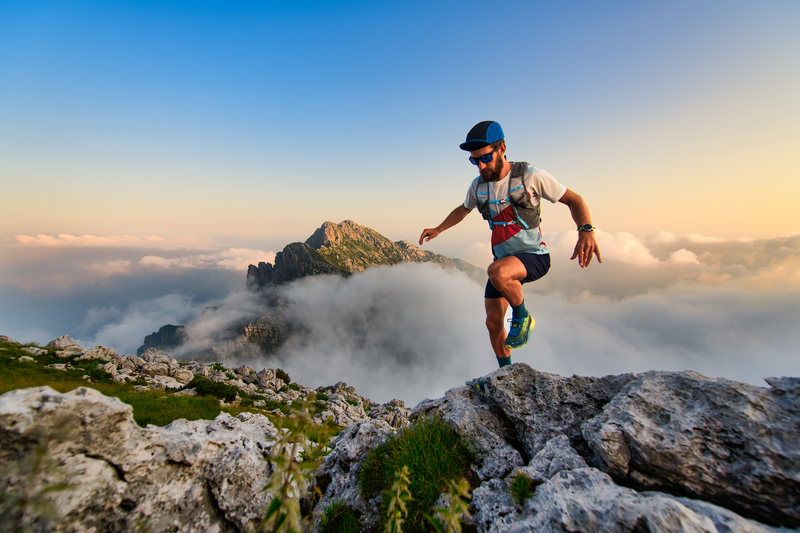
94% of researchers rate our articles as excellent or good
Learn more about the work of our research integrity team to safeguard the quality of each article we publish.
Find out more
ORIGINAL RESEARCH article
Front. Mar. Sci. , 03 June 2024
Sec. Marine Megafauna
Volume 11 - 2024 | https://doi.org/10.3389/fmars.2024.1391674
Age of cetaceans is an important data set for their conservation and management. Although the chronological age of a toothed whale has traditionally estimated from the number of annual tooth growth layer groups (GLGs), this method has potential difficulties such as errors due to the subjectivity of the age-readers and time-consuming sample processing, in addition to lethal tooth sampling in many cases. To overcome these difficulties through a non-lethal approach, considerable amount of efforts has been made in recent years to construct chronological age estimation models based on the correlation between chronological and biological age expressed as the frequency of DNA methylation at CpG (cytosine-phosphate-guanine) sites. The present study, by using next-generation sequencing on DNA extracted from the skin tissues of 30 individuals (15 males and 15 females), performed methylation analysis on multiple CpG sites in the three genes previously reported as age-linked in cetaceans (GRIA2, TET2, and CDKN2A) to construct an age estimation model for Risso’s dolphins. Key aspects of this study are: (1) the chronological ages of Risso’s dolphins of wild origins used in this study had been determined by the GLGs of teeth prior to this study, and (2) as Risso’s dolphins are said to have a lifespan of 30 years, samples were collected from individuals aged 1–29 years, covering from young to old ages. Among the 11 CpG sites in GRIA2, 6 CpG sites in TET2, and 7 CpG sites in CDKN2A analyzed, the CpG sites in GRIA2 showed a statistically significant correlation with age, whereas those in TET2 and CDKN2A did not show such a strong correlation. Using multiple regression analysis on the CpG sites in the three genes, the best fitted model was expressed as follows: y=-0.7548 + 5.6164x(GRIA2_CpG3)+2.6187x(TET2_CpG6)-1.5907x(CDKN2A_CpG3) (R²=0.7062, residual mean=2.98, standard deviation=2.3). With the mean error of 2.98 years between the estimated and known chronological ages, the accuracy of this model was found to be comparable to or higher than that of previously reported age estimation models for cetaceans. This study demonstrated for the future that the age of wild Risso’s dolphins can be estimated by using their skin samples collected with minimal invasiveness.
In order to properly implement conservation and management of cetaceans, chronological age is an important data set for understanding their biology, ecology and population dynamics. For instance, the use of population dynamics models based on age-dependent parameters, such as age at sexual maturity, lifespan, mortality rate, and age composition, enables us to evaluate the current status of the population and predict its future (e.g., Punt, 2014). Despite its critical importance, unfortunately, it is realistically difficult to determine the chronological age of wild animals based on field observations throughout their entire life from birth. Their chronological age is, therefore, often assessed indirectly by counting annual growth layer groups (GLGs: a group of layers occurring with cyclical and predictable repetition, Hohn, 2018) in the teeth or bones. This is also true for cetaceans, and currently, earplugs and teeth are widely used for determining the chronological age of baleen whales (infraorder Mysticeti) and toothed whales (infraorder Odontoceti), respectively (Hohn, 2018). However, one major disadvantage within the indirect age estimation technique using such age traits is the fact that it requires lethal sampling in many cases, preventing us from conducting research such as on endangered species as well as continued surveys of living individuals (IWC, 1980; Mayne et al., 2021a). Furthermore, these age assessments possess inherent difficulties, including possible subjective errors among age-readers during visual measurements, the time required to acquire assessment techniques to overcome such errors (Kitakado et al., 2013), and the inability to make continuous observations over animal’s lifespan. In addition, it is necessary that experienced scientists process the samples with considerable amount of time before the age-reading (Hohn, 2018). Although conventional lethal or invasive methods are still widely used today to determine the chronological age of cetaceans, there is a need to explore novel age estimation methods (e.g., Olsen et al., 2012; Polanowski et al., 2014; Boye et al., 2020).
