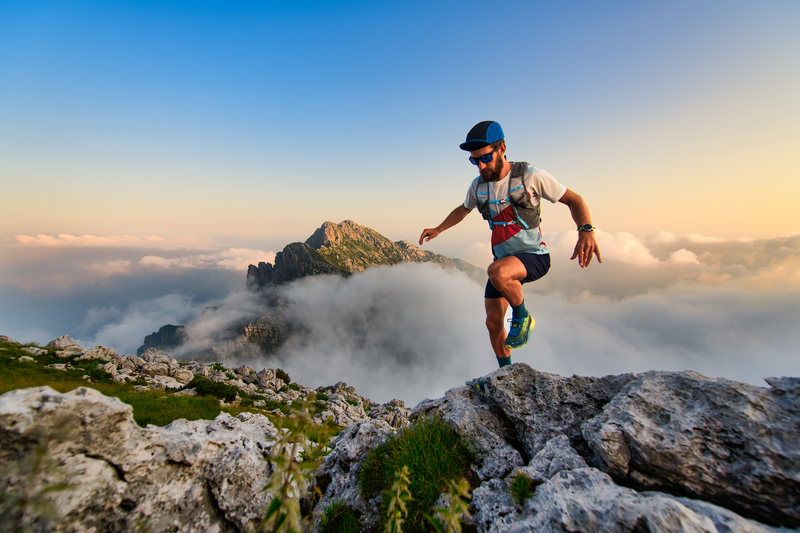
94% of researchers rate our articles as excellent or good
Learn more about the work of our research integrity team to safeguard the quality of each article we publish.
Find out more
ORIGINAL RESEARCH article
Front. Mar. Sci. , 04 January 2024
Sec. Marine Biogeochemistry
Volume 10 - 2023 | https://doi.org/10.3389/fmars.2023.1307218
The coastal environment in the Saemangeum area has experienced persistent physical stresses owing to the irregular operation of the sluice gates and related artificial disturbances since seawall construction, which has led to restricted freshwater-seawater circulation. To understand the impacts of stress, we performed long-term (1999-2022, 24 years) in situ measurements of relevant biotic and abiotic parameters and employed the random forest (RF) technique to determine the phytoplankton community response to environmental disturbance. Specifically, we estimated chlorophyll-a (Chl-a) concentrations using an RF model based on various environmental factors such as sea surface temperature (SST), sea surface salinity (SSS), dissolved oxygen saturation (DO), dissolved inorganic nitrogen (DIN), and dissolved inorganic phosphorus (DIP) as input variables. From the RF analysis, each environmental factor contributed to variation in Chl-a concentration as follows: SSS (42.91%), SST (17.88%), DIP (14.38%), DIN (13.36%), and DO (11.48%). In addition, we performed sensitivity experiments by altering the salinity, which was revealed to be the most influential environmental parameter. As a result, Chl-a concentration increased by approximately 1.79 times in lower salinity conditions (from 7 to 27 psu) compared to the normal salinity conditions prior to the seawall construction (from 12 to 32 psu) in both areas, including the inside and outside the seawall. More importantly, lower salinity conditions stimulated dinoflagellate blooms, that is, red tides, implying that restricted freshwater-seawater circulation could worsen coastal ecosystems. Thus, this study contributes to understanding the impacts of environmental changes caused by sluice gate manipulation on marine ecosystems, such as phytoplankton community dynamics. Moreover, this study recommends an ecologically suitable operation scheme for Saemangeum sluice gates to ensure a healthy coastal ecosystem.
In an estuarine region, the interplay between freshwater and seawater is influenced by dynamic physical environmental factors such as freshwater discharge and tidal flows (Ryther, 1969). Coastal ecosystems in estuarine regions are recognized as systems with high levels of biodiversity and productivity due to the nutrient supply from the land (McLusky and Elliott, 2004; Wetz et al., 2011; Cloern et al., 2014). It is also notable that estuarine areas are known to undergo eutrophication and algal blooms due to excessive nutrient input from the land (Paerl et al., 2014; Jeong and Yang, 2015). These areas are also stressed by changes in salinity (Telesh, 2004; Bianchi, 2007). Moreover, anthropogenic disturbances related to coastal development, including dam construction and the irregular exchange of freshwater and seawater, can significantly accelerate the physical stress in the coastal environment, including salinity differences (Park and Sin, 2022). Anthropogenic disturbances have the potential to induce unstable and unpredictable changes in coastal ecosystems, such as phytoplankton dynamics (Elliott and Quintino, 2007; Borja et al., 2010; Domingues et al., 2012). Recognizing that the first chain of the coastal ecosystem’s production system is primary production (Cloern et al., 2014), anthropogenic perturbations may directly lead to variations in phytoplankton biomass and community composition (Domingues et al., 2007; Choi et al., 2013). Ultimately, this affects the quantity and quality of higher trophic levels in the food web (Frederiksen et al., 2006; Kang et al., 2017), leading to changes in coastal ecosystem functioning.
The Saemangeum seawall is the longest man-made dike ever built globally, accounting for 33.9 km in length (Figure 1). The internal water of the seawall is affected by seawater (part of the Yellow Sea, YS) outside the seawall and influenced by two rivers (Dongjingang, and Mangyeonggang) inside the seawall (Lee and Ryu, 2008). After the completion of the seawall construction in 2006, the mixing of freshwater and seawater (i.e., estuary, brackish water) has been controlled through two sluice gates (Sinsi and Garyeok) on the southern part of the seawall (Figure 1B). When sluice gates are opened, ecological and hydrological changes occur due to the inflow of seawater from the outside and the outflow of freshwater from the inside, considering the tidal range and water depth of the mixing area, causing changes in the marine environment (Yang et al., 2008; Choi et al., 2013; Baek et al., 2019). The Saemangeum seawall sluice gates irregularly control freshwater and seawater exchange, contributing to increased environmental stress (Lee et al., 2009; Kim et al., 2020). In summer, when freshwater inflow is high owing to increased precipitation (Park and Sin, 2022), the influence of low salinity on freshwater becomes more pronounced, leading to strong stratification of internal waters (Kim et al., 2006; Oh and Choi, 2015). The outer region has an average salinity above 28 psu in the outer region, whereas the inner region has an average salinity below 25 psu. In addition, chlorophyll-a (Chl-a) concentration, used as a proxy for phytoplankton biomass, was nearly four times higher inside than outside the area. Choi et al. (2013) and Jeong and Yang (2015) reported a positive relationship between nutrients and Chl-a concentrations before and after seawall construction from 2006 to 2009 and 2002 to 2010, respectively. Interestingly, increased oxygen concentrations in internal waters are caused by phytoplankton photosynthesis (Choi et al., 2013). Conversely, other studies have reported that phytoplankton respiration consumes oxygen, contributing to decreased oxygen concentrations (Jeong and Yang, 2015).
Figure 1 Location of the Saemangeum seawall in South Korea adjacent to the coastal Yellow Sea (A) and the studied sampling stations (B). The blue and orange colored squares indicate the sites sampled outside and inside of the Saemangeum seawall, respectively. The bathymetry data was obtained from the GEBCO 2020 with 1-arc resolution.
Other studies in similar conditions have also articulated the environmental changes and addressed impacts driven by dike construction. Domingues et al. (2007) investigated the relationship between physicochemical environmental factors, Chl-a, and phytoplankton communities during the construction of the Alqueva Dam in the Guadiana River (Spain) from 2002 to 2003. They observed that an increase in nitrates led to an increase in Chl-a concentration and alterations in the composition of phytoplankton communities. Additional analyses for the same regions between 2007 and 2009 showed similar environmental changes to those observed by Domingues et al. (2007), while the phytoplankton community composition returned to prior conditions for dam construction (Domingues et al., 2012). These findings indicate that coastal ecosystems adapt to rapid environmental changes due to natural or anthropogenic disturbances, but challenges in identifying adaptation may begin with long-term and continuous data. Jiao et al. (2007) reported an increase in Chl-a related to reduced salinity during the construction of China’s Three Gorges Dam on the Yangtze River. Similarly, Wei et al. (2021) analyzed environmental changes in the same region during the summer seasons in 2008 and 2013, demonstrating that the combination of low salinity and high nitrate levels in the Yangtze River resulted in increased Chl-a and oxygen levels (Wei et al., 2021).
Despite scientific findings on the relationship between dike construction and its impact on the environment, these studies have focused on understanding the short-term ecological responses of coastal ecosystems based on the nutritional differences between freshwater and seawater (Jiao et al., 2007; Choi et al., 2013; Wei et al., 2021). However, two other limitations exist to understanding coastal ecosystem dynamics under anthropogenic disturbances. First, physical stresses, including stratification and river discharge, have not been considered in previous studies focusing on the nutritional perspective (Jiao et al., 2007; Choi et al., 2013). Second, the data for analysis were limited to short-term (Wei et al., 2021) and discontinuous data. Discontinuous or short-term (less than ten years) data constrain a comprehensive understanding of coastal environmental variations, such as phytoplankton adaption, shifts in phytoplankton communities, and changes in coastal ecosystem productivity. Therefore, it is essential to conduct long-term (over ten years) multiparametric data analyses, considering the complexity and variability of physical environmental changes driven by natural and anthropogenic interventions, to understand coastal ecosystems (Park and Sin, 2022). Many recent studies have employed machine learning (ML) techniques to explain the relationships between factors such as sea surface temperature (SST), nitrogen, and marine ecosystems. Park et al. (2015b) used an artificial neural network (ANN) model, specifically a support vector machine (SVM), to examine the relationship between nutrient levels (nitrate and phosphate) and phytoplankton blooms in the Nakdong River in South Korea (Kim et al., 2019). Park et al. (2020) employed a random forest (RF) model to quantify the relationships and influences of various environmental factors, including light and Chl-a.
Our study aimed to assess and quantify the long-term environmental and ecological impacts of the Saemangeum seawall construction off the west coast of the Korean Peninsula, focusing on the relationship between Chl-a and various environmental factors, including SST, salinity, and nutrients. First, we analyzed the main coastal environmental changes caused by the Saemangeum seawall structure using long-term (1999-2022) data (Section 3.1). We considered four conditions: before and after seawall construction and differences in circulation times for freshwater and seawater. Second, we assessed and explained the contribution of each environmental factor to the coastal ecosystems using the RF model (Section 3.2). Third, we simulated and examined the coastal ecosystem response to multivariate effects and the combined impacts of two or more factors on Chl-a (Section 3.3). Finally, we discuss the biological responses induced by environmental changes caused by long-term anthropogenic disturbances, such as shifts in phytoplankton community composition and the occurrence conditions for red tides caused by dinoflagellate blooms (Section 4).
