; Supplementary Figure S8).
3.2 Seasonal trends in TChla
Distinct differences in spring bloom timing (TChla > 5 mg m-3 or spring season maxima when threshold not exceeded) were observed between the fjord, channel and shelf stations (Figure 2). With monthly sampling it was not possible to accurately determine spring bloom initiation; however, in 2018 and 2019, the fjord showed spring bloom conditions at the end (2018-03-31, 6.88 mg m-3) and middle (2019-03-15, 6.54 mg m-3) of March, respectively, while blooms at the channel were first observed in late April (2018-04-28, 6.32 mg m-3) and early May (2019-05-11, 4.98 mg m-3). At the fjord, spring blooms occurred prior to seasonal decreases in wind speed and changes in direction at the Lookout weather station with lower wind speeds and less seasonal variability observed within the fjord at Ethel Island station (Supplementary Figure S4). In contrast, the channel blooms followed seasonal transitions to lower wind speeds (Lookout station) and closer to the annual freshet (Figure 2). Due to a lack of early season data it was difficult to assess conditions at the shelf, but in 2018 (only year with complete winter and spring data) the first bloom was observed in July (2018-07-14, 7.74 mg m-3), despite nutrient drawdown in May, suggesting the spring bloom occurred earlier. In 2019, the first observed bloom at the shelf occurred in conjunction with the channel spring bloom and was the time series maximum TChla (2019-05-13, 12.16 mg m-3). In 2020, spring data were largely missing, but the channel and shelf showed blooming conditions in late April/early May while the fjord showed a marginal TChla concentration that was nonetheless the annual maxima (2020-05-04, 2.68 mg m-3). With a single exception (2019 fjord autumn bloom – next paragraph), spring blooms always represented the seasonal maxima TChla at each station.
Phytoplankton biomass conditions following the spring bloom were highly variable, but some years showed a bimodal trend with decreased summer biomass following the spring bloom followed by the resurgence of a late summer/autumn bloom: These years included 2018 at the fjord and channel and 2019 at the channel. Interestingly, 2019 showed near blooming conditions at the fjord through much of the spring-summer seasons with the annual maximum TChla observed in early September (2019-10-02, 6.93 mg m-3); whereas, in 2020, a lack of summer/autumn blooms was observed at all stations.
3.3 Phytoplankton dynamics and community structure
Over the time series, 137 taxa were identified with the cryptophyte, Hillea sp., showing the highest median abundance and percent contribution (8.23 x 104 cells L-1, 20%) followed by the diatom, Skeletonema marinoi (7.32 x 104 cells L-1, 12%, Table 3) across the dataset. In comparison, CHEMTAX (only available for 2019 and 2020) showed the highest median contribution by diatoms (0.54 mg m-3, 39%) followed by cryptophytes (0.40 mg m-3, 19%) and size fractionated chlorophyll by ChlaMICRO (0.77 mg m-3, 61%) (Table 3). Yet, considerable spatial-temporal variability in protist community composition was observed across the time series.
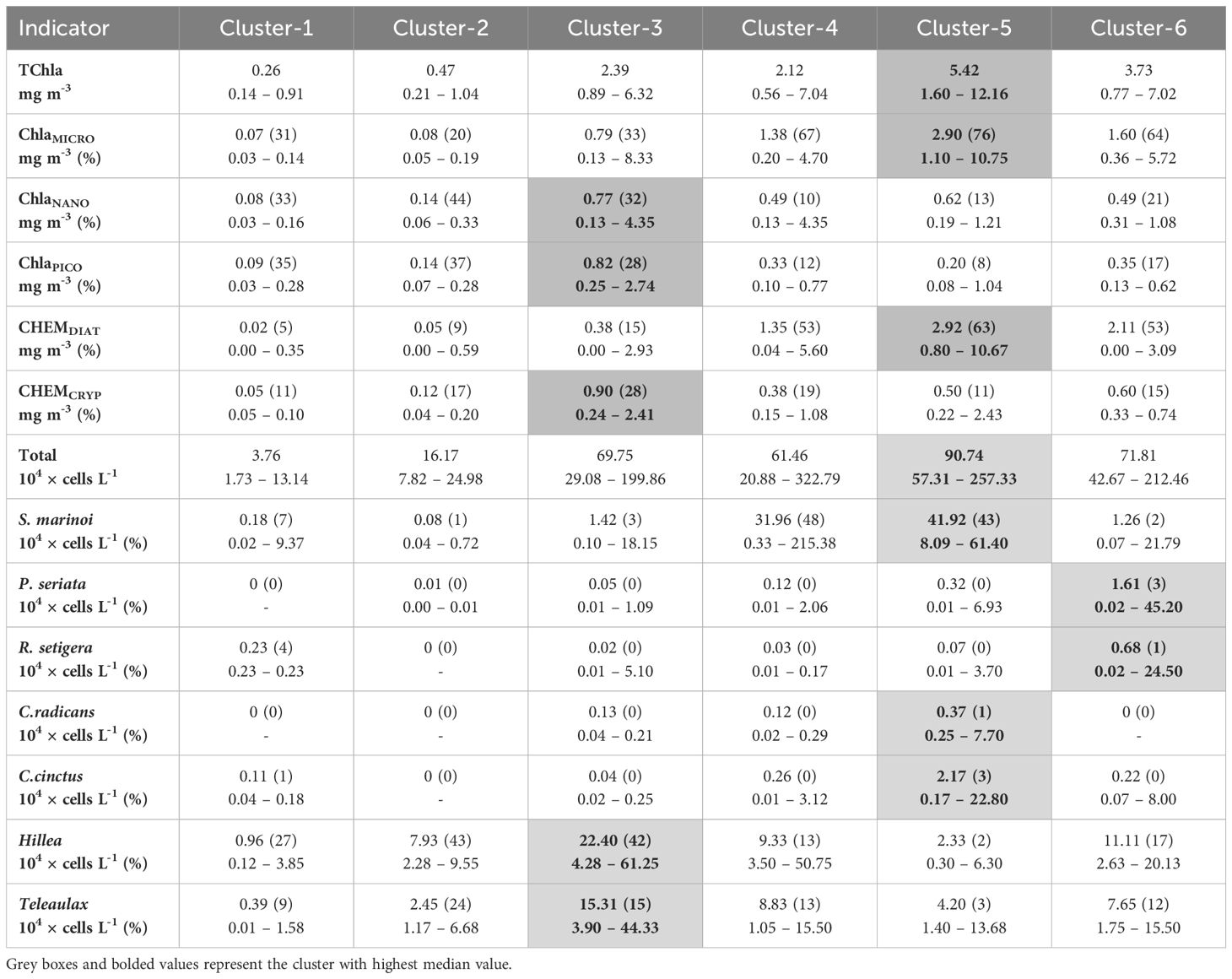
Table 3. Median abundance/biomass and percent contribution (brackets) and minimum and maximum values for TChla, ChlaMICRO/NANO/PICO, CHEMTAX diatom (CHEMTDIAT) and cryptophyte (CHEMCRYP) groups and, key indicator species for each taxonomic cluster.
Cluster analysis well resolved the temporal and spatial trends in the abundance-based (microscopy) community composition data showing six significant clusters (ANOSIM R = 0.70, p <0.001, details about concentrations and percent contributions available in Table 3 and dendrogram provided in Supplementary Figure S9).
Clusters-1 and 2 were composed of winter and late autumn samples, which generally had low total cell abundance and TChla (< 1.1 mg m-3) and were dominated by the small cryptophyte Hillea. The bulk of the chlorophyll was found in the pico and nano-size fractions during these months. No significant indicator species was found for either of these clusters. Additionally, CHEMTAX highlighted diverse phyto-flagellate communities in cluster 1 and 2 samples, with cluster-1 dominated by raphidophytes and green algae and cluster-2 by cryptophytes and dictyochophytes. Despite the differences, no clear spatial or annual differences were observed between the two clusters (Figures 3, 4, Table 3).
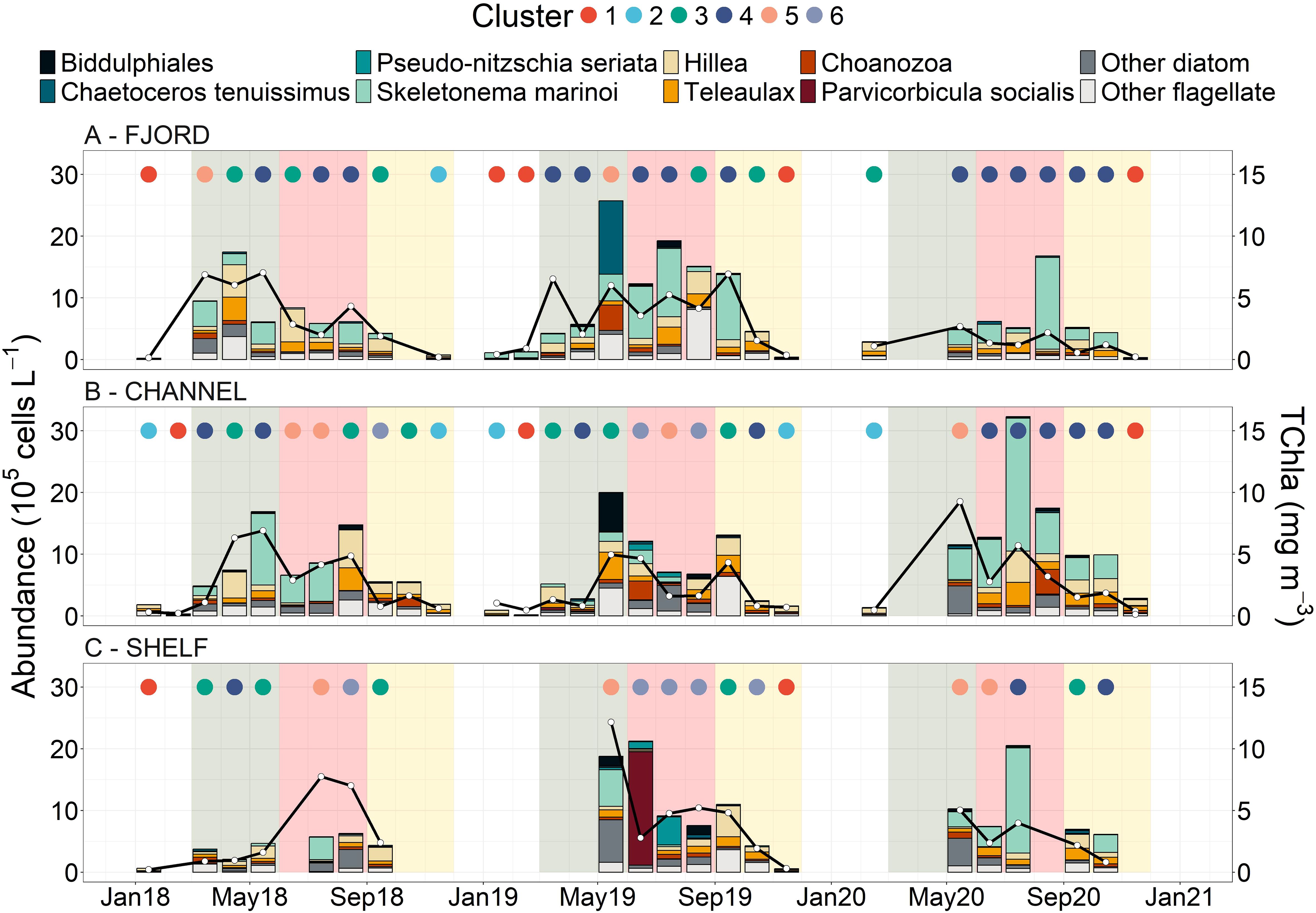
Figure 3. Time series of dominant species that showed the top 8 maximum abundances with other species placed within “other diatoms” and “other flagellates” for the (A) fjord, (B) channel and (C) shelf stations. The right y-axis corresponds with the black line and white filled dots and represents HPLC TChla (mg m-3). The colored dots at the top of each panel represents the cluster that the sample fell within and corresponds with the colors and data in Figure 4. The shaded background represents meterological seasons with spring (green = March, April , May), summer (red = June, July, August) and autumn (yellow = September, October, November).