In an attempt to develop non-lethal age estimation methods as an alternative, age estimation technique utilizing the correlation between biological age based on the frequency of DNA methylation (DNAm) and chronological age (Maegawa et al., 2010; Horvath, 2013) has been receiving a great amount of attention as a method to minimize the impact on individuals (Polanowski et al., 2014; Wright et al., 2018; Mayne et al., 2021b). Methylation frequency can be estimated using DNA extracted from skin samples obtained from non-lethal and minimally invasive approaches, such as biopsy. Furthermore, this age estimation method is greatly advantageous as it requires a shorter time than conventional one based on age traits, such as teeth. DNAm primarily occurs upon covalent binding of a methyl group to the 5-position carbon of the pyrimidine ring of cytosines at the CpG sites in cytosine-guanine sequences (5’-Cytosine-phosphate-Guanine-3’). Approximately 70% of CpG sites in mammalian DNA are thought to be impacted by methylation, and in particular, methylation tends to occur at promoter regions (Lister et al., 2009). Methylation status varies even at identical CpG sites among adjacent cells. Studies on mammals, such as humans and mice, have reported a correlation between methylation frequency within specific genes and chronological age (Maegawa et al., 2010; Hannum et al., 2013; Horvath, 2013; Yi et al., 2014). An estimation model of chronological age can be constructed based on the correlative relationship between individuals with known chronological age and methylation frequency, which enables us to estimate chronological age even in individuals whose chronological age is unknown. Since its application on humpback whales (Megaptera novaeangliae) as reported by Polanowski et al. (2014), studies applying such an age estimation model to cetaceans have been increasing (e.g., Beal et al., 2019; Barratclough et al., 2021; Bors et al., 2021; Robeck et al., 2021).
Risso’s dolphins (Grampus griseus) are the only species belonging to the genus Grampus of the family Delphinidae, infraorder Odontoceti, suborder Cetacea, order Cetartiodactyla, and they are distributed worldwide on the continental shelves and slopes around between 64°N and 46°S (Jefferson et al., 2014). In the waters near Japan, they have been sighted in a wide area on the Pacific coast south of the Kuril Islands, and they are also distributed in the Sea of Japan (Jefferson et al., 2014). The age at sexual maturity of this species is estimated to be 8–10 years for females and 10–12 years for males (Amano and Miyazaki, 2004). In Japan, they are commercially targeted by dolphin drive fisheries in Taiji, Wakayama. The Risso’s dolphins used in previous studies have been largely limited to individuals caught or bycaught from the commercial fisheries (Jefferson et al., 2014; Kanaji and Gerrodette, 2020), and various challenges still exist in expanding the knowledge on the biology, ecology and population dynamics of this species.
The present study analyzed the relationship between DNAm frequency and chronological age at the CpG sites in the three age-related genes in cetacean, namely GRIA2, TET2, and CDKN2A (Polanowski et al., 2014; Beal et al., 2019) through next-generation sequencing in order to construct an age estimation model for Risso’s dolphins for the first time. Key aspects of this study are: (1) the chronological ages of Risso’s dolphins of wild origins used in this study had been determined by the GLGs of teeth prior to this study, and (2) as Risso’s dolphin are said to have a lifespan of 30 years (Hartman et al., 2016), samples were collected from individuals aged 1–29 years, covering from young to old ages. Furthermore, this study demonstrates that for the future we only need a tiny amount of tissue as a sample for the age estimation that can be collected in a non-lethal, minimally invasive way.
This study used 30 individuals of Risso’s dolphin of wild origins (15 females and 15 males) caught by dolphin drive fisheries in Taiji, Wakayama, Japan in 2016 and 2017 (Supplementary Data Sheet). The fisheries were conducted outside the scientific community, and the use of their samples for scientific research on marine mammals is in line with the guidelines of the Society for Marine Mammalogy (2023).
The chronological ages of the individuals had been assessed by counting the GLGs present in the dentine or cementum of the teeth. Teeth were processed according to standard procedures (Hohn et al., 1989). Selected teeth (one tooth per individual) were bisected longitudinally through the midline, using a gravity-fed precision saw with a diamond blade (Isomet, Buehler, USA). Vertically split teeth were decalcified in a solution of hydrochloric acid at a concentration of 36.46 diluted to 10% for 20 minutes. Once decalcified, teeth were rinsed with water overnight and thin sectioned (24 μm, 32 μm) with a sliding freezing microtome (HM430, Thermo Fisher scientific, USA). The tin sections were prepared near the normal longitudinal section of the tooth and close to the horizontal cross section. Thin sections were then stained in Mayer’s hematoxylin in 20 minutes, then rinsed with water in 10 minutes. Thin sections blued for 5 minutes in a weak ammonia solution, rinsed with water in 5 minutes and then immersed in 100% ethanol for 5 minutes, again immersed in 100% ethanol prepared in a separate container for 5 minutes, and then mounted on slides with Euparal. Only stained section slides in good condition for GLG staining of both dentin and cementum were used. Section slides were examined under a digital microscope (VHX-7000, KEYENCE, Japan) for GLGs in the dentine (20–40×) and cementum (100–300×). The chronological ages of individuals with a closed pulp cavity was based on the cementum GLGs. For individuals without a closed pulp cavity, the GLGs of each cementum and dentin was counted. Age reading was performed three times independently by single reader and the median was treated as the chronological ages. One of the authors of this paper, Hikari Maeda who has considerable experience, conducted the age reading prior to this study. Mean absolute error (mean of difference between counted and median ages) and proportional error (mean absolute error divided by median age) were calculated.
Approximately 5 mm square of skin tissue was collected from the mandible of each individual and used as a sample for DNA extraction. These skin samples were stored at -78°C until used for DNA extraction. Genomic DNA was extracted from approximately 20 mg of a sample cut from a 5 mm square of skin tissue using the Gentra Puregene Tissue Kit (Qiagen, Germany). The extracted genomic DNA was stored at 4°C after measuring its concentration with a spectrophotometer (GeneQuant pro, GE HealthCare Technologies USA).
