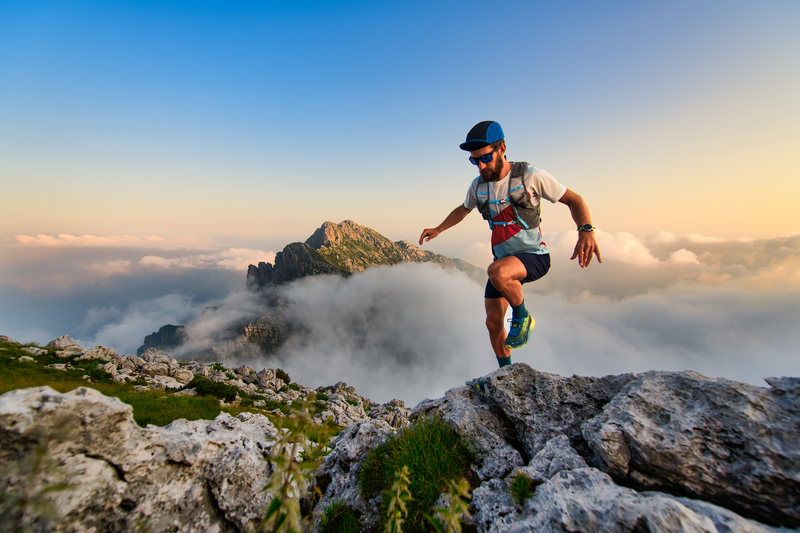
94% of researchers rate our articles as excellent or good
Learn more about the work of our research integrity team to safeguard the quality of each article we publish.
Find out more
ORIGINAL RESEARCH article
Front. Mar. Sci. , 15 January 2024
Sec. Marine Megafauna
Volume 10 - 2023 | https://doi.org/10.3389/fmars.2023.1306294
Rapid changes of marine ecosystems resulting from human activities and climate change, and the subsequent reported rise of infectious diseases in marine mammals, highlight the urgency for timely detection of unusual health events negatively affecting populations. Studies reporting pathological findings in the commonly stranded harbor porpoise (Phocoena phocoena) on North Atlantic coastlines are essential to describe new and emerging causes of mortality. However, such studies often cannot be used as long-term health surveillance tools due to analytical limitations. We tested 31 variables gained from stranding-, necropsy-, dietary- and marine debris data from 405 harbor porpoises using applied supervised and unsupervised machine learning techniques to explore and analyze this large dataset. We classified and cross-correlated the variables and characterized the importance of the different variables for accurately predicting cause-of-death categories, to allow trend assessment for good conservation decision. The variable ‘age class’ seemed most influential in determining cause-of-death categories, and it became apparent that juveniles died more often due to acute causes, including bycatch, grey-seal-predation and other trauma, while adults of infectious diseases. Neonates were found in summer, and mostly without prey in their stomach and more often stranded alive. The variables assigned as part of the external examination of carcasses, such as imprints from nets and lesions induced by predators, as well as nutritional condition were most important for predicting cause-of-death categories, with a model prediction accuracy of 75%. Future porpoise monitoring, and in particular the assessment of temporal trends, should predominantly focus on influential variables as determined in this study. Pathogen- and contaminant assessment data was not available for all cases, but would be an important step to further complete the dataset. This could be vital for drawing population-inferences and thus for long-term harbor porpoise population health monitoring as an early warning tool for population change.
Necropsies are often a key element in wildlife surveillance programs (Ryser-Degiorgis, 2013). Necropsies can be performed for various reasons, including (but not limited to) characterizing normal and abnormal morphologic features, to establish baseline health parameters and to identify cause(s) of morbidity and mortality in individuals, groups or populations (Ryser-Degiorgis, 2013; McAloose et al., 2018). In terrestrial wildlife research, the goals of health surveillance programs are usually disease-driven: the recognition of exotic or emerging disease and its potential for transmission to domestic animals or humans (Artois et al., 2009; Ryser-Degiorgis, 2013). Many marine mammal monitoring programs, however, originated with a primary conservation and management focus and the main aim of determining the rate and extent of human-related causes of morbidity and mortality (e.g., ASCOBANS, 1992). Ideally, studying cetacean pathology — the study of diseases, their cause or etiology, pathogenesis and the structural and functional changes they give rise to (McAloose et al., 2018) — should be extended with ecological and other biological disciplines and quantitative analysis, to go beyond the emphasis on specific individuals and to be able to make appropriate population inferences.
