- 1College of Geography and Environmental Science/Key Research Institute of Yellow River Civilization and Sustainable Development and Collaborative Innovation Center on Yellow River Civilization of Henan Province, Henan University, Kaifeng, China
- 2Key Laboratory of Geospatial Technology for the Middle and Lower Yellow River Regions (Henan University), Ministry of Education/National Demonstration Center for Environment and Planning, Henan University, Kaifeng, China
- 3Henan Dabieshan National Field Observation and Research Station of Forest Ecosystem, Zhengzhou, China
- 4Laboratory of Climate Change Mitigation and Carbon Neutrality, Henan University, Zhengzhou, China
- 5Xinyang Academy of Ecological Research, Xinyang, China
- 6Henan Key Laboratory of Earth System Observation and Modeling, Henan University, Kaifeng, China
- 7College of Urban and Environmental Sciences, Northwest University, Xi’an, China
- 8Department of Geography, University of Connecticut, Storrs, CT, United States
- 9The Forest Science Research Institute of Xinyang, Henan, Xinyang, China
- 10Henan Jigongshan Forest Ecosystem National Observation and Research Station, Henan, Xinyang, China
Heavy-metal contamination in river and ocean is a critical environmental issue that endangers marine ecosystems and human health. Therefore, conducting extensive research to devise effective mitigation measures is imperative. Sediment samples were taken randomly throughout the study area. Analysis was done to determine the presence of different metals, including arsenic, cadmium, chromium, nickel, copper, zinc, lead, and manganese. The assessment of different pollution levels was done by using various pollution indicators including “geo-accumulation index (Igeo), contamination factor (CF), enrichment factor (EF)” for accuracy. The Igeo measurement for Cd indicated varying pollution, ranging from moderate to significantly polluted, while Mn revealed no contamination. Elements such as Ni, Cr, Cu, and Zn showed a moderate level of contamination. The contamination factor values exhibited a range of 0.436 (Pb) to 7.637 (Cd), with average values spanning from 0.9176 (Mn) to 4.9714 (Cd), suggesting significant regional variation. EF exhibits a pattern of contamination comparable to that of Igeo. The noncarcinogenic risk associated with exposure to Cd and As exceeded the higher limit (HI > 1) for children and adults. Furthermore, the carcinogenic risk presented by pollutants such as copper (Cu), arsenic (As), nickel (Ni), cadmium (Cd), and chromium (Cr) was found to exceed the limits in children. In adults, only arsenic (As) and copper (Cu) were shown to represent a higher risk of cancer than the limit of 10−4. The PCA analysis revealed that two (PCs) accounted for more than 65% of the total variance in the River Ravi, as determined by eigenvalues greater than 1. This study underscores the importance of the ongoing monitoring and management of heavy-metal pollution to ensure sustainable marine ecosystem development and public health protection.
1 Introduction
Anthropogenic activities impact aquatic habitats, altering water and sediment quality and affecting the organisms. The marine ecosystem near developed land is exposed to various forms of pollution, such as agricultural, industrial, and health-related contaminants (Chen et al., 2022).
The issue of pollution in aquatic environments has emerged as a prominent concern in recent decades, primarily due to its significant implications for public health and the well-being of marine organisms. Underrated sources of pollution, such as the rise in navigation and maritime transport, the growth of tourism, the release of untreated wastewater into waterways, and the use of pesticides in agriculture, which often find their way into aquatic environments through estuaries, contribute significantly to both point and non-point pollutants (Svavarsson et al., 2021). This pollution disrupts the aquatic life cycle and poses a public safety risk to humans (Mofijur et al., 2021).
Coastal areas worldwide face increasing pressure from anthropogenic activities, leading to various environmental concerns, including the pollution of aquatic ecosystems by heavy metals (Zhang et al., 2015; El Zokm et al., 2020). These contaminants are of particular concern due to their toxicity, persistence, and bioaccumulation, which pose significant threats to marine life and human health through the food chain (Tao et al., 2012; Briffa et al., 2020). Coastal sediments often serve as the final repository of contaminants originating from multiple sources (e.g., riverine input, atmospheric deposition, and direct industrial discharges) and usually act as important sinks for trace elements through adsorption and subsequent sedimentation (Al-Mur, 2021). Therefore, the pollution status of marine sediments has often been used as an important criterion to evaluate the condition of coastal environments and understand the possible environmental changes caused by anthropogenic activities (Kwok et al., 2014; Beltrame et al., 2009).
Furthermore, heavy metals present in sediments and seawater are assimilated by aquatic organisms and subsequently bioaccumulate through the food chain, posing a potential threat to marine ecosystems and human health (Zhou et al., 2022). Heavy metal concentrations in fish and other marine species are indicators of environmental contamination. By investigating heavy metals in marine fauna, we are able to assess the impact of heavy metals in the ambient environment on marine biodiversity and evaluate the potential risks to ecosystems (Singh and Turner, 2009)
Heavy metal contamination has emerged as a significant environmental challenge in contemporary times (Chen et al., 2023), drawing considerable attention due to its toxic nature, long-lasting effects, tendency to accumulate in living organisms, and resistance to degradation (Palansooriya et al., 2022; Wang et al., 2022). Thus pollution not only poses threats to aquatic life but also endangers human health and the marine ecosystem (Gu et al., 2021). Within riverine ecosystems, heavy metal pollution typically arises from both natural processes and human activities (Dan et al., 2022), with anthropogenic factors being the primary drivers (Li et al., 2022). Upon entering riverine environments, heavy metals often accumulate in sediments, where they interact with the overlying water (Gu et al., 2021), maintaining an equilibrium. However, alterations in environmental conditions such as pH and redox potential can lead to the release of these metals back into the water column, subsequently entering the food web (Bao et al., 2023). This process, characterized by bioaccumulation and biomagnification, has detrimental effects on aquatic ecosystems and poses risks to human health (Gu et al., 2023).
Metals are significant environmental contaminants due to their stability in the ecosystem, capacity to accumulate in living organisms, and their movement through the food chain (Sarmiento et al., 2016). These substances possess enduring presence in the environment, the ability to be taken up by living species, and the potential to accumulate and increase in concentration within the food chain (Vicente-Martorell et al., 2009). As a result, they can pose a significant threat to living organisms over an extended period of time (Agnaou et al., 2014). These components pose both carcinogenic and non-carcinogenic concerns, particularly affecting the brain and endocrine systems. The dangers are more pronounced in children compared to adults (Issac and Kandasubramanian, 2021).
Marine organisms are highly valuable for biomonitoring research due to their biological usefulness. As a result, they are commonly used as biological indicators to assess the environmental condition. They are crucial for assessing the impact of metal contamination on the ecosystem, particularly in the initial phases. The level of metal buildup in their bodies is influenced by physiological activities, particularly feeding, as well as variations in the physico-chemical characteristics of the environment (Vicente-Martorell et al., 2009).
In a similar manner, sediments accumulate significant amounts of heavy metals due to their low degradability. The concentration of metals in sediments is positively correlated with the abundance of organic matter in the sediment (Hsu et al., 2016). As a result, heavy metals build up in the sediment at the bottom of streams and in suspended solids (SS), which can be harmful to the environment, plants, animals, and human health because of their chemical characteristics. Living creatures can become contaminated by these metallic elements either through direct exposure to the pollution or indirectly through the trophic chain (Islam et al., 2018; Lei et al., 2011).
In the context of Pakistan, several studies have reported on different rivers. Still, a comprehensive study on the River Ravi regarding environmental pollution is lacking despite the continuous influx of artificial pollutants, including heavy metals, driven by increased human activity and industrial development (Abdul et al., 2009; Shahid et al., 2023). This study aims to provide a comprehensive analysis of heavy-metal concentrations in surface sediments, uncovering the extent of contamination and its ecological impacts, with the aim of helping policymakers in the River Ravi to devise effective environmental protection strategies.
The primary objectives of this study were: 1) to investigate the levels of heavy-metal concentrations in surface sediments to evaluate the potential ecological risks posed by these contaminants; 2) to describe the spatial distribution patterns of heavy-metal concentrations in sediments and to identify and quantify the respective sources and their contributions; and 3) human health risk assessment and; 4) to explore correlations among heavy metals in the River Ravi. The ultimate goal of this study is to provide strategic guidance for the management of heavy-metal pollution in the River Ravi.
