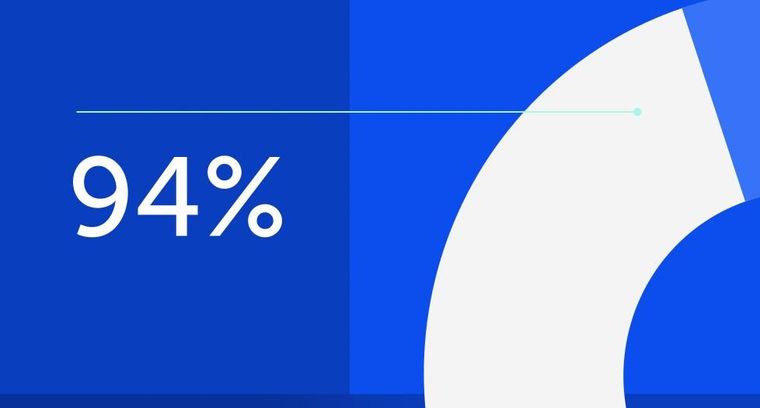
94% of researchers rate our articles as excellent or good
Learn more about the work of our research integrity team to safeguard the quality of each article we publish.
Find out more
ORIGINAL RESEARCH article
Front. Mar. Sci., 03 September 2024
Sec. Marine Fisheries, Aquaculture and Living Resources
Volume 11 - 2024 | https://doi.org/10.3389/fmars.2024.1436755
This article is part of the Research TopicTowards an Expansion of Sustainable Global Marine AquacultureView all 13 articles
The mortality of Atlantic salmon is one of the main challenges to achieving its sustainable production. This sector benefits from generating many data, some of which are collated in a standardized way, on a monthly basis at site level, and are accessible to the public. This continuously updated resource might provide opportunities to monitor mortality and prompt producers and inspectors to further investigate when mortality is higher than expected. This study aimed to use the available open-source data to develop production cycle level dynamic linear models (DLMs) for monitoring monthly mortality of maricultured Atlantic salmon in Scotland. To achieve this, several production cycle level DLMs were created: one univariate DLM that includes just mortality; and various multivariate DLMs that include mortality and different combinations of environmental variables. While environmental information is not collated in a standardized way across all sites, open-source remote-sensed satellite resources provide continuous, standardized estimates. By combining environmental and mortality data, we seek to investigate whether adding environmental variables enhanced the estimates of mortality, and if so, which variables were most informative in this respect. The multivariate model performed better than the univariate DLM (P = .004), with salinity as the only significant contributor out of 12 environmental variables. Both models exhibited uncertainty related to the mortality estimates. Warnings were generated when any observation fell above the 95% credible interval. Approximately 30% of production cycles and more than 50% of sites experienced at least one warning between 2015 and 2020. Occurrences of these warnings were non-uniformly distributed across space and time, with the majority happening in the summer and autumn months. Recommendations for model improvement include employing shorter time periods for data aggregation, such as weekly instead of on a monthly basis. Furthermore, developing a model that takes hierarchical relationships into account could offer a promising approach.
Over the past few decades, farmed Atlantic salmon has been a major contributor to the growth of international trade in fisheries and aquaculture products. It is an important global food source with a key role to play in food security and nutrition (FAO, 2022). Scotland is the third largest farmed salmon producer in the world with a production share of 7.6%, behind Norway (55.3%) and Chile (25.4%) (Iversen et al., 2020). Scottish salmon is amongst the top food export products of the UK (Department for Environment, Food & Rural Affairs, 2023), and the industry generates income in remote areas with few other opportunities (Murray et al., 2021).
The production of farmed salmon consists of two distinct phases: freshwater and seawater. To transition between these phases, salmon undergo a process called smoltification, during which they develop the ability to move from freshwater to seawater, becoming smolts (FAO, 2004). In this study, only the seawater phase was considered. Initially, salmon are raised in large tanks of freshwater for about 10 to 16 months and then moved to open net pens in seawater for about 14 to 22 months (Walde et al., 2023). There has been a tendency to keep smolts as long as possible in freshwater, where they face fewer challenges (Bjørndal and Tusvik, 2018; Hilmarsen et al., 2018). The farms in the open sea are usually referred to as sites and contain one generation of fish at a time, with the time between stocking and harvesting being one production cycle. A production cycle refers to a site-level period in which at least one pen on a site is occupied consecutively (Boerlage et al., 2017). It is recommended to fallow a site (entire site has no fish) for a minimum of 4 weeks before a new generation of salmon is introduced again (Scottish Salmon Producers Organisation, 2014). In 2022, around 55.2 million smolts were put to sea in Scotland, resulting in a total annual production of 169,194 tons (Munro, 2023).
Mortality rates are one of the main constraints to the sustainability of the industry. In Scotland, the mortality rates in the marine phase were approximately 24% in 2020, 26% in 2019 and 23% in 2018 (Munro, 2023). Mortality represents a significant economic loss to the producers and is considered an indicator of suboptimal fish welfare (Noble et al., 2018). Due to the open net-pen structures in which salmon are cultured, salmon are exposed to the natural environment that directly impacts their well-being. Salmon mortality can be influenced by several factors, including infectious and non-infectious agents. Examples of the most important infectious contributors are sea lice and sea lice treatments (Boerlage et al., 2024), and gill disease and cardiomyopathies (Mowi, 2022). Non-infectious agents are algal blooms, predators and the natural environmental conditions of the water (Sommerset et al., 2022). Optimal ranges have been determined for environmental variables, such as sea surface temperature, salinity, pH and dissolved oxygen requirements (Noble et al., 2018). Outside of these ranges, health and welfare of salmon may be impacted, resulting in increased mortalities.
Salmon aquaculture has become one of the most technologically advanced industries (FAO, 2022), with an increasing accumulation of data collected. Most producers have sensors that monitor the environment continuously. Additionally, all companies collect and store mortality data in their management programs. Some governments, such as in Scotland, collect and collate monthly mortality data from all aquaculture producers of all sites, in a standardized way, which is subsequently made publicly accessible. This valuable, continuously updated resource might provide an opportunity to develop an industry-wide monitoring model for mortality that does not require additional administrative complexities. Such a monitoring model could help identify events where mortality is higher than expected and prompt producers and inspectors to investigate the event further. Including environmental information into the monitoring model could enhance the predictions. Although salmon sites monitor and record many environmental variables, such information is not collated in a standardized way. A promising solution emerges from the widespread availability of open-source environmental data derived from satellites, which are standardized and publicly available (Thakur et al., 2018).
Using open-source databases collected by governments to model mortality of maricultured salmon has been done before. Recent studies have used different modelling methods, each with a different approach regarding the relevant variables to include. Moriarty et al. (2020) included only sea temperature and fish biomass, while others, such as Oliveira et al. (2021) and Tvete et al. (2022) included a wider range of variables such as sea temperature, sea salinity, fish weight at stocking, sea lice information or the occurrence of pancreas disease. These studies found sea temperature and salinity to be key drivers of farmed salmon mortality. However, these models assumed a fixed relationship between the response variable and the predictors, which may not hold over time as the underlying process changes.
Dynamic linear models (DLMs), a special case of state-space models, allow for time-varying parameters, which enables the model to adjust to changes in the underlying processes that generate the data over time (West and Harrison, 1997). DLMs can easily incorporate exogenous variables and it is possible to include terms to model trends and seasonality to improve the predictions. In addition, DLMs utilize a Bayesian framework where historical knowledge is combined with current data to detect changes within an observed process. This approach enables a more comprehensive understanding of the situation, facilitating well-informed decision-making (Kristensen et al., 2010). Thus, the use of DLMs is a promising approach to monitor farmed salmon mortality. In other farmed animal species, DLMs have been applied and proven effective in monitoring animal production (Dominiak et al., 2019a, 2019b; Jensen et al., 2016, 2017; Skjølstrup et al., 2022). To the farmed salmon industry, they have been applied only to a limited extent. One example of using such models for salmon aquaculture modelling is by Elghafghuf et al. (2020) who compared different state-space models in estimating the sea lice infestation pressure in salmon sites. Furthermore, a state-space monitoring model for salmon mortality and movement has been developed for wild Pacific salmon (Newman, 1998).
The purpose of this study was to use the available open-source mortality and environmental data to develop production cycle level DLMs for monitoring monthly mortality of maricultured Atlantic salmon in Scotland. We intended to assess if these already existing resources can give valuable information to be used in the surveillance of mortality for Scottish salmon aquaculture, by triggering warnings when mortality is higher than expected. This can inform producers, veterinarians and inspectors, alerting them to further investigate. More specifically, we had four objectives: (1) to create a univariate production cycle level DLM using mortality data from salmon sites in Scotland; (2) to create multivariate production cycle level DLMs combining both mortality and environmental data. With this combination, we investigated if adding environmental data improved the estimates of mortality; (3) to compare the univariate and multivariate models and select the best model for monitoring salmon mortality; (4) to create warnings when observed mortality exceeded the expected levels.
