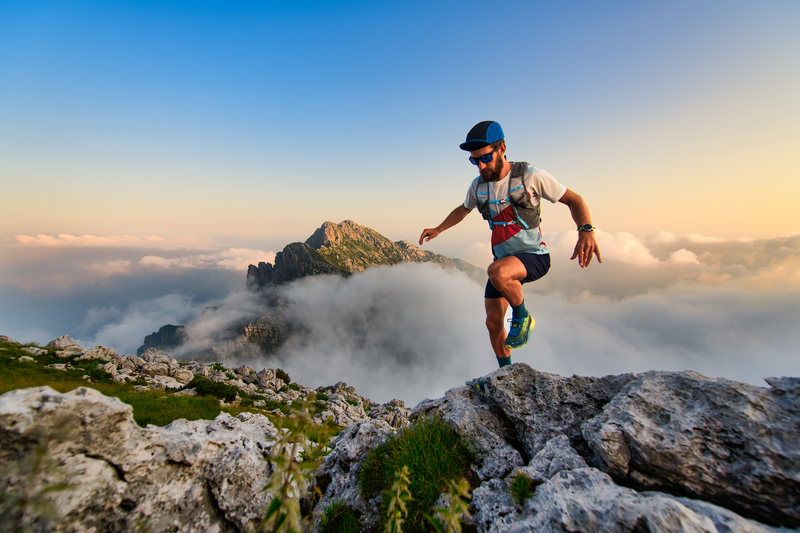
94% of researchers rate our articles as excellent or good
Learn more about the work of our research integrity team to safeguard the quality of each article we publish.
Find out more
ORIGINAL RESEARCH article
Front. Mar. Sci. , 05 September 2024
Sec. Marine Ecosystem Ecology
Volume 11 - 2024 | https://doi.org/10.3389/fmars.2024.1416359
This article is part of the Research Topic Marine Plankton: Biological and Chemical Interactions View all 6 articles
Introduction: Coastal marine ecosystems are among the most ecologically and socio-economically dynamic regions on Earth, and the study of eukaryotic planktonic communities is an important aspect of aquatic ecology. It is essential to monitor plankton biodiversity and identify influencing factors in order to measure human effects on coastal waters and help contribute to ecosystem preservation. Environmental DNA (eDNA) metabarcoding analysis is an advanced toolfor detecting the presence of aquatic organisms, which has revolutionary significance for biodiversity monitoring and real-time detection of wild environments.
Methods: In this study, eDNA metabarcoding was used to study seasonal changes in eukaryotic plankton communities in subtropical coastal waters of the northern South China Sea, to explore how various trophic groups affect the complexity and stability of the species network.
Results: The results showed that the summer interaction network is looser and had has reduce biodiversitycompared to the spring interaction network. As an important part of the planktonic community, the abundance and diversity of algae reflect the interactions between different species and the influence of environmental factors on the dominant species, particularly temperature and nutrients. In addition, producer diversity set the basis for species network complexity and indirectly affected network stability, while consumer diversity and its flexible predation strategies may directly lead to differences in network stability across seasons. There were close correlations among species interaction, environmental factors and species diversity.
Discussion: This study can help us understand the ecosystem functions throughout the year and the complex interactions of planktonic communities from a multiple trophic level perspective, and provide effective methods for sustainable resource utilization and ecological environmental protection in the future.
Plankton are found in almost all habitats on earth and cover a wide spectrum of cell sizes, shapes and taxonomic classifications. Plankton are natural inhabitants that coexist in aquatic ecosystem, playing a crucial role in maintaining biodiversity and food webs, and establishing intricate networks of ecological interactions in aquatic environments (Duffy et al., 2007; Malfatti and Francesca, 2007).
Biological community ecology has long been interested in studying the mechanisms involved in their assembly and how they respond to their environment to understanding the ecological processes determining the community assembly of plankton community (Li and Hu, 2021c). The ocean remains the least sampled habitat in a variety of natural environments, which presents difficulties in understanding the drivers of its biodiversity (Fonseca et al., 2014). Therefore, the exploration of marine environments that maintaining a large number of geographical differences will provide stronger evidence to resolve the debate over the distribution of plankton on earth (Faurby and Funch, 2011). In addition, due to the large amount of undescribed diversity, it is difficult to understand the diversity of microeukaryotes through traditional morphological methods alone (Bika et al., 2012). At present, traditional methods such as morphological identification are still commonly used for routine monitoring of coastal plankton communities. However, the effectiveness of these methods in providing comprehensive information on plankton diversity has been called into question. This is primarily due to the challenges posed by the small size of microeukaryotes and the identification of concealed immature stages, which necessitate specialized advanced classification techniques. Therefore, a fast, simple, and sensitive method is needed to monitor eukaryotic plankton in coastal ecosystems (Uusitalo et al., 2013; Thomsen and Willerslev, 2015; Thalinger et al., 2019; Castañeda et al., 2020; Yang et al., 2021; Zhang et al., 2023a).
As for the planktonic community, it may exhibit unique and distinct phenological features driven by seasonal changes in the environment, biological signatures, and biological interactions, with implications for temporal changes in biogeochemistry and ecosystem function. The causal relationship among environmental factors, species diversity and ecosystem function has always been the focus of ecological research (Chapin et al., 2000; Snelgrove et al., 2014; Dee et al., 2017). The interaction between them is always complex and highly dependent on the external environment (Chang et al., 2022). Environmental factors affect the composition and productivity of plankton, thus strongly influencing plankton diversity (Negrete-García et al., 2022; Loschi et al., 2023).
Besides, the complex relationship between species diversity and ecosystem stability has always been one of the key concerns in the field of ecology (Naeem and Li, 1997). The general conclusion of the existing studies is that the reduction of species diversity is likely to weaken the stability of the ecosystem, while in the context of global climate and environment change, the fluctuations of environmental factors are likely to have an important impact on the structure and process of the ecosystem and change the species diversity, and then change the function and stability of the ecosystem (Hooper et al., 2012; Zhou-Yuan et al., 2021). While functional redundancy (multiple species per functional group) is meaningful to maintain the stability of community function, and the conservation of biodiversity can provide enough functional redundancy for the ecosystem to maintain the long-term stability of ecosystem function (Naeem and Li, 1997). Meanwhile, complexity has also considered as an important factor affecting stability, and new research has also shown that highly diverse ecosystems maintain their own stability by reducing complexity when it exceeds a certain threshold (Yonatan et al., 2021).
Eukaryotic plankton communities, which are composed of algae, fungi, protozoa, and metazoans through complex interactions, are important players in marine ecosystems, serving as primary producers, predators, decomposers, and parasites (Sherr et al., 2007; Zubkov and Tarran, 2008). The interactions of mass and energy transport between these multiple trophic levels determine complex ecosystem processes, while the previous community studies focused on monitoring a single trophic level, ignoring the interactions between multiple trophic groups and their respective ecological functions (Brose, 2008; Zhou-Yuan et al., 2021). In recent years, network analysis has been increasingly used to study potential biological interactions and reflect ecological stability among members of microbial communities based on network topological properties, which is now an effective tool for ecological community studies. The patterns and topological features of co-occurring networks (i.e., degree, intermediacy, clustering coefficient, short path length, and modular structure) may provide new insights into interspecies interactions and ecological issues (Kara et al., 2013). Species co-occurrence networks exhibit non-random associations and modular structures, suggesting that complex interactions between different species may play an important role in controlling community aggregation and maintaining community stability (Chaffron et al., 2010). Complex interactions were recorded among members of plankton through a network of graded ecosystems along estuaries (Konopka et al., 2015). Thus, it may be a challenge to figure out the relative importance of the various controlling forces that shape plankton communities (Wu et al., 2023).