The three genes analyzed in this study were GRIA2, TET2, and CDKN2A, which had been previously studied in humpback whale (Polanowski et al., 2014) and common bottlenose dolphin (Tursiops truncatus, Beal et al., 2019) (Table 1). GRIA2 (Glutamate Receptor Ia2/AMP A2) is associated with aging-related neuronal cell death, and its methylation frequency increases with aging in humans (hypermethylation, Koch and Wagner, 2011). TET2 (Ten Eleven Translocation 2) is a proto-oncogene that has been suggested to be involved in the demethylation of other genes, and its methylation frequency was reported to increase with aging in humans (Grönnger et al., 2010). CDKN2A (Cyclin Dependent Kinase Inhibitor 2A) is a tumor suppressor gene whose expression level increases with aging in humans, and it is considered a useful biomarker of aging (Krishnamurthy et al., 2006). This study analyzed 11 CpG sites in GRIA2, 6 CpG sites in TET2, and 7 CpG sites in CDKN2A.
Bisulfite treatment, which converts non-methylated cytosine to uracil, was performed using the Methyl Detector Bisulfite Modification Kit (Active Motif, USA) on template DNA fragmented with restriction enzyme Hind III (New England Biolabs, USA). Using bisulfite-treated DNA as a template, the first PCR (Table 2) was performed to amplify the target region, after which the presence or absence of DNA amplification was examined by 2% agarose gel electrophoresis. DNA was extracted from the excised gel slice using the QIAquick Gel Extraction Kit (QIAGEN). The second PCR (Table 2) was performed using the DNA obtained from the first PCR as a template. An index sequence for sample identification (Illumina, 2015) and an adapter sequence for connecting the flow cell of the next-generation sequencer were added, which enabled the identification of up to 96 samples. After the PCR, the presence or absence of DNA amplification was examined by 2% agarose gel electrophoresis. Then, DNA was extracted from the excised gel slice using the QIAquick Gel Extraction Kit and stored at 4°C. After adjusting the concentration of the DNA samples, sequencing was performed using MiSeq (Illumina, USA) with reference to the MiSeq® System Quick Reference Guide (Illumina, 2011).
Data obtained from the sequencing (SAM and BAM files) were converted into the file format for data analysis using Samtools version 1.9 (Genome Research Limited, UK). Adapter sequences were removed with cutadapt version 1.18 (Martin, 2011), and low-quality reads with Q30 or less were removed with sickle version 1.33 (Joshi and Fass, 2011). Then, 3’ and 5’ terminal reads were merged using FLASH2 version 2.2.00 (Magoč and Salzberg, 2011), and FASTQ files were converted to FASTA files by FASTX-Toolkit version 0.0.14 and saved. A reference sequence file was created using bowtie2 version 2.3.5.1 (Langmead and Salzberg, 2012; Langmead et al., 2019), and the FASTA file of each sample was mapped using bismark version 0.23.1 (Babraham Bioinformatics, UK) to measure DNAm frequency.
To investigate the correlation between DNAm frequency and chronological age, simple and multiple regression analyses were performed using R version 4.2.2 (R Core Team, 2022) and Excel® version 2210 (Microsoft, USA). Simple regression analysis was used to analyze the correlation between DNAm frequency and chronological age at each CpG site by sex. A regression line was determined using the chronological age assessed by teeth as the independent variable and the methylation frequency as the dependent variable. The goodness of fit between the regression line and the data, as well as whether the regression coefficient was significantly greater than 0 (p<0.05), was judged by obtaining the coefficient of determination (R²) and statistical significance (p-value). To test whether there was a difference between sexes, covariance analysis was performed using the slope and intercept of the regression line. When the significance level was p>0.05, regression analysis was performed by including both sexes, assuming that there was no difference between sexes. For use as independent variables of the multiple regression model, CpG sites were selected from each gene according to the values of R² and p-value, and the multiple regression analysis was performed. Specifically, CpG sites satisfying R²>0.6 and p<0.05 were used for multiple regression analysis, assuming that the regression line could adequately express the relationship between the chronological age and methylation frequency when R²>0.6. When a gene had no CpG site satisfying the condition, a CpG site with a relatively large R² and a small p-value was used. The model of multiple regression analysis with the lowest Akaike information criterion (AIC) value was selected as the best age estimation model. Then, the value of R², p-value, mean absolute error (MAE) between chronological age and age estimated by the model, and standard deviation (SD) indicating the degree of spread of the error were determined. The 95% confidence interval (CI) and 95% prediction interval (PI) of the regression line were also obtained. In addition, the accuracy of the model by multiple regression analysis was examined by leave-one-out cross-validation (LOOCV).
The chronological ages of these individuals assessed by GLGs, showing that females and males were aged 1–29 years (mean 17.5 years) and 4–27 years (mean 14.1 years), respectively (Figure 1; Supplementary Data Sheet). Mean absolute error and proportional error with their ranges by the age readings were 0.41 (0.00–1.00) and 0.02 (0.00–0.10), respectively.
Figure 1 Chronological age distribution of male and female samples. Their ages were estimated based on growth layer groups (GLGs) in teeth.
DNAm frequencies of the CpG sites analyzed in this study were provided as Supplementary Material (Supplementary Data Sheet).
The presence of a significant correlation between the methylation frequency and chronological age was examined using simple regression analysis for each of the 11 CpG sites firstly by sex (female only and male only, Table 3). A statistically significant correlation was observed at all CpG sites in both males and females. In particular, GRIA2_CpG3 in females (R²=0.7695) and GRIA2_CpG6 in males (R²=0.7428) showed a strong positive correlation with age, and the regression line and the data fitted well. In the covariance analysis of the regression line, no difference in slope and intercept was observed between sexes at any CpG sites. Thus, single regression analysis was performed on the data from 30 individuals, and it showed a statistically significant correlation between the methylation frequency and age at all CpG sites (Table 3). Among these, GRIA2_CpG3 had the best fit of the regression equation (R²=0.7307, p=1.83×10−9), followed by GRIA2_CpG2 (R²=0.6968, p=9.79×10−9).