Data cleaning, manipulation and modelling were performed using the statistical programming environment R (R Core Team, 2022) and RStudio (Posit team, 2022). The time-series analysis workflow is freely accessible (https://doi.org/10.5281/zenodo.10617901).
We used two different types of data: salmon production data and environmental data.
Scotland’s Aquaculture website (https://aquaculture.scotland.gov.uk/; last accessed 9 February 2023) hosts an open access database containing various datasets with information on the aquaculture industry of Scotland. This study utilizes data from the “Fish Farm Monthly Biomass and Treatments” dataset owned by the Scottish Environment Protection Agency (SEPA). This dataset contains information about all fish species produced in Scotland and is submitted by all producers on a monthly basis. The salmon production data used in this study consists exclusively of data of Atlantic salmon (Salmo salar L.) from the period between 2002 and 2020 (entire period available at the time of extraction).
The environmental data used in this study is remotely sensed data from satellites. The environmental variables included were: temperature, salinity, concentration of phytoplankton, chlorophyll, dissolved oxygen, precipitation, concentration of dinoflagellates, diatoms, nanophytoplankton, picophytoplankton, pH and concentration of nitrate. Precipitation data (Huffman et al., 2019) was obtained from NASA’s Earthdata platform (https://urs.earthdata.nasa.gov; last accessed on 9 February 2023). The other variables were obtained from E.U. Copernicus Marine Service Information (Tonani et al., 2022a, 2022b) and downloaded through Copernicus Marine Environment Monitoring Service (https://marine.copernicus.eu/services-portfolio/access-to-products/; last accessed on 9 February 2023). All environmental data are open-source and reported daily. To match the production data, the time period downloaded was from 2002 to 2020.
The descriptive statistics of the variables used throughout the study are shown in Table 1.
All active marine salmon sites in Scotland between 2002 and 2020 were part of the dataset. Not all sites were active during the entire study period, either because they started production after the beginning of the study period, or because they discontinued it during the study period. Therefore, some sites made a first appearance in the study after 2002 and others disappeared before 2020. It resulted in a total of 402 sites and 2138 production cycles.
Scotland is geographically divided into six regions: Highland, Argyll & Bute, Shetland Islands, Eilean Siar, Orkney Islands, and North Ayrshire (Figure 1). The region North Ayrshire only has one site, therefore, we grouped it with the nearest region (Argyll & Bute) and only the remaining five regions were considered.
Figure 1. Salmon sites distributed across the six regions of Scotland. Created using the leaflet R package (Cheng et al., 2024).
During the data cleaning process, some sites and production cycles were excluded from the study. First, production cycles for which it could not be guaranteed that they were in the dataset from stocking to harvesting were removed from the study. Production cycles with persistently missing or zero values for mortality (kg) or biomass (kg) throughout the entire production cycle were also excluded (92 production cycles). Records that did not meet the standard production cycle for commercial purposes, which we defined as between 6 and 36 months, were dropped (224 production cycles). Sites with less than 2 years of reported data (24 months) in the study period were also removed (24 sites). Finally, incoherent production cycles where information from some months was not reported were excluded from the study (33 production cycles).
The mortality reported corresponded to the number of kilograms of dead salmon per month per site (Table 1). We converted mortality to a proportion to take the production size into account, using the reported biomasses. Estimating biomasses at fish farming sites is based on management program algorithms and food intake data, typically periodically adjusted through sampling to determine the average fish weight. This average weight is then multiplied by the number of existing fish on the site, calculated as the initial number of fish put into the sea minus the countable dead fish (Costa et al., 2006). Mortality values for analysis were calculated as:
We regarded some mortality proportions as unrealistic. Reasons possibly included data entry mistakes; or stocking, harvesting or moving fish between sites in the middle of a month, which timing and quantity of fish moved were not available to us. This absence of movement data introduced a source of error in our proportion estimates, as the reported biomasses were a snapshot of the biomasses at the end of the month, while mortality accounts for the cumulative mortalities throughout the month. Moving fish from a site during the month leads to lower biomasses reported at the end of a month relative to the mortalities observed during the month, whereas introducing fish to a site during the month leads to higher reported biomasses relative to the observed mortalities. To reduce this source of error as much as possible, we identified unrealistic mortality proportions and we made them missing. For that, we defined biomasses lower than realistic as:
and biomasses higher than realistic as:
where corresponds to the current month and to the previous month. As shown in Equations 2 and 3, we set limits of 20% deviation from expected biomasses, below and above which biomasses were considered abnormal. In Equation 3, additionally we used the feed intake reports to foresee how much the salmon where expected to grow in each month. Although the feed conversion ratio (FCR) for salmon fluctuates throughout a production cycle, for the sake of simplification, we adopted a reported FCR of 1.3 for salmon in the United Kingdom (Torrissen et al., 2011), meaning that 77% of feed intake is transformed in weight gain. Equations 2 and 3 were applied to all reported biomasses on the dataset. When the biomasses were considered lower or higher than realistic, these observations were replaced by missing values. Therefore, we restricted the analysis to only realistic biomasses, which were used to calculate mortality as defined in Equation 1.
The environmental data (2002-2020) have three dimensions: spatial, temporal and depth. These data were transformed into observations of 20 km around a site (spatial), monthly (temporal) and averaged of 0, 3, and 10 meters below the surface (depth; precipitation only at 0 meters). These transformations were accomplished using the R packages: tidyverse (Wickham et al., 2019), janitor (Firke et al., 2023), lubridate (Grolemund and Wickham, 2011), ncdf4 (Pierce, 2023), raster (Hijmans et al., 2023), sp (Pebesma et al., 2018) and sf (Pebesma et al., 2022).
Spatial coverage of environmental data from satellite images was not available for around 65% of the exact site locations for all environmental variables except for precipitation which was available for 100% of the locations. Because aquaculture sites are often next to the shore or in sea lochs, estimates for this data at the aquaculture site location are often missing. Reasons are the compromised pixel edge lengths and coastal effects, dissolved organic compounds of terrestrial origin, and weather patterns (Thakur et al., 2018). Dropping these sites would bias our study towards offshore sites and leave the study with too few observations. Therefore, we used buffer zones of 20 km around the aquaculture sites and averaged the values within that buffer as a proxy for site location. For 24 sites we could not obtain environmental data even with the 20 km buffer, as they were situated too close to the shore or far inside sea lochs. As a result, these sites were not part of the study.
Temporal coverage meant that daily environmental data obtained were aggregated into monthly data to have the same dimensions as the mortality data (Table 1). We aggregate the environmental data with the intention of looking at both extremes and variation. For extremes, we aggregated into monthly data by using the 1st and 9th deciles to best capture the amount of abnormal days with more extreme environmental factors needed to cause salmon mortality (Table 2). For the environmental variables where both increases and decreases can lead to salmon mortality, we used both deciles, while for variables where only increases were of interest, we applied only the 9th decile. The only exception is for precipitation, where we used the 9th decile even though only decreases might affect mortality. The reason is that relying solely on the 1st decile would often result in a value of 0, which would lose the impact of precipitation. Thus, we looked at its negative effect, when the 9th decile is lower than normal should lead to salmon mortality. To investigate the effect of relatively quick changes in environmental variables, i.e. their variation, we designed variables that portrayed the maximum daily variation out of a month for the variables where variability may affect mortality (Table 2). This was not the case for precipitation, nor for the phytoplankton and chlorophyll type variables (Phytoplankton, Chlorophyll, Dinoflagellates, Diatoms, Nanophytoplankton, Picophytoplankton) because they usually appear as blooms (a sudden increase of the concentration in seawater) (Brown et al., 2020). Therefore, the maximum daily variation on a month would be similar to what is given by the 9th decile.
For depth coverage, we determined the depth level to be an average of values at 0, 3 and 10 meters depth. Salmon swim vertically to the depth that better meets their physiological needs, e.g. fish swim to deeper depths in the summer than in the winter to avoid the stronger surface light (Oppedal et al., 2007). Thus, using only one of the reported depths would not be an accurate measure of what salmon were exposed to.
After cleaning both environmental and mortality data, they were combined into one dataset. Our study population was reduced to 293 seawater Scottish salmon sites, corresponding to 1610 production cycles distributed in five regions of Scotland.