Studies reporting pathological findings in commonly stranded small cetaceans in the North-East Atlantic and adjacent waters often focus on descriptions of the most frequently diagnosed lesions and causes of death (Kirkwood et al., 1997; Jauniaux et al., 2002; Siebert et al., 2001; Siebert et al., 2006; Arbelo et al., 2013; van Elk et al., 2019; Schick et al., 2020; Siebert et al., 2020; Neimanis et al., 2022). Specifically, bycatch has been a primary research focus (Clausen and Andersen, 1988; Kuiken, 1996; Kirkwood et al., 1997; IJsseldijk et al., 2021), and so have reports on the health effects of anthropogenic noise, chemical pollution and marine debris for a number of marine mammal species (Kuiken et al., 1994; Fernández et al., 2005; Jepson et al., 2005; Unger et al., 2016; Desforges et al., 2018; Sonne et al., 2020; Siebert et al., 2022). These studies are essential to describe typical or atypical causes of mortality and new or emerging infectious and non-infectious diseases, but they often cannot be used as long-term health surveillance tool due to analytical limitations. Analyzing data from post-mortem examinations is challenged by several factors — including large individual variance, varying methods, procedures and specialists involved between cases, decomposition, insufficient numbers of individuals or a lack of long-term studies — that may all hamper comparative and comprehensive (meta-) analyses. There is, however, an urgent need for assessments of patterns in biological, ecological and pathological profiles across individuals, in order to detect changes over space and time for trend analysis and timely detection of unusual health events affecting populations. This is particularly relevant given the rapid changes of marine ecosystems resulting from human influences and climate change, and the subsequent reported rise of contaminant exposure and infectious diseases in marine mammals (Gulland and Hall, 2007; Simmonds and Isaac, 2007; Halpern et al., 2008; Bossart, 2011; Gulland et al., 2022).
In the Netherlands, a long-term cetacean post-mortem surveillance program was initiated in 2008 to sample stranded animals and monitor their causes of mortality and health status. The primary species found stranded in the Netherlands is the harbor porpoise (Phocoena phocoena) (IJsseldijk et al., 2020). Pathological findings related to anthropogenic and natural cause-of-death categories in 612 harbor porpoises that stranded between 2008 and 2019 on Dutch shores were recently published (IJsseldijk et al., 2022). In this follow-up study, we applied supervised and unsupervised machine learning techniques to further explore and assess the post-mortem data and to investigate the usefulness of such methods to aid in surveillance and to further unlock information that is contained in these data. Using classification and correspondence analyses, we assessed the correlation between variables collected in stranding, necropsy, dietary and marine debris investigations and determined their association with, and importance for predicting harbor porpoise cause-of-death categories. We additionally explored what kind of hypotheses can be derived from the results of these analyses, that can provide guidance for future targeted surveillance. With the large number of individual harbor porpoises included in the analyses, robust trends in post-mortem findings in terms of anthropogenic and natural threats can be detected, resilient to small or short-term variations or simply chance, and thus relevant at the population level. This work therefore serves as a detailed example on the potential wider usefulness of collecting long-term and extensive mortality data of a protected species, applicable for wildlife across different environments and taxa.
