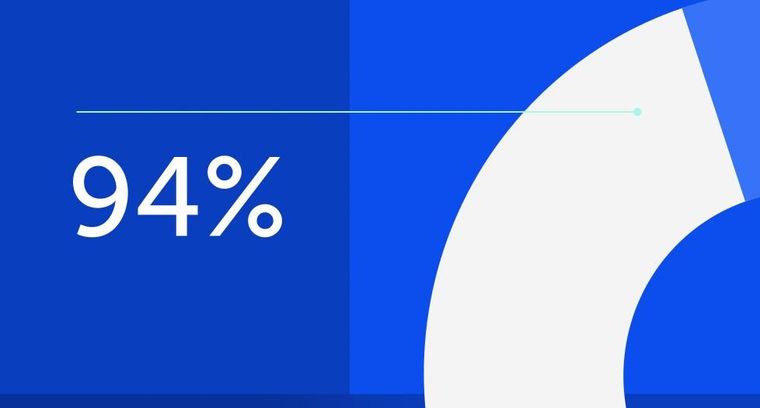
94% of researchers rate our articles as excellent or good
Learn more about the work of our research integrity team to safeguard the quality of each article we publish.
Find out more
ORIGINAL RESEARCH article
Front. Mar. Sci., 09 July 2024
Sec. Ocean Observation
Volume 11 - 2024 | https://doi.org/10.3389/fmars.2024.1396491
This article is part of the Research TopicDemonstrating Observation Impacts for the Ocean and Coupled PredictionView all 18 articles
We assess for the first time the impact of in-situ ocean observations on European Centre for Medium-Range Weather Forecasts (ECMWF) sub-seasonal forecasts of both ocean and atmospheric conditions. A series of coupled reforecasts have been conducted for the period 1993-2015, in which different sets of ocean observations were withdrawn in the production of the ocean initial conditions. Removal of all ocean in-situ observations in the initial conditions leads to significant degradation in the forecasts of ocean surface and subsurface mean state at lead times from week 1 to week 4. The negative impact is predominantly caused by the removal of the Argo observing system in recent decades. Changes in the mean state of atmospheric variables are comparatively small but significant in the forecasts of lower and upper atmospheric circulation over large regions. Our results highlight the value of continuous, real-time in-situ observations of the surface and subsurface ocean for coupled forecasts in the sub-seasonal range.
Behind every weather and climate forecast product, every extreme event and disaster managed, every forecast and predictability examined, satellite and in-situ observational data play a pivotal role. In recent decades, since the advent of global coupled forecasting, operational forecasting centers are in demand of continuous, near real-time, homogenous, ground truth observations, especially of the data-sparse ocean part, which covers more than 70% of the Earth’s system surface. Moreover, subsurface ocean, which is one of the sources of predictability in the sub-seasonal range (e.g. Vitart et al., 2015; Subramanian et al., 2019) cannot be observed remotely. Therefore, assimilation of ocean in-situ observations is key to constrain the 3-dimensional ocean state and provides better estimation of the ocean initial conditions (e.g. Balmaseda et al., 2015; Penny et al., 2019) for global coupled forecasting and climate services.
Ocean data assimilation products made in the behind-real time [Ocean-sea ice ReAnalyses (ORAs)] are also fundamental tools for global monitoring of our changing climate. Continuously changing observing systems are challenging for representing realistic long-term changes in reanalysis datasets (Balmaseda et al., 2015; Hegerl et al., 2015). Since the early 2000s, Argo profiling floats have started a new era of providing continuous, real-time global temperature, salinity profiles and ocean current observations of the upper ocean (Gould et al., 2004). Prior to the Argo period, ship-based XBT/CTD measurements and tropical mooring observations used to dominate in-situ ocean observational types.
Current ECMWF ocean-sea ice reanalysis [ORAS5 (Zuo et al., 2019)] assimilates both satellite and in-situ ocean observations and provides initial conditions for all ECMWF coupled forecasting systems in the medium-to-sub-seasonal-to-seasonal range. While the role of ocean observations has also been assessed in earlier generation of ECMWF seasonal forecasting systems (e.g. Balmaseda and Anderson, 2009), and decadal forecast experiments (Doblas-Reyes et al., 2011), it has not yet been assessed in the ECMWF extended-range forecasting system which provides the sub-seasonal forecasts up to day 46 (Vitart et al., 2019).