2 Materials and methods
2.1 Study area
The River Ravi is a river that flows across borders, starting in the northeast of the Kangra district in Himachal Pradesh and the southeast of the Chamba district in the Jammu Kashmir Region of India (Chand and Sharma, 2015). It flows across Pakistan and serves as a notable tributary of the Indus System in the Punjab state (Akhtar et al., 2014). After covering a distance of 100 km from its upper catchments in India, the Ravi River enters Pakistan. The Ravi River is the most diminutive of the five other rivers in the Indus basin system that flow through Pakistan (Aslam et al., 2020).
The total length of the Ravi River is approximately 720 kilometres. The study region is situated within the latitude range of 31° 1’ 45.30” N to 31° 55’ 19.43” N and the longitude range of 73° 20’ 6.19” E to 74° 39’ 21.00” E. The study region is the Lahore division, which has four districts: Sheikhupura, Lahore, Kasur, and Nankana Sahib (Figure 1). The length of the Ravi River within the research region is 219.87 kilometres.
2.2 Sampling and analysis
This investigation involved the collection of sediment samples from various sites along the Ravi River. The collection of these samples were done during the May-June 2023. Twenty-two stations were sampled along the mainstream of the River. The sampling locations were chosen randomly. The sediment samples were obtained using a securely sealed grab sampler from a boat. Subsequently, the specimens were enclosed in plastic bags and preserved in an ice box maintained at 4°C. In the laboratory, samples were allowed to dry naturally at the ambient temperature. Before screening, the sediment samples were subjected to disaggregation and sorted into different particle sizes using a stainless-steel mesh (Feng et al., 2017). Samples were grounded to a fine powder using an electric agate mortar for less than 20 minutes, with around ten grams of each sample. The obtained powder was subsequently employed for the analysis of heavy metal presence. To conduct heavy metal analysis, 0.5 g of the powdered samples were treated with concentrated nitric acid (HNO3) and concentrated chloric acid (HClO3) in a ratio of 3:1 until practically all the liquid evaporated (Chester et al., 1994). Subsequently, deionized distilled water was added. After dilution, the insoluble residue was eliminated, and deionized water was added until the total volume reached 25 ml. The samples were analyzed for the existence of certain heavy metals using a flame atomic absorption spectrometer (AAS, GBC-932, Australia). To ensure the highest levels of accuracy, three replicates of each measurement were performed, with variations between them not exceeding 3% (Das et al., 2022).
2.3 Heavy metals analysis
The measurement of selected metals in sediment samples was done using the methodologies from the literature with certain modifications (Bu et al., 2020). After every fifth sample run, an examination was conducted to assess contamination and drift in a certified standard and a blank solution. All the chemicals and reagents utilized were of duly confirmed analytical purity. The metals were analyzed with a Buck 230ATS Atomic Absorption Spectrophotometer (AAS). The device was utilized at its utmost sensitivity and adjusted according to the manufacturer’s guidelines. The replication findings were computed following data acquisition for each metal parameter, expressed as milligrams per kilogram (mg/kg).
2.4 Pollution indicators
Sediment quality assessment involves the use of several indices to determine the extent of heavy-metal contamination. The indices employed include geo-accumulation index (Igeo), contamination factor (CF), and enrichment factor (EF). Collectively, these indices offer a robust framework for evaluating heavy-metal pollution in sediments. The degree of ecological risk associated with heavy-metal contamination can be systematically categorized as listed below.
2.4.1 Geo-accumulation index
Muller (1969) used this idea to measure the spatial arrangement of hazardous substances and their corresponding detrimental impacts within a particular ecosystem. The Igeo index is extensively used to assess pollution levels. The geo-accumulation index (Igeo) can be determined using Equation 1.
where Cn represents the measured metal in the sediment sample, Bn is the geochemical background concentration, many researchers have employed several elements such as silicon (Si), aluminium (Al), Manganese (Mn) and Fe (Nazeer et al., 2014; Yu et al., 2017). However, the current research uses Mn to normalize heavy metals (HMs).
2.4.2 Contamination factor
It is an important criteria for determining the source and extent of pollution caused by specific pollutants in the ecosystem. It serves as a direct sediment index, allowing for the estimation of individual pollution indexes. The CF was determined by the Equation 2.
Wang et al. (2006) classified the CF into four distinct categories, ranging from 1 to (see Table 1).
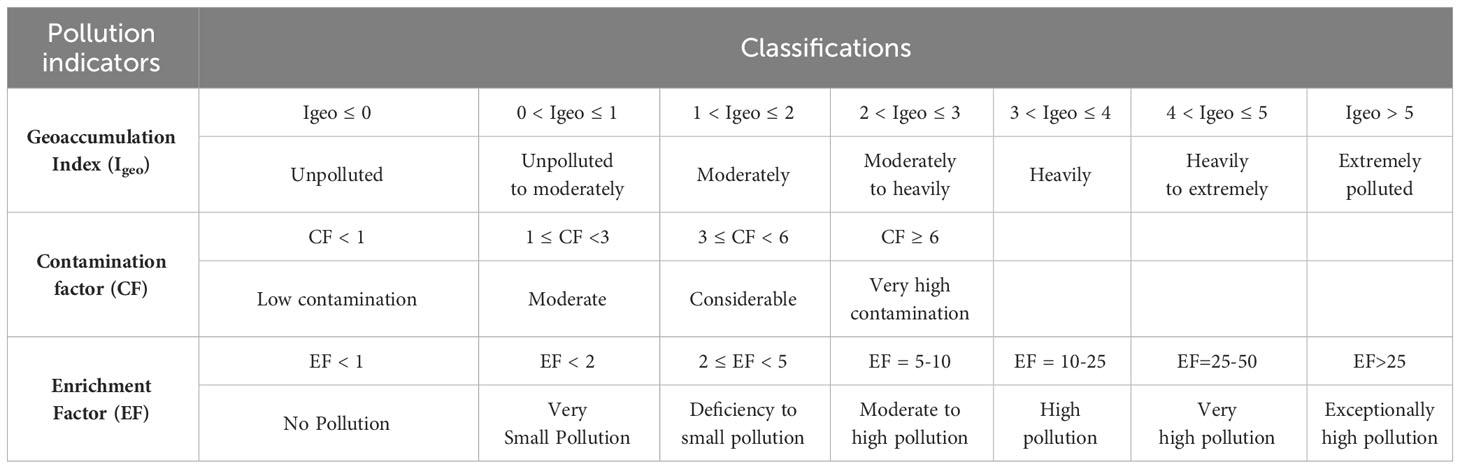
Table 1 Pollution indices used in the present study and their classifications of ecological risk degrees.
2.4.3 Enrichment factor
Enrichment factors (EF) are used to evaluate the pollution level and quantify the extent of human influence. The calculation used Equation 3 (Loska et al., 2004; Kara et al., 2014).
Ci is the metal element concentration with index i, measured in milligrams per kilogram (mg/kg). Cref represents the concentration of the reference element, also measured in mg/kg.
The data presented in Table 1 help to identify areas with elevated levels of heavy metals, prompting further investigation and remediation efforts. By proactively addressing these issues, their potential impact on both marine life and human health can be mitigated.
2.5 Health risk assessment
The non-cancerous and cancerous risk conditions due to exposure to heavy meats have been assessed by considering the overall metal content of specific heavy metals. The equations used in this investigation to calculate the human health risk assessment (HRA) associated with metal exposure are derived from existing literature and methodologies (Zheng et al., 2010; Li et al., 2015) (Table 2).
Hence, regardless of gender, children and adults were categorized. The metal exposure can occur through three main pathways: direct inhalation, dermal absorption,” and ingestion of deposited atmospheric particles as indicated in Equation 4–Equation 6
The metal content (mg/kg) is represented by C. The estimates are based on parameters provided by the literature (Li et al., 2015).
The HQ was measured per body weight and represented as milligrams per kilogram per day (mg/kg/day). To achieve this objective, the estimated ADDi values were used as the numerator, and the matching RfD values were used as the divisor to calculate HQi using Equation 7.
The study examines the maximum allowable risk of exposure to RfD, considering both children and adults, throughout a lifetime. If HQ< 1 (Zheng et al., 2010). Noncarcinogenic substances have a threshold limit below which no adverse response occurs. The RfD values employed in this study are cited by other studies (Li et al., 2015). The hazard index (HI), which is calculated by summing the hazard quotients (HQs) for the three main pathways of exposure, is represented by Equation 8 (De Miguel, 2007; Zheng et al., 2010).