Post-mortem examinations on stranded harbor porpoises have been conducted at the Faculty of Veterinary Medicine, Utrecht University since 2008, with the necropsies following internationally standardized guidelines (IJsseldijk et al., 2019, adapted from Kuiken and García Hartmann, 1991). The primary aim of the post-mortem examinations was to determine the animals’ most likely causes of death and their health status prior to death. Each case was assigned to one of ten cause-of-death categories reflecting the most likely cause of death or stranding: accidental bycatch in fisheries (referred to as bycatch), acute grey seal (Halichoerus grypus) predation, previously induced but still active bite lesions from a grey seal attack, infectious disease, emaciation, starvation, perinatal death, blunt force and sharp trauma, other and unknown (for more details see: IJsseldijk et al., 2022). Here, the necropsy reports, including photographs, gross- and histopathological descriptions and information on diet and marine debris ingestion, of these 612 cases were reassessed and scored on 31 points (referred to further as variables). Variable descriptions and categories within variables are presented in Table 1. Of the 612 cases, 405 cases had complete records of all variables, which we took to be a requirement for inclusion in this study. The excluded cases missed data on one or more of the variables, mostly due to incompleteness of the carcass or post-mortem artefacts. The dataset is published as Supplementary Table 1.
Table 1 Variables taken from stranding and necropsy reports and diet- and marine debris investigations for analytical purposes.
Data exploration and analyses were performed using R version 4.2.2 (R Core Team, 2017), with packages ggplot2 (Wickham, 2016), grid, gridExtra and RColorBrewer of CRAN for visualization. We used supervised and unsupervised machine learning techniques for data exploration and analyses. Unsupervised learning is the process of grouping unclassified data into clusters using automated methods or algorithms. This means that there is no a priori response variable and no prior labelling or classification of the data, and one seeks to understand the relationships between the measured variables using cluster analysis. With supervised learning there is a response variable; in our study this is the concluding cause-of-death category. Supervised learning models can generalize knowledge from labelled or categorized data (variables) to predict new or unlabeled cases (Berry et al., 2020). Specific packages used for the supervised and unsupervised classification of data are listed in the appropriate subsections below.
Firstly, correlation between all variables was assessed using a correlation matrix with R package corrplot (version 0.84). Cramer’s V correlation coefficient was calculated for every pair of variables, using the vcd and DescTools libraries. Cramer’s V is defined as the square root of a normalized chi-square value. It ranges between 0 and 1 and quantifies strengths of associations between categorical variables. Similar to Pearson’s r, a value close to 0 means no association (Akoglu, 2018). To further interpret Cramer’s V, we follow Akoglu (2018) with values >0.05 indicating a weak association, >0.10 a moderate association, >0.15 a strong association and >0.25 a very strong association. Because we measure association between categorical variables, the direction of associations (positive or negative) is not reflected in Cramer’s V. Certain categories however represent Likert scales (such as parasite loads) or intervals (such as age class or nutritional condition). For those associations with Cramer’s V > 0.5 (n=6), we present stacked bar charts and provide hypotheses that can be derived from these results as a guide for future targeted surveillance.
Cluster analysis attempts to group points — representing individuals, and their multivariate sample in a multidimensional Euclidean space — into disjoint sets (Gower, 1967). Different methods of clustering can be applied, depending on whether the variables are quantitative, qualitative or a combination thereof. Here, a Multiple Correspondence Analysis (MCA) using a Burt table was conducted using the FactoMineR package for analysis and the factoextra package for visualization (Husson et al., 2016; Berry et al., 2020). MCA is an extension of correspondence analysis for summarizing and visualizing a data table consisting of more than two categorical variables. As such, it is considered a generalization of Principal Component Analysis (PCA) with categorical instead of quantitative variables (Abdi and Williams, 2010). MCA shows associations between variable categories and groups of individuals with similar profiles. We focused on the categories and not on individuals in our dataset. We extracted the eigenvalues, reflecting the proportion of variance in the different dimensions (axes), and explored the percentage of inertia explained by the dimensions using scree plots. For interpretation we assessed the (square of the) correlation ratio between each variable and each dimension (eta2), the test values (v.test) to judge association between categories and a factor following a Gaussian distribution, and the R2 correlation ratio of the variables in the different dimensions. Positive test values represent positive associations, negative values represent negative associations. For visualization, we focused on the quality of the representation of variables on the factor map (squared cosine, cos2) and the contribution of variables to the definition of dimensions (contrib). Finally, the coordinates of the individuals (coord) on the principal components of the MCA were used for hierarchical classification. The main output, the dendrogram generated by the hierarchical clustering, shows the hierarchical relationship and distance between clusters (Murtagh and Contreras, 2012).