Table 3 Single regression analyses of CpG methylation as a function of chronological age at GRIA2, TET2, CDKN2A genes conducted for female and male separately and both combined.
Two females (aged 1 and 29 years) were excluded from the analysis because data could not be obtained through the sequencing resulting in a sample size of 13 females. The presence of a significant correlation between the methylation frequency and chronological age was examined by sex using simple regression analysis for each of the six CpG sites, and no CpG site with a good fit was found in either males or females (R²<0.6, Table 3). In particular, TET2_CpG1, TET2_CpG4, and TET2_CpG5 in females and TET2_CpG2, TET2_CpG3, and TET2_CpG5 in males had R²<0.1 and p>0.2, indicating no correlation with age. Covariance analysis was then performed to examine the difference between sexes in slope and intercept, and it showed a statistically significant difference only in the intercept at TET2_CpG4 (p=4.00×10−4). Thus, with assuming no difference between sexes, simple regression analysis was performed on the data from 28 individuals. The fit of the regression line was poor at all CpG sites, and no correlation was observed (R²<0.6, p>0.05). Given these, the CpG site with the highest R² was found to be TET2_CpG6.
One female (aged 29 years) was excluded from the analysis due to low DNA concentration, resulting in a sample size of 14 females. The presence of a significant correlation between the methylation frequency and chronological age was examined by sex using simple regression analysis for each of the seven CpG sites (Table 3), and no CpG site with good fit was found in either males or females (R²<0.6), as in TET2. In particular, CDKN2A_CpG2, CDKN2A_CpG4, CDKN2A_CpG6, and CDKN2A_CpG7 in females and CDKN2A_CpG2 and CDKN2A_CpG5 in males had R²<0.1 and p>0.2, indicating no correlation with age. In the covariance analysis of the regression line, no difference in slope and intercept between sexes was observed at any CpG sites. Thus, simple regression analysis was performed on the data from 29 individuals, and statistically significant results were obtained at CDKN2A_CpG1, CDKN2A_CpG3, and CDKN2A_CpG5 (p<0.05). Other CpG sites had a large variability with R²<0.5 and p>0.05, indicating a poor fit of the regression line. The CpG site with the best fit was CDKN2A_CpG1 (R²=0.2998, p=2.11×10−3), followed by CDKN2A_CpG3 (R²=0.2378, p=7.29×10−3).
Because the three genes showed no difference between sexes, an age estimation model was constructed using all the individuals irrespective of sexes. In GRIA2, among all CpG sites that had a significant age correlation, GRIA2_CpG3 (R²=0.7307, p=1.83×10−9) and GRIA2_CpG2 (R²=0.6968, p=9.79×10−9) had the best fits of the regression equation. Thus, these two CpG sites in GRIA2 were used for multiple regression analysis. In TET2, since no CpG sites had a significant age correlation, TET2_CpG6, which had the best fit (R²=0.1175, p=0.074), was used. In CDKN2A, CDKN2A_CpG1 (R²=0.2998, p=2.11×10−3) and CDKN2A_CpG3 (R²=0.2378, p=7.29×10−3), which had the best fit, were used.
Among the 10 models constructed, Model 1 had the lowest MAE between the chronological age and the chronological age estimated by the model (2.98), as well as the smallest SD (2.31, Table 4). Model 6, with R²=0.7325, had the next best fit. Model 1, having the lowest AIC, was selected as the best age estimation model with the results of LOOCV (R²=0.6398, p=2.2×10−7, SD=2.8, 95%CI=9.2) (Figure 2). Using multiple regression analysis on the CpG sites in the three genes, the chronological age estimation model based on the methylation frequencies of Risso’s dolphins was expressed as follows:
Table 4 Multiple regression analysis using CpG sites at GRIA2, TET2, and CDKN2A genes to develop the best fit chronological age estimation model.
Figure 2 Precision of the best age estimation model (model 1 in Table 4) in this study constructed using multiple CpG sites at GRIA2, TET2, and CDKN2A genes. (A) The result of multiple regression analysis (R²=0.7062) with 95% confidence interval of regression line (pale red solid line) and 95% prediction line (black dotted line) and (B) its validation using LOOCV analysis (R²=0.6398) with 95% confidence interval.
The present study focused on the three genes that had been previously reported to have a correlation with chronological age in cetaceans (Polanowski et al., 2014; Beal et al., 2019). One of the significant findings of this study is that these genes appeared to show different patterns on correlative age relationship in cetaceans. In GRIA2, all CpG sites studied in this study exhibited a significant positive correlation with chronological age regardless of whether males and females were analyzed separately or combined. This pattern was well consistent with the ones reported in humpback whales (Polanowski et al., 2014), bottlenose dolphins (Beal et al., 2019), and Antarctic minke whales (Balaenoptera bonaerensis, Goto et al., 2020; Tanabe et al., 2020). However, CpG sites with a high age correlation appeared to differ among species (e.g., Tanabe et al., 2020): although the GRIA2_CpG3 and GRIA2_CpG2 in Risso’s dolphins of this study exhibited a high age correlation, different CpG site (GRIA2_CpG8 in this study) showed the strongest age correlation in bottlenose dolphins (Beal et al., 2019). Contrary, in TET2, different trends in age correlation were observed depending on species and CpG site. Most, if not all, of the CpG sites studied in bottlenose dolphin, humpback whale, and Antarctic minke whale have been shown to have a negative age correlation (Polanowski et al., 2014; Beal et al., 2019; Goto et al., 2020), while a positive age correlation was reported in fin whale (Balaenoptera physalus) (García-Vernet et al., 2021). In Risso’s dolphin of this study, the slopes of the regression lines for TET2_CpG2, TET2_CpG4, and TET2_CpG5 differed between males and females, and only TET2_CpG3 of both sexes combined showed a negative age correlation. In addition to that, compared to GRIA2, the CpG sites in TET2 showed a poor fit of the regression line with large variability. Such a poor fit of the regression line has also been observed in other cetaceans, with an R² of approximately 0.1–0.4 in many studies (Polanowski et al., 2014; Beal et al., 2019). As with TET2, in CDKN2A, the CpG sites showed a relatively poor fit. A similar trend has been reported for other cetaceans: despite a positive correlative relationship, large variability was observed in bottlenose dolphin, humpback whale, and Antarctic minke whale (Polanowski et al., 2014; Beal et al., 2019; Goto et al., 2020). Although TET2 and CDKN2A are often studied with reference to previous reports, age estimation using these genes is considered difficult, making their priority lower as a choice of genes for the future age estimation analysis. This study strongly indicated that future studies examining age correlations should cover as many CpG sites as possible, even when selecting genes that are known to be age-linked from previous studies.
