- 1Associate Laboratory i4HB – Institute for Health and Bioeconomy, NOVA School of Science and Technology, NOVA University of Lisbon, Caparica, Portugal
- 2UCIBIO – Applied Molecular Biosciences Unit, Department of Life Sciences, NOVA School of Science and Technology, NOVA University of Lisbon, Caparica, Portugal
The vast biological and biochemical diversity of the global ocean is the driver behind marine bioprospecting for novel bioproducts. As Marine Biotechnology is gaining momentum as one of the main pillars of the ‘Brue Growth’ revolution, the ability to screen for novel compounds of interest in species with little or no genomic resources is paramount. With this respect, proteins, which are easily metabolised, can be synthetised using convenient DNA recombinant methods and can easily be modified to better meet the needs of human society, making them prized targets. Evidently, proteins that hold natural bioactivity and specificity such as toxins and other venom components, have long captured the focus of biotechnologists, leading to the merger between environmental omics and toxinology termed as ‘venomics’. Indeed, bioactive proteins such as conopeptides, conotoxins, turripeptides and others are long deemed important subjects of research. Even though current mainstream paradigms set the focus on secondary metabolites from marine organisms, transcriptomics and proteomics approaches and their combination are rising strategies for screening for thousands of proteins and peptides in non-conventional biological models, emphasising, but not limited to, marine invertebrate animals due to their abundance, biodiversity and uncanny biochemical strategies to cope with selective pressure in literally every known marine habitat. Untargeted approaches, such as RNA-Seq – based transcriptomics and tandem mass spectrometry – based proteomics, can circumvent limitations related with absent or reduced genomic annotation. The present review will outline the main contributions of ‘omics’ and computational approaches for bioprospecting for proteinaceous marine bioactives. Despite the relatively low number of ‘omics’ studies with the main purpose of discover novel compounds, there is already important literature showcasing pipelines and approaches for revolutionising the exploration of the ocean.
1 Introduction
The global ocean is arguably the widest and most diversified source of bioactives. Motivated by its vast coastline and varied biogeography from the Mediterranean to the North Sea, the European Union (EU) set high prospects for turning marine bioprospecting into one of the means to lead the global ‘Bioeconomy Revolution’ in the near future, as new marine-derived bioproducts join ranks in novel potential human and veterinary pharmaceuticals and biomaterials; feeds and nutraceuticals; eco-friendly pesticides; anti-foulants, and other applications (see for instance Rodrigo and Costa, 2019; Vieira et al., 2020; Gonçalves and Costa, 2021). However, virtually all industrialised nations partake in this race, with potentially high benefits for scientists and their networks, industrialists and the end-point consumer. In addition, it is a major contribution to exploit novel and more sustainable marine resources. Evidently, this last, but not least, gain implies the industrial production of marine bioactives rather than its harvesting from the seas.
Bioactive compounds can be simply defined as substances that can interfere with a biological system, triggering a physiological consequence that can be either a response or a direct effect. Even though the concept became popularised by nutraceutical research (refer to Kussmann et al., 2023, for a recent review), ‘natural bioactive’ is far from being exhausted by food additive research and applications. In fact, compounds that interfere with specific molecular and downstream physiological processes hold great value as pharmaceuticals, for instance, depending on their specificity, potency and safety (Lindequist, 2016; Bordon et al., 2020). Many marine organisms secrete natural antibiotics, anti-foulants and defensive repellents, without neglecting venomous and poisonous animals that inject (in the first case) cocktails of neurotoxins with potential value as painkillers into their target recipients, just to mention few examples (Shen et al., 2000; Rodrigo and Costa, 2019; Turner et al., 2020; Guryanova et al., 2023). However, despite the promises of the seas as an almost inexhaustible source of novel bioactives, the real number of marine-derived pharmaceuticals, for instance, may seem staggering low (Martins et al., 2014; Cappello and Nieri, 2021). In fact, forty years ago, Colwell (1983) in a notorious paper in Science, had already pointed out the advantages and challenges of marine biotechnology and its close association to modern genetic engineering to scale-up production. Still, decades after, only about a dozen drugs derived from marine bioactives were tested and approved for commercial use in the USA and the EU since the late 1960s. This figure includes painkillers like Ziconotide (developed from a conotoxin protein), antivirals like Vidarabine (derived from a sponge) and anti-cancer drugs like Trabectedin (from a tropical tunicate), which is an alkaloid currently under application mostly for treating sarcomas (recently reviewed by Cappello and Nieri, 2021). Nonetheless, if we consider the challenges of bioprospecting through the oceans’ immense biodiversity and how to translate this endeavour into consumer products we may, at least partly, understand these seemingly meagre results compared to bioproducts of terrestrial origin, which directly or indirectly account for roughly 60% of pharmaceutical bioactives (Montaser and Luesch, 2011; Lindequist, 2016). We can also add the many gaps in the knowledge on basic aspects of the biology of marine organisms, from systematics to ecology and physiology, plus difficulties in accessing open water and deep sea habitats for prospecting (Molinski et al., 2009; Lindequist, 2016; Rodrigo and Costa, 2019). In addition, producing a natural compound in the laboratory can be cumbersome and may require detailed knowledge of unknown metabolic pathways.
From the current state-of-the-art, we may isolate two main approaches for marine bioprospecting: i) extensively cataloguing new metabolites and ii) directing searches towards specific needs. Whereas the second could systematise bioprospecting in favour of the needs of industry but seems still a mirage in the near future, the first is currently the mainstream approach that, albeit relying on a good deal of fortuitousness, is generating important databases especially (but not exclusively) for metabolites isolated from marine invertebrates and microorganisms (e.g., Hosseini et al., 2022, for a review) yet to be translated into consumer products. Regardless of approach, type of molecule and type of prospective application, it is clear that systematising marine bioprospecting is paramount. With this respect, advances in molecular and computational tools, including public databases, permit retrieving, analysing and storing huge amounts of data from marine biological samples and revolutionising bioprospecting (Ambrosino et al., 2019; Maghembe et al., 2020). These advances include high throughput methods, designed to analyse one or few endpoints (such as, proteins or mRNAs) in multiple biological samples in a single run, and ‘omics’ approaches, conceived to analyse multiple endpoints in single runs. While goals like the identification of multiple species (e.g., microbes) in single samples can bear enormous advantages, it is ‘omics’ that enable screening for multiple metabolites, proteins and mRNAs, with which databases of bioactives and marine genomic resources are enriched, with the added value of contributing to understand how compounds of interest are produced in vivo to as a means to achieve industrial scale-up via genetic engineering using convenient models such as bacteria and yeasts (Pennington et al., 2018; Maghembe et al., 2020).
The term ‘omics’ includes a range of techniques conceived to target specific classes of biomolecules, ultimately providing not only identification of large batches of substances by contrasting spectra and sequences against ever-growing databases but also their relative or absolute quantification (e.g., Martins et al., 2019; Maghembe et al., 2020; Madeira and Costa, 2021). These methods arose about two decades ago, arguably with proteomics taking the lead by combining gel-based separation methods followed by mass spectrometry (MS) (Madeira and Costa, 2021). Oligonucleotide microarrays, albeit targeted but able to screen for thousands of cDNAs in single runs for gene expression studies, rapidly became popular and are still widely used (Goodwin et al., 2016). Nonetheless, current advances brought by high-throughput sequencing, especially RNA-Seq, and the ability to assemble de novo entire transcriptomes without a priori reference mapping revolutionised the screening for putative proteins and peptides from novel species from up dozens of thousands open reading frames (ORFs) (Wang et al., 2009; Martins et al., 2019). Most importantly, these methods are untargeted whereas metabolomics must target more specific classes of molecules due to the biochemical diversity of secondary metabolites. Modern gel-independent separation techniques and tandem MS (MS/MS) coupled with rapidly improving computational tools and databases are major players in rendering proteomics and metabolomics into highly efficient methods now capable of identifying and quantifying thousands of molecules in non-conventional model organisms (Martins et al., 2019; Alseekh et al., 2021; Madeira and Costa, 2021). In addition, omics approaches may also revolutionise bioprospecting through the investigation the mechanisms of toxicity in test organisms and predict in silico the molecular targets of bioactives in humans, for instance.
In a time of rapid technical advances but slow translation of research into bio-economical assets, omics seem rightfully placed to tackle what is simultaneously the oceans’ best advantage and biggest challenge for biotechnologists – their unsurmountable biodiversity. This review will summarise the main advances; applications and contributions of omics for the prospecting of proteinaceous marine bioactives for biotechnological purposes.
2 Peptides vs. secondary metabolites
Peptides and proteins stand out owing to their ubiquitous metabolism. In addition, their target specificity and selectivity are particularly noteworthy in the biomedical field since they rarely bind to off-target and, therefore, rarely have side effects (see, for instance, the review by Leader et al., 2008). Moreover, peptides and proteins can be modified to improve potency and selectivity before heterologous expression using DNA recombinant technology in convenient biotechnological models such as bacteria and yeast, benefitting from standardised protocols that include retrieval and purification (see, for instance, Rivera-de-Torre et al., 2022 and references therein). On the other hand, a considerable number of enzymes or non-enzymatic catalysts is likely required for the synthesis of secondary metabolites. Thus, compromising cost effectiveness, assuming the biosynthetic pathways of metabolites have been determined in the first place. Nevertheless, it should be bear in mind that the different post-translational modifications needed for the protein to be active can dictate the host system and, consequently, the cost of production (Rivera-de-Torre et al., 2022).
Still, peptides and proteins have several limitations for biomedical applications. Susceptibility to enzymatic degradation and rapid clearance lead to a short plasma half-life as well as being degraded in the gastrointestinal tract. Additionally, their membranes bear reduced permeability to such large molecules, which hinders oral uptake and bioavailability. Altogether, the best entry route for peptide and protein as therapeutics is by subcutaneous injection which can turn these drugs into less attractive solutions as it can cause discomfort or pain during administration. Some of the peptides and proteins drawbacks are now being overcome with new strategies, such as, amino acid modifications (including changing to artificial ones), backbone modifications, conjugation and new formulations (for reviews on protein and peptide therapeutics, refer to Fosgerau and Hoffmann, 2015, and Erak et al., 2018). Finally, a word must be given to the relevance of protein-directed omics for the discovery not only of bioactives per se, but also to the enzymes involved in the bioprocesses of synthesis of compounds of interest. Even though this perspective is still in its infancy, it may be paramount to produce and eventually scale-up the typically cumbersome and costly production of secondary metabolites.