As a method of supervised learning, we conducted a Random Forest (RF) classification, including the final cause-of-death category, using the R-package randomForest. Individuals with cause-of-death categories ‘other’ and ‘unknown’ were excluded (n=13). The RF algorithm is based on classification (if response is a factor) and regression (if response is continuous) tree analysis, where nodes of a tree are split using the best subset of randomly chosen predictors. This contrasts with standard trees, which split nodes into binary groups by identifying regions with the most homogenous set of responses to predictor variables (Breiman, 2001; Liaw and Wiener, 2002). RFs have two parameters: the number of variables in the random subset at each node and the number of trees in the forest. The technique is robust against overfitting and yields accurate predictions (Breiman, 2001), for a detailed explanation see Liaw and Wiener (2002).
We split our dataset randomly in a training set (bootstrap samples, set at a proportion of 0.7 of the cases) and a test set (the remaining cases). The predictive ability was tested on out-of-bag (oob) observations. Two settings needed to be chosen: the numbers of trees in the forest (Ntrees) and the number of variables randomly drawn at each split (m) (James et al., 2017). Each RF tree can provide an estimate of the importance of the variables for the predictions and of the structure of the data (proximity of data points to one another) based on the oob-data. The variable’s importance was assessed by estimating the mean decrease accuracy, which indicates how much accuracy the model loses by excluding each variable, and by the mean decrease in Gini coefficient, which measures how each variable contributes to the homogeneity of the nodes and leaves in the resulting RF (Liaw and Wiener, 2002). The higher the mean value, the greater the accuracy of the variable to the successful classification of the model.
The variables with the most and the highest Cramer’s V correlations were: ‘age class’, ‘pathology of the respiratory tract’ and ‘multiple organ inflammation’ (Figure 1; Supplementary Table 2). The total percentage of inertia (variance) explained by the first three dimensions of the MCA was 42.5% (Supplementary Figure 1) of the (in total) 56-dimensional space (eigenvalues, percentage of variance and cumulative variance presented in Supplementary Table 3 and the variable output of the MCA is given in Supplementary Tables 4–9). The first two dimensions are used in plotting the MCA-map (Supplementary Figure 2). Results of the MCA corresponds to those of the correlation analysis using Cramer’s V. Variables with the highest contribution to the first dimension of the MCA were ‘age class’ (R2 = 0.63), ‘parasites respiratory tract’ (R2 = 0.59), ‘pathology respiratory tract’ (R2 = 0.51) and ‘parasites middle ear and sinuses’ (R2 = 0.48). Variables with the highest contribution to the second dimension of the MCA were ‘season’ (R2 = 0.43), ‘age class’ (R2 = 0.42) and ‘ingested prey’ (R2 = 0.42) (Supplementary Figure 3). These variables can thus be considered most relevant in terms of explaining variance in the data.
Figure 1 Correlation matrix with Cramer’s V correlation coefficient. Cramer’s V close to 0 means no association, >0.05 indicates a weak association, >0.10 a moderately associated, >0.15 a strong association and >25 a very strong association (Akoglu, 2018).