A hazard index (HI) value of less than one is considered safe, while an HI value of more than 1 indicates the potential for non-cancerous events (Zheng et al., 2010; Hussain et al., 2015).
2.6 Statistical analysis
The heavy metals concentration was analyzed using Microsoft Office Excel to determine the minimum, maximum, and average concentrations and Pearson correlation analysis. ArcGIS software (version 10.8) was utilized for the spatial interpolations of heavy metal concentration in the River, employing spatial distribution techniques (Shetaia et al., 2023). The R programming language (Shetaia et al., 2023) was used to identify the correlation (corrplot) between heavy metals.
3 Results
3.1 Spatial distribution of the heavy metal
Figure 2 presents the statistical data and regional patterns of heavy metal concentrations in the sediment of River Ravi. The presence of heavy metals (HMs) in the soil is influenced by factors such as pH soil chemistry (including moisture, soil organic matter, clay, silt, and sand). The mean concentrations of metals were as follows: manganese (Mn) had the highest average value at 779.996 mg/kg, followed by nickel (Ni) at 131.163 mg/kg, chromium (Cr) at 129.594 mg/kg, zinc (Zn) at 128.112 mg/kg, copper (Cu) at 62.514 mg/kg, lead (Pb) at 50.504 mg/kg, arsenic (As) at 33.742 mg/kg, and cadmium (Cd) at 1.491 mg/kg. Regarding spatial distribution, most metal concentrations increase near densely inhabited and industrialized regions, particularly in the river section adjacent to Lahore. Various anthropogenic effluents are discharged into this area, such as home sewage, industrial wastes from the city, and agricultural wastes from neighbouring cultivated fields. Additionally, the presence of domestic and industrial waste may also contribute to the increased metal content. Zinc is readily absorbed by soil organic matter (SOM), although it is subsequently liberated in an acidic environment (Metsalu and Vilo, 2015; Nassar and Fahmy, 2016).
Water pollution significantly threatens aquatic life, including fish, shrimp, and crabs, thereby escalating health risks at various trophic levels (Ariyaee et al., 2015). This is particularly concerning for commercially valuable fish species because elevated levels of heavy metals could have deleterious health effects on humans, especially in vulnerable populations such as children, women, and individuals with pre-existing health conditions. Consequently, the vigilant monitoring of heavy-metal concentrations in organisms is imperative for the effective regulation and management of aquatic ecosystems, ensuring the safety of seafood for human consumption (Caeiro et al., 2005).
Table 3 summarizes the concentrations of heavy metals in the River Ravi with other rivers in Pakistan and globally. The River Ravi has moderate heavy metal concentration levels, with all measured elements falling within the range observed in other rivers, except for metals As, Cr, Ni, and Cu, which show higher levels at some sampling sites. The heightened levels of the elements in the River indicate that, in addition to the River’s natural flow, pollutants from other sources also infiltrate this unique ecosystem and contribute to the increase in pollution. Examples of causes contributing to water pollution can be categorized as anthropogenic, such as urban and industrial wastewater, and natural, connected to sediment texture and geological features (Hashemi, 2018). Upon comparing the average concentration of Arsenic (As) in various elements, it was discovered that the River Ravi exhibited a lower concentration (33.74 mg/kg) in comparison to the Zhejiang River in China (6.90–74.34 mg/kg) and the Yellow River in China (14-48 mg/kg) (Liu et al., 2009; Zhang et al., 2018). The Sediment of the River Ravi exhibited a significantly higher concentration of heavy metals compared to the Tanjan River in Iran (12.8 mg/kg) (Liu et al., 2016), the Yangtze River in China (29.9 mg/kg) (Yang et al., 2009), the Jialu River in China (2.39–14.57 mg/kg) (Fu et al., 2011), the Kabul River in Pakistan (1.26 mg/kg), the Chenab River in Pakistan (.65 mg/kg), and the Indus River in Pakistan (7.65 mg/kg) (Nawab et al., 2018). This phenomenon can be explained by the substantial influx of this element from rivers. Arsenic can be found naturally in rocks along the River, but it can also come from fertilizers and fungicides in rice crops along the River (Szolnoki et al., 2013; Wang et al., 2019).
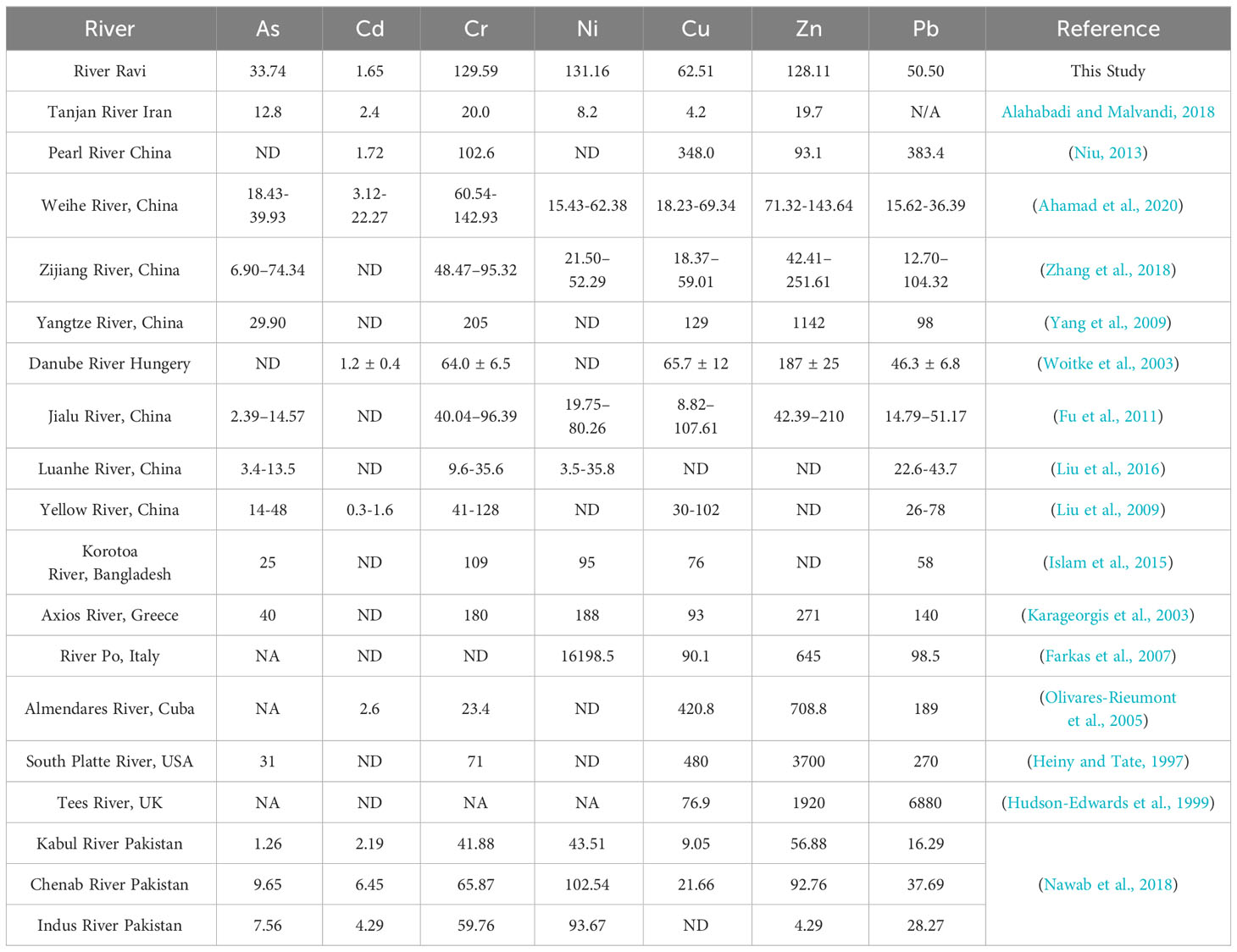
Table 3 Comparison of the heavy metal contents in the River Ravi surface sediment with other rivers of the world (unit mg/kg).