The residuals of a DLM should follow a normal distribution (West and Harrison, 1997). To assess if this assumption was met, a univariate DLM was run for each input variable individually. For some variables, a logarithmic transformation was needed (Table 2). Two variables that were subject to logarithmic transformation included zeros. On those cases, the logarithmic transformation was conducted as: where α ∈]0,1].
The variances of the different variables were adjusted to a similar scale by standardizing each variable individually. It was accomplished by subtracting the sample mean of the variable from all observations and then dividing them by the sample standard deviation.
The available data were split into a learning set (145 sites, distributed by five regions of Scotland, and 784 production cycles) for estimating parameters for the DLMs and a test set (the same 145 sites and 353 other production cycles) for validating the models.
This division was done by chronologically dividing the dataset into two parts, where the first three quarters of the data were used as a learning set, while the remaining quarter was used as the test set. A division at a specific date would cut production cycles, having parts of some production cycles in the learning set and other parts of the same production cycles in the test set. Instead, we used different cut-off dates at each site as close to the three quarters reference as possible, ensuring that production cycles would remain intact. Sites with less than two production cycles on the learning set were excluded.
For the expectation maximization (EM) algorithm, which was used in the training phase of the model, the learning set was again divided into a training set and validation set with a three quarters-one quarter split, in the same way described above. Sites where all mortality or environmental information was missing on the EM algorithm training set were excluded.
Only the sites which were present in all four sets - EM training set, EM validation set, DLM learning set and DLM test set - were included in this study. Therefore, all sets include the same sites.
Dynamic linear models (DLMs) are generally used to estimate the true state of a given variable at each time t, by filtering the random noise. DLMs use a Bayesian framework to base their estimates on observed data, while incorporating any prior knowledge available prior to a given observation. Besides, DLMs do not follow the assumption that the estimates remain constant over time, allowing them to have systematic fluctuations and changes as time passes (West and Harrison, 1997).
A DLM is represented by a combination of two equations, namely the observation equation (Equation 4) and the system equation (Equation 5).
The observation equation (Equation 4) describes how the values of an observation vector (Yt) depend on underlying (unobservable) parameters (θt). The transposed design matrix ) extracts the expected values of the observable variables from the parameter vector the system equation (Equation 5) is what makes the DLMs dynamic since it updates the values from time t – 1 to time t, through the system matrix (Gt). The observational variance-covariance matrix (Vt) is where the uncertainty about the observations is depicted. The systematic variance-covariance matrix (Wt) represents how uncertain we are about how much each element of the system will randomly change from one time step to another and how changes in one element affect changes in all other elements, and vice versa.
For a complete specification of the DLM, the matrices Ft, Gt, Vt and Wt must be given together with the initial distribution of . The prior information/belief at time (before any observations are made) is presented as D0, which consists of the initial mean and a variance-covariance matrix (C0).
In this study, the systematic variance-covariance matrix (Wt) was assumed constant, so that . The dimensions of the observational variance-covariance matrix (Vt) and the transposed design matrix ) changed over time according to which variables had missing observations at a given time t. Thus, the missing observations at any given time step were ignored. The system matrix (Gt) was not constant for the variables that do not have a seasonal pattern. In those cases, Gt was updated at each month t.
Two types of production cycle level DLMs (univariate and multivariate) were created and are explained in detail in the following subsections. The term “production cycle level” indicates that the predictions will be made individually per production cycle based on prior information given at country level. Therefore, these models do not account for the potential relationships within the same sites or regions.
A univariate production cycle level DLM (West and Harrison, 1997) was used to monitor salmon mortality on a monthly basis (mortality defined as explained in Table 2).
In this case, Yt consisted of only one value (the observed mortality for month t). The parameter vector (θt) contained the level and a trend factor for the variable mortality at time t. The initial level of m0 was calculated by fitting a spline function to the mortality data available in the learning set (all sites), which was then used to predict the mortality value for t = 0 (initial level). The spline function was created using the default settings of the smooth.spline function available in stats R package (R Core Team, 2022). It was decided to use the default settings, as the role of the spline’s exact shape is less important here than in classical static models due to the dynamic model’s inherent capability to adapt over time. The trend factor was initially set to 1, indicating that before any observations are made, we expected the system to evolve exactly in accordance with the estimated spline function. A trend component greater than 1 would then correspond to a trend (positive or negative) being faster than the average, while a trend component less than 1 would correspond to a trend which is slower than the average.
The prior variance (C0) was determined by computing the covariance between the differences amid all two consecutive mortality observations for the first six observed mortality values of each production cycle on the learning set and the first six original observed mortality values.
The matrix was represented in the univariate case as:
This structure serves to separate the level and the trend of , when is multiplied by on the observation equation (Equation 4). The result was the predicted mortality value at each time t.
The Gt matrix for the univariate case was defined as:
with
where and being the expected log-transformed mortality at times and , respectively, given by the spline function. Thus is the expected rate of change in log-transformed mortality values from time to time .
The observational variance-covariance matrix (V) expressed the uncertainty about the mortality observations. To make an initial estimate for V, which in the univariate case was a scalar (only one value), we calculated a two-sided moving average with a moving window equal to five months to each production cycle on the learning set individually. Then, the residuals between the observed and estimated values of each production cycle were combined. The variance of the combined residuals corresponds to the initial estimate for V.
Finally, matrix was defined as:
where expresses how uncertain we were about the evolution of the mortality level, expresses how uncertain we were about the evolution of the trend factor, and the off-diagonal elements & are equal and represent the systematic covariance between the evolution of mortality level and the evolution of the trend factor. The systematic variance-covariance matrix (W) was initially estimated by diving the prior variance, C0 by 10.
Afterwards, we optimized the values in V and W using the expectation maximization (EM) algorithm, as described in subsection 2.4.3.
In multivariate models, more than one variable is modelled simultaneously. Here, all environmental variables (21) and mortality (all represented in Table 2) were given as inputs. Thus, the multivariate DLM forecasted the 22 variables expected values for each month. The hypothesis was that this multivariate model would learn from previous mortality and environmental data and work on associations between the variables to give an accurate prediction of mortality considering the environmental factors of that month.
Most environmental variables had a clear seasonal pattern (see for example Figure 2). This knowledge was included in the DLMs to improve the predictions by using a linear combination of trigonometric functions (sine and cosine, also known as harmonic waves), called the Fourier form representation (West and Harrison, 1997). For each variable, we assessed the sum of harmonic waves that better reflected its seasonality. To do so, we used a linear regression to get the relationship between the observations of each variable on the learning set and a trigonometric function representing a sum of a specific number of harmonic waves. Each of the harmonic waves has its own frequency ranging from 1 cycle per year to 6 cycles per year (the Nyquist harmonic). The Nyquist harmonic corresponds to the maximum number of waves allowed for a given period (West and Harrison, 1997). In this study, the Nyquist harmonic corresponded to the 6th harmonic wave . The harmonic with the lowest frequency represents the main annual pattern, whereas those with higher frequencies account for deviations from the overall pattern. For a graphical illustration of the method, reference is made to (Dominiak et al 2019a, Figure 7).
Figure 2. Seasonal pattern in daily temperature data from 2002 to 2020, utilizing a 20 km buffer and averaging across the three depths and all sites.
Next, we measured the fit of the trigonometric function to the data using the adjusted Coefficient of determination (R2). We successively tested several sums of harmonic waves and when the adjusted R2 stopped improving, the number of harmonic waves that had the highest adjusted R2 was selected as the optimal for that variable. The best number of harmonic waves for each variable is presented in Table 3. For some variables the best number of harmonic waves is 0, meaning that those variables did not have a seasonal pattern.
In Supplementary Figure S1 is illustrated the expected patterns for each variable modelled with the respective sum of harmonic waves or with spline functions (for the variables without seasonal pattern). The data points shown are from one arbitrary site, but the waves and the splines were defined based on data from all sites. The x-axis for the variables with seasonal pattern correspond to the calendar months, whereas for the variables that did not show any seasonality correspond to the months of the production cycle since stocking.
The process of creating a multivariate DLM involves combining the univariate models required to represent each variable individually, as exemplified previously with mortality, while also considering the interdependencies between those variables.
Here, the Yt consisted on a vector with all observed values in month t for the 22 variables. Also, θt was a vector containing the underlying parameters for all variables in month t. A linear regression based on the best number of harmonic waves (Table 3) for a period of 12 months was created as previously explained for each seasonal variable individually, and its coefficient estimates were used in m0. The coefficient estimates values corresponded to the intercept and one sine and cosine wave for each harmonic wave needed. For example, for the variable d1.temp the best number of harmonic waves was 2 (Table 3) thus, 5 coefficient estimates were allocated to m0. For the variables that used all waves possible (including the Nyquist harmonic) the last sine wave could not be calculated. In those cases, only the available information (12 coefficient estimates) was added to m0. For the variables that did not show seasonal patterns, i.e. the variables related to mortality and salinity (Table 3), the levels were calculated with spline functions as explained on the univariate case and the trend factors were again defined as 1. Therefore, the unobservable parameter vector had 137 elements.
