- 1School of Biological Sciences, Georgia Institute of Technology, Atlanta, GA, United States
- 2Department of Biological Oceanography, Leibniz Institute for Baltic Sea Research Warnemünde, Rostock, Germany
- 3Lamont-Doherty Earth Observatory, Columbia University, Palisades, NY, United States
- 4Estuary and Ocean Science Center, San Francisco State University, Tiburon, CA, United States
The Western Tropical North Atlantic is a highly dynamic marine system where the Amazon River Plume (ARP) generates a patchwork of environmental conditions that favor different phytoplankton groups. To study phytoplanktonic community structure in such heterogeneous conditions, we used a set of five standard ship-based measurements taken from oceanographic surveys between 2010 and 2021 to characterize different habitat types. We then utilized a variety of multiparametric approaches to examine phytoplankton biodiversity in the different habitats to assess the biological relevance of our delineated habitats. Our approach generated a consistent set of habitat types across cruises carried out in multiple different years and the Amazon’s two predominant (wet and dry) seasons. Our phytoplankton community analyses revealed strong distinctions among all habitats along the plume gradient using in-vivo fluorescence and diagnostic pigments, and clear contrasts of diazotroph community along the mesohaline waters using direct cell-count, a pattern consistent with niche partitioning among similar species. The few apparent mismatches we found between phytoplankton community composition and habitat may reflect recent hydrographic changes driven by mixing and/or upwelling and thus may be a useful index to biologically-relevant temporal variation. Our habitat classification approach is straightforward and broadly applicable in identifying biologically distinct areas within heterogeneous and dynamic regions of the ocean.
Introduction
The Amazon River Plume (ARP) deposits nearly 20% of global freshwater discharge into the Western Tropical North Atlantic Ocean (WTNA) at an average annual rate of 120,000 m3/s and extends more than 1,800 km from the mouth (Subramaniam et al., 2008; Goes et al., 2014). From winter to peak discharge during spring, the ARP is mainly transported northward along the eastern coast of South America by the North Brazil Current (NBC). During summer through fall, as Amazon River discharge is weakening, the ARP is entrained by the North Equatorial Countercurrent (NECC) and travels eastward (Coles et al., 2013). Fed by flow through the world’s largest river basin, the ARP carries with it large amounts of terrestrially derived sediments, nutrients, as well as particulate and dissolved organic matter (Nittrouer et al., 1986; Del Vecchio and Subramaniam, 2004). During its progression to the open ocean, the ARP gradually mixes with adjacent coastal and offshore waters, generating large gradients of temperature, salinity, turbidity, and nutrient availability in the WTNA (DeMaster et al., 1996; DeMaster and Aller, 2001; Coles et al., 2013). Due to variations in flow direction, mixing, spatial extent, and mesoscale circulation, the ARP creates diverse and dynamic distributions of ecological niches in the water column.
The distribution of physical and chemical properties in the ocean water column has long been recognized as a major factor shaping the community composition of marine plankton (Sverdrup et al., 1942; Platt et al., 1991; Weber et al., 2019), calling for methods to define the different ecological niches observed. Based on physical forcings and seasonal cycles of primary production, Longhurst (1998) partitioned the upper ocean into several biogeographic provinces, providing a general framework for understanding the ocean at large regional scales, including studies of the export of carbon dioxide to the deep ocean (Honjo et al., 2008), and spatial distributions of various marine species at different trophic levels, ranging from bacteria (Li et al., 2004) to plankton (Beaugrand et al., 2002; Woodd-Walker et al., 2002; Alvain et al., 2005) to pelagic fishes (Corbineau et al., 2008; Reygondeau et al., 2012). Nevertheless, Longhurst’s scheme is often hard to implement in systems with fluctuating physical and chemical conditions and forcings over varying spatial and temporal scales. Yet, mesoscale and submesoscale forcings, which either act as hydrographical boundaries or provide nutrient injections, can shape the planktonic community structure in some of the most productive and diverse ocean regions, including coastal areas, upwelling systems, and eddies (Lévy et al., 2001; Baltar and Aristegui, 2017; Raes et al., 2018; Hernández-Hernández et al., 2020).
Physical tracers such as salinity or temperature are often used to overcome this issue and characterize the spatial distribution of biochemical processes in dynamic regions such as estuaries. Although this is a robust approach for describing water masses and the impact of mixing, single or double tracers like salinity and temperature alone cannot fully capture the complex interactions and processes that constrain primary production and phytoplankton diversity. For example, phytoplankton biomass is typically higher within the ARP (defined as water with salinity below 35 psu) than in the surrounding oceanic waters as a result of the nutrients and buoyancy discharged by the river. However, large accumulations of diatoms have been found in more saline waters far from the river mouth, where turbidity is low enough to alleviate light limitation for photosynthesis (Smith and Russell, 1995; Smith Jr and Demaster, 1996). Additionally, the phytoplankton community structure can also drive and be driven by the biogeochemical gradient along the aging plume (Goes et al., 2014; Stukel et al., 2014; Weber et al., 2017).
To capture the biological impacts of variable biogeochemical conditions, a robust method has been developed to delineate regions into distinct phytoplankton habitats using fundamental oceanographic measurements (Weber et al., 2019). This approach includes the influence of upper water column properties and extends Longhurst’s biogeochemical province concept to the smaller scale of highly variable marine environments. The approach of Weber et al. (2019) has provided insight into the potential drivers shaping phytoplankton distributions in different river-ocean systems like the Amazon and Mekong Rivers (Weber et al., 2019; Charvet et al., 2021). While previous studies have examined differences in phytoplankton communities in habitats across the ARP continuum during single expeditions, a comparison of habitats and their biological relevance across larger temporal scales is lacking.
Here, we investigate phytoplankton community structure in the context of previously defined habitats of the ARP over a multi-year span. We hypothesized that: (1) the properties of habitat types previously described are consistent across time, and (2) planktonic community structure varies significantly and consistently among habitat types.
Methods
We performed a hierarchical cluster analysis (HCA) parallel to a principal component analysis (PCA) to study a set of plankton habitats in the Amazon Plume region. Our habitat-defining variables included sea surface temperature (SST), sea surface salinity (SSS), mixed layer depth (depth of buoyancy frequency maximum, MLD), the depth of the subsurface chlorophyll maximum (ChlMD), and a nitrate availability index (NAI) as proposed by Weber et al. (2019). All surface variables in this study were defined as the nearest measurement to a five-meter reference depth. NAI is defined as:
Where [NO2/3] is the total concentration of measured nitrate and nitrite, Z is the depth of the water column, positive downward. The threshold surface concentration of nitrate and nitrite for calculation of NAI was set at 0.5 µM as it is comparable to the half-saturation constant KS range for nitrogen uptake of coastal and oceanic phytoplankton and would support considerable rate of nitrate assimilation (Eppley et al., 1969). In case the surface [NO2/3] does not reach the threshold value, we would look for the depth where [NO2/3] reaches 2 µM as in Weber et al. (2019).
Sample collection
Biological and chemical data were collected during six oceanographic cruises conducted in the Amazon River plume region from 2010 to 2021 (Table 1; Supplementary Figure 1). On all cruises, a Conductivity-Temperature-Depth (CTD) Rosette system equipped with a fluorometer was used to measure hydrographic properties in the water column Samples for measurement of nitrite (NO2-) and nitrate (NO3-) concentration across the water column were collected using Niskin-type bottles mounted on the CTD rosette and analyzed at sea and reanalyzed as needed at the Georgia Institute of Technology (Atlanta, USA) using a Lachat® QuikChem 8000 flow-injection analysis system for the first three cruises and a Lachat® QuikChem 8500 series 2 for cruises EN614 and EN640 (Knap et al., 1996). For cruise M174, nutrient analyses were conducted at sea using a Seal Analytical QuAAtro 39 continuous flow analyzer. Detailed calibration and processing protocol of CTD and bottle data for each specific cruise can be found from their corresponding source on the Biological and Chemical Oceanography Data Management Office (BCO-DMO) and Data Publisher for Earth & Environmental Science servers (See Data Availability). On each cruise, every CTD cast with a measured nutrient profile was included in our habitat type analysis (Table 1). Each cast was identified using a “cruise_station.event” code, beginning with the cruise name, followed by the station number before the decimal, and the specific event number after the decimal.
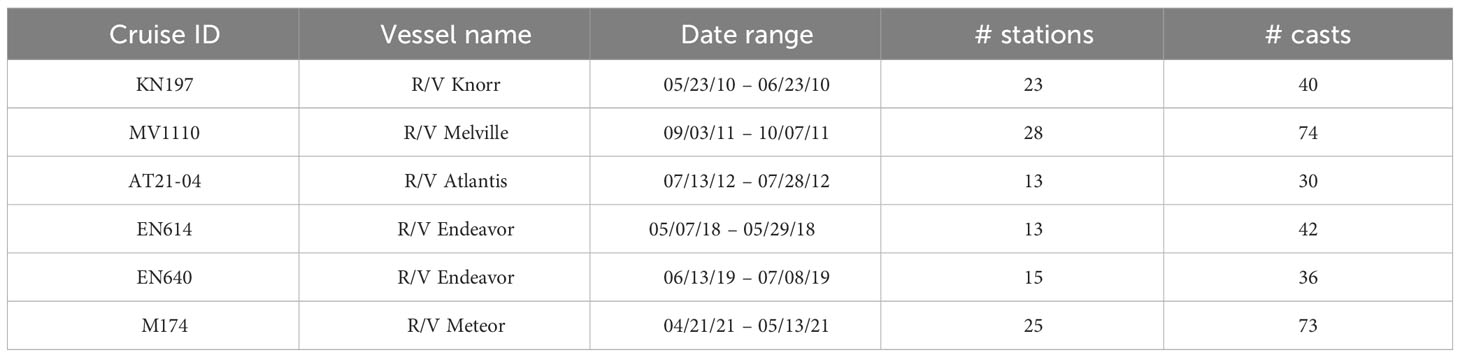
Table 1 List of oceanographic expeditions, vessel names, date ranges, and the total number of stations and casts for each cruise.
On cruises EN614, EN640, and M174, surface phytoplankton pigments at five-meter depth were quantified for assessment of the surface phytoplankton community structure (Table 2). Phytoplankton pigments were determined and analyzed either via Advanced Laser Fluorometric (ALF) analysis as described in Chekalyuk and Hafez (2008) or via high-performance liquid chromatography (HPLC) as described in Van Heukelem and Thomas (2001).
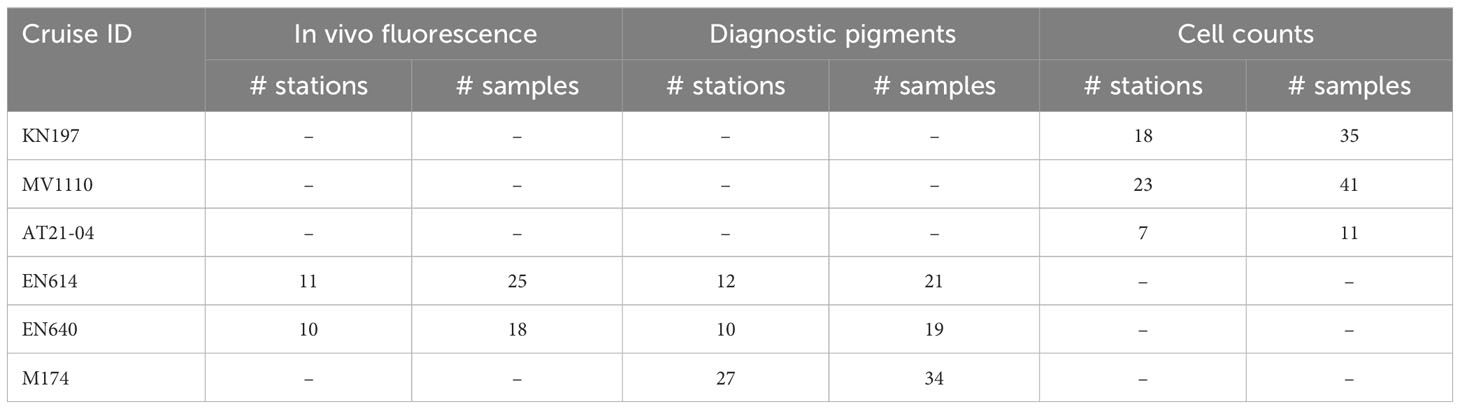
Table 2 Sample size of in vivo fluorescence, diagnostic pigments, and diazotroph cell counts used in phytoplankton diversity analysis.
Samples for ALF analysis were collected in 500 ml amber glass bottles and stored in the dark under cold temperatures to reduce non-photochemical quenching effects before analysis. Fluorescence signals were measured using the Custom Laser Analytical Spectroscopic Systems (Chekalyuk and Hafez, 2013). The Raman-normalized measurements of phytoplankton variable fluorescence (Fv/Fm), chlorophyll stimulated by a blue laser (679 nm), and three phycoerythrin (PE) fluorescence signatures stimulated by a green laser were used to quantify different groups of PE-containing phytoplankton (Chekalyuk and Hafez, 2008). These include PE-1 (565 nm) found in blue water oligotrophic cyanobacteria, PE-2 (578 nm) found in green water cyanobacteria thriving in coastal mesohaline waters, and PE-3 (590 nm) found in eukaryotic photoautotrophic cryptophytes that are abundant in coastal, estuarine, and enclosed bay waters (Chekalyuk and Hafez, 2008; Goes et al., 2014).