Assessment of the values within variables gives more insight into patterns contained in the data. The values best represented in the first dimension of the MCA were: the value present in the variable ‘pathology respiratory tract’, adult in the variable ‘age class’, present in the variable ‘multiple organ inflammation’ and present in the variable ‘pathology liver’. This suggests that the data group together for these values within the variables. We can interpret this as an indication that most adult harbor porpoises present moderate to severe pathological changes to the respiratory tract, liver and multiple (other) organs. Values best represented in the second dimension of the MCA were: absent in the variable ‘ingested prey’, summer in the variable ‘season’, neonate in the variable ‘age class’, and finally yes in the variable ‘live stranding’. This suggests that most neonates are found in summer, that they had generally no prey in their stomach and often live stranded (Figure 2; Supplementary Table 9).
Figure 2 Variable values (triangles) and individuals (circles) plotted on the Multiple Correspondence Analysis factor map. Values most influential (cos2 >0.75) are indicated in red and the values are given (see Table 1 for variable and value descriptions). Note that not all values with >0.75 are labelled for readability.
There were several variables that did not correlate to any of the other variables: ‘location’, ‘sex’, ‘mutilation’, ‘previously induced bite lesions’, ‘blunt force trauma’, ‘ingested debris’, ‘edema respiratory tract’, ‘pathology cardiovascular system’, and finally ‘miscellaneous’ (using Cramer’s V, Figure 1; Supplementary Table 2) meaning that these variables are non-influential for clustering of the data.
There were 46 combinations of variables with correlations with Cramer’s V >0.25 (Supplementary Table 2). Those with Cramer’s V >0.5 (n=6) are presented in Table 2 together with examples of hypotheses these associations suggest for future targeted studies.
Table 2 Variables with Cramer’s V >0.5, with plotted barchart as an indicator of the association between two variables, and the hypothesis that could be drawn from that.
Assessment of individuals in relation to their final cause-of-death category highlights which of the variables heavily influence categorization of causes of death. Firstly, the coordinates of the individuals on the principal components of the MCA were used for hierarchical classification. It identified three clusters in the data (Supplementary Figures 4, 5). In cluster 1, there were predominantly animals that had died perinatally or due to starvation (Table 3, blue cluster in Figure 3). This is the smallest cluster of the three. The second cluster contains mostly animals that had died due to acute causes, including bycatch, grey seal predation and other trauma, although animals with non-acute grey seal bite lesions as well as animals dying of emaciation are also represented (Table 3, yellow cluster in Figure 3). The third cluster mainly consists of harbor porpoises that had died of infectious diseases (Table 3, orange cluster in Figure 3). When cross-checking the coordinates of the clusters (Supplementary Figure 5) with the MCA-results by color-marking the points by age class (Figure 3), it is apparent that the first cluster consisted mostly of neonates, the second cluster of juveniles and some adults, and the third cluster mostly of adults. This confirms the strong relation between the variable ‘age class’ and cause-of-death categories.
Table 3 Representation of cause-of-death categories in the three clusters assigned through the multiple correspondence analysis.
Figure 3 Individuals plotted on the Multiple Correspondence Analysis factor map, colored by age class. Age class 1 are the neonates (blue), 2 the juveniles (yellow) and 3 the adults (orange). The ellipse of the different age classes are small and do not overlap, indicating that the three age class values are part of distinct clusters.
The RF model, constructed with 700 trees and m=5 (variables randomly drawn at each split), correctly classified 74.82% of the cause-of-death categories. Different runs with varying m values (range m=3-7) resulted in marginally different classification accuracies (varying from 73.72% at m=3 to 75.91% at m=7). A decrease in Ntrees to 100 (with m=5) also did not change the accuracy of the model much, with still 72.63% of the cause-of-death categories accurately predicted. We therefore conclude that it was possible to accurately predict 75% of cause-of-death categories based on the variables assessed during the necropsies of harbor porpoises.
