- 1CEFE, Univ Montpellier, CNRS, EPHE-PSL University, IRD, Montpellier, France
- 2France Energies Marines, Plouzané, France
- 3Observatoire Pelagis, Unité d'Appui et de Recherche (UAR) 3462 Centre National de la Recherche Scientifique (CNRS) - La Rochelle Université (LRUniv), La Rochelle, France
Since the first plans to develop offshore wind farms (OWFs), concerns have been raised about the impacts on marine megafauna. Today, it is required to assess these impacts over the whole lifecycle of the OWF. Before construction, initial assessments are often conducted by visual surveys, but subsequent monitoring over the lifecycle of the OWF has to be digital due to safety requirements, leading to challenges in data comparability. The aim of this study was to attempt to establish generalizable intercalibration factors for this transition between visual and digital monitoring methods. To this end, intercalibration surveys were conducted at five different sites and at different times of the year within a site, using both visual monitoring at low-altitude and digital monitoring at both low and high altitudes. We tested the potential for intercalibration of the results based on the ratio of abundance estimated from data collected by the different methods. We explored factors such as the species under study and site-specific conditions that may influence intercalibration. We computed more than 100 intercalibration factors and found that, on average, abundance estimates from digital methods were higher than those from visual methods and that flight altitude for digital monitoring did not significantly influence abundance estimates. Aside from divergent abundance estimates depending on monitoring method, the findings also revealed significant heterogeneity, only one-third of which was explained by contextual factors such as taxonomy or the sea conditions. This outcome presents a pessimistic outlook on the prospect for the intercalibration of results between an initial assessment carried out with visual observations and subsequent monitoring with digital methods after OWF construction and until decommissioning. The high heterogeneity prevents seamless transferability of intercalibration factors and highlights the importance of local context.
1 Introduction
The exponential growth of the global population and the corresponding rise of energy consumption have significantly increased the demand for energy (Château, 2022). Despite the widespread recognition that fossil fuels substantially contribute to greenhouse gas emissions and cause global warming, they still remain the primary energy source globally (Olabi and Abdelkareem, 2022). Without strong measures to curb greenhouse gases assuming the continuation of policies implemented by the end of 2020, the International Panel on Climate Change (IPCC) warns of projected planetary warming of 3.2°C by the end of the century, posing severe ecological risks and threatening human societies (Lee et al., 2023). In response to this critical challenge, governments are adapting their energy policy to shift towards renewable energy resources. According to the European Environment Agency (EEA), 23% of energy consumed in the EU in 2022 was generated from renewable sources (EEA Report, 2023). To address climate imperatives, the EU has set an ambitious target to increase the proportion of its gross final energy consumption sourced from renewables to 32% by 2030 (EU Directive, 2018). Offshore wind energy is pivotal in achieving this goal: wind energy is an affordable technology for producing clean, renewable and abundantly available energy (European Commission, 2023). There has been interest in developing offshore windfarms (OWFs) since the early 2000s (Díaz and Guedes Soares, 2020), mainly due to onshore limitations and the possibility of building larger wind farms enabling greater energy production (Enevoldsen and Valentine, 2016). In Europe, most OWFs are located in the North Sea and generate power for the northern Europe. In stark contrast, OWF development in southern Europe is in its infancy, with few sites and currently little energy production compared to northern Europe. However, OWF development is accelerating: for example, France aims to build and operate 50 OWFs by 2050, with a capacity of 40 GW to provide nearly 20% of national electricity consumption (French Ministry of Ecological Transition, 2022).
However, from the beginning, OWF development has raised concerns regarding its impacts on wildlife, and particularly on marine megafauna (large fish, turtles, seabirds and marine mammals) (Garthe and Hüppop, 2004; Madsen et al., 2006; Bailey et al., 2014; Croll et al., 2022). Seabirds and marine mammals in particular have been the focus of impact studies out of concerns of additional mortality, injuries, displacements, or loss of habitats during the construction phase or operation of OWFs. During the construction phase, pile driving could potentially cause hearing damage, masking of calls or spatial displacement of marine mammals to avoid the loud sounds emitted (Madsen et al., 2006; Bailey et al., 2014). Noise generated by ship traffic as a consequence of OWF construction can also cause displacement and disturbances (Wisniewska et al., 2018; Frankish et al., 2023). Once construction is completed, however, there is no consensus on whether marine mammals are directly impacted by OWFs (Teilmann and Carstensen, 2012; Dähne et al., 2014). During the operational phase, potential effects could be either negative (e.g. acoustic disturbance) or positive (e.g. fishing exclusion) depending on biological conditions as well as prevailing management goals (Bergström et al., 2014). For seabirds, different behavioural reactions could occur, ranging from complete avoidance to attraction (Furness et al., 2013; Dierschke et al., 2016). When attracted to OWFs, seabirds are at risk of mortality by collision with the blades (Lane et al., 2020). When avoiding OWFs (Peschko et al., 2020; Garthe et al., 2023), birds may experience functional habitat loss due to displacement, which could in turn result in increased energy costs for individuals if the alternative foraging habitat is of poorer quality or if the individuals have to travel longer distances to reach it (Masden et al., 2010). These risks can have consequences on seabird viability in addition to the environmental factors driving population dynamics (Horswill et al., 2022). Because of these potential consequences, assessing the impacts of OWFs on seabirds and marine mammals, many of which are protected, endangered or threatened species, is required by law in the EU (EU Habitats Directive, 1992; European Union, 2009). Reliable impact studies at each step of an OWF lifecycle – from commissioning through construction and operation to decommissioning – are needed, with special attention given to establishing accurate baselines against which to assess changes in the distribution and abundance of seabirds and marine mammals.
The monitoring of marine megafauna for impact studies typically relies on surveying using either ships or aircraft (Camphuysen et al., 2004; Hammond et al., 2021). In this study, we focused on aerial surveys, which are more commonly used for impact studies as they are quick to cover large area and less expensive than shipboard surveys (Certain and Bretagnolle, 2008; Buckland et al., 2012). These surveys are typically based on visual observations conducted at altitudes lower than 200 m, a limit crucial for detecting and identifying species as small as harbour porpoises (Phocoena phocoena) or seabirds (Certain and Bretagnolle, 2008). However, during the construction and operation phases of OWFs, aerial monitoring must adhere to safety regulations, requiring a minimum flyover altitude of 300 m. Because onboard observers cannot identify species at such high altitudes, digital data acquisition methods need to be employed. Digital methods for monitoring marine megafauna from an aircraft involve equipping the aircraft with cameras to film/photograph a strip underneath along pre-defined transects. Footage is then analysed to identify the animals that were present in the sampled area. High-resolution image acquisition allows for reliable species identification even at altitudes higher than 300 m (Žydelis et al., 2019), allowing effective surveillance of marine megafauna during OWF construction and operation. This has made digital census and monitoring by aircraft essential for most offshore renewable energy projects in northern Europe (Buckland et al., 2012; Garthe et al., 2023). Consequently, two different types of monitoring methods, observer-based (called ‘visual’ thereafter) and digital, are often used to monitor marine megafauna throughout the phases of the OWF. Ensuring consistency and comparability of results from these two monitoring methods is crucial to guarantee the reliability and accuracy of the data collected and enable a comprehensive assessment of the impact of OWF activities on marine megafauna.
To date, only a few studies have formally compared the results of visual and digital aerial monitoring surveys (e.g. Buckland et al., 2012 in Wales; Taylor et al., 2014 in Massachusetts; Williamson et al., 2016 in Scotland; Žydelis et al., 2019 in western Baltic Sea; Garcia-Garin et al., 2020 on the Spanish Mediterranean coast). The outcomes of these studies present contrasting results. For instance, Žydelis et al. (2019) found that the number of sightings of small and inconspicuous birds such as grebes, mergansers and auks were more than ten times more numerous in a digital survey dataset compared to a visual dataset. Buckland et al. (2012) also found increased sightings of black scoters with digital methods. Conversely, Garcia-Garin et al. (2020) concluded that observer-based methods were more effective for seabird detection during simultaneous flights, but equalled digital methods for marine mammal detection. On harbour porpoises, Williamson et al. (2016) found that digital surveys provided similarly measures of relative abundance to those obtained by visual line-transect surveys. Taylor et al. (2014) reported that digital method yielded significantly higher mean density estimates for loggerhead turtles, ocean sunfish, and blue sharks but no significant differences for leatherback turtles or basking sharks. The modalities of these studies make it possible to only partially answer questions raised when comparing one technique to another. For instance, each of the studies was carried out in a specific area, limiting the external validity of the results to other regions where characteristics of the environment and fauna are different. Moreover, these studies only produced estimates associated with the different methods, but did not formally investigate intercalibration estimates between methods.
In this study, we aimed to establish general intercalibration factors that allow the transition between aerial visual and digital monitoring methods. To this end, we carried out 14 surveys conducted across five distinct locations, using both visual monitoring at low altitude and digital monitoring at both low and high altitudes, encompassing various seasons, and focused on 15 groups of species, including marine birds, cetaceans, sharks, large fish and jellyfish. Intercalibration was tested based on the ratio of abundance estimated from data collected by the different methods. Buckland et al. (2012) emphasized how crucial it is to take into account that these factors are likely to vary across species, locations and seasons. We thus explored, by means of linear models, whether the species under study or site-specific conditions influenced intercalibration factors.
2 Materials and methods
2.1 Intercalibration surveys
The intercalibration surveys were conducted at five different sites around planned OWF projects in northwestern France: Courseulles-sur-Mer, Dieppe Le Tréport, Fécamp, Centre Manche and Bretagne Sud (Figure 1). One intercalibration survey per season was conducted at the Centre Manche, Bretagne Sud and Dieppe Le Tréport sites, and a single intercalibration survey for Fécamp and Courseulles-sur-Mer sites (Table 1).