The Saemangeum is a tidal flat located in the southwestern part of the Korean Peninsula, and the Saemangeum Seawall construction was built by a national project, the Saemangeum Reclamation Project, to reclaim regions and use them for agricultural purposes. The Saemangeum seawall is located where two rivers (Dongjingang and Mangyeonggang) meet the coastal YS and the western coast of Korea (Figure 1A). The YS affects the outside of the seawall (Jin et al., 2013). In contrast, the interior of the seawall is influenced by freshwater enriched with nutrients predominantly originating from land, including agricultural areas (approximately 50%) and forests (approximately 35%) (Jeong and Kwak, 2021). From April 2006 to December 2010, the sluice gates on the southern parts of the seawall remained open, allowing unrestricted interaction between freshwater and seawater. However, from January 2011 to August 2014, the sluice gates were opened selectively twice daily (daytime and nighttime) for one hour to manage the water levels within the inner area (Oh and Choi, 2015). The sluice gates were opened once daily for less than one hour between Sep. 2014 and Nov. 2020. Considering the water quality, the gates were opened twice daily (daytime and nighttime) (Oh and Choi, 2015). The bottom topography of the estuary inside the Saemangeum seawall is generally flat, with an average depth of approximately 20 m (Figure 1B) (Lee and Ryu, 2008; Oh and Choi, 2015; Jeong and Kwak, 2021).
In addition, the study area has a typical temperate climate and distinct seasonal variations in SST (Kim et al., 2020). It also has typical East Asian monsoon characteristics with precipitation. Based on the Korea Meteorological Administration (KMA) (https://data.kma.go.kr), the precipitation increases in summer (from July to September, 22.95 ± 8.20 cm) and decreasing in winter (from January to March, 3.81 ± 1.33 cm) (Wang et al., 2017) (Figure 2A). As a result, the Saemangeum seawall discharges a larger volume of freshwater during the summer (63.95 ± 34.62 m3s-1) than during winter (20.86 ± 8.83 m3s-1) (Oh and Choi, 2015; Cho et al., 2020) (Figure 2B). Moreover, freshwater causes lower sea surface density, resulting in strong stratification inside and outside the Saemangeum seawall (Oh and Choi, 2015).
Figure 2 Time series of monthly averaged precipitation (A), discharge (B) from 2013 to 2022. The pink and blue shade shows the winter season (from January to March) and summer season (from July to September), respectively. In B, over the zero (0) means seawater, likes outside in the seawall, inflow into the freshwater, likes inside in the seawall. Conversely, under zero (0) indicates freshwater outflow to seawater.
The SST and sea surface salinity (SSS) were measured on-site using a handheld multiparameter meter (YSI, model Pro Quatro). Dissolved oxygen saturation (DO), essential for phytoplankton respiration and growth, was measured using the YSI and converted into dissolved oxygen saturation (%) to eliminate the potential effects of seasonal water temperature and salinity variations. Dissolved inorganic nitrogen DIN (NH4+ + NO3- + NO2-) and dissolved inorganic phosphorus (DIP, PO43-) were analyzed as nutrient sources for phytoplankton. The DIN and DIP concentrations were measured using absorption spectrometry with sulfanilamide, sodium nitroprusside, and potassium persulfate methods, respectively (Armstrong et al., 1967; Jeong and Kwak, 2021). Chl-a was measured in the water samples. The collected water samples (500 mL) were passed through GF/F filters with a diameter of 47 mm. The filters were then placed in 90% acetone and stored in a dark room for 24 h before measurement with a fluorescence spectrophotometer (provided by Water and Eco-Bio Co., Ltd.). In addition, phytoplankton abundance and species composition were analyzed in samples collected between 2019 and 2022 and preserved in acidic Lugol’s solution using the settling and siphoning method (Welch, 1948). After thorough mixing, each phytoplankton species in 1 mL Sedgwick–Rafter counting chambers was counted under an optical microscope.
Outside the Saemangeum seawall, observations collected in February, May, August, and November from 1999 to 2022 were obtained from the Korea Marine Environment Management Corporation (KOEM) under the Ministry of Oceans and Fisheries (https://www.meis.go.kr). Monthly environmental factors inside the Saemangeum seawall from 2012 to 2022 were obtained from the Saemangeum Area Integrated Environmental Management System (SAIEMS), managed by the Ministry of Environment (https://www.eariul.go.kr) (Figure 1B; Table 1). Irregularly acquired data from 2014 to 2022 were provided by Water & Eco-Bio Co. Ltd. (Figure 1B; Table 1).
Table 1 Description of the in-situ measurement, including sea surface temperature (SST), sea surface salinity (SSS), dissolved oxygen saturation (DO), dissolved inorganic nitrogen (DIN), dissolved inorganic phosphorus (DIP), and chlorophyll-a concentration (Chl-a).
A total of 2,341 samples were collected, with 68% representing surface depths (shallower than 5 meters) and 32% representing bottom depths (deeper than 5 meters), following the regulations set forth by the Ministry of Oceans and Fisheries of Korea (MOF) and the Ministry of Land, Infrastructure and Transport (MOLIT). Out of these, 1,162 samples pertain to the period between 2012 and 2022 within the inner Saemangeum seawall, while 1,179 samples correspond to the period from 1999 to 2022 outside the seawall. It’s worth noting that salinity values between the surface and bottom layers exhibited similarity at most stations (though not shown), indicating well mixed within both the water masses in the inner and outer areas of the seawall (Kim et al., 2006; Jeong and Kwak, 2021). In the scope of this study, our primary focus is to investigate potential coastal ecological changes that might be associated with the construction of the Saemangeum seawall. To this end, we organized the spatially sampled data, with external samples representing a marine environment and internal samples representing a blend of freshwater and seawater circulation environments. The data underwent monthly averaging to mitigate the influence of irregular observation dates, following the methodology outlined by Oda et al. in 2018.
The RF model is a tree-based algorithm with high randomness that minimizes errors and enhances performance. The randomness in the RF model plays a critical role in preventing overfitting and enhancing the prediction performance. The model was constructed by setting two essential parameters: the number of single decision trees (Ntrees) and the number of features (Mtry) (Baek et al., 2022). Each tree randomly selects a subset of the dataset and chooses the best-split function from the selected subset to split the nodes (Park et al., 2020). Finally, the results averaged for each tree were assembled to conclude. Thus, Ntrees and Mtry are significant parameters in the RF model. In this study, we employed an empirical trial to set Ntrees to 52 single decision trees, whereas Mtry was set to five, based on the convention (Baek et al., 2022). Five variables (predictors) were used as inputs for the RF model. The predictors were the SSS and other environmental factors (SST, DO, DIN, and DIP). We determined the significance of the predictors using the Boruta algorithm, and all input data were normalized using the Z-score method. A 5-fold cross-validation method was used to evaluate the accuracy of the RF model. For the model training set, 60% (1404) of the total data were used, while 20% (468) were used for the validation set, and the remaining 20% (469) were used as the test set.
Several statistical metrics were used to evaluate the performance of the model, including the Pearson correlation coefficient (r), coefficient of determination (R2), root mean square error (RMSE), and percentage model bias (P-bias). r displays the relationship between the in-situ and predicted values, R2 represents the index of variability that the model can explain, and RMSE indicates the difference between the observed and predicted values. Finally, P-bias assesses whether the model underestimates or overestimates observations (Bae and Seo, 2018; Liao et al., 2021). A strong correlation is indicated when r > 0.6 (Liao et al., 2021), a value of R2 closer to 1, and a smaller value of RMSE indicates a better model fit to the observed data (Li et al., 2013). The P-bias indicates the model’s bias, such that values closer to zero suggest a lower bias. A P-bias negative value implied an underestimation of the observed values by the model, whereas a positive value indicated an overestimation (Bae and Seo, 2018).
To offer understandable and reasonable explanations for the ML model developed in this study, we used explainable artificial intelligence (XAI) techniques, such as variable importance (VI), the Shapley additive explanations (SHAP) approach, and partial dependence (PD) plots. The VI is a method provided by the RF model to calculate the impact of each predictor on the target variable (Breiman, 2001). The VI indicates the extent to which a model’s prediction accuracy is affected by removing a particular variable (Cutler et al., 2007). VI is a normalized value calculated by assigning scores to each feature after the model has completed training, with a sum of 1. Hence, the closer a variable’s VI value is to 1, the more essential it is for the model’s prediction. SHAP explains the impact of multiple predictors on outcomes using game theory to analyze causal relationships using modern ML techniques (Lundberg and Lee, 2017). The limitations of inherent feature importance information, such as VI, in RF models, are compensated for by utilizing SHAP values (Jang et al., 2022). The SHAP value for the input variables was the weighted average of the marginal contribution of the input variable (Eq. (1)).
where φi represents the SHAP value of the i-th input variable, F indicates the set of all input variables, and S represents all the subsets without the i-th input variable. Additionally, the subset S without the i-th input variable, and represents the dataset that includes the i-th input variable. where shows the predicted value based on . For further information, please refer to the study by Lundberg and Lee (2017). The SHAP values were calculated using Eq. (1) can indicate the contribution of each input feature to the target and its correlation and responsiveness, including the value and direction (positive or negative) of the output factor for the condition range of each input factor (Mangalathu et al., 2020; Rodríguez-Pérez and Bajorath, 2020; Smith and Alvarez, 2021). An optimized Tree SHAP was used to explain the RF model (Mangalathu et al., 2020; Rodríguez-Pérez and Bajorath, 2020).
Finally, the PD plots help interpret the prediction results of the models because they visualize the interactions between variables and explain the model results (Friedman, 2001; Park et al., 2022). In particular, PD plots show how the model’s prediction reacts to changes in the values of one or two variables (Baek et al., 2022). Thus, we can identify how a variable affects the model’s prediction and understand its operational mechanisms. The PD plot analysis was performed as follows:
where represents the variable visualized through PD plots, and denotes the variable used in the RF model (, estimated value). In general, Xs composes one or two factors and shows changes in the predicted values for complex effects among multiple variables. Set S is the set of variables desired to identify partial dependence. The PD plots for set S were calculated by designating a specific variable of and averaging all values of , which are the other variables. For further details, refer to Baek et al. (2022).
