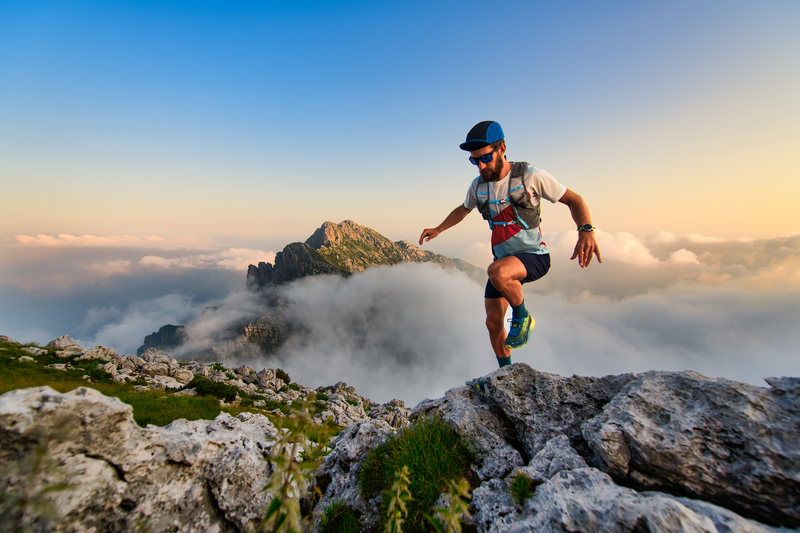
95% of researchers rate our articles as excellent or good
Learn more about the work of our research integrity team to safeguard the quality of each article we publish.
Find out more
ORIGINAL RESEARCH article
Front. Mar. Sci. , 17 September 2024
Sec. Marine Fisheries, Aquaculture and Living Resources
Volume 11 - 2024 | https://doi.org/10.3389/fmars.2024.1405303
Large-scale seaweed and shellfish aquaculture are increasingly being considered by policymakers as a source of food, animal feed and bioproducts for Europe. These aquacultures are generally thought to be low impact or even beneficial for marine ecosystems as they are ‘extractive’ – i.e., growing passively on foodstuff already available in seawater, and with potential habitat provision, fisheries, climate mitigation and eutrophication mitigation benefits. At some scale however, over-extraction of nutrients or chlorophyll could potentially have a negative effect on natural systems. Understanding the likely impacts of aquaculture production at scale is important to identify when safe limits are being approached. Taking seaweed aquaculture as the primary focus, this work uses operational oceanographic model outputs to drive prognostic growth models to predict the likely optimal distribution of seaweed farms across European waters to meet different production scenarios. A novel nutrient transport scheme is then used to model the interacting ‘footprints’ of nutrient drawdown from aquaculture facilities to demonstrate the likely spatial impact of large-scale aquaculture. Evaluation of both seaweed and shellfish contributions to CO2 balance under large scale production, and the potential impact on fisheries are also considered. The study finds that the impact of intensive seaweed aquaculture on nutrient availability could be significant where many farms are placed close together; but at the regional/basin scale even the highest level of production considered does not significantly impact total nutrient budgets. Seaweed aquaculture has the potential to extract large amounts of carbon dioxide, but the impact on carbon budgets depends on the end-use of the extracted seaweed. Shellfish aquaculture is a net source of CO2 due to the impact of calcification of shells on the carbonate system (i.e., alkalinity removal). However, gram-for-gram the CO2 impact of shellfish production is likely to be less than the impact of land-based meat production. Whilst operational oceanographic models are useful for taking a ‘broad brush’ approach to likely placement and impacts of aquaculture, reliable yield predictions for individual locations across European waters would require models integrating more physical and biogeochemical factors (wave environment, local currents, riverine inputs) at a finer scale than currently achievable.
Aquaculture plays a major role in developing strategies to address food security because it provides a relatively sustainable and renewable source of food (FAO, 2016). Global aquaculture production increased by 527% between 1990 and 2018 (FAO, 2016), however, Europe contributed very little to this increase (Gutiérrez et al., 2020). Marine aquaculture in Europe generated €2.73 billion in 2018 (Carvalho et al., 2018). Diadromous fishes are the species most produced in Europe, followed by molluscs, with macroalgae representing only 0.1% of the aquaculture production in Europe (Hough, 2022).
Seaweed and shellfish aquaculture is extractive, i.e. it removes naturally occurring food sources (inorganic nutrients, organic matter) from seawater, compared to finfish aquaculture which is maintained by addition of food (Buck et al., 2017). As such, both seaweed and shellfish aquaculture are generally considered to be low impact compared to caged fish farms and can in many cases have a net positive impact on the marine ecosystem via the provision of a range of ecosystem services. For example, macroalgae cultivation has the potential to mitigate climate change (Duarte et al., 2017) by absorbing CO2 from water. Its use as a CO2 removal mechanism for climate mitigation has been proposed (Lehahn et al., 2016; Moreira and Pires, 2016; Krause-Jensen et al., 2018), but largely depends on the fate of the seaweed grown i.e. most of the CO2 captured is released back into the atmosphere if the seaweed is eaten or ultimately decomposed. Seaweed also improves water quality (e.g., Neori et al., 1993), positively impacts biogeochemical cycling, primary production and food web dynamics (Ramus, 1992; Xie et al., 2017), provides nursery grounds and habitat for fish (e.g., Theuerkauf et al., 2022) and protects coasts and biodiversity (Sugumaran et al., 2022).
Shellfish can also act to remove excess nutrients via uptake of particulate organic nitrogen (Buck et al., 2017) and improve water clarity/quality (e.g., Capuzzo et al., 2015). However, shellfish are also typically a net source of CO2 to the atmosphere due to the effect of calcification during shell formation removing alkalinity and therefore reducing the capacity of the ocean to store CO2 (Li et al., 2021; Yang et al., 2021). However, any net negative impact on CO2 must be weighed against the impact of other protein production systems such as land-based animal sources.
Both shellfish and macroalgal farms can potentially have negative effects on the environment, in certain locations or levels of intensity (Campbell et al., 2019; Kelly et al., 2020). For example, the removal of nutrients or chlorophyll below the natural background level may impact natural macro- and micro-algal populations and/or the biogeochemistry or food webs of natural systems (Campbell et al., 2019). There is some evidence of nutrient depletion in areas of China where Saccharina japonica (formerly Laminaria japonica, Phaeophyceae) is cultured (UNDP/FAO, 1989). Removal of large amount of nutrients from the water reduces their availability to phytoplankton and other naturally occurring primary producers with consequent effects on the food chain. Therefore, it is important to consider potential impacts when developing new extractive aquaculture activities, particularly when there is a large scale of ambition.
The scale at which extractive aquaculture is likely to have negative impacts on the surrounding environment is not well understood. Aldridge et al. (2021) defined the level at which seaweed aquaculture could begin to impact local phytoplankton populations as the density of seaweed at which demand for nitrogen is the same for seaweed as for the natural phytoplankton population. Their modelling study in Strangford Loch (Northern Ireland) suggests that only at very large farm size (>10000 of 100m lines of S. latissima) would this criterion be approached. Abhilash et al. (2019) compared nutrient concentrations, pH, salinity and chlorophyll in areas surrounding a Kappaphycus alvarezii (Rhodophyta) farm in India, before during and after farm deployment and found no impact of the farm overall. Visch et al. (2020) investigated the environmental impact of Saccharina latissima (Phaeophyceae) farming in Sweden. The authors found that benthic oxygen flux, dissolved nutrient concentrations, and benthic mobile fauna did not differ between the area surrounding the farm and an area located at between 1.8 and 2.7 km from the farm (the control). Moreover, the authors found that the farm positively affected the benthic infauna and attracted pelagic mobile fauna. Other studies also find no or minimal impact of shellfish and seaweed farming on the surrounding marine environment (Zhang et al., 2009; Buschmann et al., 2014). However, as highlighted by the authors of these studies, the larger the scale of farming, the more likely are adverse impacts. As the scale of aquaculture in a region increases this could not only impact the natural marine environment but overall nutrient reduction could lead to impacts on farm productivity, both within and between farms.
Most of the seaweed farmed in the world are consumed as food (Sugumaran et al., 2022). They are also a source of phycocolloids such as agar, carrageenan and alginates (Chopin and Tacon, 2021); fibres and protein for livestock feed (Makkar et al., 2016); and compounds for pharmaceutical and cosmetic uses (Zhang and Wang, 2015; Couteau and Coiffard, 2016; Hitoe and Shimoda, 2017). The use of seaweed as a source of biofuel is also under investigation (Cassani et al., 2022) and there is potential for the use of seaweed for the production of biopolymers that can replace petroleum-based plastics (Farhan and Hani, 2017; Lim et al., 2021).
China is responsible for approximately 2/3 of global seaweed production (Zheng et al., 2019). In Europe, wild harvesting of seaweed has been the main source of macroalgal biomass to date (Buschmann et al., 2017). However, the European Commission is actively promoting production and consumption of seaweed in Europe. The EU Directorate General responsible for EU policy on maritime affairs and fisheries (DG MARE), is currently focusing on the development of an EU Algae Strategy. The aim of DG MARE is to identify areas where macroalgae cultivation can be used to contribute to the Green Deal. Moreover, the strategy will aim to exploit the potential of algae sector to contribute to the development of a sustainable European Blue Economy (Bermejo et al., 2022).
Global shellfish aquaculture has increased by 10-fold from 1985 to 2018. This industry generated an income of USD 104.55 billion in 2018 (Azra et al., 2021). Culture of bivalve molluscs in Europe represents an important commercial sector. It is estimated that 625 tonnes of shellfish were produced in Europe in 2017, generating EUR 1.26 B (EU, 2019). Mussels are the bivalves most farmed in Europe representing 35% of total aquaculture production, followed by oyster (7%) and clams (3%) (EU, 2019).