For all intercalibration surveys, transects were positioned throughout the study area, perpendicular to the coast, covering an area of between 7% and 11% of the total study area. Two planes followed these transects at different altitudes. One plane flew at a speed of between 170 and 180 km/h at an altitude of approximately 600 ft above sea level, an altitude at which observers on board could easily detect and identify the species encountered (Certain and Bretagnolle, 2008). The same plane was also equipped with a high-resolution camera photographing a strip under the plane. A second aircraft, flying at a speed of about 180 km/h at an altitude of approximately 1150 ft or 220 km/h at an altitude 1800 ft, depending on the system used (Table 1), flew over the site within a maximum of 15 min before or after the low-altitude flight. This aircraft was only equipped with high-resolution cameras capturing a strip under the aircraft.
2.2 Visual methods
In low-altitude flights, two observers were positioned on either side of the aircraft, which was equipped with bubble windows to allow for detection under the aircraft. Observers recorded information on marine megafauna (marine mammals, birds, sharks, large fish, turtles) and the sampling conditions encountered (see Lambert et al., 2019 for details). In particular, the sea state according to the Beaufort scale was recorded for all transects, from which an average sea state over the entire flight was calculated (Table 1). At regular intervals, the navigator (who recorded information) and the observers rotated to limit eye fatigue and increase detection capacity. For seabirds, sightings were recorded within a 200-m-wide strip following a standardized strip-transect protocol, assuming that all seabirds would be detected within the strip. For cetaceans, sharks, turtles and large fish, there was no observation distance limit, but observers determined the distance of each detected individual or group by measuring the angle of detection from the aircraft wings with an inclinometer (see Lambert et al., 2019). Species identification was carried out to the lowest taxonomic level possible, but some species could not be identified to species level and were grouped on the basis of common morphological criteria.
2.3 Digital methods
Digital methods were used at both high and low altitudes. High-resolution photos were taken (with a spatial resolution of at least 2 cm per pixel), regardless of altitude, and covered a 400–500-m strip under the aircraft (see Supplementary Table 1 for the technical characteristics of the different methods used). All images were then analysed following two steps: (1) detecting an object of interest in the picture, (2) identifying the detected objects. Object detection was done either automatically using automated object-detection models or by humans, depending on the systems used (Supplementary Table 1). Naturalists then carried out object identification. If unambiguous species identification was not possible, identification was limited to family or groups of morphologically similar species.
2.4 Abundance estimates
We grouped the sightings of the different surveys into 15 species groups (alcids, gannets, larids, cormorants, grebes, divers, storm petrels, shearwaters, fulmars, skuas, cetaceans, sharks, large fish, sunfish and jellyfish). The species composition of each group is presented in Supplementary Table 2. All other species not included in these different groups were excluded from this study. For each of these species groups, when sufficient data was available, we estimated abundance over the entire study area for each type of aerial monitoring method (visual, low-altitude digital, high-altitude digital) and for each intercalibration survey.
We fitted generalized additive models (GAM) using the ‘dsm’ package (Miller et al., 2022) in R version 4.2.1 (R Core Team, 2022) to estimate the number of individuals at the study site scale, with geographic coordinates as covariates. We divided the aerial transects into segments of approximately 4 km and associated each observation with the nearest segment. For digital data and visual bird sightings, we assumed that all individuals were detected on the monitored strip: we did not correct for availability or perception bias. Availability bias occurs when individuals are present but cannot be detected (e.g. diving animals). Perception bias occurs when individuals are visible but are missed by observers or automated detection algorithms (e.g. sun glare affecting visibility).We determined the size of the monitored strip depending on the system used (Supplementary Table 1). For visual data on marine mammals, sharks and large fish, we assumed that detection probability decreased with perpendicular distance from the transect line, usually in a non-linear way (Buckland et al., 2001). In contrast to strip transect methods, which assume constant detection in the strip followed by the aircraft, line transect methods assume that all individuals directly on the transect line are detected, and allow for individuals off the line to be missed. To account for distance detection heterogeneity, we applied a distance-sampling analysis using the ‘Distance’ package (Miller et al., 2019) in R to estimate the species-specific effective strip width using a half-normal detection function. We obtained the following GAM:
Where is the expected number of observations per segment j, is the effective area monitored, is an intercept, the latitude and longitude for each segment are included as smooth terms (using the default thin plate splines). The distribution of was modelled as a Tweedie distribution. Using this GAM, we predicted distribution over the whole study site using a hexagonal prediction grid of 3.46 km² (i.e. 2 km between opposite edges of the hexagonal cells).
2.5 Intercalibration factors
We estimated intercalibration factors as the ratio, on a log scale, between the abundance estimated using a first data source and the abundance estimated using a second data source. We estimated two types of intercalibration factors: visual/digital or digital low-altitude/digital high-altitude. For the visual/digital intercalibration factors, we estimated both the visual/low-altitude digital and the visual/high-altitude digital intercalibration factors. To quantify variability associated with intercalibration factors, we performed leave-one-out analyses (Supplementary 3). At each iteration, we randomly removed a part of the effort and observation corresponding to at least 10% of the survey area. We then estimated the abundance using the reduced datasets for each monitoring method with the GAM described above to obtain intercalibration factors. We computed 200 iterations to estimate the uncertainty of the intercalibration factors.
2.6 Variables influencing the intercalibration factors
We used linear models to investigate the factors driving the variability in estimated intercalibration factors. We considered the uncertainty associated with estimated intercalibration factors with a weighted likelihood approach: weights were computed so that precise intercalibration factors were given more weight than imprecise ones. First, we tested the variability associated with the group of species studied. Since species are more or less visible depending on their colour, size or behaviour, it seems likely that intercalibration factors would depend on the species group studied. We also considered the site effect and tested the digital data acquisition process as different digital processes were used for the different intercalibration surveys (Table 1) and may therefore be a source of variability in the intercalibration factors. We estimated intercalibration factors between visual and high-altitude digital datasets and visual and low-altitude digital datasets, and we also examined whether the altitude of the digital data acquisition flight explained some of the variability in the intercalibration factors. This variable was not tested in the low-altitude digital/high-altitude digital intercalibration factors as there was no change in altitude. Finally, as sea state is known to affect detection in visual surveys (Teilmann, 2003; Pollock et al., 2006), we also included this variable in our models as a linear covariate ranging from 0 to 4 on the Beaufort scale. This sea state variable was calculated and averaged over the entire visual survey for each campaign (Table 1). Only visual methods collected data on the sampling conditions encountered during all surveys, as this information was recorded as part of the protocol, which was not the case for digital methods. We also tested an interaction between group and sea state, assuming that the detection of some species groups might deteriorate when the conditions worsen. Using the AIC criterion corrected for small sample size (Anderson and Burnham, 2004) and the ‘AICcmodavg’ R package (Mazerolle, 2020), we assessed which variables accounted for the variability observed in the intercalibration factors. We included all our variables of interest in a general model and then removed step by step the variables explaining the least variability based on the AICc. If two models were less than two AICc points apart, we selected the most parsimonious one.
3 Results
3.1 Observation and abundance estimates
We analysed datasets from the 14 surveys and estimated 116 intercalibration factors to compare the different survey methods (visual, digital low-altitude, digital high-altitude) for different species groups (Supplementary Table 4). Cormorants, grebes, shearwaters, fulmars, and skuas were not included in the analysis due to insufficient data. The results revealed highly variable situations of intercalibration which we illustrated in two examples in the following. In one example, larids had nearly identical abundance estimates (Figure 2); in another, on alcids, there was pronounced divergence between estimates obtained through different data acquisition methods (Figure 3).
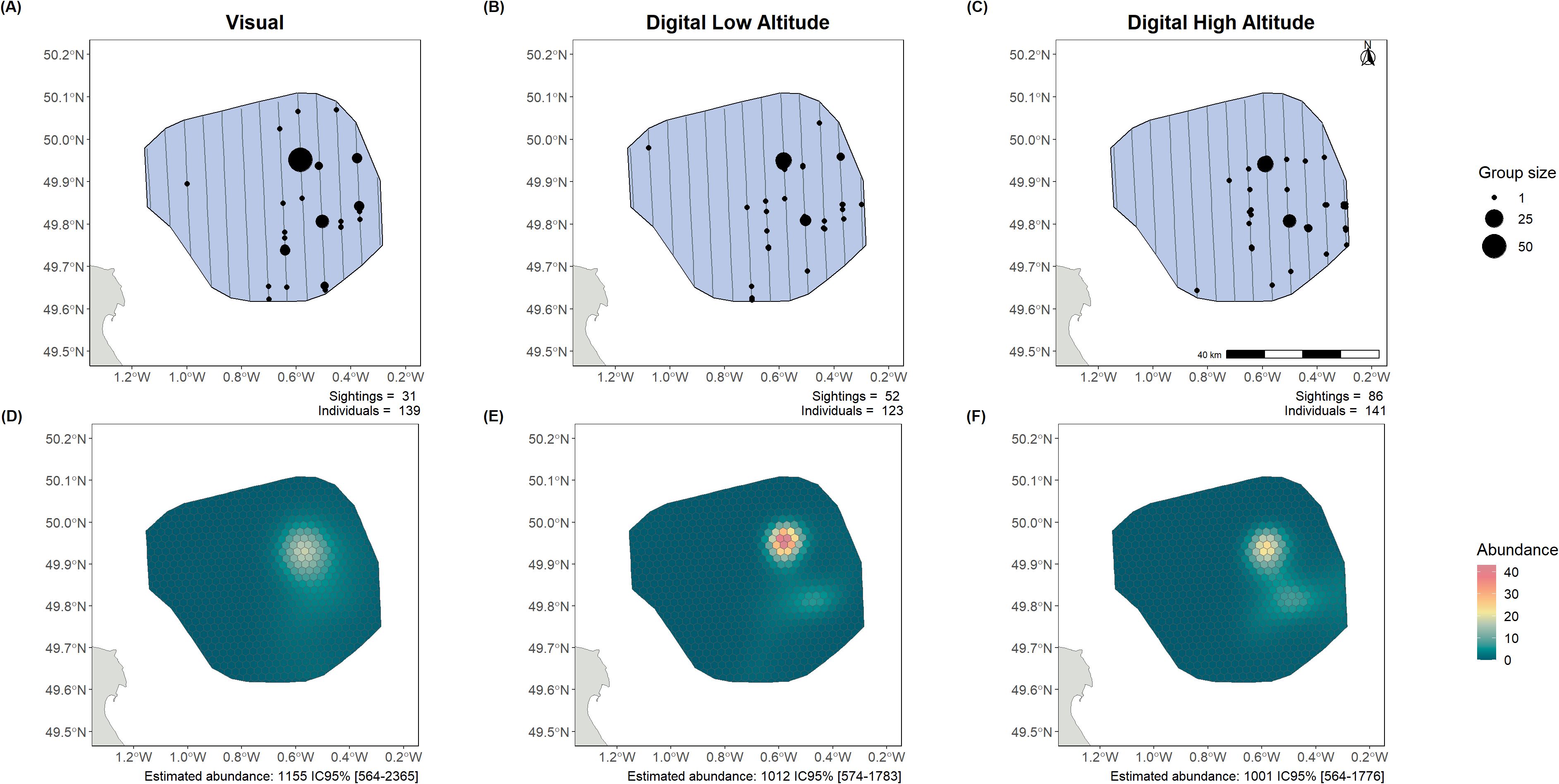
Figure 2. Larid observations (A–C) and abundance estimates (D–F) from the surveys and intercalibration analysis in Centre Manche (13/06/2022). The lines represent the flown transects, while the dots show the observations of both individual birds and groups. Each observation plot shows the number of sightings and the total number of birds detected. The estimated abundance over the whole area, with the 95% confidence intervals, is also shown below the density surface model (DSM) plots. Left panels present visual monitoring, centre panels low-altitude digital monitoring, and right panels high-altitude digital monitoring.