3.2 Pollutions levels
The heavy-metal concentrations in sediment samples can be evaluated using various pollution assessment indicators (Hahladakis et al., 2013; Liu et al., 2018; Zhou et al., 2022). These include the geo-accumulation index (Igeo), which measures the degree of contamination compared with pre-industrial levels; the contamination factor (CF), which quantifies the relative enrichment of metals; the enrichment factor (EF), which assesses the potential harm to living organisms. The levels of these indicators are listed below
The highest level of contamination, as indicated by the Igeo values, was attributed to Cd (-0.519 – 2.348), in agreement with the pollution degree defined by Igeo (Muller, 1969). The sediments in the research area are categorized as uncontaminated by copper (Cu), nickel (Ni), lead (Pb), manganese (Mn), iron (Fe), and zinc (Zn). The Igeo analysis indicates that the sediments of River Ravi displayed a range of pollution levels, ranging from moderate to extremely high, as depicted in Figure 3. The mean Igeo values ranged from -0.792 (Mn) to 1.583 (Cd), with the minimum and maximum Igeo values being -1.783 (Pb) and 2.348 (Cd), respectively. According to the criteria, it is essential to highlight that As, Cd, Cr, Ni, Cu, Zn, and Pb displayed distinct contamination patterns. Cadmium showed moderate pollution, whereas lead contamination varied from unpolluted to moderate. The soil samples primarily exhibited low pollution levels for chromium (Cr), nickel (Ni), copper (Cu), and zinc (Zn), ranging from unpolluted to moderately polluted. The soil analysis revealed varied degrees of contamination, ranging from non-polluted to moderately polluted, as indicated by the presence of As and Pb. The soil samples were sorted in descending order based on the average sequence of Igeo values as follows: Cd (1.583), As (0.654), Pb (0.467), Ni (0.328), Cr (-0.130), Zn (-0.213), Cu (-0.234), Mn (-0.792).
The contamination factor values exhibited a range of 0.436 (Pb) to 7.637 (Cd), with average values spanning from 0.9176 (Mn) to 4.9714 (Cd), suggesting significant regional variation. Analysis of the average contamination factor values from all test locations revealed no evidence of soil contamination by Mn. Nevertheless, As, Ni, Cr, Cu, and Zn in soils suggest a moderate pollution level. In contrast, Cd indicates a high level of contamination based on the criteria. The soils in different sampling sites displayed varying contamination patterns. Specifically, 9.0% of the sites had low contamination, 50% had moderate contamination, and 43.0% had considerable contamination. Regarding Cd (cadmium) levels in the soils, 9% had low contamination, 22% had moderate contamination, 36% had considerable contamination, and 33% exhibited very high contamination. Remarkably, the soil sample recovered from S-14 displayed the greatest Igeo and CF values for Cd, although elements like Cr, Cu, and Ni did not demonstrate a comparable trend. These data indicate that soil pollution in the study area is likely caused by processes other than only metal contamination.
The research region faces significant heavy metal pollution, as indicated by the Contamination Factor (CF) evaluation results. The Enrichment Factor (EF) was also utilized to assess the quantity of pollution caused by a particular metal (Figure 4). The EF analysis reveals a consistent pattern of pollution in heavy metals, which is also observed in the Igeo analysis. This indicates a certain amount of consistency in the assessed repercussions of human activities on pollution. Based on the EF categorization (Hakanson, 1980), approximately 55% of the sampling locations exhibited a moderate to high pollution level regarding Cd contamination. The average concentration of arsenic (As), lead (Pb), and nickel (Ni) indicate a low level of pollution, ranging from deficiency to minor contamination. The soil samples exhibited the following sequence of EF values: Cd (5.4581) > As (2.8254) > Pb (2.6236) > Ni (2.2159) > Cr (1.6189) > Zn (1.5159) > Cu (1.4985). The mean EF values for magnesium were below 1, suggesting a natural source. The Ni, Cr, Cu, and Zn levels ranged from 1 to 2, indicating that the sources are most likely of anthropogenic origin (Zhang et al., 2018). The concentrations of Cd, As, and Pb exhibited a moderate level of enrichment, suggesting the presence of human-induced impacts and a moderate impact from human activities (Figure 4).
3.3 Human health risk assessment
The study aimed to assess the potential human health risks from exposure to some aspects of the Ravi River. Specifically, the non-cancerous effects of arsenic (As), cadmium (Cd), chromium (Cr), nickel (Ni), copper (Cu), zinc (Zn), lead (Pb), and manganese (Mn), as well as the carcinogenic risks of As, Cd, Cr, Ni, Cu, and Pb, were investigated. The study focused on three main pathways of exposure: inhalation, ingestion, and dermal interaction. Figure 5 demonstrated that the eating route had the highest danger, followed by cutaneous interaction and inhalation. Other scholars have also documented this phenomenon (Xu et al., 2017; Ur Rehman et al., 2018). Among the metals tested, the sequence of causing health impairment in children through diverse exposure routes was as follows: Cd > Mn > Cr > Ni > Pb > As > Cu > Zn. A similar pattern was seen in adulthood except for Copper (Cu), which came before Nickel (Ni). The sequencing of the remainder of the metals in the HI sequence remained consistent. According to the health risk assessment model employed by USEPA and IRIS, it was determined that children had a higher level of exposure to metals compared to adults. This conclusion was based on many criteria and approaches. Children are more exposed to dust and soil particles than adults because of their active behaviour, extended playtime, and lack of caution while eating and drinking. Precautionary steps should be implemented to reduce children’s exposure to potentially harmful health conditions. Unlike several other studies (Xu et al., 2017; Ur Rehman et al., 2018). In our investigation, Cd exhibited the most considerable noncarcinogenic risk in adults, followed by As, Mn, Cr, Ni, Cu, Pb, and Zn. Hence, Cd, As, Mn, and Cr are the elements that should be given the highest consideration regarding their propensity to cause health concerns. Multiple paths contribute to the intake of harmful substances during the day and night. In addition to these pathways, various food products (both wet and dry) from the same area are significant sources of health hazards in toxicology and hygiene. The hazard quotient (HQ) values for children were more important than those for adults, although they were still within the safe limit (HQ < 1). However, due to exposure to Cd, the HQ (Hazard Quotient) for children exceeded the established limit of 1.08E+00. Furthermore, HQderm was discovered to exceed the acceptable threshold (HQ > 1) in both children and adults due to exposure (Aslam et al., 2020). The study demonstrates that Cd and As were the sole metals shown to have detrimental health impacts in our research. These effects are expected to intensify with an increase in the HQ value. Similarly, the computed Hazard Index (HI) values for children were higher than those for adults in all of the metals investigated. Nevertheless, the analysis revealed that all these elements’ exposure remained below the established safety threshold (HI < 1).