For HPLC analysis, we filtered one to two liters of surface seawater collected from the CTD rosette through a GF/F filter under gentle vacuum. Filters were frozen in liquid nitrogen for later analysis using an Agilent RR1200 Series Gradient system. Seven diagnostic pigments from the HPLC analysis were analyzed and their taxonomic significances at different levels were presented in Table 3, following Vidussi et al. (2001) and Wright et al. (2005) and references therein. Each diagnostic pigment was normalized by dividing for the total chlorophyll concentration measured for each cast. Detailed calibration and processing of both ALF and HPLC data can also be found on the BCO-DMO server (Data Availability).
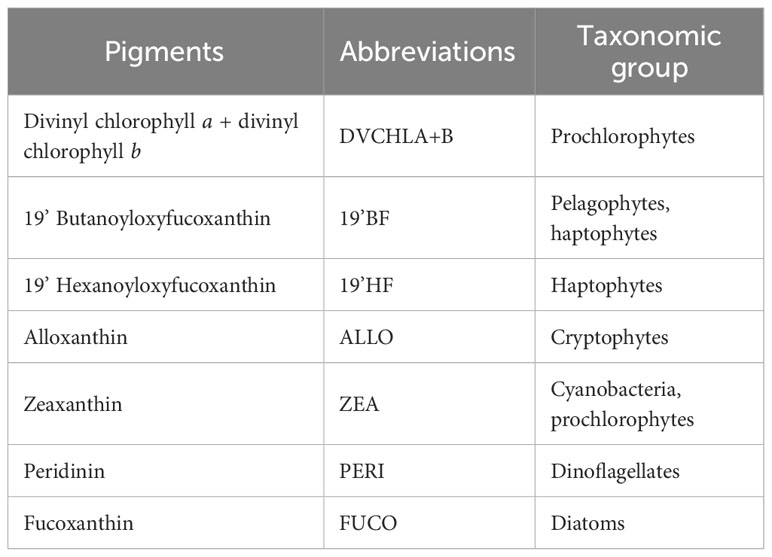
Table 3 Diagnostic pigments used in the study of phytoplankton community structures and their associated taxonomic group.
We also used direct microscopic counts to assess surface diazotrophic community structure on cruises KN197, MV1110, and AT21-04 (Table 2). Samples were collected directly from distinct depths in the upper 10 m of the water column using the shipboard CTD-rosette system. Ten liters of seawater (one full sampling bottle) were filtered through an 8 µm pore size, 47 mm diameter Nuclepore® membrane filter in an inline filter cartridge (Carpenter et al., 1999). The filter was examined and enumerated under 400x magnification using a Zeiss® Axioskop Epifluorescence microscope. Colonies and single trichomes of Trichodesmium spp., as well as Richelia spp. found in diatom-diazotroph associations (DDAs), were identified via phycoerythrin and chlorophyll excitation under green (510-560 nm) and blue (450-490 nm) illumination, respectively. The diatoms associated with Richelia spp. were identified as either Hemiaulus or Rhizosolenia spp. based on cell morphology observed using bright-field optics (Carpenter et al., 1999).
Statistical approach
For our habitat type classification, we performed HCAs and PCAs on correlations of the standardized habitat-defining variables using JMP Pro 16 software (SAS Institute Inc., Cary, USA). Distance and gap statistics were examined to select the optimal number of clusters for the HCAs. We applied this routine on the entire dataset of all six cruises and on individual cruise datasets to test for the consistency of our approach. Shapiro-Wilk and Levene’s tests were conducted for each variable to evaluate normality and homogeneity of data, respectively. Since variables are generally heterogenous and slightly non-normally distributed (Supplementary Tables 1 and 2), we conducted Welch’s ANOVA to compare the means of each variable across our habitat types. Since all variables showed significant different among the habitat types (Supplementary Table 3), we performed Games-Howell post hoc test for pairwise comparisons of the variables (Supplementary Table 4). We also conducted Wech’s ANOVA and Games-Howell post hoc test for mean comparison of each variable in each habitat type across our cruises (Supplementary Table 5). The statistical analyses were done in R using RStudio (Team, 2013).
Phytoplankton community structure/beta diversity
To test for the biological significance of the newly assigned habitat types, we compared the upper water column phytoplankton communities in the different habitat types sampled during the six cruises. We used the R package “vegan” (Oksanen et al., 2020) to carry out community structure analyses. Dissimilarity matrices of community structure were calculated for all standardized datasets. Euclidian distances were generated on the chi-square standardized ALF signal and HPLC diagnostic pigment data, which are equivalent to chi-square distances used in correspondence analysis (Legendre and Gallagher, 2001). For cell counts, Bray-Curtis dissimilarities were calculated on log(x+1) standardized abundances. Permutational multivariate analysis of variance (PERMANOVA) was performed on the dissimilarities of all datasets to examine differences in phytoplankton community diversity among habitat types (Anderson, 2001).
Since PERMANOVA tests for cell counts, diagnostic pigments, and fluorescence all showed significant differences (p< 0.001, Supplementary Tables 6–8) among habitats, distance-based redundancy analyses (dbRDA) were conducted using the “capscale” function to further examine the phytoplankton beta diversity among different habitat types. DbRDA is a multivariate constrained ordination method to explore the relationships among biological assemblages of species and their environments (Legendre and Anderson, 1999). We examined the data structure by doing eigen-analyses of the biodiversity distance matrices fitted by the predictor variable, which is the habitat assignment in this case (Anderson et al., 2008). The Pearson’s correlation of the standardized phytoplankton measurements with their respective axes was used to identify the individual pigments, fluorescence signals, or phytoplankton species that accounted for the differences observed among habitats. ANOVA-like permutation tests based on 999 permutations were performed to assess the significance of the model for all analyses (Legendre et al., 2011). While PERMANOVA can test for the biological relevance of the superimposed habitat types on the overall observed variation in the phytoplankton data, dbRDA and its subsequent tests allow us to examine the influence of the habitat assignment on the overall variation and explore trends that would be masked by the high variability or high correlation in the unconstrained ordination. Since all tests were significant (p< 0.001, Supplementary Tables 9–11), we conducted subsequent pairwise permutation tests among all the habitat types in the dbRDA to further confirm their biological significance (Kindt and Coe, 2005).
Results
Multi-cruise analysis
The HCA on the habitat-defining variables of all six cruises generated a total of eight distinct habitat types that were also highlighted by the PCA, with each habitat having a unique combination of the habitat-defining variables (Figure 1). The clusters produced by our HCA of all six cruises included five habitat types previously defined by Weber et al. (2019): young plume core (YPC), old plume core (OPC), western plume margin (WPM), eastern plume margin (EPM), and oceanic water (OSW).
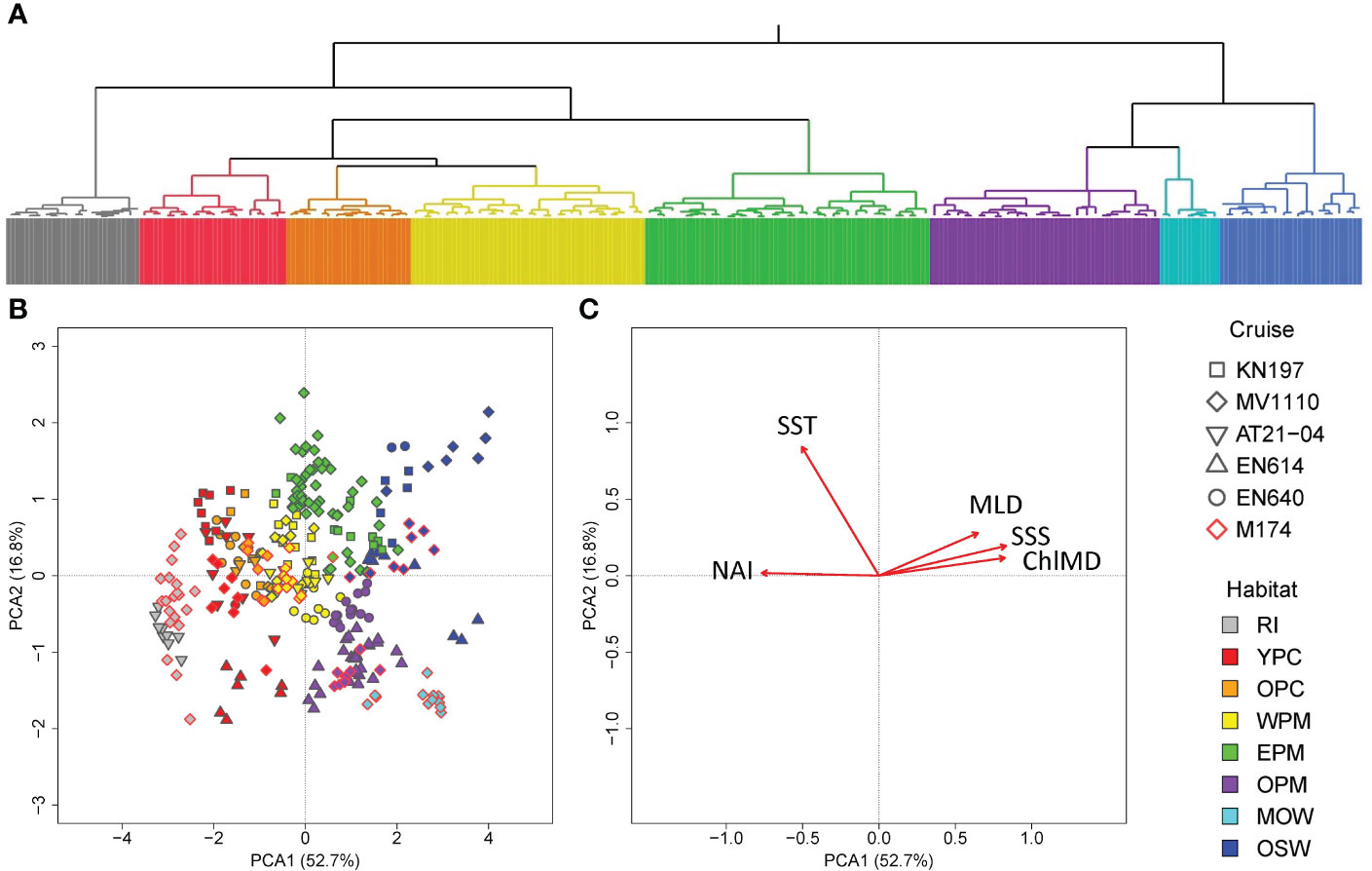
Figure 1 Wards-mean hierarchical cluster analysis (A) and principal component analysis (B, C) of CTD casts from six cruises conducted in the WTNA based on the habitat-defining variables of Weber et al. (Weber et al., 2019). (A) Dendrogram showing clustering of different groups, where colors correspond to habitat types. The detailed classification of each cast can be found in the supplementary. (B) Scatter plot of each cast’s score with respect to the first two principal components, where colors correspond to habitat types, and marker shapes correspond to cruises. (C) Loading plot showing the unrotated loading matrix of the analyzed habitat-defining variables as a function of the first two principal components. The closer the variable’s loading value is to 1 or -1, the greater the effect of the variable in driving that component. Habitat types are marked by color: riverine input (RI, gray), young plume core (YPC, red), old plume core (OPC, orange), western plume margin (WPM, yellow), eastern plume margin (EPM, green), outer plume margin (OPM, purple), modified oceanic water (MOW, cyan), and oceanic water (OSW, blue).
Many stations from our five other cruises fell into clusters representing the previously defined habitat types (Figure 1). One of our newly detected habitat types, namely riverine input, consisting of casts from cruises AT21-04 (n=9) and M174 (n=20) that formed a sister group with the plume-influenced waters (YPC, OPC, WPM, and EPM). Two clusters, one consisting of casts from cruises EN614 (n=27), EN640 (n=15), M174 (n=8), which we designated as outer plume margin (OPM), and one consisting of casts only from cruise M174 (n=13), which we designated as modified oceanic waters (MOW) both form sister groups with the OSW group. In summary, stations from our six cruises fall into a total of eight distinct habitat types, including the five habitats previously described by Weber et al. (2019) and three new categories that encompassed the riverine input (RI) and two habitats that fall between the plume influenced region and oceanic water (OPM and MOW).
The first two principal components of the PCA of all stations described a total of 79.5% of the overall variation (Figure 1). PC1 accounted for 52.7% of the total variation and was driven strongly by SSS, ChlMD, and NAI. SST was the main driver for PC2, which accounted for 16.8% of the total variation. The last defining variable used in our analysis, the MLD, mainly drove the third component (PC3), which accounted for 14.8% of the total variation (Supplementary Figure 2). In the plane defined by the first two principal component axes, the riverine input (RI), plume cores (YPC and OPC), plume margins (WPM, EPM, OPM), and oceanic waters (MOW and OSW) are arrayed along a continuum of habitat defining variables where NAI decreased as SSS, and ChlMD increased (Figure 1). We observed the same pattern on the plane of PC1 and PC3, with MLD clearly separating the oceanic waters from the plume-influenced waters (Supplementary Figure 2).
