- 1School of Life and Environmental Sciences, Faculty of Science, Engineering and Built Environment, Deakin University, Melbourne, VIC, Australia
- 2Faculty of Science, Gent University, Gent, Belgium
- 3National Fisheries Intelligence Service, Fisheries and Oceans Canada, Winnipeg, MB, Canada
Introduction: Knowledge of the hunting strategies of top predators can provide insights into the cost-benefit trade-offs of their foraging activities. Air-breathing marine predators are constrained in their foraging activities due to their metabolic expenditure at depth being supported by limited body oxygen stores. Understanding how these species adapt their behaviours to maximise foraging success is of importance in view of the anticipated alterations to marine ecosystems in response to global change. The Australian fur seal (Arctocephalus pusillus doriferus), the largest fur seal species, has a distribution restricted to south-eastern Australia, which is one of the fastest warming oceanic regions and where the abundance, distribution and diversity of prey species is expected to change in coming decades.
Methods: In the present study, combined IMU (acceleration, magnetometer, gyroscope), depth and GPS data logger information was used to reconstruct 3-dimensional tracks during diving, assess energy expenditure and quantify prey capture events in adult female Australian fur seals during benthic foraging.
Results: The results revealed that individuals ascended at steeper pitches (to reduce transit time), remained for shorter durations and travelled shorter distances at the surface, and then descended at steeper pitches on subsequent dives after predatory events on the seafloor. Higher travel speeds and more directional changes during searching for prey along the seafloor, while requiring greater energy expenditure, were associated with more prey captures. Interestingly, individuals did not display conventional Area Restricted Search, with the heading between dives not influenced by prey encounters.
Discussion: Together, these results suggest Australian fur seals undertake rapid searching along the seafloor to surprise cryptic prey and, if prey is encountered, undertake rapid surfacing (to reload body oxygen stores) and return to nearby seafloor habitat with a similar but undisturbed prey field.
1 Introduction
Individual survival and fitness are linked to the success of foraging activities (Morse and Fritz, 1987), with long-term impacts on foraging success affecting population growth and survival of the species (Pyke, 1984). Foraging activities are the result of interactions between functional performance, ecological features, and energetic needs (Toscano et al., 2016). Optimization of foraging behaviours by individuals is based on evaluating the costs (time and energy expended) and benefits (energy and nutrients gained) of engaging in a particular strategy (Mobbs et al., 2018). Over the long-term, the energy gained must be higher than the energy expended in searching, capturing and handling prey (MacArthur and Pianka, 1966) in order to balance the energy expenditure of living (Stephens et al., 2007).
The foraging behaviours of air-breathing marine predators are constrained by their oxygen stores limiting the time they can spend submerged in search of prey (Andrews and Enstipp, 2016). Two primary foraging strategies (pelagic and benthic) have been described in these species, with differences in the duration of the bottom phase of the dive (Arnould and Costa, 2006) and distribution of prey items (Doniol-Valcroze et al., 2011). Pelagic foragers use the entirety of the dive in search of prey (Arnould and Costa, 2006), exploiting high-density patches of small schooling species (fish, crustacea and cephalopods) which can be distributed throughout the water column (Chimienti et al., 2017). In contrast, benthic foragers search for larger, cryptic prey on the seafloor (Meyers et al., 2021). The high energetic costs of diving to the sea floor (Costa, 1991) are balanced by the profitability of spatial and temporal predictability of benthic prey abundance and distribution (Arnould and Costa, 2006).
Pelagic environments are characterised by spatially variable patches of food resources in high marine productivity areas (Harcourt et al., 2002). Correspondingly, once profitable habitats have been encountered, pelagic predators have been observed to undertake Area Restricted Search (ARS) (Paiva et al., 2010) to maximise the rate of prey encounter (Pinaud, 2008). This behaviour involves a series of slow and sinuous movements scanning the area for prey (Weimerskirch, 2007), increasing residence time in productive resource areas (Fauchald and Tveraa, 2003). It has been shown to be prevalent in areas of high prey abundance and low competition (Salton et al., 2022). In addition, during ARS, effective prey detection is greater than direct detection distance (Tinbergen et al., 1967) and, therefore, such a strategy is assumed to decrease energy expenditure while searching for prey (Regular et al., 2013). However, some studies have shown that ARS does not necessarily reflect foraging success and that straight line searching can be more profitable in some instances (Weimerskirch et al., 2007; Hoskins et al., 2015b). In contrast, benthic environments are characterized by more spatially consistent but lower prey abundance (Snelgrove, 2001). Relatively little is known about which strategies air-breathing marine predators use for optimizing search patterns in benthic habitats (Takahashi et al., 2003; Maxwell et al., 2012; Blakeway et al., 2021).
The Australian fur seal (Arctocephalus pusillus doriferus, hereafter referred to as AUFS) is the largest fur seal species, with mean adult female and male body mass of 76 kg and 270 kg, respectively (Kirkwood and Arnould, 2008). The breeding distribution of AUFS is mostly restricted to Bass Strait, a shallow (60-80 m) continental shelf area of low marine primary productivity between the Australian mainland and Tasmania (Kirkman and Arnould, 2018) influenced by numerous oceanographic currents (Fandry, 1981). While its population is still recovering from the over-exploitation of the commercial sealing era (18th-19th centuries), the AUFS represents the largest resident marine predator biomass in the region (Kirkwood and Arnould, 2012).
The foraging range of AUFS is restricted to the continental shelf where diving is almost exclusively benthic (Arnould and Hindell, 2001; Arnould and Kirkwood, 2007; Hoskins et al., 2017). The species is considered a generalist predator, foraging on > 60 taxa, including elasmobranchs, bony fish, and cephalopods (Deagle et al., 2009; Kernaléguen et al., 2016). Its broad-scale foraging behaviour and habitat-use, as well as the environmental factors influencing these characteristics, have been extensively investigated (e.g (Hoskins et al., 2015a, b; Speakman et al., 2020)). However, little is known of the hunting strategies the species employs or the factors influencing their energetic consequences (Foo et al., 2016).
The Bass Strait region is one of the world’s fastest-warming oceanic areas (Hobday and Pecl, 2014), with projected climate change impacts expected to lead to significant shifts in the diversity, abundance and distribution of species (Hobday and Lough, 2011). Such alterations are likely to affect the prey field of the region’s marine predators (Fulton, 2011). Knowledge of the hunting strategies they employ, and the factors influencing their success and efficiency, is crucial for understanding the behavioural, energetic and, ultimately, population trajectory responses of these species to the anticipated ecosystem modifications (Niella et al., 2022). The aims of the present study, therefore, were to determine the: 1) fine-scale dive behaviour movements; 2) prey capture and energy expenditure consequences of these movements; and 3) factors influencing hunting search strategies of AUFS.
2 Materials and methods
2.1 Study site and data collection
The study was conducted on Kanowna Island (39° 9.1’ S, 146° 18.5’ E) in northern Bass Strait, south-eastern Australia, during the winters of 2015-19. The island hosts the third largest breeding colony of AUFS, with an annual production of ca. 2500 pups (Geeson et al., 2022). Adult females suckling pups were selected at random and captured using a modified hoop net (Fuhrman Diversified, Seabrook, Texas, U.S.A.). Individuals were then anaesthetised with isoflurane delivered via a portable gas vaporiser (StringerTM, Advanced Anaesthesia Specialists, Gladesville, NSW, Australia) for the duration of handling procedures.
A combined video/depth/IMU (Inertial Measurement Unit) data logger (CD v6, Customized Animal Tracking Solutions, Moffat Beach, Australia) was glued to the fur along the dorsal midline just posterior to the scapula using quick-setting epoxy (RS Components, Corby, UK; Figure 1). The video data logger was programmed to record for 1 h at 10:00 and 14:00 (AEST) each day. The IMU recorded depth (m), video/depth/IMU data logger and ambient temperature (°C) and three axes of acceleration (m·s-2), magnetic field intensity (μT), and angular velocity (°·s-1) at 20 Hz. A GPS/dive behaviour data logger (Mk10F, Wildlife Computers, Redmond, E.A., U.S.A.) and a VHF transmitter (Sirtrack Ltd, Havelock North, NZ) were also attached in series posterior to the first device. The GPS/dive behaviour logger was programmed to record location and depth at 15 min and 5 s intervals, respectively. The VHF transmitter was used to relocate the individual at the colony to facilitate recapture. Together, the devices represented <2% body mass and <1% cross-sectional surface area and, therefore, are likely to have had a negligible impact on hydrodynamic drag (McMahon et al., 2008; Field et al., 2011).
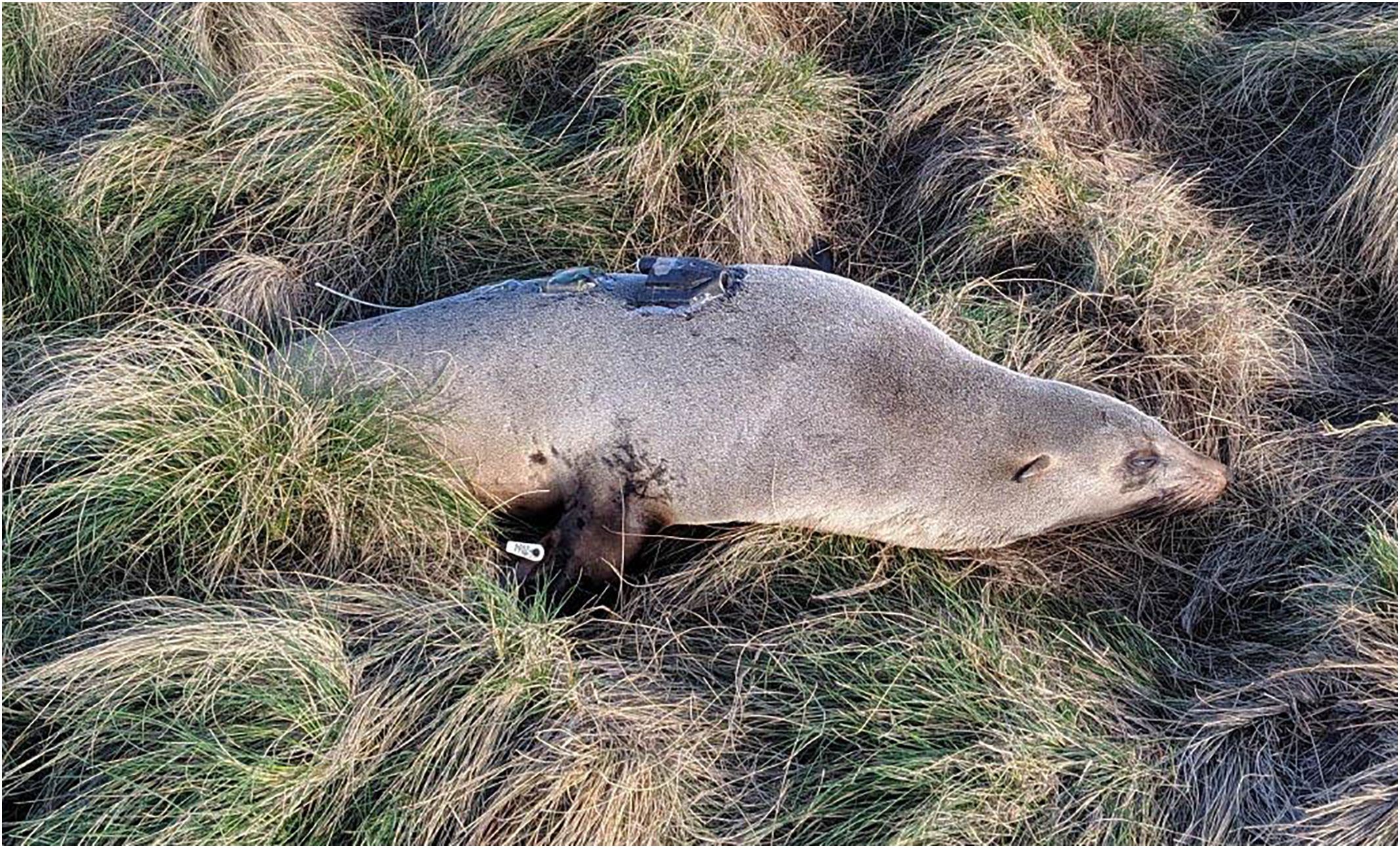
Figure 1 Adult female Australian fur seal (Arctocephalus pusillus doriferus) with (from right to left) a combined video/depth/IMU (accelerometer, magnetometer, gyroscope) datalogger, GPS/dive behaviour data logger, and VHF transmitter glued to the dorsal midline fur.