To fill this gap of information in the sub-seasonal time scale, we evaluate for the first time the overall impact of all in-situ ocean observations and Argo observations in the ECMWF extended-range forecasting system using ocean observing system experiments. The aim of the paper is to provide an overall assessment of the global observational impact with a focus on understanding the changes in the mean state of the sub-seasonal range coupled ensemble forecasts. The rest of the paper is organized as follows: Section 2 describes the methodology and independent observational datasets used for the forecast verification. In Section 3 we assess the observational impact on the changes in mean state and forecast skill of the ocean and atmospheric forecasts. Section 4 presents the summary and further remarks.
One of the methods for assessing observational impact on global numerical weather prediction systems is Observing System Experiments (OSEs) (e.g. Fujii et al., 2019). It measures the impact on forecast biases and skill of removing an observational type from the data assimilation system which produces the initial conditions for the forecasts. Here we describe the Ocean OSEs conducted for this study and its specifications.
Three ocean OSES are carried out (Table 1), using the Reanalysis and the sub-seasonal Reforecast (Retrospective forecast or Hindcast) experiments for the global domain: 1) Ref: a reference reforecast experiment which is initialized from a Reanalysis experiment where all in-situ ocean observations are assimilated in producing the initial conditions, 2) NoInsitu: a sensitivity reforecast experiment which is initialized from a Reanalysis experiment where all in-situ observations are not assimilated, and 3) NoArgo: a sensitivity Reforecast experiment which is initialized from a Reanalysis experiment where only Argo observations are not assimilated.
The initial conditions are produced using a low-resolution version of ORAS5 (Zuo et al., 2019) with ORCA 1° ocean horizontal resolution and 42 vertical levels. The first model layer is 10 m thick, and the upper 25 levels represent approximately the top 880 m. Both the horizontal and vertical resolution in our setup is lower than that of ORAS5, which has a horizontal resolution of approximately 0.25° and 75 vertical levels. ORAS5 assimilates observations of temperature and salinity profiles, altimeter-derived sea level anomalies and sea ice concentration. In order to isolate and focus on the in-situ observational impact, sea level assimilation and bias correction (which indirectly holds the effect of in-situ observational information) are also removed in the initial condition production in our study. We keep the same atmospheric forcing fluxes and experimental set up in all the 3 Reanalysis experiments except for the differences in assimilated in-situ observations.
The 5 members of ocean-sea ice initial conditions are produced by perturbing the surface forcing fields and observations assimilated as described in Zuo et al., 2017. The forcing perturbation part addresses structural and analysis uncertainties while the observation perturbation part addresses the observational representativeness error. In this study, unlike that in ORAS5, only SST and Sea Ice Concentration (SIC) fields of the forcing perturbation part, and SIC, temperature and salinity profiles of the observation perturbation part are perturbed. That means that the temperature and salinity profiles from all in-situ sources are not perturbed in the NoInsitu experiment and those from Argo observations are not perturbed in the NoArgo experiment. All 3 experiments have SST relaxation (equivalent to a restoration surface heat flux term of -200 Wm-2K-1), which is the same as in ORAS5. So the observational impact assessed in our study is exclusively the contribution of subsurface ocean in-situ observations 1) on the surface and subsurface oceanic forecasts, and 2) on the sea surface temperature forecasts and thereby the atmospheric forecasts, in spite of the SST relaxation needed in the Reanalysis experiments.
The 5-member ensemble of coupled reforecasts are performed with a low-resolution version of ECMWF extended-range forecasting system. The coupled model consists of the same ocean and sea ice model (NEMO3.4/LIM2) used for our reanalysis experiments and is coupled to the ECMWF atmospheric model, Integrated Forecast System (IFS) version 47r1. It is run with a horizontal resolution of 36 km, corresponding to a cubic octahedral reduced Gaussian grid at truncation Tco319 and 137 vertical levels. All the three reforecasts are started on the first of each month of each year from 1993 to 2015, resulting in 276 forecast start dates in total. Note that week 1 in the extended-range forecasts starts from day 5 onwards and week 4 ends on day 32.