The present study constructed an age estimation model of Risso’s dolphin using the CpG sites in the three genes by multiple regression analysis, and its MAE between estimated age and known age (i.e., chronological age assessed by GLGs in this study) was found to be 2.98 years. This estimation error is considered low given that the chronological age estimation models of humpback whale (Humpback Epigenetic Age Assay, HEAA; Polanowski et al., 2014) and bottlenose dolphin (Bottlenose dolphin Epigenetic Age estimation Tool, BEAT; Beal et al., 2019) had a mean estimation error of 3.58 years and 4.83 years, respectively. Because TET2 and CDKN2A did not show good correlation with age, age estimation was also performed only with the CpG sites in GRIA2 using simple regression analysis (y=-2.3471 + 4.8884x(GRIA2_CpG3)), and its estimation error was found to be 3.18 years, which was still smaller than those of the age estimation models of humpback whale (Polanowski et al., 2014) and bottlenose dolphin (Beal et al., 2019). The practical advantage of age estimation using CpG sites of only one gene, on the one hand, lies in the relative easiness of the analysis and age estimation. The present study, on the other hand, showed that genes and CpG sites correlated with age could differ among species as well. Furthermore, it has been pointed out that the models of such age estimation are unstable due to the possible interference with the results of neighboring CpG sites during mapping by bismark (Grönnger et al., 2010; Beal et al., 2019). These suggest that it is preferable to construct an age estimation model based on the analysis of multiple genes and CpG sites whenever possible. In recent years, another method of methylation-based age estimation that comprehensively analyzes CpG sites on the genome of cetaceans with microarray has been increasingly applied to cetaceans (Barratclough et al., 2021; Bors et al., 2021; Robeck et al., 2021; Arneson et al., 2022), and the use of such a method will dramatically increase the number of CpG sites to be analyzed, likely leading to highly accurate age estimation.
A previous report indicated the presence of a bias in age estimation due to the uneven selection of samples used for analysis in terms of their chronological ages (Polanowski et al., 2014). When studying species with a long lifespan, such as cetaceans, samples of older age individuals are particularly often scarce, leading to underestimation of the ages of older age individuals. Considering this aspect of sample bias, the present study made an effort to evenly select individuals aged 1 to 29 years from available samples of Risso’s dolphin, which are said to have an average lifespan of approximately 30 years (Hartman et al., 2016). This sample selection likely has contributed to the mean age estimation error of 2.98 years in the selected model, which was smaller compared to previously reported models. Nevertheless, the weakness of this study may be the small sample size of 30 individuals. With regard to sample size, Mayne et al. (2021a) reported that a sample size of at least 70 is required to construct a DNAm-based age estimation model and a sample size of 135 is required to construct a stable model with high reliability (Mayne et al., 2021a). Thus, raising the methylation analyses of 45 individuals of humpback whale (Polanowski et al., 2014) and 62 individuals of Bechstein’s bat (Myotis bechsteinii) (Wright et al., 2018) as examples, they cautioned us that the models constructed with a sample size of less than 70 could be reasonable but unstable. Unfortunately, even with a non-lethal-methylation-based age estimation method, increasing sample size is a difficult task to be accomplished in studies targeting endangered species or wild organisms rarely encountered in their habitats, often the cases seen in cetacean studies.
Next important issue to be discussed is that caution is needed when interpreting the results of age estimation based on methylation frequency, as it contains two types of errors: one in estimating chronological ages due to the use of indirect approaches and the other in estimating biological ages due to inter-individual differences in methylation frequency. With regard to the error in estimating chronological ages, the present study used the ages indirectly assessed by GLGs of teeth as the known chronological ages with mean absolute error of 0.41 by the single reader. It is common in the previous cetacean studies that the chronological ages of cetaceans have been assessed using natural markings, teeth, earplugs, and other markers (Polanowski et al., 2014; Beal et al., 2019; Goto et al., 2020). Ages assessed using natural markings and the counting of GLGs of teeth and earplugs inevitably contain errors as they are indirectly obtained. It has been pointed out that this type of errors tends to occur at the counting stage of the age traits, such as teeth and earplugs, due to the subjectivity as well as skill levels of age-readers, and this tendency is more evident in the age estimation for older age individuals (e.g., Kitakado et al., 2013). Because this kind of human errors are difficult to be avoided, the age structure models has taken into account such errors (e.g., Punt, 2014). Although the chronological ages of Risso’s dolphins were considered as reliable as the experienced scientist counted GLGs in teeth and the mean absolute error was small, errors caused by the age-reader need to be considered to interpret results of DNAm-based age estimation models. In this study, age reading was independently performed three times by the single reader because availability of readers was limited. It would be better to have multiple age readers in the future research to test precision and accuracy of the age reading.