The variables that most influenced the RF model were: ‘mutilation’, ‘imprints’ and ‘previously induced bite lesions’ followed by ‘multiple organ inflammation’ and ‘age class’. This indicates that the model loses accuracy by excluding each of these variables. Permuting these variables from the RF model affects the model error and we thus conclude that these are influential predictor variables. The opposite was true for the variables ‘ingested debris’, ‘sex’, ‘pathology cardiovascular system’, ‘iron accumulation hepatocytes’, ‘ingested stones’ and ‘multiple organ congestion’ (Figure 4). Permuting these non-influential variables has little to no effect on the model error. Assessment by Gini-coefficient showed overlapping results, with ‘imprints’, ‘previously induced bite lesions’ and ‘mutilation’ deemed most influential in predicting cause-of-death categories, followed by variables ‘nutritional condition’ and ‘location’. The variables ‘iron accumulation hepatocytes’, ‘pathology cardiovascular system’, ‘ingested plants’, ‘ingested debris’ and ‘pathology urogenital system’ were not influential and did thus not directly correlate to one cause-of-death category (Supplementary Figure 6).
Figure 4 The most importance variables in the classification (assessed by the mean decrease in accuracy) in decreasing order. Assessment by mean decrease in Gini is presented in Supplementary Figure 6.
We recently reported pathological findings of harbor porpoises and related these to anthropogenic and natural cause-of-death categories (IJsseldijk et al., 2022). In the present study, these stranding, necropsy, dietary and marine debris data were analyzed in more depth, to determine the classification of, and correlation between, 31 possibly relevant variables and to determine their relative importance for accurately predicting cause-of-death categories. There were three clusters defined through the unsupervised classification methods, mainly related to the variable ‘age class’. Through the supervised classification methods, we defined the variables ‘imprints’, ‘previously induced bite lesions’, ‘mutilation’ and ‘nutritional condition’ as the most important variables for predicting cause-of-death categories, with a model prediction accuracy of 75%. In both supervised and unsupervised classification methods, a range of variables were deemed non-informative for that purpose, either because they did not correlate to other variables or permuting these variables from the predictive model had little to no effect on the model outcomes. These were: ‘ingested plants’, ‘ingested debris’, ‘iron accumulation hepatocytes’, ‘iron accumulation Kupffer cells’, ‘sex’, ‘blunt force trauma’, ‘edema respiratory tract’, ‘pathology cardiovascular system’ and ‘multiple organ congestion’. These variables can therefore be considered uninformative in terms of explaining the variance in the data. We suggest that these reflect variables that either rarely occur or widely occur regardless of other variables.
The most important variable that resulted from the analyses was ‘age class’ and we can therefore conclude that age is highly influential in cause-of-death categorization. This strengthens the suggestion that harbor porpoises in the North Sea face different risks during their lifetime, as briefly discussed in IJsseldijk et al. (2022). The results of the MCA and the subsequent hierarchical clustering identified three distinct clusters, collectively explaining 42.5% of the variance in the data. The first cluster consisted predominantly of neonates. The individuals in this cluster had absence of disease, parasites and solid stomach content. They fell within the cause-of-death category ‘perinatal death’, which logically only includes neonates, and also the category ‘starvation’, which appears a common mortality cause for neonates (Kirkwood et al., 1997; Siebert et al., 2001; IJsseldijk et al., 2022). It is thus not unexpected that the neonates can rather easily be distinguished in the data based on their pathological profiles. The second cluster consisted mainly of juveniles, although some adults were also represented in this cluster. The variables indicative of acute causes of death were predominantly grouped here, revealing similarity in pathological profiles among porpoises dying of the acute cause-of death categories: ‘bycatch’, ‘grey seal predation’ (both acute through mutilations, as those that died with previously induced but active bite lesions) and ‘trauma’ (mostly ship strikes). However, also the category ‘emaciation’ was prominent in this cluster, which cannot be considered an acute cause of death. Poor nutritional condition suggests prolonged debilitation rather than acute processes. However, these porpoises had no signs of significant disease or other abnormalities that could explain their poor nutritional status, resulting in similarity in pathological profiles of these porpoises with those dying from acute and anthropogenic causes. The cause of the emaciation would likely rather be an effect of (changes in) foraging success. Finally, the third cluster consisted of mainly adults. This cluster was dominated by the infectious disease category, although numerous bycaught individuals were also part of cluster 3. This shows similarity in the pathological profiles of bycaught adult porpoises and those dying of infectious causes. We can conclude that this points towards an overall reduced health of adult harbor porpoises inhabiting the southern North Sea or an increase in susceptibility to infectious disease with age.