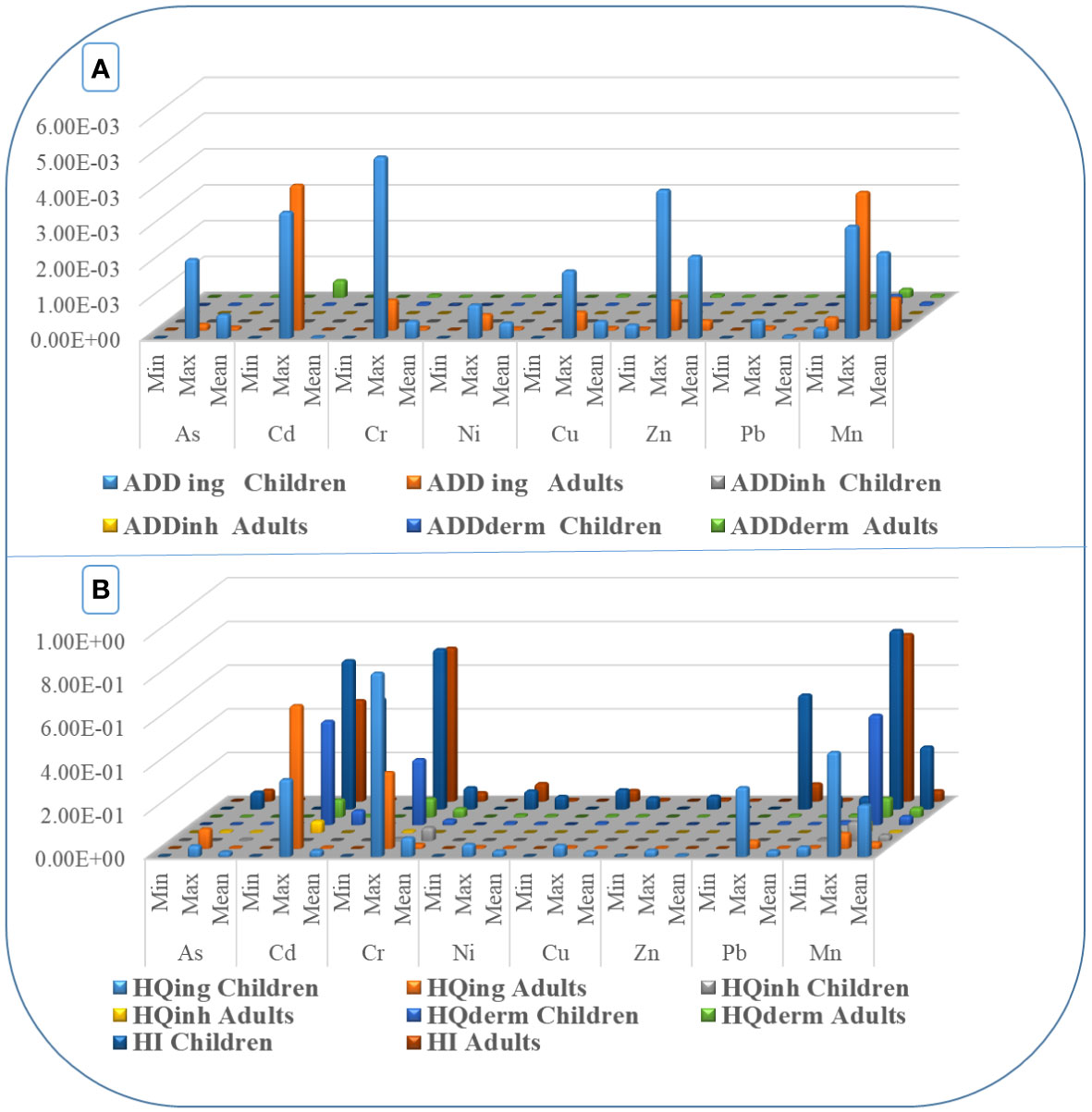
Figure 5 (A) Average Daily Dose (ADD measured in the unit of mg/kg/day) in three modes: (B) Health risk from heavy metals in the study area.
Figure 5B unambiguously demonstrated that the cancer risks associated with several metals studied much exceeded the permissible threshold of RI (1 × 10−4). The values for children were ranked in the following order: Cu (6.65E-03) > As (3.65E-03) > Ni (3.88E-04) > Cd (3.65E-04) > Cr (3.62E-04) and Pb (7.15E-07). Only As (5.22E-04) and Cu (4.22E-04) exhibited RI values above the acceptable limit for adults, indicating a high risk of human carcinogenicity. Nevertheless, Cd, Ni, Cr, and Pb concentrations were determined to be under the acceptable threshold of 1 × 10−4.
Correlation analyses can reflect whether different heavy metals share a common source or undergo similar contamination processes (Hu et al., 2013). To further elucidate the origins of the heavy metals in the sediments and quantify their respective source contributions, Positive Matrix Factorization (PMF) was employed for source apportionment and contribution elucidation.
The metal concentration in aquatic habitats is affected by anthropogenic activities and natural phenomena (Li et al., 2020). Human-induced pollution includes agricultural effluents caused by the overuse of pesticides, herbicides and fertilizers, together with traffic pollution, industrial discharges, and home wastewater (Magnusson et al., 2018; Shetaia et al., 2022). Furthermore, natural characteristics refer to an ecosystem’s chemical and physical properties, its environmental context, and the biological factors that affect it (Zhang et al., 2019; Ytreberg et al., 2022). The interrelationships among the analyzed metals can aid in determining the origin(s) of pollution (Zhao et al., 2023). Moreover, the HCA approach offers a fitting explanation for variables in the sample region that exhibit similar geochemical behaviour or have been impacted by human activities. The reference is attributed to Thiombane et al. (2018). Figure 6 displayed the correlation of the heavy metals samples.
The examination of heavy metals indicated a substantial correlation between the existence of arsenic (As) and manganese (Mn), as well as between As and chromium (Cr). Furthermore, a moderate positive association was detected between arsenic (As) and copper (Cu) elements with a correlation coefficient of 0.48. Corelation analysis of heavy metals showed that surface sediments of the River Ravi originate from natural sources and three anthropogenic sources. Anthropogenic source 1 originated primarily from industrial effluent, which is characterized by a high load of metals. Anthropogenic source 2 originated primarily from metal processing and vehicular activities. Anthropogenic source 3 originated primarily from coastal industrial activities and fossil fuel combustion.
Heavy metal pollution in rivers can have detrimental effects on aquatic ecosystems. Metals can accumulate in sediments, water, and biota, leading to toxicological effects on aquatic organisms. Rivers serve as conduits for transporting heavy metal pollution from inland areas to coastal regions and ultimately the ocean. As rivers discharge into the sea, they carry their pollutant loads, including dissolved metals and sediment-bound contaminants. Coastal ecosystems may act as sinks for heavy metals, where deposition and accumulation occur, affecting marine organisms and habitats (Zhang et al., 2019; Ytreberg et al., 2022; Zhao et al., 2023).
4 Conclusions
This study focused on the levels of heavy metals in seawater, surface sediments, and organisms in the River Ravi. A total of twenty-two stations along the main course of the River, including Sheikhupura (5), Lahore (9), Kasur (3), and Nankana Sahib (5), were sampled. The average Igeo values varied from -0.792 (Mn) to 1.583 (Cd), while the minimum and maximum Igeo values were -1.783 (Pb) and 2.348 (Cd), respectively. Cadmium exhibited a moderate pollution level, whereas lead showed contamination ranging from unpolluted to moderate. The contamination factor values varied between 0.436 (Pb) and 7.637 (Cd), with average values ranging from 0.9176 (Mn) to 4.9714 (Cd), indicating substantial regional diversity.The enrichment factor analysis indicates a consistent pattern of pollution for heavy metals, which is also observed in the Igeo analysis. Cadmium (Cd) exhibited significant pollution, ranging from moderate to high, at approximately 55% of the sampled locations, as determined by the EF categorization.
The noncarcinogenic risk associated with chromium exposure was higher than that of other selected elements for both children and adults. Furthermore, the carcinogenic risk associated with exposure to heavy metals such as Cd, Cr, and Zn in youngsters exceeded the established limits. Among adults, only Cadmium (Cd) presented a greater risk of cancer when exposed to it in comparison to other elements. The HMs showed a higher likelihood of noncarcinogenic hazards (HQ > 1) in children, ranked in decreasing order as follows: Cd > Mn > Cr > Ni > Pb > As > Cu > Zn. The analysis of heavy metals revealed a strong association between Arsenic (As) and Manganese (Mn) with a correlation coefficient (r) of 0.87. Similarly, a considerable correlation was seen between As and Chromium (Cr) with a r value 0.71.
In conclusion, As coastal-region development accelerates and the global population increasingly relies on marine resources, comprehending and mitigating the repercussions of human activities on coastal ecosystems has become imperative. The insights gained from this research are instrumental in crafting strategies that advocate sustainable development in coastal areas. These strategies strive to harmonize economic growth with the imperatives of environmental protection and public health.
Data availability statement
The original contributions presented in the study are included in the article/supplementary material. Further inquiries can be directed to the corresponding authors.
Author contributions
MIA: Conceptualization, Writing – original draft. ZY: Software, Writing – review & editing. LR: Investigation, Writing – review & editing. CZ: Data curation, Writing – review & editing. TL: Methodology, Writing – review & editing. HL: Supervision, Writing – review & editing. MSM: Formal Analysis, Writing – review & editing. AR: Validation, Writing – review & editing. MA: Visualization, Writing – review & editing. SL: Software, Writing – review & editing. WF: Writing – review & editing.
Funding
The author(s) declare financial support was received for the research, authorship, and/or publication of this article. This study is under the auspices of NSFC 42071267, the Scientific and Technological Research Projects in Henan Province (242102321158, 232102320047) and Xinyang Academy of Ecological Research Open Foundation (2023XYMS02) and the Postdoctoral startup research fund of Henan University number (FJ3050A0670814).
Acknowledgments
We thank the editor and reviewer for their valuable comments and suggestions. We are also thankful to our lab fellow and all those who assisted during this study.