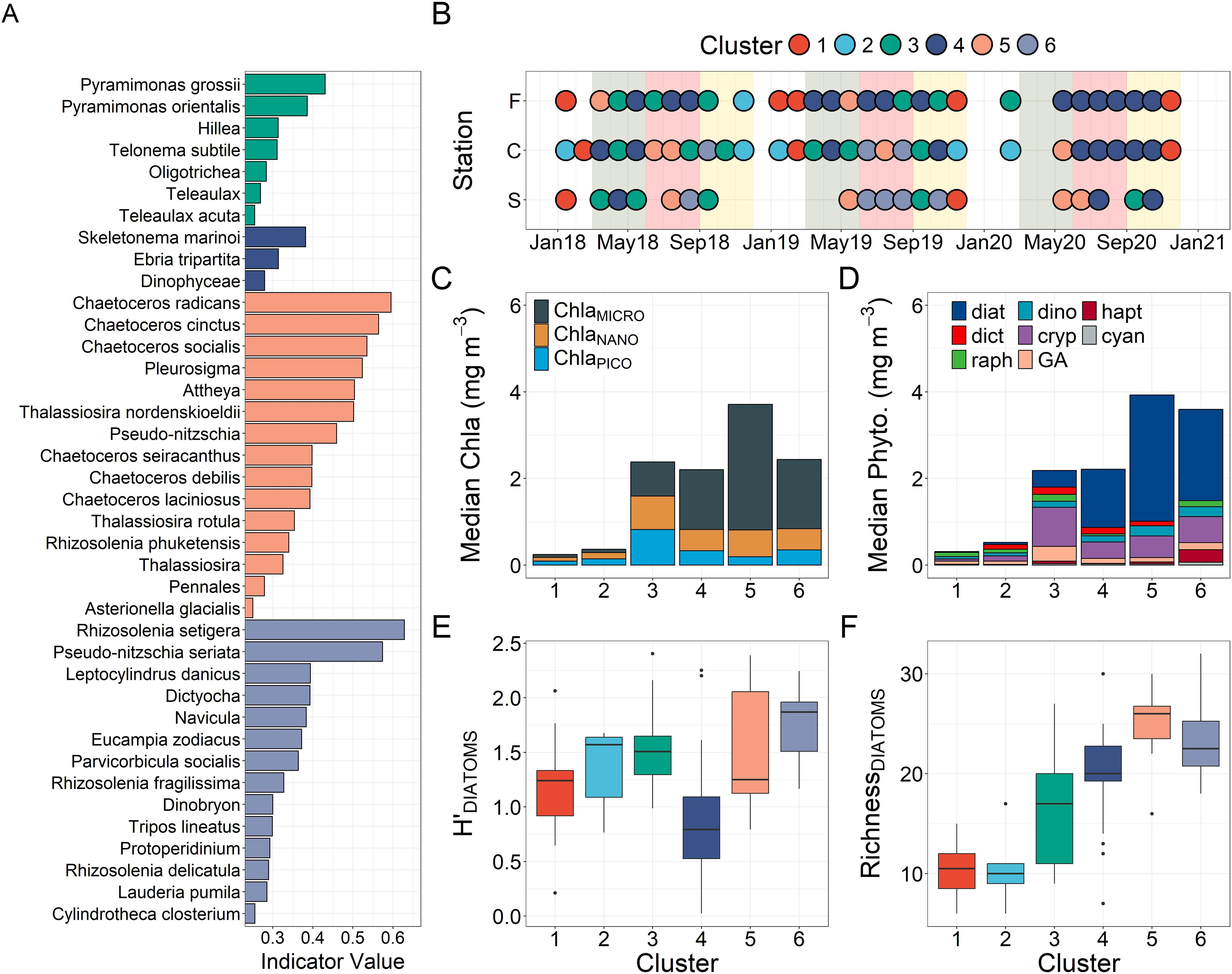
Figure 4. Cluster separated (A) significant indicator species with indicator species values (ISV) > 0.25 (note that clusters 1 and 2 did not have significant indicator species and were not included), (B) spatial-temporal distribution of sample-cluster designations across the time series for each station, (C) median size-fractionated chlorophyll contributions, (D) median CHEMTAX group level phytoplankton contributions (2019 and 2020 only) and box-plots of (E) Shannon diversity index values for diatom species and (F) richness for diatom species. In (E, F) the horizontal line within the colored box represents the median, the box-length the interquartile range, the vertical lines extending outwards from the box the 90th percentile and the dots outliers.
Cluster-3 contained late summer/early autumn bloom samples, was characterized by moderate median cell abundance and TChla, and was dominated by flagellates (Figures 3, 4). Indicator species for this cluster were largely flagellates with the cryptophytes, Hillea and Teleaulax, showing the highest median cell abundance across all clusters coincident with the highest median CHEMTAX cryptophyte contribution (Figure 4, Table 3). Samples in this cluster had relatively comparable contributions across all Chla size-fractions. Interestingly, this cluster also included the April 2018 spring bloom at the channel that was dominated by Hillea sp. and had high TChla, predominately in the nano-size fraction (Figure 3; Supplementary Figure S10) and the September 2019 channel bloom that displayed high abundance of potentially toxic raphidophyte species (Heterosigma or Chattonella sp., included as “other flagellate” in Figure 3) and dataset high CHEMTAX raphidophyte biomass (Supplementary Figure S11).
Samples dominated by diatoms were represented by Clusters-4 through 6, which combined generally spanned spring through summer conditions and were defined by distinct species composition. Cluster-4 was distinguished by S. marinoi dominance with this species being the only diatom indicator and highlighted by a low median diatom diversity (Figure 4). This cluster showed the lowest median TChla of the diatom clusters, but had relatively high median ChlaMICRO and CHEMTAX diatom contributions (Figure 4, Table 3). Spatial-temporally, this cluster was prevalent within the fjord in all years and was strongly represented by 2020 samples from all stations including the large July 2020 spikes in S. marinoi observed at both the channel and shelf (C - 215.38 and S – 170.40 x 104 cells L-1, Figure 3). In addition, this cluster included some spring samples including the May 2018 channel bloom (which corresponded with the date of the station stratification (Δρ) maxima).
Cluster-5 also showed high S. marinoi abundance, but was differentiated by having many diatom indicator species including multiple Chaetoceros and Thalassiosira species, a large range in diatom diversity values and high diatom richness (Figure 4). This cluster had the highest median total cell abundance, TChla, ChlaMICRO and CHEMTAX diatom contributions. Furthermore, this cluster represented most spring bloom samples including the May 2019 shelf bloom (high Chaetoceros cinctus abundance - 22.8 x 104 cells L-1, ISV = 0.56), the May 2019 fjord bloom (high C. tenuissimus abundance - 119.00 x 104 cells L-1, ISV = 0.34) and the 2020 channel and shelf blooms which showed high abundances of Thalassiosira pacifica (10.00 x 104 cells L-1) and nordenskioeldii (91.80 x 104 cells L-1, ISV = 0.50).
Finally, cluster-6 showed distinctively low S. marinoi abundance with its strongest indicators being Rhizosolenia setigera and Pseudo-nitzschia seriata and having the highest median diatom diversity value (Table 3, Figure 4). This cluster showed the second highest median TChla and high median ChlaMICRO and CHEMTAX diatom contributions. The relative median CHEMTAX diatom contribution for this cluster was reduced as a result of increased haptophyte contributions (10%), which was a minor group in all other clusters and showed notable contributions in summer 2019 (Supplementary Figure S11). Furthermore, cluster-6 lacked fjord and 2020 samples and encompassed much of the summer 2019 samples at the shelf which, following a large spring bloom, showed high abundances of Parvicorbicula socialis (183.75 x 104 cells L-1) in June and a Pseudo-nitzschia seriata bloom in July (45.20 x 104 cells L-1, Figure 3). By August, P. seriata abundances had declined considerably showing a diverse diatom community including relatively high L. danicus (40.30 x 104 cells L-1) and R. setigera (21.00 x 104 cells L-1) abundances.
3.4 Drivers of phytoplankton communities
Spearman’s rank order correlations were used to investigate drivers of TChla, size-fractionated Chla and CHEMTAX diatom and cryptophyte biomass between stations and across the dataset including all data (Figure 5; Supplementary Figure S12). Of the correlations, PAR was the only variable to show significant and relatively strong correlations with TChla at all stations (rs = 0.66 – 0.78, padj <0.001). The strongest correlations were observed between nutrients and TChla (DSi shown, but correlations were comparable across the other nutrients), but only at the fjord (rs = -0.79, padj <0.001) and channel (rs = -0.75, padj <0.001) with the shelf potentially lacking a correlation as a result of limited high nutrient, low TChla winter data. A similar spatial disparity was observed in the size-fractionated Chla and CHEMTAX data; however, at the channel nutrients were most strongly negatively correlated with chlaMICRO (rs ~0.80 vs < 0.60 for other sizes) and DSi was not correlated with ChlaNANO and ChlaPICO (Supplementary Figure S12). In turn, at the fjord, equally strong negative correlations were observed between all nutrients and ChlaMICRO and ChlaNANO (rs ~0.80, padj <0.001) with reduced correlations for DIP. Interestingly, while secchi depth (SD) was negatively correlated with TChla at the channel and shelf (rs = -0.77 and -0.78, respectively, padj <0.001), there was no significant correlation in the fjord, where other particle sources from riverine input were likely also abundant. Similarly, only the channel and shelf showed significant negative rain-type watershed discharge and wind speed and positive wind direction correlations with TChla. Of note, wind data from the Rivers Inlet Ethel Island station, while closer in proximity, still did not show correlations with TChla from the fjord station (not shown). The channel was the only station to show positive correlations between TChla and temperature, stratification and snow mountain-type discharge whereas the shelf was the only station to show strong positive correlations between temperature and ChlaPICO and ChlaNANO (rs = 0.75, padj <0.001).
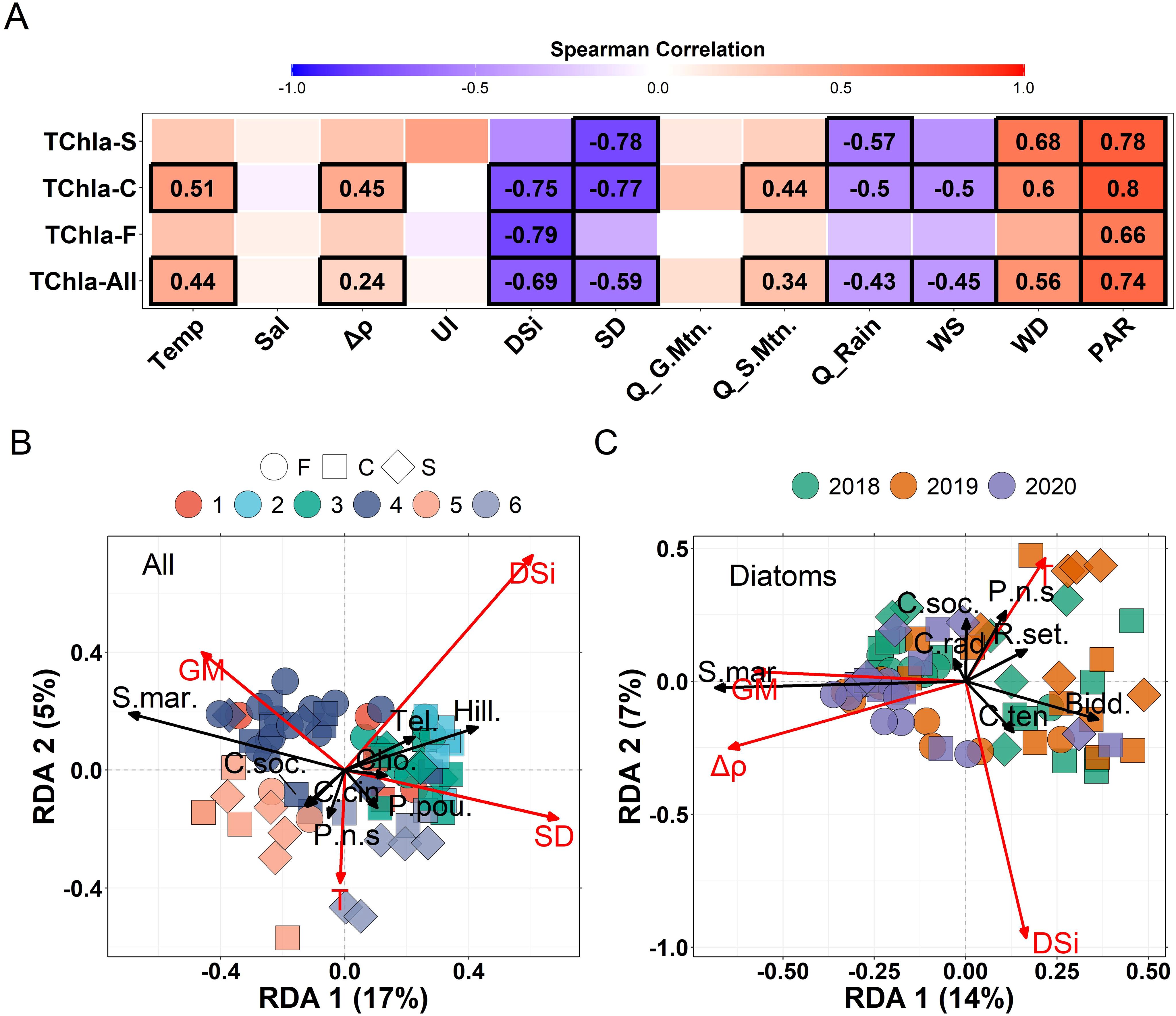
Figure 5. Investigation of environmental drivers of phytoplankton dynamics done through (A) Spearman’s rank order correlations between drivers and TChla with correlations performed independently for each station (TChla-S, TChla-C and TChla-F) and with all of the data grouped (TChla-all) and RDA analysis performed on (B) the entire community and (C) just on diatoms. In (A) the colours represent correlation strength with significant correlations having a black box and bolded rs values and non-significant correlations lacking a box and correlation number. Furthermore, Temp = 5m depth temperature, Sal = 5m depth salinity, Δp = stratification, UI = 3-day backwards averaged upwelling index, DSi = 5m depth dissolved silicate, SD = secchi depth, Q_G.Mtn = 10-day backward averaged glacierized mountain-type discharge, Q_S.Mtn = 10-day backward averaged snow mountain-type discharge, Q_Rain = 10-day backward averaged rain-type discharge, WS = 3-day backward average windspeed from the Lookout station, WD = 3-day backward average wind direction from the Lookout station, and PAR = 3-day backward average incoming photosynthetic radiation from the Lookout station. In (B) the colors represent the results of the taxonomic clustering and in (C) the colors represent years. In both (B, C) the shapes represent the different stations with circles = fjord, squares = channel and diamonds = shelf samples. Only species with the highest axes scores and abundances were plotted with S.mar, Skeletonema marinoi; C.soc, Chaetoceros socialis; C.Cin, C.cinctus; C.rad, C.radicans; Bidd, biddulphiales (unidentified centric diatoms); R.set, Rhizosolenia setigera; P.n.s, Pseudo-nitzschia seriata; Cho, Choanozoa; Tel, Telealeaux; Hill, Hillea. Ordinations were visualized using scaling-3.