Production of filter-feeding species can contribute enormously to food security for the rapidly growing world population (FAO, 2016). These species require neither land nor fertilization and they are considered an important source of animal proteins with the potential, through species diversification, to improve nutrition (FAO, 2016). Shellfish are rich in proteins, essential amino acids, bioactive peptides, long-chain polyunsaturated fatty acids, astaxanthin, and other carotenoids and vitamin B12. Shellfish are also an important source of a minerals such as zinc, potassium, selenium and iodine (Venugopal and Gopakumar, 2017). The presence of other bioactive compounds in marine mussels have also been demonstrated and their use in non-food industries is currently under investigation (Grienke et al., 2014).
Shellfish aquaculture attends to two important UN Sustainable Development Goals: minimizing biodiversity loss and achieving sustainable food production (Blanchard et al., 2017). Shellfish aquaculture can create shelter and foraging ground for other marine organisms. Farmed shellfish can also promote the growth of wild shellfish with consequent secondary effect on coastal protection and biodiversity (Suplicy, 2020).
Extractive aquaculture in European waters has the potential to feed populations and provide raw materials and growth to the bioeconomy. Furthermore, transfer of production of food, feed, pharma and fuel from land to more sustainable production methods offshore has land-use and associated biodiversity benefits as well as the potential direct ecosystem services benefits outlined above. However, key questions have been identified within the policy community regarding the relative benefits vs disbenefits of large-scale aquaculture and in particular what level of productivity can be achieved before risks outweigh the gains.
Campbell et al. (2019) identify potential negative impacts of large-scale seaweed farming and key knowledge gaps. We focus here on nutrient extraction, one of the high-risk potential impacts identified in their study. The removal of nutrients has the potential to change community structure and ‘undermine’ the food chain (Campbell et al., 2019) and thereby impact on fin fisheries as well as the wider marine ecosystem. Likewise, particulate organic matter extraction by shellfish could conceivably have a similar effect. These direct effects of the extraction of food from the natural environment are difficult to mitigate against, other than by the strategy of targeting locations where eutrophication or hyper-nutrification artificially increase nutrient and phytoplankton levels and so extraction can be considered to be a beneficial consequence (Campbell et al., 2019). However, the degree to which productivity can be limited to low/positive impact locations whilst also meeting high-yield targets for seaweed and shellfish production is unknown. The potential impacts of both seaweed and shellfish aquaculture on carbon emissions when conducted at scale are also of key importance to policymakers.
The current state-of-the-art for broad-domain evaluation of seaweed or shellfish growth potential to inform policy and decision making is to use ‘suitability indices’. These rate coastal ocean areas on the basis of a number of measures of suitability and ultimately give each location a score to indicate potential suitability (e.g. Theuerkauf et al., 2019; Thomas et al., 2019). Such approaches are able to evaluate a wide range of factors - both environmental limits to growth (nutrients, temperature, flow rate), environmental technical constraints (water depth, wave height) and socio-economic factors (workforce availability, distance from land, competing uses etc.). What they cannot address is nutrient uptake, greenhouse gas balance or potential impact of nutrient uptake on fisheries or downstream aquaculture farms. To evaluate these quantitative effects, realistic spatially-resolved estimates of yield potential are needed.
The spatial and temporal scale of the potential direct impacts of nutrient extraction by macroalgae are governed by hydrodynamics and biogeochemistry. Transport and subsequent mixing of nutrient depleted water with unaffected water will act to restore nutrientconcentrations (defined here as ‘hydrodynamic nutrient restoration’), as willnitrogen cycling (‘biogeochemical nutrient restoration’). If the timescale of biogeochemical nutrient restoration is sufficiently slow under a given set of conditions, then we would expect mixing to dominate the spatial scale of impact. Conversely biogeochemistry might control this scale if it is relatively rapid, or if the hydrodynamic nutrient restoration is impaired by multiple other seaweed farms, for instance. This would be the same for any extracted substance which is subject to biogeochemical cycling e.g., dissolved inorganic carbon or chlorophyll. Whilst processes and rates might vary (dissolved inorganic carbon, for example, being subject to air-sea exchange of CO2), given a characteristic timescale it is in theory possible to compare biogeochemical and hydrodynamic restoration to determine the ultimate mitigator of an extraction signal resulting from a farm.
The effect of alkalinity removal by shellfish growth, however, cannot be rebalanced biogeochemically or at any scale of dilution. One mole of alkalinity is removed from the ocean for every mole of calcium carbonate precipitated and thus less CO2 can be taken up by the ocean (by a factor dependent on buffering capacity of the carbonate system) (Frankignoulle et al., 1994). The only way to restore the system to its previous state is to add back a mole of alkalinity (e.g., by dissolving a mole of CaCO3). Therefore, the argument that is sometimes made that the alkalinity removal effect of shellfish can be mitigated by their uptake of inorganic carbon or via DIC uptake by downstream seaweed farms (integrated multitrophic aquaculture) is false. Whilst the local effect on CO2 uptake might be mitigated by DIC management (e.g., Li et al., 2021), the effect of alkalinity addition is global.
This paper focusses specifically on the European region, its aim being to contribute to the evidence base for European policymakers regarding the potential for extractive aquaculture in European seas to meet food and other needs of the European population, environment, and economy without leading to unacceptable negative effects. Specifically, it aims to answer questions over the possible impacts of large-scale extractive aquaculture at particular yield targets, on nutrients, carbon emissions and fisheries.
There are few examples of aquaculture yield prediction over wide spatial areas in the literature, beyond broad extrapolations from simple assumptions. Typically, mechanistic or statistical modelling-based predictions of potential yields have only been made in localised areas e.g., embayments or limited areas of open coast e.g (Broch et al., 2019; Aldridge et al., 2021); or in a few cases at the sub-basin scale (Van Der Molen et al., 2018; Kotta et al., 2022). One study does present an analysis of potential yield of macroalgae globally, by application of a mechanistic model to geospatial environmental data (Lehahn et al., 2016). While a predictive, mechanistic modelling approach at this scale has significant uncertainty associated, not least due to the challenges of validation, it is nonetheless a potentially valuable tool in evaluating potential yields and impacts of aquaculture at the continental scale.
In order to be i) tractable in terms of human- and computer-resource use, ii) consistent across the European domain and iii) reproducible and easily updated; yield modelling would ideally be driven by operational data. In this case, operational means geospatial data that is complete (i.e., no missing data), validated and updated automatically. Furthermore, potential yields must be predictable by a generalised model i.e., not relying on local adjustments or knowledge to ‘tweak’ the model. Minimum viable models are needed, with the fewest input data requirements. Simple growth models which can represent multiple species through modifying parameter values will maximise the robustness of the results.
In this study we use published seaweed and shellfish growth models to predict potential extractive aquaculture yields in European waters and a nutrient transport scheme to investigate the impacts of large-scale seaweed farming on local nutrient concentrations and regional nutrient budgets with particular focus on potential fisheries impacts.
The specific objectives of the study are:
- To develop a computationally efficient geospatial analysis framework for predicting seaweed and shellfish aquaculture potential yields and impacts using operational oceanographic model output.
- To use yield predictions to allocate seaweed or shellfish farms to meet high-yield targets for European extractive aquaculture production.
- To quantify the impact of nutrient uptake by seaweed farms on local and regional nutrient concentrations and provide an estimate of how productivity of ‘downstream’ seaweed farms might be impacted by reduced nutrient availability in the large-scale seaweed aquaculture scenarios.
- To quantify the direct impact on CO2 emissions of extractive seaweed and shellfish aquaculture.
- To outline the steps needed to improve qualitative and quantitative estimates of biomass yield, farm interactions and impacts achieved by this approach.
The seaweed growth model (Section 3.2) takes as input temperature, current velocities, nitrate, ammonium, phosphate, and PAR fields. Current velocity fields are also used in the advection model. The shellfish model (Section 3.3) requires chlorophyll-a, current velocities and temperature.
All data except the PAR (Photosynthetically Available Radiation) were sourced from the Copernicus Marine Service (CMEMS - https://resources.marine.copernicus.eu/products), the data used originating from large-scale hydrodynamic and coupled biogeochemical model runs which are run operationally by various modelling centres around Europe and the latest validated data made available through CMEMS. Henceforth we will refer to these downloaded model outputs as ‘CMEMS model outputs’ for brevity. PAR was downloaded from the OceanColor data provided by NASA (https://oceancolor.gsfc.nasa.gov/l3). Daily data were used for all the forcing parameters, except for PAR, for which month-averaged data were used, due to data availability.
CMEMS model output data are provided by areas (Arctic, Baltic Sea, Northwest Shelf, Iberia-Biscay-Ireland, Mediterranean Sea and Black Sea). Data extraction and growth model runs were conducted separately for each area. For all the areas except the Baltic Sea we used analysis and forecast 2021 data, as this was the most commonly available year across all areas. During the runs on the Baltic Sea we used reanalysis 2020 data. The analysis and forecast model outputs for the Baltic were found to have unrealistically high nutrient concentration and were therefore not useable. Reanalysis data was only available up to 2020. Full details of the originating models are provided in the Supplementary Material Table 1.1.
Macroalgal growth was modelled using a modified version of the multi-species growth model presented by Hadley et al., (2015). The model currency is nitrogen and the key state variables of the model are related to the quantity of seaweed nitrogen per unit volume of seawater. Seaweed in the model is able to uptake nutrient nitrogen into an internal store from where it is fixed into new biomass, rate limited by nutrient availability, temperature and light. Full details of model equations and setup are presented in Section 3 of the Supplementary Material. Alternative, more complex models using frond area to quantify seaweed growth and nutrient interactions are commonly used (e.g. Broch and Slagstad, 2012) and may be more refined than the per-unit-volume approach but present significant challenges in terms of additional complexity, which is undesirable when applying a model over a wide spatial domain as in this work.