Conflict of interest
The authors declare that the research was conducted in the absence of any commercial or financial relationships that could be construed as a potential conflict of interest.
Publisher’s note
All claims expressed in this article are solely those of the authors and do not necessarily represent those of their affiliated organizations, or those of the publisher, the editors and the reviewers. Any product that may be evaluated in this article, or claim that may be made by its manufacturer, is not guaranteed or endorsed by the publisher.
References
Abdul R., Muhammad J., Muhammad U., Sajid A. (2009). Assessment of heavy metals in sediments of the river Ravi, Pakistan. Int. J. Agric. Biol. 11, 197–200.
Agnaou M., Ait Alla A., Ouassas M., Bazzi L., El Alami Z., Moukrim A. (2014). Assessment of organochlorine pesticides contamination of oued souss estuary (south of Morocco): seasonal variability in sediment and a detritivore annelid Neries diversicolor. J. Mater. Environ. Sci. 5, 581–586.
Ahamad M. I., Song J., Sun H., Wang X., Mehmood M. S., Sajid M., et al. (2020). Contamination level, ecological risk, and source identification of heavy metals in the hyporheic zone of the Weihe River, China. Int. J. Environ. Res. Public Health 17, 1070. doi: 10.3390/ijerph17031070
Akhtar M., Mahboob S., Sultana S., Sultana T. (2014). Pesticides in the River Ravi and its Tributaries Between its Stretches from Shahdara to Balloki Headworks, Punjab-Pakistan. Water Environ. Res. 86, 13–19. doi: 10.2175/106143013X13807328848612
Alahabadi A., Malvandi H. (2018). Contamination and ecological risk assessment of heavy metals and metalloids in surface sediments of the Tajan River, Iran. Mar. pollut. Bull. 133, 741–749. doi: 10.1016/j.marpolbul.2018.06.030
Al-Mur B. A. (2021). Assessment of heavy metal contamination in water, sediments, and Mangrove plant of Al-Budhai region, Red Sea Coast, Kingdom of Saudi Arabia. J. Taibah Univ. Sci. 15, 423–441. doi: 10.1080/16583655.2021.1985871
Ariyaee M., Azadi N. A., Majnoni F., Mansouri B. (2015). Comparison of metal concentrations in the organs of two fish species from the zabol chahnimeh reservoirs, iran. Bull. Environ. Contamination Toxicol. 94, 715–721.
Aslam R. A., Shrestha S., Pala I., Ninsawat S., Shanmugam M. S., Anwar S. (2020). Projections of climatic extremes in a data poor transboundary river basin of India and Pakistan. Int. J. Climatol 40, 4992–5010. doi: 10.1002/joc.6501
Bao T. L., Wang P. F., Hu B., Wang X., Qian J. (2023). Mobilization of colloids during sediment resuspension and its effect on the release of heavy metals and dissolved organic matter. Sci. Total Environ. 861, 160678. doi: 10.1016/j.scitotenv.2022.160678
Beltrame M. O., De Marco S. G., Marcovecchio J. E. (2009). Dissolved and particulate heavy metals distribution in coastal lagoons. A case study from Mar Chiquita Lagoon, Argentina. Estuarine Coast. Shelf Sci. 85, 45–56. doi: 10.1016/j.ecss.2009.04.027
Briffa J., Sinagra E., Blundell R. (2020). Heavy metal pollution in the environment and their toxicological effects on humans. Heliyon 6, e04691. doi: 10.1016/j.heliyon.2020.e04691
Bu Q., Li Q., Zhang H., Cao H., Gong W., Zhang X., et al. (2020). Concentrations, spatial distributions, and sources of heavy metals in surface soils of the Coal Mining City Wuhai, China. J. Chem. 2020, 1–10. doi: 10.1155/2020/4705954
Caeiro S., Costa M. H., Ramos T. B., Fernandes F., Silveira N., Coimbra A., et al. (2005). Assessing heavy metal contamination in Sado Estuary sediment: An index analysis approach. Ecol. Indic. 5, 151–169. doi: 10.1016/j.ecolind.2005.02.001
Chand P., Sharma M. C. (2015). Glacier changes in the Ravi basin, North-Western Himalaya (India) during the last four decades, (1971–2010/13). Glob. Planet. Chang 135, 133–147. doi: 10.1016/j.gloplacha.2015.10.013
Chen D., Wang X. H., Luo X. M., Huang G. X., Tian Z., Li W. Y., et al. (2023). Delineating and identifying risk zones of soil heavy metal pollution in an industrialized region using machine learning. Environ. pollut. (Amsterdam Neth.) 318, 120932. doi: 10.1016/j.envpol.2022.120932
Chen J., Zhang H., Xue J., Yuan L., Yao W., Wu H. (2022). Study on spatial distribution, potential sources and ecological risk of heavy metals in the surface water and sediments at Shanghai Port, China. Mar. pollut. Bull. 181, 113923. doi: 10.1016/j.marpolbul.2022.113923
Chester R., Lin F. J., Basaham A. S. (1994). Trace metal solid state speciation changes associated with the down-column fluxes of oceanic particulates. J. Geol. Soc 151, 351–360. doi: 10.1144/gsjgs.151.2.0351
Dan S. F., Udoh E. C., Wang Q. Q. (2022). Contamination and ecological risk assessment of heavy metals, and relationship with organic matter sources in surface sediments of the Cross River Estuary and nearshore areas. J. Hazard Mater. 438, 129531. doi: 10.1016/j.jhazmat.2022.129531
Das H., Deka J., Singh A., Barman P. (2022). Study of adsorption of copper, lead, and zinc in their various chemical forms from the sediments of the Brahmaputra river, Assam, India. Environ. Qual. Manage. 31, 67–78. doi: 10.1002/tqem.21763
De Miguel E., Iribarren I., Chacon E., Ordonez A., Charlesworth S. (2007). Risk-based evaluation of the exposure of children to trace elements in playgrounds in madrid (Spain). Chemosphere 66 (3), 505–513.
El Zokm G., Al-Mur B., Okbah M. (2020). Ecological risk indices for heavy metal pollution assessment in marine sediments of Jeddah Coast in the Red Sea. Int. J. Environ. Analytical Chem. 102, 1–22.
Farkas A., Erratico C., Viganò L. (2007). Assessment of the environmental significance of heavy metal pollution in surficial sediments of the River Po. Chemosphere 68, 761–768. doi: 10.1016/j.chemosphere.2006.12.099
Feng D., Chen X., Tian W., Qian Q., Shen H., Liao D., et al. (2017). Pollution characteristics and ecological risk of heavy metals in ballast tank sediment. Environ. Sci. pollut. Res. 24, 3951–3958. doi: 10.1007/s11356-016-8113-z
Fu Z., Wu F., Mo C., Liu B., Zhu J., Deng Q., et al. (2011). Bioaccumulation of antimony, arsenic, and mercury in the vicinities of a large antimony mine, China. Microchem. J. 97, 12–19. doi: 10.1016/j.microc.2010.06.004
Gu Y. G., Wang Y. S., Ouyang J., Jordan R. W., Jiang S. J. (2021). Impacts of coastal aquaculture on sedimentary phosphorus speciation and fate: evidence from a seaweed cultivation area off Nan’ao Island, South China. Mar. pollut. Bull. 171, 112719. doi: 10.1016/j.marpolbul.2021.112719
Gu Y. G., Jiang S. J., Jordan R. W., Huang H. H., Wu F. X. (2023). Nonmetric multidimensional scaling and probabilistic ecological risk assessment of trace metals in surface sediments of daya bay (China) using diffusive gradients in thin films. Sci. Total Environ. 867, 161433.