Redundancy analysis was independently performed on the full taxonomic suite and then exclusively on diatom species to investigate drivers of phytoplankton taxonomy (Figure 5). The full analysis had an R2 = 0.21 with RDA1 explaining 17% of the variance (p = 0.001) and RDA2 explaining 5% of the variance (p = 0.003). Forward selected explanatory variables were Secchi depth (SD, 8% of variance, p = 0.001), temperature (T, 5%, p = 0.002), DSi (4%, p = 0.007), and glacierized mountain-type discharge (GM, 2%, p = 0.024). The RDA triplot (scaling 3) showed good separation between the planktonic protist abundance-based clusters. Cluster-4 was related to S. marinoi (S.mar) and glacierized mountain-type discharge (Q_GM), both of which were negatively correlated with secchi depth (SD). These samples lacked a correlation with nutrients and, while highly variable, tended to show moderate nutrient concentrations and higher DSi relative to DIN (Supplementary Figure S7). Cluster-5 was related to C. socialis (C.soc.) and C. cinctus (C.cin) and these samples and species were negatively correlated with DSi (and DIN and DIP due to cross correlation) and represented many of the depleted nutrient events (Supplementary Figure S7) including the 2019 spring bloom at the shelf, which showed the time series maximum TChla. Temperature was associated with cluster-6 samples and the closest species vector was for P. seriata (P.n.s). These samples were also moderately negatively correlated with nutrients. Cluster-3 (moderate biomass, flagellate-dominated) and cluster-2 (winter, flagellate-dominated) were associated with the cryptophyte species, Teleaulax (Tel.) and Hillea (Hill.), and Choanozoa (Cho.). All of these species were negatively correlated with diatom species. In addition, the two cryptophyte species were moderately positively correlated with nutrients and Choanozoa was positively correlated with Secchi depth. The RDA performed on just the diatom taxa showed comparable trends with the addition of stratification (Δρ), which was positively correlated with glacierized mountain-type discharge, S. marinoi, 2018 and 2020 channel and shelf samples, and fjord samples from all years. In addition, this analysis showed a stronger positive link between temperature, 2018 and 2019 samples and the diatom species, P. seriata and R. setigera. These species were negatively correlated with stratification, glacierized mountain-type discharge and nutrients.
4 Discussion
4.1 Bloom timing and seasonal TChla trends
Distinct differences in bloom timing were observed between stations with the fjord showing earlier blooms than the outer fjord stations. Previous studies have shown spring bloom timing within Rivers Inlet is highly dependent on freshwater discharge and channelized wind speed with strong discharge and outflow winds resulting in surface water advection, phytoplankton dilution and delayed blooms (Wolfe et al., 2016). In this study, the observed March 2018 and 2019 fjord blooms were early when compared to the March through early May timing observed at the same station by other studies over multiple years (Tommasi et al., 2013; Wolfe et al., 2016; Jackson et al., 2022) and are suggestive of an ideal balance of wind and discharge conditions. Of note, the fjord spring blooms developed while winter wind conditions persisted outside of the fjord, as highlighted by the lack of wind-TChla correlations at this station, suggesting a sheltering effect by the fjord allowing early blooms to develop. Similarly, localized early spring blooms (March) have been observed within Alaskan and British Columbian fjords and sheltered inlets, suggesting that these results may be broadly representative of fjord influenced northeast Pacific waters (Etherington et al., 2007; Gower et al., 2013; Du et al., 2023). Further south, it is theorized that early blooms within fjords may seed the spring blooms observed within the Salish Sea (Gower et al., 2013). Here, connectivity patterns are not well understood and more resolved data are required to investigate this hypothesis; however, the spring blooms observed at the channel and shelf were broadly related to other environmental drivers suggesting differing initiation mechanisms.
In contrast to the fjord, blooms at the channel were observed in the following month and were representative for this station, with monthly averages over the 2014 – 2018 period showing a bloom peak in April-May (St. Pierre et al., 2020, 2022). Bloom initiation mechanisms at this station appeared similar to those observed within the Salish Sea being coincident with seasonal reductions in wind speed, changes in direction, and higher incoming PAR (Collins et al., 2009; Allen and Wolfe, 2013; Del Bel Belluz et al., 2021). Furthermore, these blooms were timed closely with the annual freshet and across the British Columbia and Alaskan coasts, freshwater driven stratification has been shown to be an important driver of spring bloom timing (Henson, 2007; Du et al., 2023) and may potentially even trigger winter blooms (Johannessen et al., 2019). However, while a lack of data makes assessment of conditions at the shelf difficult, spring blooms at this station were observed under lower stratification and higher salinity when compared to the channel suggesting that freshwater-driven stratification may play a less important role than reduced wind and increased light conditions. Observations within Glacier Bay, Alaska, somewhat support this finding showing that in more exposed areas, the onset of spring blooms often developed prior to the seasonal increase in stratification and were more linked to increases in incident light (Etherington et al., 2007). Furthermore, large scale satellite chlorophyll analysis showed that PAR was a key driver of enhanced spring chlorophyll in the eastern Gulf of Alaska (Waite and Mueter, 2013). These observations highlight the complex balance of drivers that influence bloom timing in dynamic coastal systems (Etherington et al., 2007; Carstensen et al., 2015; Du et al., 2023).
Following the spring bloom, high variability in phytoplankton biomass was observed. Annual TChla variability was highest in the fjord, and in these freshwater influenced systems blooms typically develop under intermediate flow, or at freshwater fronts, where nutrient inputs, light, and flushing are balanced (Etherington et al., 2007; Hodal, 2011; Carstensen et al., 2015). In the studied fjord, advection and dilution can be prominent (Hodal, 2011; Wolfe et al., 2016) and the observed lack of a secchi-TChla correlation highlighted the presence of non-algal particles that may have driven light limitation and reduced biomass as documented in Alaskan fjords (Etherington et al., 2007; Arimitsu et al., 2016). Furthermore, differences in discharge and stratification may have influenced the depth of the chlorophyll maximum, which CTD fluorescence profiles suggest was periodically below the 5m sampling depth, notably during summer. The interplay of these dynamics could explain the observed lack of seasonality and variable annual biomass conditions observed at this station (Cloern et al., 1983; Salmaso and Zignin, 2010).
When considering the entire dataset, the high observed variability over small spatial scales suggests a dynamic system with many localized influences. This finding is an important consideration as a recent data-driven bio-regionalization using high spatial resolution satellite-based (Sentinel 3A, 300m) surface chlorophyll grouped the area into a single region characterized by largely invariant and moderate productive season biomass peaking in autumn (Marchese et al., 2022). These results do not align with the results of this study which generally show high growing season biomass initiated by a strong spring bloom. Our results are more comparable to satellite-based surface chlorophyll analysis done by Jackson et al. (2015) and a more recent and comprehensive analysis using a 23-year combined satellite time series (Pramlall et al., 2024); however, the longer time series analysis grouped the studied area into a broad estuarine cluster spanning much of the British Columbia coastline. Consequently, it is evident that these broad satellite based classifications can obscure important sub-regional variability with our results highlighting high variability in spring bloom timing, annual productivity and communities across relatively short distances likely with important ecological ramifications. For example, spring bloom timing is crucial for the survival of fish and invertebrate populations (Platt et al., 2007) and is linked to northeast Pacific Pink salmon productivity (Malick et al., 2015), Queen Charlotte Sound Pacific Auk and Rhinoceros Auklet breeding success (Borstad et al., 2011; Crossin et al., 2022) and in Rivers Inlet, summer zooplankton biomass and composition with implications for migrating Sockeye salmon (Tommasi et al., 2013). Higher sampling resolutions and longer time series are necessary to comprehensively investigate the mechanisms driving many of the observed biomass trends; however, the in situ data used here provided valuable information on species composition not easily derived by satellite based time series in dynamic coastal waters.
4.2 Phytoplankton compositions
4.2.1 General phytoplankton group-level trends and comparison to the Salish Sea
Seasonally, the winter clusters (clusters 1 + 2) in this study showed that small flagellates (ChlaNANO and ChlaPICO) such as cryptophytes, green algae and dictyochophytes were dominant under low TChla conditions (< 1 mg m-3), which is in-line with CHEMTAX and size-fractionated based results from further south in the Salish Sea (Del Bel Belluz et al., 2021; McLaskey et al., 2022; Nemcek et al., 2023). Furthermore, similar to the Salish Sea, spring bloom conditions in this study were largely diatom and ChlaMICRO dominated (with exception to the cryptophyte-dominated spring bloom – section 4.2.4); however, in this study, diatom and ChlaMICRO dominance often persisted through much of spring and summer. This finding is in contrast to the Salish Sea where persistent late spring through summer flagellate-dominated communities, constituted by pico-size species, are observed and attributed to high stratification and nutrient limitation (Del Bel Belluz et al., 2021; Nemcek et al., 2023). Contrasts between locations were also observed during autumn with the Salish Sea typically showing diatom-dominated autumn blooms whereas the autumn blooms observed here, on the central coast, where flagellate-dominated despite both locations showing somewhat comparable nutrient replete conditions (Del Bel Belluz et al., 2021). These contrasting summer and autumn phytoplankton communities suggest important differences in both bottom-up and top-down forcing between locations along the British Columbia Coast.
When compared to the results of this study, the stratification observed in the Salish Sea during summer was comparable to those at the channel (~Δρ 4-6 kg m-3), but was considerably lower than those at the fjord where diatoms were also often persistent. Despite this higher stratification, nutrients also tended to be higher, notably in the fjord, than in the Salish Sea where summer surface nutrient limitation is common. These findings suggest that, in this study, either freshwater was a source of nutrients or that frequent mixing events (either through tidal or wind energy) replenished the surface layer promoting summer diatom biomass. Similar trends have been observed in fjord influenced Chilean and Alaskan waters where nutrient rich stratified surface layers support high diatom biomass and carbon sequestration through much of the growing season (Etherington et al., 2007; Cuevas et al., 2019). Furthermore, in Douglas Channel, British Columbia, large diatom blooms were observed through spring and summer and vertical flux of organic materials peaked in August (Johannessen et al., 2019). Yet, while nutrient-limiting conditions tend to be more prevalent in the Salish Sea, summer nutrient limitation is periodically interrupted by wind driven breaks in stratification. These events often result in nutrient renewal and drawdown, but no corresponding increase in phytoplankton or diatom biomass and are suggestive of strong grazing (Del Bel Belluz et al., 2021). Consequently, the high summer diatom biomass observed here may also be indicative of lower summer season top-down control on diatoms when compared to the Salish Sea. Interestingly, and in contrast to summer conditions, the flagellate-dominated autumn blooms observed in this study, despite nutrient replete conditions, potentially suggests stronger autumn diatom grazing during this time of year. In the Salish Sea, recent zooplankton fatty acid analysis suggests that diatom grazing is at its highest in late summer/autumn with the transience of autumn diatom blooms likely modulated by variability in top-down control (McLaskey et al., 2024); however, similar studies have not been performed for the area studied here. Further research is required investigating the interaction of phytoplankton and zooplankton dynamics as these are important for understanding phytoplankton communities, food web dynamics and survival of higher trophic level species.
4.2.2 The prevalence of Skeletonema
In this study, Skeletonema marinoi was the dominant diatom over the time series being prevalent across a wide array of conditions. This fast-growing highly adaptable species is one of the most important components of diatom blooms across temperate coastal systems and its adaptability is likely a factor explaining its prevalence in this study (Carstensen et al., 2015). Furthermore, recent molecular studies have shown large diversity in species within the Skeletonema genus, which are often not resolvable via optical microscopy (Sarno et al., 2005; Kooistra et al., 2008). This diversity in species was not addressed or resolvable here, but differences in environmental niches between species could help explain the observed broad distribution in S. marinoi described below.