Applying the model to a large seaweed farm requires accounting for the quantity of nutrient taken up within the farm (i.e., where productivity is high, uptake in one part of the farm may affect the nutrient availability downstream in the farm). In order to make the model computationally tractable when running across the whole of European waters, the whole farm is considered as a single box in which the model operates, with parameterisations of water flow, nutrient availability and spacing between notional lines of seaweed accounting for average nutrient availability within the farm (See Section 3 of the Supplementary Information).
The present study focuses on three commonly farmed species of seaweed: Saccharina latissima, Alaria esculenta (both brown algae) and the green species Ulva lactuca. S. latissima was chosen because it is the most cultivated species in Europe and also the most studied (e.g. Bermejo et al., 2022). A. esculenta, also commonly farmed and studied, was included as an alternative kelp species that can be grown successfully at lower nutrient concentrations and colder temperatures than S. latissima (Buggeln, 1978). U. lactuca was selected for its tolerance to a broad range of temperature and nutrient concentrations. This ubiquitous species is mostly farmed in land-based facilities but lately its potential for near-shore or even deep ocean cultivation is gaining increasing attention (Lehahn et al., 2016; Steinhagen et al., 2021). Parameter values for each species were selected from a synthesis of literature values and are presented in Tables 4–6 of Section 3 of the Supplementary Material.
Tuning of the model was undertaken using time-series data for the growth of A. esculenta and S. latissima in Bantry Bay (IDREEM project, Pers. Comm.). Details are provided in section 3.2.7 of the Supplementary Material.
Validation was conducted by comparison of predicted yields vs published yields for seaweed farms of the same species. Data on yield was compiled from the literature, identifying European sites where one or more of the 3 species have been grown and sufficient ancillary is data available to reproduce the conditions appropriately. In particular, the deployment and harvest month were considered of greatest importance for validating yield, along with initial biomass (where available) and farm-specific information (cultivation depth, farm dimensions etc.), which were used to modify the model runs to best represent the farm data. Table 1 summarises the validation data used.
This exercise is a holistic validation of input data, growth model structure and parameterisation and as such divergence between modelled and observed yields is to be expected. Overall, however, the performance of the model is remarkably good, as demonstrated in Figure 1. Most of the available data are for S. latissima, but performance appears reasonable across species and is sufficient for the aims of this work. There is an issue with underperformance of the model when driven with Northwestern Shelf (NWS) model output data from 2021 (Figure 1). This leads to the underprediction of potential yields in this region in the analysis below. This affects the distribution of seaweed farms in the scenarios subsequently presented, because farms are placed in highest productivity locations, so other regions are favoured; however it does not quantitatively affect the conclusions drawn in this paper.
Figure 1. Results of validation exercise for macroalgal farm model. Error bars represent range of yield values in observed data, where applicable. Yield is presented per metre of rope.
Shellfish growth and harvest yield was modelled using an adapted version of the multi-species dynamic energy budget model presented by Hawkins et al., (2013), known as ShellSIM. The model balances energy inputs and outputs to yield net growth based on allocation between shell and soft tissue. Growth of reproductive biomass and subsequent spawning is modelled explicitly in the model. The model is an individual-based model so a simple population model was implemented to scale up to unit volume of seawater, which introduces mortality when there is insufficient food to support the extant population (described in Section 3 of the Supplementary Material). Parameter values for the Pacific Oyster, Crassotrea gigas and the Blue Mussel, Mytilus Edulis are taken from the source paper (Hawkins et al., 2013). The model is used to evaluate potential impacts on CO2 emissions from large-scale shellfish aquaculture and does not consider farm-farm interactions.
It was not possible to find suitable data to perform a robust validation of the model. Given that ShellSIM has previously been tuned and validated for the species of interest we are confident that the model results used to calculate the CO2 balance of shellfish aquaculture are sufficiently robust to have confidence in the conclusions drawn. Optimised spatial distributions and yields at any particular location derived from this analysis should however be considered uncertain.
To run simulations that account for the impact of farms on the concentration of nutrients, and subsequently on downstream farms, a model framework was set up to represent advection of the nutrient deficit resulting from uptake by seaweed farms. A coupled solution re-running the large scale hydrodynamic-biogeochemical models with multiple farm placement scenarios was not computationally tractable, nor practicable for rapid and repeatable exploration of farm placement options. Instead, we developed a post-processing scheme that models transport of nutrient impacts from farms using hydrodynamic outputs from the CMEMS model outputs. Given the complexity of implementing and running such a scheme in 3 dimensions we selected to implement the model as 2-dimensional lateral advection scheme. Vertical mixing is addressed through the restoration timescale parameter, τ (defined below).
We define, for each parameter of interest, the concentrations over time and space (Equation 1):
Where Cr (“reference”) is the concentration of a parameter (nitrate or ammonium) in the CMEMS model output; Cf (“farms”) is the total concentration of the same parameter if farms were present, and Cd (“difference”) is the difference between the two, representing the impact that farms have on their environment. We refer to Cd as the “deficit” because for Nitrate its value will always be negative and for ammonium almost always negative (in occasional cases net nitrogen uptake but net ammonium production is observed in the model).
The input data Cr was originally computed by the large scale hydrodynamic-biogeochemical models by solving numerically the advection diffusion equation (Equation 2):
With U being the current fields obtained from the physical model, and σ being the biogeochemical terms of the equation.
In the presence of farms, the total concentration Cf would follow the same equation, but with a new biogeochemical term є(Cf) added, which corresponds to the sources and sinks of the modeled parameter that are introduced by the farms:
In the absence of any aquaculture farms, є=0 and Equation 3 is equivalent to Equation 2. We assume that the natural biogeochemical cycle as modelled by the CMEMS models is not significantly altered by the presence of farms i.e. σ(Cf)≈ σ(Cr), then by linearity of the advection-diffusion equation we have (Equation 4):
We then simplify the equation to only model the 2D advection (neglecting diffusion) in a well-mixed surface layer. Under such strong simplifications and as we do not use the same grid, time step, or numerical scheme as CMEMS model outputs or the underlying models, our advection model will not fit precisely with CMEMS. To compensate, we introduce an attenuation (henceforth ‘restoration’) term which represents how, away from farms, the concentration of each parameter returns to its base value Cr, which is equivalent to Cd returning to 0. This return to the baseline represents the combined effect of the biogeochemical nutrient restoration and vertical mixing processes, which are not otherwise modelled in our equations. We represent this term as an exponential decay with a time constant τ. The 2D equation that we solved is finally (Equation 5):
This equation was numerically solved on the grid of CMEMS’ physical model outputs, restricted to one surface layer, with a Euler-upwind scheme. The time step duration was chosen at each time step to ensure that the Courant–Friedrichs–Lewy condition (Courant et al., 1967) was at most 0.9 and that the nutrient concentrations in farms never dropped by more than 90% in one time step (to avoid negative values), whichever required a shorter time step. In practice, the CFL requirement was much more restrictive than the requirement for the biogeochemical term.
The water current fields u and v obtained from CMEMS model output data and averaged over the considered upper layer are available as an average value in each grid cell. In order to use u and v in the Euler-upwind scheme, we needed values estimated at cell interfaces that also respected an incompressibility constraint. Full details of how this was achieved are available in the Supplementary Material (Section 5). In summary, we want to find the u and v values at interfaces of grid cells that are as close as possible to the arithmetic mean of the two adjacent cell values while respecting the zero-divergence constraint. This is a constrained optimization problem that we demonstrate to be analytically equivalent to a linear equation system, which is numerically solved for each day of data.
The farms modify nutrient concentration in a surface layer of uniform thickness, related to Zfarm, the depth of the algae farm. And we define the upper layer thickness h1 as:
It is assumed that the upper layer of thickness Zfarm is mixed under the effect of the momentum fluxes at the air-sea interface (wind, waves, tide) and the oscillatory movements of the algae fronds. A turbulent mixing length lm is introduced to simulate the turbulent mixing closed to the bottom of the farming layer and caused by the presence of the algae layer.
Numerous models based on algebraic relations of lm exist (Prandtl, 1932; Blackadar, 1962; Tsanis, 1989). Following Stocking et al. (2016), we write: lm = k.z (k is the Von Karman constant, k ≈0.4).
Thus (Equation 7):
Vertical fluxes of water - and consequently, nutrients - between the considered surface layer and underlying layers, are not directly computed in order to keep computation rapid. Instead, we considered that vertical dilution of the signal is represented in the restoration term, τ, of Equation 5.
τ, the nutrient restoration term in the nutrient deficit transport scheme is used to represent both vertical mixing with underlying waters, which will tend to dilute the signal, and biogeochemical nutrient restoration (i.e. the rebalancing of the nutrient concentration by the natural system via nitrogen cycling).
Seaweed lines tend to be deployed over winter and most growth and harvest happens in early spring. During this time, shallow shelf seas tend to be well mixed (i.e., no seasonal thermocline) and mixing tends to be relatively rapid due to wind and wave conditions. Typically, mixing throughout the water column in a well-mixed layer driven by tidal and wind-driven mixing is unlikely to be greater than a week under normal winter conditions, although this is dependent on both water column structure and wind regimes as well as the total depth of the water column (e.g., Rippeth, 2005). A simple restoration term is a coarse approximation to the vertical mixing and therefore we take the conservative assumption that dilution of the deficit to undetectable levels by vertical mixing will happen on a timescale of between 1 and 2 weeks i.e. ~10 days.
A host of processes will tend to act against any perturbation to the nitrogen cycle; for example, where nitrogen is removed from the surface waters, the ratio of uptake to remineralisation of inorganic nitrogen will be reduced leading to net remineralisation (Johnson et al., 2007); and in shallow waters the release from underlying sediments will increase when overlying nutrient concentrations are reduced (e.g. Couceiro et al., 2013; Hull et al., 2020). The characteristic attenuation timescale of this biogeochemical nutrient restoration is likely to be different for different nitrogen species, and again sensitive to local physical and topographic conditions. The turnover of ammonium in the surface ocean is commonly on the timescale of a few days (Johnson et al., 2007, 2013) whereas that of Nitrate is typically on a scale of 10-100 days (e.g., Weston et al., 2004).