Hahladakis J., Smaragdaki E., Vasilaki G., Gidarakos E. (2013). Use of Sediment Quality Guidelines and pollution indicators for the assessment of heavy metal and PAH contamination in Greek surficial sea and lake sediments. Environ. Monit. Assess. 185, 2843–2853. doi: 10.1007/s10661-012-2754-2
Hakanson L. (1980). An ecological risk index for aquatic pollution control. A sedimentological approach. Water Res. 14, 975–1001. doi: 10.1016/0043-1354(80)90143-8
Hashemi M. (2018). Heavy metal concentrations in bovine tissues (muscle, liver and kidney) and their relationship with heavy metal contents in consumed feed. Ecotoxicology Environ. Saf. 154, 263–267. doi: 10.1016/j.ecoenv.2018.02.058
Heiny J. S., Tate C. M. (1997). Concentration, distribution, and comparison of selected trace elements in bed sediment and fish tissue in the South Platte River Basin, USA 1992–1993. Arch. Environ. Contam. Toxicol. 32, 246–259. doi: 10.1007/s002449900182
Hsu L.-C., Chen K.-Y., Chan Y.-T., Deng Y., Hwang C.-E., Liu Y.-T., et al. (2016). MS title: catalytic oxidation and removal of arsenite in the presence of Fe ions and zero-valent Al metals. J. Hazard. Mater. 317, 237–245. doi: 10.1016/j.jhazmat.2016.05.071
Hu B., Li J., Zhao J., Yang J., Bai F., Dou Y. (2013). Heavy metal in surface sediments of the Liaodong Bay, Bohai Sea: distribution, contamination, and sources. Environ. Monit. Assess. 185, 5071–5083. doi: 10.1007/s10661-012-2926-0
Hudson-Edwards K. A., Macklin M. G., Taylor M. P. (1999). 2000 years of sediment-borne heavy metal storage in the Yorkshire Ouse basin, NE England, UK. Hydrol. Process. 13, 1087–1102. doi: 10.1002/(ISSN)1099-1085
Hussain R., Khattak S. A., Shah M. T., Ali L. (2015). Multistatistical approaches for environmental geochemical assessment of pollutants in soils of Gadoon Amazai Industrial Estate, Pakistan. J. Soils Sediment. 15, 1119–1129. doi: 10.1007/s11368-015-1075-9
Islam M. S., Ahmed M. K., Raknuzzaman M., Habibullah -Al- Mamun M., Islam M. K. (2015). Heavy metal pollution in surface water and Sediment: A preliminary assessment of an urban river in a developing country. Ecol. Indic. 48, 282–291. doi: 10.1016/j.ecolind.2014.08.016
Islam M. S., Hossain M. B., Matin A., Sarker M. S. I. (2018). Assessment of heavy metal pollution, distribution and source apportionment in the Sediment from Feni River estuary, Bangladesh. Chemosphere 202, 25e32. doi: 10.1016/j.chemosphere.2018.03.077
Issac M. N., Kandasubramanian B. (2021). Effect of microplastics in water and aquatic systems. Environ. Sci. pollut. Res. 28, 19544–19562. doi: 10.1007/s11356-021-13184-2
Kara M., Dumanoglu Y., Altiok H., Elbir T., Odabasi M., Bayram A. (2014). Spatial distribution and source identification of trace elements in topsoil from heavily industrialized region, Aliaga, Turkey. Environ. Monit. Assess. 186, 6017e6038. doi: 10.1007/s10661-014-3837-z
Karageorgis A., Nikolaidis N., Karamanos H., Skoulikidis N. (2003). Water and sediment quality assessment of the Axios River and its coastal environment. Cont. Shelf Res. 23, 1929–1944. doi: 10.1016/j.csr.2003.06.009
Kwok C. K., Liang Y., Wang H., Dong Y. H., Leung S. Y., Wong M. H. (2014). Bioaccumulation of heavy metals in fish and Ardeid at Pearl River Estuary, China. Ecotoxicology Environ. Saf. 106, 62–67. doi: 10.1016/j.ecoenv.2014.04.016
Lei F., Wei C., He X., Zhang R., Xu M., Jiang F. (2011). Heavy metals content and pollution assessment in benthon of Qinzhou Bay coastal waters. J. Guangxi Acad. Sci. 27, 351–354.
Li P., Qian H., Howard K. W., Wu J. (2015). Heavy metal contamination of Yellow River alluvial sediments, northwest China. Environ. Earth Sci. 73, 3403–3415. doi: 10.1007/s12665-014-3628-4
Li C. M., Wang H. C., Liao X. L., Xiao R., Liu K. H., Bai J. H., et al. (2022). Heavy metal pollution in coastal wetlands: a systematic review of studies globally over the past three decades. J. Hazard Mater. 424, 127312. doi: 10.1016/j.jhazmat.2021.127312
Li M., Zhang Q., Sun X., Karki K., Zeng C., Pandey A., et al. (2020). Heavy metals in surface sediments in the trans-Himalayan Koshi River catchment: distribution, source identification and pollution assessment. Chemosphere 244, 125410. doi: 10.1016/j.chemosphere.2019.125410
Liu C. C., Xu J., Liu C. C., Zhang P., Dai M. (2009). Heavy metals in the surface sediments in Lanzhou Reach of Yellow River, China. Bull. Environ. Contam. Toxicol. 82, 26–30. doi: 10.1007/s00128-008-9563-x
Liu J., Yin P., Chen B., Gao F., Song H., Li M. (2016). Distribution and contamination assessment of heavy metals in surface sediments of the Luanhe River Estuary, northwest of the Bohai Sea. Mar. pollut. Bull. 109, 633–639. doi: 10.1016/j.marpolbul.2016.05.020
Liu Y., Wang Q., Zhuang W., Yuan Y., Yuan Y., Jiao K., et al. (2018). Calculation of thallium's toxicity coefficient in the evaluation of potential ecological risk index: A case study. Chemosphere 194, 562–569.
Loska K., Wiechuła D., Korus I. (2004). Metal contamination of farming soils affected by industry. Environ. Int. 30, 159–165. doi: 10.1016/S0160-4120(03)00157-0
Magnusson K., Jalkanen J.-P., Johansson L., Smailys V., Telemo P., Winnes H. (2018). Risk assessment of bilge water discharges in two Baltic shipping lanes. Mar. pollut. Bull. 126, 575–584. doi: 10.1016/j.marpolbul.2017.09.035
Metsalu T., Vilo J. (2015). ClustVis: a web tool for visualizing clustering of multivariate data using principal component analysis and heatmap. Nucleic Acids Res. 43, W566–W570. doi: 10.1093/nar/gkv468
Mofijur M., Fattah I. M. R., Alam M. A., Islam A. B. M. S., Ong H. C., Rahman S. M. A., et al. (2021). Impact of COVID-19 on the social, economic, environmental and energy domains: lessons learnt from a global pandemic. Sustain. Prod. Consum. 26, 343–359. doi: 10.1016/j.spc.2020.10.016
Muller C. H. (1969). Allelopathy as a factor in ecological process. Vegetatio, 348–357. doi: 10.1007/BF00332847
Nassar M. Z. A., Fahmy M. A. (2016). Effects of some environmental factors on the population density and species diversity of phytoplankton in Bitter Lakes, Egypt. Rendiconti Lincei 27, 291–298. doi: 10.1007/s12210-015-0476-4
Nawab J., Farooqi S., Xiaoping W., Khan S., Khan A. (2018). Levels, dietary intake, and health risk of potentially toxic metals in vegetables, fruits, and cereal crops in Pakistan. Environ. Sci. pollut. Res. 25, 5558–5571. doi: 10.1007/s11356-017-0764-x
Nazeer S., Hashmi M. Z., Malik R. N. (2014). Heavy metals distribution, risk assessment and water quality characterization by water quality index of the River Soan, Pakistan. Ecol. Indic. 43, 262–270. doi: 10.1016/j.ecolind.2014.03.010
Niu J. (2013). Precipitation in the Pearl River basin, South China: scaling, regional patterns, and influence of large-scale climate anomalies. Stochastic Environ. Res. Risk Assess. 27, 1253–1268. doi: 10.1007/s00477-012-0661-2
Olivares-Rieumont S., de la Rosa D., Lima L., Graham D. W., D′ Alessandro K., Borroto J., et al. (2005). Assessment of heavy metal levels in Almendares River sediments—Havana City, Cuba. Water Res. 39, 3945–3953. doi: 10.1016/j.watres.2005.07.011
Palansooriya K. N., Li J., Dissanayake P. D., Suvarna M., Li L., Yuan X., et al. (2022). Prediction of soil heavy metal immobilization by biochar using machine learning. Environ. Sci. Technol. 56, 4187–4198. doi: 10.1021/acs.est.1c08302