With regard to the error in age estimation arising from inter-individual differences in methylation frequency, it has been reported that DNAm occurs differently due to different level of sunlight exposure and that of stresses caused by the surrounding environment (Grönnger et al., 2010; Crossman et al., 2021). This suggests that if individuals of the same species with the same age have been exposed to different environments, even the age-linked genes exhibit inter-individual differences in methylation frequency, and thus in estimated age. It is thought that this tendency is more evident in older age individuals. As a matter of fact, the present study that happened to contain samples from two females aged 10 years and two females aged 29 years demonstrated that the variation in methylation frequency at the 11 CpG sites in GRIA2 was on average greater between the old individuals than between the young individuals (mean difference of 0.7% for those aged 10 years and 1.2% for those aged 29 years). Although the possibility cannot be ruled out that individuals treated as the same age in this study could be actually of different ages, a 1% difference in methylation frequency results in an approximately 4.9 years difference in age estimation from our model (model 1), which is a large error in estimated age. The use of a large number of samples to the possible extent at each age is expected to improve the accuracy of a DNAm-based age estimation model. Furthermore, Goto et al. (2020) revealed the differences in methylation frequency among the tissue samples obtained from different body parts of Antarctic minke whale (i.e., ventral, lateral, and dorsal side of whale body). From the physiochemical perspective, it is of great interest to elucidate the mechanisms behind the differences in methylation level at the different part of the body, but from a practical perspective, it would be more important to simply decide how and where to take tissue samples from the body.
DNAm-based method can be a first choice for estimating ages as an alternative to the conventional age estimation methods because it is non-lethal once models are constructed, does not involve errors caused by the subjectivity of age-readers, and enable rapid age estimation. Nevertheless, studies on cetaceans have been definitely increasing but still scarce, and the method has not yet been put to practical use. Reasons for this include the difficulty in obtaining sufficient number of tissue samples, and the fact that the actual chronological ages of cetaceans in many studies are not known. In this respect, attempts to construct a universal age estimation model for toothed whales that can be applied to as many species as possible are considered effective (Robeck et al., 2021). For convenience, the use of a universal model is an option for species from which sufficient numbers of samples or samples with known chronological age cannot be obtained. Moreover, the use of a universal model is practical when the accurate determination of the age of each individual is not the primary objective, as in the case of its application to age structure model. However, as shown in the present study, even in the same gene, CpG sites used for an age estimation model may differ depending on species, and thus it is desirable to cover a large number of species to the possible extent when constructing an unbiased universal model. For example, in terms of the number of species covered, Robeck et al. (2021) constructed one of the best universal models so far for toothed whales, but it covered species mainly from the family Delphinidae, but only a few from the families Monodontidae and Phocoenidae, and none from the families Physeteridae and Ziphiidae (Robeck et al., 2021). Thus, applying a universal model to all cetaceans of the infraorder Odontoceti may be difficult at present. It is desirable to expand the number of species to which a universal age estimation model can be applied. It should be stated again that the use of species-specific age estimation models can be more reasonable for accurate age estimation if a sufficient number of samples can be obtained from the species.
This study showed that the age of Risso’s dolphin was able to be successfully estimated using the DNAm-based model. For this purpose, frequency of DNAm was analyzed through next-generation sequencing using DNA samples extracted from skin tissues. For the future, especially after the development of the age estimation model, we only need a tiny amount of tissue as a sample from each individual whale collected in a non-lethal, minimally invasive way. In recent years, progress has been made in recent years regarding the efforts to construct age structure models for bottlenose dolphin, Risso’s dolphin, and short-finned pilot whale (Globicephala macrorhynchus) caught in Japan (Kanaji et al., 2021). Age estimation based on DNAm frequency is expected to play an important role in the conservation and management of these species.
The original contributions presented in the study are included in the article/Supplementary Material, further inquiries can be directed to the corresponding author.
Ethical approval was not required for the study involving animals in accordance with the local legislation and institutional requirements because the animals used in this study were wild origins caught by fisheries which were managed by a prefectural government in Japan based on scientific information and Japan’s laws/regulations in 2016 and 2017. The fisheries were conducted outside the scientific community, and the use of their samples for scientific research on marine mammals is in line with the guidelines of the Society for Marine Mammalogy (2023).
MM: Conceptualization, Data curation, Formal analysis, Investigation, Writing – original draft, Writing – review & editing, Methodology. HMa: Conceptualization, Data curation, Funding acquisition, Resources, Writing – review & editing, Formal analysis, Investigation, Methodology. AT: Formal analysis, Investigation, Methodology, Writing – review & editing, Conceptualization, Validation. HS: Methodology, Resources, Writing – review & editing, Conceptualization, Supervision. RN: Investigation, Methodology, Writing – review & editing. HK: Supervision, Writing – review & editing, Investigation, Methodology, Resources. GN: Supervision, Writing – review & editing. NK: Conceptualization, Investigation, Methodology, Supervision, Writing – original draft, Writing – review & editing. HMu: Conceptualization, Funding acquisition, Project administration, Supervision, Writing – original draft, Writing – review & editing.
The author(s) declare financial support was received for the research, authorship, and/or publication of this article. This study was supported by Japan Fisheries Research and Education Agency and Fisheries Agency of Japan.
We thank Dr. Shingo Minamikawa (Fisheries Resources Institute) for his logistical arrangement of the samples. We also thank the Taiji Fishery Cooperative Union and Isana Organization for supporting sampling in the field.
The authors declare that the research was conducted in the absence of any commercial or financial relationships that could be construed as a potential conflict of interest.
All claims expressed in this article are solely those of the authors and do not necessarily represent those of their affiliated organizations, or those of the publisher, the editors and the reviewers. Any product that may be evaluated in this article, or claim that may be made by its manufacturer, is not guaranteed or endorsed by the publisher.
