- 1William & Mary, Virginia Institute of Marine Science, Gloucester Point, VA, United States
- 2Department of Marine and Environmental Biology, University of Southern California, Los Angeles, CA, United States
- 3Biology Department, Woods Hole Oceanographic Institution, Woods Hole, MA, United States
- 4Geosciences Department, Princeton University, Princeton, NJ, United States
- 5Biology Department, St. Mary’s College of Maryland, St. Mary’s City, MD, United States
- 6Skidaway Institute of Oceanography, University of Georgia, Savannah, GA, United States
- 7Department of Molecular and Cellular Biology, University of Arizona, Tucson, AZ, United States
- 8Center for Aquatic Cytometry, Bigelow Laboratory for Ocean Sciences, East Boothbay, ME, United States
- 9Department of Biology, Pennsylvania State University Schuylkill, Schuylkill Haven, PA, United States
- 10Institute for Biodiversity and Ecosystem Dynamics, University of Amsterdam, Amsterdam, Netherlands
- 11Department of Ecology, Evolution, and Marine Biology, University of California, Santa Barbara, Santa Barbara, CA, United States
Protist plankton can be divided into three main groups: phytoplankton, zooplankton, and mixoplankton. In situ methods for studying phytoplankton and zooplankton are relatively straightforward since they generally target chlorophyll/photosynthesis or grazing activity, while the integration of both processes within a single cell makes mixoplankton inherently challenging to study. As a result, we understand less about mixoplankton physiology and their role in food webs, biogeochemical cycling, and ecosystems compared to phytoplankton and zooplankton. In this paper, we posit that by merging conventional techniques, such as microscopy and physiological data, with innovative methods like in situ single-cell sorting and omics datasets, in conjunction with a diverse array of modeling approaches ranging from single-cell modeling to comprehensive Earth system models, we can propel mixoplankton research into the forefront of aquatic ecology. We present eight crucial research questions pertaining to mixoplankton and mixotrophy, and briefly outline a combination of existing methods and models that can be used to address each question. Our intent is to encourage more interdisciplinary research on mixoplankton, thereby expanding the scope of data acquisition and knowledge accumulation for this understudied yet critical component of aquatic ecosystems.
1 Introduction
The planktonic protist community can be divided into three main functional groups - phytoplankton, zooplankton, and mixoplankton. The physiology and the biogeochemical and food web impacts of mixoplankton - protists that combine photoautotrophy and phagotrophy for growth (Flynn et al., 2019) - are poorly understood compared to phytoplankton and zooplankton. Despite the detection of mixoplankton in aquatic systems as far back as the 1930s (Biecheler, 1936), their prevalence was not fully appreciated by the larger aquatic science community until the last decade (Flynn et al., 2013). To complicate further, the traditional methods used to study plankton communities in situ often do not distinguish between mixoplankton and other plankton groups (Millette et al., 2018). Mixoplankton and phytoplankton are often amalgamated due to the presence of chloroplasts in both types of organisms. In turn, estimations of grazing using indirect measurements (e.g., dilution and prey removal experiments) cannot distinguish between zooplankton and mixoplankton grazers (Ferreira et al., 2021).
Despite the methodological challenges, empirical evidence shows that mixoplankton are ubiquitous globally in marine (Leles et al., 2017; Faure et al., 2019; Mitra et al., 2023a) and freshwater systems (Saad et al., 2016; Hansson et al., 2019). Pioneering studies that targeted in situ mixoplankton found that they can contribute to over 50% of the bacterivory in oligotrophic regions in the ocean (Unrein et al., 2007; Zubkov and Tarran, 2008) and can be important protistan predators (Li et al., 2000; Jeong et al., 2010). These studies support theory on the competitive advantage of mixoplankton under inorganic nutrient limiting conditions (Thingstad et al., 1996). Since then, other studies quantified the abundance of in situ mixoplankton (e.g., Tsai et al., 2011; Vargas et al., 2012; Gast et al., 2014), revealing new insights into environmental gradients associated with their abundance and competitive success (Edwards, 2019). In addition, large-scale ocean models have made important predictions that mixoplankton can impact trophic dynamics and carbon cycling by increasing cell size and carbon export (Ward and Follows, 2016; Chakraborty et al., 2020). However, we still lack a mechanistic understanding of the metabolic constraints determining mixoplankton responses to environmental variation at the cellular level, which hampers our ability to evaluate the robustness of our predictions at the ecosystem level. Furthermore, most of these in situ studies have focused on mixoplankton ingestion of bacterial prey (Edwards, 2019), despite it being well known that many mixoplankton consume eukaryotic prey (Jeong et al., 2010). Overall, while several studies have focused on factors that impact mixoplankton ingestion rates within controlled experimental conditions (e.g., Sanders et al., 1989, 2000; Li et al., 2000; Jeong et al., 2005; Sanders and Gast, 2012; McKie-Krisberg et al., 2015; Millette et al., 2017; Lim et al., 2019), we know less about in situ mixoplankton presence and activity. To address these knowledge gaps, we discuss how we can combine empirical data (from cultured isolates to in situ bulk community) with different classes of models (from cells to ecosystems) to investigate various research questions and advance mixoplankton research. The integration of empirical and modeling approaches is a powerful way to boost our understanding of ecological processes. For example, it can help to infer the mechanisms behind observed relationships, identify general principles and develop theory, generate new hypotheses that can inform new experiments, and make predictions beyond the environmental conditions covered by the empirical dataset. A selection of approaches stands out for improving our understanding of mixoplankton (Box 1). However, our goal is not to provide extensive methodological details; for detailed descriptions of methods used to detect phago-mixotrophy see Beisner et al. (2019) and Wilken et al. (2019). Our objective is to establish a conceptual framework that encourages interdisciplinary collaboration in the study of mixoplankton. This will require a combination of one or more methods that include controlled laboratory experiments, the development of methods to be applied in situ, and model development. To that end, we focus on how we can integrate different modeling approaches to the empirical methods listed in Box 1 to answer key research questions (Table 1).
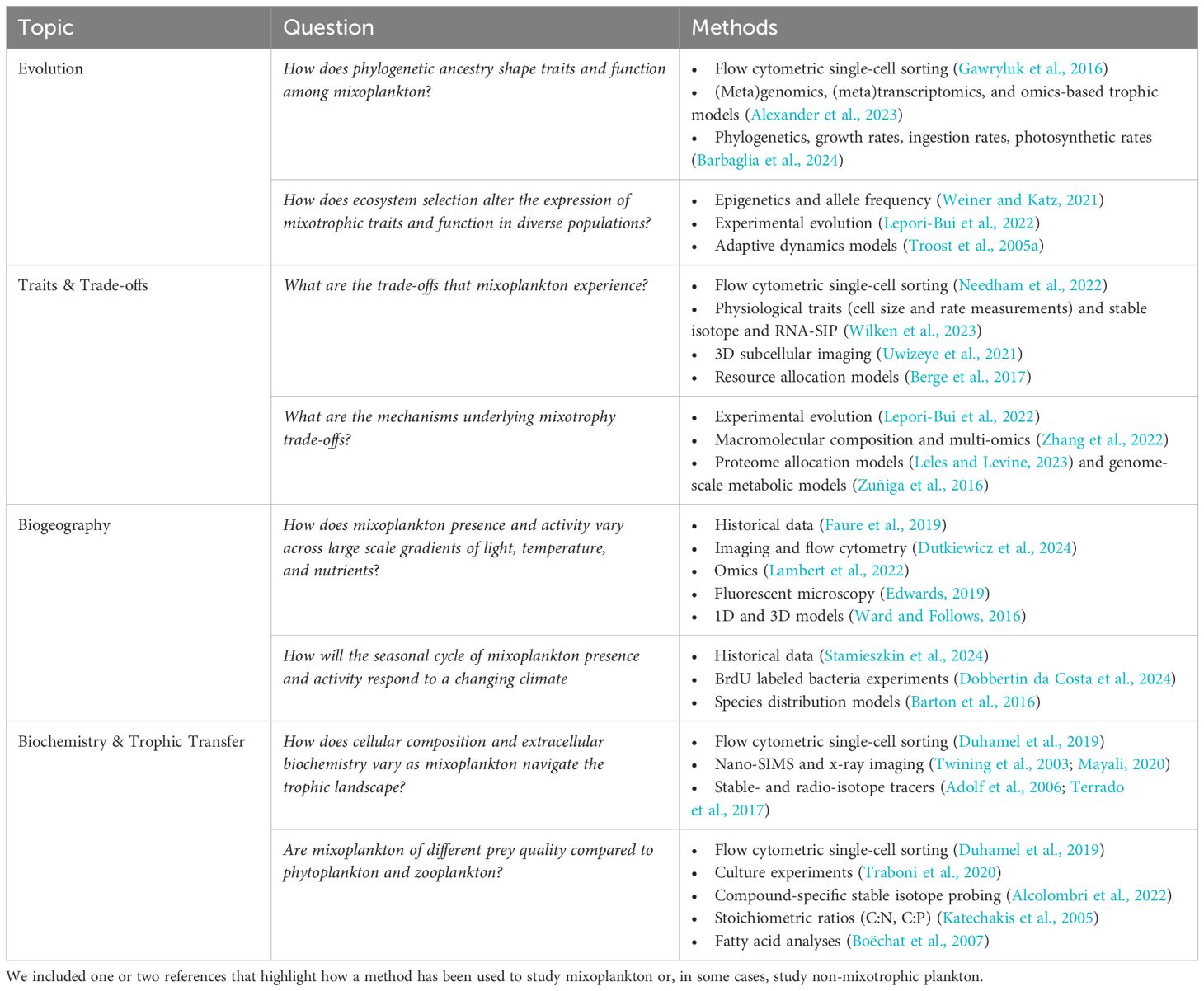
Table 1 Summary of possible research questions that could be asked within each topic area and the combination of methods that could be used to address each question.
For this exercise, we identified two example research questions related to four research priorities described in Figure 1 in Millette et al. (2023). The research priorities are: i) mixotrophy evolution, ii) traits and trade-offs, iii) ecological biogeography, and iv) biogeochemistry and trophic transfer. While our examples are by no means exhaustive or describe the only way this research could be addressed, they serve as compelling illustrations of the vast potential that emerges from connecting empirical and theoretical approaches in the context of mixoplankton research and broader scientific pursuits.
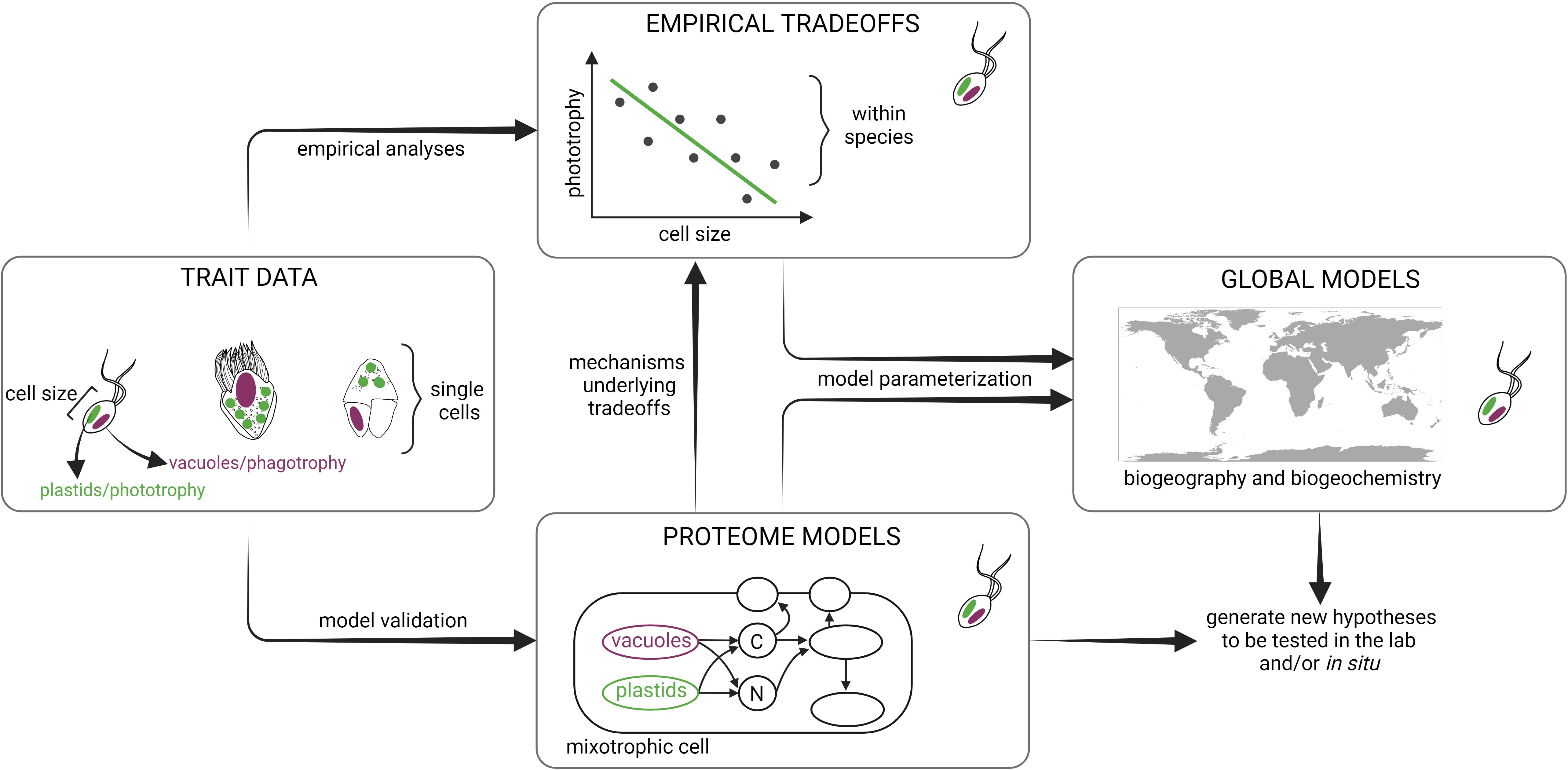
Figure 1 Trait-based framework integrating empirical and modeling approaches to study mixoplankton. Traits measured at the population-level and single-cell techniques can be applied in situ and/or under controlled experimental conditions to determine mixoplankton attributes (e.g. plastids/phototrophy, feeding vacuoles/phagotrophy, and cell size) using a variety of methods such as flow cytometry, isotope labeling, 3D cell imaging, and omics’ datasets. Empirical analyses can then reveal potential trade-offs between traits measured across different environmental conditions within a single species. Proteome allocation models can reveal the mechanisms underlying the empirically derived trade-offs by resolving fine-scale cellular processes. Both proteome models and empirical trade-offs provide critical information to parameterize ecosystem models that can predict mixoplankton biogeography and biogeochemical impact in the global ocean. Ultimately, models can generate new hypotheses that can be tested in the lab and/or in situ. Schematic created with BioRender.com.
2 Mixotrophy evolution
Mixoplankton span an immense breadth of phylogenetic and functional diversity, encompassing nearly every major eukaryotic supergroup (Stoecker et al., 2009; Selosse et al., 2017). This diversity allows us to study the origin and traits of mixoplankton comparatively and ask how mixotrophy has shaped the evolution of lineages, and conversely, how ecosystems drive mixoplankton trait selection and adaptations. Here we highlight some of the current directions and challenges of research regarding mixoplankton evolution by focusing on macroevolutionary (Section 2.1) and microevolutionary (Section 2.2) processes.
2.1 How does phylogenetic ancestry shape traits and function among mixoplankton?
An open question regarding mixoplankton diversity is how their unique evolutionary histories have shaped cell metabolism and physiology of extant protist lineages (Kim and Maruyama, 2014; Mansour and Anestis, 2021). We are gaining a better mechanistic understanding of why and how phago-mixotrophy is maintained in contemporary aquatic systems through insights into the genetic and cellular integration of photosynthetic symbionts/organelles and phago-heterotrophic hosts, and by deciphering the cryptic ancestral genetic signatures of phagotrophy. Such insights have facilitated a better understanding of both non-constitutive (NCM) and constitutive (CM) cultures through use of transcriptomics and proteomics (Hattenrath-Lehmann et al., 2021; Li et al., 2021a; Koppelle et al., 2022; Johnson et al., 2023). Efforts focusing on genetic commonalities among diverse mixoplankton may also reveal conserved traits retained from early phagotrophic ancestors, while instances of gene loss/acquisition can shed light on traits that have enabled the diversification of younger lineages. Ongoing culture work suggests a mixotrophy continuum, ranging from mostly photosynthetic groups (e.g., prasinophytes, haptophytes) to mostly phagotrophic (e.g., dictyochophytes) (Li et al., 2022; Edwards et al., 2023a), but with a high degree of variability within lineages and even among species (Calbet et al., 2011; Wilken et al., 2020).