3 An overview of omics strategies for bioprospecting proteinaceous marine bioactives
The tremendous biodiversity of the oceans is, at the same time, a major booster and challenge for bioprospecting the marine resources with the ultimate goal of unravelling novel bioactives as there is much still to be explored. The many gaps found in the knowledge of these species can be counterpointed by omics and computational tools as they can be used for attempting to characterise new biomolecules as well as to predict their interactions, mode-of-action and effects (Rodrigo and Costa, 2019). Indeed, there are already some pipelines for trying to identify new biomolecules with biotechnological potential from sample collection to isolation and production of sequences of interest with potential biomedical applications (Figure 1 and Table 1). This includes analyses like transcriptomics and (or) proteomics to discover and sorting through hundreds or even thousands of molecules. Please refer to von Reumont et al. (2022) for an overview of protocols to collect venom from different groups of animals. Moreover, distinct procedures of extraction and sample preparation are adopted according to the type of biomolecules of interest (DNA, RNA, proteins or metabolites) and the omics platform or approach. The multiple number of omics methods are related with different levels and events from gene expression and its regulation to the metabolism. Omics thus encompasses various approaches to identify, characterise and quantify many biomolecules in single runs. Therefore, transcriptomic and proteomic profiling of non-conventional model organisms may facilitate the discovery of marine proteinaceous toxins and other bioactives with biomedical applications (Rodrigo and Costa, 2019). Nevertheless, the data obtained from bioinformatic analyses should be validated on the wet lab, for instance, by PCR and/or RT-qPCR for RNA-Seq strategies and by Western blot and/or ELISA for mass spectrometry. Moreover, the predicted functions and interactions with other substances should also be assessed through the evaluation of toxicity and bioreactivity of the compounds. These functional experiments can be performed in vitro followed by in vivo testing.
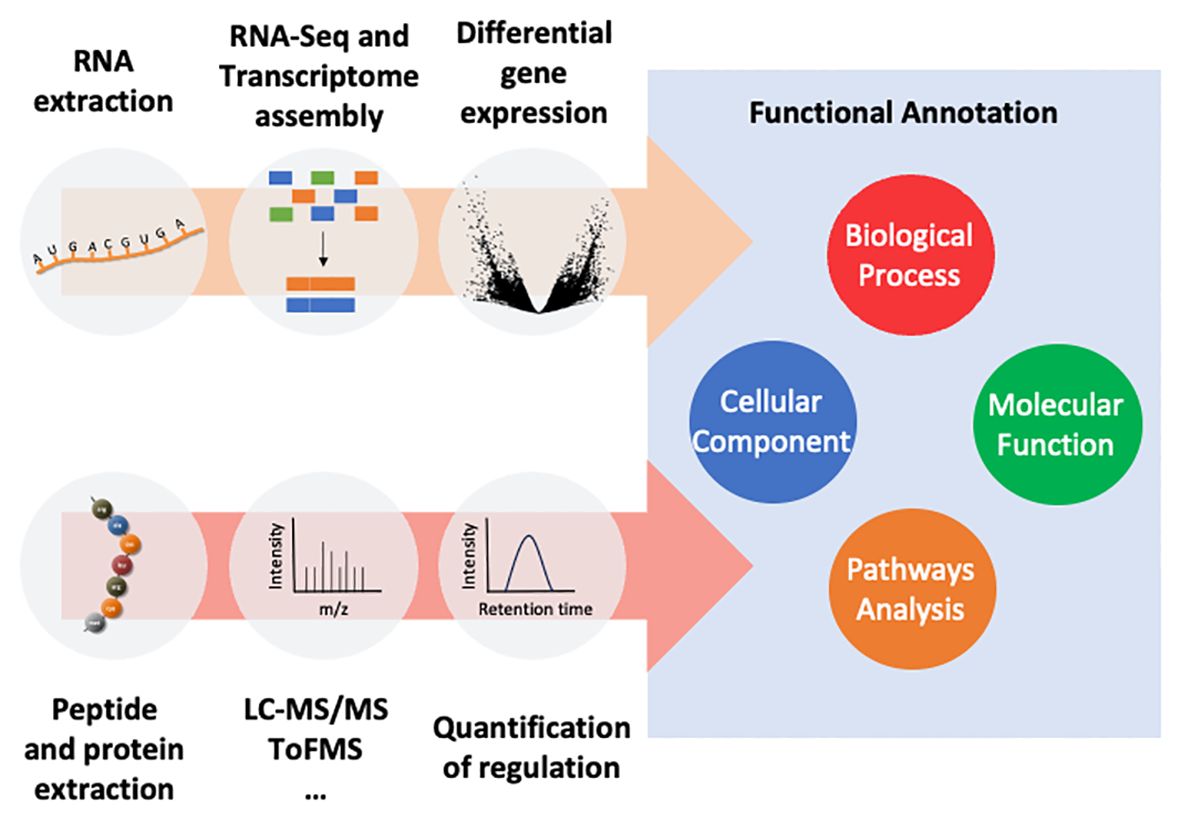
Figure 1 A pipeline to unravel novel proteinaceous bioactives through ‘omics’ strategies. Coding regions of putative genes of interest can be predicted from the assembled transcriptome. Proteomics can complement transcriptomics by validating peptides and proteins of interest. In either case, predicted coding regions or inferred peptides and proteins are functionally annotated by homology-matching against available and constantly-evolving databases. Finally, gene ontology and pathway analyses, for instance, may enable the discovery of function and physiological and molecular targets of novel biomolecules.
3.1 Transcriptomics
Due to reduced genomic resources available for marine organisms, untargeted approaches, such as next-generation RNA sequencing (RNA-Seq), allow to identify new molecules of interest through de novo transcriptome assembly and functional annotation. Afterwards, computational tools assist to reduce from many bioproducts to the ones with the most potential to be translate into the market. Nevertheless, there are some challenges associated with RNA-Seq, namely, the short length of the sequenced reads and a slight decrease in the accuracy compared to the Sanger sequencing.
Transcriptomics via RNA-Seq is an approach that aims at studying the whole-transcriptome and also quantifying gene expression (for a review, see for instance Wang et al., 2009; Stark et al., 2019). Although RNA-Seq requires massive computational resources, especially if de novo assembly is intended, and generates large amounts of data (which can turn its interpretation challenging), it is also an untargeted approach and consequently, may not require a genome or transcriptome of reference. Since all the different software and approaches to the transcriptomic pipeline are worthy of a full review, we will only focus on the most important aspects of the pipeline to provide a starting point. For a general overview, please refer to the dedicated reviews Conesa et al. (2016) and Stark et al. (2019), while for a review in RNA-Seq applied to venomics, turn to Verdes et al. (2016) and von Reumont (2018).
In most marine species, due to the absence of well-annotated genomes or transcriptomes to which map reads, de novo assembly of transcriptomes is essentially mandatory. There are several different computational applications for assembly of transcriptomes. However, as von Reumont et al. (2014) and Holding et al. (2018) pointed out, they can lead to distinct results as they have different algorithms and, consequently, can produce contigs distinct in length, number, identity and quality. Moreover, they also differ in the sensitivity to detect transcripts with low levels of expression. The performance of these software suites can be improved if the reads are paired-end and long. Holding et al. (2018) assessed the performance of software for de novo assembly of transcriptomes from venom-glands of snakes and scorpions. These authors proposed a combination of the results from multiple assemblers to recover a more complete group of transcripts encoding toxin with higher quality. While Rana et al. (2016) evaluated different software regarding de novo transcriptome assembly of killifish, Hölzer and Marz (2019) performed a comparison between software within distinct species. Altogether, the tool that outperforms the others appears to be different in these works and some authors also raised the hypothesis that comparing software is a difficult task and the best one can vary according to the species in study. Nevertheless, Trinity (Grabherr et al., 2011; Haas et al., 2013) appears to be popular in marine transcriptomic studies. Recently, MacManes (2018) proposed the Oyster River Protocol, which includes the usage of multiple software for de novo transcriptome assembly, and it has already been applied for the sea urchin transcriptome (Murano et al., 2022). As a rule, the reads for transcriptome assembly have to be mapped back against the transcriptome in order to quantify transcript abundance (alignment-based or alignment-free algorithms). The peptides and proteins with potential for biotechnological applications (for instance, toxins) might be expressed only in an organ or a few organs of interest. Therefore, a differential expression analysis between an organ of interest and a reference organ may facilitate sorting out through hundreds of thousands of transcripts.
Apart from de novo assembly, distinct methods to annotate the sequences also greatly influence the outcomes, as well as whether homology-based search employs translated or nucleotide sequences. Beforehand, predicting coding regions within the assembled transcripts must be performed using specific software like TransDecoder (Haas et al., 2013) or Trinotate (a suite that includes the first software and different tools used for annotation) (Bryant et al., 2017). For annotating the predicted ORFs (open reading frames), different approaches can be applied: a homology (reference) -based search against proteins present in public repositories, such as UniProt (The Uniprot Consortium, 2023) or RefSeq (O’Leary et al., 2016), or identification of conserved protein domains present in databases, for instance, Pfam or InterPro (Paysan-Lafosse et al., 2023). Blast (Camacho et al., 2009) is one of the most used tools for annotating proteins through homology-searches against public repositories. Since marine organism are not well-annotated, performing different runs against different databases and subsets like Tox-Prot (Jungo and Bairoch, 2005; Jungo et al., 2012) or lineage-specific (Smith and Undheim, 2018) can be an option for identifying with greater confidence a higher number of homologs such as toxins. Nevertheless, Smith and Undheim (2018) pointed out that homology-based searches are prone to erroneously identify non-toxin proteins as toxins. Despite some authors also using hmmer (Eddy, 2009) as standard for such searches, this software is better known for conserved domains.
There are other tools that help filtering the data and discover the potential function of the toxins. It could be performed a Gene Ontology (GO) analysis (The Gene Ontology Consortium, 2021) with the purpose of data mining and trying to identify and group the predicted translated sequences according to their potential function or activity. Another example is DAVID (Huang et al., 2009a, 2009b), which allows retrieving functional annotation. However, one drawback of this approach is that genes or proteins must be from a model organism. Therefore, for non-conventional marine organisms, their transcripts and proteins have to be converted into homologs. Something similar happens while using the R package multiGSEA (Ihaka and Gentleman, 1996; Canzler and Hackermüller, 2020) for performing a pathway enrichment analysis. Although this tool allows to integrate and combine data from various ‘omics’ during the analysis, it has a limitation as it can be only used with model organism. One possible solution to circumventing these annotation problems is to retrieve GO terms for close homologous proteins and perform a Fisher’s test. Other interesting tools are IPA (Krämer et al., 2014) and Cytoscape (Shannon et al., 2003), which enable analysing protein-protein interactions and retrieve non-canonical networks.