To compute the prior variance (C0), firstly the vcov function available on stats R package (R Core Team, 2022) was applied to each linear regression previously created for each seasonal variable. This function returned a variance-covariance matrix of the main parameters of each regression model. For all the variables that do not have seasonality, the variance-covariance matrices were calculated as explained in the univariate model. Then, the final variance-covariance matrix (C0) was a combination of each variable individual variance-covariance matrix.
In the multivariate case, the system matrix (Gt) was also a combination of each variable’s individual G matrix. A system submatrix describing a single harmonic wave is defined as:
where and defines the frequency. If a = 1, the frequency is 1 full cycle per year. If a = 2, the frequency is 2 cycles per year, etc. The case a = 6 corresponds to 6 cycles per year (the Nyquist harmonic), which is a special case represented as:
For the variables without cyclical patterns, the G matrix was defined as explained for the univariate case. Therefore, the system matrix for the multivariate corresponded to a 137 × 137 matrix and was defined as:
where (being the expected log-transformed mortality given by the spline function). Due to space constraints, it was not possible to present the complete Gt matrix. Thus, we decided to show the three different designs present in Gt: the system matrix with 2 harmonic waves for the variable d1.temp (upper left corner block), the Nyquist harmonic for log.d9.pico (middle block) and the system matrix for log.mortality (lower right corner block).
In the multivariate DLMs the transposed design matrix has the same aim as in the univariate case: separate the observable values from the trend factor or harmonic waves (depending on the variable). Therefore, the had repeated structures according to the number and the type of variables used (with or without seasonal patterns). The transposed design matrix in the multivariate case, when no observation was missing in month , corresponded to a 22 × 137 matrix depicted as:
Again, it was not possible to show the complete matrix due to space restrictions. Thus, we presented the designs corresponding to the same three variables shown on Gt: d1.temp (upper left corner block), log.d9.pico (middle block) and log.mortality (lower right corner block). When there was a missing observation for some variable in month t, the row corresponding to that variable on the matrix was excluded.
The observational variance-covariance matrix (Vt) was a quadratic matrix with the number of rows and columns being equal to the number of variables without missing observations in month , being defined as:
where the maximum value of was 22 (total number of variables). If d1.temp is considered variable number 1 and log.mortality variable number 22, is the observational variance of d1.temp, is the observational variance of log.mortality, and and correspond to the observational covariance between d1.temp and log.mortality. An initial estimate of V was created by placing the individual observational variances of each variable along the diagonal, while the off-diagonal values were set to . The observational variances of the variables without seasonality were calculated as explained in the univariate DLM, while the observational variances for the variables with seasonal patterns were defined by computing the variance of each linear regression residuals formerly created.
The systematic variance-covariance matrix was initially determined by dividing the prior variance by 10 (as in the univariate case), being a matrix.
As in the univariate case, the initial estimates of Vt and W were optimized using the EM algorithm as described in sub-section 2.4.3. The values located in the off-diagonal areas of Vt and W contribute with the additional information about how the different variables mutually affect each other.
We initially developed a multivariate production cycle level DLM incorporating all available variables (21 environmental variables and mortality), as previously explained in subsection 2.4.2. However, our subsequent analysis revealed that utilizing all this information might not be the most efficient strategy. Some of the environmental variables may not influence mortality and including them could result in a more complex and computationally demanding model than required. Nevertheless, we have provided a thorough explanation of the most intricate model, thus creating other models with fewer variables is a simple matter of excluding from the initial specifications the irrelevant variables.
A systematic approach would entail the construction of individual multivariate production cycle DLMs for all possible variable combinations. This would result in over 2 million possibilities. Instead, we made a DLM per variable group, and then applied the stepwise forward selection method.
In the first step, seven multivariate production cycle level DLMs were created, each one using the variables included in each environmental variable group (Temperature, Salinity, Phytoplankton and Chlorophyll, Dissolved oxygen, Precipitation, Nitrate, pH) plus the variable mortality. To compare the performance of the seven multivariate DLMs, it was necessary to evaluate how different the mortality observations were from the predictions, represented as forecast errors. This evaluation was conducted by calculating the Root Mean Squared Error (RMSE) for the collective set of mortality forecast errors across all production cycles within each DLM. A lower RMSE signifies a higher level of model precision, allowing the comparative analysis of the models. Considering our aim of investigating if adding environmental variables improves the predictions of mortality, the RMSEs from the seven multivariate models were compared against the RMSE of the univariate model. If those RMSEs were lower than the RMSE of the univariate model, it was considered to improve the mortality predictions.
The second step was to build six multivariate DLMs each one with the variables that provided the best DLM on the first step and one other environmental variable group per model. In this way, we could understand if adding any other environmental group improved the estimates of mortality. The RMSEs were compared against the RMSE of the best performing multivariate DLM from the first step.
In the third step, we wanted to assess if all variables present on the best DLM from the second step were relevant to the model. In that sense, several multivariate DLMs consisting of all possible variables combinations were created. The RMSEs were compared against the RMSE of the best model from the second step.
To see if we could improve the best DLM so far, the fourth step involved employing the stepwise forward selection method. It consisted of building a multivariate DLM with the most promising selection of variables identified thus far and adding all other variables, one at a time. The model with the lowest RMSE was designated as the best.
The expectation maximization (EM) algorithm (West and Harrison, 1997) was used to optimize the systematic variance-covariance matrix (W) and the full version of the observational variance-covariance matrix (V) for both the univariate and the multivariate versions of the DLM.
The EM algorithm is a mathematical method that estimates unknown parameters by finding the most likely outcome based on observed data. It involves a series of iterations, which implies calculating the likelihood of the data given previous estimates, and then refining those estimates based on the new information (Dethlefsen, 2001).
Running the EM algorithm is usually computationally demanding, especially if working with large datasets or using several variables simultaneously. To tackle this challenge we ran the EM algorithm using an early stopping technique. For that, we started by dividing the learning set into a training set, consisting of the first three quarters of the data and a validation set, consisting of the last quarter, as previously explain in section 2.3. After each iteration of the EM algorithm, the DLM with the most recent set of variance components (W and V) was applied to the validation set, and the root mean squared error (RMSE) of the forecast errors was calculated. When the RMSE (rounded to 4 decimal places) stopped decreasing, the EM algorithm was terminated and the variance components which minimized the RMSE were returned.
Since the initial specifications needed were already calculated (m0, C0Ft, Gt, Vt and W) the next step was to update the models. The updating procedure for the DLMs was computed by utilizing the Kalman filter updating equations (filtering) as described by West and Harrison (1997). The values were forecast at each time step, relying on the current estimate of the mean and prior information about error and variance around both the system and the data. These values were subsequently “corrected” according to the new observation, where the predicted values by the model and the actual observed values were compared, and the forecast errors were used to improve the estimated value of the next time step. As a result of the Kalman filter, we got the monthly expected values (filtered mean and variance) and the forecasts for each variable (mortality in the univariate DLM and all 22 variables in the full multivariate DLM).
The parameter vectors θt are autocorrelated to each other through the system equation. In the Kalman filter, we only used the previous information to obtain the best estimate of θt. However, owing to the autocorrelation present between the parameter vectors, the subsequent observations have as much useful information to estimate the true values of θt as the past observations. Therefore, a retrospective analysis called smoothing can be employed, where data are analyzed from the latest update and working backwards to the initial point, as outlined by West and Harrison (1997). This retrospective analysis is useful because we obtain the best possible estimates for each variable, which are important since they can provide better knowledge about the effects of specific events, like disease outbreaks (Kristensen et al., 2010).
Warnings were generated when the observed mortality values fell above the 95% credible intervals (CI). The 95% CI were calculated using the forecasted values (ft) produced by the Kalman filter, along with its respective variance Qt:
where . Whenever a warning was triggered, it indicated that the mortality was higher than expected for that time step on that production cycle and further investigation is required.
The following results were obtained by applying the DLMs to the test set while using the initial specifications calculated based on the learning set. Every production cycle in the test set (a total of 353 production cycles across 145 sites) was subjected to both univariate and multivariate DLMs individually.