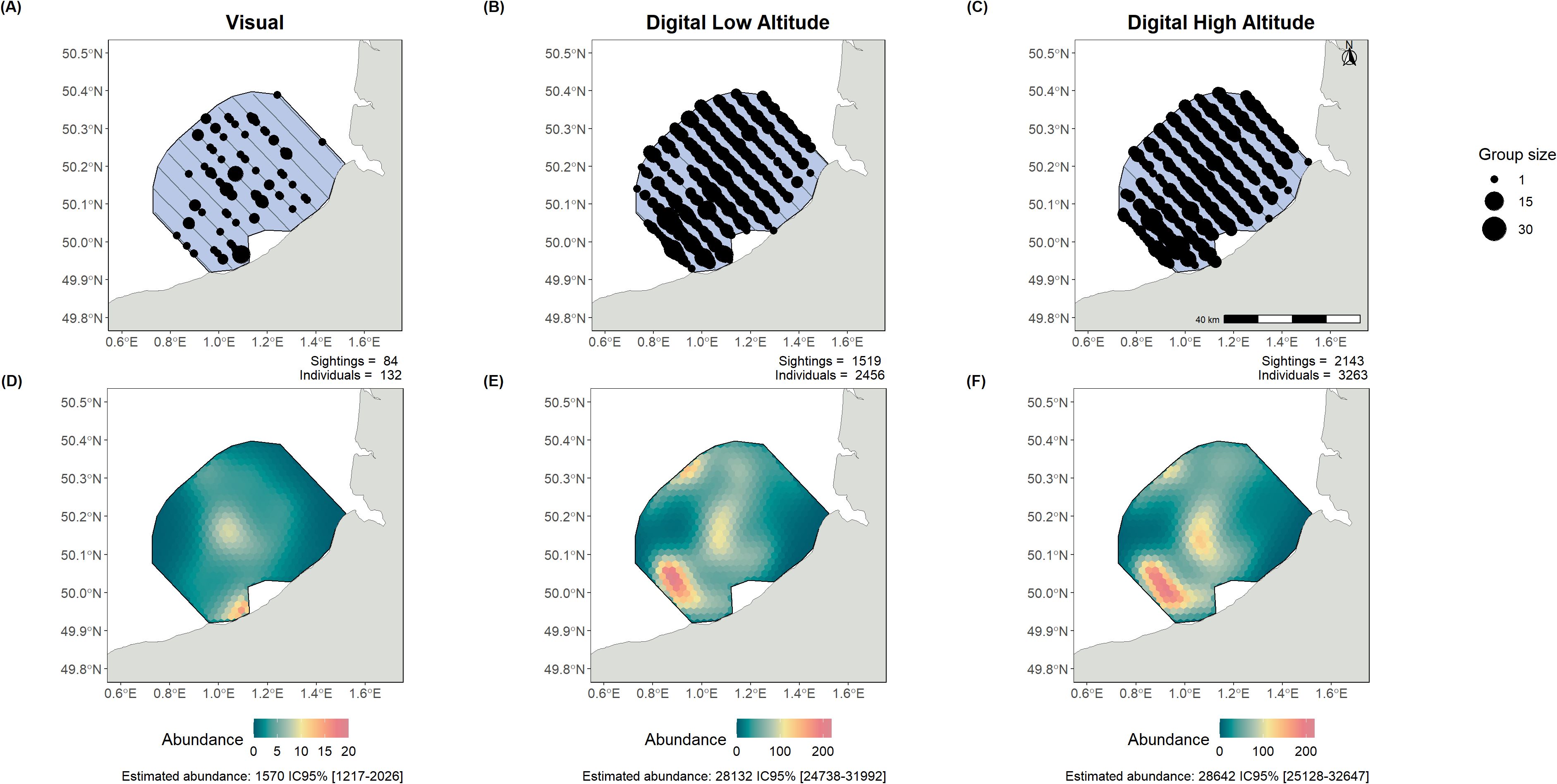
Figure 3. Alcid observations (A–C) and abundance estimates (D–F) from the surveys and intercalibration analysis of Dieppe Le Tréport (27/02/2022). The lines represent the flown transects, while the dots show the observations of both individual birds and groups. Each observation plot shows the number of sightings and the total number of birds detected. The estimated abundance over the whole area, with the 95% confidence intervals, is also shown below the density surface model (DSM) plots. Left panels present visual monitoring, centre panels low-altitude digital monitoring, and right panels high-altitude digital monitoring.
Three different datasets of larid observations obtained from three different aerial monitoring methods were used separately to fit GAMs and estimate larid abundance and distribution in the Centre Manche area (Figure 2). The three methods of data acquisition yielded abundance estimates with overlapping confidence intervals (1,155 individuals estimated CI 95% [564-2,365] with visual data; 1,012 individuals estimated CI 95% [574-1,783] with digital low-altitude data and 1,001 individuals estimated CI 95% [564-1,776] with digital high-altitude data). The projected distributions showed strong similarity, indicating a ‘hotspot’ in the northeast of the study area.
In contrast, for alcids in the English Channel, there was high variation in abundance estimations depending on the data acquisition method (Figure 3). Visual detections were much lower (Figure 3A), with 132 individuals detected, while the digital method at the same altitude detected 2,456 individuals (Figure 3B). As a result, abundance estimates between visual and digital data differed by a factor of 18, with no overlap in confidence intervals (1,570 individuals estimated CI 95% [1,217-2,026] with visual data; 28,132 individuals estimated CI 95% [24,738-31,992] with digital low-altitude data and 28,642 individuals estimated CI 95% [25,128 – 32,647] for digital high-altitude survey). However, the estimates obtained from the two digital methods in the intercalibration survey showed consistent abundance estimates and similar patterns of distribution.
3.2 Comparison between visual and digital data
Our results showed high variability in intercalibration factors (Figure 4). The average intercalibration factor (on a log scale) between visual and digital methods was -0.372 (CI 95% [-1.611–0.507]), which indicated that, on average, digital data acquisition produced higher abundance estimates than visual data acquisition.