Over the time series, S. marinoi dominated communities were observed under two different regimes represented by two clusters. Under the first regime (cluster-5), S. marinoi was the dominant diatom largely during spring bloom conditions, but was present with a high diversity of other diatom species generally including Chaetoceros and Thalassiosira spp. This trend is representative of the coastal northeast Pacific spanning the Gulf of Alaska to Oregon where Skeletonema spp. are often the main contributor to spring blooms alongside other characteristic diatoms such as Chaetoceros and Thalassiosira spp (Du et al., 2023; Harrison et al., 1983; Hobson and McQuoid, 1997). Similar to S. marinoi, these chain forming species are highly adapted to turbulent and nutrient rich coastal ecosystems and can quickly bloom when optimal conditions are met (Carstensen et al., 2015). Furthermore, many of the prominent species within this cluster are larger than S. marinoi with higher carbon and Chla contents (González et al., 2007): This finding is supported by TChla and ChlaMICRO being highest for cluster-5. The presence of these higher biomass species could help explain the RDA based negative correlation between cluster-5 samples and nutrients as larger diatoms have higher nutrient requirements when compared to Skeletonema spp. and potentially drove the strong nutrient drawdown observed following some high biomass diatom bloom conditions (e.g. in 2018, low nutrient conditions were observed through the summer at the channel when cluster-5 samples were present - Figures 2, 3) (Yano et al., 2023).
In contrast to the first regime, samples included in the second regime (cluster-4) were heavily dominated by S. marinoi and showed lower diatom diversity. Samples from this cluster did not show a relationship with nutrients and were largely represented by fjord samples, but also channel and shelf samples under high discharge summer conditions. In coastal northeast Pacific waters, it is common for Skeletonema spp. to become less prevalent following the spring bloom, but there are sheltered fjord systems such as Juan Perez Sound (Haida Gwaii, British Columbia) (Peterson et al., 2007) and Sechelt Inlet (Salish Sea, British Columbia) (Haigh et al., 1992) that also show Skeletonema spp. dominance from spring through summer. In addition, comparable observations have been made globally where Skeletonema spp. have been associated with eutrophic, turbid, low salinity and high stratification waters near river outlets or at the heads of coastal fjords, notably during high discharge periods (Alves-De-Souza et al., 2008; Katano et al., 2012; Brito et al., 2015; Elferink et al., 2017; Marić Pfannkuchen et al., 2018; Gao et al., 2022; Cavalcanti-Lima et al., 2023). These associations are supported by the RDA results of this study with S. marinoi dominated samples being correlated with increased glacierized mountain-type discharge and stratification, reduced secchi depths and moderate nutrients. Under these conditions, S. marinoi dominance may have been promoted by the species’ ability to survive and adapt to low and dynamic salinity conditions (Balzano et al., 2011; Sjöqvist et al., 2015; Gao et al., 2022; Pinseel et al., 2022) and its preference for, and ability to alter buoyancy to limit sinking out of, salt stratified surface layers (Erga et al., 2015). In contrast, many other diatom species require turbulent conditions that limit their sinking out of the surface layer (Marañón et al., 2012) as evidenced by Chaetoceros spp. often being associated with higher salinities and mixing when compared to Skeletonema spp (Peterson et al., 2007; Wasmund et al., 2011; Krawczyk et al., 2015; Elferink et al., 2017). These traits would promote the observed S. marinoi dominance within the fjord with high discharge events potentially advecting waters dominated by this species outwards to the channel and shelf. Similar observations of Skeletonema spp. dominance within the spatial extent of freshwater influenced waters has been observed elsewhere (Patil and Anil, 2011; Katano et al., 2012) and rivers plumes have been shown to have homogenizing effects on phytoplankton communities (Frame and Lessard, 2009). Nonetheless, high discharge events and freshwater influences did not always promote S. marinoi dominance (i.e. late summer 2019) suggesting more complicated mechanisms not resolved here.
The lack of S. marinoi during the late August/September 2019 discharge events, which resulted in strong freshwater signals at the channel and shelf, is in contrast to the trends observed in 2020. Timing may have been a factor with the 2019 discharge events occurring later in the summer when flagellates were typically more prevalent and, again, potentially representative of increased diatom grazing during this time of the year. An additional consideration is that the advection patterns of source waters outwards from the many local watersheds and fjords remains largely unknown and may have driven the observed differences in phytoplankton communities between years. For example, in 2019, raphidophytes were observed in lieu of S. marinoi, and it is possible that source waters interacted with seed beds of these species spurring a bloom: raphidophyte blooms (Chattonella spp. and H.akashiwo) have been linked to freshwater and warm temperatures (Katano et al., 2012; Esenkulova et al., 2021; Nemcek et al., 2023). In addition, the lack of S. marinoi could be further explained by the suspected ability of raphidophytes to inhibit its growth via allelopathy; although, the opposite has also been observed with Skeletonema spp. hindering raphidophyte growth, and as a result, it is also possible that the observed raphidophytes were present due to a lack of S. marinoi (Pratt, 1966; Haigh and Taylor, 1991; Yamasaki et al., 2007; Katano et al., 2012). Considering the observed trends, it is clear that further research is required investigating freshwater-oceanographic dynamics and advection patterns and their influence on phytoplankton communities within British Columbia fjord systems that heavily influence coastal oceanographic variability.
Despite the freshwater periods lacking S. marinoi, this species was the dominant observed diatom across the time series and its strong presence likely has important biogeochemical implications. For example, large phytoplankton support more efficient energy transfer to higher tropic level species, such as Pacific salmon, when compared to conditions dominated by smaller species (Lerner et al., 2022). In this study, S.marinoi dominated conditions showed high ChlaMICRO contributions (despite being lower than cluster-5 spring bloom samples) and persisted through much of the summer season through stratified conditions. This prevalence of S.marinoi may provide a longer window of increased energy transfer supporting higher productivity when compared to systems such as the Salish Sea, which become flagellate dominated following the spring bloom. Yet, recent preliminary evidence also suggests that high density Skeletonema spp. blooms can also reduce wild salmon feeding and consequently, the occurrence of blooms during the May to June Rivers Inlet out-migration period could negatively affect juvenile survival (Esenkulova et al., 2022). In addition, Rivers Inlet often shows intermediate water oxygen minimum zones that have been associated with remineralization of organic matter (Jackson et al., 2022). As a result, high summer diatom biomass, such as observed in the fjord in 2019, could help to promote oxygen depletion in intermediate waters that could impact salmon populations (Rosen et al., 2022). Finally, the observed coast wide Skeletonema spp. blooms and subsequent sinking of cells could represent an important source of carbon sequestration and nutrient reintroduction to coastal waters within inflowing subsurface waters (Whitney et al., 2005); however, while Skeletonema spp. can represent a dominant proportion of the total diatom cells exported from surface waters, they typically contribute less to overall vertical carbon flux than larger, but less abundant, Thalassiosira and Chaetoceros spp (González et al., 2007). Therefore, higher diatom diversity conditions, such as those observed in summer 2018 when there were few stochastic discharge events, could promote greater carbon sequestration.
4.2.3 Summer communities and harmful diatoms
The summer species cluster (cluster-6) was observed at the channel and shelf in 2018 and 2019, with the majority of samples representing the high salinity 2019 conditions. Within this cluster, Rhizosolenia setigera was the dominant indicator species and similar to this study, is often found under warm and high salinity summer conditions in coastal northeast Pacific waters (Esenkulova et al., 2021; Du et al., 2023). Furthermore, elevated R. setigera abundances only occurred during periods of low S. marinoi abundances suggesting differences in environmental preferences with R. setigera likely being characteristic of more oceanic (or less fjord influenced) waters. While not toxic, R. setigera have prominent spikes that can damage fish gills and result in finfish mortality (Esenkulova et al., 2021). In addition to this species, cluster-6 was associated with other harmful species, notably Psuedo-nitzschia seriata, which was prominent during 2019.
Similar to R. setigera, the 2019 P. seriata dominated bloom was observed predominantly at the shelf under warm and high salinity early summer conditions. Upon freshwater arrival, abundances of this species decreased dramatically. These trends are in-line with empirical observations of P. seriata showing a preference for high salinities and low tolerance to reduced salinities when compared to other Pseudo-nitzschia spp (Boivin-Rioux et al., 2022; Clark et al., 2019; Dodrill et al., 2023; Fehling et al., 2006; Weber et al., 2021). Furthermore, links between Pseudo-nitzschia spp. and warm temperatures have been observed regionally (Peterson et al., 2007; Perry et al., 2023) and specifically for P.seriata, in the English Channel (Houliez et al., 2023). In this study, these conditions appeared to be important to the proliferation of P. seriata when compared to the broadly present Pseudo-nitzschia spp., which often showed elevated abundances during cooler periods such as the spring bloom (however, it should be noted that P. seriata were also broadly present, but just in low abundances). Similar to this finding, Fehling et al. (2006) reported an RDA based link between P. seriata and warm summer conditions while other Pseudo-nitzschia spp. showed broader presence and elevated spring bloom abundances. Houliez et al. (2023) found similar temporal trends and links with temperature in the English Channel, but further highlighted that P.seriata blooms occurred in years when nutrients, particularly silicate, had been depleted by large spring blooms constituted by Chaetoceros spp. Under these conditions, the ability of P.seriata to remain viable under low silicate and DSi:DIN and to quickly capitalize on small nitrogen introductions, with a preference for urea, is thought to allow them to outcompete other diatom species and become established (Melliti Ben Garali et al., 2016; Houliez et al., 2023). Here, monthly sampling made it difficult to draw similar conclusions; however, 2019 did show the time series maxima TChla at the shelf in May, which included a diverse array of Chaetoceros spp, and coincided with nil to low DIN and DSi conditions that persisted through to the P.seriata bloom in July. Furthermore, choanoflagellate (Parvicorbicula socialis) abundances were exceptionally high in June, when P.seriata had already become the dominant diatom. This choanoflagellate species is indicative of high bacterial abundances and microbial loop conditions following the breakdown of a strong spring bloom (McKenzie et al., 1997) and may suggest the continuation of high phytoplankton biomass between the monthly sampling. Along with high temperature and salinity, these conditions may have promoted P.seriata in this year.
Of note, the observed largely monospecific P. seriata bloom was unique with the large Pseudo-nitzschia blooms often observed between northern California and Washington typically being made up of several species and rarely showing considerable P. seriata contributions (Trainer et al., 2009; McCabe et al., 2016). Yet, our results are in-line with a recent large-scale regional analysis showing P. seriata prevalence within Queen Charlotte Sound, suggesting a potential hotspot for this species (Perry et al., 2023). If so, the results of this study suggest that that warm and low freshwater conditions, potentially driven by marine heatwaves, may allow for coastward advection of P.seriata blooms which is similar to observations in the Columbia River estuary during low freshwater and marine heatwave conditions (Dodrill et al., 2023; McCabe et al., 2023). This coastward advection is an important consideration as P. seriata is capable of producing high concentrations of domoic acid, which can kill a variety of marine life and accumulates within shellfish that if consumed by humans results in potentially fatal amnesic shellfish poisoning (Fernandes et al., 2014; Perry et al., 2023).
4.2.4 Cryptophyte and flagellate blooms
The observed Hillea sp. (cryptophyte) dominated blooms and more specifically, the 2018 spring bloom at the channel, are novel observations with relatively few blooms of this genus (no literature with Hillea spp. blooms could be found) reported globally (Laza-Martínez, 2012; Bazin et al., 2014; Šupraha et al., 2014). In coastal British Columbia, Hillea sp. abundances are high, notably during warm and low nutrient stratified summer conditions, but they are seldom associated with high TChla (Del Bel Belluz et al., 2021; Nemcek et al., 2023). In contrast, the observed cryptophyte blooms in this study occurred during nutrient replete conditions, typically favored by diatoms, as highlighted by the positive RDA based cryptophyte species-nutrient correlations. The lack of diatoms during these bloom events suggest that other drivers, not studied here, may have played a role in promoting cryptophyte dominance. For instance, high dissolved organic carbon concentrations, flushed from watersheds following rain events, and subsequent increases in microbial biomass have been documented within the studied area (St. Pierre et al., 2020) and associated with increased cryptophyte abundances elsewhere (Oseji et al., 2019). These conditions may have promoted cryptophytes via mixotrophy, potentially coupled with this group’s efficient light harvesting capabilities (Unrein et al., 2007; Yoo et al., 2017), which would have been advantageous during light limiting early spring conditions. Of note, the observed 2019 spring cryptophyte bloom occurred following a prolonged period of rain-type discharge (Supplementary Figure S8). In addition, the high abundances observed in the fjord may have been due to high turbidity conditions favoring these species’ ability to quickly adapt to rapidly changing light conditions (Mendes et al., 2023). Another consideration was the role of microzooplankton grazing with mixotrophic and heterotrophic dinoflagellates and ciliates shown to exert strong controls on cryptophyte growth and more locally, nano and pico-plankton (Strom et al., 2001, 2007; Johnson et al., 2018). Consequently, reductions in microzooplankton, either driven by environmental conditions or mesozooplankton grazing, may have lifted top-down controls on cryptophyte and small flagellate growth (Carstensen et al., 2015). Similar trends have been observed in Prince William Sound, Alaska, where < 20 um Chla increased in the presence of high mesozooplankton biomass suggestive of a trophic cascade where grazing on microzooplankton released small phytoplankton from top-down control (Liu et al., 2008). Importantly, cryptophyte-dominated blooms shift the size structure of high biomass events to small species not as easily consumed by mesozooplankton. In particular, while cryptophytes can provide a nutritious food source to zooplankton (McLaskey et al., 2022), their small size increases food web length reducing the energy passed on to higher trophic levels (Lerner et al., 2022).