The observational impact on the coupled forecast skill is assessed by verifying against independent observations: ESA CCI SST and SIC products (Merchant et al., 2019), CMEMS GREP V2 ocean variables (Storto et al., 2018) and ERA5 atmospheric variables (Hersbach et al., 2020). Statistical significance is calculated using a bootstrap resampling method (Roberts et al., 2022, Supplementary Figure S1). The differences, and forecast skill scores are calculated 500 times using randomly selected samples of start dates with replacement in order to obtain a smooth approximation of the population distribution. Results are estimated as statistically significant at 10% level if the 5th and 95th percentiles of the bootstrap distribution of differences have the same sign, which is equivalent to a p-value of 0.1 for the two-tailed test.
To make a fair comparison, the observational impact is assessed as differences between the OSE reforecasts and Reference reforecasts, NoInsitu – Ref, NoArgo – Ref during the post-Argo period (2005-2015). Since NoInsitu experiment has the in-situ observations removed from the beginning of the experiment period, 1993, and the availability of Argo observations begin only after year 2000, NoArgo observational impact is absent in the NoArgo experiment during the pre-Argo period, 1993-2005. Due to this difference in memory of the observational impact, the NoInsitu impact is generally bigger than the NoArgo impact even in the post-Argo period. Spatial maps and forecast skill score cards of the changes in oceanic and atmospheric mean state are shown in Figures 1–5. Maximum impact is seen in the forecasts started in the month of November and the spatial maps are shown for November start dates to explain the coherent observational impact on both oceanic and atmospheric forecasts.
Figure 1 Observational impact on the mean state of SST forecasts: Difference maps of week 4 SST forecasts started on the 1st of November during the post-Argo period, 2005-2015, NoInsitu – Ref (top), NoArgo – Ref (bottom).
Figure 2 Observational impact on mean state of Mixed Layer Depth forecasts: Difference maps of week 4 Mixed layer Depth forecasts, started on the 1st of November during the post-Argo period, 2005-2015, NoInsitu – Ref (top), NoArgo – Ref (bottom).
Figure 3 Observational impact on bias score of surface and subsurface forecasts: Bias score card of ocean variables for lead times of week 1 to week 4, started on the 1st of each calendar month during the post-Argo period, 2005-2015, for whole of the Northern Hemisphere and tropical regions. Variables shown are sea ice concentration (ci), surface salinity (sos), mixed layer depth (mld), sea surface height (zos), zonal current velocity (ocu), meridional current velocity (ocv), depth of 20°C isotherm (t20d), average salinity in the upper 300m (sav300), and average temperature in the upper 300m. Bias scores are measures of magnitude of biases integrated across all grid points and all start months (Equation 1, Roberts et al., 2021). Size of the triangles indicate the magnitude of the bias scores. Red triangles denote significant degradation in forecasts due to the removal of ocean in-situ observations in the initial conditions.
Figure 4 Observational impact on mean state of atmospheric surface temperature forecasts: Difference maps of week 4 2-m temperature forecasts started on the 1st of November during the post-Argo period, 2005-2015, NoInsitu – Ref (top), NoArgo – Ref (bottom).
Figure 5 Observational impact on bias score of surface and upper atmospheric forecasts: Bias score card of atmospheric variables for lead times of week 1 to week 4, started on the 1st of each calendar month during the post-Argo period, 2005-2015, for whole of the Northern Hemisphere and tropical regions. The variables shown are 2 m temperature (2t), surface temperature (stl1), mean sea level pressure (msl), zonal/meridional wind (u/v), temperature (t), geopotential height (z), barotropic streamfunction (strf), velocity potential (vp), rossby wave source (rws) and sea surface temperature (sst). Numbers in variable names correspond to a specific pressure level in hPa. Bias scores are measures of magnitude of biases integrated across all grid points and all start months (Equation 1, Roberts et al., 2021). Size of the triangles indicate the magnitude of the bias scores. Red triangles denote significant degradation in forecasts due to the removal of ocean in-situ observations in the initial conditions.