The various omics approaches serve as powerful tools for investigating the evolutionary trajectories of protists, the biochemical underpinnings of mixotrophic behavior, and potentially even resource allocations between phototrophic and phagotrophic states. Gene-based models using reference databases of protistan genomes or transcriptomes can be leveraged to identify core metabolic features of phago-mixotrophy in lab cultures (Burns et al., 2018; Bock et al., 2021; Koppelle et al., 2022) and in field populations (Lambert et al., 2022; Alexander et al., 2023), enabling explorations into trophic modes of previously unexamined lineages. Comparative genomics can be particularly valuable in examining patterns of plastid gene loss or retention in lineages of mixoplankton (Dorrell et al., 2019), and transcriptomics and proteomics can furthermore shed light on the regulation of genes among closely related mixotrophs differing in trophic abilities (Lie et al., 2018). However, only a small subset of mixoplankton have been cultured in the lab, sequenced, and available in reference annotation databases which models are trained on, limiting our ability to infer phago-mixotrophy in the field based on sequences. Single-cell sorting approaches, in which mixotrophic status can be empirically ascertained and combined with single-cell amplicon, genomic or transcriptomic sequencing, offers a promising way to address this issue (Wilken et al., 2019; Needham et al., 2022). Furthermore, strengthening reference sequence databases with the inclusion of more cultured representatives and supplementing with metagenome assembled genomes (MAGs) derived from the natural environment (Delmont et al., 2022; Alexander et al., 2023) could expand our ability to identify mixoplankton taxa and metabolism. Once robust phylogenies are constructed, phylogenetic modeling can help us to infer likely gains and losses of function and understand how mixotrophic life histories affected rates of diversification (reviewed in Morlon, 2014).
2.2 How does ecosystem selection alter the expression of mixotrophic traits and function in diverse populations?
The richest body of historical research on mixoplankton has been focused on characterizing traits related to phagotrophy and photosynthesis and in identifying trophic interactions. The vast majority of these studies have used rate-based measurements to document photosynthesis and ingestion, and have helped to provide a foundational framework for understanding the occurrence of these traits among mixoplankton. Less well understood, however, are how mixotrophic behavior differs among diverse eukaryotic lineages, both as a function of the environment and due to physiological adaptations. Microevolution is a valuable lens through which to explore phago-mixotrophy, including epigenetics and other regulatory processes. Such approaches could deepen our understanding of how external conditions shape phago-mixotrophy phenotype and may help to predict mixoplankton functional biology and trophic status. For instance, how is the ecophysiology of different species related to epigenetic processes that modulate mixotrophic traits, and how do these relationships vary with ecosystem type? Such networks between resource limitation and trait expression are likely highly diverse and involve some combination of the above scenarios and would need to be broadly surveyed across phylogenetic lineages, types of mixoplankton, and across diverse ecosystems.
While the application of microevolutionary approaches such as epigenetic mechanisms or documenting the distribution and frequency of alleles across populations remain in their infancy for marine microeukaryotes, it may hold promise for understanding interactions between short-term acclimation and long-term adaptation strategies of mixoplankton. For example, DNA modifications and small non-coding RNAs may function as epigenetic mechanisms promoting diversification and driving phenotypic plasticity in microbial eukaryotes (Weiner and Katz, 2021), and could facilitate adaptation in response to environmental pressures. Phenotypic demonstrations of microevolutionary processes in mixoplankton have recently been documented with observations of cultures losing their capacity to feed due to long-term maintenance without prey (Blossom and Hansen, 2021), and in documenting metabolic and behavioral changes in response to warming temperatures (Wilken et al., 2013; Ferreira et al., 2022; Lepori-Bui et al., 2022). Understanding genetic processes that underlie such regulatory changes are needed, and studies are underway by using “experimental evolution” approaches for deciphering molecular dynamics of long-term environmental change.
In recent years an increase in the use of omics, experimental, and modeling approaches for characterizing mixotrophic metabolism and trophic modes across resource gradients has provided a wealth of new insights into mixoplankton physiology and biogeography. This information will also facilitate progress in deciphering evolutionary processes that have shaped lineage diversity of protistan phago-mixotrophs and can be used to validate existing theoretical approaches. Historically, adaptive dynamics models have been used to understand the circumstances under which mixotrophy is evolutionarily viable. These models simulate evolution as a series of small mutations in an organism’s (functional) trait(s) and are used to identify evolutionarily stable strategies (ESSs) that are robust to invasion by slightly different strategies (Diekmann, 2003). Such models have been used to identify environmental conditions under which mixotrophs should specialize (Troost et al., 2005b, 2005a), to evaluate a mixotroph’s optimal balance between phagotrophy and phototrophy as a function of environmental temperature (Gonzalez et al., 2022), and to understand the adaptiveness of kleptoplasty (Brown et al., 2023). These theoretical predictions can be tested with new data on the distribution (and plasticity) of mixotrophs in nature, and better constrained by data on the phylogeny of mixotrophs and their traits.
3 Mixotrophy traits and trade-offs
Here we define a trade-off as the advantage of a phenotypic trait in a given environment that is accompanied by a disadvantage in the same or different environmental context (Agrawal, 2020). We focus on three main traits that determine mixotrophy: photosynthesis, inorganic nutrient uptake, and phagotrophy (Andersen et al., 2015). Identifying when and why these traits trade-off is central to understanding the phenotypic and metabolic responses of mixoplankton to environmental changes. Trade-offs can arise either across and/or within species considering a range of environmental conditions. They might, for instance, be amplified by resource limitation (e.g. nutrient and light scarcity) that restricts investments into different traits, or might further be shaped by community ecology (e.g. interactions with competitors and predators) in cases where resource acquisition and protection against predation require different optimal traits. Furthermore, mixotrophic trade-offs can differ among functional types (constitutive versus non-constitutive mixoplankton). By gaining new insights into the above, we can better understand how trade-offs (or the lack thereof) constrain the impact of mixotrophy on ecological interactions and ecosystem processes, a pressing issue especially considering future climate scenarios (Figure 1). Here we address the methods that can be applied to identify mixoplankton trade-offs (Section 3.1) and the mechanisms underlying such trade-offs (Section 3.2).
3.1 What are the trade-offs that mixoplankton experience?
The fundamental constraints on mixotrophic metabolisms will likely be revealed by investigating key traits related to the three routes of resource acquisition: photosynthesis, nutrient uptake, and phagotrophy (Andersen et al., 2015). Although seemingly straightforward, trade-offs have been hard to characterize (Dolan and Pérez, 2000; Mitra et al., 2023b). The diverse metabolic strategies of mixoplankton complicate the interpretation of trade-offs, for example, not all mixoplankton can take up inorganic nutrients or survive in darkness (Schoener and McManus, 2017; Lie et al., 2018). Methodological challenges also hamper our ability to quantify these traits. For example, while it is relatively easy to quantify chlorophyll a content or photochemical yield of photosystem II (Fv/Fm) as a proxy for investment in light harvesting capacity, it is less straightforward to quantify the relative investments in nutrient uptake and phagotrophy. Furthermore, attempting to tease apart the investments into different nutrient acquisition modes in the field adds another layer of difficulty. Here we propose the application of single-cell techniques in situ (Beisner et al., 2019; Wilken et al., 2019) and traditional physiological measurements obtained in the lab to start estimating these traits across environmental gradients both within and across species. While we call for in situ approaches throughout this paper, we also acknowledge the importance of laboratory-based work with cultures to advance mixoplankton research. These can then be used to better inform trait-based models and predict the large-scale impact of mixotrophy (Figure 1).
Mixoplankton have the metabolic flexibility to modulate the different resource acquisition modes and thus must trade between investing in different cellular and metabolic “machinery”. Flow cytometric methods can potentially provide cell-level detail on an organism’s investment in photoautotrophy and phagotrophy, while distinguishing among co-occurring populations in a natural community (González-Gil et al., 1998; Anderson et al., 2017; Wilken et al., 2019). Specifically, the amount of autofluorescence from plastids can be compared with the fluorescence of acidic food vacuoles stained with acidotropic dyes, such as LysoTracker, in both laboratory and field samples (e.g., Anderson et al., 2017). As mentioned in Box 1, the latter approach is not free of caveats, but the promise of effective trait quantification at the single cell level makes it worth understanding potential biases better (Wilken et al., 2019). If applied successfully, quantification of traits related to photoautotrophy and phagotrophy, can be complemented by those related to nutrient uptake. For example, alkaline phosphatase activity can be assessed by flow cytometry and might serve as a proxy for investments into phosphorus acquisition (González-Gil et al., 1998).
Box 1. Overview of methods
Grazing Assays with Fluorescent Tracers
The use of fluorescently labeled tracer particles (fluorescently labeled prey or fluorescent microspheres) as surrogates for particulate prey is one of the earliest described techniques to quantify ingestion rates by mixoplankton and provide direct comparisons with heterotrophic forms in situ (Bird and Kalff, 1986; McManus and Fuhrman, 1986; Sherr et al., 1987; Rublee and Gallegos, 1989). When paired with epifluorescence microscopy or flow cytometry (Unrein et al., 2007; Waibel et al., 2019), researchers can quantify the uptake of fluorescently labeled tracers over time to estimate ingestion rates. It is also possible to identify putative mixotrophs in combination with catalyzed reporter deposition-fluorescence in situ hybridization (CARD-FISH, Unrein et al., 2007; Šimek et al., 2022). Accuracy of this method relies on having some prerequisite understanding of mixotroph physiology and behavior, as interpretation of results assumes that (1) tracer particles are ingested at the same rate as native prey and (2) mixotrophic organisms graze on prey items at equal rates during the diel period. Applying fluorescently labeled tracer particles is highly repeatable and requires minimal sample handling, allowing for multiple experimental incubations that can target a variety of size classes along a spatiotemporal gradient. However, issues with prey selectivity against inert or heat-killed varieties put limitations on the use of fluorescently labeled tracers, and it is unclear how methodological choices such as preservative influence final grazing assessments. Efforts to use live labeling of prey items may overcome discrimination against tracers (Boenigk et al., 2002; Bock et al., 2021; Li et al., 2021b). Yet, few other techniques allow for direct visualization of ingestion in situ and provide quantification of ingestion rates in combination with more high-throughput techniques (Gast et al., 2014) while preserving comparison with landmark studies in the field.
Flow Cytometry
Flow cytometry has emerged as an efficient and rapidly evolving technique for studying mixoplankton in their natural environment. There are numerous types of flow cytometers available, including imaging and non-imaging systems, all of which can be used to investigate mixoplankton. Non-imaging flow cytometry, based on optical properties, enables the determination of abundance, size, and functional groups of plankton communities. By incorporating fluorescent probes and tracers, such as LysoTracker Green, active mixoplankton communities can be rapidly identified by chlorophyll autofluorescence and their acidic food vacuoles (Rose et al., 2004; Anderson et al., 2017). However, challenges exist, such as misidentifying herbivorous heterotrophs as mixotrophic and staining of acidic cell compartments alongside food vacuoles in non-phagotrophic protists. As a result, caution is advised when interpreting acidotropic stain data in the field until further ground truthing is conducted in the laboratory with a variety of cultures under different conditions. To overcome these limitations, complementary techniques like fluorescently labeled prey incubations and FISH (Massana et al., 2009; Grujcic et al., 2018) can be employed to determine grazing rates and trophic interactions, respectively. On the other hand, traditional flow cytometry with imaging capabilities (i.e. Imaging FlowCytobot: McLane Research Laboratories, and FlowCam: Yokogawa Fluid Imaging Technologies) can be used to identify mixoplankton present based on morphology and estimate their abundance due to these instruments’ high taxonomic resolution (Sieracki et al., 1998; Olson and Sosik, 2007). However, these techniques are limited to morphotypes that are closely linked to a priori knowledge of trophic strategy. Flow cytometric cell sorting further advances the study of in situ mixoplankton by enabling the identification and sorting of active mixotrophs for downstream molecular characterization or measurement of cell activity and physiology (Lin and Glibert, 2019). Despite the cost and specialized equipment associated with flow cytometry, it remains one of the few methods capable of rapid and high-throughput characterization of in situ mixotrophic communities. The future of these methods depends on improved accessibility of instrumentation and the integration of techniques that both identify and directly measure mixotrophic activity.
Omics and Molecular Methods
Methodological advancements in the application of omics techniques to studying eukaryotic plankton have led to a wide range of techniques providing insight into the acclimation and adaptation of diverse protists to environmental conditions via changes in their genomes, transcriptomes, proteomes, and metabolomes. While the molecular machinery required for photosynthesis is evolutionarily conserved and well-studied (Post and Bullerjahn, 1994; Nymark et al., 2009; Cardol et al., 2011), far less is known about the proteins involved in phagotrophy, although recent efforts have begun to catalog the collection of genes associated to phagotrophy (Liu et al., 2016; Labarre et al., 2019). This complicates the identification of an organism that is utilizing both photosynthesis and phagotrophy using molecular tools. Nevertheless, comparative genomics approaches have been successful in predicting the potential for both phototrophy and phagotrophy from genome sequences, allowing for the identification of mixoplankton (Burns et al., 2015, 2018). First insights into shifts in metabolic strategies and trophic modes of mixoplankton in response to changing environmental conditions were gained from transcriptomes of cultured mixoplankton (Liu et al., 2015; Lie et al., 2018). Much more can be learned in the future by targeting a broader range of functionally distinct species, including analyses of the proteome and metabolome to understand investments in enzymatic machinery as well as their metabolic consequences. An important future goal is to improve functional annotations through ongoing development of genetic tools for a wide diversity of protistan groups (Faktorová et al., 2020), which will strengthen our ability to discern changes in physiology and behavior as a function of trophic mode and environmental conditions. Although the application of omics techniques to probe in situ mixotrophic activities remains challenging, research studies have produced promising results. These include detection of changing metabolic investments made by dinoflagellate populations across environmental gradients using a combination of meta-transcriptomics and -proteomics techniques (Cohen et al., 2021), as well as the application of machine learning techniques to recognize transcriptional patterns characteristic of mixotrophic lifestyles (Lambert et al., 2022). The interpretation of such environmental datasets relies on comparison to carefully controlled laboratory experiments with cultured organisms. In contrast, combining single-cell genomics (Yoon et al., 2011) and transcriptomics (Kolisko et al., 2014; Ku and Sebé-Pedrós, 2019) with techniques providing physiological information on the same cells (such as flow cytometry; see e.g., Gawryluk et al., 2016; Needham et al., 2022) could allow assessment of uncultured mixoplankton directly in the wild.
In addition to the development of omics techniques, DNA-based probes can be used to identify photosynthetic organisms that are actively grazing in natural communities. For example, bromodeoxyuridine (BrdU) labeled bacteria offers a way to taxonomically identify mixoplankton that are actively ingesting bacteria within a water sample (Fay et al., 2013). BrdU is a nucleotide analogue to thymidine and can be used to label bacterial DNA. When labeled prey are eaten, BrdU is transferred to the grazer genomic DNA via digestion, assimilation, and replication. Any organism that incorporates BrdU (ingests bacteria) and has chloroplasts (capable of photosynthesizing) is identified as a mixoplankton. While the BrdU method itself cannot provide absolute abundance data, the abundance of taxa identified as mixoplankton via BrdU experiments can be determined for any taxa that were also identified in corresponding microscopy samples (Millette et al., 2021; Dobbertin da Costa et al., 2024). The limited application of BrdU labeled prey to date has used labeled bacteria (Fay et al., 2013; Gast et al., 2018; Millette et al., 2021; Dobbertin da Costa et al., 2024). However, eukaryotic prey could be labeled with BrdU as well in order to target mixoplankton, but this approach will need testing before application to in situ samples.