The potential confounding effect of contaminants on immune function, and subsequently on the risk of infectious disease, could not be included in these analyses. This would be particularly relevant for the adults in our dataset. Exposure to chemicals, mainly persistent organic pollutants (POPs), occurs widely in the marine environment and could result in adverse health effects of marine animals (Jepson et al., 1999; Aguirre and Tabor, 2004; Pierce et al., 2008; Jepson et al., 2016). POPs bioaccumulate and biomagnify in marine food webs, resulting in the highest exposure for long-lived top-predators, such as the harbor porpoise (Sobek et al., 2010; Hallanger et al., 2011; Jepson et al., 2016; Desforges et al., 2018). Chemical contaminant concentrations have been analyzed in a subset of harbor porpoises from this study, with results related to age class and sex, parasite burden and grouped causes-of-death (acute causes versus infectious causes), see van den Heuvel-Greve et al. (2021). It was concluded that adult harbor porpoises, and in particular the males, had the highest contaminant burden, and the probability of a porpoise dying due to infectious disease or debilitation increased with increasing contaminant concentration. As only a subset of animals was chemically screened, the data could not be included here. Improved (retrospective) analysis of contaminant exposure targeting at least all adult animals would allow in depth assessment on the effect of contaminant concentrations on not only (categorized) cause of death, but also more subtle organ changes.
Changes in the respiratory tract were commonly found and seem to correlate to many other variables, including age class (Figure 1). Lesions in the respiratory tract and parasites in the lungs were also deemed relatively important as predictor variable in the RF modeling (Figure 4). Cetaceans are very sensitive to pulmonary disease (St. Leger et al., 2009). This is a direct consequence of their specific anatomy and physiology. Cetaceans lack nasal turbinates which hampers them from filtering particles, making it easier for particles (including pathogens) to enter the respiratory tract. Their trachea is relatively short and wide compared to that of terrestrial animals, and their lungs lack subdivision into lobes. This allows easy deposition of particles deep and extensively into pulmonary tissues and a relatively high risk for diffuse infections (St. Leger et al., 2009; Cozzi et al., 2017). Pulmonary disease, often associated with parasite presence, is one of the most common findings in harbor porpoises (Jepson et al., 2000; Kapetanou et al., 2020; Reckendorf et al., 2021; IJsseldijk et al., 2022). High incidence of lungworms in juvenile and adult porpoises (but not in neonates), may negatively affect health as it causes respiratory distress and instigates secondary bacterial infections (Jepson et al., 2000; Siebert et al., 2001; Jauniaux et al., 2002; Lehnert et al., 2005; Siebert et al., 2020; Reckendorf et al., 2021). Structural microbiological screening was beyond the scope of our study (IJsseldijk et al., 2022, and this study), it is however a recommended follow-up considering the high incidence of respiratory tract changes. Targeted respiratory surveillance could also answer to the hypotheses proposed in Table 2, with recommended follow-up studies into: the exposure-effect relationship between parasites in the respiratory tract and severity of lesions, the underlying causes explaining the increasing risk of respiratory lesions and respiratory parasites with age, the relation between pulmonary parasites and parasites in other organ systems, and, finally, the causes and associations between respiratory infection and infection in other organ systems.
Through the unsupervised classification procedure, we demonstrated the possibility of identifying pathologically relevant variables and associations between variables within this large dataset, in relation to cause-of-death categories. The analyses are based on many individuals and hence allow the detection of robust trends not influenced by minor individual variation or chance effects. If considered complete, results from these types of analyses may therefore inform us about threats relevant to the population. One could consider taking the assessments on variables that were deemed irrelevant in terms of explaining the data out of future post-mortem investigations, when aiming at determining cause-of-death categories. However, and as stated previously, pathogen-screening and contaminant analysis would be an important step to further complete the dataset and vital to be able to draw population-inferences.