The RI habitat was distinguished by the lowest SSS although they shared the same range of MLD and ChlMD with the plume core habitats (Figure 2; Supplementary Table 12). The YPC and OPC casts, having similar SSS, mostly differed in NAI, with YPC having much higher values. Four variables (SST, MLD, ChlMD, and NAI) together drive the differences among the WPM, EPM, and OPM habitats. The MOW, having distinctly low SST and deep ChlMD, shared the same range of MLD with the plum margin habitats, and its SSS fall between that of plume margin and oceanic water. The high SSS and deep MLD distinguished the OSW from the rest of the water types.
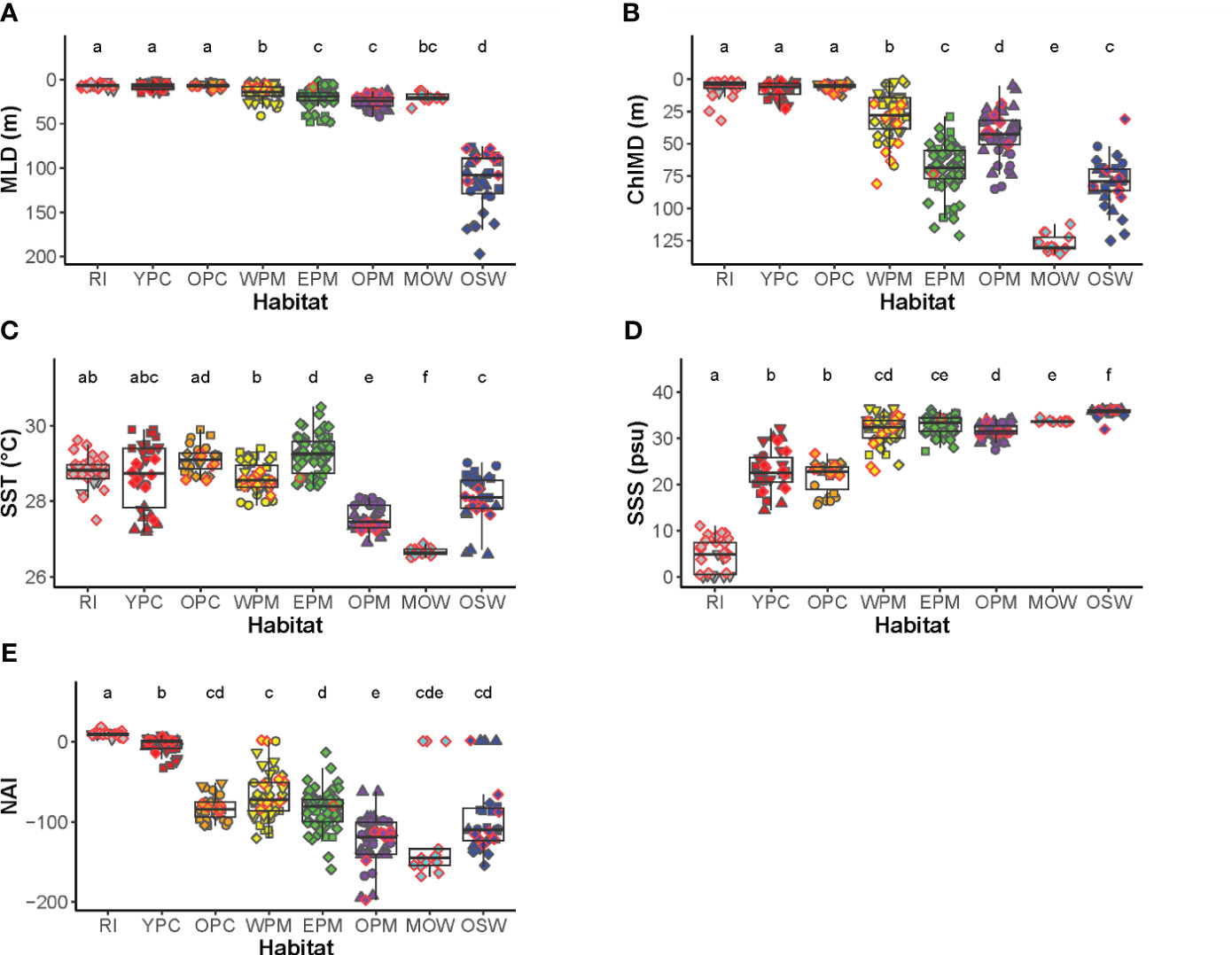
Figure 2 Habitat-defining variables of the seven studied habitat types: (A) mixed layer depth, (B) depth of maximum chlorophyll, (C) sea surface temperature, (D) sea surface salinity, (E) nitrate availability index. Each symbol represents a measurement from an individual cast. Symbol shapes and colors correspond to habitat types as in Figure 1. The boxes display the median, 25th and 75th percentiles. The upper and lower whiskers extend from the hinge to 1.5*IQR (inter-quartile range, the distance between the first and third quartiles). Data beyond the end of the whiskers are considered outliers. The letters above the whisker boxes display the Dunn’s pair-wise comparisons among the habitats (habitats with the same letter do not differ significantly).
We conducted Welch’s ANOVA to examine the differences in the habitat-defining variables among our eight habitat types and the six cruises. While we were not able to directly analyze interactions between our habitat types and the different cruises, we observed significant differences for all variables among our habitat types and among cruises for many of our habitats (Supplementary Tables 4 and 5).
Habitats and phytoplankton
To assess the biological relevance and consistency of the habitat assignment, we used in vivo fluorescence (ALF), measured during cruise EN614 and EN640, and diagnostic pigments (HPLC), also measured during the above two cruises plus cruise M174, to characterize the surface (5 m) phytoplankton communities and to explore the relationship between community composition and the habitat types. Since PERMANOVA analyses of both diagnostic pigments and fluorescence signals with 999 permutations returned p-values of 0.001 (Supplementary Tables 6 and 7), we further studied the pigment profiles using the dbRDA.
For in vivo fluorescence measured with the ALF, the dbRDA model fitted by our habitat assignment returned an R2 value of 0.51 and the permutation test returned a p-value of 0.001 (Supplementary Tables 9 and 10). The first two dbRDA axes explained 46.6% of the total observed variation and 90.5% of the variation when constrained by habitat types (Figure 3), with the first axis dbRDA1 alone accounting for 30.4% and 59% of the total and the constrained variation, and the second axis dbRDA2 accounted for 16.2% and 31.5% of the total and constrained variation. Both the pairwise PERMANOVA and the ANOVA-like comparison on the dbRDA showed significant differences among the plume core (YPC and OPC), plume margin (OPM), and oceanic water (OSW) phytoplanktonic communities (Supplementary Tables 6 and 9). On the dbRDA plane of the first two axes, the first axis, negatively correlated with chlorophyll, mainly separated the plume core (YPC and OPC) communities from the plume margin (WPM and OPM) communities. The second axis, negatively correlated with Fv/Fm, discriminated the oceanic water communities (OSW) from the rest. PE-1 correlated positively with both dbRDA1 and dbRDA2. PE-3 showed a weaker correlation in the same direction as PE-1. PE-2 showed negative correlation with the third axis dbRDA3, which contributed only 3.8% and 7.4% to the total and the constrained variation, mainly separating the YPC and some OPM stations from the rest (Supplementary Figure 3).
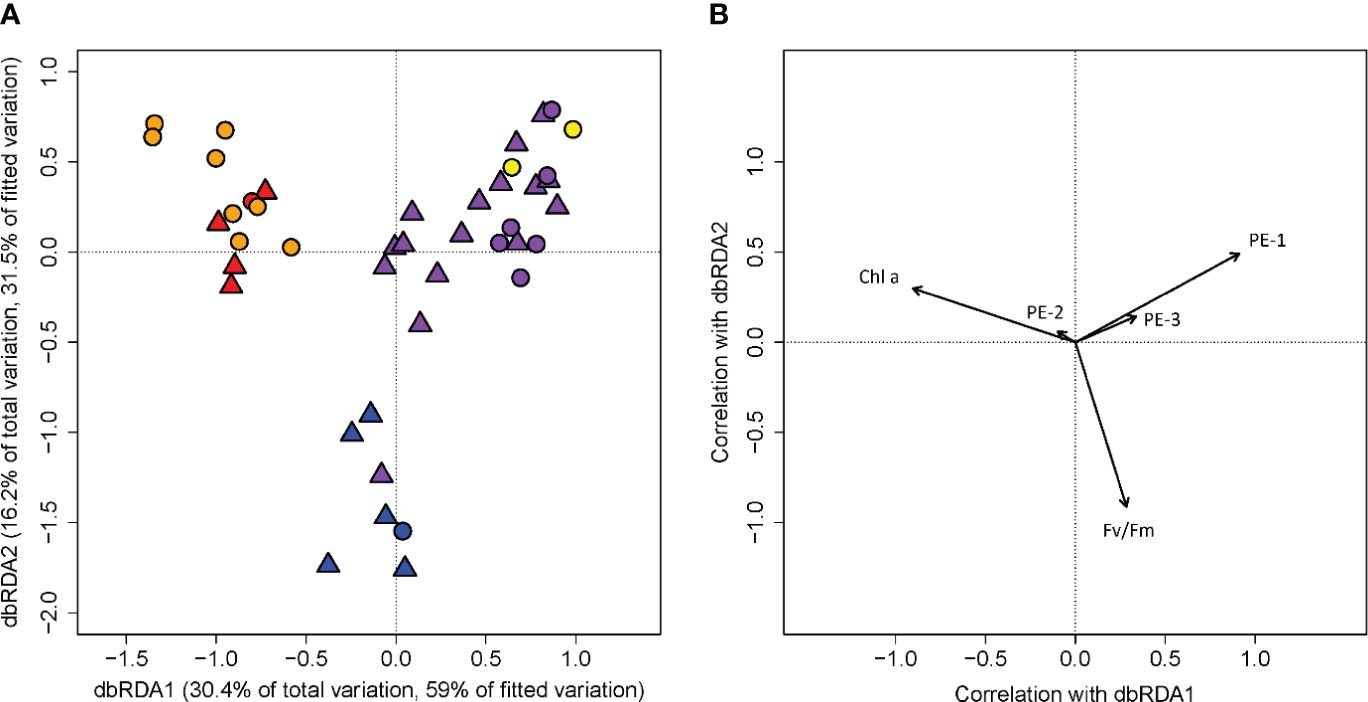
Figure 3 Distance-based redundancy analysis (dbRDA) of Advanced Laser Fluorometer (ALF) signal measurement from cruises EN614 and EN640 (R2 = 0.51, p=0.001). Panel (A) is a scatter plot of each measurement’s scores with respect to the first two axes of the dbRDA. Percentages of variation explained by dbRDA1 and dbRDA2 in the total observed variation and in the habitat-type fitted model are listed on their respective axes. Each marker corresponds to an ALF measurement from a single cast. Panel (B) is a biplot with arrows displaying the Pearson correlations of ALF measurements from cruises EN614 and EN640 with the first two dbRDA axes. The length and direction of the arrows indicates the correlation of fluorescence signal with the respective dbRDA axis. The fluorescence signatures include the normalized phytoplankton variable fluorescence (Fv/Fm), chlorophyll (Chl a), and three types of phycoerythrin (PE-1, PE-2, and PE-3). Marker shapes indicate the cruise each profile was collected as in Figure 1. Habitat types are marked by color: riverine input (RI, gray), young plume core (YPC, red), old plume core (OPC, orange), western plume margin (WPM, yellow), eastern plume margin (EPM, green), outer plume margin (OPM, purple), modified oceanic water (MOW, cyan), and oceanic water (OSW, blue).
The fitted dbRDA model of the diagnostic pigment ratio returned an R2 value of 0.55 with a p-value of 0.001 in the subsequent ANOVA-like permutation test. The first two dbRDA axes explained 45.5% of the total observed variation and 82.1% of the variation when constrained by habitat types (Figure 4). The first axis, dbRDA1, alone accounted for 32.2% and 58.1% of the total and the constrained variation, respectively. Zeaxanthin, divinyl chlorophyll a + divinyl chlorophyll b, 19’butanoyloxyfucoxanthin, and 19’ hexanoyloxyfucoxanthin all showed positive correlations with the first axis while fucoxanthin showed a negative correlation with the same axis. The second axis, dbRDA2, accounted for 13.3% and 24% of the total and constrained variation, respectively, and showed a positive correlation with alloxanthin. On the dbRDA plane of the first two components, the first axis mainly separated the riverine input (RI) and plume core (YPC and OPC) communities from the plume margin (WPM, EPM, OPM) and oceanic water (OSW) communities. The second axis distinguished most of the MOW from the rest of the habitat types. Peridinin showed a strong negative correlation with the third axis, which accounted for only 4.5% and 8.1% of the total and constrained variation, separating the riverine input and young plume core communities to some extent (Supplementary Figure 4). The pairwise PERMANOVA and permutation tests on the dbRDA confirmed the significant differences among the riverine input, young plume core, and modified oceanic water phytoplankton communities (Supplementary Tables 7 and 10). While it is difficult to observe on the dbRDA plot, the statistical tests also indicated that OSW community significantly differs from OPM and WPM while OPM and WPM showed no significant differences (Supplementary Tables 7 and 10).