Isotopes
Mixoplankton metabolism can be studied using 14C-labeled dissolved inorganic carbon (DIC) to measure photosynthesis and using radiolabeled preys (in particular using prey incubated with the amino acids 3H-leucine and 35S-methionine) to estimate bacterivory in natural samples (Zubkov and Tarran, 2008; Hartmann et al., 2012; Duhamel et al., 2019). One advantage of these methods is that radiotracers can be added in low concentration without disturbing the system. However, using radioactive material can be logistically challenging, and radiolabeled prey approaches likely underestimate feeding rates by mixoplankton since results are based on an average for all plastidic protists sorted based on their size (Duhamel et al., 2019). Radiotracer incubations combined with cell sorting by flow cytometry have also been used to quantify prey ingestion rates as well as carbon and phosphorus assimilation rates in natural communities of pigmented protists, which include strictly photoautotrophic plankton and mixoplankton (Jardillier et al., 2010; Grob et al., 2011; Hartmann et al., 2011; Rii et al., 2016b, 2016a; Duhamel et al., 2019). Such an approach would need to be combined with fluorescently-labeled prey (FLP) incubations to target mixotrophs. Alternatively, stable isotope probing (Alcolombri et al., 2022) of lipids (Wegener et al., 2016), DNA (Orsi et al., 2018), RNA (Frias-Lopez et al., 2009; Wilken et al., 2023), and single cells (Terrado et al., 2017) is emerging as a useful tool to track microbe interactions in the environment. Additions of stable isotopes in water sample incubations (e.g., 15N, 13C, 18O, 2H in the form of fully or partially labeled substrates and/or prey) can also help identify food preferences, fate of prey, and even the location of ingested particles and elements inside the mixoplankton. Flow cytometric cell sorting of plastidic protists in incubations with various stable isotope labeled molecules (Berthelot et al., 2019, 2021) and FLP followed by nanoSIMS (nanoscale secondary ion mass spectrometry) could be used to quantify carbon and nitrogen fluxes in mixoplankton. New directions include taking advantage of bulk and compound-specific natural abundance isotope composition via 15N and 13C trophic enrichment (e.g., Yun et al., 2022) and 2H metabolism response to heterotrophy in prokaryotes (Zhang et al., 2009) and eukaryotes (Maloney et al., 2024). Early experiments have indicated that 2H/1H ratios of algal biomass (Estep and Hoering, 1981) and specific lipids (Cormier et al., 2022) are sensitive to shifts from autotrophy to osmo-heterotrophy as well as shifts in bacteria capable of photoheterotrophy (Zhang et al., 2009). Tests are needed to determine if and how phago-mixotrophy influences the composition of the natural abundance of stable isotopes in biomass and biomolecules. Most of these methods are best suited for constitutive mixotrophs (CMs), since measuring mixotrophy by non-constitutive (NCM) species involves assessment of their photosynthesis rates and this is complicated in bulk, in situ conditions due to the potential for ingesting photosynthetic prey that have also taken up radio- or stable isotope-labeled dissolved inorganic C. Limitations include restricted access to specialized instruments, such as cell sorters and nanoSIMS at certain institutions, challenges in selecting prey and dyes for preparing FLP due to potential food preferences exhibited by mixotrophs, as well as potential prey egestion since samples need to be preserved (Bock et al., 2021).
Analysis of Historical Data
Historical, long-term datasets can allow for large-scale analyses with high spatial and/or temporal coverage. As such, there have been recent efforts to estimate mixoplankton abundance, presence, or proportion in publicly available plankton microscopy and sequence datasets. For these analyses, taxa are classified as mixoplankton based on experimental evidence for mixotrophy, i.e., if a taxon in the selected dataset has been previously reported as a phago-mixotroph in peer-reviewed literature. This approach allows for a rapid estimation of mixoplankton abundance/presence and proportions in a large number of samples. Datasets with associated environmental data are preferable so that conditions associated with temporal and spatial variability in potential mixoplankton can be explored. Applications of this approach include reanalysis of the Continuous Plankton Recorder (CPR) observations in the North Atlantic (Barton et al., 2013; Stamieszkin et al., 2024), the Ocean Biogeographic Information System database for the global oceans (Leles et al., 2017, 2019; Mitra et al., 2023a), Rijkswaterstaat monitoring program in the southern North Sea (Schneider et al., 2020), and the TARA Oceans dataset for the global oceans (Faure et al., 2019). However, this method is only as reliable as the available data on known mixoplankton. In many cases we do not know with certainty which taxa are mixoplankton within a given dataset, especially species within the nanoplankton size spectrum that lack defining morphological characteristics. In addition, it is uncertain whether a known mixoplankton species was actively engaging in both nutrition modes at a given time and location. As a result, this approach can either underestimate or overestimate their “true” abundance, resulting instead in a “potential” estimation of mixoplankton abundance (Stamieszkin et al., 2024). Methods such as fluorescent-labeled prey, LysoTracker, or BrdU-labeled prey have the potential to identify active mixoplankton in situ (Fay et al., 2013; Millette et al., 2021; Dobbertin da Costa et al., 2024), providing critical new information to re-analyze historical long-term datasets. However, in order for this to be attempted, scientists must first properly assign active mixoplankton as such. This will require a substantial commitment and effort in any region, but the potential to recontextualize vast amounts of historical data, and potentially provide comparisons across regions or temporal scales within a single region, is worth the effort.
Mathematical Modeling
While empirical approaches are critical for generation of new datasets, mathematical and statistical models provide powerful tools for inference. These models can integrate empirical data (including observational and experimental datasets, and field- and laboratory-based work) into predictive frameworks that can be used to interpolate and extrapolate from existing empirical measurements. Already in mixotrophy research, various models have been used to serve a number of purposes: (1) statistical – identifying connections between pieces of data (e.g., linking mixotrophic lineages to environmental conditions (Faure et al., 2019, p. 201)); (2) explanatory – testing mechanistic hypotheses (e.g., predicting coexistence between mixoplankton and specialized taxa (Crane and Grover, 2010; Moeller et al., 2019)); (3) predictive – extrapolating from existing data to understand future trends (e.g., HABs (Li et al., 2022) and the carbon cycle (Mitra et al., 2014; Ward and Follows, 2016; Dutkiewicz et al., 2021)); and (4) hypothesis generating – using existing data to make predictions that can be validated empirically (e.g., identifying trade-offs via optimal resource allocation, Ward et al., 2011; Berge et al., 2017). Because of the breadth of modelers and their tools, throughout this paper, we provide specific examples of how models can be used, in tandem with empirical methods, to address each of the research questions we highlight.
Accurate volume measurements of organelles are another way to quantify traits. In order to switch investments between strategies, mixoplankton must allocate cellular space for different structures. Novel techniques such as 3D subcellular imaging can provide detailed information on the space occupied by plastids and feeding vacuoles, with the potential to determine the metabolic flexibility of different processes (Colin et al., 2017; Uwizeye et al., 2021). As an example, these tools can be used to investigate trade-offs in CMs related to maximizing inorganic nutrient uptake through larger surface area to volume (SA/V) ratios (to accommodate nutrient transporters) versus maximizing ingestion through larger cellular biovolume (smaller SA/V ratios) occupied by feeding vacuoles. By integrating these measurements with models based on resource allocation theory, in which the mixoplankton need to build different structures to optimize the investment of carbon and nitrogen to different functions (Berge et al., 2017), it is possible to validate model predictions and generate new hypotheses about mixotrophy trade-offs (Figure 1).
Uptake kinetics have long been used to characterize trade-offs, especially across species, for both strict autotrophic and heterotrophic protists (Hansen et al., 1997; Edwards et al., 2012). In mixoplankton, the matter is complicated by potential interactions between different resource acquisition pathways. For example, do mixoplankton with higher photosynthetic rates have lower affinity for prey uptake? Recent studies provide initial insights into how these trade-offs play out among nanoplankton (Edwards et al., 2023a, 2023b; Barbaglia et al., 2024). When performed both across and within different mixotrophic species, these analyses can help to reveal the relative roles of intraspecific phenotypic plasticity on setting mixotrophy trade-offs. So far generating these types of data relies on experimentation with cultured isolates. However, stable isotope approaches with high phylogenetic resolution such as RNA based stable isotope probing (RNA-SIP) allow probing resource acquisition by mixoplankton directly within natural communities (Wilken et al., 2023). While RNA-SIP can at best be semi-quantitative, more targeted approaches such as nanoSIMS or Chip-SIP (Mayali et al., 2012) are also more quantitative. If employing isotope sources for both auto- and heterotrophic metabolism, these might also provide information on trade-offs. Such datasets can then be targeted to test modeling predictions, such as the potential correlation between mixotrophic metabolism and cell size (Chakraborty et al., 2017). On the other hand, trait correlations derived from empirical data can be used to inform mechanistic models; they can be used to define mixotrophic trade-offs in models that resolve community and ecosystem dynamics at different scales (Ward and Follows, 2016; Serra-Pompei et al., 2019). Specifically, if two traits are correlated, it is possible to derive parameters that describe this relationship and use it to constrain trait-based models (Figure 1), as it is commonly done for the allometric relationships resolving resource uptake kinetics for autotrophs and heterotrophs (Ward and Follows, 2016).
3.2 What are the mechanisms underlying mixotrophy trade-offs?
Quantification of mixoplankton traits in situ is a first step toward understanding trade-offs. Observed trait correlations might not be true trade-offs (a correlation does not equal causation) or the lack of empirically observed correlations does not exclude the possibility of a real trade-off. Therefore, if trait correlations are observed that likely reflect trade-offs, the mechanistic basis of such trade-offs needs to be understood. Such underlying mechanisms can be of different kinds. For instance, resource limitation might cause trade-offs as resources allocated to one function cannot be used for another. However, even in the presence of plentiful resources, trade-offs might arise through biophysical constraints. This includes limited cell volume or cell surface to accommodate all cellular machineries required for photosynthesis, phagotrophy and nutrient uptake, and all other cellular functions. Finally, trade-offs can arise in the interaction with other species, such as competitors, predators, or parasites. Trait correlations identified with the methods described in section 3.1 can generate hypotheses about underlying mechanisms, which will in turn determine the choice of methods required to test them.
To understand trade-offs associated with limited resource availability, the energetic costs and elemental investments involved in building cellular structures such as plastids versus digestive vacuoles should be quantified. A combination of traditional measurements of macromolecular composition, stoichiometry, and physiological rates (e.g. growth, photosynthesis, and ingestion) with the omics toolbox can be powerful to quantify the biomolecular structures and associated costs invested into each strategy. For example, proteomic approaches applied to cultured isolates in the lab can be used to link changes in strategy following an environmental perturbation to the respective costs of phenotypic adjustments (Zhang et al., 2022). Proteome models work hand-in-hand with the methods mentioned above allowing for assessment of different proteins, incorporating energetic, space, and stoichiometric constraints, and optimizing resource allocation to maximize growth across different environmental conditions (Molenaar et al., 2009; McCain et al., 2021; Leles and Levine, 2023). Such modeling frameworks can reveal new trade-offs as well as the mechanisms underpinning trait trade-offs (Figure 1). In contrast, one might choose a bottom-up approach in systems biology to simulate whole systems from (incomplete) genomes. Genome-scale metabolic models are already applied in the model microalga Chlorella to predict mixotrophic metabolism during utilization of dissolved organic carbon (Zuñiga et al., 2016) but would require further development to capture utilization of chemically much more complex prey organisms.
The optimization of the nutritional balance in mixoplankton might also be determined by constraints other than resource availability. These may be specific to certain taxonomic groups and only become apparent by comparison across species or broader taxonomic units differing in morphology. An example is the relationship between flagellar arrangements found in nano-sized protists and the flow fields they generate, which in turn affect rates of prey encounter and ingestion (Nielsen and Kiørboe, 2021). Such relationships can be resolved by video-microscopy and particle flow field analysis. A previous study suggested that flow fields created by some haptophytes do not support sufficiently high rates of prey ingestion for growth through feeding alone (Dölger et al., 2017), making the mixotrophs reliant on photosynthesis. Various techniques of live microscopy will remain important for observing the behavior of individual cells and integrating these observations with models accounting for hydrodynamics at the relevant scale.
Finally, a trade-off can arise due to direct interference of a trait with species interactions (e.g. predation defense or competition ability). Potential examples include prey searching behavior or the generation of a feeding current that can also attract predators and thus creates a trade-off between optimizing prey capture and avoiding predation (Kiørboe and Thomas, 2020). There could also be synergies for instance in mixoplankton that produce toxins to defend themselves from predators, immobilize their prey and/or inhibit competitors (Berge et al., 2012; Traboni et al., 2020). Assessing such trade-offs requires variation in the presence of interacting species, so they can best be investigated experimentally by manipulating i) the availability of prey, ii) the trophic landscape including pressure from parasites, viral infection, or predators, or iii) the presence of competitors for the same prey particles or dissolved nutrients. Gradually adding complexity to controlled experiments with forced species interactions should allow for insight into in situ community conditions and the external factors that might dictate costs and benefits for a range of traits.
4 Ecological biogeography of mixoplankton
Here we address the ecological biogeography of mixoplankton, i.e. their distributions in modern conditions. The distribution patterns of mixoplankton across environmental gradients (e.g. light, nutrients, prey, and temperature) are not well understood. What is their distribution across spatio-temporal scales and why are they distributed this way? Answering these questions will help us understand how the ecological niches of mixoplankton differ from those of phytoplankton and zooplankton. Changes in community structure can then be predicted given an environmental perturbation. This is critical to understand how plankton communities might shift due to climate change and other anthropogenic pressures and impact ecosystem functioning. Here, we propose how to conduct research on the environmental gradients that result from spatial (latitudinal, Section 4.1) and temporal (seasonal, Section 4.2) variation.
4.1 How do mixoplankton presence and activity vary across large scale gradients of light, temperature, and nutrients?
Ocean basin-scale transects can be used to examine the presence and activity of mixoplankton across large gradients of light, temperature and nutrients. Mixoplankton are able to respond to changing environmental conditions by modulating the photosynthetic and phagotrophic modes of nutrition (González-Olalla et al., 2019). When active mixoplankton are a prominent part of the protist community, a relevant number of organisms are simultaneously being producers and consumers at the bottom of the food web. This has the potential to fundamentally alter food web dynamics by increasing trophic efficiency and the size of organisms (Sanders, 1991; Ward and Follows, 2016). Latitudinal transects can thus reveal the ecological niches of mixoplankton, providing new insights into when the mixoplankton community is more likely to be functioning as producers or consumers.
There are a few on-going decadal transect studies, such as the International Global Ship-based Hydrographic Investigations Program (GO-SHIP; www.go-ship.org) where biological assessments are now included on some US ship based transects (Bio-GO-SHIP; www.biogoship.org). Along these basin-scale transects there are opportunities to monitor and examine the presence and abundance of mixoplankton using tools, such as flow and imaging cytometry at the surface using flowthrough seawater systems as well as at discrete depths. In addition, the pilot Bio-GO-SHIP program includes both eukaryotic metagenomic and transcriptomic data collection and may be analyzed for both mixotrophic presence and activity through comparative genomics. By using these transects, insight to the variability of mixoplankton abundance across different light, temperature and nutrient regimes can be assessed. Similarly, there are long research expeditions that collect plankton and molecular data that may be used to assess mixotrophy, for example, the TARA Oceans Expedition (Faure et al., 2019; Lambert et al., 2022), the Malaspina Expedition in 2010 as well as other cruises that traverse oceanic basins (e.g. Atlantic Meridional Transects).
As a part of basin-scale oceanic transects, a combination of targeted ship-board incubations and observations would be ideal. The development of routine field-based assays for assessing mixotrophic activity would be valuable tools in incorporating oceanic transits using ships involved in both oceanographic research or commercial shipping. These routine measurements could involve either periodic sampling or continuous auto-sampling for incubations coupled with FLP grazing incubations or isotope probing. Such sampling could be preserved for later analysis and could include assessment of genetic diversity. When feasible, incorporating observations that require more immediate processing, such as cell imaging, flow cytometry, or omics approaches, would greatly enhance the potential inference and scope of routine large scale sampling efforts. To conduct basin-scale transects, several suggestions can be considered. First, it is beneficial to sample at different depths within a particular location, as environmental gradients are also associated with depth. Additionally, it is essential to target traditionally under-sampled regions across the globe, such as the Indian Ocean, South Atlantic, South Pacific, and the Southern Ocean, as most data comes from easily accessible coastal marine or freshwater systems. By focusing on these areas, which have received relatively less scientific attention, we can fill critical knowledge gaps into the unique dynamics and biodiversity of mixoplankton in these ecosystems.