In this study, an eDNA metabarcoding method based on 18S rRNA gene was used to investigate the seasonal dynamic and interaction mechanism of eukaryotic plankton communities in subtropical waters. Consequently, we conducted a one-year eDNA survey in Nan’ao Island (NAO) area, a typical subtropical sea in China. This one-year survey was conducted to reflect the seasonal time changes in the sea area. Our work aimed to address the following issues: (1) Investigating the correlation between variations in the eukaryotic plankton community and its network in subtropical coastal regions, as well as their dependence on environmental factors. (2) Examining the distinct impacts of various tropical groups on biological communities. (3) Exploring the interrelationship and impact among species interaction, environmental factors and species diversity.
Our study area is located in eastern Guangdong Province, China, and is offshore waters near NAO (Figure 1). The NAO in South China Sea is a hotspot for biodiversity research (Xie et al., 2017), including Nanpeng Islands National Marine Reserve. Meanwhile, they are also the bays that are seriously affected by human activities and have the highest degree of eutrophication in east Guangdong Sea (Gu et al., 2008). We collected samples from 34 sites among above NAO (23.032-23.726°N, 116.740-117.468°E), including two summer upwelling zones, a breeding zone, an estuary zone, a critical zone of national reserve and a natural sea area, in winter (January), spring (April), summer (August) and autumn (November) of 2021. In this study, 91 surface water samples were collected from the waters near NAO. Environmental parameters, including water temperature (°C), salinity, dissolved oxygen (DO, %), chlorophyll estimated from fluorescence (Chl a, μg L-1), density (g cm-3), soluble reactive phosphorus (SRP, μmol L-1), dissolved inorganic nitrogen (DIN, μmol L-1), dissolved silicon (DSi, μmol L-1) were measured at the same time when samples collected.
Environmental DNA extraction kit (MT058, Nanjing Yijinuo Environmental Protection Technology Co., LTD.) was used to extract eDNA enriched on the filter membrane. The extracted DNA was quantified and checked for purity by Qubit™ 4 Fluorometer (Q33226) and A260/280 nm and A260/230 nm in ND5000.
PCR with 18SV9 primers (F: CCTTCYGCAGGTTCACCTAC; R: TTGTACACACCGCCC) was performed on DNA respectively. Among them, 18S were amplified by Taq polymerase using a 30 μL reaction system. The reaction system was as follows: 15μL 2*Taq Mix, 1.5μL of 10μM upstream primer, 1.5 μL of 10μM downstream primer, 1μL DNA, 11μL ddH2O. The amplification reaction was performed in a gene amplification apparatus (TC-96/G/H (b)B) under the following conditions: hot cap at 105°C, initial denaturation at 95°C for 3 min, denatured at 95°C for 15 s, annealed at 62°C for 30 s, extended at 72°C for 15 s, 28 cycles, it was extended at 72°C for 5 min and stored at 4°C.
To reduce a single PCR error, three PCR reactions were performed on each sample. PCR products were examined by 2% (w/v) agar-gel electrophoresis and Gel Doc EZ (735BR00194) gel images to check band size and specificity, and the PCR products were mixed equimolar using a fully automatic DNA pipette workstation. The PCR mixed products were purified using VAHTS DNA Clean Beads (N411-02) kit. The purified mixture was quantified using Qubit™ 4 Fluorometer (Q33226). Purified PCR mixed products were used for subsequent sequencing. Libraries were constructed using the VAHTS® Universal DNA Library Prep Kit for Ion Torrent V2 (ND702) kit, which could convert purified mixed PCR products into dedicated libraries for Ion Torrent high-throughput sequencing platforms. Prior to second-generation sequencing, the length and concentration of all DNA products were tested using gel electrophoresis and Qubit™ 4 Fluorometer. Adjust the final concentration of the sample to 100 pM. The sequencing templates were prepared and enriched with lon OneTouch 2TM and Lon OneTouch ES and sequenced in Proton Semiconductor Sequencer by lon PITM Chip Kit V3 chip.
The data analysis of high-throughput sequencing was all based on the ubuntu14.04 EcoView2.0 software. Crop, sorted, and distinguished by unique sample labels (12bp barcodes with a maximum number of mismatches set to 2) (Caporaso et al., 2010). The UPARSE (Edgar, 2013) pipeline clustered all sequences into operational taxa (OTUs) with a similarity threshold of 97%, and the 18S annotation databases were the Greengenes (DeSantis et al., 2006) and Silva databases (Pruesse et al., 2007).
The conductivity temperature-depth sensor (CTD) fitted with the research vessel was used to determine the vertical Temperature, Salinity, dissolved oxygen (DO), chlorophyll a (Chl a) and Density (amount of mass per unit volume, which related to temperature, salinity and pressure) at each sampling point.
In this study, soluble active phosphorus (SRP), dissolved silicate (DSi) and dissolved inorganic nitrogen (DIN) were determined. DIN, where soluble inorganic nitrogen is estimated by the sum of nitrate, nitrite and ammoniacal nitrogen concentrations. The measured samples were collected and put into acid-washed polyethylene bottles and frozen at -20°C for analysis. AA3 automatic nutrition analyzer spectrophotometer method was used for the determination. The inorganic nutrient data in this study are shared data from the Marine Chemistry Group on the voyage.
Alpha-diversity indices, including Shannon (measure the evenness of species in a community) and Simpson (the probability that the number of individuals obtained from two successive samples of a community species belong to the same species) were calculated using relative abundance in R package “vegan”. Alpha-diversity (the number of species in a community and the number and distribution of each species) directly shows the changes in the number of species in a community, while beta-diversity (in the form of matrix data, the differences in the number and distribution of each species in the two communities were compared one by one) can reflect the driving process of community change (Chen et al., 2015). Beta-diversity was calculated by non-metric multidimensional scaling (NMDS) analysis based on Bray-Curtis distance using the R (ver. 4.1.3) package “vegan” and “ggplot2” to study differences in eukaryote composition among four seasons. Analysis of similarity test (ANOSIM) was used to test for differences between community structures and sampling seasons in R package “vegan” (Yang et al., 2023). Redundancy analysis (RDA, based on the Bray-Curtis distance) were performed to analyze the correlation between environmental factors and the community of eukaryotic plankton in R package “vegan”.
Co-occurrence networks in NAO were constructed by using eukaryotic plankton combined to family level (this level can basically make a clear distinction between the feeding habits of species). R package “ggCLusterNet” was used to build this network using SparCC method and calculated the network indices including complexity, Robustness and Vulnerability (Kurtz et al., 2015; Wen et al., 2022). Only robust and significant correlations (|r|>0.6, p< 0.05) were incorporated into the networks (Ren et al., 2022) and the networks were visualized using the interactive Gephi 0.10.1 platform. To analyze the direct and indirect effects of environmental factors on community structure and find out the driving factors, we used a Structural equation model (SEM) to determine the paths among environmental change and network stability. Furthermore, there were four main parts in this model including environmental factors (climate: including temperature, Chl a, density, sality, DO & nutrient: including DIN, SRP and DSi), biodiversity (producers: phytoplankton and certain autotrophic protists, primary consumers: phytophagous and detritophagous plankton, predator: carnivorous zooplankton). The SEM model was constructed using R package “piecewiseSEM” (v 2.3.0).
A total of 10 206 857 operational taxonomic units (OTUs) were obtained by 18S RNA sequencing, and a total of 485 eukaryotic plankton were detected in the sequencing data set. The alpha-diversity index, including Shannon and Simpson indices, exhibited seasonal variation within the plankton composition across the four seasons. The summer exhibited the lowest Shannon index, which demonstrated a large divergence from the spring community. The Simpson index exhibited its lowest values throughout the spring and summer, with no discernible disparity between the two. Meanwhile, the alpha-diversity of winter and fall exhibited a comparable pattern in the Simpson index.