4.3 Limitations
There were multiple limitations to this study. First, monthly sampling likely missed considerable variability in phytoplankton community dynamics with coastal systems often showing high change over daily to weekly timescales (Del Bel Belluz et al., 2021). While this study documented broad patterns, higher temporal resolution sampling, potentially from moored instrumentation, are required to investigate trends such as bloom timing and phenology and the influence of freshwater pulses and tidal action on phytoplankton community composition. Second, the single sampling depth (5m) of this study likely missed important vertical variation that could influence ecosystem and biogeochemical dynamics. For example, freshwater stratified coastal waters often show high vertical phytoplankton variability, which can include strong subsurface chlorophyll maxima notably during summer stratified conditions (Cuevas et al., 2019; Du et al., 2023). Taxonomic data were not available to investigate this shortcoming, but CTD chlorophyll fluorescence profiles for each station did support the presence of summer subsurface chlorophyll maxima below the 5m sampling depth (Supplementary Figure S3). Third, top-down controls were discussed as potential drivers, but were not investigated. Zooplankton grazing is an important driver of phytoplankton biomass, composition and size-structure (Strom et al., 2001, 2007) and likely also impacted the results of this study. Fourth, optical microscopy abundance counts may have overestimated the prominence of abundant low biomass species when compared less abundant higher biomass species. In this study, the inclusion of size-fractionated and CHEMTAX data helped to address this issue at the broad size class and functional group level (e.g. the results suggest that large, but low abundance dinoflagellates did not make high biomass contributions at 5m depth). Yet, within group taxonomic differences in biomass may have been missed with our approach. Furthermore, even with microscopy, taxonomic trends indicative of different conditions may have been missed due to difficulty identifying taxa to species level based on morphology alone (e.g. Pseudo-nitzschia spp.). Finally, both microscopy and CHEMTAX struggle with separating pelagophyte, chrysophyte and dictyochophytes due to the small sizes of many of the species and their similar pigment profiles (Choi et al., 2020). Many species within these groups are not well characterized and can be important indicators of different source waters; freshwater (colonial chrysophytes, which can be underrepresented by microscopy, Krawczyk et al., 2015) or marine (small pelagophytes and dictyochophytes, Choi et al., 2020). Molecular approaches can help resolve these species and have been shown to compliment morphological and pigment-based methods (Catlett et al., 2023; Cetinić et al., 2024). Therefore, inclusion of molecular approaches in future studies could help to further characterize phytoplankton community dynamics and links to freshwater or marine conditions.
5 Conclusion
The results of this study showed high spatial-temporal variability in phytoplankton dynamics on the British Columbia continental margin. In terms of total phytoplankton biomass, notable differences were observed between fjord and channel spring bloom timing potentially as a result of a sheltering effect from winter wind conditions within the fjord. Outside of the fjord, spring blooms coincided with the relaxation of winter wind conditions, increased incoming PAR and the start of the freshet. Seasonally, biomass tended to follow a bimodal pattern with TChla maxima observed in spring, reduced summer biomass and a late summer or early autumn bloom; however, departures from this trend occurred, notably in the fjord, where dynamic freshwater and turbidity conditions likely resulted in the large differences in phytoplankton biomass between years. Compositionally, low biomass flagellate-dominated (largely cryptophytes) conditions were observed during winter. In turn, high biomass spring bloom (and some summer blooms) conditions were typically dominated by Skeletonema marinoi alongside a variety of Chaetoceros and Thalassiosira spp. and these samples were associated with nutrient drawdown. Under high stratification, S. marinoi was also dominant, but in more homogenous communities that were largely observed during the high 2020 discharge conditions. In contrast, 2019 showed warm and high salinity spring and summer conditions at the shelf with low S. marinoi and increased abundance by the potentially harmful species R. setigera and P. seriata. While spring and summer were often diatom-dominated, autumn blooms were typically flagellate-dominated despite nutrient replete conditions typically favorable for diatom blooms and suggestive of grazing. Furthermore, a rare cryptophyte-dominated spring bloom was observed at the channel in 2018 and may have been driven by reduced grazing pressure or high rainfall driven DOC concentrations.
The observed trends suggest that freshwater is a strong driver of phytoplankton community composition within the studied area. Across the coast of BC and Southeast Alaska, freshwater discharge regimes are projected to continue changing through the end of this century, with greater extent of rain-dominated regimes and earlier spring-freshet from snow/glacier-dominated regimes (Bidlack et al., 2021). In the short term, warmer temperatures will result in higher seasonal glacial discharge, but warming over the longer-term will reduce glacial inputs and summer discharge (Pitman et al., 2020; Rounce et al., 2023). Concurrently, extreme fall-winter runoff events may become more prevalent along with atmospheric rivers (Radić et al., 2015; Curry et al., 2019). Furthermore, extreme marine and terrestrial heatwaves are expected to increase in occurrence. As shown by this study, these changes will likely have profound impacts on phytoplankton community dynamics on the central coast of British Columbia.
Data availability statement
The datasets presented in this study can be found in online repositories. The names of the repository/repositories and accession number(s) can be found in the article/Supplementary Material.
Author contributions
JD: Conceptualization, Data curation, Formal analysis, Investigation, Methodology, Resources, Software, Validation, Visualization, Writing – original draft, Writing – review & editing. JJ: Conceptualization, Investigation, Writing – review & editing, Writing – original draft, Formal analysis. CK: Conceptualization, Investigation, Writing – review & editing, Writing – original draft. MP: Conceptualization, Investigation, Writing – review & editing, Writing – original draft. IG: Data curation, Investigation, Writing – review & editing, Writing – original draft. LH: Data curation, Investigation, Writing – review & editing, Writing – original draft.
Funding
The author(s) declare financial support was received for the research, authorship, and/or publication of this article. Direct funding for this research was provided by the Tula Foundation and we thank Eric Peterson and Christina Munck whose vision and long-term support for synergistic research on a remote and largely unstudied area of the central coast of British Columbia made this work possible.
Acknowledgments
We thank all of the contributing Hakai staff that worked to collect field data, conduct laboratory analysis and provide IT and logistical support including Wiley Evans, Drew Jordison, Bryn Fedje, Chris Mackenzie, Katie Pocock, Emma Myers, Leo Pontier, Katie Holmes, Kate Lansley, Rachel Walker, Breanna Knowles, Chris O’Sullivan, Bill Floyd, Maartje Korver, Rob White, Isabelle Desmarais and Emily Haughton and Mathew Foster. We thank Keith Holmes for his help with graphics. We gratefully acknowledge the help of the reviewers, whose insight greatly improved this manuscript.
Conflict of interest
The authors declare that the research was conducted in the absence of any commercial or financial relationships that could be construed as a potential conflict of interest.
Publisher’s note
All claims expressed in this article are solely those of the authors and do not necessarily represent those of their affiliated organizations, or those of the publisher, the editors and the reviewers. Any product that may be evaluated in this article, or claim that may be made by its manufacturer, is not guaranteed or endorsed by the publisher.
Supplementary material
The Supplementary Material for this article can be found online at: https://www.frontiersin.org/articles/10.3389/fmars.2024.1458677/full#supplementary-material
References
Allen S. E., Wolfe M. A. (2013). Hindcast of the timing of the spring phytoplankton bloom in the strait of Georgia 1968-2010. Prog. Oceanography 115, 6–13. doi: 10.1016/j.pocean.2013.05.026
Álvarez E., Moyano M., López-Urrutia Á., Nogueira E., Scharek R. (2014). Routine determination of plankton community composition and size structure: A comparison between FlowCAM and light microscopy. J. Plankton Res. 36, 170–184. doi: 10.1093/plankt/fbt069
Alves-De-Souza C., González M. T., Iriarte J. L. (2008). Functional groups in marine phytoplankton assemblages dominated by diatoms in fjords of southern Chile. J. Plankton Res. 30, 1233–1243. doi: 10.1093/plankt/fbn079
Amaya D. J., Miller A. J., Xie S. P., Kosaka Y. (2020). Physical drivers of the summer 2019 North Pacific marine heatwave. Nat. Commun. 11, 1903. doi: 10.1038/s41467-020-15820-w
Arimitsu M. L., Piatt J. F., Mueter F. (2016). Influence of glacier runoff on ecosystem structure in Gulf of Alaska fjords. Mar. Ecol. Prog. Ser. 560, 19–40. doi: 10.3354/meps11888
Arrigo K. R. (2005). Marine microorganisms and global nutrient cycles. Nature 437, 349–355. doi: 10.1038/nature04159
Balzano S., Sarno D., Kooistra W. H. C. F. (2011). Effects of salinity on the growth rate and morphology of ten Skeletonema strains. J. Plankton Res. 33, 937–945. doi: 10.1093/plankt/fbq150
Batten S. D., Raitsos D. E., Danielson S., Hopcroft R., Coyle K., McQuatters-Gollop A. (2018). Interannual variability in lower trophic levels on the Alaskan Shelf. Deep-Sea Res. Part II: Topical Stud. Oceanography 147, 58–68. doi: 10.1016/j.dsr2.2017.04.023
Becker S., Aoyama M., Woodward E. M. S., Bakker K., Coverly S., Mahaffey C., et al. (2020). GO-SHIP Repeat Hydrography Nutrient Manual: The Precise and Accurate Determination of Dissolved Inorganic Nutrients in Seawater, Using Continuous Flow Analysis Methods. Frontiers in Marine Science 7, 581790. doi: 10.3389/fmars.2020.581790
Bazin P., Jouenne F., Deton-Cabanillas A. F., Pérez-Ruzafa Á., Véron B. (2014). Complex patterns in phytoplankton and microeukaryote diversity along the estuarine continuum. Hydrobiologia 726, 155–178. doi: 10.1007/s10750-013-1761-9
Benjamini Y., Hochberg Y. (1995). Controlling the false discovery rate: a practical and powerful approach to multiple testing. J. R. Stat. society: Ser. B (Methodological) 57, 289–300. doi: 10.1111/j.2517-6161.1995.tb02031.x
Bidlack A. L., Bisbing S. M., Buma B. J., Fellman J. B., Floyd W. C., Giesbrecht I., et al. (2021). Climate-mediated changes to linked terrestrial and marine ecosystems across the northeast pacific coastal temperate rainforest margin. BioScience 71, 581–595. doi: 10.1093/biosci/biaa171
Blanchet F. G., Legendre P., Borcard D. (2008). Forward selection of explanatory variables. Ecology 89, 2623–2632. doi: 10.1890/07-0986.1
Boivin-Rioux A., Starr M., Chassé J., Scarratt M., Perrie W., Long Z., et al. (2022). Harmful algae and climate change on the Canadian East Coast: Exploring occurrence predictions of Dinophysis acuminata, D. norvegica, and Pseudo-nitzschia seriata. Harmful Algae 112, 102183. doi: 10.1016/j.hal.2022.102183
Borstad G., Crawford W., Hipfner J. M., Thomson R., Hyatt K. (2011). Environmental control of the breeding success of rhinoceros auklets at Triangle Island, British Columbia. Mar. Ecol. Prog. Ser. 424, 285–302. doi: 10.3354/meps08950
Brewin R. J. W., Sathyendranath S., Tilstone G., Lange P. K., Platt T. (2014). A multicomponent model of phytoplankton size structure. J. Geophysical Research: Oceans 119, 3478–3496. doi: 10.1002/2014JC009859
Brito A. C., Sá C., Mendes C. R., Brand T., Dias A. M., Brotas V., et al. (2015). Structure of late summer phytoplankton community in the Firth of Lorn (Scotland) using microscopy and HPLC-CHEMTAX. Estuarine Coast. Shelf Sci. 167, 86–101. doi: 10.1016/j.ecss.2015.07.006
Carmack E., Winsor P., Williams W. (2015). The contiguous panarctic Riverine Coastal Domain: A unifying concept. Prog. Oceanography 139, 13–23. doi: 10.1016/j.pocean.2015.07.014
Carstensen J., Klais R., Cloern J. E. (2015). Phytoplankton blooms in estuarine and coastal waters: Seasonal patterns and key species. Estuarine Coast. Shelf Sci. 162, 98–109. doi: 10.1016/j.ecss.2015.05.005
Catlett D., Siegel D. A., Matson P. G., Wear E. K., Carlson C. A., Lankiewicz T. S., et al. (2023). Integrating phytoplankton pigment and DNA meta-barcoding observations to determine phytoplankton composition in the coastal ocean. Limnology Oceanography 68, 361–376. doi: 10.1002/lno.12274
Cavalcanti-Lima L. F., Cutrim M. V. J., Feitosa F.A.do N., Flores-Montes M. de J, Dias F. J. S., et al. (2023). Effects of climate, spatial and hydrological processes on shaping phytoplankton community structure and β-diversity in an estuary-ocean continuum (Amazon continental shelf, Brazil). J. Sea Res. 193, 102384. doi: 10.1016/j.seares.2023.102384
Cetinić I., Rousseaux C. S., Carroll I. T., Chase A. P., Kramer S. J., Werdell P. J., et al. (2024). Phytoplankton composition from sPACE: Requirements, opportunities, and challenges. Remote Sens. Environ. 302, 113964. doi: 10.1016/j.rse.2023.113964
Choi C. J., Jimenez V., Needham D. M., Poirier C., Bachy C., Alexander H., et al. (2020). Seasonal and geographical transitions in eukaryotic phytoplankton community structure in the atlantic and pacific oceans. Front. Microbiol. 11. doi: 10.3389/fmicb.2020.542372
Clark S., Hubbard K. A., Anderson D. M., McGillicuddy D. J., Ralston D. K., Townsend D. W. (2019). Pseudo-nitzschia bloom dynamics in the Gulf of Maine: 2012–2016. Harmful Algae 88, 101656. doi: 10.1016/j.hal.2019.101656
Clarke K. R., Warwick R. M. (2001). Change in marine communities: An approach to statistical analysis and interpretation 2nd edition (Plymouth: PRIMER-E), 172 pages.