Modeling approaches can also be applied to investigate the ecological niches of mixoplankton. For example, Edwards (2019) combined data from studies that targeted in situ mixotrophic nanoflagellates across the global ocean with a competition model that described mixotrophs and their specialized autotroph and heterotroph competitors. However, the dataset compiled was limited in terms of spatial and temporal coverage and similar datasets are largely absent for other mixoplankton functional types. As an alternative, theoretical models that describe different mixoplankton functional types can be used to run simulations under idealized scenarios of environmental stress to evaluate competitive abilities (Leles et al., 2018; Anschütz and Flynn, 2020), with the drawback of a large number of unconstrained parameters. Large-scale models that resolve physics such as 1D (vertical resolution; water column models) and 3D (vertical and horizontal resolution) models can capture variation in, for example, temperature and nutrient concentrations that more closely reflect the real environment. Such models can then be applied to investigate the biogeography of mixotrophy at regional (Lin et al., 2018; Li et al., 2022) and global (Ward and Follows, 2016) scales, and help guide future empirical research. In addition, they can be applied to investigate species shifts and their biogeochemical roles in future climate scenarios (Dutkiewicz et al., 2021). Alternatively, Species Distribution Models (SDMs) can be powerful tools when long-term time series are available. However, their predictive skill to investigate spatial shifts of microbial taxa under future climate change scenarios was found to be low when applied to data from the Continuous Plankton Recorder program (Brun et al., 2016), which emphasizes the need for more targeted surveys.4.2. How will the seasonal cycle of mixoplankton presence and activity respond to a changing climate?
The seasonal cycle of phytoplankton and zooplankton has been well studied across aquatic systems and climate regimes for decades, particularly through long-term monitoring stations that collect plankton data alongside other environmental factors. However, mixoplankton have largely been an overlooked part of the plankton community, limiting our ability to understand drivers of plankton temporal patterns and further predict community response to climate change (Schneider et al., 2021). One way to address this research gap is through reanalyzing long-term monitoring of plankton microscopy and imaging data. Species/genera within these datasets can be classified as mixoplankton (including different types such as CMs and NCMs) based on previous experimental evidence for mixotrophy in peer-reviewed literature (Mitra et al., 2023a). An ideal dataset to analyze the seasonal cycle of mixoplankton is one with enough data to account for intra- and inter-annual variability in a given location to discern the average annual cycle and allow for statistical analysis of environmental conditions associated with variability. While long-term monitoring datasets with plankton taxa data are not common, many exist that would allow for reanalysis of mixoplankton abundance. Examples of these long-term monitoring locations include: Martha’s Vineyard Coastal Observation (Hunter-Cevera et al., 2016), the L4 station in the Western English Channel (Widdicombe et al., 2010), the Narragansett Bay Long-Term Plankton Time Series in Rhode Island (Smayda, 1998), Helgoland Roads in the North Sea (Wiltshire et al., 2010), German Long-Term Ecosystem Research Network site Lake Müggelsee (Gsell et al., 2016), and multiple locations through the French national phytoplankton monitoring network (Hernández Fariñas et al., 2015). Furthermore, NSF Long Term Ecological Research (LTER) sites such as Northeast U.S. Shelf (Fowler et al., 2020) and Northern Gulf of Alaska (Batten et al., 2018) collect phytoplankton data that could be recategorized as mixoplankton. One major drawback to simply reclassifying taxa in datasets as mixoplankton is that there is no confirmation that a specific species or genus is a mixoplankton at a location or that it was actively engaging in mixotrophy at the time of collection. This could lead to an overestimate of mixoplankton abundance and proportion.
Despite these drawbacks, historic records contain a plethora of data that can be used to rapidly expand our understanding of mixoplankton spatiotemporal distribution and associated environmental determinants. Finding a way to constrain what species/genera in a given dataset are mixoplankton and under what conditions or time of year they are most likely utilizing mixotrophy (vs. strict photoautotrophy or strict phagotrophy) would likely improve the accuracy of any abundance estimations. One approach that might be used to help identify mixoplankton within previously collected samples is BrdU labeled prey experiments. Repeated BrdU labeled bacteria experiments can help to develop a list of known mixoplankton taxa in a region where a long-term dataset exists and help identify at what time of the year or under what conditions these taxa are actively engaging in mixotrophy (Dobbertin da Costa et al., 2024). On its own, this data could expand our understanding of mixoplankton’s biogeography, however, when combined with historical datasets, this data can exponentially expand our estimations of active mixoplankton’s temporal and spatial variability. Another possible approach is a combination of flow and imaging cytometry in combination with FLP grazing incubations or isotope probing, as described in section 4.1.
Despite being able to revisit long-term time series and identify mixoplankton species, we cannot retrieve information on the relative contributions of photosynthesis and phagotrophy to their metabolism; presence of a known mixoplankton does not indicate the importance of both nutrient acquisition modes. Mathematical models that resolve carbon and nutrient fluxes and mechanistically represent plankton growth based on environmental conditions can provide insights into the potential metabolic strategy of mixoplankton over the seasonal cycle (Berge et al., 2017; Ghyoot et al., 2017; Leles et al., 2021). These models can be compared against mixoplankton biomass and other environmental variables derived from long-term time series datasets and, in turn, we can analyze the emergent metabolic strategy obtained from the simulations. For example, phagotrophy was found to be important for mixoplankton within the nanoplankton size spectrum during summer due to nutrient limitation but also during winter due to carbon limitation (Leles et al., 2021). It is noteworthy that, although a powerful tool, models are limited by our current understanding of mixoplankton trade-offs (see section 3). It is thus imperative that new datasets are generated to quantify modern in situ seasonal cycles of mixotrophs simultaneously engaging in phototrophy and phagotrophy and their associated environmental conditions. This would be a challenging endeavor that requires some combination of methods, such as uptake of fluorescent particles, LysoTracker and flow cytometry, stable isotopes, and molecular techniques (Anschutz et al., 2024). However, if activity cycles can be quantified at the same locations as long-term monitoring datasets, then it could be possible to hindcast activity using environmental and presence data.
Once the annual cycle of mixoplankton presence and/or activity are better understood, mathematical or statistical modeling can be used to investigate how mixoplankton will respond to climate induced shifts in the environment. Species distribution models (SDMs) can be used in combination with outputs from Earth System Models (ESMs; e.g., sea surface temperature, mixed layer depth, pH, chlorophyll, PAR, etc.) to evaluate shifts in the environmental envelope of mixotrophic taxa (sensu Barton et al., 2016). While SDMs are powerful for evaluating changes to the suitable habitat/environment for individual taxa, they are unable to simulate feedbacks between plankton groups and ocean biogeochemistry. In this case, mathematical models that simulate nutrient fluxes and the mechanisms driving plankton growth can be applied instead (see section 5.2).
5 Biogeochemistry and trophic transfer of mixoplankton
Mixoplankton simultaneously contribute to both primary and secondary production in aquatic food webs. However, the impact of mixotrophy on in situ carbon and nutrient cycling has not been widely studied. The contributions of photosynthesis versus phagotrophy to mixoplankton biomolecules and resulting elemental fluxes are poorly constrained as most laboratory studies do not track the phototrophic versus phagotrophic sources of biomass or the intracellular/extracellular fate of consumed carbon, other macronutrients, and trace metals. This limits our capacity to develop and parameterize mechanistic models that aim to predict how mixoplankton and mixotrophy impact carbon and nutrient fluxes at larger scales in aquatic systems, particularly as prey themselves. For example, it is predicted that mixoplankton may rely disproportionately on phagotrophy relative to phototrophy under climate change scenarios that include extended periods of stratification and nutrient limitation (Wilken et al., 2013; Gonzalez et al., 2022, p. 202; Lepori-Bui et al., 2022; Wieczynski et al., 2023). If mixoplankton begin to favor one nutrient mode over another due to climate change, then we need to understand how this will impact nutrient cycling and transfer of nutrients to lower and higher trophic levels. This section poses two foundational biogeochemical questions regarding the intra- and extracellular elemental fate of ingested particles and primary photosynthates (section 5.1), and the transfer of these elements to higher trophic levels (section 5.2). Tackling these questions with combined and/or new emerging methods will improve our understanding of energy and food web structures and help constrain fluxes of elements through, and export from, aquatic systems. Currently, methods to address the biogeochemistry and trophic transfer of mixoplankton are largely laboratory based. While studies with cultures are necessary and useful to understand inter-and intraspecific variability in mixoplankton, below we emphasize how we can start addressing these questions for the in situ mixoplankton community.
5.1 How does intra- and extracellular biochemical composition vary as mixoplankton navigate the trophic landscape?
A key parameter that needs further investigation is the extent to which phagocytized prey are assimilated by CMs into lipid, nucleic acid, protein, or carbohydrate biomass for growth versus egested, expelled, or respired. Quantifying the amount of carbon fixed through photoautotrophy compared to the carbon assimilated from prey would improve the accuracy of carbon flux predictions in modeling (Ward and Follows, 2016). Similarly, for NCMs, what percentage of assimilated carbon originates from acquired plastid photosynthetic activity versus ingested particles? Figure 2 illustrates possible intracellular and extracellular fates of consumed prey, and the potential impacts these fluxes might have on surrounding organisms. For example, variability in the mixoplankton exometabolome across different trophic conditions may influence surrounding bacteria and archaea dependent on dissolved organic matter, and other nearby phytoplankton that are competing for nutrients. Furthermore, mixoplankton likely present different intracellular biochemistry compared to phytoplankton which may impact fitness of zooplankton feeding on them (Traboni et al., 2020, 2021), see section 5.2. Importantly, such biochemical parameters are likely different for CMs versus NCMs and across different environmental conditions such as light regimes (Fischer et al., 2022).
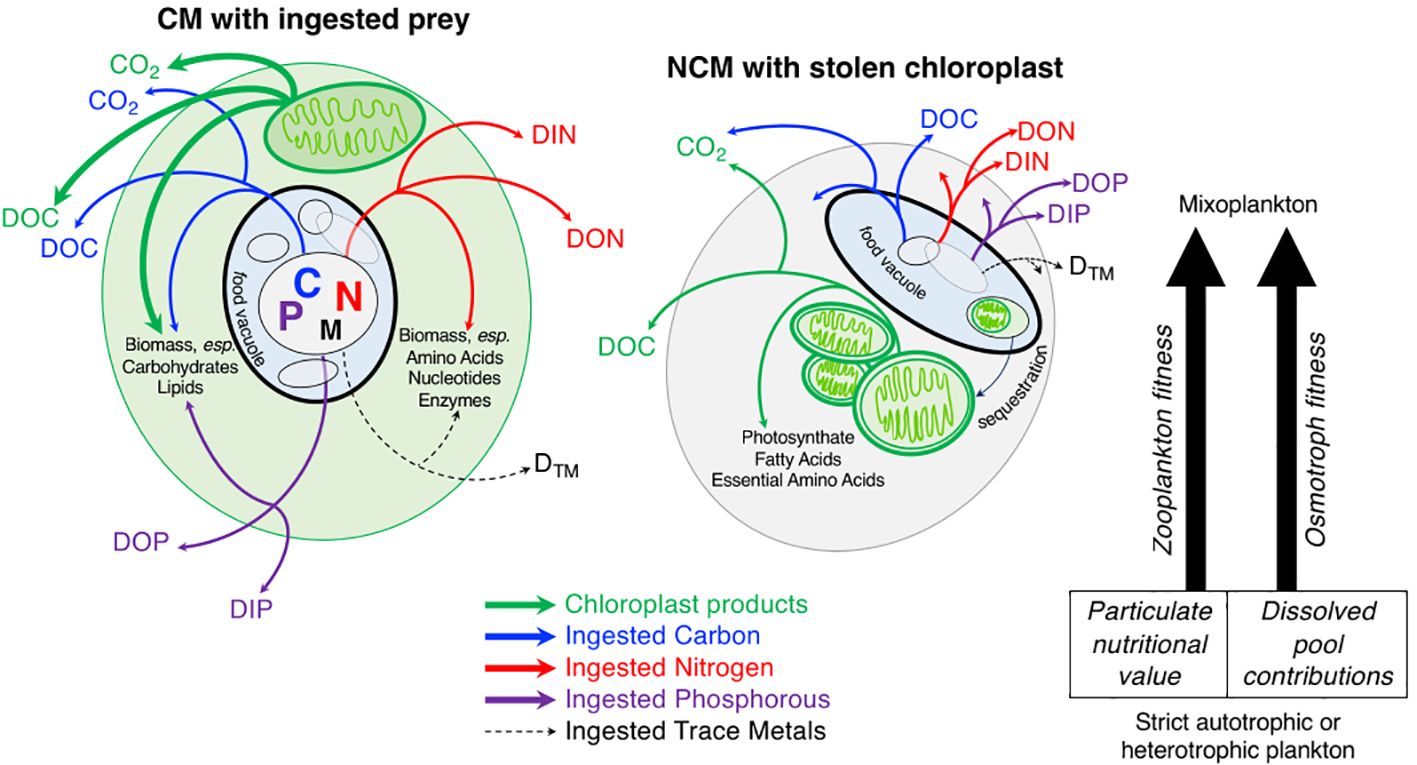
Figure 2 Following the fate of ingested particles and photosynthate products. On the left: a constitutive mixoplankton (CM) with ingested particles, arrows indicate possible fates of carbon (blue), nitrogen (red), phosphorus (purple), and trace metals (black dashed line) flux to cellular biomass or extracellular organic and inorganic material. On the right: a non-constitutive mixoplankton (NCM) with stolen chloroplast. The NCM depicted represents either a generalist or plastidic specialist NCM that acquires chloroplasts via kleptoplasty (Mitra et al., 2016). Endosymbiotic NCMs would have algae living in their cell that were providing photosynthate products (Mitra et al., 2016). There is currently almost no data on the contribution of both nutrient modes to cellular compounds. However, the limited analyses available suggests that the contribution from each nutrient mode would vary widely between species and environmental conditions for CMs and NCMs (Adolf et al., 2006; Terrado et al., 2017; Jeong et al., 2021). Green arrows indicate possible fates of photosynthates including cellular biomass such as plastidic-specific products like fatty acids and essential amino acids, or extracellular fate of any photosynthates used for cellular maintenance that are respired to inorganic carbon, or egestion/expulsion of plastid-produced organic carbon. In both cases CMs and NCMs engaged in mixotrophy have different contributions to the inorganic and organic dissolved pools compared to plankton not actively phagocytizing prey, with implications for the surrounding microbial community. Identifying and quantifying the relative fluxes of each pathway to track the fate of carbon and nutrients originating from ingested particles is the goal of section 5.1. Section 5.2 focuses on the impacts of active mixoplankton on surrounding zooplankton, if mixoplankton are better (or worse) prey particles than heterotrophic or autotrophic plankton.
New and emerging methods including high-energy imaging, labeling, and cell-sorting, will allow scientists to track the fate of ingested particles in order to understand the impact of mixotrophy on small-scale chemical changes to large-scale carbon and nutrient cycling. To illuminate the small-scale cellular impacts of mixotrophy, methods that quantify and locate intracellular elemental composition (including C, N, P), such as single-cell stable isotope probing using nanoSIMS (Mayali, 2020), energy dispersive X-ray microanalysis (Norland et al., 1995; Segura-Noguera et al., 2012, 2016) and synchrotron–based X-ray fluorescence microprobe (Twining et al., 2003) could provide insight about the effect of mixotrophic metabolism on mixoplankton stoichiometry. Meanwhile, quantifying the mass balance and stoichiometry of extracellular protist egesta is challenging (e.g., Nagata, 1996) but is needed to track the fate of ingested prey. In culture, stable and radioisotope tracer methods can track elements from labeled prey to cellular and extracellular material. In the field, the contributions of mixoplankton to the complex extracellular dissolved matter pool is much more challenging and would require isolation of protist species by flow cytometry or microscopy for both intracellular and extracellular analyses. To identify mixotrophic cells, the operator would have to target cells based on their size, chlorophyll fluorescence, and the presence of a vacuole (via vacuole staining). While the presence of ingested prey could also be used to identify mixotrophs (based on FLP also analyzed for their elemental composition), the contribution of prey to the mixotroph’s elemental composition would be difficult to tease apart in field samples.