While analysing RNA-Seq data, some assumptions must be kept in mind. Specifically, we must be aware of the importance of RNA extraction protocols, as losses and hindered integrity of RNA can occur. In addition, a considerable part of RNA in cells is rRNA. Apart from some bias originated from different sequencing platforms, hindrances may also result from computation. They can be related with de novo assembly or mapping reads against the transcriptome of reference. Here, using long-read sequencing or/and paired-end sequencing could tent to bring more robustness to predictions. Altogether, this illustrates the importance of validating the transcriptomic results, namely through PCR and/or RT-qPCR. Moreover, alternative splicing, plus the fact that not all mRNA templates are translated and that translation could happen at different rates (without prejudice of post-translation modifications), might also bias the outcomes of in silico analyses. At least in part, these issues may be resolved by coupling transcriptomics with proteomics, as already described in the works by Rodrigo et al. (2021b); Rodrigo et al. (2022), or von Reumont et al. (2020) or even Whitelaw et al. (2016).
3.2 Proteomics
Mass spectrometry-based proteomics, regardless of separation methods, which are discussed elsewhere (e.g., Madeira and Costa, 2021), is a strategy also used for bioprospecting the oceans for novel proteinaceous bioactives by targeting more directly the final products of gene expression. Moreover, the post-translational modifications (PTMs) can also be detected or at least predicted by these methods. Still, shotgun proteomics typically yields a small number of molecules when compared to transcriptomics. Nevertheless, proteins are a product of gene expression in a more downstream position compared to transcripts, which can provide a more realistic vision of metabolic condition, i.e., closer to actual phenotype (Martins et al., 2019). In addition, addressing PTMs also provides a more realistic assessment of protein structure and therefore function and ligands. It must be noted, though, that, proteomics is based on the detection of fragmented proteins (producing ionised peptides usually with 10–20 amino acids) and therefore typically does not yield full peptidic sequences. However, as it offers a more realistic screening of functional proteins in organisms, we may expect a rise in its application in bioprospecting in the near future, marine bioprospecting inclusively, concomitant with steady advances in mass spectrometry (MS) and associated computational approaches. The reader may refer to Slattery et al. (2012); Aebersold and Mann (2016) and Walker et al. (2020) for dedicated reviews on marine proteomics, proteomics and proteomics applied to the study of venoms, respectively.
Although distinct methods and instruments can be used for proteomics, there is a common pipeline that involves separation of molecules, followed by their transference into the mass spectrometer and finally, data analysis (see, for instance, Madeira and Costa, 2021). One of these strategies is LC-MS/MS (liquid chromatography-tandem mass spectrometry), which joins together liquid chromatography as a separation method with electrospray ionisation mass spectrometry. From MS/MS spectra, peptides are inferred by contrasting against m/z ratio databases. Then, other algorithms, such as Mascot Server and ProteinPilot (Perkins et al., 1999; Shilov et al., 2007), contrast the resulting peptides against a database provided by the user, e.g. the entire UniProt (The Uniprot Consortium, 2023) vs the entire RefSeq (O’Leary et al., 2016) or a customised database, in order to try to tentatively identify proteins. On the other hand, quantification of proteins can be an absolute or relative depending on whether the proteomic approach is label-dependent or free (see, for instance, Anjo et al., 2015). After protein identification and quantification, functional annotation is performed similarly to transcriptomic studies. Some tools and analyses are in fact common to both omics, including GO analysis. There are also software tools that enable to predict the bioactivity of peptides such as MS-Digest from ProteinProspector (http://prospector.ucsf.edu/prospector/mshome.htm), which allows in silico proteolytic digestion of the proteins into small peptides, which are then ranked according to their potential with PeptideRanker software (Mooney et al., 2012). Moreover, analysing the differences in the quantification of regulation between target and reference organs may also help unravelling novel proteinaceous bioactives through background subtraction. This analysis can be also a solution to overcome a major drawback of proteomics, which is the overshading of rarer proteins by more abundant molecules.
By combining both transcriptomic and proteomic techniques, proteotranscriptomic studies might be more accurate. Using the translated predicting coding regions as the reference database to identify proteins could potentially avoid the misidentification of proteins as toxins when they are instead non-toxin (Smith and Undheim, 2018; von Reumont, 2018). Joining transcriptomic and proteomic studies can also be a method for validating the results. There are other possible strategies for validating in silico results of proteomics, such as performing ELISA or Western blot, given that antibodies are available or can be raised for the purpose. The forecasted outcomes predicted by proteotranscriptomic should also be evaluated through toxicity and bioreactivity experiments.
3.3 Discovery of novel bioactives with biotechnological potential through omics and computational approaches
3.3.1 Omics studies employed to unravel promising bioactives
Non-model marine invertebrates are promising candidates for bioprospecting due to their immense biodiversity, despite reduced or absent genomic annotation. Available literature employing transcriptomic or proteomic studies on these organisms for the purpose of bioprospecting is still disappointingly low. However, the state-of-the-art indicates promising perspectives (Table 2). We may refer, for instance, to the works of Urbarova et al. (2012); Ramírez-Carreto et al. (2019) and Alcaide et al. (2024) that refer the potential of sea anemone toxins as candidates for drug discovery. Indeed, Alcaide et al. (2024) complemented a shotgun proteomic approach with in vivo bioassays with zebrafish embryos to assert toxicity and bioactivity. Urbarova et al. (2012), in turn, studied the transcriptome of two sea anemones to identify potential neurotoxins using transcriptomics. The same authors also used SWISS-MODEL (Waterhouse et al., 2018) with the purpose of predicting protein structure. Moreover, Urbarova et al. (2012) also highlighted the importance of identifying conserved protein domains (linked with the proteins reactivity and interaction with other molecules) as a mean to unravel novel peptides or proteins, as it could also give clues about their molecular functions and docking to specific molecular targets, which is paramount for drug discovery.
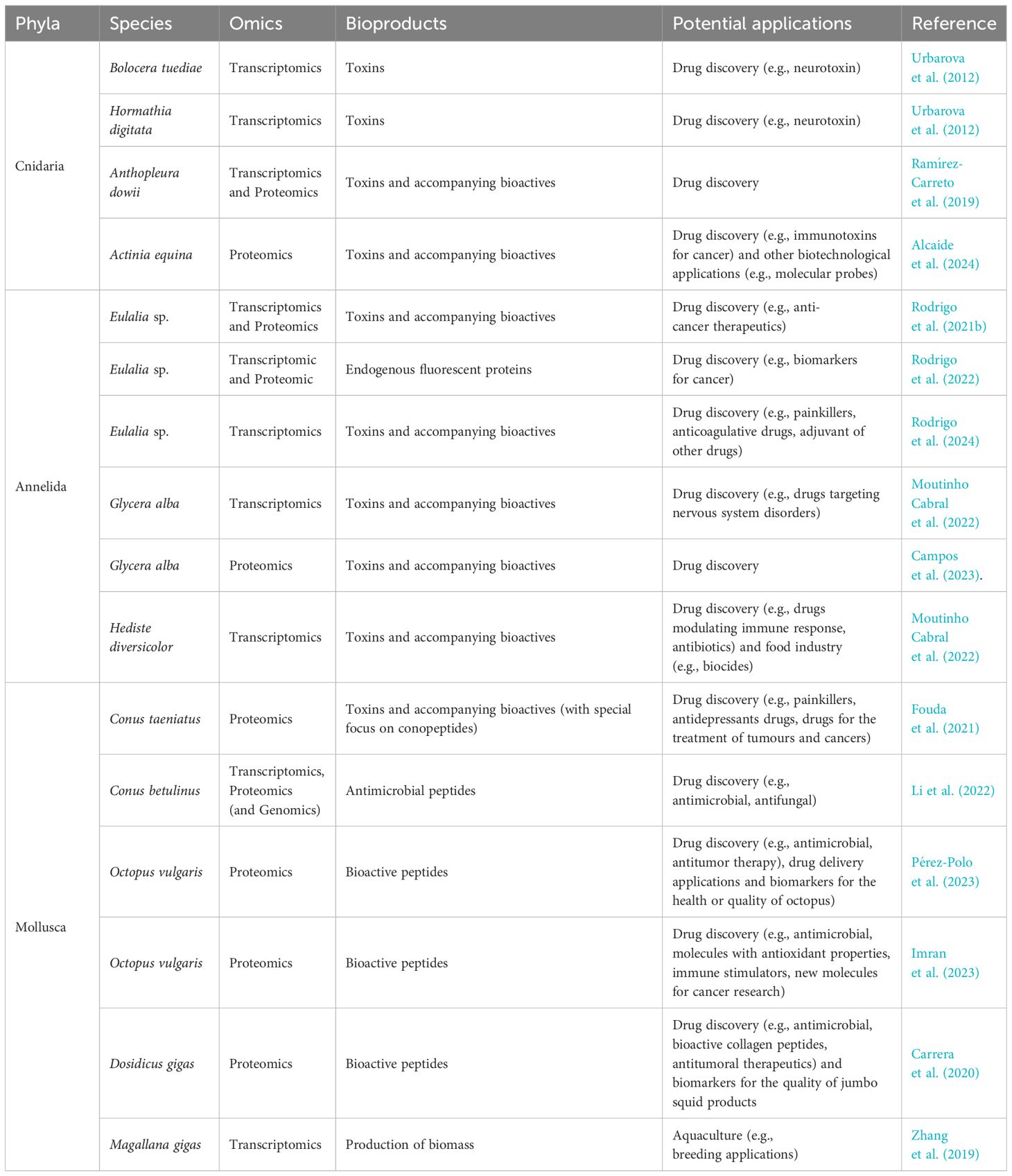
Table 2 Examples of non-conventional model marine organisms studied through ‘omics’ strategies to unravel novel bioproducts with potential biotechnological applications.
An interactome-directed analysis against the druggable human proteome (defined by Hopkins and Groom, 2002 as the human proteins that might interact with drug-like molecules) is another option to be considered before starting animals test and clinical trials. Through predicted protein-protein interactions, possible targets in the human proteome, including off-target (non-therapeutic drug targets) can be attributed to drug candidates. For this protein-protein interaction analysis, tools based on coevolutionary analysis and fragmental docking (Bai et al., 2016; Wang et al., 2020) that are being employed during the design of synthetic drugs could now potentially be applied in this novel pipeline for bioprospecting. Not only does this analysis attempt to predict the effects of the potential drugs on the human pathways, but it also might facilitate the mitigation of the side-effects. It is indeed the misidentification of targets and unexpected side-effects that lead to the cancellation of many clinical trials. Therefore, the outcome precludes possessing a shortlist of bioactives of interest. Altogether, this drug discovery approach could be a cost-effective alternative to other methods, such as the design of synthetic drugs. Despite being focused on Chordata, another interesting pipeline for bioprospecting, especially applied to drug discovery was presented in the study of Kirchhoff et al. (2021). Here, a network-based pharmacology analysis merged the transcriptomic profiling of distinct stingray species with venom bioactivity data obtained from bioassays in order to predict the toxins’ mechanism of action. In this screening assay, the stingray venom bioactivity was compared with the effects of hundreds of substances onto HeLa cells.