A reasonable representative value for τ that integrates vertical mixing and nitrogen cycle response is therefore taken to be 10 days. The sensitivity of the nutrient transport model to this key term is investigated below.
Where a large value of nutrient deficit (Cd) is propagated by the nutrient transport scheme into relatively low-nutrient-concentration water, the resulting deficit can be larger than the modelled ambient concentration of nutrient. Therefore, some cells can present apparent negative values of total nutrient concentrations. These negative values are dissipated over time in the model by the restoration term as well as by numerical diffusion, or by further advection of the large deficit to areas with greater ambient concentration values. The seaweed model is set up to stop the nutrient uptake in any cell where the total concentration value is zero or negative. Whilst negative concentrations are clearly not physically realistic, the benefit of this approach is that mass is conserved in the nutrient transport scheme. Negative values (or apparent >100% nutrient utilization values) should be interpreted as being representative of locations of extremely high nutrient pressure, where the model cannot meaningfully predict the degree of nutrient uptake or the resulting nutrient concentration. These areas require further study to fully understand the impact of intensive aquaculture on local nutrient concentrations.
In an example run with high production (IBI area, S. latissima, target production of 10MT of fresh weight), at the end date of the simulation, 29% of all grid cells affected by farm nutrient uptake (i.e. non-zero values of Cd) had negative values of nitrate but these values are relatively small compared to typical positive values of nitrate: less than 2% of grid cells had a value of nitrate lower than -0.01 mg m-3. Largely then, grid squares where Cd >= Cr are locations where there is high pressure on nutrient availability due to surrounding seaweed farms and near zero nutrient concentrations would be expected under intensive aquaculture locally.
Yield scenarios were run for seaweed and shellfish species separately. All whole-domain runs are summarised in Table 2. In the case of seaweed, 2 methods (A, B) were used to estimate farm yields. In A, each farm is treated separately and independently from all other farms, driven by unmodified nutrient fields from CMEMS model outputs. A therefore represents the potential yield of a farm in any grid square in isolation from any other farms. In B, run for the seaweed model only, farms are placed in high-productivity regions to meet target yield scenarios and the impact of these farms on nutrient fields is estimated using the nutrient transport model, allowing for the bidirectional interaction of nutrient drawdown and yield between any number of farms placed across the region.
For the target yield scenarios (1,2,5,10 Mt fresh weight), each species is considered in isolation, and farms of that species are placed, using scenario A farm locations, starting with the highest yield square and allocating farms in order of decreasing yield until the total yield target is met. Initially, farms were only placed where the total water column depth was less than 30 m, to keep to realistic present-day engineering limitations to farm deployment. For U. lactuta, farms had to be allowed up to 100 m water depth to achieve the 10 Mt yield. To minimize the nutrient impact between farms, the minimum distance between them was set initially at 10 km, and subsequently reduced to 5 km where yield at 10 km spacing was insufficient to meet the scenario target. This allocation of farms is then used in the B runs to investigate interacting nutrient impacts.
Seaweeds, as primary producers, fix carbon from dissolved CO2 into organic matter. This uptake in the water leads to a roughly equivalent quantity of CO2 being taken up from the atmosphere. This local uptake may be negated by the release of CO2 where the harvested seaweed is eaten or otherwise respired. Seaweeds have been proposed as a source of refractory organic matter to sediments and the dissolved organic carbon pool in seawater (Duarte et al., 2017; Legge et al., 2020), which would provide an addition carbon sink during seaweed cultivation. However, the fraction of macroalgal growth that is converted to this pool of carbon is highly uncertain, and possibly zero, so we do not consider this process here and therefore provide a lower estimate of carbon uptake. Local CO2 uptake is calculated from the harvested yield of seaweed (Equation 8):
Where Nf is the per-unit area macroalgal fixed N harvested from a farm, MWN is the molar weight of Nitrogen (=14 g/mol), MWCO2 is the molar weight of CO2 (=44 g/mol) and C:NMA is the molar C:N ratio of macroalgal biomass (see SM for details and source of values). Higher C:N ratio algae are responsible for greater CO2 uptake per unit fresh weight, but less nutrient uptake.
Shellfish accumulate carbon in their shells and also in their biomass. However, as heterotrophs they are net respirers of organic matter and further the process of calcification removes alkalinity from the ocean and therefore drives CO2 emission to the atmosphere. It is fair to assume that most or all the respired organic matter is readily bioavailable and would be respired by other organisms in the natural system if the shellfish were not present. Therefore, we do not consider the respiration of organic matter by shellfish as a net release of CO2. The net effect on CO2 of producing a given quantity of shellfish (expressed as dry soft tissue weight in the model) is therefore:
Where
and
In Equations 9–11, DSTW is dry soft tissue weight and DSHW is dry shell weight (state variables of the shellfish model, see Supplementary Info). C:DSTWSF is the carbon to dry soft tissue ratio of shellfish (assumed to be constant at 0.45), BF is the buffer factor in seawater which controls the response of the carbonate system to a change in alkalinity and represents the fraction of CO2 uptake/release in seawater due to alkalinity change that is emitted to the atmosphere.
Potential seaweed fresh weight yield for S. latissima, U. lactuca and A. esculenta are presented in Figure 2. These results represent the potential yield in each location assuming no influence of reduced nutrient from other farms ‘upstream’ i.e. the farm in each grid square is considered in isolation (Scenario A in Table 2).
Figure 2. Scenario A macroalgal farm potential yields across Europe for (A) S. Latissima; (B) A Esculenta and (C) U. Lactuta using default species and farm parameters (see Supplementary Material Section 3) Units of kg/m2 represents yield in a hypothetical 1x1 km farm where 40% of the area is farmed, the remaining 60% is unfarmed to allow for access, shipping and other uses of the area. Similar ‘scenario A’ maps for the shellfish species considered are provided in the Supplementary Material (Section 6).
There is broadly good agreement between the locations of near-coast high yield locations identified in the model and those areas with substantial active offshore aquaculture production (e.g., NW France, Portuguese and Spanish Atlantic coast for multiple seaweed species and Mediterranean river plumes (for U. lactuta).
There are substantial inter-species differences in both gross yield and distributions of high yield areas under Scenario A. These are due to the differing physiological properties of the 3 species, represented in the parameter sets for each species. For example, A. esculenta has lower nutrient affinity (i.e., it is able to uptake nutrients more rapidly at lower concentration) than the other two species and can thrive at lower temperatures and lower light levels. There is therefore higher potential yield in the North Sea and Norwegian coast/Arctic predicted for A. esculenta than the other species.
Note that for all species, there are clear discontinuities in model yield at CMEMS model output domain boundaries. These are due to discontinuities in the data from the CMEMS model output for different regions, due to structural and tuning differences between models run for different domains. This reflects a key challenge with utilising operational model outputs to resolve and predict potential aquaculture yields.
The nutrient deficit propagation model is used to investigate the impact of a single farm on local nutrient fields and the sensitivity of this to τ, the nutrient restoration timescale, representing both the biogeochemical restoration and vertical mixing out of the surface layer. Figure 3 presents the analysis of a single seaweed farm located in Bantry Bay, Ireland; Figure 4 the same for the Atlantic coast of Portugal. The largest nutrient deficit observed in each grid square during the growth period is presented, with values masked below 1.4 mg (N) m-3 (approximately 1% of winter ambient concentration) for nitrate and 0.3 mg (N) m-3 (approximately 10% of typical ambient concentration) for ammonium. Given the much faster biogeochemical turnover of ammonium these different cutoff values are selected to give comparable areas of influence, beyond which we can be confident of near-zero impacts on natural systems.
Figure 3. Nutrient footprint of a 1x1 km S. latissima farm located in Bantry Bay Ireland, showing the nutrient deficit propagated downstream, in units of mg (N) m-3. Each panel is a composite image showing the greatest month-averaged nutrient deficit predicted in each square over the 8 months growth period. Data only plotted where deficit is >1.4 mg m-3 (nitrate) or >0.3 mg m-3 (ammonium).
Figure 4. Nutrient footprint of a 1x1 km S. Latissima farm located off the Portuguese coast, showing the nutrient deficit propagated downstream, in units of mg (N) m-3. Each panel is a composite image of the greatest nutrient deficit observed in monthly data over the 8 months growth period. Data only plotted where deficit is >1.4 mg m-3 (nitrate) or >0.3 mg m-3 (ammonium).
As expected, the value of τ is found to be important in the size of footprint and the magnitude of the impacts in grid squares surrounding the farm. In the ‘advection only’ panels in Figures 3 and 4 the situation where only horizontal mixing dilutes the signal away with no restoration signal (τ = ∞) is presented, showing a wide area of influence, although with the most intense nutrient deficit dying away exponentially with distance from the farm. Even under this unrealistic regime of no biogeochemical restoration or vertical mixing out of the very surface layer, the impact of an individual large seaweed farm on ambient nutrient concentrations is relatively minor.
Our best estimate of restoration rate, τ, of the nutrient deficit signal, with a characteristic timescale of 10 days gives a footprint in Bantry Bay, Ireland of 5-10 km. However, in the higher-flow environment of the Portuguese coastal system, the spatial extent of the footprint is considerably greater – extending 50-100 km north and south from the farm located close to the coast at the latitude of Porto, and about 10 km in total east-west. Footprints of a similar size to those at Porto were found for a farm placed at the opening to the Ria de Betanzos in NW Spain (data not shown), a similarly high-flow environment. It is important to note that much of the area of influence, particularly in the high-flow environment, is only impacted to a very minor degree, with nutrient deficits of only a few percent of ambient concentration for nitrate or little over 10% of typical ambient for ammonium, which tends to be at much lower concentrations overall.