The outcomes of the univariate DLM for production cycle number 614 are illustrated in Figure 3. This production cycle was chosen because it has almost no missing data and no warnings were detected. Specifically, these outcomes are the filtered mean estimated by the prospective Kalman filter and the smoothed mean estimated by the retrospective smoothing (Figure 3A), and the forecasted values produced at each time step in the Kalman filter (Figure 3B). All outcomes are presented with their corresponding 95% credible interval, based on their respective variance components. The filtered mean can be interpreted as the best possible estimate of the true underlying mortality level given all previous information at each time step, while the smoothed mean can be interpreted as the best possible estimate of the true underlying mortality level given all available information prior to and after a given time step. Both means (filtered and smoothed) demonstrated a consistent alignment with the observations (Figure 3A). The 95% credible interval for the mortality forecasts (Figure 3B) was wider than the 95% credible intervals of both filtered and smoothed means (Figure 3A). This suggests that there is a higher level of uncertainty in the predictions (Figure 3B), with a RMSE value of .
Figure 3. Outcomes from the univariate DLM applied to production cycle number 614. Observations (obs) in black. (A) In green: Filtered mean (mt) and the respective 95% credible interval (CI); In blue: Smoothed mean (mts) and the respective 95% credible interval (CI). (B) In red: Forecasts (ft) and the respective 95% credible interval (CI).
Even though errors associated with the predictions existed, it was possible to detect warnings in several production cycles. Figure 4 illustrates the mortality forecasts for production cycle number 466 and it shows that the observations exceed the predictions 95% credible interval at month 11 after stocking.
Figure 4. Outcomes from the univariate DLM applied to production cycle number 466. Observations (obs) in black and the forecasts (ft) and the respective 95% credible interval (CI) in red; Circle: warning.
Between 2015 and 2020, 109 out of the 353 production cycles exhibited at least one warning. Among these 109 cycles, 77% experienced a single warning during the cycle, 20% had two warnings, and 3% encountered three.
Out of the 145 sites, 86 experienced warnings, affecting all five regions. The region of Eilean Siar had the highest occurrence rate with 28 production cycles with at least one warning out of 63 production cycles (44%) and 36 warnings in total. The region Orkney Islands showed the lowest number of warnings, having 6 production cycles with at least one warning out of 55 production cycles (11%) and 8 warnings in total, as depicted in Table 4.
Table 4. Warnings identified in the univariate and multivariate DLMs between 2015 and 2020: per region, month of the year and year.
Concerning the months of the year with more warnings (Table 4), the upward trend commenced in April with 12 warnings in 490 production cycle-months that took place during April (2.4%). July (3.7%), August (6.8%), September (5.1%), and October (4.3%) stood out as the months with the highest occurrences of warnings.
The year 2016 had the highest frequency of warnings with 42 occurrences within 903 production cycle-months (4.7%), followed by 2017 (2.9%) and 2018 (2.2%) (Table 4). In 2015, only three months of data were available, making direct comparisons not applicable.
In the process of selecting the most relevant variables to be used on the multivariate production cycle level DLM, the first step was to create seven multivariate DLMs each one using the variables included in one environmental variable group, in addition to the mortality variable (Table 5). The RMSEs from the models were compared against the RMSE of the univariate model . As shown in Table 5, the model including the salinity variables had a better performance (lowered the RMSE).
Table 5. First step - Information about the multivariate DLMs created per variable group, in addition to the mortality variable.
For the second step, six multivariate DLMs were built, each one with the variables that provided the best DLM thus far (salinity related variables and mortality) and one other environmental variable group per model. The RMSEs were compared against the RMSE of the multivariate DLM that used the salinity variables and mortality . Supplementary Table S1 shows that the models’ performances did not improve by adding any of the other environmental groups.
For the third step, we generated seven DLMs consisting of all possible combinations using the salinity variables (Table 6). Two combinations improved the performances when compared to using all salinity variables. The best DLM used the variables log.d9.sal, log.max.daily.range.sal and log.mortality (lower RMSE).
Table 6. Third step - Information about the multivariate DLMs created with all possible combinations using salinity variables, and mortality.
The fourth and last step consisted of applying the stepwise forward selection method. The results are illustrated in Supplementary Table S2, and demonstrate that the inclusion of additional variables did not lead to improved performances. Therefore, the best multivariate DLM in predicting the mortality estimates was the DLM that included the variables log.d9.sal, log.max.daily.range.sal and log.mortality, with a RMSE of .
The best multivariate DLM had a smaller RMSE than the univariate DLM ( and , respectively). To determine whether this very small difference is statistically significant or not, a paired t-test was applied to the squared forecast errors of the univariate and best multivariate DLMs. T-tests are known to be useful in studies with large sample sizes and are robust even for skewed data (Fagerland, 2012). We intend to compare the variances; therefore, it is natural to square the forecast errors. The resulting p-value was , which indicated that both models are significantly different from each other. We concluded that the best multivariate DLM performed better than the univariate DLM.
The subsequent results are based on the best and final multivariate DLM, which is henceforth referred to simply as multivariate DLM. Figures 5–7 show the outcomes of the multivariate DLM for production cycle 614 (the same presented in the univariate DLM results section). Figures 5 and 6 illustrate the filtered and the smoothed mean (A), and the forecasts (B) for the logarithmic transformation of the 9th decile of salinity (log.d9.sal) and for the maximum daily range of salinity (log.max.daily.range.sal), respectively.
Figure 5. Outcomes related to the variable log.d9.sal from the multivariate DLM applied to production cycle number 614. Observations (obs) in black. (A) In green: Filtered mean (mt) and the respective 95% credible interval (CI); In blue: Smoothed mean (mts) and the respective 95% credible interval (CI). (B) In red: Forecasts (ft) and the respective 95% credible interval (CI).
Figure 6. Outcomes related to the variable log.max.daily.range.sal from the multivariate DLM applied to production cycle number 614. Observations (obs) in black. (A) In green: Filtered mean (mt) and the respective 95% credible interval (CI); In blue: Smoothed mean (mts) and the respective 95% credible interval (CI). (B) In red: Forecasts (ft) and the respective 95% credible interval (CI).
Figure 7. Outcomes related to the variable log.mortality from the multivariate DLM applied to production cycle number 614. Observations (obs) in black. (A) In green: Filtered mean (mt) and the respective 95% credible interval (CI); In blue: Smoothed mean (mts) and the respective 95% credible interval (CI). (B) In red: Forecasts (ft) and the respective 95% credible interval (CI).
Concerning the logarithmic transformation of mortality (log.mortality), Figure 7A shows that once again the filtered and smoothed means demonstrated a consistent alignment with the observations. The 95% credible interval of the forecasts (Figure 7B) was also wider than the 95% credible intervals of both filtered and smoothed means (Figure 7A). This shows that the uncertainty in the predictions on mortality continued after adding the environmental variables considered most relevant. The multivariate DLM was also capable of giving warnings in production cycle 466, as shown in Supplementary Figure S2.
Between 2015 and 2020, 99 out of 353 production cycles experienced at least one warning from the multivariate model. All these 99 production cycles also generated warnings in the univariate model. In line with the univariate DLM, 79% of the 99 production cycles had one warning, 19% had two warnings, and 2% had three.
Among the 145 sites, 79 generated warnings, all of which were also identified when using the univariate model. All regions generated warnings (Table 4). The region of Eilean Siar recorded the highest occurrence rate (40%) and the Orkney Islands the lowest (11%), consistent with what we found in the univariate DLM.
Regarding the seasonality of the warnings produced by the multivariate model, an increase was seen in April (2.4%), with July (3.7%), August (5.5%), September (4.6%) and October (3.4%) having the highest occurrence of warnings (Table 4), similar to the pattern seen with the univariate model.
In accordance with the univariate DLM, warnings occurred most frequently in the year 2016 (4.0%), followed by 2017 at 2.5% and 2018 at 2.0%, as seen in Table 4. Once again, the year 2015 is not applicable for comparisons due to the limited availability of data.
One univariate and various multivariate production cycle level DLMs were developed using open-source data. The main goal was to investigate the value of these already collected data for monitoring monthly mortality of maricultured Atlantic salmon in Scotland. The best DLM consisted of mortality and salinity related variables. Both the univariate and the final multivariate DLMs exhibited a degree of uncertainty in the mortality predictions. Nevertheless, both models were capable of giving warnings about unexpected increases in mortality. If implemented in a near real-time surveillance system, these warnings can be used by stakeholders such as salmon producers to further investigate the observation and possibly detect (emerging) diseases. Therefore, we demonstrated that, despite the underlying data being of low resolution, the open-source data sources can be successfully used as part of a monitoring system. This has the potential to provide stakeholders with valuable information without requiring additional efforts such as more data collection or developing more data sharing agreements.