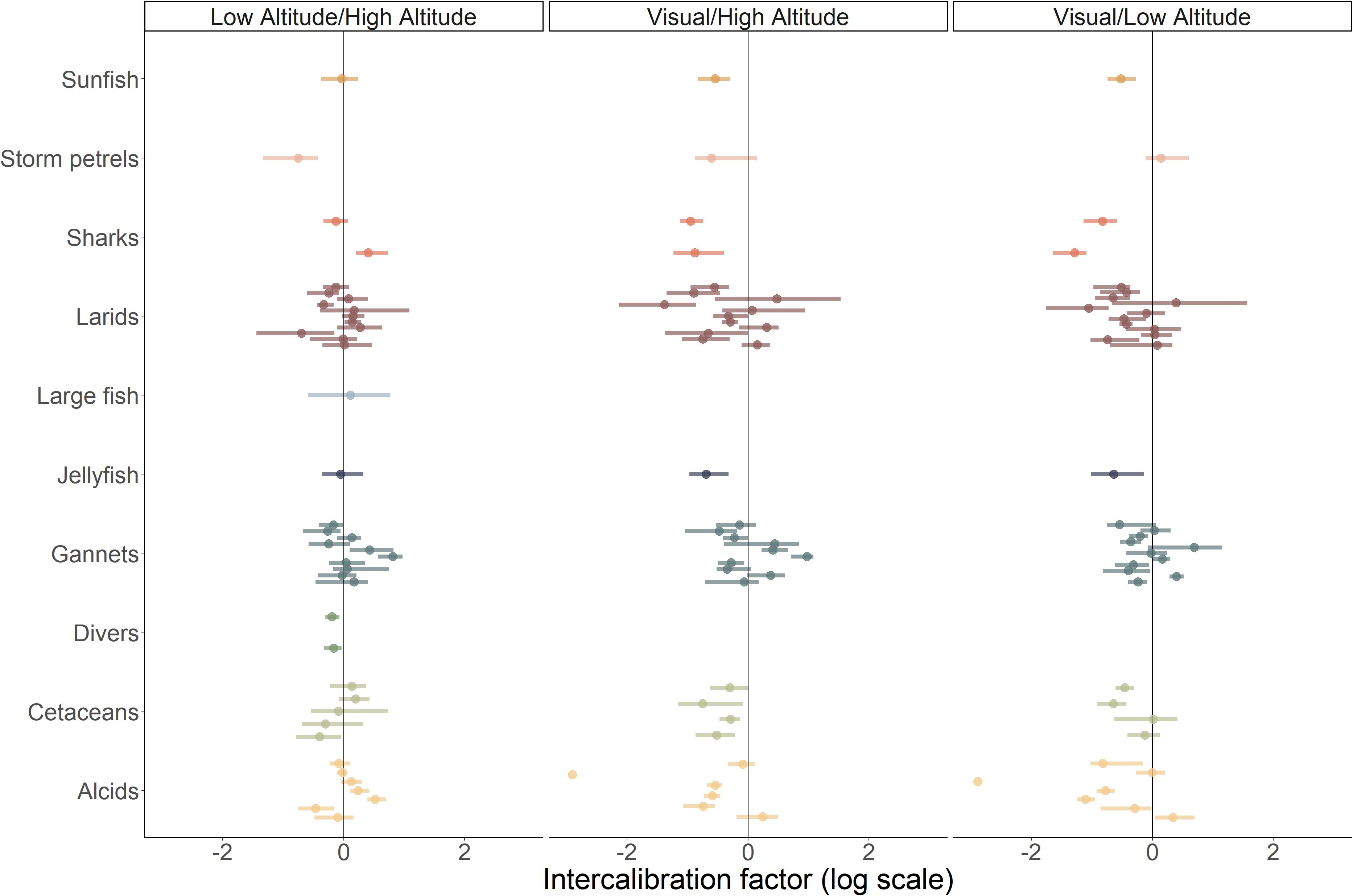
Figure 4. Intercalibration factors by species group (some species groups were not analysed because there was not enough data). The intercalibration factor is highlighted by a dot and the bar shows the 95% confidence interval. Colours represent the different species groups. Left panel corresponds to the intercalibration factor of digital methods at low and high-altitude. Centre panel corresponds to the intercalibration between the visual method and high-altitude digital method. Right panel corresponds to the intercalibration between the visual method and the low-altitude digital method.
Model selection indicated that taxonomic group and sea state were the variables that most accounted for the variance in intercalibration factors (Supplementary Table 5). However, the cumulative percentage of variability did not exceed 35%: a large proportion of the variability in intercalibration factors thus remained unaccounted for. It should also be noted that a model with site, sea state and taxonomic group covariates was within one AIC point from the selected model and explained 43% of the variability (Supplementary 5). Altitude and digital method covariates were not selected in the models with the smallest AICc nor was the interaction between species group and sea state. For this comparison between visual and digital methods, we focused on the model including a species group effect and a sea state effect.
There was high between-species group heterogeneity of intercalibration factors (Figure 5). Two species groups, large fish and divers, were not included in the model selection due to insufficient data from visual surveys. Intercalibration factors of species rarely observed during the surveys were associated with a wide confidence interval. Despite the limited intercalibration factors estimated for groups of species such as sharks, jellyfish and sunfish, the abundance estimated using visual data acquisition methods was, on average, lower than that estimated using digital methods (Figure 5). For alcids, gannets, larids and cetaceans, the taxa with the largest number of intercalibration factors estimated, the model predicted a lower intercalibration factor for alcids and a higher intercalibration factor for gannets. On average, the observed intercalibration factor for alcids was -0.78 (95% CI [-2.90–0.31]) on 13 intercalibration factors estimated, and gannets had an average intercalibration factor of 0.01 (95% CI [-0.51–0.83]) on 21 intercalibration factors estimated. Thus, for gannets, visual and digital methods produced on average relatively similar estimates, whereas for alcids the digital methods estimated on average an abundance 2.2 times higher than visual methods. For cetaceans and larids, the estimated abundance was on average higher with the digital methods compared to the visual method, with an average intercalibration factor of -0.38 (95% CI [-0.73 – -0.01]) over 8 intercalibration factors estimated for cetaceans, and an average intercalibration factor of -0.34 (95% CI [-1.20–0.43]) over 23 intercalibration factors estimated for larids.
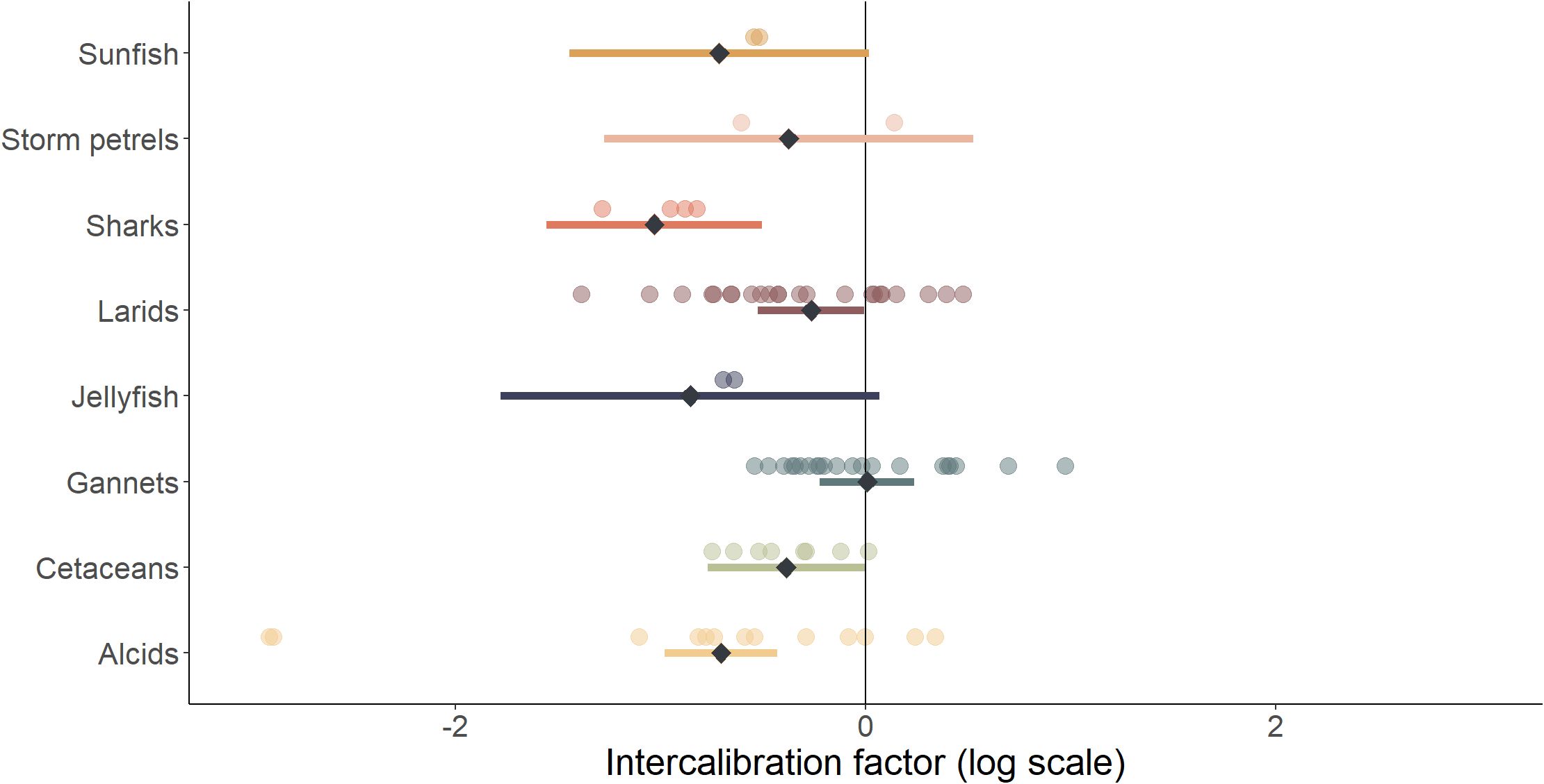
Figure 5. Estimated and model predictions of intercalibration factors by species group (some species groups were not modelled because there was not enough data). Coloured dots are the intercalibration factors estimated between visual and digital methods for the different species groups and for the different sites. Black diamonds are the intercalibration factor predicted by the selected model according to the species group, considering a sea state of 2 on the Beaufort scale. The coloured bars show the 95% confidence interval of the prediction.
The selected model also included a significant sea state effect of -0.19 (CI 95% [-0.33 – -0.05]), indicating that, on average, the intercalibration factor decreased when the sea conditions became rough (Figure 6). This means that digital methods tended to detect more individuals, and thus estimated a higher abundance, than visual methods when sea conditions were rough.