Following the attachment of data loggers, standard length and body mass data were determined using a tape measure (± 0.5 cm) and a digital suspension scale (± 0.1 kg), respectively. Uniquely numbered plastic tags (Super Tags, Dalton, Woolgoolga, Australia) were then inserted in the trailing edge of each fore-flipper for identification before the animal was left to recover from anaesthesia and resume normal behaviours. Individuals were recaptured at the colony after at least one complete foraging trip at sea following the above procedures and the data loggers removed. Data were downloaded onto portable computers in the field.
2.2 Dive behaviour, prey captures and index of energy expenditure
All dive behaviour data were determined from the depth measurements of the combined GPS/dive behaviour data logger. Due to the potential for pressure-transducer drift, depth data was first zero-offset corrected (ZOC) using the diveMove package (Luque, 2007) in R statistical environment version 4.0.3 (R Core Team, 2023). Assuming a minimum depth of 5 m for foraging dives, summary statistics were then extracted for each dive to determine dive depth (m) and durations (s) of the descent, bottom, ascent and post-dive phases. All dives which occurred below a 40 m threshold (Volpov et al., 2015) at ± 10% of the maximum depth of the preceding dive were considered benthic (Tremblay and Cherel, 2000). Since benthic dives were the focus of the present study, all non-benthic dives were excluded from further analyses. In addition, to exclude post-dive periods not associated with foraging (e.g. resting at the surface and/or commuting), all dives with a post-dive duration > 10 min (Speakman et al., 2020) were excluded from further analyses.
IMU signals were transformed from the tag reference frame to the seal reference frame following procedures described elsewhere (e.g (Johnson and Tyack, 2003; Johnson, 2011)). Peaks in the sway (Y; lateral) axis accelerometer data were used to estimate potential prey captures (PPC) using the Ethographer package (Sakamoto et al., 2009) in IgorPro (Version 6.34, Wavemetrics, USA) following the methods of Foo et al. (2016). Briefly, a 3 Hz high-pass filter was used to remove small movement noise (Viviant et al., 2010). The standard deviation (SD) of the filtered data over a 1.5 s moving window was then calculated for the whole record. Peaks over a pre-determined threshold were considered to reflect PPC (Foo et al., 2016).
To identify the optimal threshold for each individual, the frequency distribution of PPC determined with Y-acceleration SD ranging from 0.5 to 3.0 were visually inspected for discontinuity following the methods of Foo et al. (2016), the optimal threshold was the one before the discontinuity. To avoid double-counting multiple peaks from a single PPC event, a minimum interval of 3.5 s was applied between successive peaks (Volpov et al., 2015). Finally, as the minimum depth for all benthic dives recorded was 40 m, only peaks in Y-acceleration SD occurring at greater depths were considered to remove movement associated with prey handling during ascent or at the surface. The dimensions of the combined video/depth/IMU data logger precluded its attachment to the head. In addition, for the reconstruction of 3-dimensional tracks, the IMU sensor needs to be near the centre of gravity and, therefore data logger was placed on the dorsal midline just posterior to the scapula. While the majority of previous otariid seal studies using accelerometers to document PPC have involved data loggers placed on the head (Volpov et al., 2015; Foo et al., 2016), accelerometers mounted on the dorsal surface have been shown to detect PPC in northern fur seals (Callorhinus ursinus) and Antarctic fur seals (A. gazella) (Jeanniard‐Du‐Dot et al., 2017). In the present study, the PPC detection was validated by comparing accelerometer-derived data with the available video data.
To investigate the relationships between hunting strategies and foraging effort, Vectorial Dynamic Body Acceleration (VeDBA) was used as an index of energy expenditure. Previous studies have demonstrated VeDBA to be strongly correlated to energy expenditure in various taxa, including fur seals (Jeanniard‐Du‐Dot et al., 2017). VeDBA was calculated from the tri-axial accelerometer data, using the following equation:
where S is the static acceleration and A is the acceleration. A 2 s running mean of S (reflecting the positioning of the animal in space in relation to gravity) was subtracted from A to calculate dynamic acceleration related to movement (Jeanniard‐Du‐Dot et al., 2017). The mean VeDBA (g) was determined for the four different dive phases (descent, bottom, ascent and post-dive).
2.3 Reconstruction of 3-dimensional dive profiles
Preliminary analyses indicated that the magnetometer, gyroscope and accelerometer sensors in the video/depth/IMU data logger were strongly influenced by temperature and that the internal temperature of the device increased at a rate of 5°C·h-1 and 10°C·h-1 in water and air, respectively, when the video data logger was recording. To account for these biases in each sensor, two video/depth/IMU data loggers (representing the device models used in deployment data collection) were subjected to a range of in-air and in-water temperatures experienced by AUFS at sea both with, and without, the video data logger recording. A low-pass filter was applied to each axis and the filtered data was fitted to a simple linear regression with temperature. The resulting equation was then used to determine the unbiased sensor information in the deployment data according to the experienced temperature.
The position on the horizontal plane at sea surface was provided by GPS data, from which the position estimates of the start and end of the dives were extrapolated. Depth profiles were used to extrapolate the position on the vertical dimension. The position of individuals on the horizontal plane was estimated via dead-reckoning (Davis et al., 2001); i.e. by integrating the IMU signals on the seal reference frame, albeit disregarding the vertical position solution. Acceleration signals were low-pass filtered and scaled to match realistic pinniped velocities (Sato et al., 2003) and corresponding to the GPS surface track. Euler angles from the x, y, and z axes were calculated to assess roll, heading (yaw) and pitch, respectively.
Speed of descent, bottom, and ascent phases were calculated using the following formulae:
where (Xpos2 – Xpos1), (Ypos2 – Ypos1), (Zpos2 – Zpos1) are the differences in position between two consecutive points, D is the distance (m) travelled over consecutive points, TD is the total distance travelled (m), T is the time during the dive phase (s), and Sp is the speed (m·s-1). In addition, information on the longitude and latitude of the start of the descent phase and the end of the ascent phase was provided by the GPS data loggers. Through the raster (Hijmans et al., 2015) package, the distance travelled along the bottom phase, and between two dives at the surface, were calculated using the position of individuals on the horizontal plane during the dives and the coordinates of the descent and ascent phase, respectively.
To investigate the influence of sea-floor hunting activity on dive behaviour and energy expenditure, the number of substantial directional changes made by individuals was determined. Heading (yaw) values during the bottom phase of the dive were smoothed with a 3 s running mean, to remove small movements, and sampled every 3 s to document apparent changes in direction. The difference between consecutive values was determined to calculate the angular deflection of the movement and transformed into radians. The frequency distribution of directional changes was investigated in each individual to assess for discontinuities from which significant movements could be detected. Directional changes greater than this threshold were considered as intentional movements (Miller et al., 2019) related to hunting behaviour.
Mean heading during the descent phase and ascent phase were calculated. To exclude the potential influence of surface or bottom phase behaviour (e.g. prey handling) on heading during descent/ascent, only data > 5 m below the surface and > 5 m above the bottom phase were included. The difference in the mean heading during the descent phase between successive dives was then investigated to assess changes in travelling direction between subsequent dives.
2.4 Data analysis
All statistical analyses were conducted in the R statistical environment (Version 4.0.3 (R Core Team, 2023)). Linear mixed-effect models (LME) and Generalized mixed-effect models (GLME), within the nlme (Pinheiro et al., 2007) and lme4 (Bates et al., 2011) packages. To assess the factors influencing fine-scale movements, maximum depth, mean speed, mean pitch and duration of the different dive phases were used as predictor variables. Similarly, these variables were investigated as influences on energy expenditure and prey capture rates (Supplementary Table 1). In all models, the individual was considered a random factor to account for repetitive sampling. Correlation matrices were constructed to assess for collinearity between continuous factors. When correlation coefficients were r > 0.7, one of the two parameters was excluded from further analyses (Schober et al., 2018). Model comparison was conducted with AIC selection using the ‘dredge’ function in the MuMIn package (Barton and Barton, 2015). Where numerous candidate models with ΔAICc < 4 existed, model averaging was conducted and the 95% confidence intervals for the predictive variables inspected. Unless otherwise specified, data are presented as Mean ± SE and results considered significant at P < 0.05.
3 Results
3.1 Foraging trip metrics and fine-scale behaviours
Due to camera malfunction, animal-borne video data were available for only 3 individuals, for a duration of 4.5 h (2.7 ± 1.1 h) per individual during which benthic dives were made. Although available video data enabled the validation of prey captures from back-mounted accelerometers, it was insufficient to investigate relationships with seafloor habitat. Complete data sets of matching GPS, dive behaviour and IMU information were obtained from a total of 5 individuals with mean body mass of 70.5 ± 6.6 kg and standard length of 152 ± 3.8 cm (Table 1). Mean foraging trip duration was 4.6 ± 0.5 d, during which individuals travelled 234.9 ± 32.4 km within central Bass Strait. Throughout these foraging trips, individuals made 404.8 ± 57.3 benthic dives showing modes of maximum depths ranging from 75.0 - 82.9 m, with dive durations modes ranging from 2.89 - 4.56 min (Table 1).
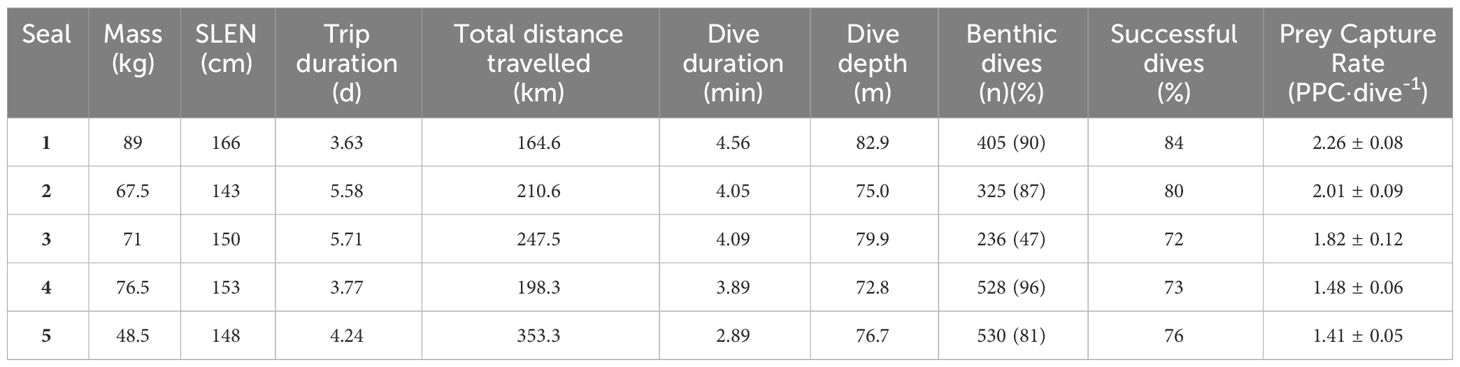
Table 1 Summary information on adult female Australian fur seals (Arctocephalus pusillus doriferus) from Kanowna Island instrumented with combined GPS/dive behaviour/IMU data loggers.