The uncertainty associated with the forecasts of mortality in both models led to wide 95% credible intervals. Many different factors have likely contributed to the uncertainty observed in the predictions of mortality. First, salmon mortality can be influenced by various factors that were not considered in this study. For example, an increase in mortality can be caused by non-infectious and infectious health challenges such as pathogens like sea lice (Noble et al., 2018), and a decrease can be caused by the mitigation measures carried out by health managers. Information on cause-specific high mortality is openly available and can be found in Salmon Scotland’s monthly mortality reports (https://www.salmonscotland.co.uk/reports; last accessed 5 January 2024), but these were unavailable during most of our study period. Incorporating these unaddressed factors into our models might have decreased the uncertainties about the predictions of mortality, but there are concerns that suboptimal validity of the cause-specific salmon mortality can lead to selection and misclassification bias when using this information to model mortality (Aunsmo et al., 2008). Second, the absence of movement data and, consequently, the indirect method used to detect the movements most likely did not capture all movements of fish. Therefore, the calculated mortality has itself associated uncertainty. Third, it would have been optimal to train the models with data where the mortality was known to be “normal” to ensure that the models learned the “normal” patterns, as was done by Jensen et al. (2016). The lack of health and welfare information about fish populations in the database made it impossible to determine what normal mortality was, and thus it was not possible to separate production cycles with only normal mortality for the learning set from those with abnormal mortality. That being said, other studies have successfully developed DLMs for monitoring purposes in the past without knowing the normal state of the animals (Bono et al., 2012, 2013, 2014; Dominiak et al., 2019a, 2019b).
Favorable environmental conditions are of paramount importance for the survival of salmon (Noble et al., 2018; Murray et al., 2022). Therefore, several multivariate DLMs including different environmental variables were made to better understand which environmental factors influence salmon mortality in Scotland. Our study demonstrated that including salinity related variables is relevant for predicting salmon mortality in Scotland. This is similar to the findings of Oliveira et al. (2021) and Tvete et al. (2022) who also described salinity as an important environmental factor. Temperature is commonly described as having significant influence in salmon mortality (Elliott and Elliott, 2010; Moriarty et al., 2020), but including it did not improve the mortality predictions further.
Uncertainty was also embedded in the environmental variables. We used buffer zones of 20 km around the aquaculture sites and averaged the environmental values within that buffer as a proxy for site location, to optimally use the satellite data. As a result, the values used did not exactly represent the environmental factors experienced by the salmon. Furthermore, we used the daily mean of three depths (0, 3, and 10 meters) to aggregate data on a monthly basis, rather than incorporating each depth in the models. While this approach saved computational time, the resulting values used in the models do not precisely correspond to the environmental conditions that salmon experienced. Despite exploration of many different types of aggregation of the environmental variables per month (e.g. different quartile levels), extremes that are known to be outside of comfort levels for salmon would always be under detected if durations were brief (less than 3 days using the 1st and 9th deciles). Moreover, suboptimal conditions may have no effect when salmon are healthy, but may affect health and welfare if salmon are stressed or have underlying conditions (Noble et al., 2018). Therefore, some important information may have been lost in the aggregations. These simplifications, such as buffer, depth and monthly aggregations could have contributed to the uncertainty seen in the mortality predictions, and could be the reason for the environmental variables considered most relevant in the literature had little effect on the predictions of mortality in this study.
Salmon farming sites monitor and record many environmental factors using sensors, which are typically more accurate and have greater resolution than satellite data (Thakur et al., 2018). However, there is a lack of standardization across all sites. Not all relevant environmental factors are measured at every site, protocols vary between sites (e.g. depths) and the data are not collected centrally from all sites at near real-time, as it is done for mortality data. Therefore, it is more suitable to use satellite data when creating models considering different sites. Sharing data between companies requires data-sharing agreements, which can be difficult. Using both satellite data and the open-source mortality dataset provided a chance to develop models without adding administrative complexities.
This study provided insights into the occurrence and distribution of warnings in Scotland. A warning indicated that salmon mortality was higher than expected. Access to raw mortality data alone does not clarify whether increased mortality is normal for the phase of the production cycle or merely a result of natural variation. With the warnings generated (using credible intervals), stakeholders are alerted to instances of increased mortality that are beyond natural variation and are higher than expected for the specific phase of the production cycle. When comparing the warnings generated by the univariate and multivariate models, it is noteworthy that both models exhibit similar results. However, the univariate model generated a greater number of warnings overall. Approximately 30% of the production cycles and more than 50% of the sites experienced at least one warning between 2015 and 2020. Considering the wide 95% credible interval of the models, the real values might be higher. Geographically, the generated warnings exhibited a non-uniform distribution across Scotland. The region of Eilean Siar had the highest warning rate and the Orkney Islands had the lowest. These findings were different from a previous study that applied a specific threshold to mortality events in Scotland between 2002 and 2009, and found that more warnings were generated on the Northern Islands (Orkney Islands and Shetland Islands) (Salama et al., 2016). In our study, most warnings happened between July and October, which is when the water temperature is higher. As temperature increases, salmon’s metabolic activity also rises, leading to a greater demand for oxygen. In addition, the amount of dissolved oxygen in the water decreases as the water temperature increases (Noble et al., 2018). Also, infectious agents, such as Neoparamoeba perurans, the causative agent of Amoebic Gill Disease (AGD), and sea lice proliferate at higher rates in warmer waters (Oldham et al., 2016; Brooker et al., 2018; Murray et al., 2022). Studies have shown that salmon mortality due to Pancreas disease is also higher in the summer months (Kilburn et al., 2012). Therefore, an increase in warnings during this period may be the result of a higher incidence of disease outbreaks and unfavorable conditions. Another interesting finding was the declining trend in the frequency of warnings from 2016 to 2020. The year 2016 had the highest prevalence of warnings in our study, while the year 2017 was reported as the one with highest total mortality between 2015 and 2020 (Munro, 2023). However, it should be noticed that the warnings generated by these models are related to unexpected changes in mortality, not necessarily to total mortality. It is unclear to us why the frequency of warnings decreased after 2016, although one of the reasons may be the establishment of gill disease that changed from emerging disease to being a consistent (and thus expected) constraint. Many other reasons could have contributed to this observation.
The warnings generated in this study were defined as any observation falling above the 95% credible interval. Nevertheless, other methods could have been used to generate warnings using the DLMs outputs. Examples of other methods include the Tabular Cumulative Sums and the V-mask (Antunes et al., 2017).
We utilized DLMs despite encountering non-normally distributed residuals in some of the original variables. To address this issue, we applied a logarithmic transformation to these variables prior to analysis and assessed whether the assumption of normality was satisfied. The practice of transforming data is frequently employed to conform to Gaussian assumptions, see for example Larsen et al. (2019). The selection of the DLM was based on its computational ease of use, allowing a more seamless execution on computer systems compared to other more complex models. The logarithmic transformation can be easily transformed back to the original values, ensuring that the interpretation of results can be conducted in terms of the original data scale.
With the available open-source data it was possible to design a monitoring model for mortality with a certain level of uncertainty. Suggestions for improvements of the models that may reduce uncertainty include using shorter time periods when aggregating the mortality data (e.g. weekly). Another approach might be to develop a novel structure that would integrate the monthly mortality data and the daily environmental data into a framework that could be utilized in the multivariate DLM. Furthermore, including reasons for mortality and movement data may improve the monitoring process. Some of these improvements require additional data collection efforts as they are not currently being collected from all producers.
The next step of this project is to develop a hierarchical DLM that will use the same mortality data used in this study. It is a more complex model in which the mutual interconnectedness between all sites in all regions are taken into account, with the sites in the same region being assumed to be more closely correlated than sites in different regions. Such a hierarchical framework has the potential to enhance the monitoring of salmon mortality, offering a more comprehensive and insightful perspective on the complex factors influencing salmon mortality.
The open-source Scottish salmon data can be used to monitor salmon mortality, allowing stakeholders to be informed when mortality is higher than expected. One key advantage of this dataset is that it has already been collated and does not require data sharing agreements. Nevertheless, a degree of uncertainty was found in the mortality predictions in both univariate and multivariate DLMs. This uncertainty may be reduced if mortality data collected on a shorter time period (such as weekly), additional data on relevant factors that influence salmon mortality and movement data are made publicly available in the future and are included in the models. Moreover, using salinity information from open-source environmental data improved the mortality predictions, even with the monthly aggregations carried out. This study presents a systematic and extensive framework for constructing univariate and multivariate DLMs, and the codes used are freely accessible. Future research will focus on creating a hierarchical DLM that considers site, regional, and country levels.
Publicly available datasets were analyzed in this study. This data can be found here: https://aquaculture.scotland.gov.uk, https://urs.earthdata.nasa.gov, https://data.marine.copernicus.eu.