We examined the environmental changes in the outer area of the Saemangeum seawall from 1999 to 2010, when freshwater and seawater exchanges were unrestricted (Figure 3; Table 2). The average SST was 14.15 ± 7.90°C, increasing to 0.83°C from 1999 to 2010 (Figure 3A). The average SSS maintained a stable 31.29 ± 0.80 psu regardless of the season. Although there was a slight increase of 0.12 psu in SSS during the same period (1999-2010), the difference was not statistically significant (Figure 3B). The mean DO was 103.45 ± 12.97%, with an increase of 1.91%p in the same period (Figure 3C). The average DIN from 1999 to 2010 was 143.14 ± 111.50 mg m-3, while the mean DIP was 19.24 ± 13.11 mg m-3. Unlike other environmental factors, DIN showed a decreasing trend of 43.11 mg m-3, and DIP showed a decrease of 2.42 mg m-3 over time (Figures 3D, E). The average Chl-a concentration was 2.76 ± 2.85 mg m-3, gradually increasing during the same period, with approximately 0.32 mg m-3 (Figure 3F).
Figure 3 Time series of spatially monthly averaged SST (A), SSS (B), DO (C), DIN (D), DIP (E), and Chl-a (F) are shown for the sampled period from 1999 to 2010. The blue bar and blue dotted line indicate each parameter value and trend. The black line shows the changed environmental conditions that completed seawall construction.
Table 2 Index of the six features statistics values from 1999 to 2010. The positive sign (+) as indicating ‘increased trend’ and the negative sign (-) as indicating ‘decreased trend’ from 1999 to 2010.
Figure 4A shows that the increasing SST trend from 2011 to 2022 inside the Saemangeum seawall was higher than outside (Table 3). The overall average SST in the outer area is 15.48 ± 7.98°C, exhibiting an increase of around 1.48°C (Table 3). On average, SST in the inner area is 14.43 ± 8.58°C and has increased by approximately 2.13°C. Figure 5A shows the seasonal SST changes in both areas inside and outside the seawall, clearly depicting the continuous increase in temperature from winter (from January to March) to summer (from July to September). In the outer area, the average seasonal temperature ranged from 5.46 ± 1.75°C in winter to 25.14 ± 1.79°C in summer. In the inner area, the average SST is 4.51 ± 2.36°C in winter and 26.12 ± 2.32°C in summer (Figure 5A). Both regions demonstrate typical seasonal variations characteristic of temperate climate zones, with the inner area experiencing a higher rate of temperature increase than the outer area. In addition, the SST warming trend in both regions increased by more than twice from 2011 to 2022 compared with the previous period (1999-2010).
Figure 4 The spatially monthly averaged changes in SST (A), SSS (B), DO (C), DIN (D), DIP (E), and Chl-a (F) are depicted from 2011 to 2022 on the outside and inside of the Saemangeum seawall. The blue square and dotted line show each variable value and trend the sampled outside the seawall, respectively. The orange circle and dotted line present values and tendencies of environmental factors inside the seawall, respectively. The green and red triangles indicated in 2017 and 2019, respectively. The orange shaded represents the period during which the sluice gates were scheduled to open and close only once per day (from September 2014 to November 2020).
Table 3 Index of the six variables statistics values divided to inside and outside of the Saemangeum seawall from 2011 to 2022.
Figure 5 Time series of monthly means of SST (A), SSS (B), DO (C), DIN (D), DIP (E), and Chl-a (F) inside and outside the Saemangeum seawall from 2011 to 2022. The blue square and orange circle indicate the monthly averaged value for each parameter inside and outside the seawall, respectively. The pink and blue shade shows the winter season (from January to March) and summer season (from July to September), respectively.
The average SSS is 30.91 ± 0.90 psu, and the SSS trend exhibited a slight decrease of 0.31 psu in the outer area from 2011 to 2022 (Figure 4B). Similarly, the inner area averaged SSS is 24.59 ± 4.83 psu, and the SSS tendency decreased by 2.77 psu, which shows a drastic change compared to the slight increase of 0.12 psu from 1999 to 2010. Notably, the inner and outer areas showed exceptionally low salinity values in 2018 and 2020 compared to other years (Figure 4B). Additionally, SSS showed a distinct seasonal variation opposite to that of SST, decreasing in summer and increasing again in winter (Figure 5B). In the outer region, conditions were characterized by seawater, with salinity changes decreasing from 31.30 ± 0.28 psu in winter to 30.73 ± 0.10 psu in summer (Kim et al., 2020). The inner part conditions as brackish water showed a more unstable pattern in terms of salinity variations compared to the outer area, with average winter salinity from 29.08 ± 0.94 psu to 20.10 ± 0.21 psu in the summer season (Figure 5B).
Both regions, including the outside and inside of the seawall, exhibit average DO levels of 98.42 ± 13.09% and 99.70 ± 15.78%, respectively (Figure 4C). Based on these results, the outer and inner areas displayed decreasing trends of 2.77% and 6.09%, respectively (Figure 4C). Moreover, the outer area of average seasonal DO ranged from 110.07 ± 6.30% in winter to 89.39 ± 6.85% in summer (Figure 5C). In inner areas, the seasonal average DO value from 2012 to 2022 during winter was 101.75 ± 3.38%, while during summer, it slightly decreased to 94.70 ± 6.40%, indicating a lower saturation level compared to winter (Figure 5C).
The average DIN concentration in the outer area was approximately 106.56 ± 84.42 mg m-3, while in the inner area, it is around 222.25 ± 132.31 mg m-3, indicating that the inner area has roughly double the nutrient level compared to the outer area (Figure 4D). Similar to DO, DIN also showed a decreasing trend in the outer and inner areas, at approximately 10.99 mg m-3 and 84.62 mg m-3, respectively. The inner and outer areas exhibited low DIN values in 2017 and 2019, respectively. In the case of seasonal variation, in the outer area, the average DIN concentration during winter and summer is about 135.77 ± 65.04 mg m-3 and 75.57 ± 25.79 mg m-3, respectively (Figure 5D). On the other hand, in the inner area, the average DIN level during winter and summer is approximately 232.93 ± 44.36 mg m-3 and 195.65 ± 40.11 mg m-3, respectively, with both regions exhibiting higher DIN concentrations during winter than summer (Figure 5D).
The average DIP concentration in the inner and outer areas is around 16.61 ± 10.68 mg m-3 and 14.57 ± 8.77 mg m-3, respectively, indicating a slight difference from DIN levels (Figures 4D, E). DIP levels showed an increasing trend of approximately 3.78 mg m-3 in the outer area, while in the inner area, it showed a decreasing trend of around 10.50 mg m-3, indicating contrasting patterns. In particular, the inner area exhibited higher DIP values in 2017, whereas the outer area showed lower DIP values in 2019, in contrast to the DIN values (Figure 4E). As in Figure 4E, the average DIP levels during winter and summer in the outer area are approximately 12.50 ± 3.27 mg m-3 and 13.54 ± 2.55 mg m-3, respectively (Figure 5E). In the inner area, the average DIP levels during winter and summer are approximately 9.42 ± 2.59 mg m-3 and 15.95 ± 3.02 mg m-3, respectively, with significant seasonal differences observed (Figure 5E). In contrast to DIN, DIP levels were higher in summer than in winter.
The average Chl-a in the outer and inner regions of the seawall is approximately 5.97 ± 4.03 mg m-3 and 13.81 ± 8.54 mg m-3, respectively, with the inner area having more than twice the values compared to the outer area (Figure 4F). The Chl-a concentration in the outer part showed an average decrease of 1.83 mg m-3, whereas that in the inner area represented an average increase of 4.68 mg m-3. Chl-a concentrations were generally higher inside than outside the seawall during the entire time series but were more pronounced during the period during which the sluice gates were only opened once per day (Figure 4F). The highest concentrations in the inner region were over 30 mg m-3 in 2019 and 2020, and the Chl-a levels were exceptionally low in 2017. In contrast, the Chl-a values in the outer area were similar to those in other years. Additionally, the average Chl-a concentration in the outer area during winter and summer is approximately 9.22 ± 0.37 mg m-3 and 5.11 ± 0.91 mg m-3, respectively (Figure 5F). In the inner area, the average Chl-a during winter and summer is approximately 9.42 ± 2.59 mg m-3 and 15.95 ± 3.02 mg m-3, respectively, indicating a higher concentration during both seasons. Interestingly, Chl-a exhibited distinct spatial and seasonal bloom patterns, with the inner area showing summer blooms and the outer area showing winter blooms (Figures 4F and 5F).
To investigate the environmental changes associated with variations in sluice gate opening times within the Saemangeum seawall, we examined fluctuations in relevant abiotic and biotic factors, including Chl-a, across three distinct periods: Period 1 (P1, from January 2011 to August 2014, with the sluice gate opened twice daily), Period 2 (P2, from September 2014 to November 2020, with the sluice gate opened once per day), and Period 3 (P3, from December 2020 to December 2022, with the sluice gate opened twice per day). The average SST in the outer areas during P1, P2, and P3 was 14.94 ± 8.87 °C, 15.72 ± 7.84 °C, and 14.92 ± 8.21 °C, respectively. In the inner areas, the mean SST for P1, P2, and P3 was 13.65 ± 9.11 °C, 14.42 ± 8.47 °C, and 15.29 ± 8.59 °C, respectively. Notably, SST in the outer areas exhibited a gradual increase, although no significant spatial or temporal differences were observed. Regarding SSS, the average values for the outer areas during P1, P2, and P3 were 30.83 ± 0.92 psu, 30.94 ± 0.95 psu, and 30.83 ± 0.69 psu, respectively. In the inner areas, the averaged SSS for P1, P2, and P3 was 26.98 ± 3.56 psu, 23.46 ± 4.98 psu, and 25.02 ± 4.74 psu, respectively. It is worth noting that the SSS inside and outside the seawall displayed significant differences concerning the sluice gate opening. Furthermore, the average DO levels in the outer areas over the same periods were 105.17 ± 9.10%, 96.25 ± 13.10%, and 101.70 ± 13.84%, respectively. In the inner areas, the average DO for P1, P2, and P3 was 96.01 ± 17.95%, 102.26 ± 16.70%, and 96.80 ± 8.08%, respectively. However, no notable changes in DO, which remained nearly saturated at all times, were associated with the operation of the sluice gate.