Stable isotope probing of biomolecules or single cells is an emerging tool that can help track the activity of specific microbes in complex environments (Alcolombri et al., 2022). For example, Terrado et al. (2017) quantified mixotrophic carbon and nitrogen acquisition in Ochromonas sp. strain BG-1 by single-cell stable isotope probing using nanoSIMS. Applied to natural environments, such approaches can help estimate carbon and nutrient fluxes in food webs. Future applications would improve our understanding of the role of mixotrophy in elemental fluxes along the continuum of mixotrophic strategies. However, it is important to acknowledge that these methods are time intensive, expensive and require specialized instrumentation and trained personnel making them unsuitable for studies conducted over global scales. Therefore, future advancements in methodology should prioritize enhancing quantification accuracy at the community level across larger scales.
Method advancement is needed to overcome challenges associated with moving from controlled laboratory culture studies to in situ assessments of the intra- and extracellular biochemistry of mixed communities that engage in a variety of trophic modes. Complications could arise in natural abundance isotope measurements and stoichiometry assessments during attempts to attribute heterotrophic metabolism to phagotrophy if a mixoplankton is simultaneously incorporating significant amounts of dissolved carbon through osmotrophy (Godrijan et al., 2020, 2022), which can also alter the biochemistry (Cecchin et al., 2018) and isotope composition (Estep and Hoering, 1981; Zhang et al., 2009; Cormier et al., 2022). Additionally, it is important to note that the methods discussed here require a sample concentration step, whereby microorganisms are typically collected on a filter membrane, or retained by it to prepare concentrated liquid samples for flow cytometry cell sorting of low-abundance samples (Berthelot et al., 2019, 2021; Duhamel et al., 2019). A major issue is that an unknown, but likely large, fraction of protists are fragile, and cells can rupture even when using gentle filtration with either vacuum, syringe or peristaltic pumps. This creates a significant bias when trying to determine important parameters in the likely majority of mixotrophs in both laboratory and field studies. Methodological improvements are therefore needed to overcome this issue, which would open exciting new applications, especially in field studies where cell abundances are relatively low.
5.2 Are mixoplankton of different prey quality compared to phytoplankton and zooplankton?
It is hypothesized that the combined use of photosynthesis and phagotrophy will enhance trophic transfer efficiency of carbon and nutrients and bolster secondary production (Ward and Follows, 2016; Moorthi et al., 2017). However, the value (or detriment) of mixoplankton to higher trophic levels in the aquatic food web remains to be extensively tested (but see Traboni et al., 2020, 2021), and the nutritional quality of mixoplankton is debated (Traboni et al., 2021; Vad et al., 2021). Mixoplankton are theorized to be of superior food quality because they maintain more stable intracellular C:N:P ratios compared to phytoplankton despite environmental variability (Katechakis et al., 2005; Moorthi et al., 2017) and synthesize essential fatty acids and sterols at a higher concentration than strict heterotrophs (Boëchat et al., 2007). The stability of mixoplankton as a food source is complicated by variability in trophic plasticity (see section 3) and innate ability to produce chloroplasts (Mitra et al., 2016). Therefore, the biochemical composition of mixoplankton taxa, and thus viability as a food source, likely differs across the various mixoplankton functional groups and environmental gradients (see section 4). Though it remains difficult to estimate the relative contribution of photosynthesis versus phagotrophy to intracellular budgets, it is likely that trophic transitions between nutritional modes are accompanied by changes in the fatty acid and sterol signature of mixoplankton (Calderini et al., 2022). This is likely to be especially complex in NCMs that have varying capacities for acquired photoautotrophy (Johnson, 2011; Leles et al., 2017).
While protist nutritional value has been considered in relation to grazer health and productivity (Jónasdóttir, 1994; Jónasdóttir et al., 2002), baseline biochemical and stoichiometric profiles for mixoplankton taxa in relation to trophic strategy are lacking (Ma et al., 2022). It is necessary to start categorizing the C:N:P ratios, fatty acid, and sterol compositions of mixoplankton functional groups under different environmental conditions so we can begin to properly understand their quality as prey items and nutrient stability under abiotic and biotic stress (e.g., nutrient, light, and prey limitation). Traditional approaches to understanding predator-prey relationships, such as trophic markers (fatty acid composition, natural abundance amino acid 15N/14N ratios) and stable isotope probing can be employed to explore how mixotrophic ingestion processes can transform prey nutrients, but interpretation of results will also require basal profiles of said prey items (e.g., heterotrophic bacteria, phytoplankton) across varying environmental conditions. The nutritional quality of mixoplankton can be deduced by elemental analysis and stable isotope probing has been used to assess the nutritional profile of phytoplankton (Grosse et al., 2017). However, as described in 5.1, applying this approach in situ will require isolation and concentration of mixoplankton.
A more complete understanding of the nutritional quality of mixoplankton requires in situ studies that assess a combination of life history traits of micro- and meso-zooplankton grazers in response to varying prey items. Most studies offer mixotrophic prey in comparison to strict nutritional specialists (Jónasdóttir, 1994; Jónasdóttir et al., 2002; Jones et al., 2002). We suggest future work that quantifies fecundity (e.g. egg production, hatching success) and growth efficiency of zooplankton predators in conjunction with a single prey species in monoculture reared in conditions along the mixotrophic spectrum or varying proportions of phagotrophy and photoautotrophy ratios (Traboni et al., 2021). Given the potential for toxicity (Hiltunen et al., 2012; Tang et al., 2020) or inedibility (Vad et al., 2020) among mixoplankton, we also see a need for prey-preference assays (as in Castellani et al., 2008; Parrish et al., 2012), but focused on mixoplankton and their varying nutritional value as prey. Advances in flow cytometric sorting and imaging allow for discrimination between potential mixotrophic prey items in situ, which could allow for studies of prey selection in mixed assemblages, though the biochemical composition of mixoplankton as prey is largely understudied.
As new data become available on mixoplankton palatability, biochemistry, and trophic transfer efficiency, they can be used to parameterize ecosystem models. These mathematical models simulate abiotic and biotic components of marine ecosystems, linking nutrient availability to primary production and grazing (e.g., Darwin; Follows et al., 2007), and, in some cases, higher trophic levels (e.g., COBALT; Stock et al., 2014). To do so in a computationally tractable manner, these models typically collapse plankton diversity into fewer dimensions (i.e. functional groups and size classes) in order to represent the feedbacks between different trophic groups and carbon/nutrient cycles (Ward and Follows, 2016; Dutkiewicz et al., 2020; Leles et al., 2021; Serra-Pompei et al., 2022). Models vary in the degree of functional complexity they represent, and in the geographic scale modeled, from regional (Leles et al., 2021) to global (Ward and Follows, 2016). Although not all ecosystem models include representations of mixotrophy, those that do have already demonstrated the importance of mixotrophy to carbon cycling (Ward and Follows, 2016). However, these models’ accuracy hinges on the quality of input data. Thus, information on mixotroph palatability can directly affect trophic linkages built into models, mixoplankton stoichiometry can be used to estimate trophic transfer efficiency, and so on.
6 Conclusions
The field of mixoplankton and phago-mixotrophy research is full of potential. We have presented eight budding research questions related to four topics and have highlighted the integration of empirical approaches and different classes of models to address these questions. We believe that to fill current knowledge gaps and generate new hypotheses, we must combine different approaches, from using established methods in new ways and investing in developing in situ single-cell techniques to identify, isolate, and study mixoplankton cells, to developing models that evolve with our understanding of the system. However, in order to accomplish that, the number of scientists collecting data on mixoplankton, and the diversity of data types being collected needs to expand. Many of the methods mentioned here have been used to study mixoplankton in some capacity, but few have been applied to in situ mixoplankton communities. Currently, it is still necessary to test most methods using cultures under controlled laboratory conditions to understand the nuances of a method and improve it before applying it in situ. Data collected from culture experiments can then be used to model mixoplankton and fill in research gaps while empirical methods for in situ data are being improved and developed. The coming decade has the potential to produce mixoplankton research that will shift our foundational understanding of plankton ecology. Even for those not directly engaged in phago-mixotrophy research, it is essential to acknowledge the presence and influence of mixoplankton in aquatic datasets, fostering a more comprehensive understanding of aquatic ecosystems. We encourage scientists of all career stages to consider these ideas for inspiration.
Author contributions
NM: Writing – original draft, Writing – review & editing. SL: Writing – original draft, Writing – review & editing. MJ: Writing – original draft, Writing – review & editing. AM: Writing – original draft, Writing – review & editing. EB: Writing – original draft, Writing – review & editing. NC: Writing – original draft, Writing – review & editing. SD: Writing – original draft, Writing – review & editing. NP: Writing – original draft, Writing – review & editing. SP: Writing – original draft, Writing – review & editing. KS: Writing – original draft, Writing – review & editing. SW: Writing – original draft, Writing – review & editing. HM: Writing – original draft, Writing – review & editing.
Funding
The author(s) declare financial support was received for the research, authorship, and/or publication of this article. The Mixotrophs and Mixotrophy working group is supported by the Ocean Carbon Biogeochemistry (OCB) Project Office, which receives funding from the National Science Foundation (NSF) Chemical and Biological Oceanography Programs (OCE-1850983) and NASA Ocean Biology and Biogeochemistry Program (80NSSC21K0413). AM received funding through a postdoctoral fellowship from the Simons Foundation (732763). NC received support from NSF ANT-2240780, NM received support from NSF OCE-2230102 and NSF ANT-2240781, and KS received support from NSF OCE-2230103. SW was supported through a Vidi grant (no 193.101) from the Dutch Research Council (NWO). HM acknowledges funding from NSF (OCE-2237017). SL received funding through a postdoctoral fellowship from the Simons Foundation (877215).
Acknowledgments
We acknowledge helpful discussion and synthesis by the Ocean Carbon Biogeochemistry Mixotrophs & Mixotrophy working group, especially participants in our 2021 and 2022 working group meetings.
Conflict of interest
The authors declare that the research was conducted in the absence of any commercial or financial relationships that could be construed as a potential conflict of interest.
Publisher’s note
All claims expressed in this article are solely those of the authors and do not necessarily represent those of their affiliated organizations, or those of the publisher, the editors and the reviewers. Any product that may be evaluated in this article, or claim that may be made by its manufacturer, is not guaranteed or endorsed by the publisher.
References
Adolf J. E., Stoecker D. K., Harding L. W. Jr. (2006). The balance of autotrophy and heterotrophy during mixotrophic growth of Karlodinium micrum (Dinophyceae). J. Plank. Res. 28, 737–751. doi: 10.1093/plankt/fbl007
Agrawal A. A. (2020). A scale-dependent framework for trade-offs, syndromes, and specialization in organismal biology. Ecology. 101, e02924. doi: 10.1002/ecy.2924
Alcolombri U., Pioli R., Stocker R., Berry D. (2022). Single-cell stable isotope probing in microbial ecology. ISME Commun. 2, 1–9. doi: 10.1038/s43705-022-00142-3
Alexander H., Hu S. K., Krinos A. I., Pachiadaki M., Tully B. J., Neely C. J., et al. (2023). Eukaryotic genomes from a global metagenomic dataset illuminate trophic modes and biogeography of ocean plankton. mBio 14 (6), e01676-23. doi: 10.1101/2021.07.25.453713
Andersen K. H., Aksnes D. L., Berge T., Fiksen Ø., Visser A. (2015). Modelling emergent trophic strategies in plankton. J. Plankton Res. 37, 862–868. doi: 10.1093/plankt/fbv054
Anderson R., Jürgens K., Hansen P. J. (2017). Mixotrophic phytoflagellate bacterivory field measurements strongly biased by standard approaches: a case study. Front. Microbiol. 8. doi: 10.3389/fmicb.2017.01398
Anschütz A.-A., Flynn K. J. (2020). Niche separation between different functional types of mixoplankton: results from NPZ-style N-based model simulations. Mar. Biol. 167, 3. doi: 10.1007/s00227-019-3612-3
Anschütz A.-A., Maselli M., Traboni C., Boon A. R., Stolte W. (2024). Importance of integrating mixoplankton into marine ecosystem policy and management - examples from the Marine Strategy Framework Directive. Integrated Environ. Assess. Manage. doi: 10.1002/ieam.4914. [Epub ahead of print].
Barbaglia G. S., Paight C., Honig M., Johnson M. D., Marczak R., Lepori-Bui M., et al. (2024). Environment-dependent metabolic investments in the mixotrophic chrysophyte Ochromonas. J. Phycol. 60, 170–184. doi: 10.1111/jpy.13418
Barton A. D., Irwin A. J., Finkel Z. V., Stock C. A. (2016). Anthropogenic climate change drives shift and shuffle in North Atlantic phytoplankton communities. Proc. Natl. Acad. Sci. 113, 2964–2969. doi: 10.1073/pnas.1519080113
Barton A. D., Pershing A. J., Litchman E., Record N. R., Edwards K. F., Finkel Z. V., et al. (2013). The biogeography of marine plankton traits. Ecol. Lett. 16, 522–534. doi: 10.1111/ele.12063
Batten S. D., Raitsos D. E., Danielson S., Hopcroft R., Coyle K., McQuatters-Gollop A. (2018). Interannual variability in lower trophic levels on the Alaskan Shelf. Deep Sea Res. Part II Top. Stud. Oceanogr. 147, 58–68. doi: 10.1016/j.dsr2.2017.04.023
Beisner B. E., Grossart H.-P., Gasol J. M. (2019). A guide to methods for estimating phago-mixotrophy in nanophytoplankton. J. Plankton Res. 41, 77–89. doi: 10.1093/plankt/fbz008
Berge T., Chakraborty S., Hansen P. J., Andersen K. H. (2017). Modeling succession of key resource-harvesting traits of mixotrophic plankton. ISME J. 11, 212–223. doi: 10.1038/ismej.2016.92
Berge T., Poulsen L. K., Moldrup M., Daugbjerg N., Juel Hansen P. (2012). Marine microalgae attack and feed on metazoans. ISME J. 6, 1926–1936. doi: 10.1038/ismej.2012.29
Berthelot H., Duhamel S., L’Helguen S., Maguer J., Cassar N. (2021). Inorganic and organic carbon and nitrogen uptake strategies of picoplankton groups in the northwestern Atlantic Ocean. Limnol. Oceanogr. 66, 3682–3696. doi: 10.1002/lno.11909
Berthelot H., Duhamel S., L’Helguen S., Maguer J.-F., Wang S., Cetinić I., et al. (2019). NanoSIMS single cell analyses reveal the contrasting nitrogen sources for small phytoplankton. ISME J. 13, 651–662. doi: 10.1038/s41396-018-0285-8
Biecheler B. (1936). Observation de la capture et de la digestion des proies chez un Péridinien vert. CR Séances Soc. Biol. 122, 1173–1175.