CM: Conceptualization, Data curation, Formal analysis, Investigation, Methodology, Software, Visualization, Writing – original draft. AB: Conceptualization, Methodology, Resources, Supervision, Writing – review & editing. AK: Conceptualization, Formal analysis, Funding acquisition, Methodology, Resources, Software, Supervision, Writing – review & editing. DJ: Conceptualization, Formal analysis, Funding acquisition, Methodology, Resources, Software, Supervision, Writing – review & editing.
The author(s) declare financial support was received for the research, authorship, and/or publication of this article. This work has received funding from the European Union’s Horizon 2020 research and innovation program under grant agreement No. 101000494 (DECIDE).
The authors declare that the research was conducted in the absence of any commercial or financial relationships that could be construed as a potential conflict of interest.
The author(s) declared that they were an editorial board member of Frontiers, at the time of submission. This had no impact on the peer review process and the final decision.
All claims expressed in this article are solely those of the authors and do not necessarily represent those of their affiliated organizations, or those of the publisher, the editors and the reviewers. Any product that may be evaluated in this article, or claim that may be made by its manufacturer, is not guaranteed or endorsed by the publisher.
This document reflects only the author’s view and the Research Executive Agency (REA) and the European Commission cannot be held responsible for any use that may be made of the information it contains.
The Supplementary Material for this article can be found online at: https://www.frontiersin.org/articles/10.3389/fmars.2024.1436755/full#supplementary-material
Antunes A. C. L., Jensen D., Halasa T., Toft N. (2017). A simulation study to evaluate the performance of five statistical monitoring methods when applied to different timeseries components in the context of control programs for endemic diseases. PloS One 12, e0173099. doi: 10.1371/journal.pone.0173099
Aunsmo A., Bruheim T., Sandberg M., Skjerve E., Romstad S., Larssen R. B. (2008). Methods for investigating patterns of mortality and quantifying cause-specific mortality in sea-farmed Atlantic salmon Salmo salar. Dis. Aquat. Organ 81, 99–107. doi: 10.3354/dao01954
Bjørndal T., Tusvik A. (2018). Økonomisk analyze av alternative produksjonsfomer innan oppdrett (in Norwegian: An economic analysis of alternative production technologies in aquaculture). SNF-prosjekt nr. 5730. Bergen, Norway.
Boerlage A. S., Shrestha S., Leinonen I., Jansen M. D., Revie C. W., Reeves A., et al. (2024). Sea lice management measures for farmed Atlantic salmon (Salmo salar) in Scotland: Costs and effectiveness. Aquaculture 580, 740274. doi: 10.1016/j.aquaculture.2023.740274
Boerlage A. S., Stryhn H., Sanchez J., Hammell K. L. (2017). Case definition for clinical and subclinical bacterial kidney disease (BKD) in Atlantic Salmon (Salmo salar L.) in New Brunswick, Canada. J. Fish Dis. 40, 395–409. doi: 10.1111/jfd.12521
Bono C., Cornou C., Kristensen A. R. (2012). Dynamic production monitoring in pig herds I: Modeling and monitoring litter size at herd and sow level. Livestock Sci. 149, 289–300. doi: 10.1016/j.livsci.2012.07.023
Bono C., Cornou C., Lundbye-Christensen S., Ringgaard Kristensen A. (2013). Dynamic production monitoring in pig herds II. Modeling and monitoring farrowing rate at herd level. Livestock Sci. 155, 92–102. doi: 10.1016/j.livsci.2013.03.026
Bono C., Cornou C., Lundbye-Christensen S., Ringgaard Kristensen A. (2014). Dynamic production monitoring in pig herds III. Modeling and monitoring mortality rate at herd level. Livestock Sci. 168, 128–138. doi: 10.1016/j.livsci.2014.08.003
Brooker A. J., Skern-Mauritzen R., Bron J. E. (2018). Production, mortality, and infectivity of planktonic larval sea lice, Lepeophtheirus salmonis (Kroyer 1837): Current knowledge and implications for epidemiological modelling. ICES J. Mar. Sci. 75, 1214–1234. doi: 10.1093/icesjms/fsy015
Brown A. R., Lilley M., Shutler J., Lowe C., Artioli Y., Torres R., et al. (2020). Assessing risks and mitigating impacts of harmful algal blooms on mariculture and marine fisheries. Rev. Aquac. 12, 1663–1688. doi: 10.1111/raq.12403
Cheng J., Schloerke B., Karambelkar B., Xie Y. (2024). leaflet: Create Interactive Web Maps with the JavaScript ‘Leaflet’. R package version 2.2.2. Available online at: https://CRAN.R-project.org/package=leaflet. (Accessed July 25, 2024).
Costa C., Loy A., Cataudella S., Davis D., Scardi M. (2006). Extracting fish size using dual underwater cameras. Aquac. Eng. 35, 218–227. doi: 10.1016/j.aquaeng.2006.02.003
Department for Environment, Food & Rural Affairs. (2023). National statistics: Agriculture in the United Kingdom 2022. Chapter 13: Overseas trade. Available online at: https://www.gov.uk/government/statistics/agriculture-in-the-united-kingdom-2022/chapter-13-overseas-trade. (Accessed March 1, 2024).
Dethlefsen C. (2001). Space Time Problems and Applications. Aalborg, Denmark: Aalborg University. [PhD dissertation].
Dominiak K. N., Hindsborg J., Pedersen L. J., Kristensen A. R. (2019a). Spatial modeling of pigs’ drinking patterns as an alarm reducing method II. Application of a multivariate dynamic linear model. Comput. Electron. Agric. 161, 92–103. doi: 10.1016/j.compag.2018.10.037
Dominiak K. N., Pedersen L. J., Kristensen A. R. (2019b). Spatial modeling of pigs’ drinking patterns as an alarm reducing method I. Developing a multivariate dynamic linear model. Comput. Electron. Agric. 161, 79–91. doi: 10.1016/j.compag.2018.06.032
Elghafghuf A., Vanderstichel R., Hammell L., Stryhn H. (2020). Estimating sea lice infestation pressure on salmon farms: Comparing different methods using multivariate state-space models. Epidemics 31, 100394. doi: 10.1016/j.epidem.2020.100394
Elliott J. M., Elliott J. A. (2010). Temperature requirements of Atlantic salmon Salmo salar, brown trout Salmo trutta and Arctic charr Salvelinus alpinus: Predicting the effects of climate change. J. Fish Biol. 77, 1793–1817. doi: 10.1111/j.1095-8649.2010.02762.x
Fagerland M. W.(2012). t-tests, non-parametric tests, and large studies-a paradox of statistical practice? BMC Med. Res. Methodol. 12. doi: 10.1186/1471-2288-12-78
FAO. (2004). Cultured Aquatic Species Information Program - Salmo salar. Available online at: https://www.fao.org/fishery/en/culturedspecies/salmo_salar/en (Accessed February 7, 2023).
FAO. (2022). The State of World Fisheries and Aquaculture 2022. Towards Blue Transformation (Rome: FAO). doi: 10.4060/cc0461en
Firke S., Denney B., Haid C., Knight R., Grosser M., Zadra J. (2023). Package “janitor”. Available online at: https://github.com/sfirke/janitorhttps://sfirke.github.io/janitor/ (Accessed February 15, 2023).
Grolemund G., Wickham H. (2011). Dates and Times Made Easy with lubridate. J. Stat. Softw. 40. Available at: http://www.jstatsoft.org/.
Hijmans R. J., van Etten J., Sumner M., Cheng J., Baston D., Bevan A., et al. (2023). Package “raster”. Available online at: https://rspatial.org/raster (Accessed February 15, 2023).
Hilmarsen Ø., Holte E. A., Brendeløkken H., Høyli R., Hognes E. S. (2018). Konsekvensanalyse av landbasert oppdrett av laks – matfisk og post-smolt (in Norwegian: Consequenses of land-bases salmon farming). Rapport nr. OC2018 A-033, prosjekt nr. 302003741. Trondheim, Norway.
Huffman G. J., Stocker E. F., Bolvin D. T., Nelkin E. J., Tan J. (2019). GPM IMERG Late Precipitation L3 1 day 0.1 degree x 0.1 degree V06 (Goddard Earth Sciences Data and Information Services Center - GES DISC). NASA, Washington DC, USA. Available at: https://disc.gsfc.nasa.gov/datasets/GPM_3IMERGDL_06/summary.