The reconstructed 3 dimensional dive profiles typically revealed individuals descending to the sea floor at a steep angle (high pitch), swimming rapidly along the sea floor in a mostly constant direction, then ascending to the surface at a shallower angle (lower pitch; Figure 2). However, there was substantial variation in dive parameters between dive phases, between dives and between individuals (Table 2). During descent, the mode of mean downward pitch (from the horizontal) of individuals ranged from 34 – 75.1° while the mode of mean descent speed ranged from 0.37 – 0.50 m·s-1, resulting in the mode of mean VeDBA ranging from 0.51 – 0.92 g (Table 2).
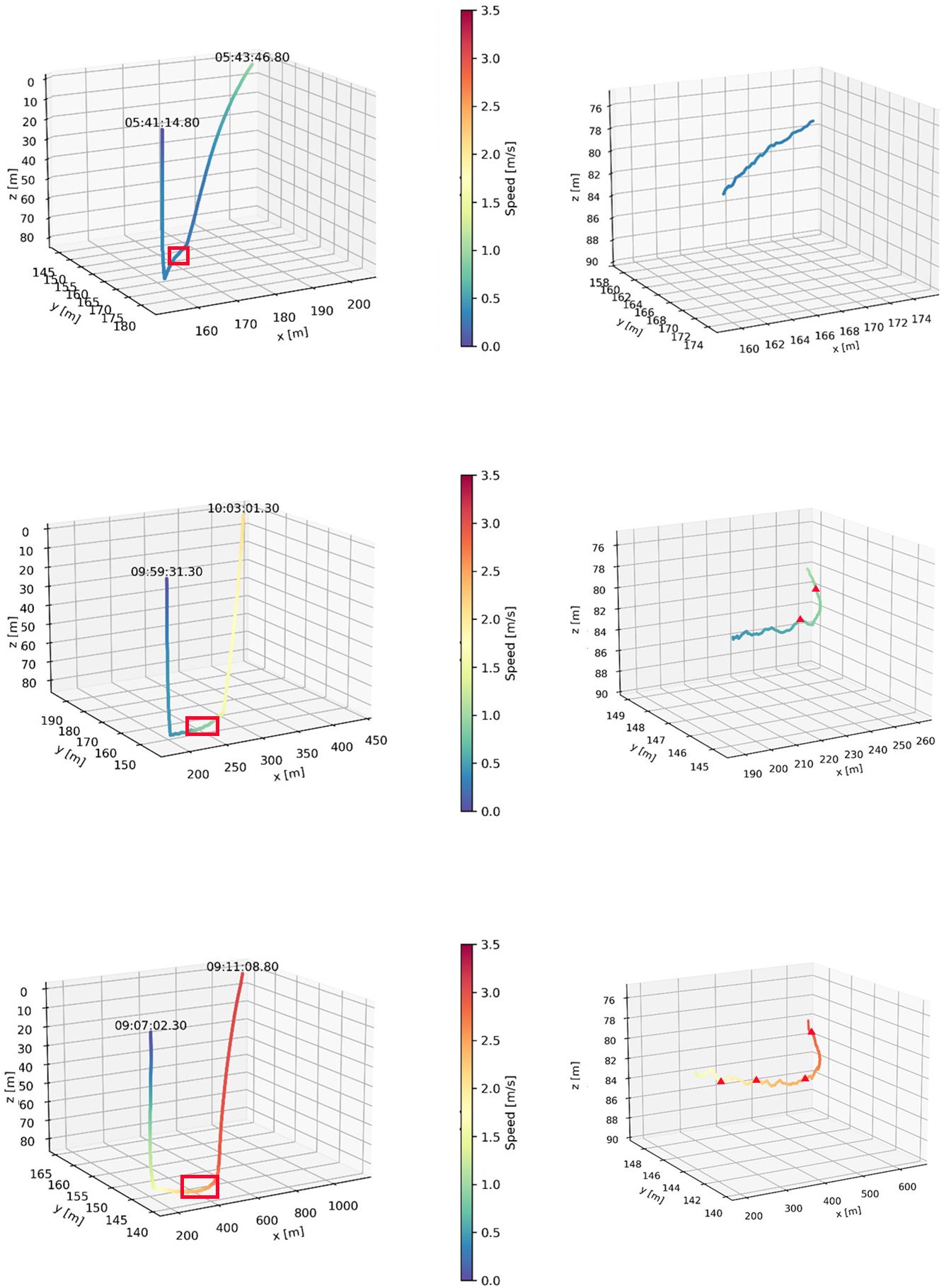
Figure 2 Representative 3-dimensional reconstructions of three foraging dives by adult female Australian fur seal (Arctocephalus pusillus doriferus) individuals from Kanowna Island in northern Bass Strait, south-eastern Australia. Swim speed along the track is indicated by the colour ramp. Right panels represent a zoomed in part of the track (indicated by red box in left panel). Red triangles denote recorded prey capturess events.

Table 2 Summary table showing modes of fine-scale behaviours for adult female Australian fur seal (Arctocephalus pusillus doriferus) individuals from Kanowna Island, in northern Bass Strait, south-eastern Australia, during the three dive phases: descent; bottom: and ascent.
During ascent, the mode of mean upward pitch (from the horizontal) of individuals ranged from 62.9 – 72.4° while the mode of mean ascent speed ranged from 0.35 – 0.53 m·s-1, resulting in the mode of mean VeDBA ranging from 0.59 – 1.13 g (Table 2). During the bottom phase of dives, where duration had a mode ranging from of 1.46 - 2.08 min, the distribution of directional changes suggested turns of > 45° indicated intentional movement changes. The number of such directional changes during bottom phases ranged from 0 - 45 with the mode for individuals ranging from 5 - 9. The mode of mean speed during the bottom phase ranged from 0.41 - 0.46 m·s-1, resulting in the mode of mean VeDBA ranging from 1.18 – 1.75 g (Table 2).
To investigate factors influencing potential foraging time within a dive (i.e. time along the sea floor), LMEs were constructed with the fine-scale diving behaviour variables. As time in the bottom phase of a dive has been shown to be influenced by dive depth (due to body oxygen store limitations (Thompson and Fedak, 1993), the bottom phase duration was converted to a proportion of total dive duration and arcsine-transformed before modelling. Since there were numerous candidate models with a ΔAICc < 4, model averaging was conducted and revealed all predictor variables to have a consistent influence (95% CI not crossing 0; Table 3). Descent pitch and maximum depth were included in all candidate models with positive influences, indicating they are the most important parameters in explaining bottom time (%) (Figures 3B, D). Mean speed during descent also positively influenced bottom phase (%) duration whereas mean speed during the bottom phase had a negative influence (Figures 3C, A).
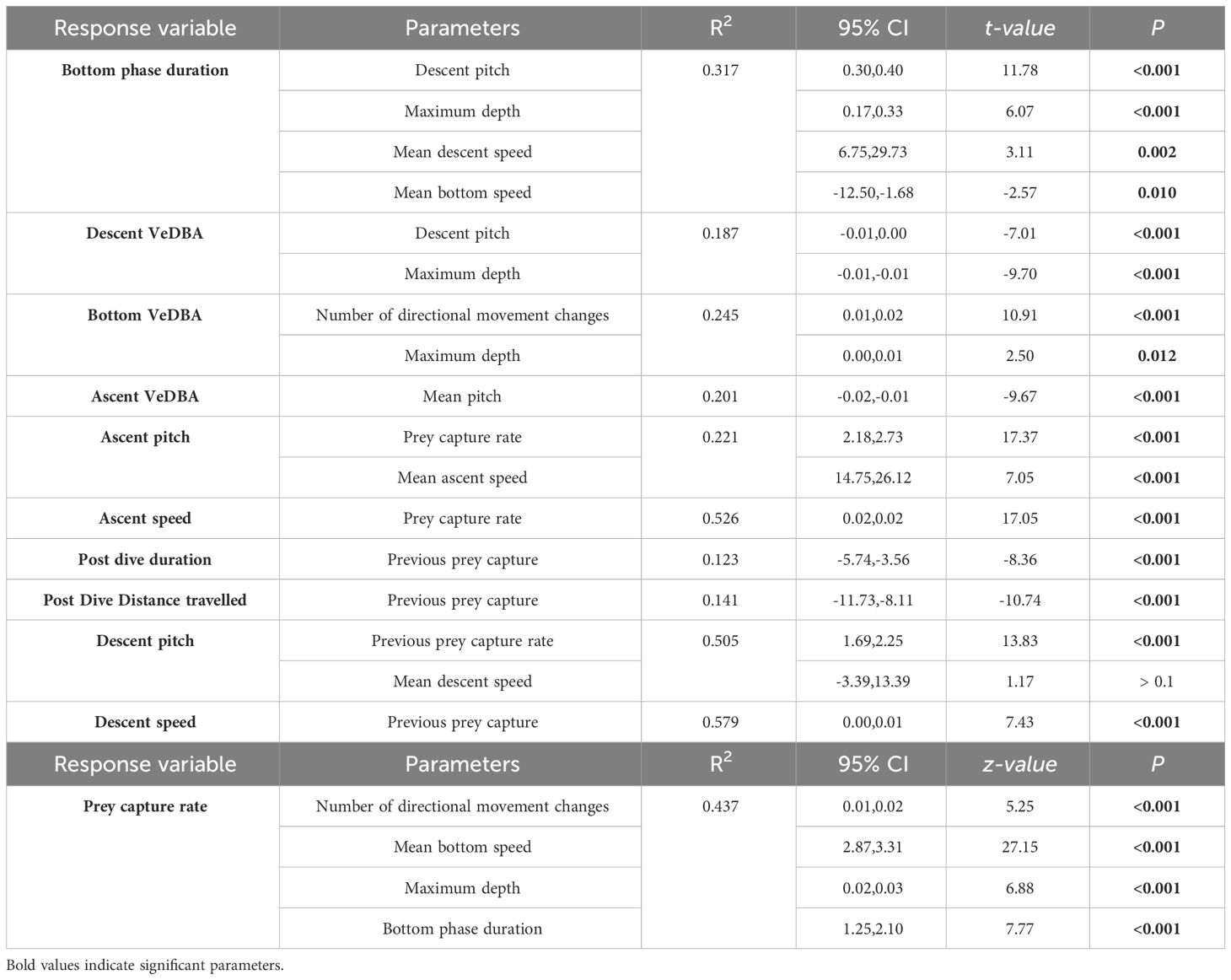
Table 3 Best models of Linear mixed-effect (LME) and Generalized mixed-effect (GLME) models predicting factors influencing inter-dive and intra-dive behaviour, and VeDBA in adult female Australian fur seals (Arctocephalus pusillus doriferus) from Kanowna Island.