In P1, the average DIN concentrations in the outer and inner areas were 67.41 ± 61.82 mg m-3 and 251.23 ± 111.65 mg m-3, respectively. In addition, the averaged DIN concentrations for P2 were 118.53 ± 78.77 mg m-3 and 223.27 ± 149.00 mg m-3 in the outer and inner areas, respectively. In P3, the averaged DIN values were 89.97 ± 66.44 mg m-3 and 188.21 ± 96.78 mg m-3 in the outer and inner areas, indicating significant spatial and temporal differences variation. In the case of the DIP, the average DIP concentrations during P1 were 9.21 ± 7.78 mg m-3 and 14.50 ± 6.94 mg m-3 in the outer and inner areas, respectively. In P2, DIP values were 15.86 ± 8.62 mg m-3 and 19.51 ± 11.48 mg m-3 on the outside and inside of the seawall, respectively. In P3, DIP levels were 11.96 ± 8.31 mg m-3 in the outer area and 8.94 ± 7.84 mg m-3 in the inner area, respectively, showing a clear temporal difference in both areas. Chl-a concentration depicted outside averages of 6.36 ± 3.69 mg m-3, 6.31 ± 4.25 mg m-3, and 4.24 ± 2.97 mg m-3 during P1, P2, and P3, respectively. In averaged Chl-a concentration, higher values in the inner area with averages of 10.15 ± 4.86 mg m-3, 15.66 ± 9.83 mg m-3, and 13.31 ± 6.53 mg m-3 in P1, P2, and P3, respectively. The Chl-a concentration changed more in the inner than the outer area according to temporal variations.
The scatter plots in Figures 6A, B illustrate the RF model performance of the validation and test sets for the in situ and predicted Chl-a. The RF model training set showed r = 0.97, R2 = 0.93, RMSEChl-a = 1.91 mg m-3 (p<.001) (not shown). The validation set (Figure 6A) had r = 0.78, R2 = 0.62, RMSEChl-a = 4.02 mg m-3 (p<.001), and the test set (Figure 6B) had r = 0.73, R2 = 0.54, RMSEChl-a = 3.73 mg m-3 (p<.001), respectively. Figures 6A and 6B display a slightly different pattern, where the values were divided into two groups centered around the Chl-a values of 10 mg m-3. The left group in Figure 6B accurately represents the Chl-a levels outside the Saemangeum seawall, whereas the right group effectively captures the higher Chl-a levels inside the seawall (Figures 3F and 6B; Tables 2, 3). Furthermore, upon evaluating the P-bias, which indicates the model’s bias, it was found that the predicted Chl-a predicted by the model was underestimated by 10.85% (training), 14.03% (validation), and 18.54% (test) compared with the in-situ Chl-a, respectively. The RF model slightly underestimated the Chl-a values higher than 31 mg m-3 because of the limited number of training data points, with only 82 (3.50%) out of 2,341 data. Although the performance of the validation and test sets decreased slightly compared to the training results, all cases showed a high correlation between predicted and in-situ Chl-a with r > 0.6 and R2 > 0.5, and P-bias was also very low (below 20%). Based on the prediction model, a performance table presented by Bae and Seo (2018), the R2 of our model was at a good level, and the P-bias was at a good, confirming that the RF model performed well (Liao et al., 2021).
Figure 6 Scatter plots of probability density estimate (PDE) compared between in-situ Chl-a and estimated Chl-a performance evaluation of a model for validation set (A) and test set (B). SHAP values of each parameter show an impact on Chl-a (C). The feature value colors signify each environmental parameter’s value, from low (blue) to high (red). In addition, each factor order shows by high contribution to estimated Chl-a.
We used the VI provided by the RF model to identify the importance of each parameter affecting Chl-a levels. The relative contribution of SSS was the highest at 42.91 ± 2.81%, followed by SST (17.88 ± 2.26%), DIP (14.38 ± 2.67%), DIN (13.36 ± 2.45%), and DO (11.48 ± 1.88%). The SHAP analysis performed to examine the quantitative relationships between individual factors and Chl-a yielded the same results as those obtained with the VI, as shown in Figure 6C. As the SHAP value on the x-axis became positive, the likelihood of the corresponding parameter contributing to an increase in Chl-a concentration increased. SSS was found to have the greatest impact on the estimated Chl-a, as shown by the highest negative correlation among the parameters (Figure 6C). In the SSS case, when the SHAP value is above 0, the mean SSS is 25.47 ± 4.51 psu, and the corresponding Chl-a is 14.01 ± 10.59 mg m-3. When the SHAP value is below 0, the average SSS is 31.18 ± 0.81 psu, and the Chl-a is 4.47 ± 4.19 mg m-3. Furthermore, the SHAP value is over 0.25 (tipping point in the feature value of SSS), an average SSS of 22.85 ± 4.31 psu, and the Chl-a is 17.53 ± 11.70 mg m-3. SST was the second most important factor in estimating Chl-a, which was also negatively correlated with Chl-a. In the SHAP value is above 0, the average SST is 9.10 ± 8.12°C, and the corresponding Chl-a is 11.09 ± 9.94 mg m-3. When the SHAP value is below 0, the average SST is 17.70 ± 6.04°C, and the Chl-a is 7.33 ± 8.16 mg m-3. Especially, SHAP value is less than -0.30, with an average SST at 2.49 ± 3.51°C, and the Chl-a is 0.55 ± 0.71 mg m-3, which indicates remarkably low temperature and Chl-a (Figure 6C).
DIP was the third most significant factor affecting the variation in Chl-a, which exhibited a negative correlation. For DIP concentrations, when the SHAP value is higher than 0, the average DIP concentrations are 5.95 ± 5.18 mg m-3, and the corresponding Chl-a level is 10.20 ± 9.65 mg m-3. When the SHAP value is under 0, the mean DIP value is 20.90 ± 11.06 mg m-3, and the Chl-a is 6.35 ± 7.42 mg m-3. In the case of DIN similar to DIP (Figure 6C), the SHAP value is higher than 0, the average DIN concentrations are 68.96 ± 61.69 mg m-3, and the corresponding Chl-a is 10.40 ± 9.73 mg m-3. Conversely, the SHAP value is lower than 0, the average DIN concentrations are 221.79 ± 125.41 mg m-3, and the corresponding Chl-a is 6.48 ± 7.51 mg m-3. Thus, as DIP and DIN decreased, Chl-a increased, resulting in a negative relationship (Morais et al., 2009; Zhou et al., 2017). The final contributor was DO, which is associated with phytoplankton respiration and photosynthesis. It was positively correlated with Chl-a. When the SHAP value in DO exceeds 0, the average DO is 103.57 ± 18.83%, and the corresponding Chl-a is 11.32 ± 10.53 mg m-3. When the SHAP value is below 0, the average DO is 96.12 ± 15.26%, with Chl-a at 6.41 ± 6.78 mg m-3. Consequently, the RF model can reveal the oceanic physicochemical relationships between the five environmental variables and Chl-a through the VI and SHAP approaches.
In Section 3.2, we found that salinity was the most influential driving factor of Chl-a variation. To further investigate the effect of SSS on Chl-a, we designed a sensitivity experiment using the RF model under three conditions: (1) SSS-5 psu, (2) SSS, and (3) SSS+5 psu (Figure 7; Table 4). The 5 psu means the difference between the salinity levels between the brackish water (average SSS at 26.25 ± 4.63 psu) and seawater (averaged SSS at 31.14 ± 1.11 psu) from 1999 to 2022. Hence, we simulated the estuarine salinity condition by reducing it by 5 psu (Case 1) and oceanic salinity condition by increasing it by 5 psu (Case 3) from the original condition. The original salinity condition (Case 2) has ranged from approximately 24 to 32 psu (averaged SSS 28.71 ± 4.15 psu), including the lowest value (12.44 psu) and the highest value (38.42 psu), with a mean Chl-a at 8.59 ± 8.99 mg m-3. Figure 7 shows the experimental results. In Case 1, when the SSS was reduced to 5 psu, the mean salinity distribution was approximately 19–27 psu (Figure 7; Table 4). At this time, Chl-a responded more sensitively, with mean values of 15.41 ± 6.71 mg m-3, about 1.79 times higher than the original condition. Conversely, when the SSS was increased for 5 psu (case 3), the mean salinity range was approximately 29 to 37 psu, with mean Chl-a of 4.22 ± 4.53 mg m-3, about half of the original condition’s levels at case 2. In the outside seawall (seawater), when SSS was decreased (maintaining SST, DO, DIN, and DIP levels at the original conditions), there was a notable increase in Chl-a. However, despite having nutrient-rich conditions in the estuary compared to the outer area, there was a decrease in Chl-a when the SSS was increased. Therefore, these results support that SSS has a negative correlation between SSS and Chl-a (Figure 7).
Figure 7 Sensitivity experiment shows Chl-a according to SSS. The test conditions include (1) a 5 psu decrease in the normal SSS (orange square and red line), (2) the SSS value (grey star and black dashed line), and (3) a 5psu increase in the normal SSS (blue circle and blue line).
Table 4 The estimated Chl-a levels according to changes in salinity condition through sensitivity test. The experiment under three conditions: SSS-5 psu (1), SSS (2), and SSS+5 psu (3).
Additionally, we identified the dominant phytoplankton communities, which are driving the decrease in Chl-a when salinity increases in the study area from 2019 to 2022 in spring (April) and summer (August). Figure 8 shows the phytoplankton abundance in each community, both inside and outside the seawall. During the research period, the phytoplankton community could be broadly classified into eight categories, including the communities of Bacillariophyceae (Baci), Dinophyceae (Dino), Raphidomonadea (Raph), Cryptophyceae (Cryp), Dictyochophyceae (Dict), Chlorophyceae (Chlo), Cyanophyceae (Cyan), and Euglenophyceae (Eugl) in both regions, as reported by Choi et al. (2013). Among the various phytoplankton communities, Baci and Dino show very high abundances compared to the other communities.
Figure 8 Variation in the phytoplankton community’s abundance inside of the seawall in April (A), in August (C), and outside of the seawall in April (B), in August (D) from 2019 to 2022. The orange shaded is restricted freshwater and seawater circulation period, and each color dotted line means total phytoplankton abundance. The bar of blue, yellow, orange, pink, cyan, crimson, brown, and purple indicate phytoplankton communities, such as Bacillariophyceae (Baci.), Dinophyceae (Dino.), Raphidomonadea (Raph.), Cryptophyceae (Cryp.), Dictyochophyceae (Dict.), Chlorophyceae (Chlo.), Cyanophyceae (Cyan.), and Euglenophyceae (Eugl.), respectively.