Therefore we can conclude that the spatial scale of significant modifications to nutrients (i.e. at a scale likely to have a measurable although not necessarily large or negative effect on natural ecosystems) downstream of an individual seaweed farm is likely to be confined to a 1-5 km area around the farm in most cases. The impact of multiple farms in close proximity could of course have a larger impact, although in most cases, where high productivity seaweed aquaculture is feasible it will be in regions of elevated nutrient concentrations due to anthropogenic activity, where large-scale removal of excess nutrients is likely to be net-beneficial to the natural environment.
In order to address the question of the degree to which farm-farm interaction could affect total yield, nutrient transport model (Scenario B) runs were undertaken with farms placed to target yields of 1,2,5 and 10 Mt, as described in Methods, with τ=10 days. The total yield of farms in each basin and for the whole domain in B runs and the percentage of the yield achieved by the same farms in A (no nutrient interactions) are presented in Table 3. Unsurprisingly, the percentage of the maximum (Scenario A) yield achieved in B runs decreases as the number of interacting farms increases, with a relatively steep drop-off between 5 and 10 Mt scenarios (Table 3, Figure 5). Results are presented for S. latissima but similar results were found with other macroalgal species.
Figure 5. Percentage of target yield achieved when nutrient interactions are included decreases rapidly as yield passes 5MT.
The data presented in Figure 5 is indicative of the inhibition of yield in large-scale intensive aquaculture across Europe as farm density reaches levels at which nutrient extraction begins to become significant. It is not possible from this analysis to predict the level at which returns from additional farms diminish to an unsustainable level (i.e., the total maximum viable yield for Europe) for the following reasons: firstly, the distribution of farms is uneven across the domain. By allocating farms based purely on highest potential yield, they are inevitably placed close together – thereby maximising the possibility of nutrient drawdown lowering overall yield. In these yield scenarios no farms are placed in Arctic or NWS model regions due to predicted lower productivity and few farms are located in the Baltic or Black seas. Secondly, we restrict farm placement to shallow water (<30m in most cases – see methods section). Therefore, with a wider spread of farms, particularly if deep water farms were permitted in the runs, a much higher total yield could likely be achieved without significant inhibition of yield by nutrient loss, albeit with a lower average productivity per individual farm.
At higher seaweed farm density, the compound effect of nutrient extraction will mean that mixing processes in surface waters will be increasingly ineffective in diluting away the nutrient deficit signal. Vertical mixing and biogeochemical nutrient restoration processes will become increasingly important in determining the timescale and therefore spatial impact in a region. Here we focus on the IBI region, which was the most productive in our scenarios (Table 3).
Figure 6 presents the maximum percentage reduction of the ambient surface nutrient concentration observed at each pixel of the IBI region throughout the growth period. A value of 100 represents the removal of all of the available nutrient during at least 1 month of the growing period. Substantial areas in these plots, particularly in the 10 Mt scenario, appear to have completely exhausted the nutrients in some areas of the coastal seas, particularly off the coast of North Africa and in the English Channel. This is an artefact of the deficit transport model as discussed in the methods section – in reality farms will only be able to extract nutrient to the point at which they are uncompetitive with microalgae or their uptake is slowed by their nutrient uptake affinity (i.e., kinetic limitation at low nutrient concentration). Values of apparent 100% nutrient utilisation indicate where the model has broken down due to extremely high nutrient pressure (see methods section). Thus, this highlights regions of potentially substantial pressure on natural algal populations and the wider ecosystem in these areas. In order to fully quantify the magnitude of this effect a more refined model is required in future studies.
Figure 6. Percentage nutrient reduction calculated from nutrient deficit signal and ambient (CMEMS model output) nutrient concentration in each grid square for farm placement in the IBI region under the (left) 1Mt and (right) 10Mt target yield scenarios for S. latissima. Colour scale cuts off at less than 1%.
The total N extraction by region is shown in Table 4. The nutrient deficit model conserves mass and therefore these regional-scale values are correct in spite of local scale artefacts (above). Considering the potential impact of such large-scale seaweed aquaculture on nutrient concentrations at a regional/basin scale, we take 3 illustrative examples:
1) The nitrogen flow into the North Sea from the English Channel is estimated to be 170 kt (N) yr-1 (Brion et al., 2004). This is ten times the nitrogen extracted across the whole IBI region by seaweed farms in the 10 Mt yield scenario.
2) The Iberian upwelling system provides a supply of new water along the Portuguese coast of between 0.3 and 6 Sv (Coelho et al., 2002; Huthnance et al., 2002)). Taking an estimated year-round average of 2 Sv for on-off shelf exchange and a modest deep water nitrate concentration of 10 µM (140 mg m-3), the total nitrogen supply to the Portuguese coastal system is estimated to be 2x106 m3s-1 x 31.536x106 (s y-1) x 140 x 10-12 kt (N) m-3 = 8830 kt (N) yr-1. Therefore, for the IBI region the basin-scale N budget is many orders of magnitude larger than the nitrogen extraction due to large-scale aquaculture at the 1-10 Mt scale (2 to 17 kt (N), Table 4). This is even before accounting for river input and atmospheric deposition.
3) For the Mediterranean Sea, inflow of water (and therefore nutrients) from the Atlantic is a relatively small term. A nitrogen budget integrating riverine, atmospheric and other inputs to the Mediterranean basin estimated a total N budget of 1.67 +/- 0.55 Mt (N) yr-1 (Strobl et al., 2008), vastly greater than the 1.9 kt of N extracted from the Mediterranean Sea in the 10 Mt yield scenario.
Thus, we can confidently conclude that the impact of aquaculture at this scale will not significantly impact nutrient budgets at the basin scale.
This analysis demonstrates that regional-scale impacts of nutrient extractions due to large-scale seaweed aquaculture are likely to be small, whereas local impacts are potentially large where areas of the sea are intensively farmed. To avoid over-exploitation of nutrients it is important to focus seaweed farms in areas of hyper-nutrification to avoid undermining food chain and maximise net beneficial impacts. This also highlights the importance of nutrient monitoring in areas of intensive seaweed aquaculture.
Table 5. lists the net effect of yield targets on CO2 emissions calculated for the seaweed S. latissima, and the shellfish M. edulis (Blue Mussel) and C. gigas (Pacific Oyster). This analysis demonstrates that, as expected, extraction of seaweed biomass results in a net uptake of CO2 from the atmosphere. This potentially contributes to climate change mitigation, depending on the fate of the extracted seaweed. If the seaweed is eaten or otherwise processed for extraction of particular biomolecules, the majority or the entirety of the fixed carbon is likely to relatively rapidly end up returned to the atmosphere through respiration by consumers, or decomposition of waste material. However, if the seaweed is harvested directly for carbon storage (e.g. for biochar), or the waste materials left over from biomolecule extraction are processed in such a way to store the carbon long-term, then a proportion of the macroalgal CO2 uptake could potentially be counted as long term storage (and therefore contribute to mitigation of atmospheric CO2 i.e. negative emissions).
Table 5. Summary of impacts on net uptake of CO2 in target yield scenarios for S. latissimi, blue mussel, M. edulis and Pacific oyster, C. gigas.
Also as expected, the net effect of shellfish production is to drive emission of CO2 from (or inhibit uptake by) the ocean. As discussed above this is a global effect that can only be ameliorated by adding more (carbonate) alkalinity to the ocean. Grinding up and dissolution of waste shells after shellfish consumption and adding back to the ocean is one approach to partially mitigate the negative effects of shellfish production on ocean acidification and CO2 balance (Morris et al., 2019).
It is interesting to note the large difference in magnitude of impact on CO2 between M. edulis and C. gigas. The reason for this is the substantial difference (~a factor of 4) in the ratio of shell to tissue weight in the two organisms. C. gigas has a much higher ratio (more shell produced per unit mass of food) and therefore the effects of calcification are much greater for a given total weight yield (and the edible proportion much smaller).
The CO2 impacts of shellfish aquaculture cannot be considered in isolation and must be weighed against the costs of protein production on land. For example, 10 Mt of C. gigas will produce roughly 0.5 Mt of Oyster meat (Mazón-Suástegui et al., 2018), for a net CO2 emission of 2.7 Mt of CO2 (Table 5). The mass ratio of animal meat production to CO2 emissions varies from 6kg CO2/kg meat for poultry to 60kg/kg for Beef (Poore and Nemecek, 2018). Therefore, an equivalent amount of 0.5Mt of chicken and beef will result in 3 and 30 Mt CO2. This is not an entirely fair comparison as we are not accounting for farming and extraction related emissions in the shellfish production, nor the production of strong greenhouse gases. Nonetheless, it appears that, if choosing less shell-heavy organisms than oysters to grow in aquaculture facilities there is a net carbon as well as land use gain from switching diets from land-based meat to shellfish.
Large scale extractive aquaculture has potential benefits, from mitigation of carbon emissions and ocean acidification to nutrient removal and reducing pressure on land use. However, it is not without risks of negative impacts, and these must be considered carefully and quantified where possible. This paper provides an initial estimate of the likely impacts of extractive aquaculture on nutrients and carbon emissions. A target extraction yield of 10 Mt of seaweed, if limited to near-shore, shallow sea locations, could have significant local impacts on nutrient concentrations, potentially impacting local natural seaweed communities and phytoplankton community structure, particularly when many farms are placed close together (i.e., within 5-10 km of each other). The larger and more intensive the farms, the greater the spacing between farms will need to be to minimise impacts under any given set of conditions.
Targeting extractive aquaculture to ameliorate eutrophication or hyper-nutrification from runoff maximises the benefit and minimises the risk of negative impacts caused by undermining the food chain. Spreading extractive aquaculture out into deeper waters would reduce impacts for a given target yield. Interspersing seaweed farms with shellfish aquaculture (to remineralize nutrients) would likely provide some mitigation of over-extraction of nutrients and could usefully be investigated in a future study.
At the wider scale, the production of 10 Mt of seaweed or shellfish (the largest amount considered in this study) distributed around European waters has only a marginal effect on regional nutrient budgets so the larger scale impacts on e.g. fisheries or natural populations of algae and natural carbon uptake by the ocean is likely to be minimal. There is inevitably some level of yield target, however, at which regional scale impacts would be seen. As aquaculture activities intensify in European waters it is essential to increase monitoring efforts for chlorophyll, nutrients and the response of the natural ecosystem to ensure negative effects are identified and mitigated at the earliest possible stage.