Iversen A., Asche F., Hermansen Ø., Nystøyl R. (2020). Production cost and competitiveness in major salmon farming countries 2003–2018. Aquaculture 522, 735089. doi: 10.1016/j.aquaculture.2020.735089
Jensen D. B., Hogeveen H., De Vries A. (2016). Bayesian integration of sensor information and a multivariate dynamic linear model for prediction of dairy cow mastitis. J. Dairy Sci. 99, 7344–7361. doi: 10.3168/jds.2015-10060
Jensen D. B., Toft N., Kristensen A. R. (2017). A multivariate dynamic linear model for early warnings of diarrhea and pen fouling in slaughter pigs. Comput. Electron. Agric. 135, 51–62. doi: 10.1016/j.compag.2016.12.018
Kilburn R., Murray A. G., Hall M., Bruno D. W., Cockerill D., Raynard R. S. (2012). Analysis of a company’s production data to describe the epidemiology and persistence of pancreas disease in Atlantic salmon (Salmo salar L.) farms off Western Scotland. Aquaculture 368-369, 89–94. doi: 10.1016/j.aquaculture.2012.09.004
Kristensen A. R., Jørgensen E., Toft N. (2010). Herd Management Science II. Advanced topics (2010 Edition), pp. 75–144.
Larsen M. L. V., Pedersen L. J., Jensen D. B. (2019). Prediction of tail biting events in finisher pigs from automatically recorded sensor data. Animals 9, 458. doi: 10.3390/ani9070458
Moriarty M., Murray A. G., Berx B., Christie A. J., Munro L. A., Wallace I. S. (2020). Modelling temperature and fish biomass data to predict annual Scottish farmed salmon, Salmo salar L., losses: Development of an early warning tool. Prev. Vet. Med. 178, 104985. doi: 10.1016/j.prevetmed.2020.104985
Munro L. A. (2023). Scottish fish farm production survey 2022 (Edinburgh: Scottish Government). Available online at: https://www.gov.scot/publications/scottish-fish-farm-production-survey-2022/pages/5/ (Accessed February 5, 2024).
Murray A., Falconer L., Clarke D., Kennerley A. (2022). Climate change impacts on marine aquaculture relevant to the UK and Ireland. MCCIP Science Review 2022, 18. doi: 10.14465/2022.reu01.aqu
Murray A. G., Ives S. C., Smith R. J., Moriarty M. (2021). A preliminary assessment of indirect impacts on aquaculture species health and welfare in Scotland during COVID-19 lockdown. Vet. Anim. Sci. 11, 100167. doi: 10.1016/j.vas.2021.100167
Newman K. B. (1998). State-Space Modeling of Animal Movement and Mortality with Application to Salmon. Biometrics 54, 1290–1314. https://www.jstor.org/stable/2533659.
Noble C., Gismervik K., Iversen M. H., Kolarevic J., Nilsson J., Stien L. H., et al. (2018). Welfare Indicators for farmed Atlantic salmon: tools for assessing fish welfare. Available online at: www.nofima.no/fishwell/english (Accessed February 24, 2023).
Oldham T., Rodger H., Nowak B. F. (2016). Incidence and distribution of amoebic gill disease (AGD) - An epidemiological review. Aquaculture 457, 35–42. doi: 10.1016/j.aquaculture.2016.02.013
Oliveira V. H. S., Dean K. R., Qviller L., Kirkeby C., Bang Jensen B. (2021). Factors associated with baseline mortality in Norwegian Atlantic salmon farming. Sci. Rep. 11, 14702. doi: 10.1038/s41598-021-93874-6
Oppedal F., Juell J. E., Johansson D. (2007). Thermo- and photoregulatory swimming behavior of caged Atlantic salmon: Implications for photoperiod management and fish welfare. Aquaculture 265, 70–81. doi: 10.1016/j.aquaculture.2007.01.050
Pebesma E., Bivand R., Racine E., Sumner M., Cook I., Keitt T., et al. (2022). Package “sf.”. Available online at: https://r-spatial.github.io/sf/ (Accessed February 15, 2023).
Pebesma E., Bivand R., Rowlingson B., Gomez-Rubio V., Hijmans R., Sumner M., et al. (2018). Package “sp.”. Available online at: https://github.com/edzer/sp/https://edzer.github.io/sp/ (Accessed February 15, 2023).
Pierce D. (2023). Package “ncdf4.”. Available online at: https://cirrus.ucsd.edu/~pierce/ncdf/ (Accessed February 15, 2023).
Posit team. (2022). RStudio: Integrated Development Environment for R. RStudio, PBC, Boston, MA. http://www.rstudio.com/.
R Core Team. (2022). R: A language and environment for statistical computing. R Foundation for Statistical Computing, Vienna, Austria. https://www.R-project.org/.
Salama N. K. G., Murray A. G., Christie A. J., Wallace I. S. (2016). Using fish mortality data to assess reporting thresholds as a tool for detection of potential disease concerns in the Scottish farmed salmon industry. Aquaculture 450, 283–288. doi: 10.1016/j.aquaculture.2015.07.023
Scottish Salmon Producers Organization (2014). Scottish Salmon farming code of good practice. Growing a sustainable industry. Durn, Isla Road, Perth.
Skjølstrup N. K., Lastein D. B., de Knegt L. V., Kristensen A. R. (2022). Using state space models to monitor and estimate the effects of interventions on treatment risk and milk yield in dairy farms. J. Dairy Sci. 105, 5870–5892. doi: 10.3168/jds.2021-21408
Sommerset I., Walde C. S., Bang Jensen B., Wiik-Nielsen J., Bornø G., Oliveira V. H. S., et al. (2022). Fiskehelserapporten 2021. Veterinærinstituttet rapportserie nr 2a/2022. Available online at: www.vetinst.no (Accessed September 14, 2023).
Thakur K. K., Vanderstichel R., Barrell J., Stryhn H., Patanasatienkul T., Revie C. W. (2018). Comparison of remotely-sensed sea surface temperature and salinity products with in situ measurements from British Columbia, Canada. Front. Mar. Sci. 5. doi: 10.3389/fmars.2018.00121
Tonani M., Ascione I., Saulter A. (2022a). Product user manual (Ocean Physical and Biogeochemical reanalysis) NWSHELF_MULTIYEAR_BGC_004_011. EU Copernicus Marine Service. doi: 10.48670/moi-00058
Tonani M., Ascione I., Saulter A. (2022b). Product user manual (Ocean Physical and Biogeochemical reanalysis) NWSHELF_MULTIYEAR_PHY_004_009. EU Copernicus Marine Service. doi: 10.48670/moi-00059
Torrissen O., Olsen R. E., Toresen R., Hemre G. I., Tacon A. G. J., Asche F., et al. (2011). Atlantic salmon (Salmo salar): the “Super-chicken” of the sea? Rev. Fisheries Sci. 19, 257–278. doi: 10.1080/10641262.2011.597890
Tvete I. F., Aldrin M., Jensen B. B. (2022). Towards better survival: Modelling drivers for daily mortality in Norwegian Atlantic salmon farming. Prev. Vet. Med. 210, 105798. doi: 10.1016/j.prevetmed.2022.105798
Walde C. S., Bang Jensen B., Stormoen M., Asche F., Misund B., Pettersen J. M. (2023). The economic impact of decreased mortality and increased growth associated with preventing, replacing or improving current methods for delousing farmed Atlantic salmon in Norway. Prev. Vet. Med. 221, 106062. doi: 10.1016/j.prevetmed.2023.106062
Keywords: salmon, mortality, dynamic linear models, aquaculture, open-source data, environmental data, state-space models, warnings
Citation: Merca C, Boerlage AS, Kristensen AR and Jensen DB (2024) Monitoring monthly mortality of maricultured Atlantic salmon (Salmo salar L.) in Scotland I. Dynamic linear models at production cycle level. Front. Mar. Sci. 11:1436755. doi: 10.3389/fmars.2024.1436755
Received: 22 May 2024; Accepted: 01 August 2024;
Published: 03 September 2024.
Edited by:
Yngvar Olsen, NTNU, NorwayCopyright © 2024 Merca, Boerlage, Kristensen and Jensen. This is an open-access article distributed under the terms of the Creative Commons Attribution License (CC BY). The use, distribution or reproduction in other forums is permitted, provided the original author(s) and the copyright owner(s) are credited and that the original publication in this journal is cited, in accordance with accepted academic practice. No use, distribution or reproduction is permitted which does not comply with these terms.
*Correspondence: Carolina Merca, Y21nbUBzdW5kLmt1LmRr
Disclaimer: All claims expressed in this article are solely those of the authors and do not necessarily represent those of their affiliated organizations, or those of the publisher, the editors and the reviewers. Any product that may be evaluated in this article or claim that may be made by its manufacturer is not guaranteed or endorsed by the publisher.
Research integrity at Frontiers
Learn more about the work of our research integrity team to safeguard the quality of each article we publish.