In the inside during spring seasons of both 2019 and 2020, there was an internal dominance of dinoflagellates, constituting 99.90% and 74.66% of the total phytoplankton abundance, respectively (Figure 8A). In the summer seasons of 2019 and 2020, diatoms contributed to phytoplankton community composition at 71.06% and 92.30%, respectively (Figure 8C). The outside environment also showed that diatoms dominated over dinoflagellates (Figures 8B, D). In the case of outside, similar to inside, dinoflagellates dominated in Apr. 2019 and 2020 at 98.51% and 84.97%, respectively. Diatoms also was most frequently represented in Aug. 2019 and 2020, at 84.95% and 97.78%, respectively. These results suggest that dinoflagellates dominate the spring blooms (Choi et al., 2013), whereas diatoms prevail during summer blooms. Spatially, the outside of the Saemangeum seawall, such as seawater, has a high diatom abundance, and the inside of the seawall, such as brackish water, has a greater abundance of dinoflagellates. However, when mixing increased to twice daily in 2020, the dominant phytoplankton community shifted from dinoflagellates to diatoms.
We provide the first comprehensive analysis of long-term (1999-2022) environmental changes due to artificial disturbances in the inner and outer areas of the Saemangeum Seawall (Figures 3–5; Tables 2, 3). Despite previous efforts to understand ecological changes in coastal ecosystems resulting from anthropogenic disturbances, most studies have focused only on short-term investigations, providing limited insights into short-term brackish water environmental changes (Lie et al., 2008; Lee et al., 2009; Oh and Choi, 2015). Consequently, we examined the spatiotemporal distributions of the long-term data, including four periods: unrestricted exchange of freshwater and seawater (1999-2010), Period 1 (P1, from January 2011 to August 2014), Period 2 (P2, from September 2014 to November 2020), and Period 3 (P3, from December 2020 to December 2022). We found that salinity was spatially and temporally predominant due to artificial disturbances, playing a dominant role in Chl-a level changes. Ultimately, the analysis of long-term data represents the environmental changes in the study area and emphasizes the essential consideration of salinity in Chl-a estimation.
Using long-term data analysis, we inferred three potential impacts on coastal environmental changes, including differences in freshwater residence time depending on the sluice gate operation, global warming, and land-based activities.
First, irregular sluice gate operations can influence the difference in freshwater residence time (Oda et al., 2018; Jeong and Kwak, 2021), leading to notable disparities in environmental conditions between the inner and outer areas of the seawall. We observed an intensified spatial salinity and nutrient gradient during P2, characterized by longer freshwater residence times than those in P1 and P3. The contrasting salinity patterns in the inner and outer areas suggest that the dominant influence of freshwater is controlled by the opening time of the sluice gate (Yang et al., 2008; Kim et al., 2020). Similar to salinity, nutrients (DIN and DIP) showed higher accumulation in the inner area than in the outer area, with longer freshwater residence times (Yang et al., 2008; Boyer et al., 2009; Kim et al., 2013; Sin et al., 2013). As a result, Chl-a showed a high concentration during P2, while the Chl-a concentration decreased toward P3 when the residence time of the freshwater decreased again. In conclusion, the operation of the sluice gate contributed to the determination of environmental changes in the study area. This has a significant effect on nutrient salinity and phytoplankton dynamics. It provides evidence of the association between freshwater residence time and the basis of coastal ecosystem based on the findings of this study (Moradi and Moradi, 2020). Thus, selecting the appropriate timing for sluice gate operation helps to enhance the ecological efficiency of the coastal ecosystem in the study area.
Second, warming trends indirectly affect physical coastal environmental changes. SST demonstrated a gradual increase in the inner and outer regions of the seawall (Figures 3A and 4A; Tables 2 and 3). The average SST warming trend was 0.14°C yr-1 from 1999 to 2022, with the outer area showing 0.10°C yr-1 and the inner area showing 0.19°C yr-1, indicating a higher warming rate on the inside compared to the outside of the seawall (Kim et al., 2020). This research area warming trend may be affected by the global warming effect in the YS, where an average temperature increase of approximately 0.05 to 0.08°C yr-1 has been reported (Park et al., 2015a; Han and Lee, 2020; Li et al., 2022). The study area showed a higher warming rate than the YS, particularly in the inner area, which increased by approximately twice as much. This suggests that as coastal regions become shallower, the warming trend amplifies (DeCarlo et al., 2017; Li et al., 2022). Furthermore, a decline in the average DO trend was observed in the study area with increasing SST. These results can be attributed to reduced oxygen solubility in brackish water and seawater owing to warmer water temperatures during the same period (Choi et al., 2013).
Warming trend is a major factor in increasing precipitation, which can influence salinity changes by enhancing river discharge through floods during the summer season (Wang et al., 2017; Park and Sin, 2022). Therefore, we compared rainfall data obtained from the KMA for the nearest location (Gunsan) to the study area with discharge data calculated according to Cho et al. (2020). Precipitation peaked in 2018 and 2020, and the river discharge outflow from the inner (brackish water) to the outer (seawater) areas was also significantly enhanced (Figure 9). In addition, the SSS had the lowest values in 2018 and 2020. Conversely, Figure 4B shows high salinity in both areas in 2017, coinciding with lower rainfall than other periods (Figure 9). Hence, increasing precipitation enhances river discharge, resulting in lower salinity (Silva et al., 2008). Ultimately, these conclusions suggest that the SST changes in the study area may be influenced not only by artificial disturbances from coastal development but also by global warming effects.
Figure 9 Time series of precipitation (A) and discharge (B) from 2013 to 2022. The orange shade represents the period during which the sluice gates were scheduled to open and close only once per day (from September 2014 to November 2020).
Third, land-based activities near the coast can cause nutrient imbalances such as P-limited conditions. In fact, the increase in Chl-a is related to the uptake of nutrients such as DIP and DIN. Thus, DIP and DIN decrease with uptake, and Chl-a increases, resulting in a negative relationship (Morais et al., 2009; Zhou et al., 2017). The estuary receives high nutrient concentrations, particularly nitrate, from fertilizers in the surrounding agricultural land. However, phosphorus is supplied in lower amounts than nitrogen, as it is preferentially removed through wastewater treatment systems on land (Flynn, 2002). Differences in nutrient levels were also observed in the study area (Figures 3 and 4; Tables 2 and 3), with the inside of the seawall (brackish water) affected by freshwater, showing higher nitrogen levels and slightly lower phosphorus levels (Section 3). Therefore, owing to the excessive supply of nutrients (especially nitrate) from surrounding agricultural areas, the DIN : DIP ratios (Redfield, 1934) on the inside and outside were 50:1 and 17:1, respectively. This suggests that the inside is in an extreme P-limited condition compared with the outside (Ptacnik et al., 2010; Sin et al., 2013; Jeong and Yang, 2015). Consequently, based on the characteristics of the surrounding land, the study area had spatially varying nutrient availability for phytoplankton uptake, with phosphate having a more significant impact on phytoplankton growth than nitrate (as described in Sections 3.1, and 3.2).
Differences in the temporal variations of potentially limiting nutrient levels were clearly observed between 2017 and 2019 (Figures 4D, E). In 2017, the DIN levels were lower, whereas the DIP concentrations remained similar to those in other years in both the inner and outer areas (Figures 4D, E). Similar to DIN, Chl-a exhibited lower values (Figure 4F). In 2019, the DIN levels were the lowest; DIP concentrations were low in the inner part and moderate in the outer part (Figures 4D, E). Chl-a was highest in the seawall (Figure 4F). The findings suggest that phytoplankton in the P-limited environment (inside) rapidly proliferated and showed a phytoplankton bloom in 2019, after sufficient uptake of DIP compared to 2017. Furthermore, in 2020 and 2021, DIN levels showed variability, whereas DIP remained consistently low. During these years, Chl-a levels were high. These findings suggest that phytoplankton blooms in the study area are affected by the sensitivity of phosphate to nitrate (Yang et al., 2008; Choi et al., 2013).
Nitrogen and phosphorus influence phytoplankton community composition (Bi et al., 2021). Phytoplankton growth can be limited in P-limited environments, potentially resulting in decreased primary production and subsequent negative feedback at higher trophic levels (Bianchi, 2007; Kang et al., 2017; Acevedo-Trejos et al., 2018). Diatoms generally grow well when the N:P ratio is low; in other words, their growth increases when phosphate levels are high (Morais et al., 2009; Zhou et al., 2017; Bi et al., 2021). In contrast, dinoflagellates grow well at high N:P ratios (Zhou et al., 2017; Zhang et al., 2022). In Section 3.4, we found that the outside of the Saemangeum seawall, such as seawater, has a high diatom abundance, and the inside (brackish water), which is presumed to be in P-limited conditions, has a greater abundance of dinoflagellates. Particularly, Skeletonema costatum (S. costatum) and Prorocentrum minimum (P. minimum) were the most frequently found species in the Baci and ino communities in the study area, respectively (Choi et al., 2013; Park et al., 2023). Especially, P.minimum is known as a species that causes red tides (Kim and Jeong, 2004; Jeong et al., 2013), and both species, including S.costatum as diatom and P.minimum as dinoflagellate, have been consistently observed since 2006 (Choi et al., 2013; Park et al., 2023). After 2020, when mixing increased to twice daily, environmental changes in salinity conditions increased, and nutrient limitation decreased. Simultaneously, the dominant phytoplankton community shifts from dinoflagellates to diatoms. Consequently, the transition from dinoflagellates to diatoms as the dominant species in the study area may indicate a potential change to a more efficient coastal ecosystem food web (Lin et al., 2005; Bi et al., 2021).