Sarmiento A. M., Bonnail E., Nieto J. M., DelValls A. (2016). Bioavailability and toxicity of metals from a contaminated sediment by acid mine drainage: linking exposure–response relationships of the freshwater bivalve Corbicula fluminea to contaminated sediment. Environ. Sci. pollut. Res. 23, 22957–22967. doi: 10.1007/s11356-016-7464-9
Shahid S. U., Abbasi N. A., Tahir A., Ahmad S., Ahmad S. R. (2023). Health risk assessment and geospatial analysis of arsenic contamination in shallow aquifer along Ravi River, Lahore, Pakistan. Environ. Sci. pollut. Res. 30, 4866–4880. doi: 10.1007/s11356-022-22458-2
Shetaia S. A., Abu Khatita A. M., Abdelhafez N. A., Shaker I. M., El Kafrawy S. B. (2022). Human-induced sediment degradation of Burullus lagoon, Nile Delta, Egypt: heavy metals pollution status and potential ecological risk. Mar. pollut. Bull. 178, 113566. doi: 10.1016/j.marpolbul.2022.113566
Shetaia S. A., Nasr R. A., El Saeed R. L., Dar M. A., Al-Mur B. A., Zakaly H. M. (2023). Assessment of heavy metals contamination of sediments and surface waters of Bitter lake, Suez Canal, Egypt: Ecological risks and human health. Mar. pollut. Bull. 192, 115096. doi: 10.1016/j.marpolbul.2023.115096
Singh N., Turner A. (2009). Trace metals in antifouling paint particles and their heterogeneous contamination of coastal sediments. Mar. pollut. Bull. 58, 559–564. doi: 10.1016/j.marpolbul.2008.11.014
Svavarsson J., Guls H. D., Sham R. C., Leung K. M. Y., Halldorsson H. P. (2021). Pollutants from shipping-new environmental challenges in the subarctic and the Arctic Ocean. Mar. pollut. Bull. 164, 112004. doi: 10.1016/j.marpolbul.2021.112004
Szolnoki Z., Farsang A., Puskas I. (2013). Cumulative impacts of human activities on urban garden soils: origin and accumulation of metals. Environ. pollut. 177, 106e115. doi: 10.1016/j.envpol.2013.02.007
Tao Y., Yuan Z., Xiaona H., Wei M. (2012). Distribution and bioaccumulation of heavy metals in aquatic organisms of different trophic levels and potential health risk assessment from Taihu lake, China. Ecotoxicology Environ. Saf. 81, 55–64. doi: 10.1016/j.ecoenv.2012.04.014
Thiombane M., Petrik A., Di Bonito M., Albanese S., Zuzolo D., Cicchella D., et al. (2018). Status, sources and contamination levels of organochlorine pesticide residues in urban and agricultural areas: a preliminary review in central–southern Italian soils. Environ. Sci. pollut. Res. 25, 26361–26382. doi: 10.1007/s11356-018-2688-5
Ur Rehman I., Ishaq M., Ali L., Khan S., Ahmad I., Din I. U., et al. (2018). Enrichment, spatial distribution of potential ecological and human health risk assessment via toxic metals in soil and surface water ingestion in the vicinity of Sewakht mines, district Chitral, Northern Pakistan. Ecotoxicology Environ. Saf. 154, 127–136. doi: 10.1016/j.ecoenv.2018.02.033
Vicente-Martorell J. J., Galindo-Riaño M. D., García-Vargas M., Granado-Castro M. D. (2009). Bioavailability of heavy metals monitoring water, sediments and fish species from a polluted estuary. J. Hazard. Mater. 162, 823–836. doi: 10.1016/j.jhazmat.2008.05.106
Wang W., Bai J., Zhang G., Jia J., Wang X., Liu X., et al. (2019). Occurrence, sources and ecotoxicological risks of polychlorinated biphenyls (PCBs) in sediment cores from urban, rural and reclamation-affected rivers of the Pearl River Delta, China. Chemosphere 218, 359e367. doi: 10.1016/j.chemosphere.2018.11.046
Wang X. N., Gu Y. G., Wang Z. H. (2022). Rare earth elements in different trophic level marine wild fish species. Environ. pollut. (Amsterdam Neth.) 292, 118346. doi: 10.1016/j.envpol.2021.118346
Wang J. X., Sun X. W., Yang Y., Huang H., Lee Y. C., Tan O. K., et al. (2006). Hydrothermally grown oriented ZnO nanorod arrays for gas sensing applications. Nanotechnology 17, 4995. doi: 10.1088/0957-4484/17/19/037
Woitke P., Wellmitz J., Helm D., Kube P., Lepom P., Litheraty P. (2003). Analysis and assessment of heavy metal pollution in suspended solids and sediments of the River Danube. Chemosphere 51, 633–642. doi: 10.1016/S0045-6535(03)00217-0
Xu Y., Wu Y., Han J., Li P. (2017). The current status of heavy metal in lake sediments from China: Pollution and ecological risk assessment. Ecol. Evol. 7, 5454–5466. doi: 10.1002/ece3.3124
Yang Z., Wang Y., Shen Z., Niu J., Tang Z. (2009). Distribution and speciation of heavy metals in sediments from the mainstream, tributaries, and lakes of the Yangtze River catchment of Wuhan, China. J. Hazard. Mater. 166, 1186–1194. doi: 10.1016/j.jhazmat.2008.12.034
Ytreberg E., Hansson K., Hermansson A. L., Parsmo R., Lagerström M., Jalkanen J.-P., et al. (2022). Metal and PAH loads from ships and boats, relative other sources, in the Baltic Sea. Mar. pollut. Bull. 182, 113904. doi: 10.1016/j.marpolbul.2022.113904
Yu S., Hong B., Ma J., Chen Y., Xi X., Gao J., et al. (2017). Surface sediment quality relative to port activities: a contaminant-spectrum assessment. Sci. Total Environ. 596–597, 342–350. doi: 10.1016/j.scitotenv.2017.04.076
Zhang Z., Lu Y., Li H., Tu Y., Liu B., Yang Z. (2018). Assessment of heavy metal contamination, distribution and source identification in the sediments from the Zijiang River, China. Sci. Total Environ. 645, 235–243. doi: 10.1016/j.scitotenv.2018.07.026
Zhang Z., Pei N., Sun Y., Li J., Li X., Yu S., et al. (2019). Halogenated organic pollutants in sediments and organisms from mangrove wetlands of the Jiulong River Estuary, South China. Environ. Res. 171, 145e152. doi: 10.1016/j.envres.2019.01.028
Zhang L., Shi Z., Zhang J., Jiang Z., Wang F., Huang X. (2015). Spatial and seasonal characteristics of dissolved heavy metals in the east and west Guangdong coastal waters, South China. Mar. pollut. Bull. 95, 419–426. doi: 10.1016/j.marpolbul.2015.03.035
Zhao Z., Liu Q., Liao Y., Yu P., Tang Y., Liu Q., et al. (2023). Ecological risk assessment of trace metals in sediments and their effect on benthic organisms from the south coast of Zhejiang province, China. Mar. pollut. Bull. 187, 114529. doi: 10.1016/j.marpolbul.2022.114529
Zheng N., Liu J., Wang Q., Liang Z. (2010). Health risk assessment of heavy metal exposure to street dust in the zinc smelting district, Northeast of China. Sci. Total Environ. 408, 726e733. doi: 10.1016/j.scitotenv.2009.10.075
Keywords: heavy metals, risk assessment, geoaccumulation index, carcinogenic risk, River Ravi
Citation: Ahamad MI, Yao Z, Ren L, Zhang C, Li T, Lu H, Mehmood MS, Rehman A, Adil M, Lu S and Feng W (2024) Impact of heavy metals on aquatic life and human health: a case study of River Ravi Pakistan. Front. Mar. Sci. 11:1374835. doi: 10.3389/fmars.2024.1374835
Received: 22 January 2024; Accepted: 20 February 2024;
Published: 01 May 2024.
Edited by:
Abida Farooqi, Quaid-i-Azam University, PakistanReviewed by:
Xian Sun, Sun Yat-sen University, ChinaSivalingam Periyasamy, National Research Council (CNR), Italy
Copyright © 2024 Ahamad, Yao, Ren, Zhang, Li, Lu, Mehmood, Rehman, Adil, Lu and Feng. This is an open-access article distributed under the terms of the Creative Commons Attribution License (CC BY). The use, distribution or reproduction in other forums is permitted, provided the original author(s) and the copyright owner(s) are credited and that the original publication in this journal is cited, in accordance with accepted academic practice. No use, distribution or reproduction is permitted which does not comply with these terms.
*Correspondence: Heli Lu, luheli@vip.henu.edu.cn; Siqi Lu, siqi.lu@uconn.edu