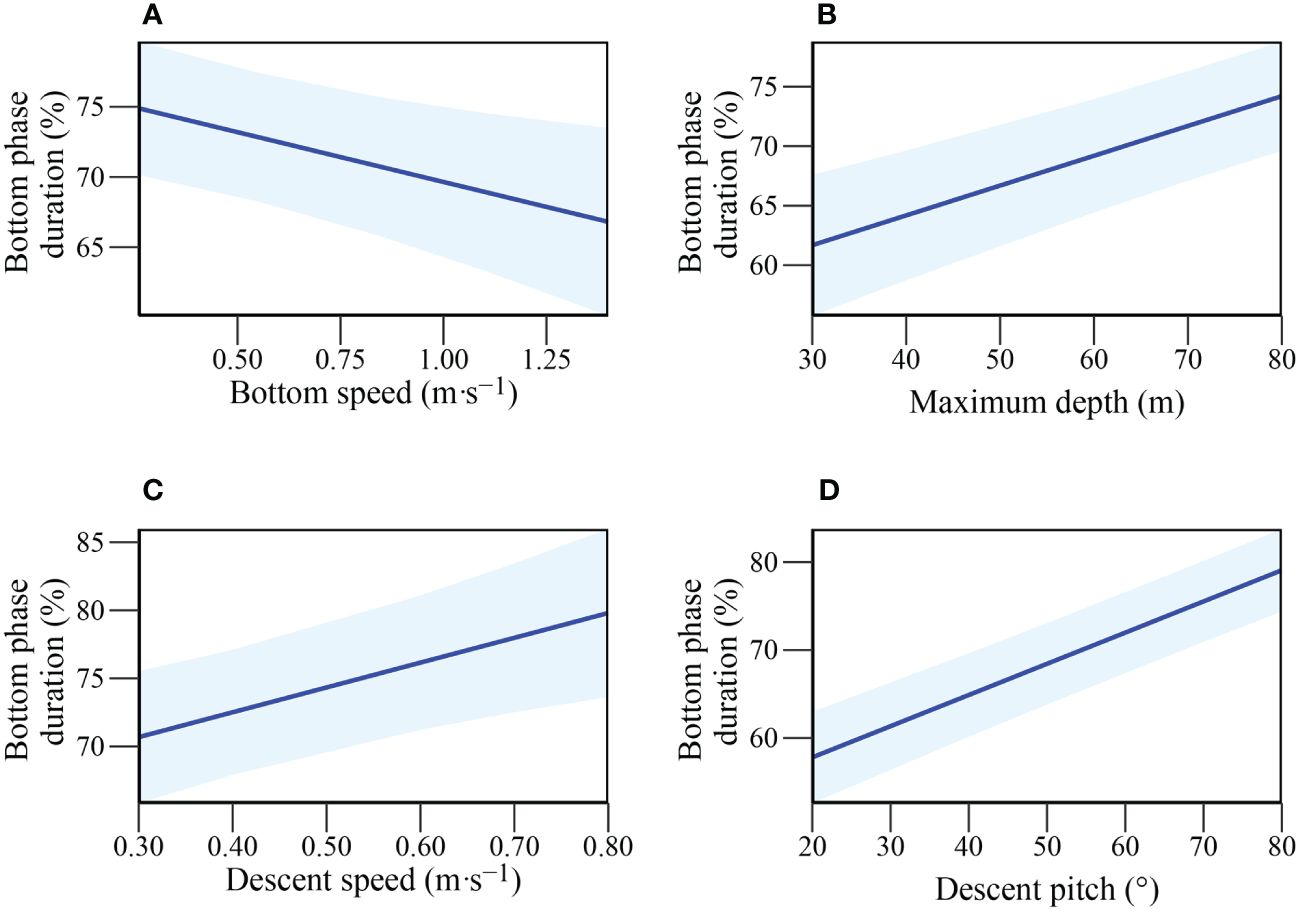
Figure 3 Relationship of Bottom phase duration (%) with: (A) Mean bottom speed (m·s-1); (B) Maximum depth (m); (C) Mean descent speed (m·s-1); (D) Descent pitch (°) in adult female Australian fur seals (Arctocephalus pusillus doriferus).
Factors influencing VeDBA during different dive phases were investigated with LMEs (Table 3). Individuals had a reduced VeDBA during the descent phase of dives with increasing (steeper) pitch and deeper maximum depths. Similarly, VeDBA was reduced during the ascent phase with increasing (steeper) pitch. During the bottom phase of dives, VeDBA was found to be positively influenced by the number of directional changes initiated and with greater depths (Table 3).
3.2 Factors influencing prey captures and inter-dive foraging strategies
A total of 150 benthic prey encounter events were recorded in the video data collection (50 ± 9 per individual). All were detected as PPC in the analysis of the back-mounted accelerometer data, while no false-positive events were recorded, validating the technique for quantifying potential prey captures. A total of 3524 PPC were recorded in 1559 dives (77% of the total benthic dives), with the number of PPC ranging from 0 - 10 per dive. Mean prey capture rate varied between individuals from 1.4 ± 0.1 PPC·dive-1 to 2.3 ± 0.1 PPC·dive-1 (Table 1).
Parameters influencing prey capture rate were investigated using GLME with the number of directional changes, mean bottom speed, proportion of bottom phase duration, and maximum depth as predictor variables. The full model was the most parsimonious (Table 3), showing that all measured variables positively influence prey capture rate (Figure 4).
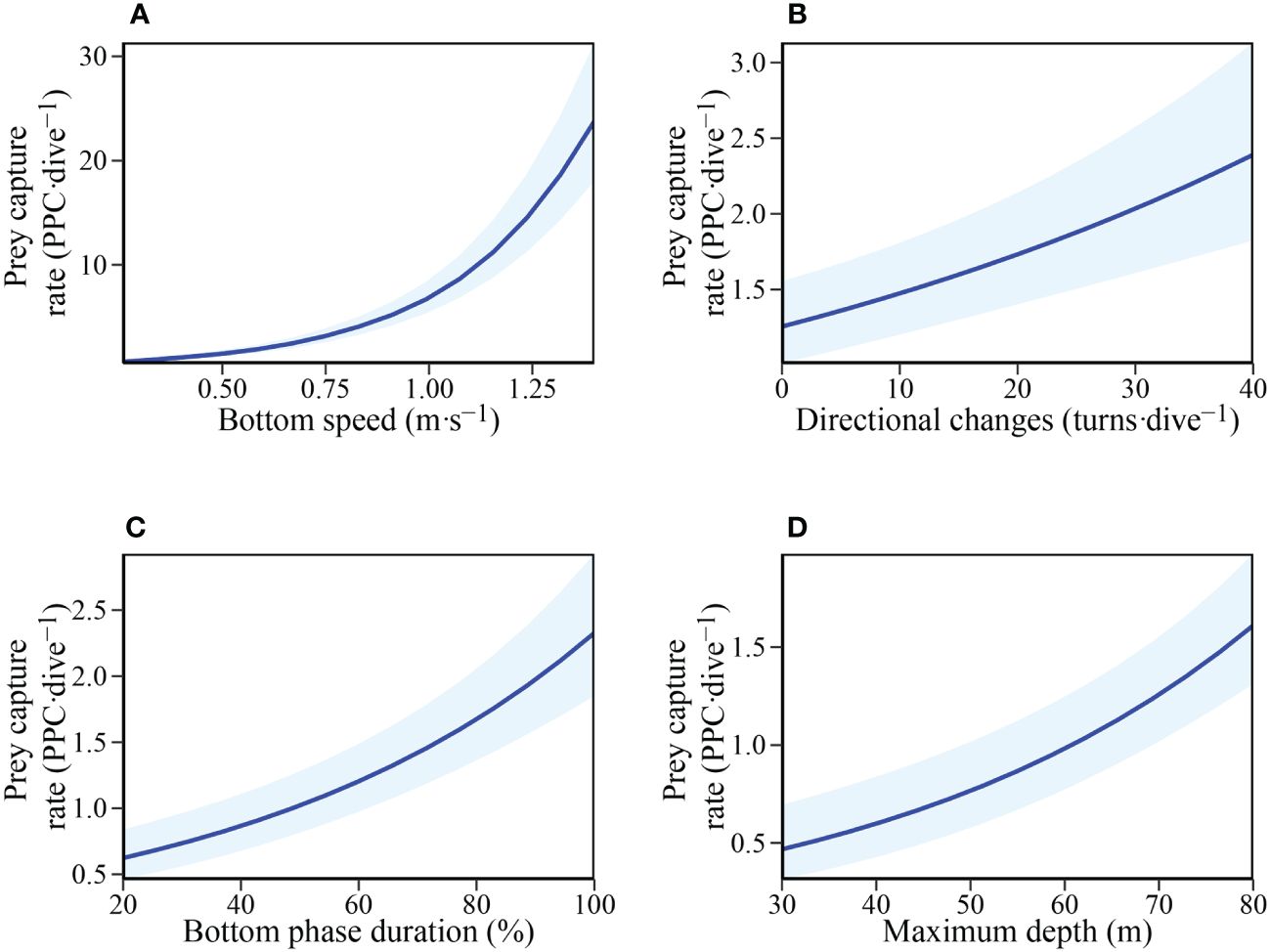
Figure 4 Relationship between Prey capture rate (Potential prey capture (PPC) ·dive-1) and: (A) Mean bottom phase speed (m·s-1); (B) Number of directional changes (turns·dive−1); (C) Bottom phase duration (%); and (D) Maximum depth (m) in adult female Australian fur seals (Arctocephalus pusillus doriferus).
The hunting success experienced by individuals during the bottom phase of dives was found to influence numerous aspects of subsequent fine-scale behaviours (Table 3). LME model selection revealed that individuals ascended to the surface at a steeper pitch and faster swim speed with increasing prey captures during the bottom phase (Figures 5A, B). In addition, post-dive duration and the distance travelled before the next dive were both negatively influenced by the number of prey captures during the bottom phase of the previous dive (Figures 5C, D). Furthermore, the descent pitch and speed during a dive were positively influenced by the number of prey captures in the preceding dive (Figures 5E, F).