We identified the dominant environmental conditions for diatoms and dinoflagellates using the DT model, which formed the basis for the RF model (Figure 10). Therefore, we used a Gini coefficient of 0 for the nodes comprising the decision tree as the selection criterion for environmental conditions. As the Gini coefficient approaches 0, the nodes are considered to be fully purified and free of impurities (Tangirala, 2020). Additionally, red tide level is determined by the dinoflagellates abundance of more than 2000 cells mL-1 according to empirical trial (Tango et al., 2005; Jeong et al., 2013). The first result from the DT model, diatom can be present when the salinity exceeds 30.30 psu (like ocean environment), and the DIP concentration was below 13.51 mg m-3, while the temperature was above approximately 17.25°C (Figure 10A). These conditions indicate that diatom characteristics, with a higher contribution from S. S.costatum, prevail in environments with higher salinity than do dinoflagellates (Tian et al., 2002). Notably, DIP is a characteristic of diatoms thriving in phosphate-rich environments, suggesting that lower DIP levels in estuaries paradoxically indicate a result of enhanced uptake. On the other hand, the second result from the DT model is that red tide can occur when the salinity is under 27.83 psu (like estuarine environment), and the temperature range is approximately 7.65 to 27.30°C, along with DO levels lower than 102.44 mg m-3, as shown in Figure 10B. These conditions, such as SSS and SST, revealed the P.minimum proliferation states reported by Tango et al. (2005) and included typical dinoflagellate bloom conditions, as described by Xiao et al. (2018). Significantly, the reason why the possibility of red tide occurrence increases as low oxygen levels means that oxygen consumption increases as respiration due to an increase in phytoplankton biomass, resulting in oxygen depletion.
Figure 10 Flowchart of classification for the phytoplankton community, including Bacillariophyceae (A) and red tide (dinophyceae) (B). The blue and red box show differences in salinity conditions for diatom and red tide, respectively.
Mainly, the most influential factor in distinguishing between diatoms and dinoflagellates is SSS, which is supported by the research findings in changing the foundation of the coastal ecosystem according to changes in salinity. Comprehensively, diatoms are known to mainly live in stable environments with limited environmental changes compared to dinoflagellates and are known to respond sensitively to changes in salinity and nutrients, as well as temperature (Zhou et al., 2017). Whereas dinoflagellates are known to adapt better to extreme environments than diatoms and can survive well even in rapid environmental changes such as salinity and temperature. In addition, unlike diatoms, dinoflagellates grow well the high DIN and low DIP condition (Zhou et al., 2017), so it is inferred that dinoflagellates showed a high abundance even in the inside of seawall, which is classified as a relatively P-limited environment. Therefore, through the results of the DT model, it was possible to derive environmental characteristics in which diatoms and dinoflagellates can thrive. In addition, the above results revealed objective environmental data that the phytoplankton community shifts from dinoflagellates to diatoms in response to anthropogenic disturbances, especially changes in salinity and nutrient levels. This phytoplankton succession (from dinoflagellates to diatoms) signifies an improvement in the efficiency of coastal ecosystems (Lin et al., 2005; Bi et al., 2021).
In this study, we conducted research using a regression learner provided by MATLAB R2020a was used to select the optimal model. Four representative models commonly used to estimate Chl-a were tested: a linear regression model (Baek et al., 2019), exponential Gaussian process regression (EGPR) (Pasolli et al., 2010), and RF. The linear regression model’s performance (only test set) was R2 = 0.35 and RMSEChl-a = 5.68 mg m-3, and the SVM model showed an R2 = 0.50 and RMSEChl-a = 4.52 mg m-3. Both models displayed high error values when Chl-a was below 1 mg m-3 or above 30 mg m-3. The EGPR model’s performance showed an R2 = 0.66 and RMSEChl-a = 3.79 mg m-3, and RF had an R2 = 0.64 and RMSEChl-a = 3.22 mg m-3. All models displayed a bias towards overestimating the values when Chl-a was less than 10 mg m-3, with the linear model showing the highest bias, followed by SVM, EGPR, and RF. The training time (s) was the fastest for the simple linear regression model, taking less than 3 s; SVM and RF took less than 5 s; and EGPR took over 20 s. Overall, ML techniques were shown to be more suitable for predicting Chl-a, as they reflected the nonlinear relationships between factors and represented higher accuracy and lower error compared to linear statistical models. EGPR and RF exhibited the highest accuracy, with RF displaying the lowest errors and biases. These results support the conclusion that the pilot study achieved the best performance.
Despite these efforts, constraints, and limitations remain in the precise estimation of Chl-a concentration. Light is essential for phytoplankton growth and primary productivity, as are the upper ocean’s temperature, salinity, and nutrient conditions (Domingues et al., 2005; Yuan et al., 2022). Light controls phytoplankton growth, typically due to high-turbidity water or strengthened stratification levels during the winter (Lu and Gan, 2015). Domingues et al. (2007) suggested the possibility of light limitation in coastal areas with transparency of approximately 1 m (Sin et al., 2013). After analyzing the irregularly acquired Secchi depth, the transparency levels were observed to be within 0.3 to 4 m for the inner region and 1 to 7 m for the outer region, with shallower values during the summer season. It was found that the average depth of the inner area was 1.30 m, and the outer area was 2.39 m, both exceeding 1 m. Furthermore, the Chl-a values in the study area during the summer season, when the transparency is expected to be the lowest, were also very high. Physical environmental factors, such as SST and SSS, also showed similar values from the surface to the bottom layer. Therefore, the study area is estimated to have a low probability of light limitation. Nevertheless, the possibility of light limitations still exists because of the absence of continuous PAR data. Hence, the possibility of light limitations in the inner and outer regions of the seawall cannot be completely ruled out. In addition, we verified the temperature and salinity to consider the stratification that may occur owing to low salinity in freshwater. Physical environmental factors exhibited similar values from the surface to the bottom layers (not shown). Thus, it is assumed that the study area is a water mass in which the surface-to-bottom layers are very well mixed; thus, the impact on buoyancy and stratification is expected to be low. Nevertheless, the possibility of stratification caused by the influence of buoyant plumes, which may occur because of heavy rainfall in summer (Figures 2 and 9), cannot be neglected (Guerreiro et al., 2013).
In this study, we investigated the impact of artificial disturbances on the coastal ecosystem response during the Saemangeum seawall construction. The key findings are as follows. (i) We conducted a spatiotemporal analysis using long-term (1999-2022) environmental data (SST, SSS, DO, DIN, DIP, and Chl-a). This analysis emphasized the significance of salinity variations by observing changes based on spatial distribution. Furthermore, following the seawall construction, restricted freshwater and seawater circulation once daily led to substantial alterations, especially in salinity and nutrient levels; (ii) thus, We employed the RF model to predict the quantitative impact of the five factors influenced by the coastal ecosystem. The estimated Chl-a showed high accuracy (R2 = 0.54) and low difference (RMSEChl-a = 3.79 mg m-3) compared to the observed Chl-a, within the Chl-a ranges, which including inside and outside of Chl-a concentration, of about 0.0 to 45 mg m-3. Through the VI and SHAP approaches to assess the contributions of each factor, it was revealed that SSS was the most influential controller (42.91%) of the coastal ecosystem changes in the study area. The next most important contributor was the SST, followed by the DIP. This order supports that the RF model considers the physicochemical relationships between phytoplankton and environmental factors. (iii) The results of a sensitivity test showed that Chl-a levels increased approximately 1.79 times when the salinity was 5 psu lower (from 7 to 27 psu) than the normal salinity range (from 12 to 32 psu) in outside and inside the seawall. Conversely, when salinity (from 17 to 37 psu) reduced the Chl-a levels by half in the same areas. Moreover, multivariate analysis identified the optimal environmental conditions for abundant Chl-a when salinity levels were below 30 psu, regardless of variations in other environmental factors. These findings emphasize the dominant role of salinity in coastal ecosystems. Furthermore, this study revealed shifts in phytoplankton species in response to salinity changes resulting from artificial disturbances through long-term ecological analysis. In conclusion, the results of this study not only enhance our understanding of the coastal ecosystem’s response to physical environmental changes resulting from anthropogenic disturbances but also contribute to the comprehension of ecological changes, including red tides.
The raw data supporting the conclusions of this article will be made available by the authors, without undue reservation.
J-YB: Conceptualization, Data curation, Formal Analysis, Investigation, Methodology, Software, Validation, Visualization, Writing – original draft, Writing – review & editing. CG: Writing – review & editing, Formal Analysis. JK: Formal Analysis, Resources, Writing – review & editing. JN: Funding acquisition, Writing – review & editing. Y-HJ: Conceptualization, Funding acquisition, Project administration, Supervision, Writing – review & editing.
The author(s) declare financial support was received for the research, authorship, and/or publication of this article. This research was supported by Korea Institute of Marine Science & Technology Promotion (KIMST) funded by the Ministry of Oceans and Fisheries, Korea (20140257). Also, This paper was supported by KIMST funded by the Ministry of Oceans and Fisheries, Korea (20180456). In addition, this work was supported by 2022 BK21 FOUR Program of Pusan National University (PNU-Global Fellowship program). Furthermore, CG currently benefits from a research grant funded by FCT (contract CEECIND/00752/ 2018/CP1534/CT0011) linked to project CHASE (/www.chase-dust.com).
The authors declare that the research was conducted in the absence of any commercial or financial relationships that could be construed as a potential conflict of interest.
All claims expressed in this article are solely those of the authors and do not necessarily represent those of their affiliated organizations, or those of the publisher, the editors and the reviewers. Any product that may be evaluated in this article, or claim that may be made by its manufacturer, is not guaranteed or endorsed by the publisher.
Acevedo-Trejos E., Marañón E., Merico A. (2018). Phytoplankton size diversity and ecosystem function relationships across oceanic regions. Proc. R. Soc. B: Biol. Sci. 285 (1879), 20180621. doi: 10.1098/rspb.2018.0621
Armstrong F. A. J., Stearns C. R., Strickland J. D. H. (1967). The measurement of upwelling and subsequent biological process by means of the Technicon Autoanalyzer® and associated equipment. Deep Sea Res. Oceanographic Abstracts 14 (3), 381–389. doi: 10.1016/0011-7471(67)90082-4
Bae S., Seo D. (2018). Analysis and modeling of algal blooms in the Nakdong River, Korea. Ecol. Model. 372, 53–63. doi: 10.1016/j.ecolmodel.2018.01.019
Baek J. Y., Jo Y. H., Kim W., Lee J. S., Jung D., Kim D. W., et al. (2019). A new algorithm to estimate chlorophyll-a concentrations in turbid yellow sea water using a multispectral sensor in a low-altitude remote sensing system. Remote Sens. 11 (19), 2257. doi: 10.3390/rs11192257
Baek J. Y., Park J., Kim D. W., Lee J. S., Lee J. Y., Lee S. J., et al. (2022). Role of aerosols in spring blooms in the central yellow sea during the COVID-19 lockdown by China. Front. Mar. Sci. 9, 911819. doi: 10.3389/fmars.2022.911819
Bi R., Cao Z., Ismar-Rebitz S. M., Sommer U., Zhang H., Ding Y., et al. (2021). Responses of marine Diatom-Dinoflagellate competition to multiple environmental drivers: Abundance, elemental, and biochemical aspects. Front. Microbiol. 12, 731786. doi: 10.3389/fmicb.2021.731786
Bianchi T. S. (2007). Biogeochemistry of estuaries (United Kingdom: Oxford University Press on Demand).