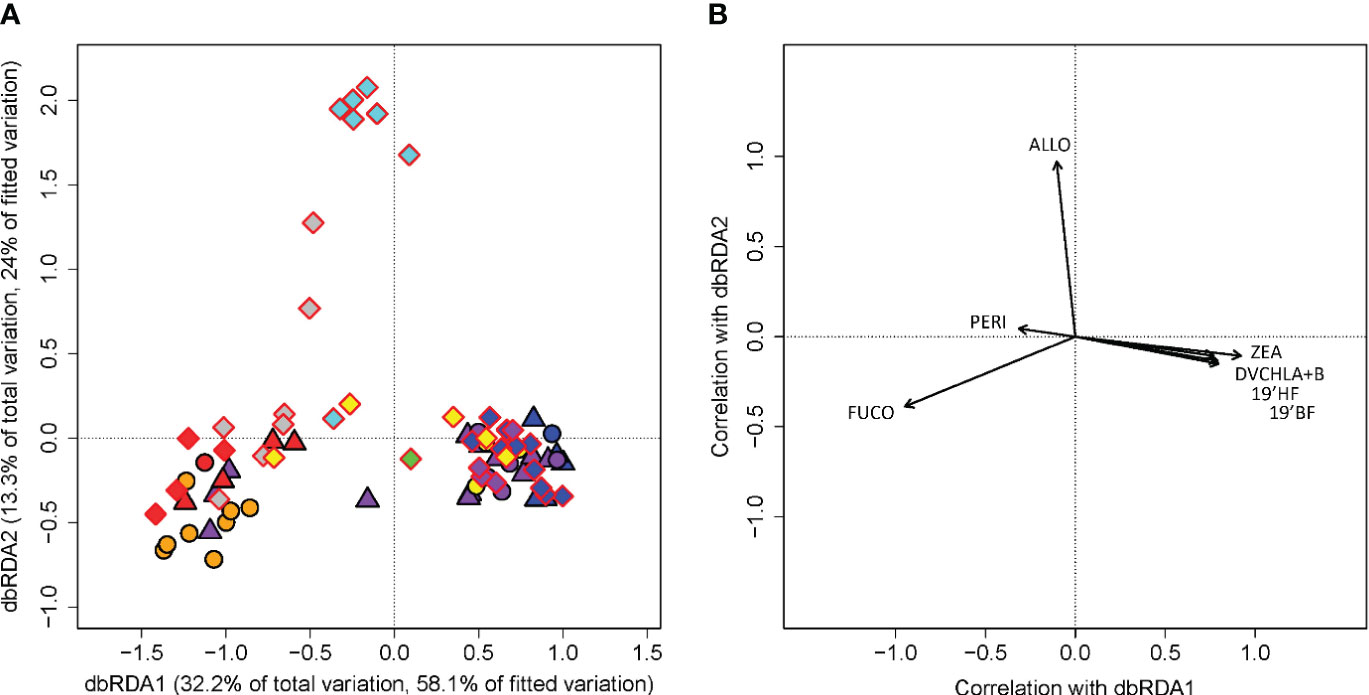
Figure 4 Distance-based redundancy analysis (dbRDA) of diagnostic pigment to total chlorophyll ratio from cruises EN614, EN640, and M174 (R2 = 0.55, p=0.001). Panel (A) is a scatter plot of each measurement’s scores with respect to the first two axes of the dbRDA. Percentages of variation explained by dbRDA1 and dbRDA2 in the total observed variation and in the model fitted with the habitat assignment are listed on their respective axes. Each marker corresponds to a phytoplankton pigment profile from a single cast. Panel (B) is a biplot with arrows displaying the Pearson correlations of phytoplankton pigments relative to the first two dbRDA axes. The length and direction of the arrows indicates the correlation of each pigment with the respective dbRDA axis. The pigments analyzed include: divinyl chlorophyll a + divinyl chlorophyll b (DVCHLA+B), 19’ butanoyloxyfucoxanthin (19’BF), 19’ hexanoyloxyfucoxanthin (19’HF), alloxanthin (ALLO), zeaxanthin (ZEA), peridinin (PERI), and fucoxanthin (FUCO). The phytoplankton groups corresponding to each pigment are presented in Table 3. Marker shapes indicate the cruise each profile was collected as in Figure 1. Habitat types are marked by color: riverine input (RI, gray), young plume core (YPC, red), old plume core (OPC, orange), western plume margin (WPM, yellow), eastern plume margin (EPM, green), outer plume margin (OPM, purple), modified oceanic water (MOW, cyan), and oceanic water (OSW, blue).
To further evaluate the biological significance of the habitat types, we draw on diazotroph cell counts of colonies and free trichomes of Trichodesmium spp. and diatom-diazotroph associations of Richelia spp. in the upper 10 m of the water column collected from cruise KN197, MV1110, and AT21-04. While cell counts showed large deviation within each habitat (Supplementary Table 16), PERMANOVA indicated a significance difference among our habitat types (p-value = 0.001) with the post hoc test showing the largest differences among WPM, EPM, and OSW (Supplementary Table 8). A distance-based redundancy analysis was conducted to further explore the relationship between the phytoplankton cell counts and the habitat types. The dbRDA model of cell counts fitted by habitat assignment returned an R2 value of 0.14 and the permutation test returned a p-value of 0.001. The first two dbRDA axes of cell counts explained 12.6% of the total observed variation and 90.1% of the variation fitted by habitat types (Figure 5). The first axis, dbRDA1, accounted for 9.6% and 68.7% of the total and constrained variation, respectively, and was negatively correlated with Richelia-Hemiaulus hauckii DDA. The second axis, dbRDA2, accounted for 3% and 21.4% of the total and constrained variation, respectively, and correlated positively with Richelia-Rhizosolenia clevei DDA. Both colonial and free Trichodesmium spp., as well as Richelia-Hemiaulus membranaceus DDAs, were positively correlated with dbRDA1 and negatively correlated with dbRDA2. The ordination plot showed that dbRDA1 separated WPM from EPM to some extent, with Richelia-H. hauckii highly correlated with most WPM casts while Richelia-H. membranaceus correlated with most of the EPM communities. Richelia-R. clevei DDAs were found in all habitat types and correlated with most OSW communities. Both colonial and free Trichodesmium spp. were found in all habitat types. Permutation comparisons on the dbRDA confirmed the significant differences among the WPM, EPM, and OSW communities (Supplementary Table 11). Phytoplankton cell count profiles of YPC and OPC were distributed haphazardly across the ordination plot.
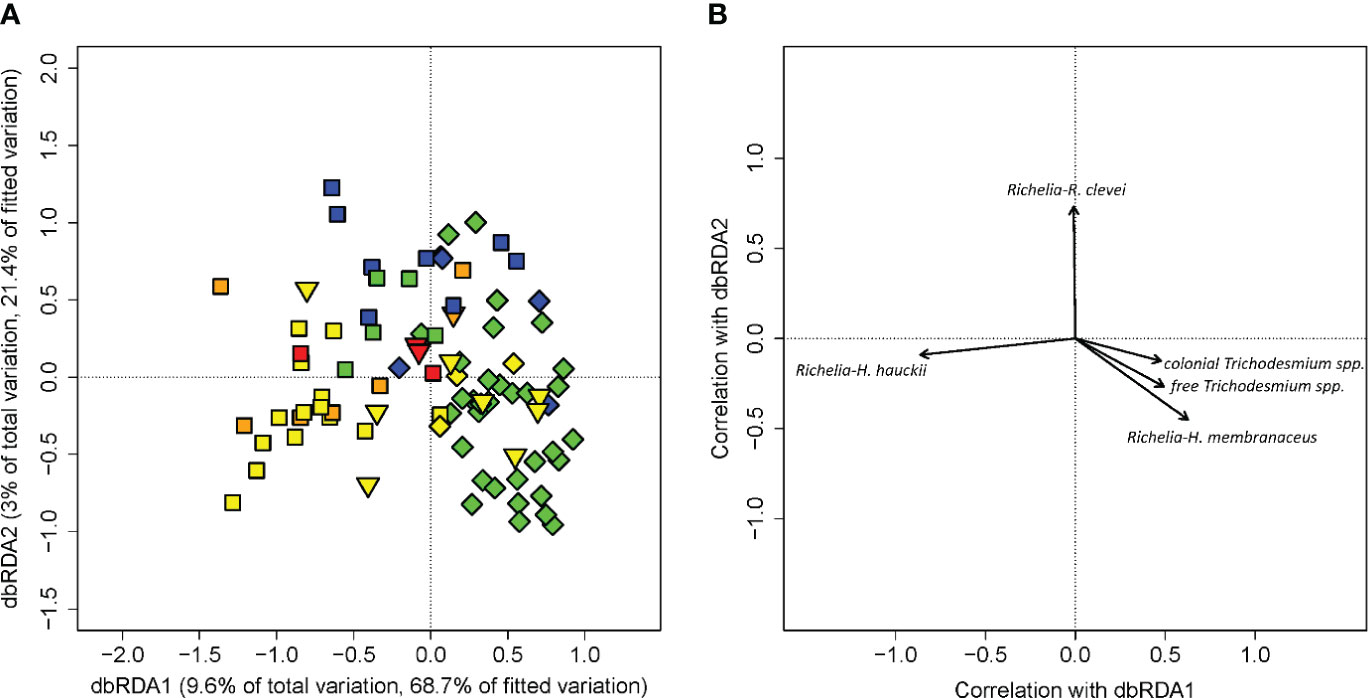
Figure 5 DbRDA of diazotroph cell count data from cruises KN197, MV1110 and AT21-04 (R2 = 0.14, p=0.001). Panel (A) is a scatter plot of each score with respect to the first two axes of the dbRDA. Percentages of variation explained by dbRDA1 and dbRDA2 in the habitat-type fitted model and in the total observed variation are listed on their respective axes. Each marker corresponds to a phytoplankton composition from one single cast. Panel (B) is a biplot with arrows displaying the Pearson correlations of phytoplankton species with the first two dbRDA axes. The length and direction of the arrows indicates the correlation of the species with the respective dbRDA axis. Marker shapes indicate the cruise each profile was collected as in Figure 1. Habitat types are marked by color: riverine input (RI, gray), young plume core (YPC, red), old plume core (OPC, orange), western plume margin (WPM, yellow), eastern plume margin (EPM, green), outer plume margin (OPM, purple), modified oceanic water (MOW, cyan), and oceanic water (OSW, blue).
Discussion
We drew on data collected during cruises in six different years between 2010 and 2021 to explore the robustness of the approach of Weber et al. (2019) for defining planktonic habitats. Hierarchical clustering of stations from all six cruises revealed a consistent classification of stations into the five previously defined habitats as well as three additional habitats revealed in the larger data set, which is more extensive spatially and includes both spring/summer (wet season) and summer/autumn (dry season) cruises. Our approach has increased the resolution of Longhurst’s biogeochemical provinces concept (1998) in the dynamic system of the Amazon River Plume along the Guianas coastal water (Figure 6). We included all stations in our PCA, which provided insight into the differences among the clusters associated with each habitat and its habitat-defining variables. We first consider the patterns evident in our full data set and then explore the differences among our six cruises.
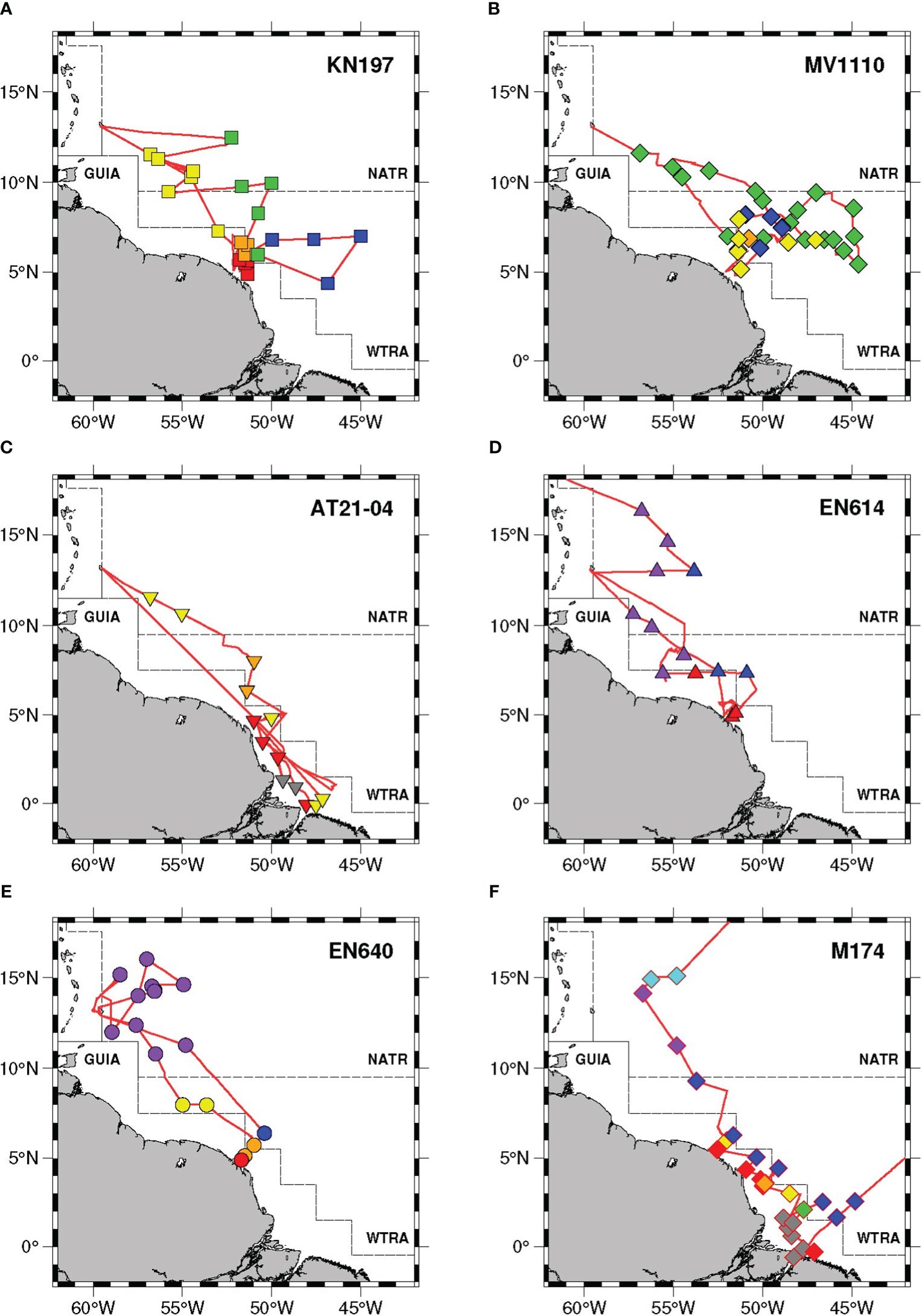
Figure 6 Station map of the six cruises studied (A–F) where each marker denotes a single station. Marker shapes denote the different cruises as in Figure 1. Marker colors show the most common habitat type assigned for the casts of each station: riverine input (RI, gray), young plume core (YPC, red), old plume core (OPC, orange), western plume margin (WPM, yellow), eastern plume margin (EPM, green), outer plume margin (OPM, purple), modified oceanic water (MOW, cyan), and oceanic water (OSW, blue). The red lines denote the individual cruise track. The black dashed lines denote the boundaries of Longhurst’s biogeochemical provinces: North Atlantic tropical gyral (NATR), Western Tropical Atlantic (WTRA), and Guianas coast (GUIA). Habitat types are marked by color: riverine input (RI, gray), young plume core (YPC, red), old plume core (OPC, orange), western plume margin (WPM, yellow), eastern plume margin (EPM, green), outer plume margin (OPM, purple), modified oceanic water (MOW, cyan), and oceanic water (OSW, blue).