We have presented a novel modelling approach to predicting the capacity for large scale seaweed aquaculture, accounting for nutrient interactions between farms, without the need to run coupled aquaculture – biogeochemistry – 3D transport models. This represents a first effort towards such a framework, which already yields useful results and provides valuable insights as presented. However, further refinements will be necessary before this approach could be used as a policy or planning tool.
Specifically, the nutrient deficit restoration term, τ, is a workaround for two unrelated issues – firstly the lack of vertical dilution of the nutrient deficit signal and secondly the mitigation of the nutrient deficit through biogeochemical cycling. In a future version of the model, separation of these two restoration terms and improved constraint on their values should be attempted. Ideally, spatially variable restoration terms based on physical and biogeochemical outputs from the CMEMS model data would be implemented. Such improved restoration terms would be likely to mitigate somewhat the complex interactions between nutrient deficits and spatially variable concentrations leading to zero or negative apparent nutrient concentrations. Nevertheless, this phenomenon will always be a possibility within the framework, where intensive aquaculture scenarios are explored. Better constraint of the nutrient restoration terms will improve the utility of zero or negative concentrations in the model as a red flag for over-intensive aquaculture. Furthermore, a future version of the model might incorporate the potential to place shellfish farms and seaweed farms in the same model run to facilitate nutrient regeneration scenarios, thus increasing potential yield.
Using operational oceanographic model output to predict potential yields comes with significant challenges. For example, there are discontinuities visible in the potential yield maps in Figure 2. These are due to the differing input data from CMEMS for the different regions. Different models are used in the different regions, with differing tunings and sometimes with structural differences. Therefore, it is possible to get substantially differing yield predictions for the same location arising from two different CMEMS model outputs.
This is particularly the case in near-shore locations where river influence is most important. In a comparison of the yield predictions driven by the CMEMS NWS and IBI regional model outputs, the near-coast behaviour is quite different. This is partly due to model resolution but largely due to the riverine inputs being used to drive the different models. Figure 7 compares modelled nitrate concentrations in the same section of the coast of SW Ireland and clearly shows the signal of riverine inputs to Bantry Bay in IBI but absent in NWS data. Furthermore, there is a different intensity of nutrient input further north, from the Shannon estuary. While the seaweed yield predictions for Bantry bay are good in the seaweed model when driven by IBI inputs, they substantially underpredict when driven by NWS data, due to the absence of nutrients. In Kenmare Bay, the next embayment north from Bantry Bay, yield predictions are similar between NWS and IBI, but both significantly underpredict the true seaweed yield due to the absence of riverine input to Kenmare Bay in both models.
Figure 7. Annual mean modelled nitrate concentration in CMEMS model output for (left) NWS regional model and (right) IBI regional model.
Riverine inputs used to drive regional scale operational oceanographic and biogeochemical models are poorly documented and have previously been recognised as a significant shortcoming of European operational modelling strategy (Capet et al., 2020) and the differences between salinity fields due to river inputs to different regional models and from different forcing datasets has been recently highlighted (Sotillo et al., 2021). When evaluating the potential for aquaculture, which is typically undertaken near to coasts for both practical (access, shelter) and operational (availability of high nutrient concentrations from river inputs) reasons, these shortcomings and differences between models are significant impediments to consistent and representative estimates of near-shore production potential, whatever yield modelling or suitability index approach is used. This is a key area of improvement needed in operational models to allow better near-shore productivity estimates.
A further challenge to such modelling effort is the applicability of complex seaweed and shellfish growth models across wide spatial domains covering a broad range of environmental forcing values. Growth models are generally tuned for a particular locale to make predictive evaluation of yield potential. This corrects the models for i) the traits of local organisms of interest; ii) the role of all real-world environmental forcings not considered by the model and iii) details of local aquaculture practices, conventions and equipment set-up. None of these factors can be accounted for at a large geographic scale and therefore, whilst the yield predictions are useful for indicative distribution of farms in high-yield scenarios of European aquaculture, site-specific estimates of yield could not be relied upon for e.g. precise optimal location of a farm or economic modelling of farm viability, without local tuning of the growth models and potentially additional input data.
Therefore, the analyses presented here should be revisited as European operational oceanographic model outputs improve and converge over the coming years. The application of these models to yield predictions for specific locations could only be operationalised with a new generation of adaptive models incorporating local parameter variability.
The datasets presented in this study can be found in online repositories. The names of the repository/repositories and accession number(s) can be found below: https://github.com/ARGANS/shellfish_and_algae-MODEL.
MJo: Conceptualization, Formal Analysis, Investigation, Methodology, Software, Validation, Visualization, Writing – original draft, Writing – review & editing, Funding acquisition. QJ: Conceptualization, Data curation, Formal Analysis, Investigation, Methodology, Project administration, Resources, Software, Validation, Visualization, Writing – original draft, Writing – review & editing, Funding acquisition. MJa: Formal Analysis, Methodology, Software, Validation, Visualization, Writing – original draft, Writing – review & editing. NM: Methodology, Software, Visualization, Writing – original draft, Writing – review & editing. MB: Writing – original draft, Writing – review & editing. CG: Conceptualization, Funding acquisition, Investigation, Methodology, Writing – original draft, Writing – review & editing. DM: Conceptualization, Investigation, Methodology, Visualization, Writing – original draft, Writing – review & editing. SP: Conceptualization, Investigation, Methodology, Writing – original draft, Writing – review & editing. JM: Conceptualization, Funding acquisition, Investigation, Methodology, Project administration, Supervision, Writing – original draft, Writing – review & editing. AM: Conceptualization, Data curation, Funding acquisition, Investigation, Methodology, Project administration, Software, Supervision, Writing – original draft, Writing – review & editing. PB: Conceptualization, Data curation, Formal Analysis, Funding acquisition, Investigation, Methodology, Project administration, Software, Supervision, Validation, Visualization, Writing – original draft, Writing – review & editing.
The author(s) declare financial support was received for the research, authorship, and/or publication of this article. This work was funded by the Executive Agency for Small and Medium-sized Enterprises (EASME) the European Climate Infrastructure and Environment Executive Agency (CINEA) and the Directorate-General for Maritime Affairs and Fisheries (DG MARE) to support the European Green Deal: Lot 1 Shellfish and algae (EMFF/2020/1.3.1.16), acting under the powers delegated by the European Commission.
Authors MJo, DM, SP, and JM were employed by Bantry Marine Research Station, Gearhies, Co.
Author MJo was employed by Ecodiversity Ltd., Schull, Co.
Authors QJ, MJa, NM, AM, and PB were employed by ACRI-ST.
Authors QJ, MJa, NM, and PB were employed by ARGANS, Ltd.
Authors MB and CG were employed by Cofrepeche.
Author SP was employed by AquaBioTech Group.
All claims expressed in this article are solely those of the authors and do not necessarily represent those of their affiliated organizations, or those of the publisher, the editors and the reviewers. Any product that may be evaluated in this article, or claim that may be made by its manufacturer, is not guaranteed or endorsed by the publisher.
The Supplementary Material for this article can be found online at: https://www.frontiersin.org/articles/10.3389/fmars.2024.1405303/full#supplementary-material
Aldridge J. N., Mooney K., Dabrowski T., Capuzzo E. (2021). Modelling effects of seaweed aquaculture on phytoplankton and mussel production. Application to Strangford Lough (Northern Ireland). Aquaculture 536, 736400. doi: 10.1016/j.aquaculture.2021.736400
Azra M. N., Okomoda V. T., Tabatabaei M., Hassan M., Ikhwanuddin M. (2021). The contributions of shellfish aquaculture to global food security: assessing its characteristics from a future food perspective. Front. Mar. Sci. 8. doi: 10.3389/FMARS.2021.654897/BIBTEX
Bermejo R., Buschmann A., Capuzzo E., Cottier-Cook E., Fricke A., Hernández I., et al. (2022). State of knowledge regarding the potential of macroalgae cultivation in providing climate-related and other ecosystem services. Report prepared by an Eklipse Working Group. ISBN: 978-3-944280-28-8
Blackadar A. K. (1962). The vertical distribution of wind and turbulent exchange in a neutral atmosphere. J. Geophysical Res. 67 (8), 3095–3102.