Removal of in-situ observations in the initial conditions has significantly changed the mean state of the ocean forecasts in week 1 and the impact persists up to week 4. Overall cooling (Figure 1) and freshening (Supplementary Figure S1) is seen in the SST and sea surface salinity (SSS) forecasts. The magnitude of changes seen, albeit small, is comparable to that of the SST biases in the Reference experiment (Supplementary Figure S2) but the sign of the changes is not always in the direction of the degradation of the existing bias. It could be because 1) the errors in SST forecasts are not always due to the quality of the observational constraint in the initial conditions; SST and SSS biases could arise due to the physical response of errors elsewhere in the forward model, and 2) inadequate constraint of sub-surface temperature and salinity in NoInSitu and NoArgo leads to the development of surface impacts later in the forecast, despite the constraint of SSTs in the initial conditions. Note that the differences seen in the SST forecasts are in spite of the SST constraint that is used to generate all initial conditions. It suggests that sub-surface observations are important for SST forecasts even if SST is constrained during the data assimilation.
Since the subsurface ocean provides an important source of predictability in the sub-seasonal range, we look at the changes in the upper ocean mean state using the depth of 20 degree isotherm (t20d) and mixed layer depth. Consistent to the changes in the surface temperature and salinity pattern seen in Section 3.1 the subsurface density has also changed. There are significant changes seen in the upper 300m averaged temperature and salinity forecasts (not shown), mean mixed layer (Figure 2) and depth of 20 degree isotherm (Supplementary Figure S3) forecasts, altering the thermocline gradients. Large patterns of change in the mixed layer processes are seen over the north Atlantic deep water formation sites and subtropics in both the hemispheres (Figure 2).
Mostly positive biases are generally seen in the depths of thermocline over the Gulf Stream, Gulf of Mexico, and Kuroshio regions, tropics and subtropics (not shown). Removing observations has deepened the thermocline over these regions and shoaled it over the northern subtropical gyre and tropical Pacific regions. Since the verification of these forecasts are against the multi-model reanalysis dataset, GREP V2, where these variables are not very well-constrained, the quality of the verification could be subjective. Nevertheless, both the NoInsitu and NoArgo impact show similar patterns of change with a pronounced signal over the Gulf Stream region indicating tentatively the role of in-situ observations, predominantly Argo observations, in forecasting the path of Gulf Stream and tropical cyclones in different ocean basins.
The evolution of changes in the mean state for a collection of ocean surface and subsurface variables from week 1 (5-11 days) to week 4 (26-32 days), for all the 12 start months is depicted in the bias score card (Figure 3). Significant degradation in the depth of 20 degree isotherm (t20d), top 300m averaged temperature (tav300) and salinity (sav300), surface salinity (sos), mixed layer depth (mld), zonal and meridional ocean current velocity (ocu, ocv) are found over the Northern Hemisphere and tropics in both NoInsitu and NoArgo experiments (red triangles). A small improvement (blue triangles) in sea surface height (zos) forecasts is the only exception.
As explained in Sections 3.1 and 3.2, the significant changes seen in SST, MLD and t20d by removing in-situ observations do impact the atmospheric forecasts, but smaller in magnitude compared to that on the oceanic forecasts. The significant impact on near-surface temperature (T2m) forecasts is overall cooling and is similar to the changes in the SST over the oceans (Figure 4) except for the Arctic region. Though statistically insignificant, notable warming is present in NoInsitu and NoArgo over the Gulf of Mexico region in the ocean subsurface, SST, T2m and temperature at 850 hPa (T850) and at 500 hPa (T500) pressure levels (not shown).
Observational impact on forecasts of atmospheric mean state for all the 12 start months is summarized as bias scores in Figure 5. Results for the lower and upper atmosphere are not as clear as the ocean forecasts with the current sample size. However, small and significant changes in bias scores over tropics and Northern Hemisphere are seen on mean sea level pressure (mslp), geopotential height at 500 hPa (Z500) and barotropic streamfunction forecasts at 200 hPa (strf200). Both NoInsitu and NoArgo observational impacts are seen as significant changes in Z500 and strf200 over large tropical and mid-latitude regions (Supplementary Figure S4). Prominent changes in precipitation (tprate) forecasts over subtropical convergence zones are seen (not shown). There is some indication that in-situ ocean observations could have a role in changing the upper atmospheric circulation via changing the propagation of the Northern Hemisphere subtropical wave guide. The Rossby wave source forecasts at 200 hPa show noisy but consistent changes (not shown).
Our results on the ocean observational impact on the atmospheric forecasts are more robust for near-surface fields over the tropics and Northern Hemisphere. Robustness of both the lower and upper atmospheric impact needs to be confirmed by carrying out OSE experiments with increased sample size and operational ocean resolution in future studies.