All cruises
Despite the differences in temporal and spatial coverage among our six cruises, our habitat classification approach cleanly separated all stations into distinct habitat types, most of which were defined previously by Weber et al. (2019). Our habitat definition approach clearly resolves the combinations of biologically relevant features of the WTNA that are persistent across years. Our inclusion of all stations from cruise KN197 previously described by Weber et al. (2019) provides an internal test of the consistency of the habitat assignments across time and space.
Habitat characteristics
The river input (RI) habitat represented the freshwater discharge at the river mouth (Figure 6). The casts belonging to this habitat can be easily identified by very fresh surface water (4.6 ± 3.6 psu) and high nitrate availability index, with a maximum of 18.7 (Supplementary Table 12). This value, representing the surface NO2-+NO3- concentration in the river mouth, illustrates the potential of the Amazon River for fostering primary production in the region (Howarth, 1988; DeMaster and Aller, 2001).
The brackish warm core of the plume extending northwest of the river mouth (YPC and OPC, Figure 6) can also be identified using salinity alone (Figure 2; Supplementary Table 12), reflecting the mixing of river discharge with oligotrophic ocean water. The two habitat types falling within the plume core can be differentiated by the sharp decline of the nitrate availability index from the young plume core (-4.5 ± 10.2) to the old plume core (-82.2 ± 15.2).
Further north, our habitat analysis revealed three distinct habitat types associated with the mixing of the aging plume and oceanic waters: WPM, EPM, and OPM (Figure 6). These habitats are characterized by increased SSS compared to the plume core, and gradual deepening of the MLD from WPM to EPM to OPM (Figure 2), reflecting the reduced influence of the river with increasing distance from the river mouth. Two of these habitats, WPM and EPM, were originally named based on their locations to the west and east of the plume axis during cruise KN197 (Figure 6). However, our multi-year dataset analysis reveals that these two distinct habitats actually represent different degrees of riverine influence, which is greater in the WPM than in the EPM habitat. The relative geolocalization of one habitat or the other could simply arise from the seasonal variability in the Amazon River Plume trajectory, itself tied to an array of forcing factors, such as river discharge, precipitation, and oceanic current structure (Molleri et al., 2010; Coles et al., 2013; Varona et al., 2019).
Our newly defined outer plume margin habitat was located well north of the plume core, where the previously defined WPM and EPM habitats frequently co-occur (Figure 6). OPM waters shared the same range of SSS (31.6 ± 1.5 psu) with the other plume margin habitats but was characterized by the relatively cold water (27.5 ± 0.3°C), the slight deepening of the mixed layer depth, and a decrease by a factor of 2 in nitrate availability to the surface water (Figure 2), showing weaker riverine influence relative to the other plume margin habitats.
The modified oceanic water (MOW) habitat was detected only during cruise M174, located well north of the plume core, in a region where we typically found OPM waters during the previous cruises (Figure 6). This habitat shared the same range of mixed layer depth with the plume margin habitats but was characterized by the coldest water (26.7 ± 0.1°C), a slight increase in surface salinity and a steep decline in surface nitrate availability relative to the plume margin habitats and an extremely deep chlorophyll maximum depth (126.9 ± 7.0 m, Figure 2). Thus, the only evident influence of the river plume in this habitat was the shoaling of the mixed layer depth compared to that typical of the oceanic water.
Oceanic water (OSW), which encompassed the oligotrophic waters surrounding the plume, was typically found to the east of the plume region (Figure 6). The only exception was during cruise MV1110 when the plume retroflected eastward with the NECC, and patches of OSW were found intermingled with EPM waters. OSW waters were distinguished by their deep mixed layer depth and high sea surface salinity (Figure 2).
Inter-cruise habitat comparisons
Most of the habitats described earlier from cruise KN197 were sampled during the 6 additional cruises (Figure 6). The freshwater (RI) habitat was only sampled during two cruises, AT21-04 and M174. We sampled the OPM habitat only during the last three cruises (EN614, EN640, and M174) and the MOW habitat only during the last cruise (M174). Similarly, we largely missed the EPM habitat on the middle three cruises of our series (AT21-04, EN614, and EN640) and only captured one instance of the EPM habitat on our most recent cruise (M174). These contrasts in habitats sampled reflect both the varied sampling regions among cruises and the dynamic nature of the plume margin and the distribution of properties associated with it (Molleri et al., 2010; Coles et al., 2013; Varona et al., 2019).
Cruise KN197 was conducted from late spring to early summer of 2010, during peak river discharged following the Amazonia wet season, and sampled the river plume as well as both the western and eastern margins of the plume axis (Supplementary Figures 5 and 6; Liang et al., 2020). The resulting five habitat types originally described by Weber et al. (2019) represented a continuum of water age and mixing history of the plume with oceanic waters (Figure 6A). All of the habitat types originally defined by Weber et al. (2019) are evident in our multi-cruise data set, and about ninety percent of the station assignments were unchanged in our global analysis. Only four casts from cruise KN197 were reassigned when analyzed in the context of our full data set: two casts shifted from WPM to EPM, and two casts shifted from OPC to WPM (Supplementary Text; Supplementary Table 2). These reassignments reflect a refinement of our ability to distinguish the three habitat types based on our larger data set.
Cruise MV1110 was conducted in the early fall of 2011, right before the driest month of the Amazon River basin (Liang et al., 2020). During this time, the river discharge weakened, and the ARP traveled eastward as it was entrained in the NECC (Supplementary Figures 5, 6). We classified the MV1110 stations into 4 of the previously defined habitat types, with most of the casts assigned to the EPM habitat (Figure 6B). As a result of the river plume being mixed with oceanic water, we found a collection of habitat types from the plume core (OPC) to the plume margins (WPM and EPM) to oceanic water (OSW), with most of the casts showing the relatively high salinity and greater depth of the chlorophyll maximum, characteristic of the EPM habitat. Interestingly, the stations in the northwest region of the plume and located to the west of the plume axis were also grouped as EPM, reflecting the generally reduced influence of river discharge to this area in comparison to what we observed on cruise KN197. When we applied our habitat classification routine on the dataset of cruise MV1110 alone, four casts shifted from OPC to WPM and four casts shifted from WPM to EPM, changes that illustrate the dependence of the hierarchical clustering algorithm on the diversity of the dataset available (Supplementary Text; Supplementary Table 13). Since most of the casts from cruise MV1110 had properties similar to those of the plume margins and oceanic water due to strong mixing as the river discharge retroflected toward the Central Tropical Atlantic Ocean, the smaller number of plume-core-like casts closer to the river mouth did not form a distinct grouping.
Cruise AT 21-04 was conducted during summer 2012, around the start of the dry season, when the river discharge slightly weakened and started to retroflect to the NECC (Supplementary Figures 5, 6; Liang et al., 2020). This cruise focused on the western area of the plume region and sampled the freshest portion of the plume near the Amazon River mouth (Supplementary Figures 5, 6). Our multi-cruise approach divided the casts from cruise AT21-04 into 4 habitat types, including the new riverine input (RI), the plume core (YPC and OPC), and western plume margin (WPM, Figure 6C). The RI habitat was distinguished by very fresh water with a mean NAI value of 9.41 ± 2.8, which was slightly lower than the riverine NO2+NO3 concentrations (11.5 µM) measured during peak river discharge in May 2010 (Goes et al., 2014). The depths of the mixed layer and the chlorophyll maximum of RI casts were similar to those of YPC and OPC. The influence of the river discharge extended both northward and southward of the river mouth as some stations to the south of the outflow were classified as either YPC or WPM, reflecting the importance of mixing in determining habitats. Our single-cruise analysis was dominated by stations strongly affected by the river plume, making it difficult to distinguish among stations in the plume margin (Supplementary Text; Supplementary Table 13).
Cruise EN614 was conducted in spring 2018, during river peak discharge after wet season (Supplementary Figures 5 and 6). The stations from cruise EN614 were assigned to 2 previously defined habitat types, including YPC, OSW, and a new habitat type we named the “Outer Plume Margin” (OPM), extending northward of the plume region (Figure 6D). This habitat shared the same ranges of mixed layer depth and surface salinity as WPM and EPM but was characterized by much cooler surface water and lower surface nitrate availability. The habitat classification of cruise EN614 reflected a sharp decline of plume influence northward of the plume core from YPC to OPM without any intermediate waters (WPM or EPM) as previously observed. In the single-cruise analysis of cruise EN614, two casts from the same station (EN614_08), located northwest of the river mouth, were shifted from YPC to OPM (Supplementary Text; Supplementary Table 13). Sea surface temperature and salinity of this station fell within the range of the plume margin waters (27°C and 30 psu), hence they were classified as OPM in the single-cruise analysis. However, the classification of these two casts in the more extensive analysis was strongly driven by the high nitrate availability in the upper water column (-5.9), a defining characteristic of the YPC habitat, and intermediate depths of the mixed layer and chlorophyll maximum (14 m and 20 m, respectively). The contrast between the physical (SSS and SST) and biogeochemical (ChlMD and NAI) properties of this station may reflect the mismatch in rate between the more dynamic river mixing and the slower biological processes (e.g., nitrate uptake, phytoplankton growth).
Cruise EN640 was conducted in summer 2019, as the river discharge started to relax, and sampled across the plume core as well as around Barbados, well north of the plume area (Supplementary Figures 5 and 6). Stations from cruise EN640 were assigned to 5 habitat types, including a single representative of YPC (EN640_06.01) and OSW (Figure 6E). Most of the stations of cruise EN640 were classified as either OPM, with some stations falling into the OPC and WPM habitats. On the whole, cruise EN640 sampled a region with reduced exposure to the plume, with low surface nitrate availability at all stations except for the single YPC station and one WPM station. In our single cruise analysis, two casts shifted from YPC to WPM and from WPM to OPM (Supplementary Text; Supplementary Table 13).
Cruise M174 was conducted in spring 2021, when the river discharge had nearly reached its annual peak after the wet season, and covered the entire plume region from the river mouth to northern waters in the EEZ of Barbados (Supplementary Figures 5 and 6; Liang et al., 2020). Stations from cruise M174 were assigned to all eight habitat types (Figure 6F). We captured multiple RI and OSW stations but only one station in the EPM habitat. We also found a new habitat type during cruise M174, namely modified oceanic water (MOW). The casts classified into this habitat were located well north of the plume proper, where we typically found OPM waters on our previous cruises. The MOW had a similar range of mixed layer depth and a slightly higher surface salinity than the plume margin waters, but was characterized by much colder water with deep chlorophyll maximum depth and deficient nitrate in the upper water column. Hence, this habitat has properties closer to that of the oceanic water, with only a slight influence of the river discharge, evident in the shoaling of the mixed layer depth. Among our expeditions, cruise M174 sampled the most extensive region of the plume and its environs and captured all of our previously defined habitats, reinforcing the consistency of these habitats over a decadal time span. We found only one change in our single-cruise analysis, in which the only EPM cast shifted to WPM (Supplementary-Text; Supplementary Table 13).