Among multi-level omics applied to biotechnology, we may refer to Rodrigo et al. (2021b) and Rodrigo et al. (2022), who complemented transcriptomics with proteomics to identify proteins with potential for biotechnological applications in the secretions of the polychaeta Eulalia sp. Rodrigo et al. (2021b) also performed network analysis based on protein-protein interaction using STRING (Szklarczyk et al., 2023) by finding the human homologs of the main proteins common to transcriptomic and proteomic data. With this work, the authors tried to shed some light on the mechanism that could be behind the fact that the secretions of Eulalia sp. targeted the cell cycle by provoking a cell arrest and inducing extrinsic programmed cell death in ovarian cancer cells. Moreover, through omics, Rodrigo et al. (2022) tried to identify peptides and proteins within Eulalia sp. secretions. The same authors also pointed out the presence of fluorescent proteinaceous complexes that can be uptaked by human cancer cells for potential use as probes. From the transcriptomic data of Eulalia sp., Rodrigo et al. (2024) successfully isolated six transcripts that encode potentially drug candidates for a range of different biomedical applications: from targeting the nervous system to influencing the immune response, ultimately aiming at heterologous expression and bioactivity testing.
Another possible approach to identify bioactives through omics was the comparative transcriptomics between two Polychaeta (Glycera alba and Hediste diversicolor) performed by Moutinho Cabral et al. (2022). Despite being sympatric, these annelids present different ecology, which can translate into expression of distinct toxins with potentially different molecular targets and thereby, biotechnological applications. The authors also performed an interactome-directed analysis against the human proteome using the HuRI platform (Luck et al., 2020). For the later analysis, human homologs of predicted ORFs were used for analyses, which can introduce bias. Altogether, Moutinho Cabral et al. (2022) showed that while the predator Glycera alba secretes proteinaceous toxins that might be applied to the treatment and management of diseases from the nervous systems, Hediste diversicolor might produce bioactives that could be used for modulating immune response or as biocides.
Transcriptomics and proteomics should also be complemented with an assessment of the interaction of the protein of interest with biological systems (e.g., toxicity and bioreactivity testing). An example of a work that joined proteomics in the search for toxins and other proteinaceous molecules of interest with bioassays for testing the cytotoxicity of the venom is Campos et al. (2023). Apart from this, the study of Glycera alba together with its transcriptomic analysis (Moutinho Cabral et al., 2022) further validate in silico analysis and reiterate the importance of complementing multi-level omics analyses to validate the discoveries. With this study, Campos et al. (2023) highlighted the detection of proteins bearing potential for biomedical applications, namely, glycerotoxin due to its possible interference with neuromuscular calcium channels.
Another example of interest is gastropod Conus taeniatus, whose proteome was analysed by Fouda et al. (2021). These authors matched the outcomes from MS/MS proteomics against a lineage-specific (Conus) database. The authors highlighted that some venom components of this cone snail could be candidates from drug discovery due to their possibility of interfering with ion channels or having antimicrobial proprieties. Li et al. (2022) study instead another Conus species. Here, the authors proposed a strategy to identify and characterise antimicrobial peptides through the use multi-level omics data. Apart from employing in silico tools for discovering physicochemical proprieties and predicting 3D structures for putative proteins, they also synthesised a few peptides and performed successful antimicrobial assays, thus highlighting the already acknowledged interest of cone snails for biotechnologists. In turn, Leal et al. (2022) performed a high-throughput search for antimicrobial peptides, but this time instead the analysis was purely in silico. This study on Gastropoda characterised several putative peptides and bioactivity was determined using PASS (Pirtskhalava et al., 2021).
Within the Mollusca, cephalopods are promising targets for bioprospecting. Distinct omics studies in Octopus vulgaris identified candidate proteins and peptides for biotechnological applications in the mucus produced by the skin and in the ink as well, namely molecules that might have antimicrobial, antitumoral, antiviral activities (among others), or even be used as potential immune stimulators (Imran et al., 2023; Pérez-Polo et al., 2023). These studies applied a similar methodology to identified peptides and proteins of interest by employing a shotgun proteomic strategy coupled with GO, pathway and network analyses. They attempted to predict peptides with bioactive potential through different software and databases, such as MS-Digest (http://prospector.ucsf.edu/prospector/mshome.htm) and PeptideRanker software as well as the databases included in BIOPEP-UWM or CAMP (Thomas et al., 2010; Mooney et al., 2012; Minkiewicz et al., 2019). Interestingly, Carrera et al. (2020) also used a similar approach for mining through the skin proteome of the jumbo squid (Dosidicus gigas). Here, the authors highlight putative peptides with several proprieties, such as antimicrobial and antitumoral, as well as bioactive collagen peptides. Nevertheless, Carrera et al. (2020) advocated the need to screen for the bioactivity of any recombinant form of these peptides. Moreover, they also pointed out advantages of using computational approaches to narrow the number of potential candidates.
While the previously mentioned marine organisms are of particular interest due to biomedical potential of their bioactives, there are also organisms worthy of mention for other biotechnological applications such as aquaculture. We may refer, for instance, to the study performed by Zhang et al. (2019) on the oyster Magallana gigas. Here, a comparative transcriptomic analysis and analysis of alternative splicing were employed between a line of oyster artificially-selected to have a fast growth and the wildtype. With this study, the authors intended to provide the first approach to the discovery of the molecular mechanism faster growth in these high-value bivalves.
3.3.2 Omics and computational approaches highlight organisms of interest
Not all marine omics studies aim directly at bioprospecting. In fact, the aim of multiple studies of “- omes” from non-conventional marine invertebrates ranges from pure descriptions of venoms to phylogenetic analyses. Nevertheless, these works can point out good roadmaps and organisms of interest, or even indirectly, molecules of interest from a pharmacological or biotechnological point of view. Altogether, these studies already indicate that, for instance, cephalopods, gastropods, cnidarians are examples of groups of marine invertebrates that could be enticing to further bioprospecting. Indeed, Ruder et al. (2013); Caruana et al. (2016); Whitelaw et al. (2016) and Almeida et al. (2020) performed a characterisation of the transcriptome or the proteome of cephalopods, which revealed the production of toxins, making this group of marine organisms particularly interesting. Nevertheless, they highlighted the need for further studies regarding cephalopods toxins and their function and targets. More specifically, apart from the transcriptomic and proteomic study of the slime produced by squid, Caruana et al. (2016) also performed a bioassay with shrimp (Artemia salina) to assess its toxicity. This is particularly noteworthy as in silico analyses only predict bioreactivity and forecast outcomes, which must be complement with further toxicity studies. While Ruder et al. (2013) employed a phylogenetic and structure analyses on coleoid venom components revealing interesting proteins, Whitelaw et al. (2016) also tried to predict post-translation modifications in toxins in the transcriptomic and proteomic study of the posterior salivary glands of two octopus. As toxins are bioproducts that are particularly interesting to be bioprospected, there is a direct link between venomics and drug discovery. On the other hand, Almeida et al. (2020) performed a cutting-edge shotgun proteomics study of posterior salivary glands (PSG) in Octopus vulgaris as the database used for the identification of peptides and proteins was a composite, which included UniProtKB data, the translated transcriptomes of PSG from cephalopods and antimicrobial peptides. These authors showed the presence of putative antimicrobial compounds, putative histones related with inflammatory responses and putative toxins in these salivary glands and raised the hypothesis of these substances integrate a first line of the host defensive barrier against pathogens.
A purely descriptive transcriptomic and proteomic study can also facilitate unravelling novel peptides for biotechnological purposes, namely within the domain of biomedicine (see for instance, Violette et al., 2012, for a work in a cone snail). Likewise, Brinkman et al. (2015) studied the transcriptome and proteome of a jellyfish and stated that this could be used as basis for different applications, from the search of novel bioactives in drug discovery to improve anti-envenomation therapy. It is also worthy to mention that these authors compared the venom profile of this species to other cnidarians. On the other hand, some venomics studies profiled the transcriptome or the proteome of marine invertebrate organisms without linking toxins to their biotechnological potential, i.e., aiming at more descriptive and ecological perspectives. Nevertheless, they are worthy of mention as they are roadmap for organisms of interest. See for instance, von Reumont et al. (2014) and Verdes et al. (2018) for further studies on Polychaeta, Modica et al. (2015) and Bose et al., 2017 for research on Gastropoda, von Reumont et al. (2020) for Nemertea and Macrander et al. (2016), as well as Klompen et al. (2021), for Cnidaria. Another example is Madio et al. (2017) work that integrated the proteomic data obtained from the ‘milked’ venom with the transcriptome of the tentacles of a sea anemone, while using strategies that can be employed to filter the data in order to reveal the proteins of interest. More specifically, the authors performed homology-directed searches against Tox-Prot and used the SignalP (Teufel et al., 2022) tool to forecast secreted proteins by searching for a signal peptide, which is an amino acid sequence that is characteristic of translocated or secreted proteins and might be present in toxin precursors.
Among the works that used omics to investigate the adaptive value of bioactives, Rodrigo et al. (2021a), through a combination between transcriptomics and microscopy, classified the Polychaeta Eulalia as a toxungen-secreting animal. Toxungens are toxins applied by surface contact as opposed to ‘venomous’, which stands for venom-injecting (see Nelsen et al., 2014, for disambiguation of terms). This work also included alignment and phylogenetic analyses of venom components, as well as a multigene phylogenic analysis performed using MrBayes 3 (Ronquist et al., 2012). Moreover, this same work also highlighted that toxins could affect neuromuscular responses, hold anti-coagulant action and promote permeabilisation of tissues, altogether serving as a roadmap for proteins of interest that were studied in subsequent works Rodrigo et al. (2021b); Rodrigo et al. (2022) and Rodrigo et al. (2024). Finally, a word must be given to the combination of omics and other endpoints, such as toxicity testing, not just as validation but also to assist refining research. However, this strategy is not yet part of mainstream approaches. For instance, D’Ambrosio et al. (2022) showed that both annelids Glycera alba and Hediste diversicolor secrete toxins with different purposes, namely predation and defence, respectively, through toxicity testing onto marine bivalves as a surrogate for realistic targets. These results steered research into specific substances of interest in the transcriptomic study of these two annelids (Moutinho Cabral et al., 2022). Another example is the work of Magdy et al. (2023), where it was shown that the venom of Conus flavidus is at least two-fold more cytotoxic to the HepG2 cancer cell line than to a normal cell line. Joining this interesting work with the analysis of the transcriptome and proteome of this species described in Himaya et al. (2022) could lead to the identification of novel candidates for drug discovery.