The NMDS analysis of the eukaryotic plankton community revealed that the communities in the four seasons exhibited some degree of overlap. Specifically, the winter, spring, and autumn communities showed a higher level of overlap, while the summer community only partially overlapped with the spring community. The ANOSIM analysis revealed a significant difference in the community groups throughout the four seasons (R=0.58, p-value <0.001). While the species composition remained steady during autumn and winter, it underwent significant changes during spring and summer (Figure 2). In summer, there was a substantial decline in the population of Cryptophyta, which was one of the dominant species belonging to algae. Conversely, there was a big increase in the population of Bacillariophyta. The population of Ciliophora, one kind of protozoa, showed a noticeable decrease throughout the summer season. The primary constituents in order level included Arthropoda, Bacillariophyta, Dinophyta, Chlorophyta, Cryptophyta, and Ciliophora. The top five phylum species collectively represented more than 80% of each season (Figure 3).
Figure 2. Diversity of eukaryotic community and influence of environmental factors. (A) alpha-diversity of eukaryotic community in four seasons including Shannon index and Simpson index, the * means p < 0.01, ** means p < 0.005 and *** means p < 0.001; (B) beta-diversity of eukaryotic community in four seasons; (C) RDA analysis of eukaryotic community including 8 environmental factors, the arrows represent different environmental factors, the larger dots represent different samples, and the smaller yellow dots represent different samples.
Figure 3. Composition in four seasons at phylum level. The postfixs of sample names mean the different season, “.1” means winter, “.2” means spring, “.3” means summer and “.4” means autumn.
There were obvious seasonal changes in environmental factors (Supplementary Table S1). Seasonal variations of average salinity, temperature and fluorescence chlorophyll showed that the highest average salinity occurred in summer, while the lowest was in winter. The values of nutrient salts were low in spring and summer while high in winter and spring.
The impact of environmental factors on the eukaryotic plankton community was not consistent. The RDA analysis revealed that the impact of nutrient salts (such as DIN, SRP, DSI) and physicochemical parameters (such as temperature, salinity, dissolved oxygen, chlorophyll, density) on the eukaryotic plankton communities displayed contrasting trends. RDA1 accounted for 31.7% of the explanation, while RDA2 accounted for 18.47% (Figure 2). The winter community saw significant impacts mostly due to nutrient salts, whereas the autumn community was primarily influenced by the physical and chemical qualities of water. In summer, when temperature fluctuations were greatest, plankton communities showed the most diverse composition.
Network analysis is increasingly being used to study potential interactions between members of biomes and ecological stability based on network topological properties. The patterns and topological features of co-occurrence networks (i.e., degree, intermediacy, clustering coefficient, short path length, and modular structure) may provide new insights into interspecies interactions and ecological issues (Kara et al., 2013). Seasonal environmental changes had significant effects on plankton network structure. In summary, topological feature parameters indicated that the eukaryotic plankton community network was more complex and compact in spring and simplest in summer (Figure 4). Network properties showed that the index related to network complexity was lowest in summer and peaks in spring. Spring networks exhibited a high degree of modularity, complexity, and functional redundancy, while summer networks exhibited the opposite (Figure 5).
Figure 4. Network analysis of eukaryotic community. (A) results of modular network analysis in four seasons; (B) comparison of 9 network topological properties in four seasons; the * means p < 0.01, ** means p < 0.005 and *** means p < 0.001. NS means not significant.
Figure 5. Network analysis of eukaryotic community. (A) presents the network analysis results of the interaction between nodes; (B) Zi-Pi analysis of four networks, based on the Zi and Pi values, network nodes can be divided into the following four categories: Module Hubs (central point of a module, referring to nodes with high connectivity within a module, Zi > 2.5 and Pi < 0.62), Connectors (Connectors, referring to nodes with high connectivity between two modules, Zi < 2.5 and Pi > 0.62), Network Hubs (nodes with high connectivity in the network, Zi > 2.5 and Pi > 0.62) and Peripherals (peripheral nodes, nodes without high connectivity within and between modules, Zi < 2.5 and Pi <0.62); (C) community stability including robustness and vulnerability.
Combining environmental factors, biodiversity of different groups and ecological network attributes, the following models are constructed in SEM to explore the factors of plankton community diversity change and its influence on community structure. Our result of SEM showed that in the eukaryotic plankton community, the factors influencing the characteristics of the species co-occurrence network may result from changes in the diversity of various trophic groups brought about by the external environment (Figure 6). Species diversity of different trophic levels showed obvious seasonal changes (Figure 7), which were related to environmental factors (climate: including temperature, Chl a, density, sality, DO & nutrient: including DIN, SRP and DSi) and network stability to a certain extent. The study found that distinct trophic groups (like predator and producer) exhibited varying or even opposite effects on the network’s stability and complexity, while the two categories of environmental factors—nutrient salts and climatic conditions—had opposing effects on the properties of the trophic group and the network. The complexity of the network was the most significantly impacted by the diversity of producers (phytoplankton and certain autotrophic protists) (path coefficient = 0.35, which measures the strength and direction of the relationship between variables). In contrast, the stability of the network was primarily determined by the diversity of consumers, and its vulnerability was primarily determined by the diversity of primary consumers (phytophagous and detritophagous plankton) (path coefficient = -0.25). The diversity of primary and secondary consumers (carnivorous zooplankton) had a major impact on robustness. In addition, network complexity had a greater impact on network vulnerability (path coefficient = 0.87). Different environmental factors had opposing impacts on the same factor and primarily impacted trophic group diversity as opposed to directly affecting network stability. Among them, primary consumers were mostly affected by the two types of environmental factors.
Figure 6. The influence paths among environmental factors, species diversity of different trophic groups, network complexity and stability analyzed by SEM. (A) path analysis among environmental factors, species diversity of different trophic groups, network complexity and robustness; (B) path analysis among environmental factors, species diversity of different nutrient groups, network complexity and vulnerability; the * means p < 0.01, ** means p < 0.005 and *** means p < 0.001.
Figure 7. Comparison of species diversity among different trophic groups (A) alpha-diversity of producer; (B) alpha-diversity of primary consumer; (C) alpha-diversity of predator; the * means p < 0.01, ** means p < 0.005 and *** means p < 0.001.
Coastal marine ecosystems are the most ecologically and socio-economically vital zones on the planet (Harley et al., 2006), and the study of eukaryotic plankton communities is an important part of aquatic ecology research (Sprong et al., 2020). Monitoring eukaryotic plankton biodiversity is essential in measuring anthropogenic influence on coastal waters, which also helps ecosystem protection (Fišer et al., 2018). Now eDNA metabarcoding, as a rapidly developing technology, can overcome the limitations of conventional methods to rapidly and accurately identify microeukaryotes (Deiner et al., 2017). Besides, seasonal data of plankton communities could also provide valuable information on the occurrences, movements, and distributions of aquatic species (Sigsgaard et al., 2017), which is helpful to better understand the internal structural changes of biomes and the driving factors.
As for the planktonic community, it may exhibit unique and distinct phenological features driven by seasonal changes in the environment, biological signatures, and biological interactions, with implications for temporal changes in biogeochemistry and ecosystem function.