Inter-cruise contrasts
We further conducted Welch’s ANOVA and subsequent post hoc analyses to compare the habitat-defining variables across different sampling times following the Amazonia wet (five cruises) and dry (one cruise) seasons for each habitat type. As the MOW habitat was solely detected during one cruise, only seven of the eight habitats were analyzed.
Sea surface salinity showed significant differences in the waters with higher plume influenced including RI, OPC, and WPM while YPC showed high within-cruise variations (Supplementary Figure 5; Supplementary Table 5), reflecting the different hydrological conditions of the Amazon basin that drive the river discharge and in turn, the salinity of the plume (Liang et al., 2020). Sampling area can also be a major driver in the observed salinity differences in the riverine input, where cruise AT21-04 had some stations closer to the river mouth and thus sampled much fresher water. Cruise M174, on the other hand, followed full tidal cycles at single stations, where we observed pronounced variations in the depth of the halocline.
Sea surface temperature appeared to vary the most among cruises (Supplementary Figure 5), showing a gradual increase from May to October in the Amazon Plume region, which could be driven by both the Amazon and Pará rivers hydrology (Varona et al., 2019). Interestingly, all three assigned habitats of cruise EN614, sampled from May to June 2018, had lower surface water temperatures than those of the same habitat type in other cruises (Supplementary Figure 6; Supplementary Table 5). This could reflect the cooling of both the Amazon basin and Tropical North Atlantic due to the influence of La Niña during rainy season 2017/2018 (DiNezio et al., 2017; Moura et al., 2019; Wu et al., 2020). Adversely, the slightly higher SST observed during cruise KN197 in June 2010 might have been driven by the 2009/2010 El Niño event (Moura et al., 2019; Wu et al., 2020).
The depths of the mixed layer showed some significant differences among cruises, with most notable changes in the OSW, where we observed a gradual deepening of the mixed layer depth from wet to dry season (Supplementary Figure 5; Supplementary Table 5), reflecting differences in plume influence across the different seasons and spatial coverage (peak discharge vs retroflecting plume) of each cruise. The shoaling of the OSW mixed layer depth in cruise KN197 could also reflect the increased temperature due to El Nino as previously discussed. The temporal similarity observed in the riverine input and plume core reflects the shallow well-mixed water in these areas.
We observed little temporal differences for depth of chlorophyll maximum and surface nitrate availability, both of which seem to vary within each cruise more than between cruises (Supplementary Figure 5; Supplementary Table 5). We found both high and low outliers in the ChlMD and NAI distributions, which were either single casts or groups of casts from the same stations (Figure 2). These outliers might reflect local physical forcings such as upwelling or eddies that may inject inorganic nitrogen into surface waters and in turn affect phytoplankton growth. Biological activities can also control the nitrate availability to the surface, both through consumption of surface nutrients by phytoplankton blooms and production of nitrate through nitrification.
The strong seasonality of the Amazon River discharge drives the plume intensity and geolocalization, which in turn creates varied gradients of hydrological and biogeochemical properties along its path (Coles et al., 2013; Liang et al., 2020). The variations we found among cruises in the individual parameters we considered illustrate the difficulty of characterizing planktonic systems using just one or two indices. By integrating five complementary and routinely measured parameters, our approach produced a consistent habitat classification across cruises carried out in different years and both the wet and dry seasons.
Phytoplankton diversity
To further investigate the effect of the Amazon River dynamics on primary producer community, we combined multiple approaches to characterize phytoplankton community composition at different taxonomic levels and to explore the relationship between phytoplankton communities and the delineated habitat types. In vivo fluorescence and diagnostic pigment analyses provided a high-level assessment of major taxonomic groups, while microscopic cell counts provided insight into the phytoplankton community at the genus or species level.
Both ALF and HPLC pigment measurements from cruises EN614, EN640, and M174 showed clear contrasts among the strongly plume-influenced waters, including the riverine input and plume cores, the intermediate plume margins, and the oceanic waters (Figures 3 and 4), indicating a shift in phytoplankton communities down the plume gradient. The dbRDA routines showed that our habitat assignments captured approximately half of the total observed variation in the surface phytoplankton community composition at these broad taxonomic levels. When the data structures were examined through the models fitted with the habitat assignment, the first two dbRDA axes explained more than 90% of the variation in the in-vivo fluorescence and 75% of the variation in the diagnostic pigments, indicating that our routines captured most of the significant patterns in both data sets.
In vivo fluorescence measured by ALF showed a high level of PE-1, an indication of blue-water cyanobacteria, among the WPM and OPM communities, most of which were located well north of the plume core during cruises EN614 and EN640 (Figure 3). Cell count data previously reported for cruise KN197 suggested a high abundance of Trichodesmium spp. and blue water Synechococcus spp. in areas with high PE-1 signal, especially the northern area of the plume region with salinity above 28 psu, where inorganic phosphate was not a limiting factor for phytoplankton growth (Goes et al., 2014). Although the two habitats share roughly the same ranges of salinity, temperature, and nitrate available to the surface, the high signal of PE-1 measured in the plume-influenced northern area (OPM) compared to the eastern oligotrophic water (OSW) suggests the important role of other nutrients (e.g., phosphate) from the river discharge in supporting primary production, as evidenced by the shoaling of the mixed layer depth at the OPM stations (Figures 2). Signal of green water cyanobacteria (PE-2), primarily DDAs (Goes et al., 2014), were found to some extent in the YPC and OPM stations (Supplementary Figure 3). While YPC represented a nutrient-repleted environment along the river plume, providing optimal conditions for DDAs growth (Shipe et al., 2006; Subramaniam et al., 2008; Foster et al., 2011), OPM is more nutrient-limited, whose high PE-2 signal indicated other sources of nutrient supporting growth of green water cyanobacteria. Fluorescence signature for eukaryotic photoautotrophic cryptophytes (PE-3), which often dominate coastal and estuarine waters (Chekalyuk and Hafez, 2008; Chekalyuk et al., 2012; Goes et al., 2014), were also present in plume margin waters (Figure 3). Unsurprisingly, ALF-derived chlorophyll concentrations were high around the fresh surface waters of the plume cores (YPC and OPC) with shallow chlorophyll maximum depths. While these two habitats have surface salinity comparable to the “estuarine type” of phytoplankton community (below 28 psu) described by Goes et al. (2014) in this region, we observed higher signal of chlorophyll in most slightly N-deplete OPC stations (2.8 ± 2.3 RFU) comparing to the nutrient-rich YPC stations (0.6 ± 0.2 RFU, Supplementary Table 14). The low signal of green water cyanobacteria (PE-2) among the OPC stations compared to those of YPC also indicates the different community compositions between the two plume core habitats sharing the same salinity range (Supplementary Table 14).
Diagnostic pigment measured via HPLC showed that diatoms (FUCO) strongly correlated with the riverine input and plume core habitats (Figure 4) where nitrate availability was higher than in OSW and enough light was available to support their photosynthesis and growth. Interestingly, we also observed instances of high fucoxanthin concentration in some WPM and OPM casts, suggesting different modes of nutrient repletion. The strong vertical stratification of the favored diatom habitats could support the retention of phytoplankton cells in the upper water column (Weber et al., 2019; Farias et al., 2022), explaining their accumulation in the surface waters of these habitats, contributing to the rapid draw down of inorganic nitrogen across the plume core area (Subramaniam et al., 2008).
Cyanobacteria (ZEA) and haptophytes (19’HF and 19’BF), which are commonly found in subtropical and temperate marine environments (Andersen, 2004) also correlated with plume margins and oceanic waters (Figure 4). Zeaxanthin provided a broad indication for cyanobacteria, including the prochlorophytes (DVCHLA+B) that are ubiquitous in oligotrophic waters and the free-living diazotrophs that thrive in environments characterized by a low N:P nutrient ratio, due to their ability to fix atmospheric nitrogen (Zehr et al., 2001; Vrede et al., 2009; Goes et al., 2014; Dupouy et al., 2018). Haptophytes have low irradiance and nutrient requirements for growth, giving them a competitive advantage over diatoms under nitrate-limited conditions (Araujo et al., 2017).
Prochlorophytes (DVCHLA+B), commonly found in oceanic waters (Weber et al., 2019; Farias et al., 2022), correlated strongly with our nutrient-deprive OPM and OSW casts (Figures 4). Their large surface-area-to-volume ratio is advantageous for nutrient uptake in oligotrophic environments and is consistent with their higher diagnostic pigment ratio found in the OSW than the OPM habitat (Supplementary Table 15). In addition, this group presents a suite of genetic ecotypes adapted to a wide range of irradiance, enabling prochlorophytes to thrive across the often deep mixed layer of oceanic environments (Moore et al., 1998; Partensky et al., 1999). We found lower concentrations of prochlorophytes in some WPM casts located well north of the plume area (Supplementary Table 15), which fits the definition of these habitats as a transition between the nutrient-replete plume water and oligotrophic oceanic environments. The much clearer distinction between the OPM and OSW observed in the ALF signals (driven by PE-1, Figure 3) compared to the differences in the diagnostic pigments (Figure 4) reflect the varied capabilities of each method in identifying the phytoplankton groups.
Cryptophytes (ALLO), common in fresh and brackish waters (Chekalyuk and Hafez, 2008; Chekalyuk et al., 2012; Weber et al., 2019), correlated strongly with the modified oceanic water and were detected in some of the riverine input communities during cruise M174 (Figure 4; Supplementary Table 15). The presence of cryptophytes at the edges of the plume (MOW) is not surprising as SiO2 becomes increasingly limiting with distance from the River Mouth (Goes et al., 2014) and cryptophytes are known to succeed diatoms when SiO2 becomes strongly limiting (Dokulil and Skolaut, 1986). Additionally, cryptophytes could have thrived under the high turbidity, light-limited conditions of the riverine input due to their capability to absorb a wide range of wavelengths of light (Haxo and Fork, 1959) and also take up organic compounds (Lewitus and Caron, 1991; Gervais, 1997) potentially supplied by the plume (DeMaster and Aller, 2001).
Dinoflagellates (PERI) were found in most habitat types and correlated with the riverine input and young plume core communities (Supplementary Figure 4; Supplementary Table 15), matching the microscopic cell count data for cruise KN197 plume core stations (Goes et al., 2014). High nutrient contents from the river discharge appeared to support growth of dinoflagellate as NAI was the main driver separating the RI and YPC from the rest of the habitat types. However, this group could be underestimated in our pigment analysis as some dinoflagellates are characterized by other pigments such as fucoxanthin or chlorophyll b as a result of secondary endosymbiosis (Zapata et al., 2012; Farias et al., 2022).
Microscopic counts of diazotrophs from three cruises (KN197, MV1110, and AT21-04) showed clear distinction along the intermediate and oceanic waters (Figure 5). The Richelia-Hemiaulus hauckii DDA correlated strongly with the OPC and WPM habitats while the Richelia-Hemiaulus membranaceus DDA correlated with the EPM waters, a distribution similar to the DDA-dominated “mesohaline” communities described in Goes et al. (2014), where they play a crucial role in nitrogen fixation and carbon sequestration, extending the plume impact hundreds of kilometer further away from the nutrient-rich area near the river mouth (Shipe et al., 2006; Subramaniam et al., 2008; Yeung et al., 2012). More oligotrophic conditions (OSW) appeared favorable for Richelia-Rhizosolenia clevei symbioses (Foster et al., 2007). Biological interactions such as niche partitioning among planktonic species may also play an important role in driving community variation within as well as among habitats (Weber et al., 2017). For example, the small, rapidly growing diatom H. hauckii may outcompete H. membranaceus and R. clevei in the regions with more influence from the plume filaments (Villareal, 1989; Hasle et al., 1996; Foster et al., 2007).
Interestingly, while Trichodesmium spp. are often found in mesohaline and oligotrophic waters, and was previously reported to correlate strongly with PE-1, dominating the “oceanic type” community (salinity over 35 psu) together with blue water Synechococcus spp. (Goes et al., 2014; Weber et al., 2017), we found no geographical distinction in our cell count data. High instances of Trichodesmium spp. were recorded throughout the plume gradient, from nutrient-rich plume cores to oligotrophic waters. The mismatch between our high PE-1 measurement in the outer plume margins and the ubiquitous Trichodesmium spp. cell counts suggests that other oceanic cyanobacterial groups, including Synechococcus spp., may contributed greatly to the elevated PE-1 in the outer plume margins. Our cell count observation fit other findings that DDAs distributions were driven by the phosphate and silicate gradient along the plume-influenced waters while Trichodesmium spp. were less effected by the riverine input (Louchard et al., 2023).
It is worth noting that our cell count dataset focuses on diazotrophs and may not reflect the complexity of the entire surface phytoplankton community. Additionally, our cell count analysis mainly focused on the dry season of the Amazon River (cruises MV1110 and AT21-04), which might result in fewer observations of strongly plume-influenced waters and their associated diazotroph communities. Since phytoplankton community composition reflects growth over some period of time before sampling, the mismatch between the water biogeochemical properties and the phytoplanktonic communities could be a result of temporal and spatial disparities between ocean environmental conditions and the observed phytoplankton communities, indicating recent changes in the system.