3.3.3 Marine natural products translated into drugs: approved or in clinical trials
There are marine toxins that have already been translated into approved marine-based drugs, despite its number still being disappointing (for dedicated reviews, the reader may refer to Molinski et al., 2009; Martins et al., 2014 and Cappello and Nieri, 2021). A landmark in marine biotechnology was the approval of Ziconotide for management of severe chronic pain (Williams et al., 2008). This drug is a synthetic peptide of a conotoxin produced by the cone-snail Conus magus (Olivera et al., 1987). Even though this conotoxin was not discovered and described through an ‘omics’ study (nor could it in the late 1970s’), we may reasonably argue that a larger number of peptides and proteins can be predicted or identified using transcriptomics or proteomics beforehand.
Toxins are not, however, the only peptidic or protein-based marine drugs already in the market. Brentuximab vedotin is an example of an approved antibody-drug conjugate for the treatment of lymphomas (Makita et al., 2020). This drug is derived from dolastatin, a depsipeptide with antimitotic activity isolated from the sea hare Dolabella auricolaria (Pettit et al., 1987; Bai et al., 1990). Interestingly, some studies revealed that this molecule is the product of a symbiotic cyanobacteria (Luesch et al., 2001). Another example, Plitidepsin is an approved drug in the treatment of multiple myeloma in Australia (see, for instance, Alonso-Álvarez et al., 2017), currently under Phase III clinical trials for the treatment for COVID-19 (White et al., 2021). This cyclic depsipeptide is produced by the sea tunicate Aplidium albicans (Urdiales et al., 1996).
Among other compounds based on or derived from marine peptides and protein that are currently under clinical trials, we may find dalazatide. This is a synthetic peptide derived from the toxin ShK isolated from sun anemone Stichodactyla helianthus that completed Phase I clinical trials and revealed promising results for the treatment of autoimmune diseases (Castañeda et al., 1995; Tarcha et al., 2017). We may also refer to the turripeptide ubi3a as potential candidate for drug development. This peptide, which was isolated from the gastropod Unedogemmula bisaya from the Central Pacific Ocean, was found to be a promising neuromodulator as it provoked tremors and an impaired locomotion on mice for a few hours upon its intracranial injection (Omaga et al., 2017).
4 Conclusions
The oceans’ immense biodiversity is simultaneously an asset and a challenge for biotechnologists. Protein-derived or inspired natural bioactives, offer multiple advantages for the development of novel drugs or safer pesticides and antifoulants since they can be easily metabolised, synthetised and modified to maximise efficacy and safety. Even though marine bioprospecting for novel proteinaceous bioactives is far from systematised, there are already approved drugs derived from proteins from marine organisms, especially invertebrates, which illustrates an immense potential for applied research. Despite the lack of standardisation, which arguably results from the pioneering nature of most research involving marine omics, recent research has been disclosing that transcriptomics, proteomics and, moreover, their combination, can offer a cross-validated screening for novel proteins of interest. Indeed, due to their unbiased disposition to assemble transcriptomes and proteomes de novo, transcriptomics and proteomics have been very successfully applied and develop to face the challenges of handling with non-conventional models if not almost obscure species altogether. The ability to merge biomedical-directed databases in search for possible targets from the novel proteins in the human interactome or druggable proteome can greatly systematise marine bioprospecting for the purpose of drug discovery and therapeutics. In addition, these omics can effectively assist finding enzymes partaking in biosynthetic processes for metabolites of interest, which is paramount, e.g., for industrial scale-up using convenient microbiological models. Moreover, even though proteinaceous biomaterials have not been the subject of the present review, they also represent a promising target for bioprospecting. Finally, it must not be neglected that transcriptomic and proteomics can be employed to screen for bioactivity and toxicity at the molecular level in selected organisms.
Author contributions
IMC: Data curation, Writing – original draft, Writing – review & editing. CG: Writing – original draft, Writing – review & editing. ARG: Conceptualization, Supervision, Writing – original draft, Writing – review & editing. PMC: Conceptualization, Funding acquisition, Supervision, Writing – original draft, Writing – review & editing.
Funding
The author(s) declare financial support was received for the research, authorship, and/or publication of this article. Fundo Azul co-financed project MARVEN (FA_05_2017_007). The Portuguese Foundation for Science and Technology (FCT), I. P., funded project WormALL (PTDC/BTA-BTA/28650/2017). This work was also financed by the National Funds from FCT in the scope of the project UIDP/04378/2020 and UIDB/04378/2020 of the Research Unit on Applied Molecular Biosciences – UCIBIO and the project LA/P/0140/2020 of the Associate Laboratory Institute for Health and Bioeconomy – i4HB. FCT is also acknowledged for the grants SFRH/BD/144914/2019 to CG and 2022.11150.BD to IMC.
Acknowledgments
Open-access publication fees were covered by the state of Bremen.
Conflict of interest
The authors declare that the research was conducted in the absence of any commercial or financial relationships that could be construed as a potential conflict of interest.
The author(s) declared that they were an editorial board member of Frontiers, at the time of submission. This had no impact on the peer review process and the final decision.
Publisher’s note
All claims expressed in this article are solely those of the authors and do not necessarily represent those of their affiliated organizations, or those of the publisher, the editors and the reviewers. Any product that may be evaluated in this article, or claim that may be made by its manufacturer, is not guaranteed or endorsed by the publisher.
References
Aebersold R., Mann M. (2016). Mass-spectrometric exploration of proteome structure and function. Nature 537, 347–355. doi: 10.1038/nature19949
Alcaide M., Moutinho Cabral I., Carvalho L., Mendes V. M., Alves de Matos A. P., Manadas B., et al. (2024). A comparative analysis of the venom system between two morphotypes of the sea anemone. Anim. (Basel) 14, 981. doi: 10.3390/ani14060981
Almeida D., Domínguez-Pérez D., Matos A., Agüero-Chapin G., Osório H., Vasconcelos V., et al. (2020). Putative antimicrobial peptides of the posterior salivary glands from the cephalopod Octopus vulgaris revealed by exploring a composite protein database. Antibiotics (Basel) 9, 757. doi: 10.3390/antibiotics9110757
Alonso-Álvarez S., Pardal E., Sánchez-Nieto D., Navarro M., Caballero M. D., Mateos M. V., et al. (2017). Plitidepsin: design, development, and potential place in therapy. Drug Des. Devel Ther. 11, 253–264. doi: 10.2147/DDDT.S94165
Alseekh S., Aharoni A., Brotman Y., Contrepois K., D’Auria J., Ewald J., et al. (2021). Mass spectrometry-based metabolomics: a guide for annotation, quantification and best reporting practices. Nat. Methods 18, 747–756. doi: 10.1038/s41592–021-01197–1
Ambrosino L., Tangherlini M., Colantuono C., Esposito A., Sangiovanni M., Miralto M., et al. (2019). Bioinformatics for marine products: an overview of resources, bottlenecks, and perspectives. Mar. Drugs 17, 576. doi: 10.3390/md17100576
Anjo S. I., Santa C., Manadas B. (2015). Short GeLC-SWATH: a fast and reliable quantitative approach for proteomic screenings. Proteomics 15, 757–762. doi: 10.1002/pmic.201400221
Bai F., Morcos F., Cheng R. R., Jiang H., Onuchic J. N. (2016). Elucidating the druggable interface of protein-protein interactions using fragment docking and coevolutionary analysis. Proc. Natl. Acad. Sci. U S A. 113, E8051–E8058. doi: 10.1073/pnas.1615932113
Bai R., Pettit G. R., Hamel E. (1990). Dolastatin 10, a powerful cytostatic peptide derived from a marine animal. Inhibition of tubulin polymerization mediated through the vinca alkaloid binding domain. Biochem. Pharmacol. 39, 1941–1949. doi: 10.1016/0006–2952(90)90613-p
Barrett T., Troup D. B., Wilhite S. E., Ledoux P., Evangelista C., Kim I. F., et al. (2011). NCBI GEO: archive for functional genomics data sets-10 years on. Nucleic Acids Res. 39, D1005–D1010. doi: 10.1093/nar/gkq1184
Benson D. A., Cavanaugh M., Clark K., Karsch-Mizrachi I., Lipman D. J., Ostell J., et al. (2013). GenBank. Nucleic Acids Res. 41, D36–D42. doi: 10.1093/nar/gks1195
Bordon K. C. F., Cologna C. T., Fornari-Baldo E. C., Pinheiro-Júnior E. L., Cerni F. A., Amorim F. G., et al. (2020). From animal poisons and venoms to medicines: achievements, challenges and perspectives in drug discovery. Front. Pharmacol. 11. doi: 10.3389/fphar.2020.01132
Bose U., Wang T., Zhao M., Motti C. A., Hall M. R., Cummins S. F. (2017). Multiomics analysis of the giant triton snail salivary gland, a crown-of-thorns starfish predator. Sci. Rep. 7, 6000. doi: 10.1038/s41598–017-05974-x
Bray N. L., Pimentel H., Melsted P., Pachter L. (2016). Near-optimal probabilistic RNA-seq quantification. Nat. Biotechnol. 34, 525–527. doi: 10.1038/nbt.3519
Brinkman D. L., Jia X., Potriquet J., Kumar D., Dash D., Kvaskoff D., et al. (2015). Transcriptome and venom proteome of the box jellyfish Chironex fleckeri. BMC Genomics 16, 407. doi: 10.1186/s12864–015-1568–3
Bryant D. M., Johnson K., DiTommaso T., Tickle T., Couger M. B., Payzin-Dogru D., et al. (2017). A tissue-mapped axolotl de novo transcriptome enables identification of limb regeneration factors. Cell Rep. 18, 762–776. doi: 10.1016/j.celrep.2016.12.063
Camacho C., Coulouris G., Avagyan V., Ma N., Papadopoulos J., Bealer K., et al. (2009). BLAST+: architecture and applications. BMC Bioinf. 10, 421. doi: 10.1186/1471–2105-10–421
Campos S., Rodrigo A. P., Moutinho Cabral I., Mendes V. M., Manadas B., D’Ambrosio M., et al. (2023). An exploration of novel bioactives from the venomous marine annelid Glycera alba. Toxins (Basel) 15, 655. doi: 10.3390/toxins15110655
Canzler S., Hackermüller J. (2020). multiGSEA: A GSEA-based pathway enrichment analysis for multi-omics data. BMC Bioinf. 21, 561. doi: 10.1186/s12859–020-03910-x
Cappello E., Nieri P. (2021). From life in the sea to the clinic: The marine drugs approved and under clinical trial. Life 11, 1390. doi: 10.3390/life11121390
Carrera M., Ezquerra-Brauer J. M., Aubourg S. P. (2020). Characterization of the jumbo squid (Dosidicus gigas) skin by-product by shotgun proteomics and protein-based bioinformatics. Mar. Drugs 18, 31. doi: 10.3390/md18010031
Caruana N. J., Cooke I. R., Faou P., Finn J., Hall N. E., Norman M., et al. (2016). A combined proteomic and transcriptomic analysis of slime secreted by the southern bottletail squid, Sepiadarium austrinum (Cephalopoda). J. Proteomics 148, 170–182. doi: 10.1016/j.jprot.2016.07.026
Castañeda O., Sotolongo V., Amor A. M., Stöcklin R., Anderson A. J., Harvey A. L., et al. (1995). Characterization of a potassium channel toxin from the Caribbean Sea anemone. Stichodactyla helianthus Toxicon. 33, 603–613. doi: 10.1016/0041–0101(95)00013-c
Colwell R. R. (1983). Biotechnology in the marine sciences. Science 222, 19–24. doi: 10.1126/science.222.4619.19
Conesa A., Madrigal P., Tarazona S., Gomez-Cabrero D., Cervera A., McPherson A., et al. (2016). A survey of best practices for RNA-seq data analysis. Genome Biol. 17, 13. doi: 10.1186/s13059–016-0881–8
Cox J., Mann M. (2008). MaxQuant enables high peptide identification rates, individualized p.p.b.-range mass accuracies and proteome-wide protein quantification. Nat. Biotechnol. 26, 1367–1372. doi: 10.1038/nbt.1511
D’Ambrosio M., Ramos Í., Martins C., Costa P. M. (2022). An investigation into the toxicity of tissue extracts from two distinct marine Polychaeta. Toxicon. X. 14, 100116. doi: 10.1016/j.toxcx.2022.100116
Deutsch E. W., Bandeira N., Perez-Riverol Y., Sharma V., Carver J. J., Mendoza L., et al. (2023). The ProteomeXchange consortium at 10 years: 2023 update. Nucleic Acids Res. 51, D1539–D1548. doi: 10.1093/nar/gkac1040
Dominguez C., Boelens R., Bonvin A. M. J. J. (2003). HADDOCK: a protein-protein docking approach based on biochemical or biophysical information. J. Am. Chem. Soc 125, 1731–1737. doi: 10.1021/ja026939x
Eddy S. R. (2009). A new generation of homology search tools based on probabilistic inference. Genome Inform. 23, 205–211.