Our results also proved that plankton communities had different successional patterns in different seasons, and this succession pattern was largely the result of seasonal changes in physical and chemical properties of water. In our results, the resilience and vulnerability of species networks also showed similar results to network complexity. In addition, the proportion of negative correlations reflecting prey-predator associations in the plankton network was lowest in summer and highest in winter (Käse et al., 2021), suggesting that summer may be mostly bottom-up regulated with less top-down regulation. In this subtropical sea area, summer temperature was the highest throughout the year, followed by spring, while these two seasons showed completely different community structure patterns (Figure 2), with the resembled nutrient salt. This may be one of the reasons for the seasonal variation of phytoplankton dominant species. Phytoplankton, as the main producers in marine ecosystems, is sensitive to subtle environmental disturbances in aquatic ecosystems, and its community characteristics and dynamic changes are usually the result of spatiotemporal integration of multiple environmental factors (Jiang et al., 2014; Lin et al., 2023).
Among them, fluctuation of temperature is likely to cause large differences in the network between spring and summer in our result, which also be confirmed in previous study that water temperature is considered to be the strongest environmental parameter (Forster et al., 2021). Temperature has been proved to play an important role in the composition of phytoplankton and the replacement of dominant species. As an important ecological factor, water temperature can directly or indirectly affect the growth of algae by influencing the enzymatic reaction of algae. Different phytoplankton have different temperature adaptation ranges, or have different competitiveness in different temperature ranges (Zhang et al., 2012). It is generally believed that Bacillariophyta and Chlorophyta belong to the high-temperature tolerant type of algae (Poste et al., 2013), while Cryptophytes belong to the cold-water type of algae, and their abundance is often negatively correlated with temperature (Chen, 2014; Xiao et al., 2019; Lin et al., 2023), which also conformed the result of this study and the abundance of Cryptophytes was the lowest of the whole year. Studies had shown that in aquatic systems with mixed algae, Bacillariophyta was dominate at 20°C and Chlorophyta was dominate at 30°C (Xu et al., 2014). In addition, the existence of summer upwelling in the study area was more conducive to the growth of Bacillariophyta while it doesn’t conform to the grow condition of Chlorophyta (Chen, 2014; Morais et al., 2018), which also made Bacillariophyta the significantly dominated species of phytoplankton in summer. In the case of phytoplankton communities, the decline in species diversity is likely to be caused by the dominance of a particular species (Srichandan et al., 2019).
Temperature is a limiting factor for most aquatic organisms and determines important ecological processes in communities, such as primary productivity respiration and carbon cycle (Zhang et al., 2023b), which is also suggests a negative relationship between climate condition and diversity of producer in our result (Figure 6). Thus, we need to pay more attention to the negative impacts on plankton communities caused by global climate warming, which are considered a major threat to aquatic ecosystems (Mei et al., 2022). Meanwhile, due to the decrease of nutrient concentration in summer, the interspecific competition of phytoplankton is likely to increase, and the species diversity was likely to decrease due to the intense competitive exclusion between species (Bužančić et al., 2016). The seasonal fluctuation of environmental factors influenced the change of plankton community to a certain extent, and became the limiting factor or promoting factor of biological growth at different periods, while temperature seemed to play the most crucial role in driving changes in the community composition among them in our result. Both these may be the important reasons for the decline of producer alpha-diversity. Besides, as an important and more specific food of Ciliates, the fluctuation of Cryptophytes abundance significantly affected the abundance of Ciliates, showing an obvious positive correlation (Supplementary Figure S1). As key herbivores in the plankton ecosystem, Ciliates undertake important intermediate carriers that transfer carbon to higher levels of the food web (Kosiba and Krztoń, 2022). This strong bottom-up regulation is also evident in our research, especially in algae and herbivores. Seasonal succession of plankton communities has been well documented and studied in many aquatic ecosystems (Gleason et al., 2011; Forster et al., 2021; Käse et al., 2021). Although the dynamic changes of species in the community are regulated by top-down and bottom-up, the bottom-up climate processes dominate the dynamic changes of plankton in the community (Lynama et al., 2017).
In marine plankton communities, keystone or dominant species are often determined by interactions between biological, chemical, and physical drivers that often change rapidly. Considering the synergistic changes of physical and chemical properties of water bodies (especially temperature) and nutrients on predator-prey interaction and nutrient transfer of ecosystem output, the interaction between environmental factors, producers and consumers is complex, including various direct and indirect effects (Franzè et al., 2023). Combined with the environment, interspecific interaction and network, the role of bottom-up in this kind of area is worthy of attention.
Ecosystem stability is a multi-dimensional concept, among which the most commonly used are resistance, resilience, persistence and variability (Li et al., 2021d). In this study, we considered that network stability indicators including vulnerability and robustness, which may be more suitable to measure network stability compared with others. Although these two indicators measure network stability from different dimensions, different trophic groups have different effects on stability (Figure 6). It has been showed that complexity was significantly correlated with network stability, especially its impact on network vulnerability in our results. How the stability of an ecosystem depends on its complexity is a challenging question that can be roughly measured by the diversity of species in the ecosystem and their interactions (Johnson et al., 1996; Loreau and de Mazancourt, 2013). And it is generally believed that the stability of food webs increases with the complexity of food chains resulting from species diversity within limits (Li et al., 2021a, b). In our data results, producers indirectly influenced network stability by positively influencing network complexity, while consumer diversity directly influences network stability, especially phytoplankton-feeding primary consumers. Species diversity is thought to play an important role in maintaining the ecological stability of plankton communities (D’Alelio et al., 2016). Among them, producer diversity has been shown to improve a range of ecosystem service functions, such as ecosystem primary productivity, nutrient cycling, and organic matter degradation (Hector et al., 1999; Fridley, 2003). It has been pointed out that the diversity of phytoplankton, as the main producers in an ecosystem, has a positive effect on the biodiversity and ecological function of consumers, and that plant diversity has a positive effect on the diversity and nutrient interaction at higher trophic levels (Poisot et al., 2013; Fornoff et al., 2019; Chen et al., 2022). Meanwhile, high phytoplankton diversity is often matched by high functional diversity, meaning that high species diversity is a fundamental characteristic necessary to maintain the functioning of the plankton ecosystem (D’Alelio et al., 2016). What’s more, the effects of plant diversity loss were most pronounced in herbivores, with a weaker response at higher trophic levels (Scherber et al., 2010; Schuldt et al., 2019; Wang et al., 2020).
At the same time, consumer diversity also has an important impact on ecosystem function. Existing studies had shown that the impact of consumer diversity on ecosystem function is mainly realized through sampling effect and resource sharing in two basic ways, while indirect, non-additive interactions between trophic levels can only correct the degree of influence (Yan et al., 2008). Predators in plankton have flexible prey rather than specific prey, and flexible food web interactions have been shown to be more common than specific predatory relationships in eukaryotic plankton communities (Chang et al., 2022). The opportunistic feeding strategies of zooplankton greatly increased the difficulty of extracting predator-prey dynamics in this community (Käse et al., 2021), and further complicated the trophic levels of predators. In the context of the current decline in global biodiversity extinction, topological simulation studies have found that food webs with higher species richness or higher connectivity are more resistant to species extinction and produce fewer secondary extinctions, therefore the food webs have higher robustness (Dunne et al., 2002). Based on dynamic simulations, it was found that food webs with more nodes were less resistant to species extinction, while food webs with higher connectivity were more resistant to species extinction (Zhao et al., 2016).
Overall, the relationship between diversity, complexity and stability has always been the focus of attention in the field of ecology, especially the complexity correlation among diversity and stability, which is not a simple liner correlation (Brose, 2008; Landi et al., 2018). The early conclusions were mostly focused on the impact of single trophic level of primary producers on ecosystems. The negative effects of diversity loss were well understood, and diversity effected appear to be surprisingly common in ecosystems (Cardinale et al., 2011). With the deepening of research, it was proved that the diversity and stability relationship of multi-trophic community were different from that of single trophic community (Jiang and Pu, 2009). In nature, ecosystem processes and stability are usually determined not by the diversity of trophic level, but by the interactions of matter and energy transport between different trophic levels (Zhou-Yuan et al., 2021). In a multitrophic community, the horizontal diversity within the trophic level can promote the resilience and resistance stability of the ecosystem, but the vertical diversity between the trophic levels may have the opposite effect (Zhao et al., 2019). There was a direct and significantly positive correlation between producer diversity and network complexity, thus affecting network stability. However, due to the flexible rather than specific predator-survival strategies of consumers in the plankton community, the variable interaction relationship and the change of trophic level may lead to the non-simple positive correlation between the diversity and network stability.