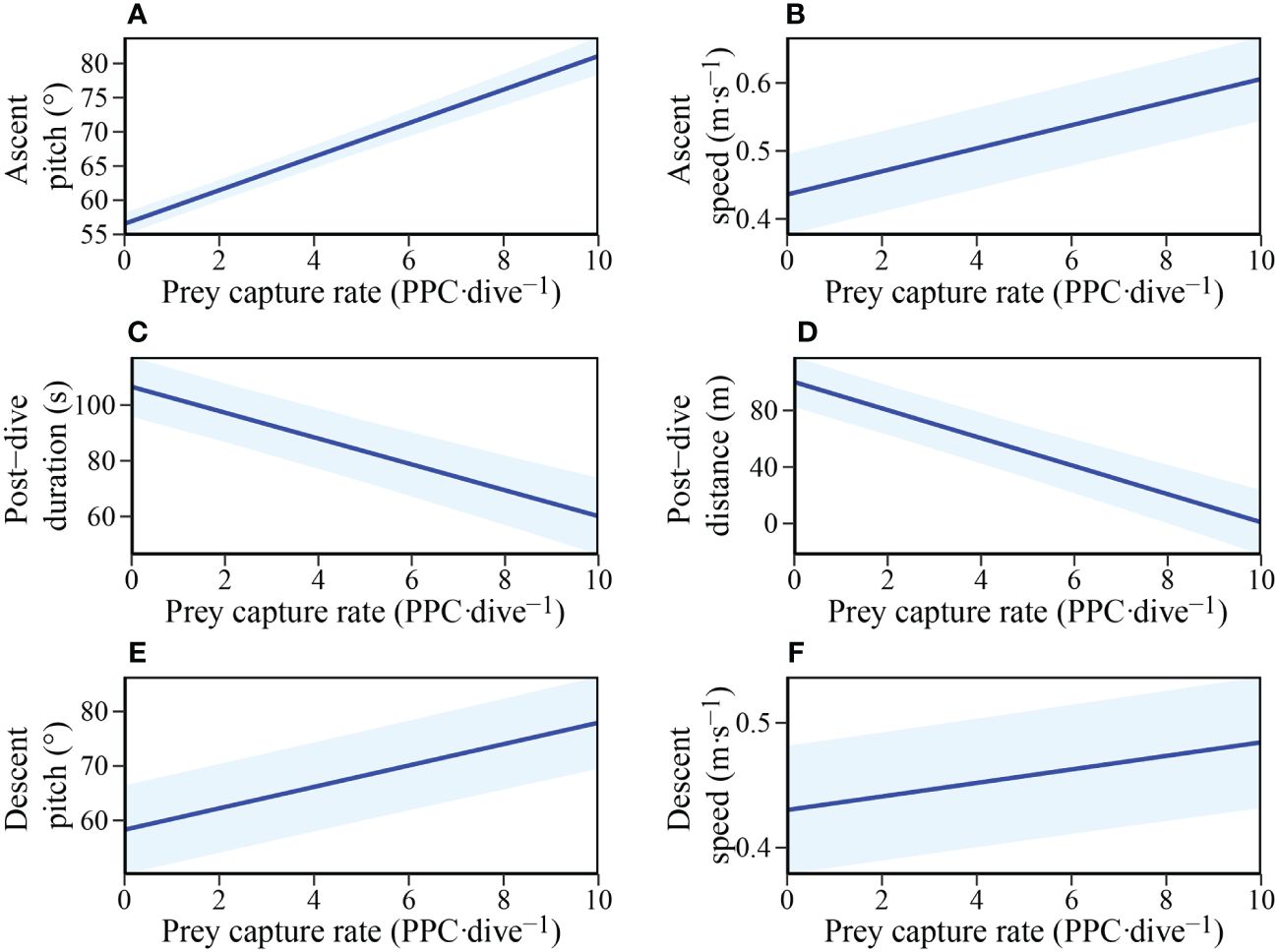
Figure 5 The influence of Prey capture rate (PPC·dive-1) within a dive on: (A) Ascent pitch (°); (B) Mean ascent speed (m·s-1); (C) Post-dive duration (s); (D) Post-dive surface travel distance (m); (E) Next dive descent pitch (°); and (F) Next dive mean descent speed (m·s-1) in adult female Australian fur seals (Arctocephalus pusillus doriferus).
Directional heading during the descent and ascent phases of dives were generally consistent. In addition, while individuals on some dives made numerous directional changes during the bottom phase, travel throughout dives was in a relatively constant direction. To investigate whether individuals altered travel direction between dives, potentially as part of a prey searching strategy, the difference between the mean heading of descent during sequential dives was analysed. The change in heading varied considerably throughout trips and between individuals (mode: 35.0°, range: 0.02 - 179°). There was no significant influence of prey capture rate on change of direction (LME: t = -1.421; P > 0.1), indicating individuals did not alter their spatial search pattern in response to the success experienced in the preceding dive.
4 Discussion
Animals must adjust their behaviour in response to their prey field in order to optimise foraging success (Dylda and Wang, 2022). For benthic foraging air-breathing vertebrates, there is a need to maximise their time along the sea floor. The results of the present study suggest adult female AUFS optimise search time along the sea floor during benthic dives by travelling at high speeds to increase prey encounters. In addition, they alter pitch and swim speed during ascent and then subsequent descent phases in response to the success on the sea floor. Furthermore, the findings suggest AUFS do not adopt conventional Area Restricted Search (ARS) behaviour (i.e. increased track tortuosity) in response to encountering successful foraging areas. Rather, in response to successful dives, individuals reduce the time away from the sea floor, reduce the horizontal distance travelled at the surface, and maintain heading, potentially to maximise searching in similarly profitable but undisturbed habitats of cryptic benthic prey. However, the present study was limited to 5 individuals and, therefore, the interpretation of the results should be viewed within this constraint.
4.1 Within-dive behaviour to optimise benthic foraging success
Since the foraging zone for benthic foragers is on the sea floor, transit periods to/from the sea floor are non-profitable areas and should be minimised in favour of longer bottom durations (Wilson and Wilson, 1988). A smaller proportion of the dive duration spent at the bottom might be expected in deep dives due to the greater travel distance consuming more energy/oxygen. However, consistent with previous findings in penguins (Zimmer et al., 2010) and other pinniped species (Schreer et al., 2001), the proportion of the dive duration spent on the sea floor (foraging zone) was positively influenced by dive depth in the present study. In addition, mean speed and pitch during descent was positively correlated with the proportion of the dive’s bottom phase, indicating individuals prioritised rapid transit to the sea floor in order to maximise time in the foraging zone. Similar findings have been observed in rockhopper penguins (Eudyptes chrysocome), where greater descent rates and lower descent times were observed during benthic dives than pelagic ones, leading to greater bottom phase durations (Tremblay and Cherel, 2000).
The proportion of the dive duration spent on the sea floor by female AUFS was negatively influenced by swim speed in this dive phase. This is consistent with increased swim speeds being associated with higher metabolic costs (Feldkamp, 1987) and, thus, a greater use of available body oxygen stores (Hvas et al., 2017). However, the results of the present study suggest an apparent benefit of greater swimming speeds along the sea floor is increased prey encounter rates. Indeed, as AUFS prey mostly on cryptic benthic prey (e.g. numerous species of octopus (Octopus), gurnards (Triglidae) and leatherjackets (Monacanthidae)) (Kernaléguen et al., 2016), it may be advantageous to travel at high speeds to detect and capture unsuspecting prey before they disperse/hide (Zhang and Richardson, 2007). This is in contrast to harbour seals (Phoca vitulina) which were observed to swim at slower speeds when hunting cryptic prey in comparison to when hunting conspicuous species (Bowen et al., 2002).
Interestingly, in the present study, prey capture rate on benthic dives was found to increase with increasing sea floor depth. Foraging by female AUFS from Kanowna Island is almost exclusively restricted to central Bass Strait (Arnould and Kirkwood, 2007; Kirkwood and Arnould, 2012) where maximum depths range 60-80 m (Baird and Ridgway, 2012). Previous studies have shown an increase in benthic species diversity with depth in Bass Strait (Coleman et al., 1997). Consequently, foraging at these greater depths may provide AUFS greater prey diversity and, thus, better foraging opportunities.
The present study also found prey capture rate was positively related to the number of directional changes along the sea floor. While this could reflect an increase in prey encounters due to changes in search direction (Bell, 2012), the directional changes may reflect intentional movements towards detected prey (Bianco et al., 2011). Indeed, previous studies of AUFS instrumented with animal-borne video cameras have revealed individuals often make sharp turns upon detecting prey with a high degree of capture success (73%) (Meyers et al., 2021). However, without additional video data, it is not possible to disentangle the observed relationship between prey capture rate and directional changes during sea floor searches by AUFS. Nonetheless, such angular directional changes are likely to incur energetic costs and are likely undertaken only when foraging outcomes are more favourable than proceeding in a straight line (Wilson et al., 2013). This is supported by the observed greater mean VeDBA during the bottom phase of dives when more directional changes were made.
Individuals in the present study were found to have lower VeDBA when descending or ascending with greater (steeper) pitches. These findings may reflect the influence of buoyancy on mechanical power and the cost of transportation (Williams et al., 2000; Wilson et al., 2010). When buoyancy forces are taken into consideration, locomotion costs are lower when maintaining a vertical dive pitch (Thompson et al., 1993). For example, northern elephant seals (Mirounga angustirostris) had a higher cost of transport when a non-vertical pitch was adopted while diving compared to a vertical one (Miller et al., 2012). Hence, the most optimal technique to reach the seafloor foraging zone faster and with less energy or the sea surface for oxygen replenishment is to have higher (steeper) pitches.
4.2 Inter-dive foraging behaviour strategies
Optimal diving models propose individuals should maximise the bottom phase of dives to enhance prey encounter opportunities (Boyd et al., 1997). Indeed, the results of the present study suggest benthic diving AUFS maximise search time on the sea floor by minimising oxygen consumption (i.e. energy expenditure) during the descent and ascent phases. However, knowing where to dive for encountering productive habitats leads to optimal foraging (Humphries et al., 2010). Air-breathing pelagic marine predators foraging in the water column have been shown to employ ARS at the surface to optimise foraging success (Fauchald, 2009; Weimerskirch et al., 2009). Since pelagic prey species form transitory and unpredictable aggregations (Weber et al., 2021), a strategy of increasing sinuosity, decreasing speed, and focusing search in small areas has been shown to lead to increases in energy intake in pelagic predators (Corbeau et al., 2019).
In contrast, relatively little is known of the search patterns of benthic foragers (Blakeway et al., 2021). Unlike pelagic foragers, benthic divers do not appear to have clearly defined bouts of diving related to patch quality (Shoji et al., 2015). In addition, previous studies suggest benthic foragers spend a large portion of their time at sea diving (Costa et al., 2004) while in a constant travel direction (i.e. commuting along the sea floor) (Ramasco et al., 2015). While this could be a mechanism for avoiding predation at the surface (Brown et al., 2010), it may also be a strategy for optimising search time (Mattern et al., 2007).
Australian fur seals primarily feed on benthic prey, as their large body size makes chasing small, highly agile pelagic species inefficient when in low abundance (Arnould and Hindell, 2001; Arnould and Costa, 2006; Hoskins and Arnould, 2014). Correspondingly, individuals should maximise search time along the seafloor. In the present study, following prey captures during the bottom phase of a dive, individuals increased both the pitch and the speed of ascent (Figure 5). This strategy could potentially be undertaken to minimise the transit time to the surface for oxygen replenishment once a profitable foraging habitat has been found. The increase in ascent pitch would also result in less horizontal distance being travelled from the productive habitat. In addition, the horizontal distance individuals travelled at the surface decreased with increasing prey capture rate on previous dives. Furthermore, a decrease in post-dive duration after successful dives was also recorded, suggesting individuals may remain at the surface only to replenish body oxygen stores before returning to profitable seafloor habitats as rapidly as possible (Cook et al., 2008). Lastly, prey capture rate of the previous dive elicited an increase descent pitch, again potentially minimising the horizontal distance travelled from the previously exploited productive habitat, and descent swim speed, minimising transit time to the seafloor. Together, these findings suggest that central place foraging adult female AUFS individuals provisioning pups on land adopt fine-scale movement strategies at sea to maximise hunting opportunities in profitable seafloor habitats (Foo et al., 2016). Furthermore, the results of the present study suggest that benthic foraging adult female AUFS do not display conventional ARS behaviour. This is consistent with a previous study of the species which highlighted that areas of apparent ARS did not coincide with diving activity (Hoskins et al., 2015b). Indeed, the directional change for subsequent dives was not related to prey capture rate, suggesting that individuals do not focus searching activity in already exploited successful areas and, instead, move to new areas. This is in contrast to what has been observed in numerous pelagic marine predators where individuals adopt high sinuosity in search patterns once productive foraging areas are encountered (Freitas et al., 2018). One potential reason for the strategy observed in AUFS could be the prey type encountered by benthic foragers. Prey density is known to be the key factor for the adjustment of predator foraging behaviour (Mori et al., 2007). For example, the number of feeding lunges during dives by blue whales (Balaenoptera musculus) was proportional to krill density (Hazen et al., 2015). In the presence of predators, cryptic prey reduce movements and activity (Stein and Magnuson, 1976; Dill and Fraser, 1984) and/or move to and hide in protective habitats (Werner et al., 1983; Power et al., 1985). Once a predator has been detected, cryptic prey may hide for a very long time (Edmunds, 1974). In addition, prey have been found to reduce and randomize their movements to improve survivorship (Mitchell and Lima, 2002). As a counter-move, predators have been shown to delay their follow-up attacks (Mitchell, 2009) and expand their hunting activity over several areas (Brown et al., 1999; Lima, 2002). For example, sharp-shinned hawks (Accipiter striatus) have been found to use their foraging area unpredictably on a spatial and temporal degree, avoiding prey hotspots where prey vigilance is higher (Roth and Lima, 2007). Hence, for AUFS hunting cryptic benthic prey such as octopus, gurnards and leatherjackets (Kernaléguen et al., 2016), the best option may be to search in similar, nearby habitats rather than going over the same area again. Indeed, while successful dives did not elicit a change in heading, the horizontal distance travelled was reduced, potentially increasing the probability of covering similar productive but undisturbed habitats. In contrast, after unsuccessful dives, individuals had shallower pitch in ascent, travelled further at the surface and descended on the next dive at a shallower pitch, increasing the distance from an unproductive foraging zone to potentially increase the probability of encountering prey in a different habitat (Figure 6). A similar strategy of consistent heading in successful foraging routes has been suggested for benthic-foraging yellow-eyed penguins (Mattern et al., 2007). Studies of fine-scale hunting movement in other benthic-foraging marine predators are needed to determine whether this is a wide-spread strategy. In summary, the present study revealed adult female AUFS individuals adjust their fine-scale movements in relation to their prey field. The proportion of dive time spent in the bottom phase (foraging zone) was increased by travelling to and from the surface at steeper pitches and faster swim speeds following successful dives. An increase in the number of directional changes along the seafloor resulted in greater energetic costs during the bottom phase but this was compensated by an increase in prey capture rate. In addition, individuals did not adopt conventionally-described ARS, as successful dives were not associated with increased path tortuosity. Rather, individuals appear to adopt a strategy of hunting in similar but undisturbed seafloor habitats by maintaining a relatively constant heading and reducing the horizontal distance travelled after successful dives. These findings reveal important new insights into how air-breathing marine benthic predators exploit their foraging habits and provide a basis for future understanding of how animals could respond to environmental changes.