Conclusions
We applied the habitat-definition approach of Weber et al. (2019) to a suite of cruises spanning different portions of the Amazon’s plume region in different years across its two predominant (wet and dry) seasons. The five previously defined habitats were distinguishable in all years of our study, and most of the initial habitat assignments of Weber et al. (2019) based on a single cruise were preserved in the larger analysis. Comparison of single- and multi-cruise analyses demonstrated the general robustness of our classification and the importance of adequately sampling the entire range of habitat variation in the region. Our larger data set revealed three additional habitat types, including the fresh river discharge (RI) at the mouth of the Amazon River, water slightly influenced by the river plume (OPM), locating to the northern edge of the plume core, and the more oceanic-like water (MOW), located well north of the plume region. The high number of mismatches between single-cruise and multi-cruise classification in cruise MV1110 and AT21-04, conducted during dry season, might be a result of our higher focus on the peak discharge period of the Amazon River.
We have explored the biological relevance and consistency of this habitat-defining approach among the six cruises using a collection of phytoplankton community measurements. We were able to observed much clearer differences among habitats using in-vivo fluorescence and diagnostic pigment measurements conducted in three cruises during river peak discharge (Table 2), than with cell counts. We found clear distinctions of DDAs among the habitats in the mesohaline region using direct cell counts. The single and colonial Trichodesmium spp. differed less consistently among habitats, which partially reflect the under-sampled dry season and suggest that inter-species interactions may play an important role in structuring the broader plankton community. The mismatch between some phytoplankton communities on the ordination space and their assigned habitat type may reflect the uncoupling between often rapid changes in physical and chemical properties and the growth responses of the phytoplankton populations. Overall, our habitat classifications coincided with major patterns in phytoplankton distribution in the Amazon River Plume region, showing that our approach delineated robust and consistent habitats that supported distinct phytoplankton communities.
Data availability
All data are available on request and are/will be available via these following sources.
CTD and bottle data.
Montoya, J. (2019) AN10/KN197-08 CTD Nutrients and Particles. Biological and Chemical Oceanography Data Management Office (BCO-DMO). (Version 2) Version Date 2019-03-13. http://lod.bco-dmo.org/id/dataset/762169.
Yager, P. L. (2018) CTD Averaged Bottle Data from R/V Melville cruise MV1110 in the Amazon River plume, NE coast of South America from September to October of 2011 (ANACONDAS project). Biological and Chemical Oceanography Data Management Office (BCO-DMO). (Version 1) Version Date 2018-07-30. http://lod.bco-dmo.org/id/dataset/742833.
Montoya, J. (2019) AN12/AT21-04 CTD Nutrients and Particles. Biological and Chemical Oceanography Data Management Office (BCO-DMO). (Version 2) Version Date 2019-03-13. http://lod.bco-dmo.org/id/dataset/762179.
Montoya, J., Peterson, R., Subramaniam, A. (2019) Hydrographic data collected during casts with a CTD-rosette system on R/V Endeavor cruise EN614 from May to June 2018. Biological and Chemical Oceanography Data Management Office (BCO-DMO). (Version 1) Version Date 2019-03-06. doi:10.1575/1912/bco-dmo.757784.1.
Montoya, J., Subramaniam, A. (2021) Dissolved, inorganic nutrient data integrated with bottle data from R/V Endeavor cruise EN614 in May 2018. Biological and Chemical Oceanography Data Management Office (BCO-DMO). (Version 1) Version Date 2021-10-26. doi:10.26008/1912/bco-dmo.861206.1.
Montoya, J., Peterson, R. N., Subramaniam, A. (2021) Binned profiles of CTD measurements from R/V Endeavor cruise EN640 from June-July 2019. Biological and Chemical Oceanography Data Management Office (BCO-DMO). (Version 3) Version Date 2021-03-19. doi:10.26008/1912/bco-dmo.846170.3.
Montoya, J., Subramaniam, A. (2021) Dissolved, inorganic nutrient data integrated with bottle data from R/V Endeavor cruise EN640 in June-July 2019. Biological and Chemical Oceanography Data Management Office (BCO-DMO). (Version 1) Version Date 2021-09-24. doi:10.26008/1912/bco-dmo.861378.1.
Mohrholz, Volker; Heene, Toralf; Mars, Robert; Söder, Jens; Subramaniam, Ajit (2022): Hydrographic properties of water masses in the Amazonas River plume obtained in April/May 2021 by CTD measurements during RV METEOR cruise M174. PANGAEA, https://doi.org/10.1594/PANGAEA.942346.
Phytoplankton data.
Carpenter, E. J. (2014) Phytoplankton: Microphytoplankton Station Counts from R/V Atlantis, R/V Knorr, R/V Melville AT21-04, KN197-08, MV1110 in the Amazon River plume; NE coast of South America from 2010-2012 (ANACONDAS project). Biological and Chemical Oceanography Data Management Office (BCO-DMO). (Version 02 January 2014) Version Date 2014-01-02. http://lod.bco-dmo.org/id/dataset/473803.
Subramaniam, A. (2020) Phytoplankton diagnostic pigments from HPLC from samples collected on R/V Endeavor cruise EN614 in the tropical North Atlantic during May 2018. Biological and Chemical Oceanography Data Management Office (BCO-DMO). (Version 1) Version Date 2019-06-05. doi:10.26008/1912/bco-dmo.769601.1.
Subramaniam, A. (2021) Phytoplankton diagnostic pigments from HPLC from samples collected on R/V Endeavor cruise EN640 in the tropical North Atlantic during June-July 2019. Biological and Chemical Oceanography Data Management Office (BCO-DMO). (Version 1) Version Date 2021-12-08. http://lod.bco-dmo.org/id/dataset/866351.
Subramaniam, A. (2022) Data from custom instrument (CLASS) measured on R/V Endeavor cruise (EN614) in May 2018. Biological and Chemical Oceanography Data Management Office (BCO-DMO). (Version 1) Version Date 2022-06-23. http://lod.bco-dmo.org/id/dataset/876170.
Data availability statement
The datasets presented in this study can be found in online repositories. The names of the repository/repositories and accession number(s) can be found in the article/Supplementary Material.
Author contributions
AP: Conceptualization, Data curation, Formal analysis, Investigation, Visualization, Writing – original draft. NC: Writing – review & editing, Investigation. AF-C: Writing – review & editing, Investigation. AS: Data curation, Funding acquisition, Writing – review & editing. ES: Data curation, Writing – review & editing. EC: Data curation, Writing – review & editing. MV: Data curation, Writing – review & editing. JM: Conceptualization, Data curation, Funding acquisition, Writing – original draft.
Funding
The author(s) declare financial support was received for the research, authorship, and/or publication of this article. This work was supported by NSF grants to JM (OCE-0934025 and OCE-1737078) and AS (OCE-1737128) and NASA grant 80NSSC21K0439 to AS. NC and AF-C were supported by DFG grants VO487/14-1. The M174 cruise was supported by grant GPF 19-1-13.
Acknowledgments
We thank the officers and crew of the RV Knorr, RV Melville, RV Atlantis, RV Meteor and RV Endeavor for their support of our work at sea. We also thank J. Landrum, R. Horak, N. Loick-Wilde, A. Martin, S. Agard, and B. Ramcharitar for their assistance and collaboration at sea and ashore.
Conflict of interest
The authors declare that the research was conducted in the absence of any commercial or financial relationships that could be construed as a potential conflict of interest.
The reviewer, HD-N, declared a past co-authorship with the authors, AS, MV, JM.
Publisher’s note
All claims expressed in this article are solely those of the authors and do not necessarily represent those of their affiliated organizations, or those of the publisher, the editors and the reviewers. Any product that may be evaluated in this article, or claim that may be made by its manufacturer, is not guaranteed or endorsed by the publisher.
Supplementary material
The Supplementary Material for this article can be found online at: https://www.frontiersin.org/articles/10.3389/fmars.2024.1287497/full#supplementary-material
References
Alvain S., Moulin C., Dandonneau Y., Bréon F.-M. (2005). Remote sensing of phytoplankton groups in case 1 waters from global SeaWiFS imagery. Deep Sea Res. Part I: Oceanographic Res. Papers 52, 1989–2004. doi: 10.1016/j.dsr.2005.06.015
Andersen R. A. (2004). Biology and systematics of heterokont and haptophyte algae. Am. J. Bot. 91, 1508–1522. doi: 10.3732/ajb.91.10.1508
Anderson M. J. (2001). A new method for non-parametric multivariate analysis of variance. Austral Ecol. 26, 32–46. doi: 10.1111/j.1442-9993.2001.01070.pp.x
Anderson M., Gorley R., Clarke K. (2008). PERMANOVA+ for PRIMER: guide to software and statistical methods (UK: Plymouth).
Araujo M. L. V., Mendes C. R. B., Tavano V. M., Garcia C. A. E., Baringer M. O. N. (2017). Contrasting patterns of phytoplankton pigments and chemotaxonomic groups along 30 S in the subtropical South Atlantic Ocean. Deep Sea Res. Part I: Oceanographic Res. Papers 120, 112–121. doi: 10.1016/j.dsr.2016.12.004
Baltar F., Aristegui J. (2017). Fronts at the surface ocean can shape distinct regions of microbial activity and community assemblages down to the bathypelagic zone: The Azores Front as a case study. Front. Mar. Sci. 4, 252. doi: 10.3389/fmars.2017.00252
Beaugrand G., Ibañez F., Lindley J. A., Reid P. C. (2002). Diversity of calanoid copepods in the North Atlantic and adjacent seas: species associations and biogeography. Mar. Ecol. Prog. Ser. 232, 179–195. doi: 10.3354/meps232179
Carpenter E. J., Montoya J. P., Burns J., Mulholland M. R., Subramaniam A., Capone D. G. (1999). Extensive bloom of a N2-fixing diatom/cyanobacterial association in the tropical Atlantic Ocean. Mar. Ecol. Prog. Ser. 185, 273–283. doi: 10.3354/meps185273
Charvet S., Kim E., Subramaniam A., Montoya J., Duhamel S. (2021). Small pigmented eukaryote assemblages of the western tropical North Atlantic around the Amazon River plume during spring discharge. Sci. Rep. 11, 1–14. doi: 10.1038/s41598-021-95676-2
Chekalyuk A., Hafez M. (2008). Advanced laser fluorometry of natural aquatic environments. Limnology Oceanography: Methods 6, 591–609. doi: 10.4319/lom.2008.6.591
Chekalyuk A., Hafez M. (2013). Next generation Advanced Laser Fluorometry (ALF) for characterization of natural aquatic environments: new instruments. Optics express 21, 14181–14201. doi: 10.1364/OE.21.014181
Chekalyuk A. M., Landry M. R., Goericke R., Taylor A. G., Hafez M. A. (2012). Laser fluorescence analysis of phytoplankton across a frontal zone in the California Current ecosystem. J. Plankton Res. 34, 761–777. doi: 10.1093/plankt/fbs034
Coles V. J., Brooks M. T., Hopkins J., Stukel M. R., Yager P. L., Hood R. R. (2013). The pathways and properties of the Amazon River Plume in the tropical North Atlantic Ocean. J. Geophysical Research: Oceans 118, 6894–6913. doi: 10.1002/2013JC008981
Corbineau A., Rouyer T., Cazelles B., Fromentin J.-M., Fonteneau A., Ménard F. (2008). Time series analysis of tuna and swordfish catches and climate variability in the Indian Ocean, (1968-2003). Aquat. Living Resour. 21, 277–285. doi: 10.1051/alr:2008045
Del Vecchio R., Subramaniam A. (2004). Influence of the Amazon River on the surface optical properties of the western tropical North Atlantic Ocean. J. Geophysical Research: Oceans 109, C11001. doi: 10.1029/2004JC002503
DeMaster D. J., Aller R. C. (2001). Biogeochemical processes on the Amazon shelf: changes in dissolved and particulate fluxes during river/ocean mixing. Biogeochemistry Amazon Basin 16, 328–357. doi: 10.1093/oso/9780195114317.003.0020
DeMaster D., Smith W. Jr., Nelson D., Aller J. (1996). Biogeochemical processes in Amazon shelf waters: chemical distributions and uptake rates of silicon, carbon and nitrogen. Continental Shelf Res. 16, 617–643. doi: 10.1016/0278-4343(95)00048-8
DiNezio P. N., Deser C., Karspeck A., Yeager S., Okumura Y., Danabasoglu G., et al. (2017). A 2 year forecast for a 60–80% chance of La Niña in 2017–2018. Geophysical Res. Lett. 44, 11,624–11,635. doi: 10.1002/2017GL074904
Dokulil M., Skolaut C. (1986). Succession of phytoplankton in a deep stratifying lake: Mondsee, Austria. Hydrobiologia 138, 9–24. doi: 10.1007/BF00027229
Dupouy C., Frouin R., Tedetti M., Maillard M., Rodier M., Lombard F., et al. (2018). Diazotrophic Trichodesmium impact on UV–Vis radiance and pigment composition in the western tropical South Pacific. Biogeosciences 15, 5249–5269. doi: 10.5194/bg-15-5249-2018
Eppley R. W., Rogers J. N., Mccarthy J. J. (1969). Half-saturation constants for uptake of nitrate and ammonium by marine phytoplankton 1. Limnology oceanography 14, 912–920. doi: 10.4319/lo.1969.14.6.0912
Farias G. B., Molinero J.-C., Carré C., Bertrand A., Bec B., De Castro Melo P. A. M. (2022). Uncoupled changes in phytoplankton biomass and size structure in the western tropical Atlantic. J. Mar. Syst. 227, 103696. doi: 10.1016/j.jmarsys.2021.103696
Foster R. A., Kuypers M. M., Vagner T., Paerl R. W., Musat N., Zehr J. P. (2011). Nitrogen fixation and transfer in open ocean diatom–cyanobacterial symbioses. ISME J. 5, 1484–1493. doi: 10.1038/ismej.2011.26
Foster R., Subramaniam A., Mahaffey C., Carpenter E., Capone D., Zehr J. (2007). Influence of the Amazon River plume on distributions of free-living and symbiotic cyanobacteria in the western tropical North Atlantic Ocean. Limnology oceanography 52, 517–532. doi: 10.4319/lo.2007.52.2.0517
Gervais F. (1997). Light-dependent growth, dark survival, and glucose uptake by cryptophytes isolated from a freshwater chemocline. J. Phycology 33, 18–25. doi: 10.1111/j.0022-3646.1997.00018.x
Goes J. I., Do Rosario Gomes H., Chekalyuk A. M., Carpenter E. J., Montoya J. P., Coles V. J., et al. (2014). Influence of the Amazon River discharge on the biogeography of phytoplankton communities in the western tropical North Atlantic. Prog. Oceanography 120, 29–40. doi: 10.1016/j.pocean.2013.07.010
Hasle G. R., Syvertsen E. E. (1996). “Marine diatoms”, in Identifying Marine Phytoplankton ed. Tomas C. R. (San Diego, CA: Elsevier)), 5–385. doi: 10.1016/b978-012693015-3/50005-x
Haxo F., Fork D. (1959). Photoreactive pigments in flagellates: photosynthetically active accessory pigments of cryptomonads. Nature 184, 1051–1052. doi: 10.1038/1841051a0
Hernández-Hernández N., Arístegui J., Montero M. F., Velasco-Senovilla E., Baltar F., Marrero-Díaz Á., et al. (2020). Drivers of plankton distribution across mesoscale eddies at submesoscale range. Front. Mar. Sci. 7. doi: 10.3389/fmars.2020.00667
Honjo S., Manganini S. J., Krishfield R. A., Francois R. (2008). Particulate organic carbon fluxes to the ocean interior and factors controlling the biological pump: A synthesis of global sediment trap programs since 1983. Prog. Oceanography 76, 217–285. doi: 10.1016/j.pocean.2007.11.003
Howarth R. W. (1988). Nutrient limitation of net primary production in marine ecosystems. Annu. Rev. Ecol. Systematics 19, 89–110. doi: 10.1146/annurev.es.19.110188.000513
Kindt R., Coe R. (2005). Tree diversity analysis: a manual and software for common statistical methods for ecological and biodiversity studies (Nairobi: World Agroforestry Centre (ICRAF)).