Erak M., Bellmann-Sickert K., Els-Heindl S., Beck-Sickinger A. G. (2018). Peptide chemistry toolbox - Transforming natural peptides into peptide therapeutics. Bioorg. Med. Chem. 26, 2759–2765. doi: 10.1016/j.bmc.2018.01.012
Fosgerau K., Hoffmann T. (2015). Peptide therapeutics: Current status and future directions. Drug Discovery Today 20, 122–128. doi: 10.1016/j.drudis.2014.10.003
Fouda M. M. A., Abdel-Wahab M., Mohammadien A., Germoush M. O., Sarhan M. (2021). Proteomic analysis of Red Sea Conus taeniatus venom reveals potential biological applications. J. Venom. Anim. Toxins Incl. Trop. Dis. 27, e20210023. doi: 10.1590/1678–9199-JVATITD-2021–0023
Gonçalves C., Costa P. M. (2021). Cephalotoxins: A hotspot for marine bioprospecting? Front. Mar. Sci. 8. doi: 10.3389/fmars.2021.647344
Goodwin S., McPherson J. D., McCombie W. R. (2016). Coming of age: ten years of next-generation sequencing technologies. Nat. Rev. Genet. 17, 333–351. doi: 10.1038/nrg.2016.49
Grabherr M. G., Haas B. J., Yassour M., Levin J. Z., Thompson D. A., Amit I., et al. (2011). Full-length transcriptome assembly from RNA-Seq data without a reference genome. Nat. Biotechnol. 29, 644–652. doi: 10.1038/nbt.1883
Guryanova S. V., Balandin S. V., Belogurova-Ovchinnikova O. Y., Ovchinnikova T. V. (2023). Marine invertebrate antimicrobial peptides and their potential as novel peptide antibiotics. Mar. Drugs 21, 503. doi: 10.3390/md21100503
Haas B. J., Papanicolaou A., Yassour M., Grabherr M., Blood P. D., Bowden J., et al. (2013). De novo transcript sequence reconstruction from RNA-seq using the Trinity platform for reference generation and analysis. Nat. Protoc. 8, 1494–1512. doi: 10.1038/nprot.2013.084
Himaya S. W. A., Arkhipov A., Yum W. Y., Lewis R. J. (2022). Comparative venomics of C. flavidus and C. frigidus and closely related vermivorous cone snails. Mar. Drugs 20, 209. doi: 10.3390/md20030209
Holding M. L., Margres M. J., Mason A. J., Parkinson C. L., Rokyta D. R. (2018). Evaluating the performance of de novo assembly methods for venom-gland transcriptomics. Toxins (Basel) 10, 249. doi: 10.3390/toxins10060249
Hölzer M., Marz M. (2019). De novo transcriptome assembly: A comprehensive cross-species comparison of short-read RNA-Seq assemblers. Gigascience 8, giz039. doi: 10.1093/gigascience/giz039
Hopkins A. L., Groom C. R. (2002). The druggable genome. Nat. Rev. Drug Discovery 1, 727–730. doi: 10.1038/nrd892
Hosseini H., Al-Jabri H. M., Moheimani N. R., Siddiqui S. A., Saadaoui I. (2022). Marine microbial bioprospecting: Exploitation of marine biodiversity towards biotechnological applications-a review. J. Basic Microbiol. 62, 1030–1043. doi: 10.1002/jobm.202100504
Huang D. W., Sherman B. T., Lempicki R. A. (2009a). Bioinformatics enrichment tools: paths toward the comprehensive functional analysis of large gene lists. Nucleic Acids Res. 37, 1–13. doi: 10.1093/nar/gkn923
Huang D. W., Sherman B. T., Lempicki R. A. (2009b). Systematic and integrative analysis of large gene lists using DAVID bioinformatics resources. Nat. Protoc. 4, 44–57. doi: 10.1038/nprot.2008.211
Ihaka R., Gentleman R. (1996). R: A language for data analysis and graphics. J. Comput. Graph. Stat. 5, 299–314. doi: 10.1080/10618600.1996.10474713
Imran M. A. S., Carrera M., Pérez-Polo S., Pérez J., Barros L., Dios S., et al. (2023). Insights into common octopus (Octopus vulgaris) ink proteome and bioactive peptides using proteomic approaches. Mar. Drugs 21, 206. doi: 10.3390/md21040206
Jones G., Willett P., Glen R. C., Leach A. R., Taylor R. (1997). Development and validation of a genetic algorithm for flexible docking. J. Mol. Biol. 267, 727–748. doi: 10.1006/jmbi.1996.0897
Jungo F., Bairoch A. (2005). Tox-Prot, the toxin protein annotation program of the Swiss-Prot protein knowledgebase. Toxicon 45, 293–301. doi: 10.1016/j.toxicon.2004.10.018
Jungo F., Bougueleret L., Xenarios I., Poux S. (2012). The UniProtKB/Swiss-Prot Tox-Prot program: A central hub of integrated venom protein data. Toxicon 60, 551–557. doi: 10.1016/j.toxicon.2012.03.010
Kanehisa M., Furumichi M., Sato Y., Kawashima M., Ishiguro-Watanabe M. (2023). KEGG for taxonomy-based analysis of pathways and genomes. Nucleic Acids Res. 51, D587–D592. doi: 10.1093/nar/gkac963
Kirchhoff K. N., Billion A., Voolstra C. R., Kremb S., Wilke T., Vilcinskas A. (2021). Stingray venom proteins: mechanisms of action revealed using a novel network pharmacology approach. Mar. Drugs 20, 27. doi: 10.3390/md20010027
Klompen A. M. L., Kayal E., Collins A. G., Cartwright P. (2021). Phylogenetic and selection analysis of an expanded family of putatively pore-forming jellyfish toxins (Cnidaria: Medusozoa). Genome Biol. Evol. 13, evab081. doi: 10.1093/gbe/evab081
Krämer A., Green J., Pollard J., Tugendreich S. (2014). Causal analysis approaches in Ingenuity Pathway Analysis. Bioinformatics 30, 523–530. doi: 10.1093/bioinformatics/btt703
Kussmann M., Abe Cunha D. H., Berciano S. (2023). Bioactive compounds for human and planetary health. Front. Nutr. 10. doi: 10.3389/fnut.2023.1193848
Leader B., Baca Q. J., Golan D. E. (2008). Protein therapeutics: a summary and pharmacological classification. Nat. Rev. Drug Discovery 7, 21–39. doi: 10.1038/nrd2399
Leal E., Múnera M., Suescún-Bolívar L. P. (2022). In silico characterization of Cnidarian’s antimicrobial peptides. Front. Mar. Sci. 9. doi: 10.3389/fmars.2022.1065717
Li B., Dewey C. N. (2011). RSEM: accurate transcript quantification from RNA-Seq data with or without a reference genome. BMC Bioinf. 12, 323. doi: 10.1186/1471–2105-12–323
Li R., Huang Y., Peng C., Gao Z., Liu J., Yin X., et al. (2022). High-throughput prediction and characterization of antimicrobial peptides from multi-omics datasets of Chinese tubular cone snail (Conus betulinus). Front. Mar. Sci. 9. doi: 10.3389/fmars.2022.1092731
Lindequist U. (2016). Marine-derived pharmaceuticals - challenges and opportunities. Biomol. Ther. (Seoul) 24, 561–571. doi: 10.4062/biomolther.2016.181
Love M. I., Huber W., Anders S. (2014). Moderated estimation of fold change and dispersion for RNA-seq data with DESeq2. Genome Biol. 15, 550. doi: 10.1186/s13059–014-0550–8
Luck K., Kim D. K., Lambourne L., Spirohn K., Begg B. E., Bian W., et al. (2020). A reference map of the human binary protein interactome. Nature 580, 402–408. doi: 10.1038/s41586–020-2188-x
Luesch H., Moore R. E., Paul V. J., Mooberry S. L., Corbett T. H. (2001). Isolation of dolastatin 10 from the marine cyanobacterium Symploca species VP642 and total stereochemistry and biological evaluation of its analogue symplostatin 1. J. Nat. Prod. 64, 907–910. doi: 10.1021/np010049y
MacManes M. D. (2018). The Oyster River Protocol: A multi-assembler and kmer approach for de novo transcriptome assembly. PeerJ 6, e5428. doi: 10.7717/peerj.5428
Macrander J., Broe M., Daly M. (2016). Tissue-specific venom composition and differential gene expression in sea anemones. Genome Biol. Evol. 8, 2358–2375. doi: 10.1093/gbe/evw155
Madeira C., Costa P. M. (2021). “Proteomics in systems toxicology,” in Advances in protein chemistry and structural biology, vol. 127 . Ed. Donev R. (Academic Press, London, UK), 55–91. doi: 10.1016/bs.apcsb.2021.03.001
Madio B., Undheim E. A. B., King G. F. (2017). Revisiting venom of the sea anemone Stichodactyla haddoni: Omics techniques reveal the complete toxin arsenal of a well-studied sea anemone genus. J. Proteomics 166, 83–92. doi: 10.1016/j.jprot.2017.07.007
Magdy N. A., Nafie M. S., El-Naggar M. S., Abu El-Regal M. A., Abdel Azeiz A. Z., Abdel-Rahman M. A., et al. (2023). Cytotoxicity and apoptosis induction of the marine Conus flavidus venom in HepG2 cancer cell line. J. Biomol. Struct. Dyn. 41, 7786–7793. doi: 10.1080/07391102.2022.2125075
Maghembe R., Damian D., Makaranga A., Nyandoro S. S., Lyantagaye S. L., Kusari S., et al. (2020). Omics for bioprospecting and drug discovery from bacteria and microalgae. Antibiotics (Basel) 9, 229. doi: 10.3390/antibiotics9050229
Makita S., Maruyama D., Tobinai K. (2020). Safety and efficacy of Brentuximab Vedotin in the treatment of classic Hodgkin lymphoma. Onco Targets Ther. 13, 5993–6009. doi: 10.2147/OTT.S193951