Results on the impact of ocean in-situ observations in the ECMWF coupled sub-seasonal forecasting system using ocean observing system experiments are discussed. One of the dominant types of ocean in-situ observations, Argo observations were removed in the production of the ocean-sea ice initial conditions. By comparing the difference in the reforecast sets, we have assessed the impact on the forecasts of ocean and atmospheric variables in the lead times of 1 to 4 weeks.
The ocean in-situ observations have significant impact on the mean state of forecast ocean and atmospheric variables. Overall cooling and freshening of the ocean surface and subsurface and changes in the structure of thermocline gradients are found. It is related to local air-sea interaction due to fast changes in ocean mixed layer in the ocean initial conditions. The magnitude of observational impact on SST forecasts is comparable to that of systematic forecast model bias. The impact is predominantly contributed by the Argo observing system in the recent decades. It does not always get translated into improvements, since the biases in the atmospheric model are not exclusively due to SST errors. Significant negative impact on the probabilistic forecast skill scores are found in the surface and subsurface ocean forecasts over the northern hemisphere and tropics (Supplementary Figure S5). The dominant impact of Argo observations on forecasts of ocean variables are consistent to earlier studies carried out using ocean OSEs of Mercator Ocean analyses and ocean-only forecasts for a single year (Turpin et al., 2016).
The impact on atmospheric mean state is relatively small, but significant in mean near-surface temperature, and lower and upper atmospheric circulation over large regions. Impact on the atmospheric forecast skill scores are not statistically significant in our results (Supplementary Figure S6).
Specific process-based aspects of the role of in-situ ocean observations and air-sea interaction on regional forecasts are discussed in Du et al., 2023 and Wei et al., 2023. Surface and subsurface tropical Pacific ocean forecasts benefit from in-situ observations. The cold tongue bias found over the Nino regions, central and eastern tropical Pacific is significantly reduced in the ECMWF forecast model when in-situ ocean observations are assimilated (Wei et al., 2023). In-situ observational role in the existing forecast errors in Madden-Julian Oscillation (MJO) propagation over the Maritime Continent is explored and it is found that biases in the forecasts of atmospheric meridional moisture advection due to intraseasonal meridional wind biases dominate the MJO forecast errors rather than the assimilation of in-situ observations and subsequent SST errors over the region (Du et al., 2023).
The impact of in-situ observations on the coupled forecasts in our study is also limited due to initialization shocks arising from inconsistencies between the coupled forecast model and the uncoupled reanalyses as discussed in Wei et al., 2023. Future studies where the ocean observations are assimilated in a coupled framework with coupled data assimilation is a way forward to disentangle observing system evaluation with coupled model initialization issues.
Novel methods for model error and observation error correction during the assimilation are expected to increase the information content of the ocean observations, especially on the poorly sampled areas such as the deep ocean. This in turn will benefit the synergies between the in-situ and remotely sensed observations such as SST and altimeter. Machine learning methods could also be used to learn the sensitivities of a forecasting system to observational network, that has the potential to provide feedback without the need of expensive OSE experiments. Additionally, diverse type of in-situ observations of both Lagrangian and Eulerian nature needs to be explored for better representation of observational errors in the subsurface ocean, which is particularly relevant for extreme weather prediction.
Our results indicate a) the value of consistent, global upper ocean observational coverage for both operational numerical weather prediction, coupled sub-seasonal-to-seasonal forecasting and climate monitoring services, b) the role of ocean observations on the forecasts of atmospheric mean state in the sub-seasonal to seasonal range, and c) the importance of initializing the upper ocean density structure in sub-seasonal forecasts. This work necessitates the need of robust, uptodate assessment of ocean observational impact in operational resolution and the intercomparison with other leading operational forecasting systems through international cooperation and investment.
The raw data supporting the conclusions of this article will be made available by the authors, without undue reservation.
BB-S: Data curation, Formal analysis, Funding acquisition, Investigation, Methodology, Software, Validation, Visualization, Writing – original draft, Writing – review & editing. MAB: Conceptualization, Funding acquisition, Methodology, Resources, Supervision, Writing – review & editing. FV: Conceptualization, Methodology, Resources, Supervision, Writing – review & editing. CDR: Software, Validation, Writing – review & editing. HZ: Funding acquisition, Methodology, Software, Writing – review & editing. ST: Software, Supervision, Validation, Writing – review & editing. MM: Writing – review & editing.