Bird D. F., Kalff J. (1986). Bacterial grazing by planktonic lake algae. Science 231, 493–495. doi: 10.1126/science.231.4737.493
Blossom H. E., Hansen P. J. (2021). The loss of mixotrophy in Alexandrium pseudogonyaulax: Implications for trade-offs between toxicity, mucus trap production, and phagotrophy. Limnol. Oceanogr. 66, 528–542. doi: 10.1002/lno.11621
Bock N. A., Charvet S., Burns J., Gyaltshen Y., Rozenberg A., Duhamel S., et al. (2021). Experimental identification and in silico prediction of bacterivory in green algae. ISME J. 15, 1987–2000. doi: 10.1038/s41396-021-00899-w
Boëchat I. G., Weithoff G., Krüger A., Gücker B., Adrian R. (2007). A biochemical explanation for the success of mixotrophy in the flagellate. Ochromonas sp. Limnol. Oceanogr. 52, 1624–1632. doi: 10.4319/lo.2007.52.4.1624
Boenigk J., Matz C., Jürgens K., Arndt H. (2002). Food concentration-dependent regulation of food selectivity of interception-feeding bacterivorous nanoflagellates. Aquat. Microb. Ecol. 27, 195–202. doi: 10.3354/ame027195
Brown A. L., Casarez G. A., Moeller H. V. (2023). Acquired phototrophy as an evolutionary path to mixotrophy. Am. Nat. 202, 458–470. doi: 10.1086/725918
Brun P., Kiørboe T., Licandro P., Payne M. R. (2016). The predictive skill of species distribution models for plankton in a changing climate. Glob. Change Biol. 22, 3170–3181. doi: 10.1111/gcb.13274
Burns J. A., Paasch A., Narechania A., Kim E. (2015). Comparative genomics of a bacterivorous green alga reveals evolutionary causalities and consequences of phago-mixotrophic mode of nutrition. Genome Biol. Evol. 7, 3047–3061. doi: 10.1093/gbe/evv144
Burns J. A., Pittis A. A., Kim E. (2018). Gene-based predictive models of trophic modes suggest Asgard archaea are not phagocytotic. Nat. Ecol. Evol. 2, 697–704. doi: 10.1038/s41559-018-0477-7
Calbet A., Bertos M., Fuentes-Grünewald C., Alacid E., Figueroa R., Renom B., et al. (2011). Intraspecific variability in Karlodinium veneficum: Growth rates, mixotrophy, and lipid composition. Harmful Algae 10, 654–667. doi: 10.1016/j.hal.2011.05.001
Calderini M. L., Salmi P., Rigaud C., Peltomaa E., Taipale S. J. (2022). Metabolic plasticity of mixotrophic algae is key for their persistence in browning environments. Mol. Ecol. 31, 4726–4738. doi: 10.1111/mec.16619
Cardol P., Forti G., Finazzi G. (2011). Regulation of electron transport in microalgae. Biochim. Biophys. Acta BBA - Bioenerg. 1807, 912–918. doi: 10.1016/j.bbabio.2010.12.004
Castellani C., Irigoien X., Mayor D. J., Harris R. P., Wilson D. (2008). Feeding of Calanus finmarchicus and Oithona similis on the microplankton assemblage in the Irminger Sea, North Atlantic. J. Plankton Res. 30, 1095–1116. doi: 10.1093/plankt/fbn074
Cecchin M., Benfatto S., Griggio F., Mori A., Cazzaniga S., Vitulo N., et al. (2018). Molecular basis of autotrophic vs mixotrophic growth in Chlorella sorokiniana. Sci. Rep. 8, 6465. doi: 10.1038/s41598–018-24979–8
Chakraborty S., Cadier M., Visser A. W., Bruggeman J., Andersen K. H. (2020). Latitudinal variation in plankton traits and ecosystem function. Glob. Biogeochem. Cycles 34, e2020GB006564. doi: 10.1029/2020GB006564
Chakraborty S., Nielsen L. T., Andersen K. H. (2017). Trophic strategies of unicellular plankton. Am. Nat. 189, E77–E90. doi: 10.1086/690764
Cohen N. R., McIlvin M. R., Moran D. M., Held N. A., Saunders J. K., Hawco N. J., et al. (2021). Dinoflagellates alter their carbon and nutrient metabolic strategies across environmental gradients in the central Pacific Ocean. Nat. Microbiol. 6, 173–186. doi: 10.1038/s41564-020-00814-7
Colin S., Coelho L. P., Sunagawa S., Bowler C., Karsenti E., Bork P., et al. (2017). Quantitative 3D-imaging for cell biology and ecology of environmental microbial eukaryotes. eLife 6, e26066. doi: 10.7554/eLife.26066.043
Cormier M., Berard J., Bougaran G., Trueman C. N., Mayor D. J., Lampitt R. S., et al. (2022). Deuterium in marine organic biomarkers: toward a new tool for quantifying aquatic mixotrophy. New Phytol. 234, 776–782. doi: 10.1111/nph.18023
Crane K. W., Grover J. P. (2010). Coexistence of mixotrophs, autotrophs, and heterotrophs in planktonic microbial communities. J. Theor. Biol. 262, 517–527. doi: 10.1016/j.jtbi.2009.10.027
Delmont T. O., Gaia M., Hinsinger D. D., Frémont P., Vanni C., Fernandez-Guerra A., et al. (2022). Functional repertoire convergence of distantly related eukaryotic plankton lineages abundant in the sunlit ocean. Cell Genomics 2, 100123. doi: 10.1016/j.xgen.2022.100123
Dobbertin da Costa M., Gast R. J., Millette N. C. (2024). Temporal and spatial variability of constitutive mixotroph abundance and proportion. FEMS Microbiol. Ecol. 100, fiae015. doi: 10.1093/femsec/fiae015
Dolan J. R., Pérez M. T. (2000). Costs, benefits and characteristics of mixotrophy in marine oligotrichs: Mixotrophy in marine oligotrichs. Freshw. Biol. 45, 227–238. doi: 10.1046/j.1365-2427.2000.00659.x
Dölger J., Nielsen L. T., Kiørboe T., Andersen A. (2017). Swimming and feeding of mixotrophic biflagellates. Sci. Rep. 7, 39892. doi: 10.1038/srep39892
Dorrell R. G., Azuma T., Nomura M., Audren de Kerdrel G., Paoli L., Yang S., et al. (2019). Principles of plastid reductive evolution illuminated by nonphotosynthetic chrysophytes. Proc. Natl. Acad. Sci. 116, 6914–6923. doi: 10.1073/pnas.1819976116
Duhamel S., Kim E., Sprung B., Anderson O. R. (2019). Small pigmented eukaryotes play a major role in carbon cycling in the P-depleted western subtropical North Atlantic, which may be supported by mixotrophy. Limnol. Oceanogr. 64, 2424–2440. doi: 10.1002/lno.11193
Dutkiewicz S., Boyd P. W., Riebesell U. (2021). Exploring biogeochemical and ecological redundancy in phytoplankton communities in the global ocean. Glob. Change Biol. 27, 1196–1213. doi: 10.1111/gcb.15493
Dutkiewicz S., Cermeno P., Jahn O., Follows M. J., Hickman A. E., Taniguchi D. A. A., et al. (2020). Dimensions of marine phytoplankton diversity. Biogeosciences 17, 609–634. doi: 10.5194/bg-17-609-2020
Dutkiewicz S., Follett C. L., Follows M. J., Henderikx-Freitas F., Ribalet F., Gradoville M. R., et al. (2024). Multiple biotic interactions establish phytoplankton community structure across environmental gradients. Limnol Oceanogr. doi: 10.1002/lno.12555. [Epub ahead of print].
Edwards K. F. (2019). Mixotrophy in nanoflagellates across environmental gradients in the ocean. Proc. Natl. Acad. Sci. 116, 6211–6220. doi: 10.1073/pnas.1814860116
Edwards K. F., Li Q., McBeain K. A., Schvarcz C. R., Steward G. F. (2023a). Trophic strategies explain the ocean niches of small eukaryotic phytoplankton. Proc. R. Soc B 290, 20222021. doi: 10.1098/rspb.2022.2021
Edwards K. F., Li Q., Steward G. F. (2023b). Ingestion kinetics of mixotrophic and heterotrophic flagellates. Limnol. Oceanogr. 68, 917–927. doi: 10.1002/lno.12320
Edwards K. F., Thomas M. K., Klausmeier C. A., Litchman E. (2012). Allometric scaling and taxonomic variation in nutrient utilization traits and maximum growth rate of phytoplankton. Limnol. Oceanogr. 57, 554–566. doi: 10.4319/lo.2012.57.2.0554
Estep M. F., Hoering T. C. (1981). Stable hydrogen isotope fractionations during autotrophic and mixotrophic growth of microalgae. Plant Physiol. 67, 474–477. doi: 10.1104/pp.67.3.474
Faktorová D., Nisbet R. E. R., Fernández Robledo J. A., Casacuberta E., Sudek L., Allen A. E., et al. (2020). Genetic tool development in marine protists: emerging model organisms for experimental cell biology. Nat. Methods 17, 481–494. doi: 10.1038/s41592-020-0796-x
Faure E., Not F., Benoiston A.-S., Labadie K., Bittner L., Ayata S.-D. (2019). Mixotrophic protists display contrasted biogeographies in the global ocean. ISME J. 13, 1072–1083. doi: 10.1038/s41396-018-0340-5
Fay S. A., Gast R. J., Sanders R. W. (2013). Linking bacterivory and phyletic diversity of protists with a marker gene survey and experimental feeding with BrdU-labeled bacteria. Aquat. Microb. Ecol. 71, 141–153. doi: 10.3354/ame01674
Ferreira G. D., Grigoropoulou A., Saiz E., Calbet A. (2022). The effect of short-term temperature exposure on vital physiological processes of mixoplankton and protozooplankton. Mar. Environ. Res. 179. doi: 10.1016/j.marenvres.2022.105693
Ferreira P. S., Santos G. A., Souza I.T.de, Neiro S.M.da S., Cardoso V. L., Batista F. R. X. (2021). Mixotrophic algal-protein production and techno-economic analysis based in medium formulation. Res. Soc Dev. 10, e54110414186. doi: 10.33448/rsd-v10i4.14186
Fischer R., Kitzwögerer J., Ptacnik R. (2022). Light-dependent niche differentiation in two mixotrophic bacterivores. Environ. Microbiol. Rep. 14, 530–537. doi: 10.1111/1758-2229.13071
Flynn K. J., Mitra A., Anestis K., Anschütz A. A., Calbet A., Ferreira G. D., et al. (2019). Mixotrophic protists and a new paradigm for marine ecology: where does plankton research go now? J. Plankton Res. 41, 375–391. doi: 10.1093/plankt/fbz026
Flynn K. J., Stoecker D. K., Mitra A., Raven J. A., Glibert P. M., Hansen P. J., et al. (2013). Misuse of the phytoplankton–zooplankton dichotomy: the need to assign organisms as mixotrophs within plankton functional types. J. Plankton Res. 35, 3–11. doi: 10.1093/plankt/fbs062
Follows M. J., Dutkiewicz S., Grant S., Chisholm S. W. (2007). Emergent biogeography of microbial communities in a model ocean. Science 315, 1843–1846. doi: 10.1126/science.1138544
Fowler B. L., Neubert M. G., Hunter-Cevera K. R., Olson R. J., Shalapyonok A., Solow A. R., et al. (2020). Dynamics and functional diversity of the smallest phytoplankton on the Northeast US Shelf. Proc. Natl. Acad. Sci. 117, 12215–12221. doi: 10.1073/pnas.1918439117
Frias-Lopez J., Thompson A., Waldbauer J., Chisholm S. W. (2009). Use of stable isotope-labelled cells to identify active grazers of picocyanobacteria in ocean surface waters. Environ. Microbiol. 11, 512–525. doi: 10.1111/j.1462–2920.2008.01793.x
Gast R. J., Fay F. A., Sanders R. W. (2018). Mixotrophic activity and diversity of Antarctic marine protists in Austral summer. Front. Mar. Sci. 5. doi: 10.3389/fmars.2018.00013
Gast R. J., McKie-Krisberg Z. M., Fay S. A., Rose J. M., Sanders R. W. (2014). Antarctic mixotrophic protist abundances by microscopy and molecular methods. FEMS Microbiol. Ecol. 89, 388–401. doi: 10.1111/fem.2014.89.issue-2
Gawryluk R. M. R., del Campo J., Okamoto N., Strassert J. F. H., Lukeš J., Richards T. A., et al. (2016). Morphological identification and single-cell genomics of marine diplonemids. Curr. Biol. 26, 3053–3059. doi: 10.1016/j.cub.2016.09.013
Ghyoot C., Lancelot C., Flynn K. J., Mitra A., Gypens N. (2017). Introducing mixotrophy into a biogeochemical model describing an eutrophied coastal ecosystem: The Southern North Sea. Prog. Oceanogr. 157, 1–11. doi: 10.1016/j.pocean.2017.08.002
Godrijan J., Drapeau D., Balch W. M. (2020). Mixotrophic uptake of organic compounds by coccolithophores. Limnol. Oceanogr. 65, 1410–1421. doi: 10.1002/lno.11396
Godrijan J., Drapeau D. T., Balch W. M. (2022). Osmotrophy of dissolved organic carbon by coccolithophores in darkness. New Phytol. 233, 781–794. doi: 10.1111/nph.17819
Gonzalez L. M., Proulx S. R., Moeller H. V. (2022). Modeling the metabolic evolution of mixotrophic phytoplankton in response to rising ocean surface temperatures. BMC Ecol. Evol. 22, 136. doi: 10.1186/s12862-022-02092-9
González-Gil S., Keafer B. A., Jovine R. V. M., Aguilera A., Lu S., Anderson D. M. (1998). Detection and quantification of alkaline phosphatase in single cells of phosphorus-starved marine phytoplankton. Mar. Ecol. Prog. Ser. 164, 21–35. doi: 10.3354/meps164021
González-Olalla J. M., Medina-Sánchez J. M., Carrillo P. (2019). Mixotrophic trade-off under warming and UVR in a marine and a freshwater alga. J. Phycol. 55, 1028–1040. doi: 10.1111/jpy.12865
Grob C., Hartmann M., Zubkov M. V., Scanlan D. J. (2011). Invariable biomass-specific primary production of taxonomically discrete picoeukaryote groups across the Atlantic Ocean: Invariable primary production of picoeukaryotes. Environ. Microbiol. 13, 3266–3274. doi: 10.1111/j.1462-2920.2011.02586.x
Grosse J., van Breugel P., Brussaard C. P. D., Boschker H. T. S. (2017). A biosynthesis view on nutrient stress in coastal phytoplankton: Algal biomolecules under N/P limitation. Limnol. Oceanogr. 62, 490–506. doi: 10.1002/lno.10439
Grujcic V., Nuy J. K., Salcher M. M., Shabarova T., Kasalicky V., Boenigk J., et al. (2018). Cryptophyta as major bacterivores in freshwater summer plankton. ISME J. 12, 1668–1681. doi: 10.1038/s41396-018-0057-5
Gsell A. S., Özkundakci D., Hébert M.-P., Adrian R. (2016). Quantifying change in pelagic plankton network stability and topology based on empirical long-term data. Ecol. Indic. 65, 76–88. doi: 10.1016/j.ecolind.2015.11.014
Hansen P. J., Bjørnsen P. K., Hansen B. W. (1997). Zooplankton grazing and growth: Scaling within the 2–2,-μm body size range. Limnol. Oceanogr. 42, 687–704. doi: 10.4319/lo.1997.42.4.0687
Hansson T. H., Grossart H.-P., del Giorgio P. A., St-Gelais N. F., Beisner B. E. (2019). Environmental drivers of mixotrophs in boreal lakes. Limnol. Oceanogr. 64, 1688–1705. doi: 10.1002/lno.11144
Hartmann M., Grob C., Scanlan D. J., Martin A. P., Burkill P. H., Zubkov M. V. (2011). Comparison of phosphate uptake rates by the smallest plastidic and aplastidic protists in the North Atlantic subtropical gyre: Phosphate uptake by oceanic protists. FEMS Microbiol. Ecol. 78, 327–335. doi: 10.1111/j.1574-6941.2011.01160.x
Hartmann M., Grob C., Tarran G. A., Martin A. P., Burkill P. H., Scanlan D. J., et al. (2012). Mixotrophic basis of Atlantic oligotrophic ecosystems. Proc. Natl. Acad. Sci. 109, 5756–5760. doi: 10.1073/pnas.1118179109
Hattenrath-Lehmann T. K., Nanjappa D., Zhang H., Yu L., Goleski J. A., Lin S., et al. (2021). Transcriptomic and isotopic data reveal central role of ammonium in facilitating the growth of the mixotrophic dinoflagellate, Dinophysis acuminata. Harmful Algae 104, 102031. doi: 10.1016/j.hal.2021.102031
Hernández Fariñas T., Bacher C., Soudant D., Belin C., Barillé L. (2015). Assessing phytoplankton realized niches using a French national phytoplankton monitoring network. Estuar. Coast. Shelf Sci. 159, 15–27. doi: 10.1016/j.ecss.2015.03.010
Hiltunen T., Barreiro A., Hairston N. G. (2012). Mixotrophy and the toxicity of Ochromonas in pelagic food webs: Toxicity of a mixotroph to competitors and consumers. Freshw. Biol. 57, 2262–2271. doi: 10.1111/fwb.12000