Borja Á., Dauer D. M., Elliott M., Simenstad C. A. (2010). Medium-and long-term recovery of estuarine and coastal ecosystems: patterns, rates and restoration effectiveness. Estuaries Coasts 33, 1249–1260. doi: 10.1007/s12237-010-9347-5
Boyer J. N., Kelble C. R., Ortner P. B., Rudnick D. T. (2009). Phytoplankton bloom status: Chlorophyll a biomass as an indicator of water quality condition in the southern estuaries of Florida, USA. Ecol. Indic. 9 (6), S56–S67. doi: 10.1016/j.ecolind.2008.11.013
Cho C. W., Song Y. S., Bang K. Y. (2020). A study on the influence of the Saemangeum sluice-gates effluent discharge using the particle tracking model (Korean). J. Korean Soc. Coast. Ocean Engineers 32 (4), 211–222. doi: 10.9765/KSCOE.2020.32.4.211
Choi J. H., Oh C. S., Cho Y. K., Ahn C. H. (2013). Consideration on the Operation of water level management and Environmental Change Associated with Inner Dike Constructions in Saemangeum Reservoir (Korean). J. Korean Soc. Mar. Environ. Energy 16 (4), 290–298. doi: 10.7846/JKOSMEE.2013.16.4.290
Cloern J. E., Foster S. Q., Kleckner A. E. (2014). Phytoplankton primary production in the world's estuarine-coastal ecosystems. Biogeosciences 11 (9), 2477–2501. doi: 10.5194/bg-11-2477-2014
Cutler D. R., Edwards T. C. Jr., Beard K. H., Cutler A., Hess K. T., Gibson J., et al. (2007). Random forests for classification in ecology. Ecology 88 (11), 2783–2792. doi: 10.1890/07-0539.1
DeCarlo T. M., Cohen A. L., Wong G. T., Davis K. A., Lohmann P., Soong K. (2017). Mass coral mortality under local amplification of 2 C ocean warming. Sci. Rep. 7 (1), 44586. doi: 10.1038/srep44586
Domingues R. B., Barbosa A., Galvao H. (2005). Nutrients, light and phytoplankton succession in a temperate estuary (the Guadiana, south-western Iberia). Estuarine Coast. Shelf Sci. 64 (2-3), 249–260. doi: 10.1016/j.ecss.2005.02.017
Domingues R. B., Barbosa A. B., Sommer U., Galvão H. M. (2012). Phytoplankton composition, growth and production in the Guadiana estuary (SW Iberia): Unraveling changes induced after dam construction. Sci. Total Environ. 416, 300–313. doi: 10.1016/j.scitotenv.2011.11.043
Domingues R. B., Sobrino C., Galvão H. (2007). Impact of reservoir filling on phytoplankton succession and cyanobacteria blooms in a temperate estuary. Estuarine Coast. Shelf Sci. 74 (1-2), 31–43. doi: 10.1016/j.ecss.2007.03.021
Elliott M., Quintino V. (2007). The estuarine quality paradox, environmental homeostasis and the difficulty of detecting anthropogenic stress in naturally stressed areas. Mar. pollut. Bull. 54 (6), 640–645. doi: 10.1016/j.marpolbul.2007.02.003
Flynn K. J. (2002). How critical is the critical N: P ratio? J. Phycology 38 (5), 961–970. doi: 10.1046/j.1529-8817.2002.t01-1-01235.x
Frederiksen M., Edwards M., Richardson A. J., Halliday N. C., Wanless S. (2006). From plankton to top predators: bottom-up control of a marine food web across four trophic levels. J. Anim. Ecol. 75 (6), 1259–1268. doi: 10.1111/j.1365-2656.2006.01148.x
Friedman J. H. (2001). Greedy function approximation: a gradient boosting machine. Ann. Stat. 1189–1232. doi: 10.1214/aos/1013203451
Guerreiro C., Oliveira A., De Stigter H., Cachão M., Sá C., Borges C., et al. (2013). Late winter coccolithophore bloom off central Portugal in response to river discharge and upwelling. Continental Shelf Res. 59, 65–83. doi: 10.1016/j.csr.2013.04.016
Han I. S., Lee J. S. (2020). Change the annual amplitude of sea surface temperature due to climate change in a recent decade around the Korean Peninsula. J. Korean Soc Mar. Environ. Saf. 26, 233–241. doi: 10.7837/kosomes.2020.26.3.233
Jang E., Kim Y. J., Im J., Park Y. G., Sung T. (2022). Global sea surface salinity via the synergistic use of SMAP satellite and HYCOM data based on machine learning. Remote Sens. Environ. 273, 112980. doi: 10.1016/j.rse.2022.112980
Jeong H. J., Du Yoo Y., Lee K. H., Kim T. H., Seong K. A., Kang N. S., et al. (2013). Red tides in Masan Bay, Korea in 2004–2005: I. Daily variations in the abundance of red-tide organisms and environmental factors. Harmful Algae 30, S75–S88. doi: 10.1016/j.hal.2013.10.008
Jeong Y. H., Kwak D. H. (2021). Factors affecting behavior and distribution of dissolved organic matter in an artificial coastal reservoir. Regional Stud. Mar. Sci. 44, 101786. doi: 10.1016/j.rsma.2021.101786
Jeong Y. H., Yang J. S. (2015). The long-term variations of water qualities in the Saemangeum salt-water lake after the sea-dike construction (Korean). J. Korean Soc. Mar. Environ. Energy 18 (2), 51–63. doi: 10.7846/JKOSMEE.2015.18.2.51
Jiao N., Zhang Y., Zeng Y., Gardner W. D., Mishonov A. V., Richardson M. J., et al. (2007). Ecological anomalies in the East China Sea: impacts of the three gorges dam? Water Res. 41 (6), 1287–1293. doi: 10.1016/j.watres.2006.11.053
Jin J., Liu S. M., Ren J. L., Liu C. G., Zhang J., Zhang G. L. (2013). Nutrient dynamics and coupling with phytoplankton species composition during the spring blooms in the Yellow Sea. Deep Sea Res. Part II: Topical Stud. Oceanography 97, 16–32. doi: 10.1016/j.dsr2.2013.05.002
Kang J. J., Joo H., Lee J. H., Lee J. H., Lee H. W., Lee D., et al. (2017). Comparison of biochemical compositions of phytoplankton during spring and fall seasons in the northern East/Japan Sea. Deep Sea Res. Part II: Topical Stud. Oceanography 143, 73–81. doi: 10.1016/j.dsr2.2017.06.006
Kim H. G., Hong S., Jeong K. S., Kim D. K., Joo G. J. (2019). Determination of sensitive variables regardless of hydrological alteration in artificial neural network model of chlorophyll a: case study of Nakdong River. Ecol. Model. 398, 67–76. doi: 10.1016/j.ecolmodel.2019.02.003
Kim J. S., Jeong H. J. (2004). Feeding by the heterotrophic dinoflagellates Gyrodinium dominans and G. spirale on the red-tide dinoflagellate Prorocentrum minimum. Mar. Ecol. Prog. Ser. 280, 85–94. doi: 10.3354/meps280085
Kim T. I., Lee H. R., Chang K. I. (2006). Seasonal variation of density stratification in the Saemangeum waters, Korea (Korean). Ocean Polar Res. 28 (3), 339–352. doi: 10.4217/OPR.2006.28.3.339
Kim T. W., Lee K., Lee C. K., Jeong H. D., Suh Y. S., Lim W. A., et al. (2013). Interannual nutrient dynamics in Korean coastal waters. Harmful Algae 30, S15–S27. doi: 10.1016/j.hal.2013.10.003
Kim J. H., Park S. H., Baek S. H., Jang M. H., Yoon J. D. (2020). Changes in fish assemblages after dike construction in the Saemangeum area. Ocean Sci. J. 55, 129–142. doi: 10.1007/s12601-020-0008-8
Lee C. R., Kang H. K., Noh J. H. (2009). Temporal and spatial variation of zooplankton community structure post construction of Saemangeum dyke (Korean). Ocean Polar Res. 31 (4), 327–338. doi: 10.4217/OPR.2009.31.4.327
Lee H. J., Ryu S. O. (2008). Changes in topography and surface sediments by the Saemangeum dyke in an estuarine complex, west coast of Korea. Continental Shelf Res. 28 (9), 1177–1189. doi: 10.1016/j.csr.2008.03.008
Li M. F., Tang X. P., Wu W., Liu H. B. (2013). General models for estimating daily global solar radiation for different solar radiation zones in mainland China. Energy conversion Manage. 70, 139–148. doi: 10.1016/j.enconman.2013.03.004
Li C. L., Yang D. Z., Zhai W. D. (2022). Effects of warming, eutrophication and climate variability on acidification of the seasonally stratified North Yellow Sea over the past 40 years. Sci. Total Environ. 815, 152935. doi: 10.1016/j.scitotenv.2022.152935
Liao Z., Zang N., Wang X., Li C., Liu Q. (2021). Machine learning-based prediction of chlorophyll-a variations in receiving reservoir of world's largest water transfer project—a case study in the miyun reservoir, North China. Water 13 (17), 2406. doi: 10.3390/w13172406
Lie H. J., Cho C. H., Lee S., Kim E. S., Koo B. J., Noh J. H. (2008). Changes in marine environment by a large coastal development of the Saemangeum reclamation project in Korea. Ocean Polar Res. 30 (4), 475–484. doi: 10.4217/OPR.2008.30.4.475
Lin C. L., Ning X. R., Su J. L., Lin Y., Xu B. (2005). Environmental changes and the responses of the ecosystems of the Yellow Sea during 1976–2000. J. Mar. Syst. 55 (3-4), 223–234. doi: 10.1016/j.jmarsys.2004.08.001
Lu Z., Gan J. (2015). Controls of seasonal variability of phytoplankton blooms in the Pearl River Estuary. Deep Sea Res. Part II: Topical Stud. Oceanography 117, 86–96. doi: 10.1016/j.dsr2.2013.12.011
Lundberg S. M., Lee S. I. (2017). “A unified approach to interpreting model predictions,” Proceedings of the Advances in Neural Information Processing Systems 30, 4765–4774.