Knap A., Michaels A., Close A., Ducklow H., Dickson A. (1996). Protocols for the joint global ocean flux study (JGOFS) core measurements. JGOFS, Reprint of the IOC Manuals and Guides No. 29 (Paris: UNESCO). 1994, 19.
Legendre P., Anderson M. J. (1999). Distance-based redundancy analysis: testing multispecies responses in multifactorial ecological experiments. Ecol. Monogr. 69, 1–24. doi: 10.1890/0012-9615(1999)069[0001:DBRATM]2.0.CO;2
Legendre P., Gallagher E. D. (2001). Ecologically meaningful transformations for ordination of species data. Oecologia 129, 271–280. doi: 10.1007/s004420100716
Legendre P., Oksanen J., Ter Braak C. J. (2011). Testing the significance of canonical axes in redundancy analysis. Methods Ecol. Evol. 2, 269–277. doi: 10.1111/j.2041-210X.2010.00078.x
Lévy M., Klein P., Treguier A.-M. (2001). Impact of sub-mesoscale physics on production and subduction of phytoplankton in an oligotrophic regime. J. Mar. Res. 59, 535–565. doi: 10.1357/002224001762842181
Lewitus A. J., Caron D. A. (1991). Physiological responses of phytoflagellates to dissolved organic substrate additions. 2. Dominant role of autotrophic nutrition in Pyrenomonas salina (Cryptophyceae). Plant Cell Physiol. 32, 791–801. doi: 10.1093/oxfordjournals.pcp.a078130
Li W. K., Head E. J., Harrison W. G. (2004). Macroecological limits of heterotrophic bacterial abundance in the ocean. Deep Sea Res. Part I: Oceanographic Res. Papers 51, 1529–1540. doi: 10.1016/j.dsr.2004.06.012
Liang Y.-C., Lo M.-H., Lan C.-W., Seo H., Ummenhofer C. C., Yeager S., et al. (2020). Amplified seasonal cycle in hydroclimate over the Amazon river basin and its plume region. Nat. Commun. 11, 4390. doi: 10.1038/s41467-020-18187-0
Louchard D., Münnich M., Gruber N. (2023). On the role of the amazon river for N 2 fixation in the Western Tropical Atlantic. Global Biogeochemical Cycles 37. doi: 10.1029/2022GB007537
Molleri G. S., Novo E. M. D. M., Kampel M. (2010). Space-time variability of the Amazon River plume based on satellite ocean color. Continental Shelf Res. 30, 342–352. doi: 10.1016/j.csr.2009.11.015
Moore L. R., Rocap G., Chisholm S. W. (1998). Physiology and molecular phylogeny of coexisting Prochlorococcus ecotypes. Nature 393, 464–467. doi: 10.1038/30965
Moura M. M., Dos Santos A. R., Pezzopane J. E. M., Alexandre R. S., Da Silva S. F., Pimentel S. M., et al. (2019). Relation of El Niño and La Niña phenomena to precipitation, evapotranspiration and temperature in the Amazon basin. Sci. Total Environ. 651, 1639–1651. doi: 10.1016/j.scitotenv.2018.09.242
Nittrouer C., Curtin T., Demaster D. (1986). Concentration and flux of suspended sediment on the Amazon continental shelf. Continental Shelf Res. 6, 151–174. doi: 10.1016/0278-4343(86)90058-0
Oksanen J., Guillaume Blanchet F., Friendly M., Kindt R., Legendre P., Mcglinn D., et al. (2020). vegan: community ecology package. R package version 2, pp. 5-2
Partensky F., Hess W. R., Vaulot D. (1999). Prochlorococcus, a marine photosynthetic prokaryote of global significance. Microbiol. Mol. Biol. Rev. 63, 106–127. doi: 10.1128/MMBR.63.1.106-127.1999
Platt T., Caverhill C., Sathyendranath S. (1991). Basin-scale estimates of oceanic primary production by remote sensing: The North Atlantic. J. Geophysical Research: Oceans 96, 15147–15159. doi: 10.1029/91JC01118
Raes E. J., Bodrossy L., Van De Kamp J., Bissett A., Ostrowski M., Brown M. V., et al. (2018). Oceanographic boundaries constrain microbial diversity gradients in the South Pacific Ocean. Proc. Natl. Acad. Sci. 115, E8266–E8275. doi: 10.1073/pnas.1719335115
Reygondeau G., Maury O., Beaugrand G., Fromentin J. M., Fonteneau A., Cury P. (2012). Biogeography of tuna and billfish communities. J. Biogeography 39, 114–129. doi: 10.1111/j.1365-2699.2011.02582.x
Shipe R. F., Curtaz J., Subramaniam A., Carpenter E. J., Capone D. G. (2006). Diatom biomass and productivity in oceanic and plume-influenced waters of the western tropical Atlantic ocean. Deep Sea Res. Part I: Oceanographic Res. Papers 53, 1320–1334. doi: 10.1016/j.dsr.2006.05.013
Smith W. O. Jr, Demaster D. J. (1996). Phytoplankton biomass and productivity in the Amazon River plume: correlation with seasonal river discharge. Continental Shelf Res. 16, 291–319. doi: 10.1016/0278-4343(95)00007-N
Smith W. O., Russell G. J. (1995). Phytoplankton biomass and nutrient distributions in the Amazon River plume: environmental correlates. Geo-Marine Lett. 15, 195–198. doi: 10.1007/BF01204463
Stukel M. R., Coles V. J., Brooks M., Hood R. R. (2014). Top-down, bottom-up and physical controls on diatom-diazotroph assemblage growth in the Amazon River plume. Biogeosciences 11, 3259–3278. doi: 10.5194/bg-11-3259-2014
Subramaniam A., Yager P., Carpenter E., Mahaffey C., Björkman K., Cooley S., et al. (2008). Amazon River enhances diazotrophy and carbon sequestration in the tropical North Atlantic Ocean. Proc. Natl. Acad. Sci. 105, 10460–10465. doi: 10.1073/pnas.0710279105
Sverdrup H. U., Johnson M. W., Fleming R. H. (1942). The Oceans: Their physics, chemistry, and general biology (New York: Prentice-Hall New York).
TEAM, R. C. (2013). R: A language and environment for statistical computing. Available at: https://www.r-project.org.
Van Heukelem L., Thomas C. S. (2001). Computer-assisted high-performance liquid chromatography method development with applications to the isolation and analysis of phytoplankton pigments. J. Chromatogr. A 910, 31–49. doi: 10.1016/S0378-4347(00)00603-4
Varona H., Veleda D., Silva M., Cintra M., Araujo M. (2019). Amazon River plume influence on Western Tropical Atlantic dynamic variability. Dynamics Atmospheres Oceans 85, 1–15. doi: 10.1016/j.dynatmoce.2018.10.002
Vidussi F., Claustre H., Manca B. B., Luchetta A., Marty J. C. (2001). Phytoplankton pigment distribution in relation to upper thermocline circulation in the eastern Mediterranean Sea during winter. J. Geophysical Research: Oceans 106, 19939–19956. doi: 10.1029/1999JC000308
Villareal T. A. (1989). Division cycles in the nitrogen-fixing Rhizosolenia (Bacillariophyceae)-Richelia (Nostocaceae) symbiosis. Br. Phycological J. 24, 357–365. doi: 10.1080/00071618900650371
Vrede T., Ballantyne A., Mille-Lindblom C., Algesten G., Gudasz C., Lindahl S., et al. (2009). Effects of N: P loading ratios on phytoplankton community composition, primary production and N fixation in a eutrophic lake. Freshw. Biol. 54, 331–344. doi: 10.1111/j.1365-2427.2008.02118.x
Weber S. C., Carpenter E. J., Coles V. J., Yager P. L., Goes J., Montoya J. P. (2017). Amazon River influence on nitrogen fixation and export production in the western tropical North Atlantic. Limnology Oceanography 62, 618–631. doi: 10.1002/lno.10448
Weber S. C., Subramaniam A., Montoya J. P., Doan-Nhu H., Nguyen-Ngoc L., Dippner J. W., et al. (2019). Habitat delineation in highly variable marine environments. Front. Mar. Sci. 6. doi: 10.3389/fmars.2019.00112
Woodd-Walker R. S., Ward P., Clarke A. (2002). Large-scale patterns in diversity and community structure of surface water copepods from the Atlantic Ocean. Mar. Ecol. Prog. Ser. 236, 189–203. doi: 10.3354/meps236189
Wright S., Jeffrey S., Mantoura R. (2005). Phytoplankton pigments in oceanography: guidelines to modern methods (Paris: Unesco Pub).
Wu R., Lin M., Sun H. (2020). Impacts of different types of El Niño and La Niña on northern tropical Atlantic sea surface temperature. Climate Dynamics 54, 4147–4167. doi: 10.1007/s00382-020-05220-7
Yeung L. Y., Berelson W. M., Young E. D., Prokopenko M. G., Rollins N., Coles V. J., et al. (2012). Impact of diatom-diazotroph associations on carbon export in the Amazon River plume. Geophysical Res. Lett. 39. doi: 10.1029/2012GL053356
Zapata M., Fraga S., Rodríguez F., Garrido J. L. (2012). Pigment-based chloroplast types in dinoflagellates. Mar. Ecol. Prog. Ser. 465, 33–52. doi: 10.3354/meps09879
Keywords: Amazon River plume, Western Tropical North Atlantic, phytoplankton, habitat classification, hierachical clustering
Citation: Pham AH, Choisnard N, Fernández-Carrera A, Subramaniam A, Strope EK, Carpenter EJ, Voss M and Montoya JP (2024) Planktonic habitats in the Amazon Plume region of the Western Tropical North Atlantic. Front. Mar. Sci. 11:1287497. doi: 10.3389/fmars.2024.1287497
Received: 01 September 2023; Accepted: 04 January 2024;
Published: 29 January 2024.
Edited by:
Alex J. Poulton, Heriot-Watt University, United StatesReviewed by:
Ana Paula B. Moreira, Federal University of Rio de Janeiro, BrazilHai Doan-Nhu, Institute of Oceanography in Nhatrang, Vietnam
Copyright © 2024 Pham, Choisnard, Fernández-Carrera, Subramaniam, Strope, Carpenter, Voss and Montoya. This is an open-access article distributed under the terms of the Creative Commons Attribution License (CC BY). The use, distribution or reproduction in other forums is permitted, provided the original author(s) and the copyright owner(s) are credited and that the original publication in this journal is cited, in accordance with accepted academic practice. No use, distribution or reproduction is permitted which does not comply with these terms.
*Correspondence: Anh H. Pham, YW5ocGhAZ2F0ZWNoLmVkdQ==
†Present address: Ana Fernández-Carrera, Oceanografía Biológica, Instituto de Oceanografía y Cambio Global (IOCAG), Universidad de Las Palmas de Gran Canaria, Las Palmas de Gran Canaria, Spain