Hunter-Cevera K. R., Neubert M. G., Olson R. J., Solow A. R., Shalapyonok A., Sosik H. M. (2016). Physiological and ecological drivers of early spring blooms of a coastal phytoplankter. Science 354, 326–329. doi: 10.1126/science.aaf8536
Jardillier L., Zubkov M. V., Pearman J., Scanlan D. J. (2010). Significant CO2 fixation by small prymnesiophytes in the subtropical and tropical northeast Atlantic Ocean. ISME J. 4, 1180–1192. doi: 10.1038/ismej.2010.36
Jeong H. J., Kang H. C., Lim A. S., Jang S. H. (2021). Feeding diverse prey as an excellent strategy of mixotrophic dinoflagellates for global dominance. Sci. Adv. 7, eabe4214. doi: 10.1126/sciadv.abe4214
Jeong H. J., Yoo Y. D., Kim J. S., Seong K. A., Kan N. S., Kim T. H. (2010). Growth, feeding and ecological roles of the mixotrophic and heterotrophic dinoflagellates in marine planktonic food webs. Ocean Sci. J. 45, 65–91. doi: 10.1007/s12601-010-0007-2
Jeong H. J., Yoo Y. D., Park J. Y., Song J. Y., Kim S. T., Lee S. H., et al. (2005). Feeding by phototrophic red-tide dinoflagellates: five species newly revealed and six species previously known to be mixotrophic. Aquat. Microb. Ecol. 40, 133–150. doi: 10.3354/ame040133
Johnson M. D. (2011). The acquisition of phototrophy: adaptive strategies of hosting endosymbionts and organelles. Photosynth. Res. 107, 117–132. doi: 10.1007/s11120-010-9546-8
Johnson M. D., Moeller H. V., Paight C., Kellogg R. M., McIlvin M. R., Saito M. A., et al. (2023). Functional control and metabolic integration of stolen organelles in a photosynthetic ciliate. Curr. Biol. 33, 973–980.e5. doi: 10.1016/j.cub.2023.01.027
Jónasdóttir S. H. (1994). Effects of food quality on the reproductive success of Acartia tonsa and Acartia hudsonica: laboratory observations. Mar. Biol. 121, 67–81. doi: 10.1007/BF00349475
Jónasdóttir S., Gudfinnsson H., Gislason A., Astthorsson O. (2002). Diet composition and quality for Calanus finmarchicus egg production and hatching success off south-west Iceland. Mar. Biol. 140, 1195–1206. doi: 10.1007/s00227-002-0782-0
Jones R., Flynn K., Anderson T. (2002). Effect of food quality on carbon and nitrogen growth efficiency in the copepod Acartia tonsa. Mar. Ecol. Prog. Ser. 235, 147–156. doi: 10.3354/meps235147
Katechakis A., Haseneder T., Kling R., Stibor H. (2005). Mixotrophic versus photoautotrophic specialist algae as food for zooplankton: The light : nutrient hypothesis might not hold for mixotrophs. Limnol. Oceanogr. 50, 1290–1299. doi: 10.4319/lo.2005.50.4.1290
Kim E., Maruyama S. (2014). A contemplation on the secondary origin of green algal and plant plastids. Acta Societatis Botanicorum Poloniae. 83, 331–336. doi: 10.5586/asbp.2014.040
Kiørboe T., Thomas M. K. (2020). Heterotrophic eukaryotes show a slow-fast continuum, not a gleaner–exploiter trade-off. Proc. Natl. Acad. Sci. 117, 24893–24899. doi: 10.1073/pnas.2008370117
Kolisko M., Boscaro V., Burki F., Lynn D. H., Keeling P. J. (2014). Single-cell transcriptomics for microbial eukaryotes. Curr. Biol. 24, R1081–R1082. doi: 10.1016/j.cub.2014.10.026
Koppelle S., López-Escardó D., Brussaard C. P. D., Huisman J., Philippart C. J. M., Massana R., et al. (2022). Mixotrophy in the bloom-forming genus Phaeocystis and other haptophytes. Harmful Algae 117, 102292. doi: 10.1016/j.hal.2022.102292
Ku C., Sebé-Pedrós A. (2019). Using single-cell transcriptomics to understand functional states and interactions in microbial eukaryotes. Philos. Trans. R. Soc B Biol. Sci. 374, 20190098. doi: 10.1098/rstb.2019.0098
Labarre A., Obiol A., Wilken S., Forn I., Massana R. (2019). Expression of genes involved in phagocytosis in uncultured heterotrophic flagellates. Limnol. Oceanogr. 65, S149–S160. doi: 10.1002/lno.11379
Lambert B. S., Groussman R. D., Schatz M. J., Coesel S. N., Durham B. P., Alverson A. J., et al. (2022). The dynamic trophic architecture of open-ocean protist communities revealed through machine-guided metatranscriptomics. Proc. Natl. Acad. Sci. 119, e2100916119. doi: 10.1073/pnas.2100916119
Leles S. G., Bruggeman J., Polimene L., Blackford J., Flynn K. J., Mitra A. (2021). Differences in physiology explain succession of mixoplankton functional types and affect carbon fluxes in temperate seas. Prog. Oceanogr. 190, 102481. doi: 10.1016/j.pocean.2020.102481
Leles S. G., Levine N. M. (2023). Mechanistic constraints on the trade-off between photosynthesis and respiration in response to warming. Sci. Adv. 9, eadh8043. doi: 10.1126/sciadv.adh8043
Leles S. G., Mitra A., Flynn K. J., Stoecker D. K., Hansen P. J., Calbet A., et al. (2017). Oceanic protists with different forms of acquired phototrophy display contrasting biogeographies and abundance. Proc. R. Soc B Biol. Sci. 284, 20170664. doi: 10.1098/rspb.2017.0664
Leles S. G., Mitra A., Flynn K. J., Tillmann U., Stoecker D., Jeong H. J., et al. (2019). Sampling bias misrepresents the biogeographical significance of constitutive mixotrophs across global oceans. Glob. Ecol. Biogeogr. 28, 418–428. doi: 10.1111/geb.12853
Leles S. G., Polimene L., Bruggeman J., Blackford J., Ciavatta S., Mitra A., et al. (2018). Modelling mixotrophic functional diversity and implications for ecosystem function. J. Plankton Res. 40, 627–642. doi: 10.1093/plankt/fby044
Lepori-Bui M., Paight C., Eberhard E., Mertz C. M., Moeller H. V. (2022). Evidence for evolutionary adaptation of mixotrophic nanoflagellates to warmer temperatures. Glob. Change Biol. 28, 7094–7107. doi: 10.1111/gcb.16431
Li M., Chen Y., Zhang F., Song Y., Glibert P. M., Stoecker D. K. (2022). A three-dimensional mixotrophic model of Karlodinium veneficum blooms for a eutrophic estuary. Harmful Algae 113, 102203. doi: 10.1016/j.hal.2022.102203
Li Q., Edwards K. F., Schvarcz C. R., Selph K. E., Steward G. F. (2021b). Plasticity in the grazing ecophysiology of Florenciella (Dichtyochophyceae), a mixotrophic nanoflagellate that consumes Prochlorococcus and other bacteria. Limnol. Oceanogr. 66, 47–60. doi: 10.1002/lno.11585
Li H., Li L., Yu L., Yang X., Shi X., Wang J., et al. (2021a). Transcriptome profiling reveals versatile dissolved organic nitrogen utilization, mixotrophy, and N conservation in the dinoflagellate Prorocentrum shikokuense under N deficiency. Sci. Total Environ. 763, 143013. doi: 10.1016/j.scitotenv.2020.143013
Li A., Stoecker D. K., Coats D. W. (2000). Spatial and temporal aspects of Gyrodinium galatheanum in Chesapeake Bay: distribution and mixotrophy. J. Plankton Res. 22, 2105–2124. doi: 10.1093/plankt/22.11.2105
Lie A. A. Y., Liu Z., Terrado R., Tatters A. O., Heidelberg K. B., Caron D. A. (2018). A tale of two mixotrophic chrysophytes: Insights into the metabolisms of two Ochromonas species (Chrysophyceae) through a comparison of gene expression. PloS One 13, e0192439. doi: 10.1371/journal.pone.0192439
Lim A. S., Jeong H. J., Ok J. H., You J. H., Kang H. C., Kim S. J. (2019). Effects of light intensity and temperature on growth and ingestion rates of the mixotrophic dinoflagellate Alexandrium pohangense. Mar. Biol. 166, 98. doi: 10.1007/s00227-019-3546-9
Lin C.-H., Flynn K. J., Mitra A., Glibert P. M. (2018). Simulating effects of variable stoichiometry and temperature on mixotrophy in the harmful dinoflagellate Karlodinium veneficum. Front. Mar. Sci. 5. doi: 10.3389/fmars.2018.00320
Lin C.-H., Glibert P. M. (2019). Mixotrophy with multiple prey species measured with a multiwavelength-excitation PAM fluorometer: case study of Karlodinium veneficum. J. Plankton Res. 41, 46–62. doi: 10.1093/plankt/fby049
Liu Z., Campbell V., Heidelberg K. B., Caron D. A. (2016). Gene expression characterizes different nutritional strategies among three mixotrophic protists. FEMS Microbology Ecol. 92, fiw106. doi: 10.1093/femsec/fiw106
Liu Z., Jones A. C., Campbell V., Hambright K. D., Heidelberg K. B., Caron D. A. (2015). Gene expression in the mixotrophic prymnesiophyte, Prymnesium parvum, responds to prey availability. Front. Microbiol. 6. doi: 10.3389/fmicb.2015.00319
Ma M., Wei C., Chen M., Wang H., Gong Y., Hu Q. (2022). Effects of nutritional mode on the physiological and biochemical characteristics of the mixotrophic flagellate Poterioochromonas malhamensis and the potential ecological implications. Microorganisms 10, 852. doi: 10.3390/microorganisms10050852
Maloney A. E., Kopf S. H., Zhang Z., McFarlin J., Nelson D. B., Masterson A. L., et al. (2024). Large enrichments in fatty acid 2H/1H ratios distinguish respiration from aerobic fermentation in yeast Saccharomyces cerevisiae. Proc. Natl. Acad. Sci. 121, e2310771121. doi: 10.1073/pnas.2310771121
Mansour J. S., Anestis K. (2021). Eco-evolutionary perspectives on mixoplankton. Front. Mar. Sci. 8. doi: 10.3389/fmars.2021.666160
Massana R., Unrein F., Rodríguez-Martínez R., Forn I., Lefort T., Pinhassi J., et al. (2009). Grazing rates and functional diversity of uncultured heterotrophic flagellates. ISME J. 3, 588–596. doi: 10.1038/ismej.2008.130
Mayali X. (2020). NanoSIMS: Microscale quantification of biogeochemical activity with large-Scale impacts. Annu. Rev. Mar. Sci. 12, 449–467. doi: 10.1146/annurev-marine-010419-010714
Mayali X., Weber P. K., Brodie E. L., Mabery S., Hoeprich P. D., Pett-Ridge J. (2012). High-throughput isotopic analysis of RNA microarrays to quantify microbial resource use. ISME J. 6, 1210–1221. doi: 10.1038/ismej.2011.175
McCain J. S. P., Tagliabue A., Susko E., Achterberg E. P., Allen A. E., Bertrand E. M. (2021). Cellular costs underpin micronutrient limitation in phytoplankton. Sci. Adv. 7, eabg6501. doi: 10.1126/sciadv.abg6501
McKie-Krisberg Z. M., Gast R. J., Sanders R. W. (2015). Physiological responses of three species of antarctic mixotrophic phytoflagellates to changes in light and dissolved nutrients. Microb. Ecol. 70, 21–29. doi: 10.1007/s00248-014-0543-x
McManus G. B., Fuhrman J. A. (1986). Bacterivory in seawater studied with the use of inert fluorescent particles. Limnol. Oceanogr. 31, 420–426. doi: 10.4319/lo.1986.31.2.0420
Millette N. C., Costa M., Mora J. W., Gast R. J. (2021). Temporal and spatial variability of phytoplankton and mixotrophs in a temperate estuary. Mar. Ecol. Prog. Ser. 677, 17–31. doi: 10.3354/meps13850
Millette N. C., Gast R. J., Luo J. Y., Moeller H. V., Stamieszkin K., Andersen K. H., et al. (2023). Mixoplankton and mixotrophy: future research priorities. J. Plankton Res. 45, 576–596. doi: 10.1093/plankt/fbad020
Millette N. C., Grosse J., Johnson W. M., Jungbluth M. J., Suter E. A. (2018). Hidden in plain sight: The importance of cryptic interactions in marine plankton. Limnol. Oceanogr. Lett. 3, 341–356. doi: 10.1002/lol2.10084
Millette N. C., Pierson J. J., Aceves A., Stoecker D. K. (2017). Mixotrophy in Heterocapsa rotundata: A mechanism for dominating the winter phytoplankton. Limnol. Oceanogr. 62, 836–845. doi: 10.1002/lno.10470
Mitra A., Caron D. A., Faure E., Flynn K. J., Leles S. G., Hansen P. J., et al. (2023a). The Mixoplankton Database (MDB): Diversity of photo-phago-trophic plankton in form, function, and distribution across the global ocean. J. Eukaryot. Microbiol. 70, e12972. doi: 10.1111/jeu.12972
Mitra A., Flynn K. J., Burkholder J. M., Berge T., Calbet A., Raven J. A., et al. (2014). The role of mixotrophic protists in the biological carbon pump. Biogeosciences 11, 995–1005. doi: 10.5194/bg-11-995-2014
Mitra A., Flynn K. J., Stoecker D. K., Raven J. A. (2023b). Trait trade-offs in phagotrophic microalgae: the mixoplankton conundrum. Eur. J. Phycol. 0, 1–20. doi: 10.1080/09670262.2023.2216259
Mitra A., Flynn K. J., Tillmann U., Raven J. A., Caron D., Stoecker D. K., et al. (2016). Defining planktonic protist functional groups on mechanisms for energy and nutrient acquisition: incorporation of diverse mixotrophic strategies. Protist 167, 106–120. doi: 10.1016/j.protis.2016.01.003
Moeller H. V., Neubert M. G., Johnson M. D. (2019). Intraguild predation enables coexistence of competing phytoplankton in a well-mixed water column. Ecology 100, e02874. doi: 10.1002/ecy.2874
Molenaar D., van Berlo R., de Ridder D., Teusink B. (2009). Shifts in growth strategies reflect tradeoffs in cellular economics. Mol. Syst. Biol. 5, 323. doi: 10.1038/msb.2009.82
Moorthi S. D., Ptacnik R., Sanders R. W., Fischer R., Busch M., Hillebrand H. (2017). The functional role of planktonic mixotrophs in altering seston stoichiometry. Aquat. Microb. Ecol. 79, 235–245. doi: 10.3354/ame01832
Morlon H. (2014). Phylogenetic approaches for studying diversification. Ecol. Lett. 17, 508–525. doi: 10.1111/ele.12251
Nagata T. (1996). “Picopellets’ produced by phagotrophic nanoflagellates: role in the material cycling within marine environments,” in Dynamics and Characterization of Marine Organic Matter Ocean Sciences Research (OSR). Eds. Handa N., Tanoue E., Hama T. (Springer Netherlands, Dordrecht), 241–256. doi: 10.1007/978–94-017–1319-1_12
Needham D. M., Poirier C., Bachy C., George E. E., Wilken S., Yung C. C. M., et al. (2022). The microbiome of a bacterivorous marine choanoflagellate contains a resource-demanding obligate bacterial associate. Nat. Microbiol. 7, 1466–1479. doi: 10.1038/s41564-022-01174-0
Nielsen L. T., Kiørboe T. (2021). Foraging trade-offs, flagellar arrangements, and flow architecture of planktonic protists. Proc. Natl. Acad. Sci. 118, e2009930118. doi: 10.1073/pnas.2009930118
Norland S., Fagerbakke K. M., Heldal M. (1995). Light element analysis of individual bacteria by x-ray microanalysis. Appl. Environ. Microbiol. 61, 1357–1362. doi: 10.1128/aem.61.4.1357-1362.1995
Nymark M., Valle K. C., Brembu T., Hancke K., Winge P., Andresen K., et al. (2009). An integrated analysis of molecular acclimation to high light in the marine diatom phaeodactylum tricornutum. PloS One 4, e7743. doi: 10.1371/journal.pone.0007743
Olson R. J., Sosik H. M. (2007). A submersible imaging-in-flow instrument to analyze nano-and microplankton: Imaging FlowCytobot. Limnol. Oceanogr. Methods 5, 195–203. doi: 10.4319/lom.2007.5.195
Orsi W. D., Wilken S., del Campo J., Heger T., James E., Richards T. A., et al. (2018). Identifying protist consumers of photosynthetic picoeukaryotes in the surface ocean using stable isotope probing: Identifying grazers of picoeukaryotes. Environ. Microbiol. 20, 815–827. doi: 10.1111/1462-2920.14018