The Supplementary Material for this article can be found online at: https://www.frontiersin.org/articles/10.3389/fmars.2024.1391674/full#supplementary-material
Amano M., Miyazaki N. (2004). Composition of a school of Risso’s dolphins, Grampus griseus. Mar. Mammal Sci. 20, 152–160. doi: 10.1111/j.1748-7692.2004.tb01146.x
Arneson A., Haghani A., Thompson M. J., Pellegrini M., Kwon S. B., Vu H., et al. (2022). A mammalian methylation array profiling methylation levels at conserved sequences. Nat. Commun. 13, 783. doi: 10.1038/s41467-022-28355-z
Barratclough A., Smith C. R., Gomez F. M., Photopoulou T., Takeshita R., Pirotta E., et al. (2021). Accurate epigenetic aging in bottlenose dolphins (Tursiops truncatus), an essential step in the conservation of at-risk dolphins. J. Zool. Bot. Gard 2, 416–420. doi: 10.3390/jzbg2030030
Beal A. P., Kiszka J. J., Wells R. S., Eirin-Lopez J. M. (2019). The Bottlenose Dolphin Epigenetic Aging Tool (BEAT): A molecular age estimation tool for small cetaceans. Front. Mar. Sci. 6. doi: 10.3389/fmars.2019.00561
Bors E. K., Baker C. S., Wade P. R., O’Neill K. B., Shelden K. E. W., Thompson M. J., et al. (2021). An epigenetic clock to estimate the age of living beluga whales. Evol. Appl. 14, 1263–1273. doi: 10.1111/eva.13195
Boye T. K., Garde E., Nielsen J., Hedeholm R., Olsen J., Simon M. (2020). Estimating the age of West Greenland humpback whales through aspartic acid racemization and eye lens bomb radiocarbon methods. Front. Mar. Sci. 6. doi: 10.3389/fmars.2019.00811
Crossman C. A., Barrett-Lennard L. G., Frasier T. R. (2021). An example of DNA methylation as a means to quantify stress in wildlife using killer whales. Sci. Rep. 11, 16822. doi: 10.1038/s41598–021-96255–1
García-Vernet R., Martín B., Peinado M. A., Víkingsson G., Riutort M., Aguilar A. (2021). CpG methylation frequency of TET2, GRIA2, and CDKN2A genes in the North Atlantic fin whale varies with age and between populations. Mar. Mammal Sci. 37, 1230–1244. doi: 10.1111/mms.12808
Goto M., Kitakado T., Pastene L. A. (2020). A preliminary study of epigenetic estimation of age of the Antarctic minke whale Balaenoptera bonaerensis. Cetacean Popul. Stud. 2, 5–14. doi: 10.34331/cpops.2.1_5
Grönnger E., Weber B., Heil O., Peters N., Stäb F., Wenck H., et al. (2010). Aging and chronic sun exposure cause distinct epigenetic changes in human skin. PloS Genet. 6, e1000971. doi: 10.1371/journal.pgen.1000971
Hannum G., Guinney J., Zhao L., Zhang L., Hughes G., Sadda S., et al. (2013). Genome-wide methylation profiles reveal quantitative views of human aging rates. Mol. Cell. 49, 359–367. doi: 10.1016/j.molcel.2012.10.016
Hartman K. L., Wittich A., Cai J. J., van der Meulen F. H., Azevedo J. M. N. (2016). Estimating the age of Risso’s dolphins (Grampus griseus) based on skin appearance. J. Mamm. 97, 490–502. doi: 10.1093/jmammal/gyv193
Hohn A. A. (2018) “Age Estimation,” in Encyclopedia of Marine Mammals, 3rd ed. Eds. Würsig B., Thewissen J. G. M., Kovacs K. M. (London, UK: Academic Press), 10–14.
Hohn A. A., Scott D. M., Wells S. R., Sweeney C. J., Irvine B. A. (1989). Growth layers in teeth from known-age, free-ranging bottlenose dolphins. Mar. Mamm. Sci. 5, 315–342. doi: 10.1111/j.1748-7692.1989.tb00346.x
Horvath S. (2013). DNA Methylation age of human tissues and cell types. Genome Biol. 14, R115. doi: 10.1186/gb-2013-14-10-r115
Illumina. (2011). MiSeq® System Quick Reference Guide. Available online at: https://jp.illumina.com/content/dam/illumina-marketing/apac/Japan/documents/pdf/quickguide_miseq.pdf (Accessed March 22, 2022).
Illumina. (2015). illumina Adapter Sequences. Available online at: https://dnatech.genomecenter.ucdavis.edu/wp-content/uploads/2013/06/illumina-adapter-sequences_1000000002694–00.pdf (Accessed December 27, 2022).
International Whaling Commission. (1980). Special issue 3: Age Determination of Toothed Whales and Sirenians (Cambridge: International Whaling Commission).
Jefferson T. A., Weir C. R., Anderson R. C., Ballance L. T., Kenney R. D., Kiszka J. J. (2014). Global distribution of Risso’s dolphin Grampus griseus: A review and critical evaluation. Mamm. Rev. 44, 56–68. doi: 10.1111/mam.12008
Joshi N. A., Fass J. N. (2011) Sickle: A sliding-window, adaptive, quality-based trimming tool for FastQ files (version 1.33) [software]. Available online at: https://github.com/najoshi/sickle (Accessed February 9, 2024).