Martins C., Dreij K., Costa P. M. (2019). The state-of-the art of environmental toxicogenomics: Challenges and perspectives of “omics” approaches directed to toxicant mixtures. Int. J. Environ. Res. Public Health 16, 4718. doi: 10.3390/ijerph16234718
Martins A., Vieira H., Gaspar H., Santos S. (2014). Marketed marine natural products in the pharmaceutical and cosmeceutical industries: tips for success. Mar. Drugs 12, 1066–1101. doi: 10.3390/md12021066
McCarthy D. J., Chen Y., Smyth G. K. (2012). Differential expression analysis of multifactor RNA-Seq experiments with respect to biological variation. Nucleic Acids Res. 40, 4288–4297. doi: 10.1093/nar/gks042
Minkiewicz P., Iwaniak A., Darewicz M. (2019). BIOPEP-UWM database of bioactive peptides: current opportunities. Int. J. Mol. Sci. 20, 5978. doi: 10.3390/ijms20235978
Modica M. V., Lombardo F., Franchini P., Oliverio M. (2015). The venomous cocktail of the vampire snail Colubraria reticulata (Mollusca, Gastropoda). BMC Genomics 16, 441. doi: 10.1186/s12864–015-1648–4
Molinski T. F., Dalisay D. S., Lievens S. L., Saludes J. P. (2009). Drug development from marine natural products. Nat. Rev. Drug Discovery 8, 69–85. doi: 10.1038/nrd2487
Montaser R., Luesch H. (2011). Marine natural products: a new wave of drugs? Future Med. Chem. 3, 1475–1489. doi: 10.4155/fmc.11.118
Mooney C., Haslam N. J., Pollastri G., Shields D. C. (2012). Towards the improved discovery and design of functional peptides: common features of diverse classes permit generalized prediction of bioactivity. PloS One 7, e45012. doi: 10.1371/journal.pone.0045012
Moutinho Cabral I., Madeira C., Grosso A. R., Costa P. M. (2022). A drug discovery approach based on comparative transcriptomics between two toxin-secreting marine annelids: Glycera alba and Hediste diversicolor. Mol. Omics 18, 731–744. doi: 10.1039/d2mo00138a
Murano C., Zuccarotto A., Leone S., Sollitto M., Gerdol M., Castellano I., et al. (2022). A survey on the distribution of ovothiol and ovoa gene expression in different tissues and cells: A comparative analysis in sea urchins and mussels. Mar. Drugs 20, 268. doi: 10.3390/md20040268
Nelsen D. R., Nisani Z., Cooper A. M., Fox G. A., Gren E. C. K., Corbit A. G., et al. (2014). Poisons, toxungens, and venoms: redefining and classifying toxic biological secretions and the organisms that employ them. Biol. Rev. Camb. Philos. Soc 89, 450–465. doi: 10.1111/brv.12062
O’Leary N. A., Wright M. W., Brister J. R., Ciufo S., Haddad D., McVeigh R., et al. (2016). Reference sequence (RefSeq) database at NCBI: Current status, taxonomic expansion, and functional annotation. Nucleic Acids Res. 44, D733–D745. doi: 10.1093/nar/gkv1189
Olivera B. M., Cruz L. J., de Santos V., LeCheminant G. W., Griffin D., Zeikus R., et al. (1987). Neuronal calcium channel antagonists. Discrimination between calcium channel subtypes using omega-conotoxin from Conus magus venom. Biochemistry 26, 2086–2090. doi: 10.1021/bi00382a004
Omaga C. A., Carpio L. D., Imperial J. S., Daly N. L., Gajewiak J., Flores M. S., et al. (2017). Structure and biological activity of a turripeptide from Unedogemmula bisaya venom. Biochemistry 56, 6051–6060. doi: 10.1021/acs.biochem.7b00485
Patro R., Duggal G., Love M. I., Irizarry R. A., Kingsford C. (2017). Salmon provides fast and bias-aware quantification of transcript expression. Nat. Methods 14, 417–419. doi: 10.1038/nmeth.4197
Paysan-Lafosse T., Blum M., Chuguransky S., Grego T., Pinto B. L., Salazar G. A., et al. (2023). InterPro in 2022. Nucleic Acids Res. 51, D418–D427. doi: 10.1093/nar/gkac993
Pennington M. W., Czerwinski A., Norton R. S. (2018). Peptide therapeutics from venom: current status and potential. Bioorg. Med. Chem. 26, 2738–2758. doi: 10.1016/j.bmc.2017.09.029
Pérez-Polo S., Imran M. A. S., Dios S., Pérez J., Barros L., Carrera M., et al. (2023). Identifying natural bioactive peptides from the common octopus (Octopus vulgaris Cuvier 1797) skin mucus by-products using proteogenomic analysis. Int. J. Mol. Sci. 24, 7145. doi: 10.3390/ijms24087145
Perkins D. N., Pappin D. J., Creasy D. M., Cottrell J. S. (1999). Probability-based protein identification by searching sequence databases using mass spectrometry data. Electrophoresis 20, 3551–3567. doi: 10.1002/(SICI)1522–2683(19991201)20:18<3551::AID-ELPS3551>3.0.CO;2–2
Pettersen E. F., Goddard T. D., Huang C. C., Couch G. S., Greenblatt D. M., Meng E. C., et al. (2004). UCSF Chimera-a visualization system for exploratory research and analysis. J. Comput. Chem. 25, 1605–1612. doi: 10.1002/jcc.20084
Pettit G. R., Kamano Y., Herald C. L., Tuinman A. A., Boettner F. E., Kizu H., et al. (1987). The isolation and structure of a remarkable marine animal antineoplastic constituent: dolastatin 10. J. Am. Chem. Soc. 109, 6883–6885. doi: 10.1021/ja00256a070
Pirtskhalava M., Amstrong A. A., Grigolava M., Chubinidze M., Alimbarashvili E., Vishnepolsky B., et al. (2021). DBAASP v3: Database of antimicrobial/cytotoxic activity and structure of peptides as a resource for development of new therapeutics. Nucleic Acids Res. 49, D288–D297. doi: 10.1093/nar/gkaa991
Ramírez-Carreto S., Vera-Estrella R., Portillo-Bobadilla T., Licea-Navarro A., Bernaldez-Sarabia J., Rudiño-Piñera E., et al. (2019). Transcriptomic and proteomic analysis of the tentacles and mucus of Anthopleura dowii Verril. Mar. Drugs 17, 436. doi: 10.3390/md17080436
Rana S. B., Zadlock F. J., Zhang Z., Murphy W. R., Bentivegna C. S. (2016). Comparison of de novo transcriptome assemblers and k-mer strategies using the killifish, Fundulus heteroclitus. PloS One 11, e0153104. doi: 10.1371/journal.pone.0153104
Ritchie M. E., Phipson B., Wu D., Hu Y., Law C. W., Shi W., et al. (2015). limma powers differential expression analyses for RNA-sequencing and microarray studies. Nucleic Acids Res. 43, e47. doi: 10.1093/nar/gkv007
Rivera-de-Torre E., Rimbault C., Jenkins T. P., Sørensen C. V., Damsbo A., Saez N. J., et al. (2022). Strategies for heterologous expression, synthesis, and purification of animal venom toxins. Front. Bioeng. Biotechnol. 9. doi: 10.3389/fbioe.2021.811905
Robinson M. D., McCarthy D. J., Smyth G. K. (2010). edgeR: a Bioconductor package for differential expression analysis of digital gene expression data. Bioinformatics 26, 139–140. doi: 10.1093/bioinformatics/btp616
Rodrigo A. P., Costa P. M. (2019). The hidden biotechnological potential of marine invertebrates: The Polychaeta case study. Environ. Res. 173, 270–280. doi: 10.1016/j.envres.2019.03.048
Rodrigo A. P., Grosso A. R., Baptista P. V., Fernandes A. R., Costa P. M. (2021a). A transcriptomic approach to the recruitment of venom proteins in a marine annelid. Toxins (Basel) 13, 97. doi: 10.3390/toxins13020097
Rodrigo A. P., Lopes A., Pereira R., Anjo S. I., Manadas B., Grosso A. R., et al. (2022). Endogenous fluorescent proteins in the mucus of an intertidal Polychaeta: Clues for biotechnology. Mar. Drugs 20, 224. doi: 10.3390/md20040224
Rodrigo A. P., Mendes V. M., Manadas B., Grosso A. R., Alves de Matos A. P., Baptista P. V., et al. (2021b). Specific antiproliferative properties of proteinaceous toxin secretions from the marine annelid. Mar. Drugs 19, 31. doi: 10.3390/md19010031
Rodrigo A. P., Moutinho Cabral I., Alexandre A., Costa P. M. (2024). Exploration of toxins from a marine annelid: an analysis of phyllotoxins and accompanying bioactives. Anim. (Basel) 14, 635. doi: 10.3390/ani14040635
Ronquist F., Teslenko M., van der Mark P., Ayres D. L., Darling A., Höhna S., et al. (2012). MrBayes 3.2: Efficient Bayesian phylogenetic inference and model choice across a large model space. Syst. Biol. 61, 539–542. doi: 10.1093/sysbio/sys029
Ruder T., Sunagar K., Undheim E. A., Ali S. A., Wai T. C., Low D. H., et al. (2013). Molecular phylogeny and evolution of the proteins encoded by coleoid (cuttlefish, octopus, and squid) posterior venom glands. J. Mol. Evol. 76, 192–204. doi: 10.1007/s00239–013-9552–5
Shannon P., Markiel A., Ozier O., Baliga N. S., Wang J. T., Ramage D., et al. (2003). Cytoscape: A software environment for integrated models of biomolecular interaction networks. Genome Res. 13, 2498–2504. doi: 10.1101/gr.1239303