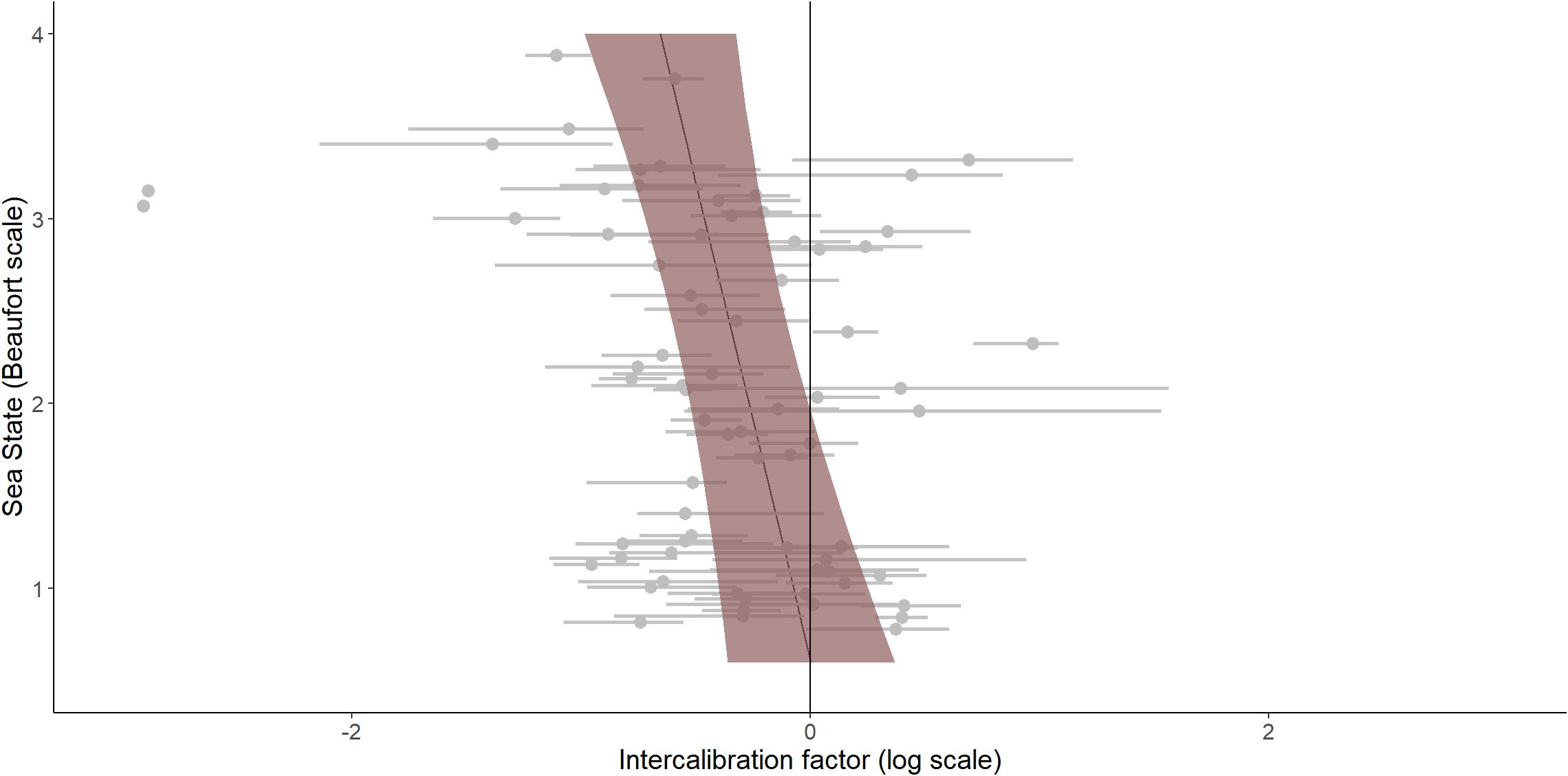
Figure 6. Model predictions of intercalibration factors according to sea state. Coloured area (shading shows the 95% CI) is the intercalibration factor predicted by the selected model according to the sea state from 0 to 4 on the Beaufort scale, fixing the group as larids. Grey dots and lines are the estimated intercalibration factors and the 95% confidence interval associated for each species group during each intercalibration analysis.
3.3 Comparison between low-altitude digital data and high-altitude digital data
The average intercalibration factor between the digital low-altitude dataset and the high-altitude datasets for each survey and species group was 0.018 (95% CI [-0.699–0.516]), indicating that digital data acquisition at low and high altitudes provided, on average, similar abundance estimates (Figure 4). Model selection indicated that the model without any covariates was within one AIC point of the model with the smallest AICc (Supplementary Table 5) and was selected. The model with the smallest AICc indicated that sea state and method covariates explained 18% of the observed variability.
4 Discussion
As the true number of individuals in the different areas studied was unknown, we only compared the abundance estimates obtained by the different survey methods and assessed their agreement or incongruence. The results highlighted that, on average, the analysis of data from digital methods led to higher abundance estimates than from visual methods. Intercalibration factors between visual and digital methods also varied greatly according to sea state and the species studied. On the other hand, abundance estimates between digital methods at high and low altitudes were more consistent on average, although the observed variability was poorly explained by the covariates tested.
4.1 Variables affecting visual–digital data intercalibration
On average, the abundance estimated through digital methods at both high or low altitudes was higher than those obtained through visual methods. This result is consistent with other studies comparing visual and digital methods which also found higher density estimates using digital methods on seabirds (Buckland et al., 2012; Žydelis et al., 2019) or on blue sharks and sunfish (Taylor et al., 2014). These results underline the need to take precautions when switching from one method to another. A key aspect of our study was the establishment of intercalibration factors for long-term monitoring of marine megafauna using these different approaches. However, intercalibration factors estimates revealed high heterogeneity, of which only one-third was explained by contextual factors such as species group or sea state, rendering intercalibration a complex task.
4.1.1 Species groups
4.1.1.1 Gannets
In our analyses, gannets had an intercalibration factor close to zero (on a log scale), which was expected due to their highly visible colour and size. However, variability around the mean showed that in some cases, the visual method recorded more individuals than the digital method, or the opposite (lower detection by the visual method compared to the digital method). Gannets and larids form large groups of individuals when they encounter favourable feeding conditions or fishing boats (Hudson and Furness, 1989; Camphuysen and Webb, 1999). As a result, the estimated density maps were often strongly influenced by these larger groups of individuals, that act as ‘hotspots’ of individuals throughout the area. If observers mistakenly record individuals that were beyond the 200-m-wide strip or if they overestimate the number of individuals in large groups (Frederick et al., 2003), this could result in overestimated abundance using the visual method. In digital methods, when a large group is present on several overlapping images, it can be difficult to avoid double or triple counting of the same individuals, which could lead to overestimating the number of individuals in these hotspots (Brack et al., 2018). Trained human operators in charge of identifying the targets detected by automated detection algorithms (as in our study) should be able to identify such duplicates, but some errors are likely to occur. Conversely, if individuals are mistaken for duplicates even though they are different individuals, this can lead to underestimating the number of individuals in the hotspot. Therefore, for larids and gannets, a large part of the heterogeneity observed in the intercalibration factors may be due to the precision of the counts of large groups depending on the method.
4.1.1.2 Alcids, divers, grebes
Alcids, divers, and grebes are considered inconspicuous due to their small size, dark plumage, and the fact that they are often seen resting on the water. Among these species, the alcids were the only ones that were sufficiently detected by the different methods to be included in our analyses. The abundance estimate for alcids was, on average, twice as low using the visual method compared to digital methods, with a high degree of variability. There were extreme cases of difference in abundance estimates between the two methods for this inconspicuous species. For example, in one intercalibration survey the number of alcids estimated was 18 times lower using the visual method compared to the two digital methods. In contrast, at the same site one year later, digital and visual methods produced similar estimates for alcids. In another intercalibration survey, several hundred alcids were detected using the digital methods but none using the visual method. Similarly, divers were sometimes observed in large numbers using digital methods, but considerably less using the visual method. This lack of detection prevented us from including this group in our analyses. Žydelis et al. (2019) also reported notable discrepancies between visual and digital methods for these species. They found that abundance estimates were about five times lower using visual methods compared to digital methods for alcids, and 15% lower for divers. Such differences might be explained by identification errors in visual methods. For example, divers might have been detected visually, but identified as alcids. However, even when summing all these inconspicuous species, our results showed fewer visual detections compared to those made using digital methods. It is therefore quite likely that individuals are under-detected by visual methods. For instance, it is known that human eye-based object detection at sea relies heavily on movement or colour contrasts with the ocean (Tasker et al., 1984), while divers and alcids are most often resting on the surface and have dark plumage that limits their detection. It is also possible that the 200 m detection limit set for seabirds on either side of the aircraft in the visual method might exceed the observer’s actual detection capability, leading to an underestimation of abundance within the study area. In a study about detection bias in strip-transect aerial methods, Certain and Bretagnolle (2008) suggested that a maximum observation distance of 150 m should prevent detection-distance bias. Barbraud and Thiebot (2009) also found that when the strip width monitored exceeded 100 m, as in our study, the probability of the visual detection of the smallest species dropped considerably compared with larger species. As a consequence, these inconspicuous species might be more easily missed by observers, resulting in bias and abundance underestimation compared to digital methods.
4.1.1.3 Larids
Larids, which are easier to detect than alcids, and generally slightly less easy to detect than gannets, as they are smaller and with more diverse plumage, had an intercalibration factor intermediate to these two species groups, a finding that is consistent given these detection characteristics. Our results indicated that, on average, estimated abundance of larids was 1.4 times higher with digital methods than visual methods. However, it is important to note the wide variability between surveys.
4.1.1.4 Storm petrels
Only one survey allowed for intercalibration of storm petrels. Contrasting abundance estimates were observed between the two digital methods: the estimate was nearly twice as high using the high-altitude digital method compared to the low-altitude digital method. Consequently, visual/digital intercalibration factors were contrasted, with a near-zero intercalibration at low altitudes and overestimation by the digital method at higher altitudes. In another survey, only the visual method recorded approximately 50 individuals, while both digital methods failed to detect any. Storm petrels are the smallest seabird species group detected in the survey areas. They are visually detectable due to their frequent movement and their conspicuous white rump. Conversely, digital methods are likely to overlook these individuals or to misinterpret a motionless white rump in images as a reflection of sunlight.