Kanaji Y., Gerrodette T. (2020). Estimating abundance of Risso’s dolphins using a hierarchical Bayesian habitat model: A framework for monitoring stocks of animals inhabiting a dynamic ocean environment. Deep. Res. Part II Top. Stud. Oceanogr. 175, 104699. doi: 10.1016/j.dsr2.2019.104699
Kanaji Y., Maeda H., Okamura H., Punt A. E., Branch T. (2021). Multiple-model stock assessment frameworks for precautionary management and conservation on fishery-targeted coastal dolphin populations off Japan. J. Appl. Ecol. 58, 2479–2492. doi: 10.1111/1365-2664.13982
Kitakado T., Lockyer C., Punt A. E. (2013). A statistical model for quantifying age-reading errors and its application to the Antarctic minke whales. J. Cetacean Res. Manage. 13, 181–190. doi: 10.47536/jcrm.v13i3.534
Koch C. M., Wagner W. (2011). Epigenetic-aging-signature to determine age in different tissues. Aging 3, 1018–1027. doi: 10.18632/aging.v3i10
Krishnamurthy J., Ramsey M. R., Ligon K. L., Torrice C., Koh A., Bonner-Weir S., et al. (2006). p16INK4a induces an age-dependent decline in islet regenerative potential. Nature 443, 453–457. doi: 10.1038/nature05092
Langmead B., Salzberg S. (2012). Fast gapped-read alignment with Bowtie 2. Nat. Methods 9, 357–359. doi: 10.1038/nmeth.1923
Langmead B., Wilks C., Antonescu V., Charles R. (2019). Scaling read aligners to hundreds of threads on general-purpose processors. Bioinformatics 35, 421–432. doi: 10.1093/bioinformatics/bty648
Lister R., Pelizzola M., Dowen R. H., Hawkins. R. D., Hon G., Tonti-Filippini J., et al. (2009). Human DNA methylomes at base resolution show widespread epigenomic differences. Nature 462, 315–322. doi: 10.1038/nature08514
Maegawa S., Hinkal G., Kim H. S., Shen L., Zhang L., Zhang J., et al. (2010). Widespread and tissue specific age-related DNA methylation changes in mice. Genome Res. 20, 332–340. doi: 10.1101/gr.096826.109
Magoč T., Salzberg S. L. (2011). FLASH: Fast length adjustment of short reads to improve genome assemblies. Bioinformatics 27, 2957–2963. doi: 10.1093/bioinformatics/btr507
Martin M. (2011). Cutadapt removes adapter sequences from high-throughput sequencing reads. EMBnet.journal 17, 10–12. doi: 10.14806/ej.17.1.200
Mayne B., Berry O., Jarman S. (2021a). Optimal sample size for calibrating DNA methylation age estimators. Mol. Ecol. Resour. 21, 2316–2323. doi: 10.1111/1755-0998.13437
Mayne B., Espinoza T., Roberts D., Butler G. L., Brooks S., Korbie D., et al. (2021b). Nonlethal age estimation of three threatened fish species using DNA methylation: Australian lungfish, Murray cod and Mary River cod. Mol. Ecol. Resour. 21, 2324–2332. doi: 10.1111/1755-0998.13440
Olsen M. T., Bérubé M., Robbins J., Palsbøll P. J. (2012). Empirical evaluation of humpback whale telomere length estimates; quality control and factors causing variability in the singleplex and multiplex qPCR methods. BMC Genet. 13, 77. doi: 10.1186/1471-2156-13-77
Polanowski A. M., Robbins J., Chandler D., Jarman S. N. (2014). Epigenetic estimation of age in humpback whales. Mol. Ecol. Resour. 14, 976–987. doi: 10.1111/1755-0998.12247
Punt A. E. (2014). A summary history of the application of statistical catch-at-age analysis (SCAA) to Antarctic minke whales. J. Cetacean Res. Manage. 14, 81–92. doi: 10.47536/jcrm.v14i1.526
R Core Team. (2022). R: A language and environment for statistical computing. Available online at: https://www.R-project.org/.
Robeck T. R., Fei Z., Lu A. T., Haghani A., Jourdain E., Zoller J. A., et al. (2021). Multi-species and multi-tissue methylation clocks for age estimation in toothed whales and dolphins. Commun. Biol. 4, 642. doi: 10.1038/s42003-021-02179-x
Tanabe A., Shimizu R., Osawa Y., Suzuki M., Ito S., Goto M., et al. (2020). Age estimation by DNA methylation in the Antarctic minke whale. Fish. Sci. 86, 35–41. doi: 10.1007/s12562-019-01371-7
The Society for Marine Mammalogy. (2023). Guideline for Treatment of Marine Mammals. Available online at: https://marinemammalscience.org/about-us/ethics/marine-mammal-treatment-guidelines/ (Accessed August 11, 2023).
Wright P. G. R., Mathews F., Schofield H., Morris C., Burrage J., Smith A., et al. (2018). Application of a novel molecular method to age free-living wild Bechstein’s bats. Mol. Ecol. Resour. 18, 1374–1380. doi: 10.1111/1755-0998.12925
Keywords: North Pacific, Risso’s dolphin, DNA methylation (DNAm), age estimation model, next generation sequencing
Citation: Mori M, Maeda H, Tanabe A, Sahara H, Nozaki R, Kondo H, Nakamura G, Kanda N and Murase H (2024) Estimation of chronological age of Risso’s dolphin (Grampus griseus) based on DNA methylation frequency. Front. Mar. Sci. 11:1391674. doi: 10.3389/fmars.2024.1391674
Received: 26 February 2024; Accepted: 02 May 2024;
Published: 03 June 2024.
Edited by:
Roberto Carlucci, University of Bari Aldo Moro, ItalyReviewed by:
Duminda Senevirathna, Uva Wellassa University, Sri LankaCopyright © 2024 Mori, Maeda, Tanabe, Sahara, Nozaki, Kondo, Nakamura, Kanda and Murase. This is an open-access article distributed under the terms of the Creative Commons Attribution License (CC BY). The use, distribution or reproduction in other forums is permitted, provided the original author(s) and the copyright owner(s) are credited and that the original publication in this journal is cited, in accordance with accepted academic practice. No use, distribution or reproduction is permitted which does not comply with these terms.
*Correspondence: Hiroto Murase, aG11cmFzMEBrYWl5b2RhaS5hYy5qcA==
†These authors share last authorship
Disclaimer: All claims expressed in this article are solely those of the authors and do not necessarily represent those of their affiliated organizations, or those of the publisher, the editors and the reviewers. Any product that may be evaluated in this article or claim that may be made by its manufacturer is not guaranteed or endorsed by the publisher.
Research integrity at Frontiers
Learn more about the work of our research integrity team to safeguard the quality of each article we publish.