The author(s) declare financial support was received for the research, authorship, and/or publication of this article. This work has been partly funded by the European Union’s H2020 AtlantOS project (grant no: 633211) and Copernicus Marine Environment Monitoring Service’s GLORAN project (contract no: 21003-COP-GLORAN-LOT3).
We acknowledge the funding, computing facility and datasets which helped to carry out this work. BB-S would like to thank Antje Weisheimer and Tim Palmer for their constructive feedback on the findings.
The authors declare that the research was conducted in the absence of any commercial or financial relationships that could be construed as a potential conflict of interest.
All claims expressed in this article are solely those of the authors and do not necessarily represent those of their affiliated organizations, or those of the publisher, the editors and the reviewers. Any product that may be evaluated in this article, or claim that may be made by its manufacturer, is not guaranteed or endorsed by the publisher.
The Supplementary Material for this article can be found online at: https://www.frontiersin.org/articles/10.3389/fmars.2024.1396491/full#supplementary-material
Balmaseda M., Anderson D. (2009). Impact of initialization strategies and observations on seasonal forecast skill. Geophys. Res. Lett. 36, 1. doi: 10.1029/2008GL035561
Balmaseda M., Hernandez F., Storto A., Palmer M., Alves O., Shi L., et al. (2015). The ocean reanalyses intercomparison project (ORA-IP). J. Oper. Oceanogr. 8, s80–s97. doi: 10.1080/1755876X.2015.1022329
Doblas-Reyes F. J., Balmaseda M. A., Weisheimer A., Palmer T. N. (2011). Decadal climate prediction with the European Centre for Medium-Range Weather Forecasts coupled forecast system: Impact of ocean observations. J. Geophys. Res.: Atmos. 116, D19111. doi: 10.1029/2010JD015394
Du D., Subramanian A. C., Han W., Wei H. H., Sarojini B. B., Balmaseda M., et al. (2023). Assessing the impact of ocean in situ observations on MJO propagation across the maritime continent in ECMWF sub-seasonal forecasts. J. Adv. Model. Earth Syst. 15, e2022MS003044. doi: 10.1029/2022MS003044
Fujii Y., Rémy E., Zuo H., Oke P., Halliwell G., Gasparin F., et al. (2019). Observing system evaluation based on ocean data assimilation and prediction systems: on-going challenges and a future vision for designing and supporting ocean observational networks. Front. Mar. Sci. 6. doi: 10.3389/fmars.2019.00417
Gould J., Roemmich D., Wijffels S., Freeland H., Ignaszewsky N., Jianping X., et al. (2004). Argo profiling floats bring new era of in situ ocean observations. EOS T. Am. Geophys. Un. 85, 185–191. doi: 10.1029/2004EO190002
Hegerl G. C., Black E., Allan R. P., Ingram W. J., Polson D., Trenberth K. E., et al. (2015). Challenges in quantifying changes in the global water cycle. Bull. Am. Meteorol. Soc. 96, 1097–1115. doi: 10.1175/BAMS-D-13-00212.1
Hersbach H., Bell B., Berrisford P., Hirahara S., Horanyi A., Munoz-Sabater J., et al. (2020). The ERA5 global reanalysis. Q. J. R. Meteor. Soc 146, 1999–2049. doi: 10.1002/qj.3803
Merchant C. J., Embury O., Bulgin C. E., Block T., Corlett G. K., Fiedler E., et al. (2019). Satellite-based time-series of sea-surface temperature since 1981 for climate applications. Sci. Data 6, 1–18. doi: 10.1038/s41597-019-0236-x
Penny S. G., Akella S., Balmaseda M. A., Browne P., Carton J. A., Chevallier M., et al. (2019). Observational needs for improving ocean and coupled reanalysis, S2S prediction, and decadal prediction. Front. Mar. Sci. 6. doi: 10.3389/fmars.2019.00391
Roberts C. D., Balmaseda M. A., Tietsche S., Vitart F. (2022). Sensitivity of ECMWF coupled forecasts to improved initialization of the ocean mesoscale. Q. J. R. Meteorol. Soc. 148, 3694–3714. doi: 10.1002/qj.4383