Recent studies had shown that the effects of species replacement on aquatic communities may not be sufficient to reflect changes in taxonomic diversity, but changes in community structure were often more easily observed (Bruno et al., 2019; Finderup et al., 2019; Zhang et al., 2022; Li et al., 2023). The functional consequences of biodiversity decline in multiple trophic taxa were difficult to predict from studies that focus on a single taxon because the functional effects of different taxa may complement or oppose each other (Li et al., 2023). Multiple trophic level analysis plays an important role in analyzing the determinants of biodiversity and community composition (Doi et al., 2013). Compared with other existing technologies, environmental DNA technology has more and more obvious advantages in the spatial and temporal distribution of species communities and multi-group biodiversity survey, and its non-invasive, sensitive, convenient and low-cost nature is making it more and more applied (Goldberg et al., 2011). Our results showed that the alpha-diversity of eukaryotic plankton community did not change significantly in the four seasons, but there were significant differences in the species co-occurrence network (Figures 4, 5), and the complexity of the network was significantly related to the seasonal changes of environmental factors. In addition, the change of species diversity within the trophic group changed the co-occurrence network, with the core Hubs of the network were different in each season. The change of key species and network may be due to the change of diversity of a trophic group in the plankton community, which affected the species interaction (such as predation, symbiosis) within the community (Doi et al., 2013). Changes in species diversity among different plankton groups in the same community are not uniform, resulting in seasonal differences in community structure. Our results also showed that eDNA datasets were useful for providing cross-species community information (Taberlet et al., 2012), and could provide feedback on the seasonal responses of key taxa and species co-occurrence networks and the dynamics of different trophic groups, rather than simple species-specific abundance or diversity changes (Li et al., 2023).
We concluded that eDNA matabarcoding can be employed to provide cross-species community information, as well as seasonal change of key taxa and species co-occurrence networks. Furthermore, the eDNA matabarcoding technology offers a valuable method for investigating the impact of abiotic factors on the structure and distribution of biological communities in coastal ecosystems, as well as for identifying the primary factors. This serves as a scientific foundation for the future preservation of the coastal ecosystems.
The eDNA sequencing data generated in this work have been deposited in the Figshare repository and can be download from https://doi.org/10.6084/m9.figshare.26531350.v1.
All applicable international, national, and/or institutional guidelines for the care and use of animals were followed by the authors.
XL: Visualization, Investigation, Formal analysis, Writing – original draft, Methodology, Data curation. KL: Methodology, Data curation, Writing – original draft, Formal analysis. HZ: Writing – review & editing, Investigation, Formal analysis. YG: Writing – review & editing, Investigation, Formal analysis. ZHZ: Validation, Methodology, Writing – review & editing, Formal analysis. LW: Investigation, Writing – review & editing, Methodology, Formal analysis. XW: Writing – review & editing, Investigation. ES: Writing – review & editing. ZZ: Writing – review & editing, Investigation, Formal analysis. QG: Writing – review & editing, Formal analysis. SL: Writing – review & editing, Formal analysis. PL: Writing – review & editing. XY: Investigation, Writing – review & editing. ZC: Investigation, Writing – review & editing. JL: Writing – original draft, Supervision, Funding acquisition, Formal analysis, Conceptualization, Investigation. WL: Funding acquisition, Project administration, Writing – original draft, Writing – review & editing, Conceptualization.
The author(s) declare financial support was received for the research, authorship, and/or publication of this article. This work was supported by the Key Program of the National Natural Science Foundation of China (42230413), Guangdong Basic and Applied Basic Research Foundation (2024A1515011082), the Key Special Project for Introduced Talents Team of Southern Marine Science and Engineering Guangdong Laboratory (GML2019ZD0606), the Key Program of Marine Economy Development (Six Marine Industries) Special Foundation of Department of Natural Resources of Guangdong Province (GDNRC[2022]48), the Scientific Research Foundation for Talents, STU (NTF21026), the Science and Technology Plan Projects of Guangdong Province (No. 2021B1212050025) and the Program for University Innovation Team of Guangdong Province (2022KCXTD008).
The authors acknowledge Tong Chen at Shantou University and all the members in our team for their help in sample collection.
The authors declare that the research was conducted in the absence of any commercial or financial relationships that could be construed as a potential conflict of interest.
All claims expressed in this article are solely those of the authors and do not necessarily represent those of their affiliated organizations, or those of the publisher, the editors and the reviewers. Any product that may be evaluated in this article, or claim that may be made by its manufacturer, is not guaranteed or endorsed by the publisher.
The Supplementary Material for this article can be found online at: https://www.frontiersin.org/articles/10.3389/fmars.2024.1416359/full#supplementary-material
Supplementary Figure 1 | The correlation between Cryptophyta abundance and Ciliophora abundance fitted by Generalized additive model (GAM).
Bika H. M., Sunga W., De Ley P., Baldwinb J. G., Sharma J., Rocha-Olivaresd A., et al. (2012). Metagenetic community analysis of microbial eukaryotes illuminates biogeographic patterns in deep-sea and shallow water sediments. Mol. Ecol. 21, 1048–1059. doi: 10.5061/dryad.qm8f3
Brose U. (2008). Complex food webs prevent competitive exclusion among producer species. Proc. R. Soc. B: Biol. Sci. 275, 2507–2514. doi: 10.1098/rspb.2008.0718
Bruno D., Belmar O., Maire A., Morel A., Dumont B., Datry T. (2019). Structural and functional responses of invertebrate communities to climate change and flow regulation in alpine catchments. Glob Chang Biol. 25, 1612–1628. doi: 10.1111/gcb.14581
Bužančić M., Gladan Z. N., Marasović I., Kušpilić G., Grbec B. (2016). Eutrophication influence on phytoplankton community composition in three bays on the eastern adriatic coast. Oceanologia 58, 302–316. doi: 10.1016/j.oceano.2016.05.003