4.1.1.5 Cetaceans
For small cetaceans, abundance estimates were higher with digital than with visual methods. This was surprising as this is a group of species for which higher estimates might be expected with visual methods compared to digital ones. Indeed, observers are able to monitor larger areas than cameras and they are able to incorporate detection cues for sightings that typically cannot be seen in imagery (Koski et al., 2013). For example, cetaceans below the surface may be masked when photographed or confused with waves during digital processing, whereas signs of presence (diving, spouting/blowing, etc.) are more likely to be detected visually. Consequently, visual methods should estimate higher abundance than digital methods. However, in their comparison between digital and visual methods, Garcia-Garin et al. (2020) found that both methods were equally effective for detecting cetaceans and Williamson et al. (2016) suggested that digital survey can provide similarly robust measures of relative abundance to those obtained by visual surveys. In contrast, our results showed variability in the intercalibration factors, with overall higher abundance estimates from digital data at the two sites where there were enough sightings for modelling. These outcomes could be the result of more accurate digital counting of group sizes for cetaceans. Or perhaps small inconspicuous species such as porpoises that are difficult to detect visually are better detected by automated detection algorithms. In particular, these species might be overlooked or underreported when there are hotspots of other target species. For example, Lambert et al. (2019) found that flying seabirds had a negative effect on small cetacean perception. However, one clear limitation of the generalization of our intercalibration results on cetaceans was the need to pool all species of cetaceans to obtain a large enough sample size for analyses. Species with potentially differing detection probability (e.g. common dolphins, bottlenose dolphins and harbour porpoises) due to their behaviour or group size (Barlow et al., 2001; Authier et al., 2018; Gilles et al., 2023) were lumped into one taxonomic group. Given the high taxonomic heterogeneity, it is unclear whether the resulting intercalibration factor can be applied to each of the cetacean species. Another contextual factor limiting the external validity of the cetacean intercalibration factor is the overall low density of harbour porpoises in Atlantic waters compared to the North Sea (Gilles et al., 2023). Our results rely on only two sites (Sud Bretagne with three intercalibration surveys and Dieppe Le Tréport with one intercalibration survey) where there were enough sightings for modelling. All else being equal, a lower density would result in less detections and increased estimation noise compared to a higher density area. The effect of density on intercalibration factors remained to be investigated. In the meantime, it cannot be assumed that one intercalibration factor estimated in one site can be accurately used in other sites, and this was supported by our results.
4.1.1.6 Sharks, sunfish, jellyfish
Regarding sharks, jellyfish and sunfish, although the number of intercalibration factors estimated was too limited to allow a precise understanding of the differences in estimates between methods, our results showed that digital methods consistently yielded higher average abundance estimates than visual observations. In a similar way, Taylor et al. (2014) found that smaller sharks, such as blue sharks, showed significantly higher abundance estimates with digital methods compared to visual methods, whereas the estimates for larger species, such as basking sharks, were similar between the two methods.This tendency might be explained by the inherent complexity of detecting small submerged individuals for humans, especially considering the constrained detection time afforded by a moving aircraft. Moreover, these species might be overlooked or underreported when there are hotspots of other target species. In contrast, images captured by digital methods using automated detection algorithms enable the identification of shapes and patterns distinct from the surrounding sea (Berg et al., 2022) and are not limited by time. This capability might considerably enhance the detection of submerged animals such as small sharks, jellyfish and sunfish, which remain predominantly underwater and elusive to visual observation methods, contributing to higher abundance estimates compared to human visual detection method.
These results show the difficulty of defining general intercalibration factors for comparing abundance estimates obtained by visual and digital methods. Average intercalibration factors vary greatly between groups of species, between -1 and 0, and therefore cannot be estimated generally for all megafauna data. It is thus crucial to carry out specific intercalibration on each group of species. Adding to this complexity, the species effect is not the only factor to be considered, as our results showed that sea state conditions also contributed significantly to the observed differences in intercalibration factors.
4.1.2 Sea state
As sea conditions deteriorated, intercalibration factors between visual and digital data decreased, with digital methods tending to estimate higher abundance compared to visual methods in rougher seas. Human detection capability is affected by the presence of waves, impeding the accurate detection of marine megafauna (Teilmann, 2003; Pollock et al., 2006). Our results suggest that digital detection may be less impacted by adverse sea conditions. The difference in estimates is unlikely to be the result of an overestimation by digital methods, as in our study all targets detected by algorithms were validated by a trained human operator, eliminating false positives that could potentially arise from wave interference (Boudaoud et al., 2019). The interaction between sea state and species group was not significant, despite the expectation that rougher sea conditions could further impede the detection of inconspicuous species. An analysis focusing on sources of heterogeneity in the visual detection of gannets and alcids highlighted a decrease in sighting density when sea conditions worsened and this decrease occurred similarly for both species (Certain and Bretagnolle, 2008). However, it remains unclear from this analysis whether sea state affected the detection of these species equally or whether both taxa selected areas where sea conditions were calmer. It should be noted that digital methods can also be affected by conditions at sea. For example, Aniceto et al. (2018) found that the reliability of digital detection using unmanned aerial vehicles could be limited in the same way as visual methods and Koski et al. (2009) showed that detection probability using unmanned airborne systems decreased considerably in Beaufort sea states greater than 2. Moreover, since digital methods rely in part on anomaly detection, when the background of an image becomes more heterogeneous due to the presence of waves, animals can be mistaken for image noise (Berg et al., 2022). Overall, our results demonstrate the difficulty of generalizing intercalibration factors that can be used in all meteorological conditions that could be encountered.
4.1.3 Site
Although not retained in the best model, our results showed that site effect could also be a source of variability in the intercalibration factors. This effect likely amalgamated several sources of variability, such as methodological variations between sites or heterogeneity between observers, the processing of digital data, from detection (heterogeneity of algorithms) to identification of animals (heterogeneity between naturalists), and site-specific conditions such as environmental conditions or different abundance of species between sites. These variations, when combined, underscore the complexity and multifaceted nature of the site effect in explaining the observed discrepancy in abundance estimations between monitoring methods. As a result, an intercalibration study carried out for one site does not allow generalization to other sites.
4.2 Digital data acquisition
On average, the estimated intercalibration factor between high and low altitudes with digital methods was close to 0 (on a log scale). Because the resolution of images remained constant on the ground whatever the flight altitude, digital methods might be expected to produce consistent results despite this change in altitude. Conversely, differences in detection were observed with visual methods according to flight height. For example, when examining the effect of doubling altitude, Marsh and Sinclair (1989) found a significantly higher density of turtles at lower altitudes. This result is particularly important, as flying at sufficient altitudes during digital surveys enhances operational safety, especially within or near offshore windfarms.
Across all the comparisons carried out, we observed variability in the intercalibration factor between digital methods, with a 95% confidence interval ranging from -0.699 to 0.516. Covariates relating to sea state and the digital method used explained 18% of this variability. This variability indicated that digital methods were not devoid of bias. Firstly, automated detection algorithms or human analysts reviewing images might inadvertently overlook individuals (Hong et al., 2019; Akçay et al., 2020), especially in challenging environmental conditions. Additionally, species identification heavily relies on the ability of trained human operators with experience of what a species looks like from above, the quality of images, and the number of available images (Brack et al., 2018). Implementing robust quality analyses, involving the reanalysis of a random subset of the dataset by different operators, could help to identify potential detection or identification bias and, if necessary, lead to a correction of the dataset. By reducing these sources of bias, the variability between the digital methods should be reduced.
A limitation of our study was the inability to account for environmental factors affecting images, such as glare or wave presence. Information about sun glare was not consistently recorded for digital methods, and when it was, the metrics used differed between methods, limiting our ability to incorporate this information into our analyses. Consequently, the effect of glare was not included in the detection process for either method, even though glare is known to limit effective coverage in digital monitoring (Kemper et al., 2016) and reduce detection probability in visual monitoring (Certain and Bretagnolle, 2008). Regarding the effect of sea state on intercalibration factors, we relied entirely on the sampling conditions recorded by visual observers during surveys. However, when digital methods are employed without complementary visual monitoring, it becomes essential to obtain information on wave presence directly from the imagery. More broadly, it would be crucial to establish a standardized and automatized metric for characterizing sun glare and wave presence on images across all digital methods. Nevertheless, it is important to note that each detection algorithm among the different digital methods may have different capabilities depending on its training dataset.
These findings underscore the importance of employing a consistent methodology and surveying during favourable weather conditions to minimize variation in intercalibration factors. Yet since the variability explained by the different covariates is low, we must be cautious when assessing trends over time using different digital methods, as this might not necessarily reflect a difference in species abundance but could arise from variability between digital methods. Moreover, digital methods are expected to evolve over time, benefiting from improved automated detection algorithms, finer resolution, and higher-quality images. Consequently, these developments could reduce detection errors. This will need to be considered when monitoring populations over time: interpreting long-term trends in abundance using methods that will continue to improve requires great caution.
4.3 Implications for long-term ecological monitoring of offshore windfarms
Our initial aim was to estimate intercalibration factors that would facilitate a transition between visual and digital methods in the context of monitoring a single windfarm over its lifecycle, from its planning and construction to its decommissioning. In France, offshore windfarm development has lagged behind northern EU Member States, presenting an opportunity for designing adequate impact studies. This gave rise to the Offshore Wind Farm Surveys of Marine Megafauna (OWFSOMM) project. Initial state assessments started with the planning phase of offshore windfarms: i.e. on average, ten years before construction. Initial assessments followed the latest protocols for data acquisition on marine megafauna by means of visual surveys over large areas (Laran et al., 2017). In the meantime, digital data acquisition has become more accessible, and will de facto replace visual surveys due to security reasons for flying over an OWF. This change in flight height was not fully anticipated in the original protocols, but has allowed an opportunity to investigate intercalibration in the OWFSOMM project. Our study was carried out in this framework. The results highlight the pronounced variability of these intercalibration factors, some of which is accounted for by species group and sea state, but a substantial proportion (roughly two-thirds) of this variance remains unexplained. Consequently, even when monitoring the same species group in the same sea state, considerable variability persists. Other factors could contribute to this heterogeneity, such as skill heterogeneity between onboard observers for visual methods and between human operators identifying targets for digital methods, or site idiosyncrasies, or differences in digital processing such as the algorithms used in the study.