Roberts C. D., Vitart F., Balmaseda M. A. (2021). Hemispheric impact of North Atlantic SSTs in sub-seasonal forecasts. Geophys. Res. Lett. 48.4, e2020GL0911446. doi: 10.1029/2020GL091446
Storto A., Masina S., Simoncelli S., Iovino D., Cipollone A., Drevillon M., et al. (2018). The added value of the multi-system spread information for ocean heat content and steric sea level investigations in the CMEMS GREP ensemble reanalysis product. Clim. Dyn. 53, 287–312. doi: 10.1007/s00382-018-4585-5
Subramanian A. C., Balmaseda M. A., Centurioni L., Chattopadhyay R., Cornuelle B. D., DeMott C., et al. (2019). Ocean observations to improve our understanding, modeling, and forecasting of sub-seasonal-to-seasonal variability. Front. Mar. Sci. 6. doi: 10.3389/fmars.2019.00427
Turpin V., Remy E., Le Traon P. Y. (2016). How essential are Argo observations to constrain a global ocean data assimilation system? Ocean Sci. 12, 257–274. doi: 10.5194/os-12-257-2016
Vitart F., Balmaseda M., Ferranti L., Benedetti A., Balan Sarojini B., Tietsche S., et al. (2019). Extended-range prediction. Technical Memorandum Vol. 854 (Reading, UK: ECMWF). doi: 10.21957/pdivp3t9m
Vitart F., Robertson A. W., S2S Steering Group (2015). “Sub-seasonal to seasonal prediction: Linking weather and climate,” in Seamless Prediction of the Earth System: From Minutes to Months. Eds. Brunet G., Jones S., Ruti P. M. (Geneva, Switzerland: WMO-1156, World Meteorological Organization), 385–401. Available at: http://library.wmo.int/pmb_ged/wmo_1156_en.pdf.
Wei H. H., Subramanian A. C., Karnauskas K. B., Du D., Balmaseda M. A., Sarojini B. B., et al. (2023). The role of in situ ocean data assimilation in ECMWF sub-seasonal forecasts of sea-surface temperature and mixed-layer depth over the tropical Pacific ocean. Q. J. R. Meteorol. Soc. 149, 3513–3524. doi: 10.1002/qj.4570
Zuo H., Balmaseda M., De Boisseson E., Hirahara S., Chrust M., De Rosnay P. (2017). A generic ensemble generation scheme for data assimilation and ocean analysis. ECMWF technical memorandum. (Reading, UK), 795. doi: 10.21957/cub7mq0i4
Keywords: ocean in-situ observational impact, Argo observations, ocean observing system experiment, sub-seasonal forecasts, coupled prediction, ocean reanalysis, ocean initialization, observing system impact
Citation: Balan-Sarojini B, Balmaseda MA, Vitart F, Roberts CD, Zuo H, Tietsche S and Mayer M (2024) Impact of ocean in-situ observations on ECMWF sub-seasonal forecasts. Front. Mar. Sci. 11:1396491. doi: 10.3389/fmars.2024.1396491
Received: 05 March 2024; Accepted: 25 June 2024;
Published: 09 July 2024.
Edited by:
Elisabeth Remy, Mercator Ocean, FranceReviewed by:
Gianmaria Sannino, Energy and Sustainable Economic Development (ENEA), ItalyCopyright © 2024 Balan-Sarojini, Balmaseda, Vitart, Roberts, Zuo, Tietsche and Mayer. This is an open-access article distributed under the terms of the Creative Commons Attribution License (CC BY). The use, distribution or reproduction in other forums is permitted, provided the original author(s) and the copyright owner(s) are credited and that the original publication in this journal is cited, in accordance with accepted academic practice. No use, distribution or reproduction is permitted which does not comply with these terms.
*Correspondence: Beena Balan-Sarojini, YmVlbmEuYmFsYW5zYXJvamluaUBwaHlzaWNzLm94LmFjLnVr
Disclaimer: All claims expressed in this article are solely those of the authors and do not necessarily represent those of their affiliated organizations, or those of the publisher, the editors and the reviewers. Any product that may be evaluated in this article or claim that may be made by its manufacturer is not guaranteed or endorsed by the publisher.
Research integrity at Frontiers
Learn more about the work of our research integrity team to safeguard the quality of each article we publish.