Caporaso J. G., Kuczynski J., Stombaugh J., Bittinger K., Bushman F. D., Costello E. K., et al. (2010). Qiime allows analysis of high-throughput community sequencing data. Nat. Methods 7, 335–336. doi: 10.1038/nmeth.f.303
Cardinale B. J., Matulich K. L., Hooper D. U., Byrnes J. E., Duffy E., Gamfeldt L., et al. (2011). The functional role of producer diversity in ecosystems. Am. J. Bot. 98, 572–592. doi: 10.3732/ajb.1000364
Castañeda R. A., Van Nynatten A., Crookes S., Ellender B. R., Heath D. D., MacIsaac H. J., et al. (2020). Detecting native freshwater fishes using novel non-invasive methods. Front. Environ. Sci. 8, 29. doi: 10.3389/fenvs.2020.00029
Chaffron S., Rehrauer H., Pernthaler J., von Mering C. (2010). A global network of coexisting microbes from environmental and whole-genome sequence data. Genome Res. 20, 947–959. doi: 10.1101/gr.104521.109
Chang C., Miki T., Ye H., Souissi S., Adrian R., Anneville O., et al. (2022). Causal networks of phytoplankton diversity and biomass are modulated by environmental context. Nat. Commun. 13, 1140. doi: 10.1038/s41467-022-28761-3
Chapin I. I. I., Zavaleta E. S., Eviner V. T., Naylor R. L., Vitousek P. M., Reynolds H. L., et al. (2000). Consequences of changing biodiversity. Nature 405, 234–242. doi: 10.1038/35012241
Chen N. T. (2014). Dynamic monitoring and analysis of planktonic algae in Meixi reach of lower Hanjiang River. J. China Urban Water Assoc. 1, 32–34 18. doi: 10.3969/j.issn.1002-8420.2014.01.010
Chen X. L., Chen G. J., Lu H. B., Liu X. D., Zhang H. C. (2015). Long-term diatom biodiversity responses to productivity in lakes of Fuxian and Dianchi. Biodiversity Sci. 23, 89–100. doi: 10.17520/biods.2014213
Chen J., Wang M., Li Y., Chesters D., Luo A., Zhang W., et al. (2022). Functional and phylogenetic relationships link predators to plant diversity via trophic and non-trophic pathways. R. Soc. 290, 20221658. doi: 10.6084/m9.figshare
D’Alelio D., Libralato S., Wyatt T., Ribera d’Alcalà M. (2016). Ecological-network models link diversity, structure and function in the plankton food-web. Sci. Rep. 6, 21806. doi: 10.1038/srep21806
Dee L. E., Allesina S., Bonn A., Eklöf A., Gaines S. D., Hines J., et al. (2017). Operationalizing network theory for ecosystem service assessments. Trends Ecol. Evol. 32, 118–130. doi: 10.1016/j.tree.2016.10.011
Deiner K., Bik H. M., Mächler E., Seymour M., Lacoursière Roussel A., Altermatt F., et al. (2017). Environmental dna metabarcoding: transforming how we survey animal and plant communities. Mol. Ecol. 26, 5872–5895. doi: 10.1111/mec.14350
DeSantis T. Z., Hugenholtz P., Larsen N., Rojas M., Brodie E. L., Keller K., et al. (2006). Greengenes, a chimera-checked 16S rRNA gene database and workbench compatible with ARB. Appl. Environ. Microbiol. 72, 5069–5072. doi: 10.1128/AEM.03006-05
Doi H., Chang K. H., Nishibe Y., Imai H., Nakano S. (2013). Lack of congruence in species diversity indices and community structures of planktonic groups based on local environmental factors. PloS One 8, e69594. doi: 10.1371/journal.pone.0069594
Duffy J. E., Cardinale B. J., France K. E., McIntyre P. B., Thébault E., Loreau M. (2007). The functional role of biodiversity in ecosystems: incorporating trophic complexity. Ecol. Lett. 10, 522–538. doi: 10.1111/j.1461-0248.2007.01037.x
Dunne J. A., Williams R. J., Martinez N. D. (2002). Biodiversity loss and ecological network structure. Ecol. Lett. 5, 558–567. doi: 10.1046/j.1461-0248.2002.00354.x
Edgar R. C. (2013). Uparse: highly accurate otu sequences from microbial amplicon reads. Nat. Methods 10, 996–998. doi: 10.1038/nmeth.2604
Faurby S., Funch P. (2011). Size is not everything: a meta-analysis of geographic variation in microscopic eukaryotes. Glob Ecol. Biogeogr. 20, 475–485. doi: 10.1111/j.1466-8238.2010.00609.x
Finderup N. T., Sand-Jensen K., Dornelas M., Bruun H. H. (2019). More is less: net gain in species richness, but biotic homogenization over 140 years. Ecol. Lett. 22, 1650–1657. doi: 10.1111/ele.13361
Fišer C., Robinson C. T., Malard F. (2018). Cryptic species as a window into the paradigm shift of the species concept. Mol. Ecol. 27, 613–635. doi: 10.1111/mec.14486
Fonseca V. G., Carvalho G. R., Nichols B., Quince C., Johnson H. F., Neill S. P., et al. (2014). Metagenetic analysis of patterns of distribution and diversity of marine meiobenthic eukaryotes. Glob Ecol. Biogeogr. 23, 1293–1302. doi: 10.1111/geb.12223
Fornoff F., Klein A., Thgen N. B., Staab M. (2019). Tree diversity increases robustness of multi-trophic interactions. R. Soc. 286, 20182399. doi: 10.6084/m9
Forster D., Qu Z., Pitsch G., Bruni E. P., Kammerlander B., Pröschold T., et al. (2021). Lake ecosystem robustness and resilience inferred from a climate-stressed protistan plankton network. Microorganisms 9, 549. doi: 10.3390/microorganisms9030549
Franzè G., Anderson S. I., Kling J. D., Wilburn P., Hutchins D. A., Litchman E., et al. (2023). Interactive effects of nutrients and temperature on herbivorous predation in a coastal plankton community. Limnol. Oceanogr. 68, S144–S157. doi: 10.1002/lno.12289
Fridley J. D. (2003). The influence of species diversity on ecosystem productivity: how, where, and why? Oikos 93, 514–526. doi: 10.1034/j.1600-0706.2001.930318.x
Gleason F. H., Küpper F. C., Amon J. P., Picard K., Gachon C. M. M., Marano A.V., et al. (2011). Zoosporic true fungi in marine ecosystems: a review. Mar. Freshw. Res. 62, 383–393. doi: 10.1071/MF10294
Goldberg C. S., Pilliod D. S., Arkle R. S., Waits L. P. (2011). Molecular detection of vertebrates in stream water: a demonstration using rocky mountain tailed frogs and idaho giant salamanders. PloS One 6, e22746. doi: 10.1371/journal.pone.0022746
Gu Y. G., Wang C. H., Lu S. H., Liang Y., Fu Y. H. (2008). Study on the horizontal distribution of biogenic silica and organic matter in the surface sediments from Eastern coastal areas of Guangdong province. J. Of Anhui Agric. Sci. 36, 13323–13325. doi: 10.3969/j.issn.0517-6611.2008.30.131
Harley C. D., Randall H. A., Hultgren K. M., Miner B. G., Sorte C. J., Thornber C. S., et al. (2006). The impacts of climate change in coastal marine systems. Ecol. Lett. 9, 228–241. doi: 10.1111/j.1461-0248.2005.00871.x
Hector A., Schmid B., Beierkuhnlein C., Caldeira M. C., Diemer M., Dimitrakopoulos P. G., et al. (1999). Plant diversity and productivity experiments in European grasslands. Science 286, 1123–1127. doi: 10.1126/science.286.5442.1123
Hooper D. U., Adair E. C., Cardinale B. J., Byrnes J. E. K., Hungate B. A., Matulich K. L., et al. (2012). A global synthesis reveals biodiversity loss as amajor driver of ecosystem change. Nature 486, 105–108. doi: 10.1038/nature11118
Jiang Y., He W., Liu W., Qin N., Ouyang H., Wang Q., et al. (2014). The seasonal and spatial variations of phytoplankton community and their correlation with environmental factors in a large eutrophic Chinese lake (Lake Chaohu). Ecol. Indic. 40, 58–67. doi: 10.1016/j.ecolind.2014.01.006