Blanchard J. L., Watson R. A., Fulton E. A., Cottrell R. S., Nash K. L., Bryndum-Buchholz A., et al. (2017). Linked sustainability challenges and trade-offs among fisheries, aquaculture and agriculture. Nat. Ecol. Evol. 1, 1240–1249. doi: 10.1038/s41559-017-0258-8
Boderskov T., Nielsen M. M., Rasmussen M. B., Balsby T. J. S., Macleod A., Holdt S. L., et al. (2021). Effects of seeding method, timing and site selection on the production and quality of sugar kelp, Saccharina latissima: A Danish case study. Algal. Res. 53, 102160. doi: 10.1016/j.algal.2020.102160
Brion N., Baeyens W., de Galan S., Elskens M., Laane R. W. P. M. (2004). The North Sea: source or sink for nitrogen and phosphorus to the Atlantic Ocean? Biogeochemistry 68, 3. doi: 10.1023/B:BIOG.0000031041.38663.aa
Broch O. J., Alver M. O., Bekkby T., Gundersen H., Forbord S., Handå A., et al. (2019). The kelp cultivation potential in coastal and offshore regions of Norway. Front. Mar. Sci. 5. doi: 10.3389/fmars.2018.00529
Broch O. J., Slagstad D. (2012). Modelling seasonal growth and composition of the kelp Saccharina latissima. J. Appl. Phycol. 24, 759–776. doi: 10.1007/s10811-011-9695-y
Buck B. H., Nevejan N., Wille M., Chambers M. D., Chopin T. (2017). “Offshore and multi-use aquaculture with extractive species: Seaweeds and bivalves,” in Buck B., Langan R. eds. Aquaculture Perspective of Multi-Use Sites in the Open Ocean: The Untapped Potential for Marine Resources in the Anthropocene. Springer, Cham, 23–69. doi: 10.1007/978-3-319-51159-7_2
Buggeln R. G. (1978). Physiological investigations on Alaria esculenta (Laminariales, Phaeophyceae). IV. Inorganic and Organic nitrogen in the blade. J. Phycol. 14, 156–160. doi: 10.1111/j.1529-8817.1978.tb02441.x
Buschmann A. H., Camus C., Infante J., Neori A., Israel Á., Hernández-González M. C., et al. (2017). Seaweed production: overview of the global state of exploitation, farming and emerging research activity. Eur. J. Phycol. 52, 391–406. doi: 10.1080/09670262.2017.1365175
Buschmann A. H., Prescott S., Potin P., Faugeron S., Vásquez J. A., Camus C., et al. (2014). The status of kelp exploitation and marine agronomy, with emphasis on macrocystis pyrifera, in chile. Adv. Botanical Res. 71, 161–188. doi: 10.1016/B978-0-12-408062-1.00006-8
Campbell I., Macleod A., Sahlmann C., Neves L., Funderud J., Øverland M., et al. (2019). The environmental risks associated with the development of seaweed farming in Europe - prioritizing key knowledge gaps. Front. Mar. Sci. 6. doi: 10.3389/FMARS.2019.00107/BIBTEX
Capet A., Fernández V., She J., Dabrowski T., Umgiesser G., Staneva J., et al. (2020). Operational modeling capacity in european seas–an EuroGOOS perspective and recommendations for improvement. Front. Mar. Sci. 7. doi: 10.3389/FMARS.2020.00129
Capuzzo E., Stephens D., Silva T., Barry J., Forster R. M. (2015). Decrease in water clarity of the southern and central North Sea during the 20th century. Global Change Biol. 21, 2206–2214. doi: 10.1111/gcb.12854
Cassani L., Lourenço-Lopes C., Barral-Martinez M., Chamorro F., Garcia-Perez P., Simal-Gandara J., et al. (2022). Thermochemical characterization of eight seaweed species and evaluation of their potential use as an alternative for biofuel production and source of bioactive compounds. Int. J. Mol. Sci. 23, 2355. doi: 10.3390/ijms23042355
Chopin T., Tacon A. G. J. (2021). Importance of seaweeds and extractive species in global aquaculture production. Rev. Fish. Sci. Aquacult. 29, 139–148. doi: 10.1080/23308249.2020.1810626
Coelho H. S., Neves R. J. J., White M., Leitão P. C., Santos A. J. (2002). A model for ocean circulation on the Iberian coast. J. Mar. Syst. 32, 153–179. doi: 10.1016/S0924-7963(02)00032-5
Couceiro F., Fones G. R., Thompson C. E. L., Statham P. J., Sivyer D. B., Parker R., et al. (2013). Impact of resuspension of cohesive sediments at the Oyster Grounds (North Sea) on nutrient exchange across the sediment-water interface. Biogeochemistry 113, 37–52. doi: 10.1007/s10533-012-9710-7
Courant R., Friedrichs K., Lewy H. (1967). On the partial difference equations of mathematical physics. IBM J. Res. Dev. 11, 215–234. doi: 10.1147/rd.112.0215
Couteau C., Coiffard L. (2016). Seaweed application in cosmetics. In Seaweed in health and disease prevention. Fleurence J., Levine I. (Eds). Academic Press. p. 423–441. doi: 10.1016/B978-0-12-802772-1.00014-2
Duarte C. M., Wu J., Xiao X., Bruhn A., Krause-Jensen D. (2017). Can seaweed farming play a role in climate change mitigation and adaptation? Front. Mar. Sci. 4. doi: 10.3389/fmars.2017.00100
FAO (2016). The state of world fisheries and aquaculture 2016. Contributing to food security and nutrition for all. FAO, Rome.
Farhan A., Hani N. M. (2017). Characterization of edible packaging films based on semi-refined kappa-carrageenan plasticized with glycerol and sorbitol. Food Hydrocolloids 64, 48–58. doi: 10.1016/j.foodhyd.2016.10.034
Forbord S., Steinhovden K. B., Solvang T., Handå A., Skjermo J. (2020). Effect of seeding methods and hatchery periods on sea cultivation of Saccharina latissima (Phaeophyceae): a Norwegian case study. J. Appl. Phycol. 32, 2201–2212. doi: 10.1007/S10811-019-01936-0/FIGURES/10
Frankignoulle M., Canon C., Gattuso J.-P. (1994). Marine calcification as a source of carbon dioxide: Positive feedback of increasing atmospheric CO2. Limnol. Oceanogr. 39, 458–462. doi: 10.4319/lo.1994.39.2.0458
Grienke U., Silke J., Tasdemir D. (2014). Bioactive compounds from marine mussels and their effects on human health. Food Chem. 142, 48–60. doi: 10.1016/j.foodchem.2013.07.027
Gutiérrez E., Lozano S., Guillén J. (2020). Efficiency data analysis in EU aquaculture production. Aquaculture 520, 734962. doi: 10.1016/j.aquaculture.2020.734962
Hadley S., Wild-Allen K., Johnson C., Macleod C. (2015). Modeling macroalgae growth and nutrient dynamics for integrated multi-trophic aquaculture. J. Appl. Phycol. 27, 901–916. doi: 10.1007/s10811-014-0370-y
Hawkins A. J. S., Pascoe P. L., Parry H., Brinsley M., Black K. D., McGonigle C., et al. (2013). Shellsim: A generic model of growth and environmental effects validated across contrasting habitats in bivalve shellfish 32, 2, 237–253. doi: 10.2983/035.032.0201
Hitoe S., Shimoda H. (2017). Seaweed fucoxanthin supplementation improves obesity parameters in mildly obese Japanese subjects. Funct. Foods Health Dis. 7, 246–262. doi: 10.31989/2160-3855
Hough C. (2022). Regional review on status and trends in aquaculture development in Europe - 2020. FAO Fisheries and Aquaculture Circular No. 1232/1. Rome: FAO. doi: 10.4060/cb7809en
Hull T., Johnson M., Greenwood N., Kaiser J. (2020). Bottom mixed layer oxygen dynamics in the Celtic Sea. Biogeochemistry 149, 263–289. doi: 10.1007/s10533-020-00662-x
Huthnance J. M., Van Aken H. M., White M., Barton E. D., Le Cann B., Coelho E. F., et al. (2002). Ocean margin exchange—water flux estimates. J. Mar. Syst. 32, 107–137. doi: 10.1016/S0924-7963(02)00034-9
Johnson M. T., Greenwood N., Sivyer D. B., Thomson M., Reeve A., Weston K., et al. (2013). Characterising the seasonal cycle of dissolved organic nitrogen using Cefas SmartBuoy high-resolution time-series samples from the southern North Sea. Biogeochemistry 113, 23–36. doi: 10.1007/S10533-012-9738-8/FIGURES/4
Johnson M., Sanders R., Avgoustidi V., Lucas M., Brown L., Hansell D., et al. (2007). Ammonium accumulation during a silicate-limited diatom bloom indicates the potential for ammonia emission events. Mar. Chem. 106, 63–75. doi: 10.1016/j.marchem.2006.09.006
Kelly E. L. A., Cannon A. L., Smith J. E. (2020). Environmental impacts and implications of tropical carrageenophyte seaweed farming. Conserv. Biol. 34, 326–337. doi: 10.1111/cobi.13462
Kerrison P. D., Innes M., Macleod A., Mccormick E., Elbourne P. D., Stanley M. S., et al. (2020). Comparing the effectiveness of twine and binderseeding in the Laminariales species Alaria esculenta and Saccharina latissima. J. Appl. Phycol. 32, 2173–2181. doi: 10.1007/s10811-020-02069-5
Kotta J., Raudsepp U., Szava-Kovats R., Aps R., Armoskaite A., Barda I., et al. (2022). Assessing the potential for sea-based macroalgae cultivation and its application for nutrient removal in the Baltic Sea. Sci. Total Environ. 839, 156230. doi: 10.1016/j.scitotenv.2022.156230
Krause-Jensen D., Lavery P., Serrano O., Marba N., Masque P., Duarte C. M. (2018). Sequestration of macroalgal carbon: The elephant in the Blue Carbon room. Biol. Lett. 14, 20180236. doi: 10.1098/rsbl.2018.0236
Lehahn Y., Ingle K. N., Golberg A. (2016). Global potential of offshore and shallow waters macroalgal biorefineries to provide for food, chemicals and energy: feasibility and sustainability. Algal. Res. 17, 150–160. doi: 10.1016/j.algal.2016.03.031
Legge O., Johnson M., Hicks N., Jickells T., Diesing M., Aldridge J., et al. (2020). Carbon on the northwest european shelf: Contemporary budget and future influences. Front. Mar. Sci. 7, 472547. doi: 10.3389/FMARS.2020.00143
Li J., Zhang W., Ding J., Xue S., Huo E., Ma Z., et al. (2021). Effect of large-scale kelp and bivalve farming on seawater carbonate system variations in the semi-enclosed Sanggou Bay. Sci. Total Environ. 753, 142065. doi: 10.1016/j.scitotenv.2020.142065
Lim C., Yusoff S., Ng C. G., Lim P. E., Ching Y. C. (2021). Bioplastic made from seaweed polysaccharides with green production methods. J. Environ. Chem. Eng. 9, 105895. doi: 10.1016/j.jece.2021.105895
Makkar H. P. S., Tran G., Heuzé V., Giger-Reverdin S., Lessire M., Lebas F., et al. (2016). Seaweeds for livestock diets: A review. Anim. Feed Sci. Technol. 212, 1–17. doi: 10.1016/j.anifeedsci.2015.09.018
Mazón-Suástegui J. M., Betanzos-Vega A., Capetillo-Piñar N., Latisnere-Barragán H., Ortiz-Cornejo N. L. (2018). Oyster production and meat yield in Crassostrea spp. (bivalvia: Ostreidae) in Pinar del Rio, Cuba. Ecosistemas y Recursos Agropecuarios 5, 501–510. doi: 10.19136/era.a5n15.1241
Monteiro J. P., Melo T., Skjermo J., Forbord S., Broch O. J., Domingues P., et al. (2021). Effect of harvesting month and proximity to fish farm sea cages on the lipid profile of cultivated Saccharina latissima. Algal. Res. 54, 102201. doi: 10.1016/j.algal.2021.102201
Moreira D., Pires J. C. M. (2016). Atmospheric CO2 capture by algae: Negative carbon dioxide emission path. Biores. Technol. 215, 371–379. doi: 10.1016/j.biortech.2016.03.060
Morris J. P., Backeljau T., Chapelle G. (2019). Shells from aquaculture: a valuable biomaterial, not a nuisance waste product. Rev. Aquacult. 11, 42–57. doi: 10.1111/raq.12225
Neori A., Ellner S. P., Boyd C. E., Krom M. D. (1993). The integration of seaweed biofilters with intensive fish ponds to improve water quality and recapture nutrients. In: Constructed Wetlands for Water Quality Improvement. Boca Raton, Florida: CRC Press, 603–607. doi: 10.1201/9781003069997-77
Peteiro C., Freire Ó. (2013). Biomass yield and morphological features of the seaweed Saccharina latissima cultivated at two different sites in a coastal bay in the Atlantic coast of Spain. J. Appl. Phycol. 25, 205–213. doi: 10.1007/S10811-012-9854-9/FIGURES/5
Peteiro C., Sánchez N., Dueñas-Liaño C., Martínez B. (2014). Open-sea cultivation by transplanting young fronds of the kelp Saccharina latissima. J. Appl. Phycol. 26, 519–528. doi: 10.1007/S10811-013-0096-2/TABLES/3
Poore J., Nemecek T. (2018). Reducing food’s environmental impacts through producers and consumers. Science 360, 987–992. doi: 10.1126/science.aaq0216
Prandtl L. (1932). Meteorogische an wendung der stromungslehre. Beiträge zur Physik der Atmosphäre 19, 188–202.