Using site- and time-invariant intercalibration factors to switch from one method to another thus seems complicated, if possible at all. It is very difficult to comprehensively control all influencing factors without multiple intercalibration analyses for each new study site. In addition, numerous other variables further complexify the quest for robust intercalibration, necessitating the consideration of a potentially large panel of variables. Consequently, we caution against establishing any long-term trends based on abundance obtained using different visual and digital methods. Transitioning from one method to another without accounting for their differences has high potential for inaccurate inference and thus a misleading interpretation of changes in marine megafauna abundance over the lifecycle of an OWF.
Data availability statement
The datasets presented in this study can be visualized in online repositories. The names of the repository/repositories and accession number(s) can be found below: https://github.com/maudqueroue/OWFSOMM/tree/main/data.
Ethics statement
Ethical approval was not required for the study involving animals in accordance with the local legislation and institutional requirements because the research methods were purely observational.
Author contributions
MQ: Data curation, Formal analysis, Investigation, Methodology, Software, Visualization, Writing – original draft, Writing – review & editing. MA: Conceptualization, Funding acquisition, Methodology, Resources, Supervision, Validation, Writing – review & editing. AB: Conceptualization, Funding acquisition, Methodology, Resources, Supervision, Validation, Writing – review & editing. KH: Funding acquisition, Project administration, Resources, Supervision, Validation, Writing – review & editing.
Funding
The author(s) declare financial support was received for the research, authorship, and/or publication of this article. This work is part of the collaborative OWFSOMM (Offshore Wind Farm Surveys Of Marine Megafauna) project, carried out at France Energies Marines. The OWFSOMM project received funding from France Energies Marines, its members and partners, the French Office for Biodiversity, the General Direction for Energy and Climate as well as French State funding managed by the French National Research Agency under the France 2030 Investment Plan (ANR-10-IEED-0006-34).
Acknowledgments
We would like to thank all 13 project partners (FEM, UBS, CNRS, LRU-PELAGIS, WIPSEA, EDR-RE, Ocean Winds, EOLFI, RWE, Ifremer, CE-Sigma, ENSTA Bretagne, GIS EMDT) for their contributions throughout the duration of the OWFSOMM project. We are grateful to everyone who participated in the aerial surveys, collected all the data and analyzed the images, including Hytech-Imaging, Biotope, Setec In-vivo consulting agencies and Bretagne Vivante. We also thank EDF-RE, Oceanwinds and the French government (“Direction Générale de l’Energie et du Climat”) whom provided dataset from aerial surveys conducted in the framework of regulatory environmental assessment studies. We would like to thank Eve Brunelle for her work on understanding the factors influencing the detection of digital methods.
Conflict of interest
The authors declare that the research was conducted in the absence of any commercial or financial relationships that could be construed as a potential conflict of interest.
Publisher’s note
All claims expressed in this article are solely those of the authors and do not necessarily represent those of their affiliated organizations, or those of the publisher, the editors and the reviewers. Any product that may be evaluated in this article, or claim that may be made by its manufacturer, is not guaranteed or endorsed by the publisher.
Supplementary material
The Supplementary Material for this article can be found online at: https://www.frontiersin.org/articles/10.3389/fmars.2024.1432798/full#supplementary-material
References
Akçay H. G., Kabasakal B., Aksu D., Demir N., Öz M., Erdoğan A. (2020). Automated bird counting with deep learning for regional bird distribution mapping. Animals 10, 1207. doi: 10.3390/ani10071207
Anderson D., Burnham K. (2004). Model selection and multi-model inference. Second. NY Springer-Verlag 63, 10.
Aniceto A. S., Biuw M., Lindstrøm U., Solbø S. A., Broms F., Carroll J. (2018). Monitoring marine mammals using unmanned aerial vehicles: quantifying detection certainty. Ecosphere 9, e02122. doi: 10.1002/ecs2.2122
Authier M., Dorémus G., Van Canneyt O., Boubert J.-J., Gautier G., Doray M., et al. (2018). Exploring change in the relative abundance of marine megafauna in the Bay of Biscay 2004–2016. Prog. Oceanogr. 166, 159–167. doi: 10.1016/j.pocean.2017.09.014
Bailey H., Brookes K. L., Thompson P. M. (2014). Assessing environmental impacts of offshore wind farms: Lessons learned and recommendations for the future. Aquat. Biosyst. 10, 1–13. doi: 10.1186/2046-9063-10-8
Barbraud C., Thiebot J. B. (2009). On the importance of estimating detection probabilities from at-sea surveys of flying seabirds. J. Avian Biol. 40, 584–590. doi: 10.1111/j.1600-048X.2009.04653.x
Barlow J., Gerrodette T., Forcada J. (2001). Factors affecting perpendicular sighting distances on shipboard line-transect surveys for cetaceans. J. Cetacean Res. Manage. 3, 201–212. doi: 10.47536/jcrm.v3i2
Berg P., Santana Maia D., Pham M.-T., Lefèvre S. (2022). Weakly supervised detection of marine animals in high resolution aerial images. Remote Sens. 14, 339. doi: 10.3390/rs14020339
Bergström L., Kautsky L., Malm T., Rosenberg R., Wahlberg M., Capetillo N.Å., et al. (2014). Effects of offshore wind farms on marine wildlife—a generalized impact assessment. Environ. Res. Lett. 9, 34012.
Boudaoud L., Maussang F., Garello R., Chevallier A. (2019). Marine bird detection based on deep learning using high-resolution aerial images. OCEANS 2019-Marseille, 1–7. doi: 10.1109/OCEANSMarseille36106.2019
Brack I. V., Kindel A., Oliveira L. F. B. (2018). Detection errors in wildlife abundance estimates from Unmanned Aerial Systems (UAS) surveys: Synthesis, solutions, and challenges. Methods Ecol. Evol. 9, 1864–1873. doi: 10.1111/2041-210X.13026
Buckland S. T., Anderson D. R., Burnham K. P., Laake J. L., Borchers D. L., Thomas L. (2001). Introduction to distance sampling: estimating abundance of biological populations (Oxford university press).
Buckland S. T., Burt M. L., Rexstad E. A., Mellor M., Williams A. E., Woodward R. (2012). Aerial surveys of seabirds : the advent of digital methods. J. Appl. Ecol. 49, 960–967. doi: 10.1111/j.1365-2664.2012.02150.x
Camphuysen C. J., Fox A. D., Leopold M. F., Petersen I. K. (2004). Towards Standardised Seabirds at Sea Census Techniques in Connection with Environmental Impact Assessments for Offshore Wind Farms in the UK. Report for COWRIE: COWRIE-BAM-02-2002.
Camphuysen C. J., Webb A. (1999). Multi-species feeding associations in North Sea seabirds: jointly exploiting a patchy environment. ARDEA-WAGENINGEN- 87, 177–198.
Certain G., Bretagnolle V. (2008). Monitoring seabirds population in marine ecosystem: The use of strip-transect aerial surveys. Remote Sens. Environ. 112, 3314–3322. doi: 10.1016/j.rse.2008.01.019
Château B. (2022). “Energy Demand Drivers,” in The Palgrave Handbook of International Energy Economics. Eds. Hafner M., Luciani G. (Springer International Publishing, Cham), 511–543. doi: 10.1007/978-3-030-86884-0_26
Croll D. A., Ellis A. A., Adams J., Cook A. S. C. P., Garthe S., Goodale M. W., et al. (2022). Framework for assessing and mitigating the impacts of offshore wind energy development on marine birds. Biol. Conserv. 276, 109795. doi: 10.1016/j.biocon.2022.109795
Dähne M., Peschko V., Gilles A., Lucke K. (2014). Marine mammals and windfarms: Effects of alpha ventus on harbour porpoises. In Ecological Research at the Offshore Windfarm alpha ventus: Challenges, Results and Perspectives. (Wiesbaden: Springer Fachmedien Wiesbaden), pp. 133–149.
Díaz H., Guedes Soares C. (2020). Review of the current status, technology and future trends of offshore wind farms. Ocean Eng. 209, 107381. doi: 10.1016/j.oceaneng.2020.107381
Dierschke V., Furness R. W., Garthe S. (2016). Seabirds and offshore wind farms in European waters: Avoidance and attraction. Biol. Conserv. 202, 59–68. doi: 10.1016/j.biocon.2016.08.016
EEA Report (2023). Share of energy consumption from renewable sources in Europe. Available online at: https://www.eea.europa.eu/en/analysis/indicators/share-of-energy-consumption-from. (accessed November 2023).
Enevoldsen P., Valentine S. V. (2016). Do onshore and offshore wind farm development patterns differ? Energy Sustain. Dev. 35, 41–51. doi: 10.1016/j.esd.2016.10.002
EU Directive (2018). Directive (EU) 2018/2001 of the European Parliament and of the Council of 11 December 2018 on the promotion of the use of energy from renewable sources. Available online at: https://eur-lex.europa.eu/legal-content/EN/TXT/?uri=uriserv:OJ.L_.2018.328.01.0082.01.ENG. (accessed November 2023).
EU Habitats Directive (1992). Council Directive 92/43/EEC of 21 May 1992 on the conservation of natural habitats and of wild fauna and flora. Available online at: https://eur-lex.europa.eu/legal-content/EN/TXT/?uri=CELEX:31992L0043. (accessed November 2023).
European Commission (2023). “Communication from the Commission to the European Parliament, the Council, the European Economic and Social Committee and the Committee of the Regions,” in European Wind Power Action Plan (European Commission Brussels, Belgium).
European Union (2009). Directive 2009/147/EC of the European Parliament and of the Council of 30 November 2009 on the conservation of wild birds (Birds Directive). Off. J. Eur. Union L 20, 7–25. Available at: https://eur-lex.europa.eu/legal-content/EN/TXT/?uri=CELEX%3A32009L0147.