Jiang L., Pu Z. (2009). Different effects of species diversity on temporal stability in single - trophic and multitrophic communities. The American Naturalist. 174, 651–659. doi: 10.1086/605961
Johnson K. H., Vogt K. A., Clark H. J., Schmitz O. J., Vogt D. J. (1996). Biodiversity and the productivity and stability of ecosystems. Trends Ecol. Evol. 11, 372–377. doi: 10.1016/0169-5347(96)10040-9
Kara E. L., Hanson P. C., Hu Y. H., McMahon L. W. K. D. (2013). A decade of seasonal dynamics and co-occurrences within freshwater bacterioplankton communities from eutrophic Lake Mendota, WI, USA. Isme J. 7, 680–684. doi: 10.1038/ismej.2012.118
Käse L., Metfies K. A., Kraberg A. C., Neuhaus S., Meunier C., Wiltshire K., et al. (2021). Metabarcoding analysis suggests that flexible food web interactions in the eukaryotic plankton community are more common than specific predator–prey relationships at Helgoland roads, North Sea. Ices J. Mar. Sci. 78, 3372–3386. doi: 10.1093/icesjms/fsab058
Konopka A., Lindemann S., Fredrickson J. (2015). Dynamics in microbial communities: unraveling mechanisms to identify principles. Isme J. 9, 1488–1495. doi: 10.1038/ismej.2014.251
Kosiba J., Krztoń W. (2022). Insight into the role of cyanobacterial bloom in the trophic link between ciliates and predatory copepods. Hydrobiologia 849, 1195–1206. doi: 10.1007/s10750-021-04780-x
Kurtz Z. D., Muller C. L., Miraldi E. R., Littman D. R., Blaser M. J., Bonneau R. A., et al. (2015). Sparse and compositionally robust inference of microbial ecological networks. PloS Comput. Biol. 11, e1004226. doi: 10.1371/journal.pcbi.1004226
Landi P., Minoarivelo H. O., Brännström Å., Hui C., Dieckmann U. (2018). Complexity and stability of ecological networks: a review of the theory. Popul. Ecol. 60, 319–345. doi: 10.1007/s10144-018-0628-3
Li Y. L., Hu C. X. (2021c). Biogeographical patterns and mechanisms of microbial community assembly that underlie successional biocrusts across northern China. NPJ Biofilms Microbiomes 7, 15. doi: 10.1038/s41522-021-00188-6
Li F., Qin S., Zhang Y., Yang Z. (2023). Environmental dna metabarcoding reveals the impact of different land use on multitrophic biodiversity in riverine systems. Sci. Total Environ. 855, 158958. doi: 10.1016/j.scitotenv.2022.158958
Li H. D., Wu X. W., Xiao Z. S. (2021a). Assembly, ecosystem functions, and stability in species interaction networks. J. Plant Ecol. 45, 1049–1063. doi: 10.17521/cjpe.2019.0159
Li X. X., Yang W., Sun T., Cui B. S., Shao D. D. (2021b). The relationship between food web complexity and diversity in aquatic ecosystems. Acta ecologica Sin. 41, 3856–3864. doi: 10.5846/stxb202009192439
Li Z. Y., Ye X. Z., Wang S. P. (2021d). Ecosystem stability and its relationship with biodiversity. Chin. J. Plant Ecol. 45, 1127–1139. doi: 10.17521/cjpe.2020.0116
Lin X. Z., Hu Y. L., Wang R. X., Li D. M., Lin H. S., Cha G. C., et al. (2023). Phytoplankton community structure and water quality assessment in Chaozhou section of Hanjiang River, Guangdong Province. J. hydroecol. 44, 52–60. doi: 10.15928/j.1674-3075.202109280340
Loreau M., de Mazancourt C. (2013). Biodiversity and ecosystem stability: a synthesis of underlying mechanisms. Ecol. Lett. 16, 106–115. doi: 10.1111/ele.12073
Loschi M., Alelio D. D., Camatti E., BernardiAubry F., Beran A., Libralato S. (2023). Planktonic ecological networks support quantifcation of changes in ecosystem health and functioning. Sci. Rep. 13, 16683. doi: 10.1038/s41598-023-43738-y
Lynama C. P., Llopeb M., Möllmannd C., Helaouëte P., Bayliss-Brownf G. A., Stenseth N. C. (2017). Interaction between top down and bottom up control in marine food webs. Pnas 114, 1952–1957. doi: 10.1073/pnas.1621037114
Malfatti F., Francesca A. (2007). Microbial structuring of marine ecosystems. Nat. Rev. Microbiol. 5, 782–791. doi: 10.1038/nrmicro1747
Mei X., Gao S., Liu Y., Hu J., Razlustkij V., Rudstam L. G., et al. (2022). Effects of elevated temperature on resources competition of nutrient and light between benthic and planktonic algae. Front. Environ. Sci. 10, 908088. doi: 10.3389/fenvs.2022.908088
Morais J. V., Custódio A. L., Marques G. M. (2018). Calibration of parameters in dynamic energy budget models using direct-search methods. J. Math Biol. 78, 1439–1458. doi: 10.1007/s00285-018-1315-x
Naeem S., Li S. (1997). Biodiversityenhances ecosystem reliability. Nature 390, 507–509. doi: 10.1038/37348
Negrete-García G., Luo J. Y., Long M. C., Lindsay K., Levy M., Barton A. D. (2022). Plankton energy flows using a global size-structured and trait-based model. Prog. Oceanogr. 209, 102898. doi: 10.1016/j.pocean.2022.102898
Poisot T., Mouquet N., Gravel D. (2013). Trophic complementarity drives the biodiversity–ecosystem functioning relationship in food webs. Ecol. Lett. 16, 853–861. doi: 10.1111/ele.12118
Poste A. E., Hecky R. E., Guildford S. J. (2013). Phosphorus enrichment and carbon depletion contribute to high microcystis biomass and microcystin concentrations in Ugandan lakes. Limnol. Oceanogr. 58, 1075–1088. doi: 10.4319/lo.2013.58.3.1075
Pruesse E., Quast C., Knittel K., Fuchs B. M., Ludwig W., Peplies J., et al. (2007). SILVA: a comprehensive online resource for quality checked and aligned ribosomal RNA sequence data compatible with ARB. Nucleic Acids Res. 35, 7188–7196. doi: 10.1093/nar/gkm864
Ren Z., Ma K., Jia X., Wang Q., Zhang C., Li X. (2022). Community assembly and co-occurrence patterns of microeukaryotes in thermokarst lakes of the yellow river source area. Microorganisms 10, 481. doi: 10.3390/microorganisms10020481
Scherber C., Eisenhauer N., Weisser W. W., Schmid B., Voigt W., Fischer M., et al. (2010). Bottom-up effects of plant diversity on multitrophic interactions in a biodiversity experiment. Nature 468, 553–556. doi: 10.1038/nature09492
Schuldt A., Ebeling A., Kunz M., Staab M., Guimaraes-Steinicke C., Bachmann D., et al. (2019). Multiple plant diversity components drive consumer communities across ecosystems. Nat. Commun. 10, 1460. doi: 10.1038/s41467-019-09448-8
Sherr B., Sherr E., Caron D., Vaulot D., Worden A. (2007). Oceanic protists. Oceanogr. (Wash D C) 20, 130–134. doi: 10.5670/oceanog.2007.57
Sigsgaard E. E., Nielsen I. B., Carl H., Krag M. A., Knudsen S. W., Xing Y. C., et al. (2017). Seawater environmental dna reflects seasonality of a coastal fish community. Mar. Biol. 164, 128. doi: 10.1007/s00227-017-3147-4
Snelgrove P. V., Thrush S. F., Wall D. H., Norkko A. (2014). Real world biodiversity-ecosystem functioning: a seafloor perspective. Trends Ecol. Evol. 29, 398–405. doi: 10.1016/j.tree.2014.05.002
Sprong P. A. A., Fofonova V., Wiltshire K. H., Neuhaus S., Ludwichowski K. U., Käse L., et al. (2020). Spatial dynamics of eukaryotic microbial communities in the German bight. J. Sea Res. 163, 101914. doi: 10.1016/j.seares.2020.101914