Shen G. S., Layer R. T., McCabe R. T. (2000). Conopeptides: from deadly venoms to novel therapeutics. Drug Discovery Today 5, 98–106. doi: 10.1016/s1359–6446(99)01454–3
Shilov I. V., Seymour S. L., Patel A. A., Loboda A., Tang W. H., Keating S. P., et al. (2007). The Paragon Algorithm, a next generation search engine that uses sequence temperature values and feature probabilities to identify peptides from tandem mass spectra. Mol. Cell Proteomics 6, 1638–1655. doi: 10.1074/mcp.T600050-MCP200
Slattery M., Ankisetty S., Corrales J., Marsh-Hunkin K. E., Gochfeld D. J., Willett K. L., et al. (2012). Marine proteomics: A critical assessment of an emerging technology. J. Nat. Prod. 75, 1833–1877. doi: 10.1021/np300366a
Smith J. J., Undheim E. A. B. (2018). True lies: Using proteomics to assess the accuracy of transcriptome-based venomics in centipedes uncovers false positives and reveals startling intraspecific variation in Scolopendra Subspinipes. Toxins (Basel) 10, 96. doi: 10.3390/toxins10030096
Stark R., Grzelak M., Hadfield J. (2019). RNA sequencing: the teenage years. Nat. Rev. Genet. 20, 631–656. doi: 10.1038/s41576–019-0150–2
Szklarczyk D., Kirsch R., Koutrouli M., Nastou K., Mehryary F., Hachilif R., et al. (2023). The STRING database in 2023: protein-protein association networks and functional enrichment analyses for any sequenced genome of interest. Nucleic Acids Res. 51, D638–D646. doi: 10.1093/nar/gkac1000
Tarcha E. J., Olsen C. M., Probst P., Peckham D., Muñoz-Elías E. J., Kruger J. G., et al. (2017). Safety and pharmacodynamics of dalazatide, a Kv1.3 channel inhibitor, in the treatment of plaque psoriasis: A randomized phase 1b trial. PloS One 12, e0180762. doi: 10.1371/journal.pone.0180762
Teufel F., Almagro Armenteros J. J., Johansen A. R., Gíslason M. H., Pihl S. I., Tsirigos K. D., et al. (2022). SignalP 6.0 predicts all five types of signal peptides using protein language models. Nat. Biotechnol. 40, 1023–1025. doi: 10.1038/s41587–021-01156–3
The Gene Ontology Consortium (2021). The Gene Ontology resource: Enriching a GOld mine. Nucleic Acids Res. 49, D325–D334. doi: 10.1093/nar/gkaa1113
The Uniprot Consortium (2023). UniProt: The universal protein knowledgebase in 2023. Nucleic Acids Res. 51, D523–D531. doi: 10.1093/nar/gkac1052
Thomas S., Karnik S., Barai R. S., Jayaraman V. K., Idicula-Thomas S. (2010). CAMP: a useful resource for research on antimicrobial peptides. Nucleic Acids Res. 38, D774–D780. doi: 10.1093/nar/gkp1021
Turner A., Kaas Q., Craik D. J. (2020). Hormone-like conopeptides - new tools for pharmaceutical design. RSC Med. Chem. 11, 1235–1251. doi: 10.1039/d0md00173b
Urbarova I., Karlsen B. O., Okkenhaug S., Seternes O. M., Johansen S. D., Emblem Å. (2012). Digital marine bioprospecting: mining new neurotoxin drug candidates from the transcriptomes of cold-water sea anemones. Mar. Drugs 10, 2265–2279. doi: 10.3390/md10102265
Urdiales J. L., Morata P., Núñez De Castro I., Sánchez-Jiménez F. (1996). Antiproliferative effect of dehydrodidemnin B (DDB), a depsipeptide isolated from Mediterranean tunicates. Cancer Lett. 102, 31–37. doi: 10.1016/0304–3835(96)04151–1
Verdes A., Anand P., Gorson J., Jannetti S., Kelly P., Leffler A., et al. (2016). From mollusks to medicine: A venomics approach for the discovery and characterization of therapeutics from Terebridae peptide toxins. Toxins (Basel) 8, 117. doi: 10.3390/toxins8040117
Verdes A., Simpson D., Holford M. (2018). Are fireworms venomous? Evidence for the convergent evolution of toxin homologs in three species of fireworms (Annelida, Amphinomidae). Genome Biol. Evol. 10, 249–268. doi: 10.1093/gbe/evx279
Vieira H., Leal M. C., Calado R. (2020). Fifty shades of blue: How Blue Biotechnology is shaping the bioeconomy. Trends Biotechnol. 38, 940–943. doi: 10.1016/j.tibtech.2020.03.011
Violette A., Biass D., Dutertre S., Koua D., Piquemal D., Pierrat F., et al. (2012). Large-scale discovery of conopeptides and conoproteins in the injectable venom of a fish-hunting cone snail using a combined proteomic and transcriptomic approach. J. Proteomics 75, 5215–5225. doi: 10.1016/j.jprot.2012.06.001
von Reumont B. M. (2018). Studying smaller and neglected organisms in modern evolutionary venomics implementing RNASeq (transcriptomics) - A critical guide. Toxins (Basel) 10, 292. doi: 10.3390/toxins10070292
von Reumont B. M., Anderluh G., Antunes A., Ayvazyan N., Beis D., Caliskan F., et al. (2022). Modern venomics - Current insights, novel methods, and future perspectives in biological and applied animal venom research. Gigascience 11, giac048. doi: 10.1093/gigascience/giac048
von Reumont B. M., Campbell L. I., Richter S., Hering L., Sykes D., Hetmank J., et al. (2014). A polychaete’s powerful punch: Venom gland transcriptomics of Glycera reveals a complex cocktail of toxin homologs. Genome Biol. Evol. 6, 2406–2423. doi: 10.1093/gbe/evu190
von Reumont B. M., Lüddecke T., Timm T., Lochnit G., Vilcinskas A., von Döhren J., et al. (2020). Proteo-transcriptomic analysis identifies potential novel toxins secreted by the predatory, prey-piercing ribbon worm. Mar. Drugs 18, 407. doi: 10.3390/md18080407
Walker A. A., Robinson S. D., Hamilton B. F., Undheim E. A. B., King G. F. (2020). Deadly proteomes: A practical guide to proteotranscriptomics of animal venoms. Proteomics 20, 1900324. doi: 10.1002/pmic.201900324
Wang Z., Gerstein M., Snyder M. (2009). RNA-Seq: A revolutionary tool for transcriptomics. Nat. Rev. Genet. 10, 57–63. doi: 10.1038/nrg2484
Wang J., Yazdani S., Han A., Schapira M. (2020). Structure-based view of the druggable genome. Drug Discovery Today 25, 561–567. doi: 10.1016/j.drudis.2020.02.006
Waterhouse A., Bertoni M., Bienert S., Studer G., Tauriello G., Gumienny R., et al. (2018). SWISS-MODEL: Homology modelling of protein structures and complexes. Nucleic Acids Res. 46, W296–W303. doi: 10.1093/nar/gky427
White K. M., Rosales R., Yildiz S., Kehrer T., Miorin L., Moreno E., et al. (2021). Plitidepsin has potent preclinical efficacy against SARS-CoV-2 by targeting the host protein eEF1A. Science 371, 926–931. doi: 10.1126/science.abf4058
Whitelaw B. L., Strugnell J. M., Faou P., da Fonseca R. R., Hall N. E., Norman M., et al. (2016). Combined transcriptomic and proteomic analysis of the posterior salivary gland from the southern blue-ringed octopus and the southern sand octopus. J. Proteome Res. 15, 3284–3297. doi: 10.1021/acs.jproteome.6b00452
Williams J. A., Day M., Heavner J. E. (2008). Ziconotide: an update and review. Expert Opin. Pharmacother. 9, 1575–1583. doi: 10.1517/14656566.9.9.1575
wwPDB consortium (2019). Protein Data Bank: the single global archive for 3D macromolecular structure data. Nucleic Acids Res. 47, D520–D528. doi: 10.1093/nar/gky949
Yuan D., Ahamed A., Burgin J., Cummins C., Devraj R., Gueye K., et al. (2024). The European nucleotide archive in 2023. Nucleic Acids Res. 52, D92–D97. doi: 10.1093/nar/gkad1067
Keywords: marine biotechnology, blue growth, next-generation sequencing, computational biology, bioinformatics, bioproducts, proteins, marine invertebrates
Citation: Moutinho Cabral I, Gonçalves C, Grosso AR and Costa PM (2024) Bioprospecting and marine ‘omics’: surfing the deep blue sea for novel bioactive proteins and peptides. Front. Mar. Sci. 11:1362697. doi: 10.3389/fmars.2024.1362697
Received: 28 December 2023; Accepted: 20 May 2024;
Published: 04 June 2024.
Edited by:
Simon Jungblut, University of Bremen, GermanyReviewed by:
Camino Gestal, Spanish National Research Council (CSIC), SpainFederica Di Costanzo, Anton Dohrn Zoological Station Naples, Italy
Copyright © 2024 Moutinho Cabral, Gonçalves, Grosso and Costa. This is an open-access article distributed under the terms of the Creative Commons Attribution License (CC BY). The use, distribution or reproduction in other forums is permitted, provided the original author(s) and the copyright owner(s) are credited and that the original publication in this journal is cited, in accordance with accepted academic practice. No use, distribution or reproduction is permitted which does not comply with these terms.
*Correspondence: Inês Moutinho Cabral, imf.cabral@campus.fct.unl.pt; Pedro M. Costa, pmcosta@fct.unl.pt