Ramus J. (1992). “Productivity of seaweeds,” in Falkowski P. G., Woodhead A. D., Vivirito K. eds. Primary productivity and biogeochemical cycles in the sea. Environmental Science Research vol 43. (Boston, MA: Springer).
Rippeth T. P. (2005). Mixing in seasonally stratified shelf seas: A shifting paradigm. Philos Trans A Math Phys Eng Sci. 363, 2837–2854. doi: 10.1098/rsta.2005.1662
Sotillo M. G., Campuzano F., Guihou K., Lorente P., Olmedo E., Matulka A., et al. (2021). River freshwater contribution in operational ocean models along the european atlantic façade: Impact of a new river discharge forcing data on the CMEMS IBI regional model solution. J. Mar. Sci. Eng. 9 (4), 401. doi: 10.3390/JMSE9040401
Carvalho N., Nielsen R., Guillen J. (2018). Economic report of the EU aquaculture sector (STECF-18-19), European Commission, Joint Research Centre, Scientific, Technical and Economic Committee for Fisheries, EU Publications Office, Luxembourg. https://data.europa.eu/doi/10.2760/45076.
Steinhagen S., Enge S., Larsson K., Olsson J., Nylund G. M., Albers E., et al. (2021). Sustainable large-scale aquaculture of the northern hemisphere sea lettuce, ulva fenestrata, in an off-shore seafarm. J. Mar. Sci. Eng. 9, 615. doi: 10.3390/jmse9060615
Stocking J. B., Rippe J. P., Reidenbach M. A. (2016). Structure and dynamics of turbulent boundary layer flow over healthy and algae-covered corals. Coral Reefs 35, 1047–1059.
Strobl R., Evans B., Somma F., Garcia G. E., Stips A., Zaldivar C. J. (2008). Feasibility study of the application of the LOICZ budget to the Mediterranean sea. Joint Research Centre Scientific and Technical Reports, Joint Research Centre Institute for Environment and Sustainability, Luxembourg. doi: 10.2788/80138
Sugumaran R., Padam B. S., Yong W. T. L., Saallah S., Ahmed K., Yusof N. A. (2022). A retrospective review of global commercial seaweed production-current challenges, biosecurity and mitigation measures and prospects. Int. J. Environ. Res. Public Health 19 (12), 7087. doi: 10.3390/ijerph19127087
Suplicy F. M. (2020). A review of the multiple benefits of mussel farming. Rev. Aquacult. 12, 204–223. doi: 10.1111/raq.12313
Theuerkauf S. J., Barrett L. T., Alleway H. K., Costa-Pierce B. A., St. Gelais A., Jones R. C. (2022). Habitat value of bivalve shellfish and seaweed aquaculture for fish and invertebrates: Pathways, synthesis and next steps. Rev. Aquacult. 14, 54–72. doi: 10.1111/raq.12584
Theuerkauf S. J., Morris J. A., Waters T. J., Wickliffe L. C., Alleway H. K., Jones R. C. (2019). A global spatial analysis reveals where marine aquaculture can benefit nature and people. PloS One 14, e0222282. doi: 10.1371/journal.pone.0222282
Thomas J. B. E., Ramos F. S., Gröndahl F. (2019). Identifying suitable sites for macroalgae cultivation on the Swedish west coast. Coast. Manage. 47, 88–106. doi: 10.1080/08920753.2019.1540906
Tsanis I. K. (1989). Simulation of wind-induced water currents. J. Hydraulic Eng. 115 (8), 1113–1134.
UNDP / FAO. (1989). Culture of Kelp (Laminaria japonica) in China. RAS/86/024 Training Manual, 89, 140–140.
Van Der Molen J., Ruardij P., Mooney K., Kerrison P., O’Connor N. E., Gorman E., et al. (2018). Modelling potential production of macroalgae farms in UK and Dutch coastal waters. Biogeosciences 15, 1123–1147. doi: 10.5194/bg-15-1123-2018
Venugopal V., Gopakumar K. (2017). Shellfish: nutritive value, health benefits, and consumer safety. Compr. Rev. Food Sci. Food Saf. 16, 1219–1242. doi: 10.1111/1541-4337.12312
Visch W., Bergström P., Nylund G. M., Peterson M., Pavia H., Lindegarth M. (2020). Spatial differences in growth rate and nutrient mitigation of two co-cultivated, extractive species: The blue mussel (Mytilus edulis) and the kelp (Saccharina latissima). Estuarine Coast. Shelf Sci. 246, 107019. doi: 10.1016/J.ECSS.2020.107019
Weston K., Jickells T. D., Fernand L., Parker E. R. (2004). Nitrogen cycling in the southern North Sea: consequences for total nitrogen transport. Estuarine Coast. Shelf Sci. 59, 559–573. doi: 10.1016/j.ecss.2003.11.002
Xie X., He Z., Hu X., Yin H., Liu X., Yang Y. (2017). Large-scale seaweed cultivation diverges water and sediment microbial communities in the coast of nan'ao island, south china sea. Sci. Total Environ. 598, 97–108.
Yang B., Gao X., Zhao J., Liu Y., Lui H. K., Huang T. H., et al. (2021). Massive shellfish farming might accelerate coastal acidification: A case study on carbonate system dynamics in a bay scallop (Argopecten irradians) farming area, North Yellow Sea. Sci. Total Environ. 798, 149214. doi: 10.1016/j.scitotenv.2021.149214
Zhang J., Hansen P. K., Fang J., Wang W., Jiang Z. (2009). Assessment of the local environmental impact of intensive marine shellfish and seaweed farming–application of the MOM system in the sungo bay, china. Aquaculture 287 (3-4), 304–310. doi: 10.1016/j.aquaculture.2008.10.008
Zhang L., Wang H. (2015). Multiple mechanisms of anti-cancer effects exerted by astaxanthin. Mar. Drugs 13, 4310–4330. doi: 10.3390/md13074310
Keywords: extractive aquaculture, macroalgae cultivation, nutrients, carrying capacity, environmental impacts, numerical modelling
Citation: Johnson M, Jutard Q, Jaouen M, Maltsev N, Boyer M, Guillerme C, McElligott D, Paolacci S, Maguire J, Mangin A and Bryère P (2024) Potential nutrient, carbon and fisheries impacts of large-scale seaweed and shellfish aquaculture in Europe evaluated using operational oceanographic model outputs. Front. Mar. Sci. 11:1405303. doi: 10.3389/fmars.2024.1405303
Received: 22 March 2024; Accepted: 29 July 2024;
Published: 17 September 2024.
Edited by:
Carter Newell, Pemaquid Mussel Farms (United States), United StatesReviewed by:
Leonel Pereira, University of Coimbra, PortugalCopyright © 2024 Johnson, Jutard, Jaouen, Maltsev, Boyer, Guillerme, McElligott, Paolacci, Maguire, Mangin and Bryère. This is an open-access article distributed under the terms of the Creative Commons Attribution License (CC BY). The use, distribution or reproduction in other forums is permitted, provided the original author(s) and the copyright owner(s) are credited and that the original publication in this journal is cited, in accordance with accepted academic practice. No use, distribution or reproduction is permitted which does not comply with these terms.
*Correspondence: Martin Johnson, bWpvaG5zb25AYm1ycy5pZQ==
Disclaimer: All claims expressed in this article are solely those of the authors and do not necessarily represent those of their affiliated organizations, or those of the publisher, the editors and the reviewers. Any product that may be evaluated in this article or claim that may be made by its manufacturer is not guaranteed or endorsed by the publisher.
Research integrity at Frontiers
Learn more about the work of our research integrity team to safeguard the quality of each article we publish.