Mangalathu S., Hwang S. H., Jeon J. S. (2020). Failure mode and effects analysis of RC members based on machine-learning-based SHapley Additive exPlanations (SHAP) approach. Eng. Structures 219, 110927. doi: 10.1016/j.engstruct.2020.110927
McLusky D. S., Elliott M. (2004). The estuarine ecosystem: ecology, threats and management (United Kingdom: OUP Oxford).
Moradi M., Moradi N. (2020). Correlation between concentrations of chlorophyll-a and satellite derived climatic factors in the Persian Gulf. Mar. pollut. Bull. 161, 111728. doi: 10.1016/j.marpolbul.2020.111728
Morais P., Chícharo M. A., Chícharo L. (2009). Changes in a temperate estuary during the filling of the biggest European dam. Sci. total Environ. 407 (7), 2245–2259. doi: 10.1016/j.scitotenv.2008.11.037
Oda Y., Nakano S., Suh J. M., Oh H. J., Jin M. Y., Kim Y. J., et al. (2018). Spatiotemporal variability in a copepod community associated with fluctuations in salinity and trophic state in an artificial brackish reservoir at Saemangeum, South Korea. PloS One 13 (12), e0209403. doi: 10.1371/journal.pone.0209403
Oh C. S., Choi J. H. (2015). Consideration on changes of density stratification in Saemangeum Reservoir (Korean). J. Korean Soc. Mar. Environ. Energy 18 (2), 81–93. doi: 10.7846/JKOSMEE.2015.18.2.81
Paerl H. W., Hall N. S., Peierls B. L., Rossignol K. L. (2014). Evolving paradigms and challenges in estuarine and coastal eutrophication dynamics in a culturally and climatically stressed world. Estuaries coasts 37 (2), 243–258. doi: 10.1007/s12237-014-9773-x
Park Y., Cho K. H., Park J., Cha S. M., Kim J. H. (2015b). Development of early-warning protocol for predicting chlorophyll-a concentration using machine learning models in freshwater and estuarine reservoirs, Korea. Sci. Total Environ. 502, 31–41. doi: 10.1016/j.scitotenv.2014.09.005
Park J., Kim H. C., Bae D., Jo Y. H. (2020). Data reconstruction for remotely sensed chlorophyll-a concentration in the ross sea using ensemble-based machine learning. Remote Sens. 12 (11), 1898. doi: 10.3390/rs12111898
Park S. H., Kim J. G., Myung G. O., Kwon M. S. (2023). Relationship between phytoplankton distribution and salinity in the giant artificial brackish Lake Saemangeum. Ecohydrology Hydrobiology 23 (2), 251–260. doi: 10.1016/j.ecohyd.2023.01.003
Park K. A., Lee E. Y., Chang E., Hong S. (2015a). Spatial and temporal variability of sea surface temperature and warming trends in the Yellow Sea. J. Mar. Syst. 143, 24–38. doi: 10.1016/j.jmarsys.2014.10.013
Park J., Lee W. H., Kim K. T., Park C. Y., Lee S., Heo T. Y. (2022). Interpretation of ensemble learning to predict water quality using explainable artificial intelligence. Sci. Total Environ. 832, 155070. doi: 10.1016/j.scitotenv.2022.155070
Park S., Sin Y. (2022). Characteristics of water quality and chlorophyll-a in the seawater zone of the yeongsan river estuary: long-term, (2009-2018) data analysis (Korean). Ocean Polar Res. 44 (1), 13–27. doi: 10.4217/OPR.2022001
Pasolli L., Melgani F., Blanzieri E. (2010). Gaussian process regression for estimating chlorophyll concentration in subsurface waters from remote sensing data. IEEE Geosci. Remote Sens. Lett. 7 (3), 464–468. doi: 10.1109/LGRS.2009.2039191
Ptacnik R., Andersen T., Tamminen T. (2010). Performance of the Redfield ratio and a family of nutrient limitation indicators as thresholds for phytoplankton N vs. P limitation. Ecosyst. 13, 1201–1214. doi: 10.1007/s10021-010-9380-z
Redfield A. C. (1934). On the proportions of organic derivatives in sea water and their relation to the composition of plankton (Vol. 1) (Liverpool: university press of liverpool).
Rodríguez-Pérez R., Bajorath J. (2020). Interpretation of machine learning models using shapley values: application to compound potency and multi-target activity predictions. J. computer-aided Mol. design 34, 1013–1026. doi: 10.1007/s10822-020-00314-0
Ryther J. H. (1969). Photosynthesis and Fish Production in the Sea: The production of organic matter and its conversion to higher forms of life vary throughout the world ocean. Science 166 (3901), 72–76. doi: 10.1126/science.166.3901.72
Silva A., Palma S., Moita M. T. (2008). Coccolithophores in the upwelling waters of Portugal: Four years of weekly distribution in Lisbon bay. Continental Shelf Res. 28 (18), 2601–2613. doi: 10.1016/j.csr.2008.07.009
Sin Y., Hyun B., Jeong B., Soh H. Y. (2013). Impacts of eutrophic freshwater inputs on water quality and phytoplankton size structure in a temperate estuary altered by a sea dike. Mar. Environ. Res. 85, 54–63. doi: 10.1016/j.marenvres.2013.01.001
Smith M., Alvarez F. (2021). Identifying mortality factors from Machine Learning using Shapley values–a case of COVID19. Expert Syst. Appl. 176, 114832. doi: 10.1016/j.eswa.2021.114832
Tangirala S. (2020). Evaluating the impact of GINI index and information gain on classification using decision tree classifier algorithm. Int. J. Advanced Comput. Sci. Appl. 11 (2), 612–619. doi: 10.14569/IJACSA.2020.0110277
Tango P. J., Magnien R., Butler W., Luckett C., Luckenbach M., Lacouture R., et al. (2005). Impacts and potential effects due to Prorocentrum minimum blooms in Chesapeake Bay. Harmful Algae 4 (3), 525–531. doi: 10.1016/j.hal.2004.08.014
Telesh I. V. (2004). Plankton of the Baltic estuarine ecosystems with emphasis on Neva Estuary: a review of present knowledge and research perspectives. Mar. pollut. Bull. 49 (3), 206–219. doi: 10.1016/j.marpolbul.2004.02.009
Tian Y., Mingjiang Z., Peiyuan Q. (2002). Combined effects of temperature, irradiance and salinity on growth of diatom Skeletonema costatum. Chin. J. Oceanology Limnology 20 (3), 237–243. doi: 10.1007/BF02848852
Wang H., Shao Z., Gao T., Zou T., Liu J., Yuan H. (2017). Extreme precipitation event over the Yellow Sea western coast: Is there a trend? Quaternary Int. 441, 1–17. doi: 10.1016/j.quaint.2016.08.014
Wei Q., Wang B., Zhang X., Ran X., Fu M., Sun X., et al. (2021). Contribution of the offshore detached Changjiang (Yangtze River) Diluted Water to the formation of hypoxia in summer. Sci. Total Environ. 764, 142838. doi: 10.1016/j.scitotenv.2020.142838
Wetz M. S., Hutchinson E. A., Lunetta R. S., Paerl H. W., Christopher Taylor J. (2011). Severe droughts reduce estuarine primary productivity with cascading effects on higher trophic levels. Limnology Oceanography 56 (2), 627–638. doi: 10.4319/lo.2011.56.2.0627
Xiao W., Liu X., Irwin A. J., Laws E. A., Wang L., Chen B., et al. (2018). Warming and eutrophication combine to restructure diatoms and dinoflagellates. Water Res. 128, 206–216. doi: 10.1016/j.watres.2017.10.051
Yang J. S., Jeong Y. H., Ji K. H., Kim H. S., Choi J. H., Kim W. J. (2008). The early-stage changes of water qualities after the Saemangeum sea-dike construction (Korean). J. Korean Soc. Mar. Environ. Energy 11 (4), 199–213.
Yuan X., Wang S., Fan F., Dong Y., Li Y., Lin W., et al. (2022). Spatiotemporal dynamics and anthropologically dominated drivers of chlorophyll-a, TN and TP concentrations in the Pearl River Estuary based on retrieval algorithm and random forest regression. Environ. Res. 215, 114380. doi: 10.1016/j.envres.2022.114380
Zhang X., Fang C., Wang Y., Lou X., Su Y., Huang D. (2022). Review of effects of dam construction on the ecosystems of river estuary and nearby marine areas. Sustainability 14 (10), 5974. doi: 10.3390/su14105974
Keywords: anthropogenic disturbance, chlorophyll-a concentration, random forest, coastal environmental changes, phytoplankton community
Citation: Baek J-Y, Guerreiro CV, Kim J, Nam J and Jo Y-H (2024) Coastal environmental changes after the Saemangeum seawall construction. Front. Mar. Sci. 10:1307218. doi: 10.3389/fmars.2023.1307218
Received: 04 October 2023; Accepted: 12 December 2023;
Published: 04 January 2024.
Edited by:
Zhanfei Liu, The University of Texas at Austin, United StatesReviewed by:
Sun Cuici, Chinese Academy of Sciences (CAS), ChinaCopyright © 2024 Baek, Guerreiro, Kim, Nam and Jo. This is an open-access article distributed under the terms of the Creative Commons Attribution License (CC BY). The use, distribution or reproduction in other forums is permitted, provided the original author(s) and the copyright owner(s) are credited and that the original publication in this journal is cited, in accordance with accepted academic practice. No use, distribution or reproduction is permitted which does not comply with these terms.
*Correspondence: Young-Heon Jo, am95b3VuZ0BwdXNhbi5hYy5rcg==
Disclaimer: All claims expressed in this article are solely those of the authors and do not necessarily represent those of their affiliated organizations, or those of the publisher, the editors and the reviewers. Any product that may be evaluated in this article or claim that may be made by its manufacturer is not guaranteed or endorsed by the publisher.
Research integrity at Frontiers
Learn more about the work of our research integrity team to safeguard the quality of each article we publish.