Srichandan S., Baliarsingh S. K., Prakash S., Lotliker A. A., Parida C., Sahu K. C. (2019). Seasonal dynamics of phytoplankton in response to environmental variables in contrasting coastal ecosystems. Environ. Sci. pollut. Res. Int. 26, 12025–12041. doi: 10.1007/s11356-019-04569-5
Taberlet P., Coissac E., Mehrdad Hajibabaei L. H. R. (2012). Environmental dna. Mol. Ecol. doi: 10.1111/j.1365-294x.2012.05542.x
Thalinger B., Wolf E., Traugott M., Wanzenböck J. (2019). Monitoring spawning migrations of potamodromous fish species via edna. Sci. Rep. 9, 15388. doi: 10.1038/s41598-019-51398-0
Thomsen P. F., Willerslev E. (2015). Environmental dna – an emerging tool in conservation for monitoring past and present biodiversity. Biol. Conserv. 183, 4–18. doi: 10.1016/j.biocon.2014.11.019
Uusitalo L., Fleming-Lehtinen V., Hallfors H., Jaanus A., Hallfors S., London L. (2013). A novel approach for estimating phytoplankton biodiversity. Ices J. Mar. Sci. 70, 408–417. doi: 10.1093/icesjms/fss198
Wang M. Q., Li Y., Chesters D., Bruelheide H., Ma K., Guo P. F., et al. (2020). Host functional and phylogenetic composition rather than host diversity structure plant–herbivore networks. Mol. Ecol. 29, 2747–2762. doi: 10.1111/mec.15518
Wen T., Xie P., Yang S., Niu G., Liu X., Ding Z., et al. (2022). Ggclusternet: an r package for microbiome network analysis and modularity-based multiple network layouts. Imeta 1, e32. doi: 10.1002/imt2.32
Wu J. N., Zhu Z., Waniek J. J., Niu M. Y., Wang Y. T., Zhang Z. R., et al. (2023). The biogeography and co-occurrence network patterns of bacteria and microeukaryotes in the estuarine and coastal waters. Mar. Environ. Res. 184, 105873. doi: 10.1016/j.marenvres.2023.105873
Xiao W., Laws E. A., Xie Y., Wang L., Liu X., Chen J., et al. (2019). Responses of marine phytoplankton communities to environmental changes: new insights from a niche classification scheme. Water Res. 166, 115070. doi: 10.1016/j.watres.2019.115070
Xie X., He Z., Hu X., Yin H., Liu X., Yang Y. (2017). Large-scale seaweed cultivation diverges water and sediment microbial communities in the coast of nan’ao island, South China Sea. Sci. Total Environ. 598, 97–108. doi: 10.1016/j.scitotenv.2017.03.233
Xu J. D., Cai S. Z., Xuan L. L., Qiu Y., Zhou X. W., Zhu D. Y. (2014). Observational study on summertime upwelling in coastal seas between eastern Guangdong and southern Fujian. J. Trop. Oceanogr. 33, 1–9. doi: 10.3969/j.issn.1009-5470.2014.02.001
Yan L., Guo-Yong L., Jun-Peng M., Shu-Cun S. (2008). Effects of consumer diversity on food web structure and ecosystem functioning:current knowledge and perspectives. Acta Ecologica Sin. 28, 388–398. doi: 10.3321/j.issn:1000-0933.2008.01.045
Yang J., Zhang X. W., Jin X. W., Seymour M., Richter C., Logares R., et al. (2021). Recent advances in environmental dna- based biodiversity assessment and conservation. Divers. Distrib. 27, 1876–1879. doi: 10.1111/ddi.13415
Yang Q., Zhang P., Li X., Yang S. X., Chao X., Liu H. Q., et al. (2023). Distribution patterns and community assembly processes of eukaryotic microorganisms along an altitudinal gradient in the middle reaches of the yarlung Zangbo River. Water Res. 239, 120047. doi: 10.1016/j.watres.2023.120047
Yonatan Y., Guy A., Friedman J., Bashan A. (2021). Complexity-stability relationship in empirical microbial ecosystems. Biorxiv. doi: 10.1101/2021.07.29.454345
Zhang J. F., Chen W., Song Y. X., Zhou L. F., Yang Y., Yang H., et al. (2023b). Spatio-temporal variation of phytoplankton community structure and environmental driving factors in lower reaches of Yalong River. Resour. Environ. Yangtze Basin 32, 950–960. doi: 10.11870/cjlyzyyhj202305006
Zhang M., Duan H., Shi X., Yu Y., Kong F. (2012). Contributions of meteorology to the phenology of cyanobacterial blooms: implications for future climate change. Water Res. 46, 442–452. doi: 10.1016/j.watres.2011.11.013
Zhang Z., Li J., Li H., Wang L., Zhou Y., Li S., et al. (2023a). Environmental dna metabarcoding reveals the influence of human activities on microeukaryotic plankton along the chinese coastline. Water Res. 233, 119730. doi: 10.1016/j.watres.2023.119730
Zhang L., Yang J., Zhang Y., Shi J., Yu H., Zhang X. (2022). Edna biomonitoring revealed the ecological effects of water diversion projects between Yangtze River and Tai lake. Water Res. 210, 117994. doi: 10.1016/j.watres.2021.117994
Zhao Q., Van den Brink P. J., Carpentier C., Wang Y. X. G., Rodríguez Sánchez P., Xu C., et al. (2019). Horizontal and vertical diversity jointly shape food web stability against small and large perturbations. Ecol. Lett. 22, 1152–1162. doi: 10.1111/ele.13282
Zhao L., Zhang H., O’Gorman E. J., Tian W., Ma A., Moore J. C., et al. (2016). Weighting and indirect effects identify keystone species in food webs. Ecol. Lett. 19, 1032–1040. doi: 10.1111/ele.12638
Zhou-Yuan L., Xiao-Zhou Y., Shao-Peng W. (2021). Ecosystem stability and its relationship with biodiversity. Chin J Plant Ecol 45, 1127–1139. doi: 10.17521/cjpe.2020.0116
Keywords: environmental DNA, plankton community, subtropical marine ecologcial system, co-occurrence network, multitrophic levels, seasonal changes
Citation: Lin X, Li K, Zhao H, Gao Y, Zhang Z, Wang L, Wang X, Sanganyado E, Zheng Z, Gutang Q, Liu S, Li P, Yan X, Chen Z, Lin J and Liu W (2024) Seasonal changes of plankton community and its influencing factors in subtropical coastal marine areas revealed by eDNA-based network analysis. Front. Mar. Sci. 11:1416359. doi: 10.3389/fmars.2024.1416359
Received: 12 April 2024; Accepted: 07 August 2024;
Published: 05 September 2024.
Edited by:
Claudia J. Hernández-Guerrero, Centro Interdisciplinario de Ciencias Marinas, MexicoReviewed by:
Xiaoshou Liu, Ocean University of China, ChinaCopyright © 2024 Lin, Li, Zhao, Gao, Zhang, Wang, Wang, Sanganyado, Zheng, Gutang, Liu, Li, Yan, Chen, Lin and Liu. This is an open-access article distributed under the terms of the Creative Commons Attribution License (CC BY). The use, distribution or reproduction in other forums is permitted, provided the original author(s) and the copyright owner(s) are credited and that the original publication in this journal is cited, in accordance with accepted academic practice. No use, distribution or reproduction is permitted which does not comply with these terms.
*Correspondence: Wenhua Liu, d2hsaXVAc3R1LmVkdS5jbg==; Jianqing Lin, bGluamlhbnFpbmdAc3R1LmVkdS5jbg==
Disclaimer: All claims expressed in this article are solely those of the authors and do not necessarily represent those of their affiliated organizations, or those of the publisher, the editors and the reviewers. Any product that may be evaluated in this article or claim that may be made by its manufacturer is not guaranteed or endorsed by the publisher.
Research integrity at Frontiers
Learn more about the work of our research integrity team to safeguard the quality of each article we publish.