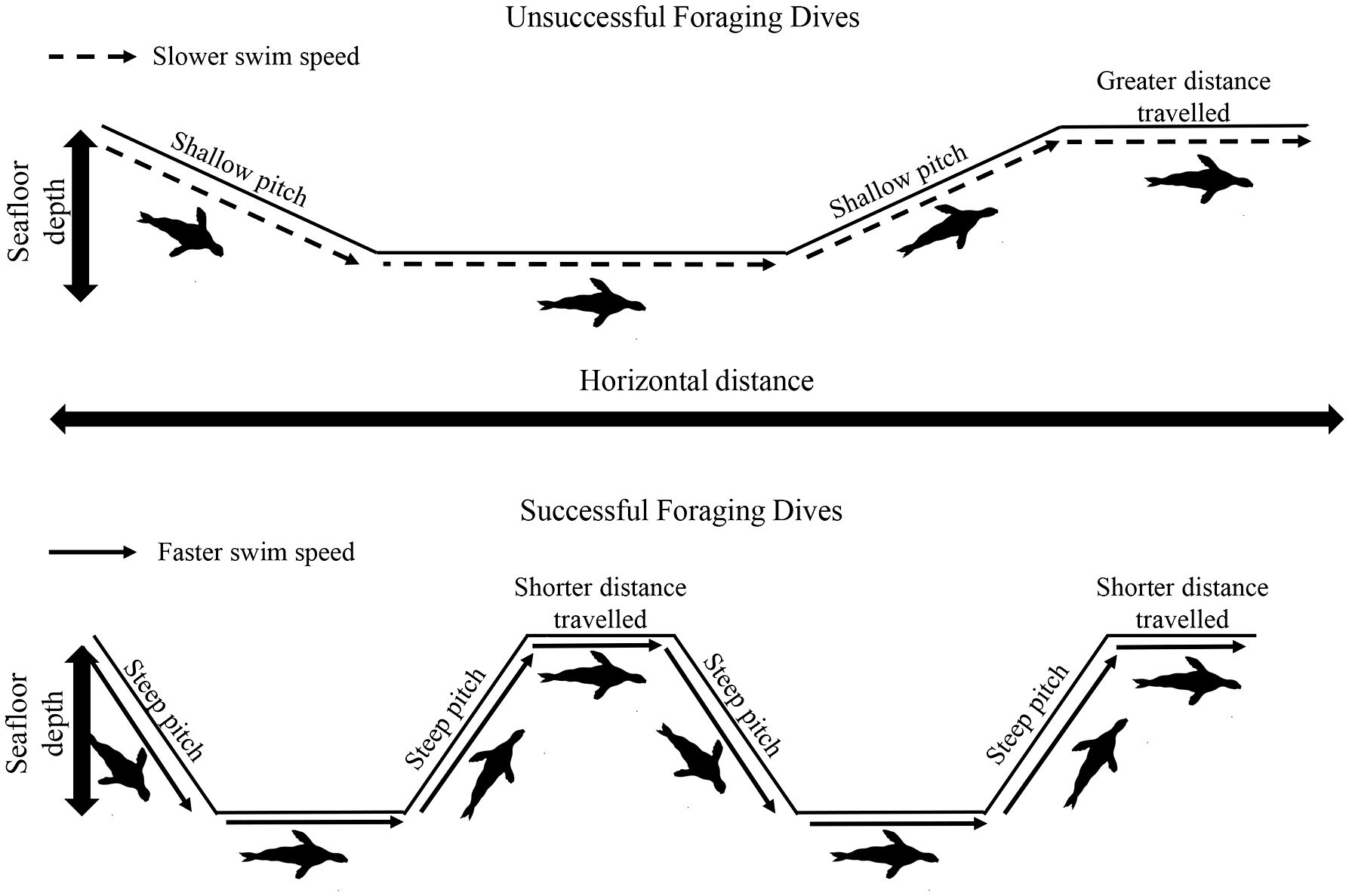
Figure 6 Graphical representation of diving behaviour in adult female Australian fur seals (Arctocephalus pusillus doriferus) associated with unsuccessful and successful foraging dives.
Data availability statement
The datasets presented in this study can be found in online repositories. The names of the repository/repositories and accession number(s) can be found below:Zenodo repository [10.5281/zenodo.10467539] https://zenodo.org/records/10467540?token=eyJhbGciOiJIUzUxMiJ9.eyJpZCI6IjAwODE2NTYyLTM5ZTItNGQ0Ni05NWE3LTY5NWNmMTBmYThlNSIsImRhdGEiOnt9LCJyYW5kb20iOiIzMmM5ZTljNmFlMTFjNWFiZjkxOTE4NTgxYzUzZDEwZiJ9.vsulFZILi0cLE56suJG06oh-d4mcQpermvojoGRFTsJxtPvKrYZVJr7yVlec0NAFTkooAz8OOOIEWHvieMH4jw.
Ethics statement
All research procedures were approved by the Deakin University Animal Ethics Committee (A16-2008, A14-2011, B16-2014) and conducted under a Department of Land, Water Environment and Planning (Victoria, Australia) Wildlife Research Permits (10005484, 10007153, 1000826).
Author contributions
PS: Data curation, Formal analysis, Methodology, Writing – original draft, Writing – review & editing, Conceptualization, Investigation. SL: Data curation, Formal analysis, Writing – original draft, Writing – review & editing, Conceptualization, Methodology. JA: Conceptualization, Data curation, Funding acquisition, Methodology, Project administration, Resources, Supervision, Writing – original draft, Writing – review & editing, Investigation.
Funding
The author(s) declare financial support was received for the research, authorship, and/or publication of this article. The study was supported by Deakin University internal research fund.
Acknowledgments
We thank Parks Victoria and Best Helicopters (in particular Dan Rogers and Sean Best, respectively) for their logistical support and the assistance of the many field workers over the study period is gratefully acknowledged.
Conflict of interest
The authors declare that the research was conducted in the absence of any commercial or financial relationships that could be construed as a potential conflict of interest.
Publisher’s note
All claims expressed in this article are solely those of the authors and do not necessarily represent those of their affiliated organizations, or those of the publisher, the editors and the reviewers. Any product that may be evaluated in this article, or claim that may be made by its manufacturer, is not guaranteed or endorsed by the publisher.
Supplementary material
The Supplementary Material for this article can be found online at: https://www.frontiersin.org/articles/10.3389/fmars.2024.1368756/full#supplementary-material
References
Andrews R. D., Enstipp M. R. (2016). Diving physiology of seabirds and marine mammals: relevance, challenges and some solutions for field studies. Comp. Biochem. Physiol. Part A: Mol. Integr. Physiol. 202, 38–52. doi: 10.1016/j.cbpa.2016.07.004
Arnould J. P. Y., Costa D. (2006). “Sea lions in drag, fur seals incognito: insights from the otariid deviants,” in Sea Lions of the World (Alaska Sea Grant College Program, Fairbanks), 309–323. doi: 10.4027/slw.2006
Arnould J. P. Y., Hindell M. A. (2001). Dive behaviour, foraging locations, and maternal-attendance patterns of Australian fur seals (Arctocephalus pusillus doriferus). Can. J. Zool. 79, 35–48. doi: 10.1139/z00-178
Arnould J. P. Y., Kirkwood R. (2007). Habitat selection by female Australian fur seals (Arctocephalus pusillus doriferus). Aquat. Conserv.: Mar. Freshw. Ecosys. 17, S53–S67. doi: 10.1002/(ISSN)1099-0755
Baird M. E., Ridgway K. R. (2012). The southward transport of sub-mesoscale lenses of Bass Strait Water in the centre of anti-cyclonic mesoscale eddies. Geophys. Res. Lett. 39, L02603. doi: 10.1029/2011GL050643
Barton K., Barton M. K. (2015). Package ‘mumin’. Version 1, 439. Available at: https://CRAN.R-project.org/package=MuMIn.
Bates D., Maechler M., Bolker B., Walker S., Christensen R. H. B., Singmann H., et al. (2011). “Package ‘lme4’,” in Linear mixed-effects models using S4 classes. R package version (Innsbruck, Austria: Journal of Statistical Software), vol. 1.
Bell W. J. (2012). Searching behaviour: the behavioural ecology of finding resources (London: Springer Science & Business Media).