Frankish C. K., von Benda-Beckmann A. M., Teilmann J., Tougaard J., Dietz R., Sveegaard S., et al. (2023). Ship noise causes tagged harbour porpoises to change direction or dive deeper. Mar. pollut. Bull. 197, 115755. doi: 10.1016/j.marpolbul.2023.115755
Frederick P. C., Hylton B., Heath J. A., Ruane M. (2003). Accuracy and variation in estimates of large numbers of birds by individual observers using an aerial survey simulator. J. F. Ornithol. 74, 281–287. doi: 10.1648/0273-8570-74.3.281
French Ministry of Ecological Transition (2022). L’éolien : état des lieux et axes de développement à l’heure de la transition énergétique. Available online at: https://www.ecologie.gouv.fr/parcs-eoliens-en-mer-mediterranee-gouvernement-annonce-selection-13-candidats-participer-deuxieme. (accessed November 2023).
Furness R. W., Wade H. M., Masden E. A. (2013). Assessing vulnerability of marine bird populations to offshore wind farms. J. Environ. Manage. 119, 56–66. doi: 10.1016/j.jenvman.2013.01.025
Garcia-Garin O., Aguilar A., Borrell A., Gozalbes P., Lobo A., Penadés-Suay J., et al. (2020). Who’s better at spotting? A comparison between aerial photography and observer-based methods to monitor floating marine litter and marine mega-fauna. Environ. pollut. 258, 113680. doi: 10.1016/j.envpol.2019.113680
Garthe S., Hüppop O. (2004). Scaling possible adverse effects of marine wind farms on seabirds: Developing and applying a vulnerability index. J. Appl. Ecol. 41, 724–734. doi: 10.1111/j.0021-8901.2004.00918.x
Garthe S., Schwemmer H., Peschko V., Markones N., Müller S., Schwemmer P., et al. (2023). Large-scale effects of offshore wind farms on seabirds of high conservation concern. Sci. Rep. 13, 1–13. doi: 10.1038/s41598-023-31601-z
Gilles A., Authier M., Ramirez-Martinez N. C., Araujo H., Blanchard A., Carlstrom J., et al. (2023). Estimates of cetacean abundance in European Atlantic waters in summer 2022 from the SCANS-IV aerial and shipboard surveys (University of Veterinary Medicine Hannover).
Hammond P. S., Francis T. B., Heinemann D., Long K. J., Moore J. E., Punt A. E., et al. (2021). Estimating the abundance of marine mammal populations. Front. Mar. Sci. 8. doi: 10.3389/fmars.2021.735770
Hong S.-J., Han Y., Kim S.-Y., Lee A.-Y., Kim G. (2019). Application of deep-learning methods to bird detection using unmanned aerial vehicle imagery. Sensors 19, 1651. doi: 10.3390/s19071651
Horswill C., Miller J. A. O., Wood M. J. (2022). Impact assessments of wind farms on seabird populations that overlook existing drivers of demographic change should be treated with caution. Conserv. Sci. Pract. 4, e12644. doi: 10.1111/csp2.12644
Hudson A. V., Furness R. W. (1989). The behaviour of seabirds foraging at fishing boats around Shetland. Ibis (Lond. 1859). 131, 225–237. doi: 10.1111/j.1474-919X.1989.tb02765.x
Kemper G., Weidauer A., Coppack T. (2016). Monitoring seabirds and marine mammals by georeferenced aerial photography. Int. Arch. Photogramm. Remote Sens. Spat. Inf. Sci. 41, 689–694. doi: 10.5194/isprs-archives-XLI-B8-689-2016
Koski W. R., Allen T., Ireland D., Buck G., Smith P. R., Macrander A. M., et al. (2009). Evaluation of an unmanned airborne system for monitoring marine mammals. Aquat. Mamm. 35, 347. doi: 10.1578/AM.35.3.2009.347
Koski W. R., Thomas T. A., Funk D. W., Macrander A. M. (2013). Marine mammal sightings by analysts of digital imagery versus aerial surveyors: a preliminary comparison. J. Unmanned Veh. Syst. 1, 25–40. doi: 10.1139/juvs-2013-0015
Lambert C., Authier M., Dorémus G., Gilles A., Hammond P., Laran S., et al. (2019). The effect of a multi-target protocol on cetacean detection and abundance estimation in aerial surveys. R. Soc Open Sci. 6 (9), 190296. doi: 10.1098/rsos.190296
Lane J. V., Jeavons R., Deakin Z., Sherley R. B., Pollock C. J., Wanless R. J., et al. (2020). Vulnerability of northern gannets to offshore wind farms; seasonal and sex-specific collision risk and demographic consequences. Mar. Environ. Res. 162, 105196. doi: 10.1016/j.marenvres.2020.105196
Laran S., Authier M., Blanck A., Doremus G., Falchetto H., Monestiez P., et al. (2017). Seasonal distribution and abundance of cetaceans within French waters- Part II: The Bay of Biscay and the English Channel. Deep. Res. Part II Top. Stud. Oceanogr. 141, 31–40. doi: 10.1016/j.dsr2.2016.12.012
Lee H., Calvin K., Dasgupta D., Krinner G., Mukherji A., Thorne P., et al. (2023). IPCC 2023: Climate Change 2023: Synthesis Report, Summary for Policymakers. In: Contribution of Working Groups I, II and III to the Sixth Assessment Report of the Intergovernmental Panel on Climate Change. Eds. Lee H., Romero J. (Geneva, Switzerland: IPCC).
Madsen P. T., Wahlberg M., Tougaard J., Lucke K., Tyack P. (2006). Wind turbine underwater noise and marine mammals: Implications of current knowledge and data needs. Mar. Ecol. Prog. Ser. 309, 279–295. doi: 10.3354/meps309279
Marsh H., Sinclair D. F. (1989). An experimental evaluation of dugong and sea turtle aerial survey techniques. Wildl. Res. 16, 639–650. doi: 10.1071/WR9890639
Masden E. A., Haydon D. T., Fox A. D., Furness R. W. (2010). Barriers to movement: Modelling energetic costs of avoiding marine wind farms amongst breeding seabirds. Mar. pollut. Bull. 60, 1085–1091. doi: 10.1016/j.marpolbul.2010.01.016
Miller D. L., Rexstad E., Burt L., Bravington M. V., Hedley S., Model A. (2022). Package ‘dsm.’. doi: 10.32614/CRAN.package.sm
Miller D. L., Rexstad E., Thomas L., Laake J. L., Marshall L. (2019). Distance sampling in R. J. Stat. Software 89, 1–28. doi: 10.18637/jss.v089.i01
Olabi A. G., Abdelkareem M. A. (2022). Renewable energy and climate change. Renew. Sustain. Energy Rev. 158, 112111. doi: 10.1016/j.rser.2022.112111
Peschko V., Mercker M., Garthe S. (2020). Telemetry reveals strong effects of offshore wind farms on behaviour and habitat use of common guillemots (Uria aalge) during the breeding season. Mar. Biol. 167, 118. doi: 10.1007/s00227-020-03735-5
Pollock K. H., Marsh H. D., Lawler I. R., Alldredge M. W. (2006). Estimating animal abundance in heterogeneous environments: an application to aerial surveys for dugongs. J. Wildl. Manage. 70, 255–262. doi: 10.2193/0022-541X(2006)70[255:EAAIHE]2.0.CO;2
R Core Team (2022). R: A language and environment for statistical computing (Vienna, Austria: R Foundation for Statistical Computing). Available at: https://www.r-project.org/.
Tasker M. L., Jones P. H., Dixon T. I. M., Blake B. F. (1984). Counting seabirds at sea from ships: a review of methods employed and a suggestion for a standardized approach. Auk 101, 567–577. doi: 10.1093/auk/101.3.567
Taylor J. K. D., Kenney R. D., LeRoi D. J., Kraus S. D. (2014). Automated vertical photography for detecting pelagic species in multitaxon aerial surveys. Mar. Technol. Soc J. 48, 36–48. doi: 10.4031/MTSJ.48.1.9
Teilmann J. (2003). Influence of sea state on density estimates of harbour porpoises (Phocoena phocoena). J. Cetacean Res. Manage. 5, 85–92. doi: 10.47536/jcrm.v5i1
Teilmann J., Carstensen J. (2012). Negative long term effects on harbour porpoises from a large scale offshore wind farm in the Baltic - Evidence of slow recovery. Environ. Res. Lett. 7 (4), 045101. doi: 10.1088/1748-9326/7/4/045101
Williamson L. D., Brookes K. L., Scott B. E., Graham I. M., Bradbury G., Hammond P. S., et al. (2016). Echolocation detections and digital video surveys provide reliable estimates of the relative density of harbour porpoises. Methods Ecol. Evol. 7, 762–769. doi: 10.1111/2041-210X.12538
Wisniewska D. M., Johnson M., Teilmann J., Siebert U., Galatius A., Dietz R., et al. (2018). High rates of vessel noise disrupt foraging in wild harbour porpoises (Phocoena phocoena). Proc. R. Soc B Biol. Sci. 285 (1872), 20172314. doi: 10.1098/rspb.2017.2314
Keywords: digital surveys, visual surveys, seabirds, small cetaceans, impact assessment surveys, offshore wind farms
Citation: Quéroué M, Authier M, Besnard A and Heerah K (2024) Going digital: challenges in monitoring marine megafauna when comparing results from visual and digital aerial surveys. Front. Mar. Sci. 11:1432798. doi: 10.3389/fmars.2024.1432798
Received: 14 May 2024; Accepted: 02 October 2024;
Published: 23 October 2024.
Edited by:
Mark Jessopp, University College Cork, IrelandReviewed by:
Dominik André Nachtsheim, University of Veterinary Medicine Hannover, GermanyOriol Giralt Paradell, University College Cork, Ireland
Copyright © 2024 Quéroué, Authier, Besnard and Heerah. This is an open-access article distributed under the terms of the Creative Commons Attribution License (CC BY). The use, distribution or reproduction in other forums is permitted, provided the original author(s) and the copyright owner(s) are credited and that the original publication in this journal is cited, in accordance with accepted academic practice. No use, distribution or reproduction is permitted which does not comply with these terms.
*Correspondence: Maud Quéroué, bWF1ZC5xdWVyb3VlQGdtYWlsLmNvbQ==