Cloern J. E., Alpinea A. E., Colea B. E., Wow R. L. J., Arthurb J. F., Ballb M. D. (1983). River discharge controls phytoplankton dynamics in the northern san francisco bay estuary. Estuarine Coast. Shelf Sci. 16, 415–429. doi: 10.1016/0272-7714(83)90103-8
Cloern J. E., Jassby A. D. (2008). Complex seasonal patterns of primary producers at the land-sea interface. Ecol. Lett. 11, 1294–1303. doi: 10.1111/j.1461-0248.2008.01244.x
Collins K. A., Allen S. E., Pawlowicz R. (2009). The role of wind in determining the timing of the spring bloom in the Strait of Georgia. Can. J. Fisheries Aquat. Sci. 66, 1597–1616. doi: 10.1139/F09-071
Crossin G. T., Filgueira R., Studholme K. R., Hipfner J. M. (2022). Phenological cues to breeding and the differential response of Pacific auks to variation in marine productivity. Mar. Ecol. Prog. Ser. 687, 163–172. doi: 10.3354/meps14015
Cuevas L. A., Tapia F. J., Iriarte J. L., González H. E., Silva N., Vargas C. A. (2019). Interplay between freshwater discharge and oceanic waters modulates phytoplankton size-structure in fjords and channel systems of the Chilean Patagonia. Prog. Oceanography 173, 103–113. doi: 10.1016/j.pocean.2019.02.012
Curry C. L., Islam S. U., Zwiers F. W., Déry S. J. (2019). Atmospheric rivers increase future flood risk in western Canada’s largest pacific river. Geophysical Res. Lett. 46, 1651–1661. doi: 10.1029/2018GL080720
Del Bel Belluz J., Peña M. A., Jackson J. M., Nemcek N. (2021). Phytoplankton composition and environmental drivers in the northern strait of Georgia (Salish sea), british columbia, Canada. Estuaries Coasts 44, 1419–1439. doi: 10.1007/s12237-020-00858-2
Di Lorenzo E., Mantua N. (2016). Multi-year persistence of the 2014/15 North Pacific marine heatwave. Nat. Climate Change 6, 1042–1047. doi: 10.1038/nclimate3082
Dodrill T. N., Pan Y., Peterson T. D. (2023). River discharge mediates extent of phytoplankton and harmful algal bloom habitat in the columbia river estuary (USA) during north pacific marine heat waves. Estuaries Coasts 46, 166–181. doi: 10.1007/s12237-022-01129-y
Du X., Campbell R., Kibler S. (2023). Seasonal changes of microphytoplankton community in prince william sound, alaska in 2019. Estuaries Coasts 46, 388–403. doi: 10.1007/s12237-022-01144-z
Dufrene D. M., Legendre P. (1997). Species assemblages and indicator species: the need for a flexible asymmetrical approach. Ecol. Monogr. 67, 345–366. doi: 10.1890/0012-9615(1997)067[0345:SAAIST]2.0.CO;2
Elferink S., Neuhaus S., Wohlrab S., Toebe K., Voß D., Gottschling M., et al. (2017). Molecular diversity patterns among various phytoplankton size-fractions in West Greenland in late summer. Deep-Sea Res. Part I: Oceanographic Res. Papers 121, 54–69. doi: 10.1016/j.dsr.2016.11.002
Erga S. R., Lie G. C., Aarø L. H., Frette Ø., Hamre B. (2015). Migratory behaviour of Skeletonema grethae (Bacillariophyceae) in stratified waters. Diatom Res. 30, 13–25. doi: 10.1080/0269249X.2014.943808
Esenkulova S., Neville C., DiCicco E., Pearsall I. (2022). Indications that algal blooms may affect wild salmon in a similar way as farmed salmon. In. Harmful Algae 118, 102310. doi: 10.1016/j.hal.2022.102310
Esenkulova S., Suchy K. D., Pawlowicz R., Costa M., Pearsall I. A. (2021). Harmful algae and oceanographic conditions in the strait of Georgia, Canada based on citizen science monitoring. Front. Mar. Sci. 8. doi: 10.3389/fmars.2021.725092
Etherington L. L., Hooge P. N., Hooge E. R., Hill D. F. (2007). Coastal and estuarine research federation oceanography of glacier bay, alaska: implications for biological patterns in a glacial fjord. Source: Estuaries Coasts 30, 927–944. doi: 10.1007/BF02841386
Fehling J., Davidson K., Bolch C., Tett P. (2006). Seasonality of Pseudo-nitzschia spp. (Bacillariophyceae) in western Scottish waters. Mar. Ecol. Prog. Ser. 323, 91–105. doi: 10.3354/meps323091
Fernandes L. F., Hubbard K. A., Richlen M. L., Smith J., Bates S. S., Ehrman J., et al. (2014). Diversity and toxicity of the diatom Pseudo-nitzschia Peragallo in the Gulf of Maine, Northwestern Atlantic Ocean. Deep-Sea Res. Part II: Topical Stud. Oceanography 103, 139–162. doi: 10.1016/j.dsr2.2013.06.022
Foreman M. G. G., Pal B., Merryfield W. J. (2011). Trends in upwelling and downwelling winds along the British Columbia shelf. J. Geophysical Research: Oceans 116(C10). doi: 10.1029/2011JC006995
Frame E. R., Lessard E. J. (2009). Does the Columbia River plume influence phytoplankton community structure along the Washington and Oregon coasts? J. Geophysical Research: Oceans 114(C2). doi: 10.1029/2008JC004999
Gao Y., Jiang Z., Chen Y., Liu J., Zhu Y., Liu X., et al. (2022). Spatial variability of phytoplankton and environmental drivers in the turbid sanmen bay (East China sea). Estuaries Coasts 45, 2519–2533. doi: 10.1007/s12237-022-01104-7
Giesbrecht I. J. W., Floyd W. C., Tank S. E., Lertzman K. P., Hunt B. P. V., Korver M. C., et al. (2021). The Kwakshua Watersheds Observatory, central coast of British Columbia, Canada. Hydrological Processes 35 (6), e14198. doi: 10.1002/hyp.14198
Giesbrecht I. J. W., Tank S. E., Frazer G. W., Hood E., Gonzalez Arriola S. G., Butman D. E., et al. (2022). Watershed classification predicts streamflow regime and organic carbon dynamics in the northeast pacific coastal temperate rainforest. Global Biogeochemical Cycles 36 (2), e2021GB007047. doi: 10.1029/2021GB007047
Goñi M. A., Welch K. A., Ghazi L., Pett-Ridge J. C., Haley B. A. (2023). Mobilization and export of particulate and dissolved solids and organic carbon from contrasting mountainous river watersheds in California and Oregon. J. Geophysical Research: Biogeosciences 128 (4), e2022JG007084. doi: 10.1029/2022JG007084
González H. E., Menschel E., Aparicio C., Barría C. (2007). Spatial and temporal variability of microplankton and detritus, and their export to the shelf sediments in the upwelling area off Concepción, Chile (∼36°S), during 2002-2005. Prog. Oceanography 75, 435–451. doi: 10.1016/j.pocean.2007.08.025
Gower J., King S., Statham S., Fox R., Young E. (2013). The Malaspina dragon: A newly-discovered pattern of the early spring bloom in the Strait of Georgia, British Columbia, Canada. Prog. Oceanography 115, 181–188. doi: 10.1016/j.pocean.2013.05.024
Haigh R., Taylor E. J. R. (1991). Mosaicism of microplankton communities in the northern Strait of Georgia, British Columbia. Mar. Biol. 110, 301–314. doi: 10.1007/BF01313717
Haigh R., Taylor F. J. R., Sutherland T. F. (1992). Phytoplankton ecology of Sechelt Inlet, a fjord system on the British Columbia coast. I. General features of the nano- and microplankton. Mar. Ecol. Prog. Ser. 89, 117–134. doi: 10.3354/meps089117
Hallegraeff G. M. (2010). Ocean climate change, phytoplankton community responses, and harmful algal blooms: A formidable predictive challenge. J. Phycology 46, 220–235. doi: 10.1111/j.1529-8817.2010.00815.x
Halverson M., Jackson J., Richards C. G., Melling H., Hunt B., Brunsting R., et al. (2017). Guidelines for processing RBR CTD profiles. Can. Tech. Rep. Hydrography Ocean Sci. 314, iv + 38 p.
Hare A., Evans W., Pocock K., Weekes C., Gimenez I. (2020). Contrasting marine carbonate systems in two fjords in British Columbia, Canada: Seawater buffering capacity and the response to anthropogenic CO2 invasion. PloS One 15 (9), e0238432. doi: 10.1371/journal.pone.0238432
Harley C. D. G., Hughes A. R., Hultgren K. M., Miner B. G., Sorte C. J. B., Thornber C. S., et al. (2006). The impacts of climate change in coastal marine systems. Ecol. Lett. 9, 228–241. doi: 10.1111/j.1461-0248.2005.00871.x
Harrison P. J., Syvertsen E. E. (1996). Marine diatoms. In Tomas C. (ed) Identification of marine diatoms and dinoflagellates. Academic Press, San Diego. Pp 5–385.
Hasle G. R., Syvertsen E. E. (1996). Marine diatoms. In Tomas C. (ed) Identification of marine diatoms and dinoflagellates. Academic Press, San Diego. Pp 5–385.
Henson S. A. (2007). Water column stability and spring bloom dynamics in the Gulf of Alaska. J. Mar. Res. 65, 715–736. doi: 10.1357/002224007784219002
Henson S. A., Cael B. B., Allen S. R., Dutkiewicz S. (2021). Future phytoplankton diversity in a changing climate. Nat. Commun. 12 (1), 5372. doi: 10.1038/s41467-021-25699-w
Hobson L. A. (2009). Phytoplankton ecology of the northeast Pacific Ocean: An illustrated guide (Victoria, B.C., Canada: LCJLMES Press), 34 pp. 83 plates.
Hobson L. A., McQuoid M. R. (1997). Temporal variations among planktonic diatom assemblages in a turbulent environment of the southern Strait of Georgia, British Columbia, Canada. Mar. Ecol. Press Ser. 150, 263–274. doi: 10.3354/meps150263
Hodal M. (2011). Net Physical Transports, Residence Times, and New Production for Rivers Inlet, British Columbia (Doctoral dissertation, University of British Columbia).
Holm-Hansen O., Lorenzen C. J., Holmes R. W., Strickland J. D. H. (1965). Fluorometric determination of chlorophyll. ICES J. Mar. Sci. 30, 3–15. doi: 10.1093/icesjms/30.1.3
Hooker S. B., Thomas C. S., Van Heukelem L., Schlüter L., Russ M. E., Ras J., et al. (2010). The fourth seaWiFS HPLC analysis round-robin experiment (SeaHARRE-4). (No. NASA/TM-2010-215857).