Bianco I. H., Kampff A. R., Engert F. (2011). Prey capture behavior evoked by simple visual stimuli in larval zebrafish. Front. Syst. Neurosci. 5. doi: 10.3389/fnsys.2011.00101
Blakeway J. A., Arnould J. P. Y., Hoskins A. J., Martin-Cabrera P., Sutton G. J., Huckstadt L. A., et al. (2021). Influence of hunting strategy on foraging efficiency in Galapagos sea lions. PeerJ 9, e11206. doi: 10.7717/peerj.11206
Bowen W. D., Tully D., Boness D. J., Bulheier B. M., Marshall G. J. (2002). Prey-dependent foraging tactics and prey profitability in a marine mammal. Mar. Ecol. Prog. Series. 244, 235–245. doi: 10.3354/meps244235
Boyd I., Mccafferty D., Walker T. (1997). Variation in foraging effort by lactating Antarctic fur seals: response to simulated increased foraging costs. Behav. Ecol. Sociobiol. 40, 135–144. doi: 10.1007/s002650050326
Brown J. S., Laundré J. W., Gurung M. (1999). The ecology of fear: optimal foraging, game theory, and trophic interactions. J. mammal. 80, 385–399. doi: 10.2307/1383287
Brown A. C., Lee D. E., Bradley R. W., Anderson S. (2010). Dynamics of white shark predation on pinnipeds in California: effects of prey abundance. Copeia 2, 232–238. doi: 10.1643/CE-08-012
Chimienti M., Cornulier T., Owen E., Bolton M., Davies I. M., Travis J. M. J., et al. (2017). Taking movement data to new depths: Inferring prey availability and patch profitability from seabird foraging behavior. Ecol. Evol. 7, 10252–10265. doi: 10.1002/ece3.3551
Coleman N., Gason A. S. H., Poore G. C. B. (1997). High species richness in the shallow marine waters of south-east Australia. Mar. Ecol. Prog. Series. 154, 17–26. doi: 10.3354/meps154017
Cook T. R., Lescroël A., Tremblay Y., Bost C.-A. (2008). To breathe or not to breathe? Optimal breathing, aerobic dive limit and oxygen stores in deep-diving blue-eyed shags. Anim. Behav. 76, 565–576. doi: 10.1016/j.anbehav.2008.02.010
Corbeau A., Collet J., Fontenille M., Weimerskirch H. (2019). How do seabirds modify their search behaviour when encountering fishing boats? PloS One 14, e0222615. doi: 10.1371/journal.pone.0222615
Costa D. P. (1991). Reproductive and foraging energetics of high latitude penguins, albatrosses and pinnipeds: implications for life history patterns. American Zoologist, 31 (1), 111–130. doi: 10.1093/icb/31.1.111
Costa D. P., Kuhn C. E., Weise M. J., Shaffer S. A., Arnould J. P. Y. (2004). “When does physiology limit the foraging behaviour of freely diving mammals?,” in International congress series, vol. 1275. (Elsevier), 359–366. doi: 10.1016/j.ics.2004.08.058
Davis R. W., Fuiman L. A., Williams T. M., Le Boeuf B. J. (2001). Three-dimensional movements and swimming activity of a northern elephant seal. Comp. Biochem. Physiol. Part A: Mol. Integr. Physiol. 129, 759–770. doi: 10.1016/S1095-6433(01)00345-2
Deagle B. E., Kirkwood R., Jarman S. N. (2009). Analysis of Australian fur seal diet by pyrosequencing prey DNA in faeces. Mol. ecol. 18, 2022–2038. doi: 10.1111/j.1365-294X.2009.04158.x
Dill L. M., Fraser A. H. (1984). Risk of predation and the feeding behavior of juvenile coho salmon (Oncorhynchus kisutch). Behav. Ecol. Sociobiol. 16, 65–71. doi: 10.1007/BF00293105
Doniol-Valcroze T., Lesage V., Giard J., Michaud R. (2011). Optimal foraging theory predicts diving and feeding strategies of the largest marine predator. Behav. Ecol. 22, 880–888. doi: 10.1093/beheco/arr038
Dylda E., Wang K. H. (2022). Prior actions influence cost-benefit related decision-making during mouse foraging behaviors. Eur. J. Neurosci. 56, 3861–3874. doi: 10.1111/ejn.15689
Edmunds M. (1974). Defence in animals: a survey of anti-predator defences (New York: Longman Publishing Group).
Fandry C. B. (1981). Development of a numerical model of tidal and wind-driven circulation in Bass Strait. Mar. Freshw. Res. 32, 9–29. doi: 10.1071/MF9810009
Fauchald P. (2009). Spatial interaction between seabirds and prey: review and synthesis. Mar. Ecol. Prog. Series. 391, 139–151. doi: 10.3354/meps07818
Fauchald P., Tveraa T. (2003). Using first-passage time in the analysis of area-restricted search and habitat selection. Ecology 84, 282–288. doi: 10.1890/0012-9658(2003)084[0282:UFPTIT]2.0.CO;2
Feldkamp S. D. (1987). Swimming in the California sea lion: morphometrics, drag and energetics. J. Exp. Biol. 131, 117–135. doi: 10.1242/jeb.131.1.117
Field I., Harcourt R., Boehme L., Nico De Bruyn P. J., Charrassin J. B., Mcmahon C., et al. (2011). Refining instrument attachment on phocid seals. Mar. Mammal. Sci. 28, E325–E332. doi: 10.1111/j.1748-7692.2011.00519.x
Foo D., Semmens J. M., Arnould J. P. Y., Dorville N., Hoskins A. J., Abernathy K., et al. (2016). Testing optimal foraging theory models on benthic divers. Anim. Behav. 112, 127–138. doi: 10.1016/j.anbehav.2015.11.028
Freitas C., Caldeira R., Reis J., Dellinger T. (2018). Foraging behavior of juvenile loggerhead sea turtles in the open ocean: from Lévy exploration to area-restricted search. Mar. Ecol. Prog. Series. 595, 203–215. doi: 10.3354/meps12581
Fulton E. A. (2011). Interesting times: winners, losers, and system shifts under climate change around Australia. ICES J. Mar. Sci. 68, 1329–1342. doi: 10.1093/icesjms/fsr032
Geeson J. J., Hobday A. J., Speakman C. N., Arnould J. P. Y. (2022). Environmental influences on breeding biology and pup production in Australian fur seals. R. Soc. Open sci. 9, 211399. doi: 10.1098/rsos.211399
Harcourt R. G., Bradshaw C. J. A., Dickson K., Davis L. S. (2002). Foraging ecology of a generalist predator, the female New Zealand fur seal. Mar. Ecol. Prog. Series. 227, 11–24. doi: 10.3354/meps227011
Hazen E. L., Friedlaender A. S., Goldbogen J. A. (2015). Blue whales (Balaenoptera musculus) optimize foraging efficiency by balancing oxygen use and energy gain as a function of prey density. Sci. Adv. 1, e1500469. doi: 10.1126/sciadv.1500469
Hijmans R. J., Van Etten J., Cheng J., Mattiuzzi M., Sumner M., Greenberg J. A., et al. (2015). Package ‘raster’. R package. (Innsbruck, Austria: Journal of Statistical Software) 734. Available at: https://CRAN.R-project.org/package=raster.
Hobday A. J., Lough J. M. (2011). Projected climate change in Australian marine and freshwater environments. Mar. Freshw. Res. 62, 1000–1014. doi: 10.1071/MF10302
Hobday A. J., Pecl G. T. (2014). Identification of global marine hotspots: sentinels for change and vanguards for adaptation action. Rev. Fish Biol. Fish. 24, 415–425. doi: 10.1007/s11160-013-9326-6
Hoskins A. J., Arnould J. P. Y. (2014). Relationship between long-term environmental fluctuations and diving effort of female Australian fur seals. Mar. Ecol. Prog. Ser. 511, 285–295. doi: 10.3354/meps10935
Hoskins A. J., Costa D. P., Arnould J. P. Y. (2015b). Utilisation of intensive foraging zones by female Australian fur seals. PloS One 10, e0117997. doi: 10.1371/journal.pone.0117997
Hoskins A. J., Costa D. P., Wheatley K. E., Gibbens J. R., Arnould J. P. Y. (2015a). Influence of intrinsic variation on foraging behaviour of adult female Australian fur seals. Mar. Ecol. Prog. Series. 526, 227–239. doi: 10.3354/meps11200
Hoskins A. J., Schumann N., Costa D. P., Arnould J. P. Y. (2017). Foraging niche separation in sympatric temperate-latitude fur seal species. Mar. Ecol. Prog. Series. 566, 229–241. doi: 10.3354/meps12024
Humphries N. E., Queiroz N., Dyer J. R. M., Pade N. G., Musyl M. K., Schaefer K. M., et al. (2010). Environmental context explains Lévy and Brownian movement patterns of marine predators. Nature 465, 1066–1069. doi: 10.1038/nature09116
Hvas M., Folkedal O., Imsland A., Oppedal F. (2017). The effect of thermal acclimation on aerobic scope and critical swimming speed in Atlantic salmon, Salmo salar. J. Exp. Biol. 220, 2757–2764. doi: 10.1242/jeb.154021
Jeanniard‐Du‐Dot T., Guinet C., Arnould J. P. Y., Speakman J. R., Trites A. W. (2017). Accelerometers can measure total and activity‐specific energy expenditures in free‐ranging marine mammals only if linked to time‐activity budgets. Funct. Ecol. 31, 377–386. doi: 10.1111/1365-2435.12729
Johnson M. (2011). Measuring the orientation and movement of marine animals using inertial and magnetic sensors-a tutorial. Fine-scale Anim. Move. Workshop 22, 1–22.
Johnson M. P., Tyack P. L. (2003). A digital acoustic recording tag for measuring the response of wild marine mammals to sound. IEEE J. ocean. engineer. 28, 3–12. doi: 10.1109/JOE.2002.808212
Kernaléguen L., Dorville N., Ierodiaconou D., Hoskins A. J., Baylis A. M. M., Hindell M. A., et al. (2016). From video recordings to whisker stable isotopes: a critical evaluation of timescale in assessing individual foraging specialisation in Australian fur seals. Oecologia 180, 657–670. doi: 10.1007/s00442-015-3407-2
Kirkman S. P., Arnould J. P. Y. (2018). “Cape and Australian fur seals: Arctocephalus pusillus pusillus and A. p. doriferus,” in Encyclopedia of Marine Mammals 3rd ed. Eds. Würsig B., Thewissen J. G. M., and Kovacs K. M. (Amsterdam, The Netherlands: Academic Press), 158–161. doi: 10.1016/B978-0-12-804327-1.00083-2
Kirkwood R., Arnould J. P. Y. (2008). “Australian fur seal,” in The mammals of Australia, 3rd edn. (Eds S. Van Dyck and R. Strahan.). (Reed New Holland: Sydney) 723–725.
Kirkwood R., Arnould J. P. Y. (2012). Foraging trip strategies and habitat use during late pup rearing by lactating Australian fur seals. Aust. J. Zool. 59, 216–226. doi: 10.1071/ZO11080
Lima S. L. (2002). Putting predators back into behavioral predator–prey interactions. Trends Ecol. Evol. 17, 70–75. doi: 10.1016/S0169-5347(01)02393-X
Luque S. P. (2007). Diving behaviour analysis in R. R news. 7, 8–14. Available at: https://CRAN.R-project.org/package=diveMove.