Parrish C. C., French V. M., Whiticar M. J. (2012). Lipid class and fatty acid composition of copepods (Calanus finmarchicus, C. glacialis, Pseudocalanus sp., Tisbe furcata and Nitokra lacustris) fed various combinations of autotrophic and heterotrophic protists. J. Plankton Res. 34, 356–375. doi: 10.1093/plankt/fbs003
Post A. F., Bullerjahn G. S. (1994). The photosynthetic machinery in prochlorophytes: Structural properties and ecological significance. FEMS Microbiol. Rev. 13, 393–413. doi: 10.1111/fmr.1994.13.issue-4
Rii Y. M., Duhamel S., Bidigare R. R., Karl D. M., Repeta D. J., Church M. J. (2016b). Diversity and productivity of photosynthetic picoeukaryotes in biogeochemically distinct regions of the South East Pacific Ocean. Limnol. Oceanogr. 61, 806–824. doi: 10.1002/lno.10255
Rii Y., Karl D., Church M. (2016a). Temporal and vertical variability in picophytoplankton primary productivity in the North Pacific Subtropical Gyre. Mar. Ecol. Prog. Ser. 562, 1–18. doi: 10.3354/meps11954
Rose J. M., Caron D. A., Sieracki M. E., Poulton N. (2004). Counting heterotrophic nanoplanktonic protists in cultures and aquatic communities by flow cytometry. Aquat. Microb. Ecol. 34, 263–277. doi: 10.3354/ame034263
Rublee P. A., Gallegos C. L. (1989). Use of fluorescently labelled algae (FLA) to estimate microzooplankton grazing. Mar. Ecol. Prog. Ser. 51, 221–227. doi: 10.3354/meps051221
Saad J. F., Unrein F., Tribelli P. M., López N., Izaguirre I. (2016). Influence of lake trophic conditions on the dominant mixotrophic algal assemblages. J. Plankton Res. 38, 818–829. doi: 10.1093/plankt/fbw029
Sanders R. W. (1991). Mixotrophic protists in marine and freshwater ecosystems. J. Protozool. 38, 76–81. doi: 10.1111/j.1550-7408.1991.tb04805.x
Sanders R. W., Berninger U. G., Lim E. L., Kemp P. F., Caron D. A. (2000). Heterotrophic and mixotrophic nanoplankton predation on picoplankton in the Sargasso Sea and on Georges Bank. Mar. Ecol. Prog. Ser. 192, 103–118. doi: 10.3354/meps192103
Sanders R. W., Gast R. J. (2012). Bacterivory by phototrophic picoplankton and nanoplankton in Arctic waters. FEMS Microbiol. Ecol. 82, 242–253. doi: 10.1111/fem.2012.82.issue-2
Sanders R. W., Porter K. G., Bennett S. J., DeBiase A. E. (1989). Seasonal patterns of bacterivory by flagellates, ciliates, rotifers, and cladocerans in a freshwater planktonic community. Limnol. Oceanogr. 34, 673–687. doi: 10.4319/lo.1989.34.4.0673
Schneider L. K., Flynn K. J., Herman P. M. J., Troost T. A., Stolte W. (2020). Exploring the trophic spectrum: Placing mixoplankton into marine protist communities of the Southern North Sea. Front. Mar. Sci. 7. doi: 10.3389/fmars.2020.586915
Schneider L. K., Gypens N., Troost T. A., Stolte W. (2021). Modeling mixoplankton along the biogeochemical gradient of the Southern North Sea. Ecol. Model. 459, 109690. doi: 10.1016/j.ecolmodel.2021.109690
Schoener D. M., McManus G. B. (2017). Growth, grazing, and inorganic C and N uptake in a mixotrophic and a heterotrophic ciliate. J. Plankton Res. 39, 379–391. doi: 10.1093/plankt/fbx014
Segura-Noguera M., Blasco D., Fortuño J.-M. (2012). An improved energy-dispersive X-ray microanalysis method for analyzing simultaneously carbon, nitrogen, oxygen, phosphorus, sulfur, and other cation and anion concentrations in single natural marine microplankton cells: X-ray microanalysis of microphytoplankton. Limnol. Oceanogr. Methods 10, 666–680. doi: 10.4319/lom.2012.10.666
Segura-Noguera M., Blasco D., Fortuño J.-M. (2016). Taxonomic and environmental variability in the elemental composition and stoichiometry of individual dinoflagellate and diatom cells from the nw mediterranean sea. PloS One 11, e0154050. doi: 10.1371/journal.pone.0154050
Selosse M.-A., Charpin M., Not F. (2017). Mixotrophy everywhere on land and in water: the grand écart hypothesis. Ecol. Lett. 20, 246–263. doi: 10.1111/ele.12714
Serra-Pompei C., Hagstrom G. I., Visser A. W., Andersen K. H. (2019). Resource limitation determines temperature response of unicellular plankton communities. Limnol. Oceanogr. 64, 1627–1640. doi: 10.1002/lno.11140
Serra-Pompei C., Ward B. A., Pinti J., Visser A. W., Kiørboe T., Andersen K. H. (2022). Linking plankton size spectra and community composition to carbon export and its efficiency. Glob. Biogeochem. Cycles 36, e2021GB007275. doi: 10.1029/2021GB007275
Sherr B. F., Sherr E. B., Fallon R. D. (1987). Use of monodispersed, fluorescently labeled bacteria to estimate in situ protozoan bacterivory. Appl. Environ. Microbiol. 53, 958–965. doi: 10.1128/aem.53.5.958-965.1987
Sieracki C. K., Sieracki M. E., Yentsch C. S. (1998). An imaging-in-flow system for automated analysis of marine microplankton. Mar. Ecol. Prog. Ser. 168, 285–296. doi: 10.3354/meps168285
Šimek K., Mukherjee I., Nedoma J., de Paula C. C. P., Jezberová J., Sirová D., et al. (2022). CARD-FISH and prey tracer techniques reveal the role of overlooked flagellate groups as major bacterivores in freshwater hypertrophic shallow lakes. Environ. Microbiol. 24, 4256–4273. doi: 10.1111/1462–2920.15846
Smayda T. J. (1998). Patterns of variability characterizing marine phytoplankton, with examples from Narragansett Bay. ICES J. Mar. Sci. 55, 562–573. doi: 10.1006/jmsc.1998.0385
Stamieszkin K., Millette N. C., Luo J. Y., Follett E., Record N. R., Johns D. G. (2024). Large protistan mixotrophs in the North Atlantic Continuous Plankton Recorder time series: associated environmental conditions and trends. Front. Mar. Sci. 11. doi: 10.3389/fmars.2024.1320046
Stock C. A., Dunne J. P., John J. G. (2014). Global-scale carbon and energy flows through the marine planktonic food web: An analysis with a coupled physical–biological model. Prog. Oceanogr. 120, 1–28. doi: 10.1016/j.pocean.2013.07.001
Stoecker D. K., Johnson M. D., Vargas C., Not F. (2009). Acquired phototrophy in aquatic protists. Aquat. Microb. Ecol. 57, 279–310. doi: 10.3354/ame01340
Tang H., Zhu S., Wang N., Xu Z., Huang J., Gu L., et al. (2020). The inhibitory effect of mixotrophic Ochromonas gloeopara on the survival and reproduction of Daphnia similoides sinensis. Environ. Sci. pollut. Res. 27, 29068–29074. doi: 10.1007/s11356-020-09291-1
Terrado R., Pasulka A. L., Lie A. A.-Y., Orphan V. J., Heidelberg K. B., Caron D. A. (2017). Autotrophic and heterotrophic acquisition of carbon and nitrogen by a mixotrophic chrysophyte established through stable isotope analysis. ISME J. 11, 2022–2034. doi: 10.1038/ismej.2017.68
Thingstad T. F., Havskum H., Garde K., Riemann B. (1996). On the strategy of “eating your competitor”: a mathematical analysis of algal mixotrophy. Ecology 77, 2108–2118. doi: 10.2307/2265705
Traboni C., Calbet A., Saiz E. (2020). Effects of prey trophic mode on the gross-growth efficiency of marine copepods: the case of mixoplankton. Sci. Rep. 10, 12259. doi: 10.1038/s41598–020-69174-w
Traboni C., Calbet A., Saiz E. (2021). Mixotrophy upgrades food quality for marine calanoid copepods. Limnol. Oceanogr. 66, 4125–4139. doi: 10.1002/lno.11948
Troost T. A., Kooi B. W., Kooijman S. A. L. M. (2005a). Ecological specialization of mixotrophic plankton in a mixed water column. Am. Nat. 166, E45–E61. doi: 10.1086/432038
Troost T. A., Kooi B. W., Kooijman S. A. L. M. (2005b). When do mixotrophs specialize? Adaptive dynamics theory applied to a dynamic energy budget model. Math. Biosci. 193, 159–182. doi: 10.1016/j.mbs.2004.06.010
Tsai A.-Y., Gong G.-C., Sanders R. W., Chen W.-H., Chao C.-F., Chiang K.-P. (2011). Importance of bacterivory by pigmented and heterotrophic nanoflagellates during the warm season in a subtropical western Pacific coastal ecosystem. Aquat. Microb. Ecol. 63, 9–18. doi: 10.3354/ame01470
Twining B. S., Baines S. B., Fisher N. S., Maser J., Vogt S., Jacobsen C., et al. (2003). Quantifying trace elements in individual aquatic protist cells with a synchrotron x-ray fluorescence microprobe. Anal. Chem. 75, 3806–3816. doi: 10.1021/ac034227z
Unrein F., Massana R., Alonso-Sáez L., Gasol J. M. (2007). Significant year-round effect of small mixotrophic flagellates on bacterioplankton in an oligotrophic coastal system. Limnol. Oceanogr. 52, 456–469. doi: 10.4319/lo.2007.52.1.0456
Uwizeye C., Decelle J., Jouneau P.-H., Flori S., Gallet B., Keck J.-B., et al. (2021). Morphological bases of phytoplankton energy management and physiological responses unveiled by 3D subcellular imaging. Nat. Commun. 12, 1049. doi: 10.1038/s41467-021-21314-0
Vad C. F., Schneider C., Fischer R., Kainz M. J., Ptacnik R. (2021). From adverse to beneficial: Contrasting dietary effects of freshwater mixotrophs on zooplankton. Freshw. Biol. 66, 2272–2282. doi: 10.1111/fwb.13832
Vad C. F., Schneider C., Lukić D., Horváth Z., Kainz M. J., Stibor H., et al. (2020). Grazing resistance and poor food quality of a widespread mixotroph impair zooplankton secondary production. Oecologia 193, 489–502. doi: 10.1007/s00442-020-04677-x
Vargas C. A., Contreras P. Y., Iriarte J. L. (2012). Relative importance of phototrophic, heterotrophic, and mixotrophic nanoflagellates in the microbial food web of a river-influenced coastal upwelling area. Aquat. Microb. Ecol. 65, 233–248. doi: 10.3354/ame01551
Waibel A., Peter H., Sommaruga R. (2019). Importance of mixotrophic flagellates during the ice-free season in lakes located along an elevational gradient. Aquat. Sci. 81, 45. doi: 10.1007/s00027-019-0643-2
Ward B. A., Dutkiewicz S., Barton A. D., Follows M. J. (2011). Biophysical aspects of resource acquisition and competition in algal mixotrophs. Am. Nat. 178, 98–112. doi: 10.1086/660284
Ward B. A., Follows M. J. (2016). Marine mixotrophy increases trophic transfer efficiency, mean organism size, and vertical carbon flux. Proc. Natl. Acad. Sci. 113, 2958–2963. doi: 10.1073/pnas.1517118113
Wegener G., Kellermann M. Y., Elvert M. (2016). Tracking activity and function of microorganisms by stable isotope probing of membrane lipids. Curr. Opin. Biotechnol. 41, 43–52. doi: 10.1016/j.copbio.2016.04.022
Weiner A. K. M., Katz L. A. (2021). Epigenetics as driver of adaptation and diversification in microbial eukaryotes. Front. Genet. 12. doi: 10.3389/fgene.2021.642220
Widdicombe C. E., Eloire D., Harbour D., Harris R. P., Somerfield P. J. (2010). Long-term phytoplankton community dynamics in the Western English Channel. J. Plankton Res. 32, 643–655. doi: 10.1093/plankt/fbp127
Wieczynski D. J., Moeller H. V., Gibert J. P. (2023). Mixotrophic microbes create carbon tipping points under warming. Funct. Ecol. 37, 1774–1786. doi: 10.1111/1365-2435.14350
Wilken S., Choi C. J., Worden A. Z. (2020). Contrasting mixotrophic lifestyles reveal different ecological niches in two closely related marine protists. J. Phycol. 56, 52–67. doi: 10.1111/jpy.12920
Wilken S., Huisman J., Naus-Wiezer S., Van Donk E. (2013). Mixotrophic organisms become more heterotrophic with rising temperature. Ecol. Lett. 16, 225–233. doi: 10.1111/ele.12033
Wilken S., Yung C. C. M., Hamilton M., Hoadley K., Nzongo J., Eckmann C., et al. (2019). The need to account for cell biology in characterizing predatory mixotrophs in aquatic environments. Philos. Trans. R. Soc B Biol. Sci. 374, 20190090. doi: 10.1098/rstb.2019.0090
Wilken S., Yung C. C. M., Poirier C., Massana R., Jimenez V., Worden A. Z. (2023). Choanoflagellates alongside diverse uncultured predatory protists consume the abundant open-ocean cyanobacterium Prochlorococcus. Proc. Natl. Acad. Sci. 120, e2302388120. doi: 10.1073/pnas.2302388120
Wiltshire K. H., Kraberg A., Bartsch I., Boersma M., Franke H.-D., Freund J., et al. (2010). Helgoland roads, north sea: 45 years of change. Estuaries Coasts 33, 295–310. doi: 10.1007/s12237-009-9228-y
Yoon H. S., Price D. C., Stepanauskas R., Rajah V. D., Sieracki M. E., Wilson W. H., et al. (2011). Single-cell genomics reveals organismal interactions in uncultivated marine protists. Science 332, 714–717. doi: 10.1126/science.1203163
Yun H. Y., Larsen T., Choi B., Won E., Shin K. (2022). Amino acid nitrogen and carbon isotope data: Potential and implications for ecological studies. Ecol. Evol. 12, e8929. doi: 10.1002/ece3.8929
Zhang X., Gillespie A. L., Sessions A. L. (2009). Large D/H variations in bacterial lipids reflect central metabolic pathways. Proc. Natl. Acad. Sci. 106, 12580–12586. doi: 10.1073/pnas.0903030106
Zhang H., Gu B., Zhou Y., Ma X., Liu T., Xu H., et al. (2022). Multi-Omics profiling reveals resource allocation and acclimation strategies to temperature changes in a marine dinoflagellate. Appl. Environ. Microbiol. 88, e01213–e01222. doi: 10.1128/aem.01213-22
Zubkov M. V., Tarran G. A. (2008). High bacterivory by the smallest phytoplankton in the North Atlantic Ocean. Nature 455, 224–226. doi: 10.1038/nature07236
Zuñiga C., Li C.-T., Huelsman T., Levering J., Zielinski D. C., McConnell B. O., et al. (2016). Genome-scale metabolic model for the green alga Chlorella vulgaris utex 395 accurately predicts phenotypes under autotrophic, heterotrophic, and mixotrophic growth conditions. Plant Physiol. 172, 589–602. doi: 10.1104/pp.16.00593
Keywords: mixoplankton, mixotrophy, methods, evolution, trade-offs, biogeography, food webs
Citation: Millette NC, Leles SG, Johnson MD, Maloney AE, Brownlee EF, Cohen NR, Duhamel S, Poulton NJ, Princiotta SD, Stamieszkin K, Wilken S and Moeller HV (2024) Recommendations for advancing mixoplankton research through empirical-model integration. Front. Mar. Sci. 11:1392673. doi: 10.3389/fmars.2024.1392673
Received: 27 February 2024; Accepted: 20 May 2024;
Published: 05 June 2024.
Edited by:
Bernardo Antonio Perez Da Gama, Fluminense Federal University, BrazilReviewed by:
Claudia Traboni, Anton Dohrn Zoological Station Naples, ItalyQian Li, Shanghai Jiao Tong University, China
Copyright © 2024 Millette, Leles, Johnson, Maloney, Brownlee, Cohen, Duhamel, Poulton, Princiotta, Stamieszkin, Wilken and Moeller. This is an open-access article distributed under the terms of the Creative Commons Attribution License (CC BY). The use, distribution or reproduction in other forums is permitted, provided the original author(s) and the copyright owner(s) are credited and that the original publication in this journal is cited, in accordance with accepted academic practice. No use, distribution or reproduction is permitted which does not comply with these terms.
*Correspondence: Nicole C. Millette, bm1pbGxldHRlQHZpbXMuZWR1
†Present address: Ashley E. Maloney, Department of Geological Sciences, University of Colorado Boulder, Boulder, CO, United States