Horn S., Meunier C. L., Fofonova V., Wiltshire K. H., Sarker S., Pogoda B., et al. (2021). Toward improved model capacities for assessment of climate impacts on coastal bentho-pelagic food webs and ecosystem services. Front. Mar. Sci. 8. doi: 10.3389/fmars.2021.567266
Houliez E., Schmitt F. G., Breton E., Skouroliakou D. I., Christaki U. (2023). On the conditions promoting Pseudo-nitzschia spp. blooms in the eastern English Channel and southern North Sea. Harmful algae 125, 102424. doi: 10.1016/j.hal.2023.102424
Jackson J. M., Johannessen S., Del Bel Belluz J., Hunt B. P. V., Hannah C. G. (2022). Identification of a seasonal subsurface oxygen minimum in rivers inlet, British Columbia. Estuaries Coasts 45, 754–771. doi: 10.1007/s12237-021-00999-y
Jackson J. M., Johnson G. C., Dosser H. V., Ross T. (2018). Warming from recent marine heatwave lingers in deep British Columbia fjord. Geophysical Res. Lett. 45, 9757–9764. doi: 10.1029/2018GL078971
Jackson J. M., Thomson R. E., Brown L. N., Willis P. G., Borstad G. A. (2015). Satellite chlorophyll off the British Columbia Coast 1997-2010. J. Geophysical Research: Oceans 120, 4709–4728. doi: 10.1002/2014JC010496
Johannessen S. C., Macdonald R. W., Wright C. A. (2019). Rain, runoff, and diatoms: the effects of the north pacific 2014–2015 warm anomaly on particle flux in a Canadian west coast fjord. Estuaries Coasts. 42, 1052–1065. doi: 10.1007/s12237-018-00510-0
Johnson M. D., Beaudoin D. J., Frada M. J., Brownlee E. F., Stoecker D. K. (2018). High grazing rates on cryptophyte algae in chesapeake bay. Front. Mar. Sci. 5. doi: 10.3389/fmars.2018.00241
Katano T., Yoshino K., Matsubara T., Hayami Y. (2012). Wax and wane of Chattonella (Raphidophyceae) bloom with special reference to competition between Skeletonema (Bacillariophyceae) in the Ariake Sea, Japan. J. Oceanography 68, 497–507. doi: 10.1007/s10872-012-0112-1
Knepel K., Bogren K. (2008). Determination of orthophosphate by flow injection analysis. QuikChem Method 31-115-01-1-H (Loveland, Colorado: Lachat Instruments).
Kooistra W. H. C. F., Sarno D., Balzano S., Gu H., Andersen R. A., Zingone A. (2008). Global diversity and biogeography of skeletonema species (Bacillariophyta). Protist 159, 177–193. doi: 10.1016/j.protis.2007.09.004
Korver M. C., Haughton E., Floyd W. C., Giesbrecht I. J. W. (2022). High-resolution streamflow and weather data, (2013-2019) for seven small coastal watersheds in the northeast Pacific coastal temperate rainforest, Canada. Earth System Sci. Data 14, 4231–4250. doi: 10.5194/essd-14-4231-2022
Krawczyk D. W., Arendt K. E., Juul-Pedersen T., Sejr M. K., Blicher M. E., Jakobsen H. H. (2015). Spatial and temporal distribution of planktonic protists in the East Greenland fjord and offshore waters. Mar. Ecol. Prog. Ser. 538, 99–116. doi: 10.3354/meps11439
Laza-Martínez A. (2012). Urgorri complanatus GEN. ET SP. NOV. (Cryptophyceae), A red-tide-forming species in brackish waters. J. Phycology 48, 423–435. doi: 10.1111/j.1529-8817.2012.01130.x
Legendre P., Gallagher E. D. (2001). Ecologically meaningful transformations for ordination of species data. Oecologia 129, 271–280. doi: 10.1007/s004420100716
Lerner J. E., Marchese C., Hunt B. P. V. (2022). Stable isotopes reveal that bottom-up omnivory drives food chain length and trophic position in eutrophic coastal ecosystems. ICES J. Mar. Sci. 79, 2311–2323. doi: 10.1093/icesjms/fsac171
Lewitus A. J., White D. L., Tymowski R. G., Geesey M. E., Hymel S. N., Noble P. A. (2005). Adapting the CHEMTAX method for assessing phytoplankton taxonomic composition in southeastern US estuaries. Estuaries 28, 160–172. doi: 10.1007/BF02732761
Liu H., Dagg M. J., Napp J. M., Sato Liu R., Liu H., Kong J Dagg H. M., et al. (2008). Mesozooplankton grazing in the coastal Gulf of Alaska: Neocalanus spp. vs. other mesozooplankton. ICES J. Mar. Sci. 65, 351–360. doi: 10.1093/icesjms/fsm175
Longobardi L., Dubroca L., Sarno D., Zingone A. (2023). Global long-Term observations reveal wide geographic divergence in coastal phytoplankton species niches. J. Plankton Res. 45, 163–179. doi: 10.1093/plankt/fbac050
Mackey M. D., Mackey D. J., Higgins H. W., Wright S. W. (1996). CHEMTAX-a program for estimating class abundances from chemical markers: application to HPLC measurements of phytoplankton. Mar. Ecol. Prog. Ser. 144, 265–283. doi: 10.3354/meps144265
Malick M. J., Cox S. P., Mueter F. J., Peterman R. M. (2015). Linking phytoplankton phenology to salmon productivity along a north-south gradient in the Northeast Pacific Ocean. Can. J. Fisheries Aquat. Sci. 72, 697–708. doi: 10.1139/cjfas-2014-0298
Marañón E., Cermeño P., Latasa M., Tadonléké R. D. (2012). Temperature, resources, and phytoplankton size structure in the ocean. Limnology Oceanography 57, 1266–1278. doi: 10.4319/lo.2012.57.5.1266
Marchese C., Hunt B. P. V., Giannini F., Ehrler M., Costa M. (2022). Bioregionalization of the coastal and open oceans of British Columbia and Southeast Alaska based on Sentinel-3A satellite-derived phytoplankton seasonality. Front. Mar. Sci. 9. doi: 10.3389/fmars.2022.968470
Marić Pfannkuchen D., Godrijan J., Smodlaka Tanković M., Baričević A., Djakovac T., Pustijanac E., et al. (2018). The ecology of one cosmopolitan, one newly introduced and one occasionally advected species from the genus skeletonema in a highly structured ecosystem, the northern adriatic. Microbial Ecol. 75, 674–687. doi: 10.1007/s00248-017-1069-9
McCabe R. M., Hickey B. M., Kudela R. M., Lefebvre K. A., Adams N. G., Bill B. D., et al. (2016). An unprecedented coastwide toxic algal bloom linked to anomalous ocean conditions. Geophysical Res. Lett. 43, 10,366–10,376. doi: 10.1002/2016GL070023
McCabe R. M., Hickey B. M., Trainer V. L. (2023). The pacific northwest harmful algal blooms bulletin. Harmful Algae 127, 102480. doi: 10.1016/j.hal.2023.102480
McKenzie C. H., Deibel D., Thompson R. J., MacDonald B. A., Penney R. W. (1997). Distribution and abundance of choanoflagellates (Acanthoecidae) in the coastal cold ocean of Newfoundland, Canada. Mar. Biol. 129, 407–416. doi: 10.1007/s002270050181
McLaskey A. K., Forster I., Del Bel Belluz J., Hunt B. P. V. (2022). A high-resolution time series of particulate matter fatty acids reveals temporal dynamics of the composition and quality available to zooplankton in a temperate coastal ocean. Prog. Oceanography 206, 102843. doi: 10.1016/j.pocean.2022.102843
McLaskey A. K., Forster I., Hunt B. P. V. (2024). Distinct trophic ecologies of zooplankton size classes are maintained throughout the seasonal cycle. Oecologia 204, 227–239. doi: 10.1007/s00442-023-05501-y
Melliti Ben Garali S., Sahraoui I., de la Iglesia P., Chalghaf M., Diogène J., Ksouri J., et al. (2016). Effects of nitrogen supply on Pseudo-nitzschia calliantha and Pseudo-nitzschia cf. seriata: field and laboratory experiments. Ecotoxicology 25, 1211–1225. doi: 10.1007/s10646-016-1675-1
Menden-Deuer S., Morison F., Montalbano A. L., Franzè G., Strock J., Rubin E., et al. (2020). Multi-instrument assessment of phytoplankton abundance and cell sizes in mono-specific laboratory cultures and whole plankton community composition in the North Atlantic. Front. Mar. Sci. 7. doi: 10.3389/fmars.2020.00254
Mendes C. R. B., Costa R. R., Ferreira A., Jesus B., Tavano V. M., Dotto T. S., et al. (2023). Cryptophytes: An emerging algal group in the rapidly changing Antarctic Peninsula marine environments. Global Change Biol. 29, 1791–1808. doi: 10.1111/gcb.16602
Mouw C. B., Barnett A., McKinley G. A., Gloege L., Pilcher D. (2016). Phytoplankton size impact on export flux in the global ocean. Global Biogeochemical Cycles 30, 1542–1562. doi: 10.1002/2015GB005355
Mutshinda C. M., Finkel Z. V., Widdicombe C. E., Irwin A. J. (2016). Ecological equivalence of species within phytoplankton functional groups. Funct. Ecol. 30, 1714–1722. doi: 10.1111/1365-2435.12641
Nemcek N., Hennekes M., Sastri A., Perry R. I. (2023). Seasonal and spatial dynamics of the phytoplankton community in the Salish Sea 2015–2019. Prog. Oceanography 217, 103108. doi: 10.1016/j.pocean.2023.103108
Oksanen J., Blanchet F., Fiendly M., Kindt R., Legendre P., McGlinn D., et al. (2019). Vegan: Community ecology package. R package version 2.5-6. Available online at: https://www.cran.r-project.org/web/packages/vegan/index.html (Accessed February 11, 2023).
Olli K., Tamminen T., Ptacnik R. (2023). Predictable shifts in diversity and ecosystem function in phytoplankton communities along coastal salinity continuum. Limnology Oceanography Lett. 8, 173–180). doi: 10.1002/lol2.10242
Oseji O. F., Fan C., Chigbu P. (2019). Composition and dynamics of phytoplankton in the Coastal Bays of Maryland, USA, revealed by microscopic counts and diagnostic pigments analyses. Water (Switzerland) 11 (2), 368. doi: 10.3390/w11020368
Patil J. S., Anil A. C. (2011). Variations in phytoplankton community in a monsoon-influenced tropical estuary. Environ. Monit. Assess. 182, 291–300. doi: 10.1007/s10661-011-1876-2
Peña M. A., Fine I., Callendar W. (2019a). ). Interannual variability in primary production and shelf-offshore transport of nutrients along the northeast Pacific Ocean margin. Deep-Sea Res. Part II: Topical Stud. Oceanography, 169, 104637. doi: 10.1016/j.dsr2.2019.104637
Peña M. A., Nemcek N., Robert M. (2019b). Phytoplankton responses to the 2014–2016 warming anomaly in the northeast subarctic Pacific Ocean. Limnology Oceanography 64, 515–525. doi: 10.1002/lno.11056
Perry R. I., Nemcek N., Hennekes M., Sastri A., Ross A. R. S., Shannon H., et al. (2023). Domoic acid in Canadian Pacific waters, from 2016 to 2021, and relationships with physical and chemical conditions. Harmful Algae 129, 102530. doi: 10.1016/j.hal.2023.102530
Peterson T. D., Toews H. N. J., Robinson C. L. K., Harrison P. J. (2007). Nutrient and phytoplankton dynamics in the Queen Charlotte Islands (Canada) during the summer upwelling seasons of 2001-2002. J. Plankton Res. 29, 219–239. doi: 10.1093/plankt/fbm010
Pinseel E., Nakov T., Van den Berge K., Downey K. M., Judy K. J., Kourtchenko O., et al. (2022). Strain-specific transcriptional responses overshadow salinity effects in a marine diatom sampled along the Baltic Sea salinity cline. ISME J. 16, 1776–1787. doi: 10.1038/s41396-022-01230-x
Pitman K. J., Moore J. W., Sloat M. R., Beaudreau A. H., Bidlack A. L., Brenner R. E., et al. (2020). Glacier retreat and pacific salmon. BioScience 70, 220–236. doi: 10.1093/biosci/biaa015
Platt T., Sathyendranath S., Fuentes-Yaco C. (2007). Biological oceanography and fisheries management: perspective after 10 years. ICES J. Mar. Sci. 64, 863–869. doi: 10.1093/icesjms/fsm072
Pramlall S., Jackson J. M., Konik M., Costa M. (2023). Merged multi-sensor ocean colour chlorophyll product evaluation for the british columbia coast. Remote Sens. 15 (3), 687. doi: 10.3390/rs15030687
Pramlall S., Jackson J. M., Marchese C., Suchy K. D., Hunt B. P. V., Costa M. (2024). Mapping phenoregions and phytoplankton seasonality in Northeast Pacific marine coastal ecosystems via a satellite-based approach. Prog. Oceanography 228, 103336. doi: 10.1016/j.pocean.2024.103336
Pratt D. M. (1966). Competition between Skeletonema costatum and Olisthodiscus luteus in Narragansett Bay and in culture. Limnology Oceanography 11, 447–455. doi: 10.4319/lo.1966.11.4.0447
Radić V., Cannon A. J., Menounos B., Gi N. (2015). Future changes in autumn atmospheric river events in British Columbia, Canada, as projected by CMIP5 global climate models. J. Geophysical Res. 120, 9279–9302. doi: 10.1002/2015JD023279
Ramette A. (2007). Multivariate analyses in microbial ecology. FEMS Microbiol. Ecol. 62, 142–160. doi: 10.1111/j.1574-6941.2007.00375.x
Redfield A. C., Ketchum B. H., Richards F. A. (1963). The influence of organisms on the composition of seawater. sea 2, 26–77.