MacArthur R. H., Pianka E. R. (1966). On optimal use of a patchy environment. Am. Natural. 100, 603–609. doi: 10.1086/282454
Mattern T., Ellenberg U., Houston D. M., Davis L. S. (2007). Consistent foraging routes and benthic foraging behaviour in yellow-eyed penguins. Mar. Ecol. Prog. Series. 343, 295–306. doi: 10.3354/meps06954
Maxwell S. M., Frank J. J., Breed G. A., Robinson P. W., Simmons S. E., Crocker D. E., et al. (2012). Benthic foraging on seamounts: A specialized foraging behavior in a deep-diving pinniped. Mar. Mammal. Sci. 28, E333. doi: 10.1111/j.1748-7692.2011.00527.x
McMahon C. R., Field I. C., Bradshaw C. J. A., White G. C., Hindell M. A. (2008). Tracking and data–logging devices attached to elephant seals do not affect individual mass gain or survival. J. Exp. Mar. Biol. Ecol. 360, 71–77. doi: 10.1016/j.jembe.2008.03.012
Meyers N., Speakman C. N., Dorville N. A. Y., Hindell M. A., Semmens J. M., Monk J., et al. (2021). The cost of a meal: factors influencing prey profitability in Australian fur seals. PeerJ 9, e12608. doi: 10.7717/peerj.12608
Miller P. J. O., Biuw M., Watanabe Y. Y., Thompson D., Fedak M. A. (2012). Sink fast and swim harder! Round-trip cost-of-transport for buoyant divers. J. Exp. Biol. 215, 3622–3630. doi: 10.1242/jeb.070128
Miller H. J., Dodge S., Miller J., Bohrer G. (2019). Towards an integrated science of movement: converging research on animal movement ecology and human mobility science. Int. J. Geograph. Inf. Sci. 33, 855–876. doi: 10.1080/13658816.2018.1564317
Mitchell W. A. (2009). Multi-behavioral strategies in a predator–prey game: an evolutionary algorithm analysis. Oikos 118, 1073–1083. doi: 10.1111/j.1600-0706.2009.17204.x
Mitchell W. A., Lima S. L. (2002). Predator-prey shell games: large-scale movement and its implications for decision-making by prey. Oikos 99, 249–259. doi: 10.1034/j.1600-0706.2002.990205.x
Mobbs D., Trimmer P. C., Blumstein D. T., Dayan P. (2018). Foraging for foundations in decision neuroscience: insights from ethology. Nat. Rev. Neurosci. 19, 419–427. doi: 10.1038/s41583-018-0010-7
Mori Y., Mitani Y., Watanabe Y., Sato K. (2007). A behavioral indicator of prey patch richness derived from diving behavior: the proportion of residence time to the standard time. Israel J. Ecol. Evol. 53, 347–354. doi: 10.1560/IJEE.53.3.347
Morse D. H., Fritz R. S. (1987). “The consequences of foraging for reproductive success,” in Foraging behavior (Boston, MA: Springer US.), 443–455. doi: 10.1007/978-1-4613-1839-2_14
Niella Y., Butcher P., Holmes B., Barnett A., Harcourt R. (2022). Forecasting intraspecific changes in distribution of a wide-ranging marine predator under climate change. Oecologia 198, 111–124. doi: 10.1007/s00442-021-05075-7
Paiva V. H., Geraldes P., Ramírez I., Garthe S., Ramos J. A. (2010). How area restricted search of a pelagic seabird changes while performing a dual foraging strategy. Oikos 119, 1423–1434. doi: 10.1111/j.1600-0706.2010.18294.x
Pinaud D. (2008). Quantifying search effort of moving animals at several spatial scales using first-passage time analysis: effect of the structure of environment and tracking systems. J. Appl. Ecol. 45, 91–99. doi: 10.1111/j.1365-2664.2007.01370.x
Pinheiro J., Bates D., Debroy S., Sarkar D. (2007). Linear and nonlinear mixed effects models. R package version, Vol. 3. 1–89. Available at: https://CRAN.R-project.org/package=nlme.
Power M. E., Matthews W. J., Stewart A. J. (1985). Grazing minnows, piscivorous bass, and stream algae: dynamics of a strong interaction. Ecology 66, 1448–1456. doi: 10.2307/1938007
Pyke G. H. (1984). Optimal foraging theory: a critical review. Annu. Rev. Ecol. system. 15, 523–575. doi: 10.1146/annurev.es.15.110184.002515
Ramasco V., Barraquand F., Biuw M., Mcconnell B., Nilssen K. T. (2015). The intensity of horizontal and vertical search in a diving forager: the harbour seal. Move. ecol. 3, 1–16. doi: 10.1186/s40462-015-0042-9
R Core Team (2023). R: A Language and Environment for Statistical Computing (Vienna, Austria: R Foundation for Statistical Computing). Available at: https://www.R-project.org/.
Regular P. M., Hedd A., Montevecchi W. A. (2013). Must marine predators always follow scaling laws? Memory guides the foraging decisions of a pursuit-diving seabird. Anim. Behav. 86, 545–552. doi: 10.1016/j.anbehav.2013.06.008
Roth T. C. II, Lima S. L. (2007). Use of prey hotspots by an avian predator: purposeful unpredictability? Am. Nat. 169, 264–273. doi: 10.1086/510605
Sakamoto K. Q., Sato K., Ishizuka M., Watanuki Y., Takahashi A., Daunt F., et al. (2009). Can ethograms be automatically generated using body acceleration data from free-ranging birds? PloS One 4, e5379. doi: 10.1371/journal.pone.0005379
Salton M., Bestley S., Gales N., Harcourt R. (2022). Environmental drivers of foraging behaviour during long-distance foraging trips of male Antarctic fur seals. Anim. Behav. 183, 103–116. doi: 10.1016/j.anbehav.2021.11.006
Sato K., Mitani Y., Cameron M. F., Siniff D. B., Naito Y. (2003). Factors affecting stroking patterns and body angle in diving Weddell seals under natural conditions. J. Exp. Biol. 206, 1461–1470. doi: 10.1242/jeb.00265
Schober P., Boer C., Schwarte L. A. (2018). Correlation coefficients: appropriate use and interpretation. Anesth. Analg. 126, 1763–1768. doi: 10.1213/ANE.0000000000002864
Schreer J. F., Kovacs K. M., O’hara Hines R. J. (2001). Comparative diving patterns of pinnipeds and seabirds. Ecol. Monogr. 71, 137–162. doi: 10.1890/0012-9615(2001)071[0137:CDPOPA]2.0.CO;2
Shoji A., Elliott K. H., Greenwood J. G., Mcclean L., Leonard K., Perrins C. M., et al. (2015). Diving behaviour of benthic feeding Black Guillemots. Bird Study. 62, 217–222. doi: 10.1080/00063657.2015.1017800
Snelgrove P. V. (2001). “Diversity of marine species,” in Encyclopedia of Ocean Sciences. Eds. Steele J., Thorpe S., Turekian K. (Academic Press, Oxford), 748–757.
Speakman C. N., Hoskins A. J., Hindell M. A., Costa D. P., Hartog J. R., Hobday A. J., et al. (2020). Environmental influences on foraging effort, success and efficiency in female Australian fur seals. Sci. Rep. 10, 1–16. doi: 10.1038/s41598-020-73579-y
Stein R. A., Magnuson J. J. (1976). Behavioral response of crayfish to a fish predator. Ecology 57, 751–761. doi: 10.2307/1936188
Stephens D. W., Brown J. S., Ydenberg R. C. (2007). Foraging: Behavior and Ecology (Chicago: Univ. Press, Chicago).
Takahashi A., Dunn M. J., Trathan P. N., Sato K., Naito Y., Croxall J. P. (2003). Foraging strategies of chinstrap penguins at Signy Island, Antarctica: importance of benthic feeding on Antarctic krill. Mar. Ecol. Prog. Series. 250, 279–289. doi: 10.3354/meps250279
Thompson D., Fedak M. A. (1993). Cardiac responses of grey seals during diving at sea. J. Exp. Biol. 174, 139–154. doi: 10.1242/jeb.174.1.139
Thompson D., Hiby A., Fedak M. (1993). “How fast should I swim? Behavioral implications of diving physiology. In marine mammals: advances in behavioural and population biology: proceedings of a symposium held at the zoological society of London on 9th and 10th April 1992 ed. Boyd I. L. Clarendon Press, 349–368.
Tinbergen N., Impekoven M., Franck D. (1967). An experiment on spacing-out as a defence against predation. Behaviour 28, 307–320. doi: 10.1163/156853967X00064
Toscano B. J., Gownaris N. J., Heerhartz S. M., Monaco C. J. (2016). Personality, foraging behavior and specialization: integrating behavioral and food web ecology at the individual level. Oecologia 182, 55–69. doi: 10.1007/s00442-016-3648-8
Tremblay Y., Cherel Y. (2000). Benthic and pelagic dives: a new foraging behaviour in rockhopper penguins. Mar. Ecol. Prog. Series. 204, 257–267. doi: 10.3354/meps204257
Viviant M., Trites A. W., Rosen D. A., Monestiez P., Guinet C. (2010). Prey capture attempts can be detected in Steller sea lions and other marine predators using accelerometers. Polar Biol. 33, 713–719. doi: 10.1007/s00300-009-0750-y
Volpov B. L., Hoskins A. J., Battaile B. C., Viviant M., Wheatley K. E., Marshall G., et al. (2015). Identification of prey captures in Australian fur seals (Arctocephalus pusillus doriferus) using head-mounted accelerometers: field validation with animal-borne video cameras. PloS One 10, e0128789. doi: 10.1371/journal.pone.0128789
Weber S. B., Richardson A. J., Brown J., Bolton M., Clark B. L., Godley B. J., et al. (2021). Direct evidence of a prey depletion “halo” surrounding a pelagic predator colony. Proc. Natl. Acad. Sci. 118, e2101325118. doi: 10.1073/pnas.2101325118
Weimerskirch H. (2007). Are seabirds foraging for unpredictable resources? Deep Sea Res. Part II: Topic. Stud. Oceanogr. 54, 211–223. doi: 10.1016/j.dsr2.2006.11.013
Weimerskirch H., Le Corre M., Gadenne H., Pinaud D., Kato A., Ropert-Coudert Y., et al. (2009). Relationship between reversed sexual dimorphism, breeding investment and foraging ecology in a pelagic seabird, the masked booby. Oecologia 161, 637–649. doi: 10.1007/s00442-009-1397-7
Weimerskirch H., Pinaud D., Pawlowski F., Bost C. A. (2007). Does prey capture induce area-restricted search? A fine-scale study using GPS in a marine predator, the wandering albatross. Am. Nat. 170, 734–743. doi: 10.1086/522059
Werner E. E., Gilliam J. F., Hall D. J., Mittelbach G. G. (1983). An experimental test of the effects of predation risk on habitat use in fish. Ecology 64, 1540–1548. doi: 10.2307/1937508
Williams T. M., Davis R. W., Fuiman L. A., Francis J., Le Boeuf B. J., Horning M., et al. (2000). Sink or swim: strategies for cost-efficient diving by marine mammals. Science 288, 133–136. doi: 10.1126/science.288.5463.133
Wilson R. P., Griffiths I. W., Legg P. A., Friswell M. I., Bidder O. R., Halsey L. G., et al. (2013). Turn costs change the value of animal search paths. Ecol. letters. 16, 1145–1150. doi: 10.1111/ele.12149
Wilson R. P., Shepard E. L. C., Laich A. G., Frere E., Quintana F. (2010). Pedalling downhill and freewheeling up; a penguin perspective on foraging. Aquat. Biol. 8, 193–202. doi: 10.3354/ab00230
Wilson R. P., Wilson M. P. T. (1988). Foraging behaviour in four sympatric cormorants. J. Anim. Ecol. 57, 943–955. doi: 10.2307/5103
Zhang Y., Richardson J. S. (2007). Unidirectional prey–predator facilitation: apparent prey enhance predators’ foraging success on cryptic prey. Biol. Letters. 3, 348–351. doi: 10.1098/rsbl.2007.0087
Keywords: foraging ecology, Arctocephalus pusillus doriferus, hunting strategies, GPS tracking, 3D models
Citation: Salzeri P, Luque SP and Arnould JPY (2024) Fine-scale hunting strategies in Australian fur seals. Front. Mar. Sci. 11:1368756. doi: 10.3389/fmars.2024.1368756
Received: 11 January 2024; Accepted: 28 March 2024;
Published: 19 April 2024.
Edited by:
Mark E. Bond, Florida International University, United StatesReviewed by:
Aaron Wirsing, University of Washington, United StatesMichael R. Heithaus, Florida International University, United States
Copyright © 2024 Salzeri, Luque and Arnould. This is an open-access article distributed under the terms of the Creative Commons Attribution License (CC BY). The use, distribution or reproduction in other forums is permitted, provided the original author(s) and the copyright owner(s) are credited and that the original publication in this journal is cited, in accordance with accepted academic practice. No use, distribution or reproduction is permitted which does not comply with these terms.
*Correspondence: John P. Y. Arnould, john.arnould@deakin.edu.au