Rosen S., Bianucci L., Jackson J. M., Hare A., Greengrove C., Monks R., et al. (2022). Seasonal near-surface hypoxia in a temperate fjord in Clayoquot Sound, British Columbia. Front. Mar. Sci. 9. doi: 10.3389/fmars.2022.1000041
Rounce D. R., Hock R., Maussion F., Hugonnet R., Kochtitzky W., Huss M., et al. (2023). Global glacier change in the 21st century: Every increase in temperature matters. Science 379, 78–83. doi: 10.1126/science.abo1324
Salmaso N., Zignin A. (2010). At the extreme of physical gradients: Phytoplankton in highly flushed, large rivers. Hydrobiologia 639, 21–36. doi: 10.1007/s10750-009-0018-0
Sarno D., Kooistra W. H. C. F., Medlin L. K., Percopo I., Zingone A. (2005). Diversity in the genus Skeletonema (Bacillariophyceae). II. An assessment of the taxonomy of S. costatum-like species with the description of four new species. J. Phycology 41, 151–176. doi: 10.1111/j.1529-8817.2005.04067.x
Schmidt K., Birchill A. J., Atkinson A., Brewin R. J. W., Clark J. R., Hickman A. E., et al. (2020). Increasing picocyanobacteria success in shelf waters contributes to long-term food web degradation. Global Change Biol. 26, 5574–5587. doi: 10.1111/gcb.15161
Sejr M. K., Bruhn A., Dalsgaard T., Juul-Pedersen T., Stedmon C. A., Blicher M., et al. (2022). Glacial meltwater determines the balance between autotrophic and heterotrophic processes in a Greenland fjord. Proc. Natl. Acad. Sci. United States America 119 (52), e2207024119. doi: 10.1073/pnas.2207024119
Sjöqvist C., Godhe A., Jonsson P. R., Sundqvist L., Kremp A. (2015). Local adaptation and oceanographic connectivity patterns explain genetic differentiation of a marine diatom across the North Sea-Baltic Sea salinity gradient. Mol. Ecol. 24, 2871–2885. doi: 10.1111/mec.13208
Smith P. J. (1991). Taxonomic survey of nanoplankton in Saanich Inlet, B.C. (M.Sc. Thesis, University of Victoria), Pp 1–198.
Smith P., Bogren K. (2003). Determination of nitrate and/or nitrite in brackish or seawater by flow injection analysiscolorimetry. QuikChem Method 31-107-04-1-E (Loveland, Colorado: Lachat Instruments).
Spalding M. D., Fox H. E., Allen G. R., Davidson N., Ferdana Z. A., Finlayson M., et al. (2007). Marine ecoregions of the world: A bioregionalization of coastal and shelf areas. Bioscience 57, 573–583. doi: 10.1641/B570707
Steidinger K. A., Tangen K. (1996). Dinoflagellates. In Tomas C. (ed) Identification of marine diatoms and dinoflagellates. Academic Press, San Diego, Pp 387–598.
St. Pierre K. A., Hunt B. P. V., Giesbrecht I. J. W., Tank S. E., Lertzman K. P., Del Bel Belluz J., et al. (2022). Seasonally and spatially variable organic matter contributions from watershed, marine macrophyte, and pelagic sources to the northeast pacific coastal ocean margin. Front. Mar. Sci. 9. doi: 10.3389/fmars.2022.863209
St. Pierre K. A., Oliver A. A., Tank S. E., Hunt B. P. V., Giesbrecht I., Kellogg C. T. E., et al. (2020). Terrestrial exports of dissolved and particulate organic carbon affect nearshore ecosystems of the Pacific coastal temperate rainforest. Limnology Oceanography 65, 2657–2675. doi: 10.1002/lno.11538
Strom S. L., Brainard M. A., Holmes J. L., Olson M. B. (2001). Phytoplankton blooms are strongly impacted by microzooplankton grazing in coastal North Pacific waters. Mar. Biol. 138, 355–368. doi: 10.1007/s002270000461
Strom S. L., Macri E. L., Olson M. B. (2007). Microzooplankton grazing in the coastal Gulf of Alaska: Variations in top-down control of phytoplankton. Limnology Oceanography 52, 1480–1494. doi: 10.4319/lo.2007.52.4.1480
Strom S. L., Olson M. ,. B., Macri E. L., Mordy C. W. (2006). Cross-shelf gradients in phytoplankton community structure, nutrient utilization, and growth rate in the coastal Gulf of Alaska. Mar. Ecol. Prog. Ser. 328, 75–92. doi: 10.3354/meps328075
Šupraha L., Bosak S., Ljubešić Z., Mihanović H., Olujić G., Mikac I., et al. (2014). Cryptophyte bloom in a Mediterranean estuary: High abundance of Plagioselmis cf. prolonga in the Krka River estuary (eastern Adriatic Sea). Scientia marina 78, 329–338. doi: 10.3989/scimar.03998.28C
Swan C. M., Vogt M., Gruber N., Laufkoetter C. (2016). A global seasonal surface ocean climatology of phytoplankton types based on CHEMTAX analysis of HPLC pigments. Deep-Sea Res. I 109, 137–156. doi: 10.1594/PANGAEA.855412
Taucher J., Bach L. T., Prowe A. E. F., Boxhammer T., Kvale K., Riebesell U. (2022). Enhanced silica export in a future ocean triggers global diatom decline. Nature 605, 696–700. doi: 10.1038/s41586-022-04687-0
Throndsen J. (1993). The planktonic marine flagellates. In Tomas C. (ed) Marine phytoplankton. Academic Press, San Diego, pp. 7–145.
Taylor F., Haigh R., Sutherland T. (1994). Phytoplankton ecology of Sechelt Inlet, a fjord system on the British Columbia coast. II. Potentially harmful species. Mar. Ecol. Prog. Ser. 104, 151–164. doi: 10.3354/meps104151
Tommasi D., Hunt B. P. V., Pakhomov E. A., Mackas D. L. (2013). Mesozooplankton community seasonal succession and its drivers: Insights from a British Columbia, Canada, fjord. J. Mar. Syst. 115–116, 10–32. doi: 10.1016/j.jmarsys.2013.01.005
Trainer V. L., Hickey B. M., Lessard E. J., Cochlan W. P., Trick C. G., Wells M. L., et al. (2009). Variability of Pseudo-nitzschia and domoic acid in the Juan de Fuca eddy region and its adjacent shelves. Limnology Oceanography 54, 289–308. doi: 10.4319/lo.2009.54.1.0289
Trainer V. L., Kudela R. M., Hunter M. V., Adams N. G., McCabe R. M. (2020). Climate extreme seeds a new domoic acid hotspot on the US west coast. Front. Climate 2. doi: 10.3389/fclim.2020.571836
Tréguer P., Bowler C., Moriceau B., Dutkiewicz S., Gehlen M., Aumont O., et al. (2018). Influence of diatom diversity on the ocean biological carbon pump. Nat. Geosciences 11 (1), 27–37. doi: 10.1038/s41561-017-0028-x
Tucker S. (2010). “Determination of silicate in brackish or seawater by flow injection analysis,” in QuikChem method 31-114-27-2-A (Lachat Instruments, Loveland, Colorado).
Unrein F., Massana R., Alonso-Sáez L., Gasol J. M. (2007). Significant year-round effect of small mixotrophic flagellates on bacterioplankton in an oligotrophic coastal system. Limnology Oceanography 51, 456–469. doi: 10.4319/lo.2007.52.1.0456
Utermöhl H. (1931). Neue Wege in der quantitativen Erfassung des Planktons (mit besonderer Berücksichtigung des Ultraplanktons). Verh. int. Ver. theor. angew. Limnol. 5, 567–596.
Waite J. N., Mueter F. J. (2013). Spatial and temporal variability of chlorophyll-a concentrations in the coastal Gulf of Alaska 1998-2011, using cloud-free reconstructions of SeaWiFS and MODIS-Aqua data. Prog. Oceanography 116, 179–192. doi: 10.1016/j.pocean.2013.07.006
Wasmund N., Tuimala J., Suikkanen S., Vandepitte L., Kraberg A. (2011). Long-term trends in phytoplankton composition in the western and central Baltic Sea. J. Mar. Syst. 87, 145–159. doi: 10.1016/j.jmarsys.2011.03.010
Weber C., Olesen A. K. J., Krock B., Lundholm N. (2021). Salinity, a climate-change factor affecting growth, domoic acid and isodomoic acid C content in the diatom Pseudo-nitzschia seriata (Bacillariophyceae). Phycologia 60, 619–630. doi: 10.1080/00318884.2021.1973789
Whitney F. A., Crawford W. R., Harrison P. J. (2005). Physical processes that enhance nutrient transport and primary productivity in the coastal and open ocean of the subarctic NE Pacific. Deep-Sea Res. Part II: Topical Stud. Oceanography 52, 681–706. doi: 10.1016/j.dsr2.2004.12.023
Widdicombe C. E., Eloire D., Harbour D., Harris R. P., Somerfield P. J. (2010). Long-term phytoplankton community dynamics in the Western English Channel. J. Plankton Res. 32, 643–655. doi: 10.1093/plankt/fbp127
Winder M., Sommer U. (2012). Phytoplankton response to a changing climate. Hydrobiologia 698, 5–16. doi: 10.1007/s10750-012-1149-2
Wolfe A. M., Allen S. E., Hodal M., Pawlowicz R., Hunt B. P. V., Tommasi D. (2016). Impact of advection loss due to wind and estuarine circulation on the timing of the spring phytoplankton bloom in a fjord. ICES J. Mar. Sci. 73, 1589–1609. doi: 10.1093/icesjms/fsv151
Xu T., Newman M., Capotondi A., Stevenson S., Di Lorenzo E., Alexander M. A. (2022). An increase in marine heatwaves without significant changes in surface ocean temperature variability. Nat. Commun. 13 (1), 7396. doi: 10.1038/s41467-022-34934-x
Yamasaki Y., Nagasoe S., Matsubara T., Shikata T., Shimasaki Y., Oshima Y., et al. (2007). Allelopathic interactions between the bacillariophyte Skeletonema costatum and the raphidophyte Heterosigma akashiwo. Mar. Ecol. Prog. Ser. 339, 83–92. doi: 10.3354/meps339083
Yano R., Ohara S., Koike K. (2023). High light stress under phosphorus limitation in summer may accelerate diatom shift from Skeletonema to Chaetoceros in an oligotrophic coastal area of Japan. Front. Mar. Sci. 10. doi: 10.3389/fmars.2023.1095762
Yoo Y., Seong K. A., Jeong H. J., Yih W., Rho J. R., Nam S. W., et al. (2017). Mixotrophy in the marine red-tide cryptophyte Teleaulax amphioxeia and ingestion and grazing impact of cryptophytes on natural populations of bacteria in Korean coastal waters. Harmful Algae 68, 105–117. doi: 10.1016/j.hal.2017.07.012
Keywords: phytoplankton composition, Skeletonema, Pseudo-nitzschia, cryptophyte blooms, coastal, British Columbia, northeast Pacific
Citation: Del Bel Belluz J, Jackson JM, Kellogg CTE, Peña MA, Giesbrecht IJW and Hobson LA (2024) Phytoplankton community composition links to environmental drivers across a fjord to shelf gradient on the central coast of British Columbia. Front. Mar. Sci. 11:1458677. doi: 10.3389/fmars.2024.1458677
Received: 03 July 2024; Accepted: 06 November 2024;
Published: 09 December 2024.
Edited by:
Alberto Basset, University of Salento, ItalyReviewed by:
Jose Luis Iriarte, Austral University of Chile, ChileIan Salter, Alfred Wegener Institute Helmholtz Centre for Polar and Marine Research (AWI), Germany
Copyright © 2024 Del Bel Belluz, Jackson, Kellogg, Peña, Giesbrecht and Hobson. This is an open-access article distributed under the terms of the Creative Commons Attribution License (CC BY). The use, distribution or reproduction in other forums is permitted, provided the original author(s) and the copyright owner(s) are credited and that the original publication in this journal is cited, in accordance with accepted academic practice. No use, distribution or reproduction is permitted which does not comply with these terms.
*Correspondence: Justin Del Bel Belluz, anVzdGluLmJlbGx1ekBoYWthaS5vcmc=