Through the supervised classification RF method, we were able to accurately predict cause-of-death categories based on the variables we identified. Logically, predictions are highly influenced by the variables included in the model, as well as their mutual relationship and variance. Our dataset has a combination of common but rather unspecific variables, such as congestion or edema; combined variables, such as multiple organ inflammation; very specific variables that could have been combined, such as ingested non-food items; variables directly informative upon a specific cause of death, such as the presence of imprints from nets inferring to bycatch (Kuiken, 1996; IJsseldijk et al., 2021) or mutilations and bite lesions consistent with grey seal predation (Leopold et al., 2015a; Leopold et al., 2015b; Foster et al., 2019). It is not surprising that specific variables were influential in the predictive modeling. Knowledge and experience of researchers, and involvement of different specialists over the period of a long-term monitoring program, is also influential in assessing organ change and subsequent diagnosing of causes. To reduce subjectivity, working with decision-trees to come to diagnostic conclusions is key. These methods are available to determine death due to grey seal attacks (Leopold et al., 2015b; van Neer et al., 2020) but still needed to accurately recognize lesions associated to anthropogenic threats, including bycatch and ship strike. Composing diagnostic protocols or best practices should, evidently, be accomplished at the relevant regional or international level, and therefore within the frameworks of treaties such as ASCOBANS (1992) and merged in analytical procedures like ours. Finally, peer-review or team-discussion of diagnostics of individual case reports as part of these protocols could reduce observer bias resulting from differences in experience.
Although the pathologically relevant variables were deemed indicative in the subdivision of the clusters, it is clear that they do not address the full range of lesions found in individuals. Especially because cases with rare disorders are difficult to qualify and are not picked-up by the methods presented here (Warns-Petit et al., 2010). This also explains why variables with many zero values, such as ‘ingested plants’, ‘ingested debris’, or ‘miscellaneous’ were deemed irrelevant in order to subdivide or predict cause-of-death categories. In addition, vulnerability of an individual to infections or lesions can vary as an effect of age, season, immunity, severity or undetermined mechanisms. Finally, grouping of cases into values as either presence/absence or on Likert scale can be considered rather crude. It is evident that the methods applied here are not sufficient to study pathogenesis, etiological agents, or cause-effect relationships and should thus be considered complementary and not as an alternative to in-depth case studies or targeted surveillance.
Using multiple correspondence analysis for disease monitoring, or determining the risk factors associated with disease outbreaks and mortality events, is a frequently used approach in terrestrial wildlife health studies (Warns-Petit et al., 2010; Sichewo et al., 2019; Orozco et al., 2020; Sichewo et al., 2020). Defining distinct pathological entities aid in early outbreak detection or determination of the most frequently occurring diseases and their distinctive characteristics (Warns-Petit et al., 2010). This was recently demonstrated when a large-scale mortality event due Erysipelothrix rhusiopathiae affected harbor porpoises in the North Sea (IJsseldijk et al., 2023). The classification of cases based on pathological profiles has additional practical value, because when precise diagnosis is not needed, it allows for a rapid, reliable and rather inexpensive mean of scanning wildlife health data (Warns-Petit et al., 2010). For cetaceans, we demonstrate that it is important to not only assess pathology but to incorporate biological and ecological factors into these analyses. Specifically species-specific data on interspecific or predator interaction, age and diet, and a broad range of anthropogenic threats, should not be omitted. However, terrestrial wildlife health programs demonstrate the importance of infectious disease surveillance, which mostly lack in marine mammal health programs. Marine mammal infectious disease outbreaks are, however, on the rise (Gulland and Hall, 2007; Sanderson and Alexander, 2020), highlighting momentum for change in surveillance procedures. Increased and improved marine mammal health and infectious disease surveillance programs will be vital for long-term population health monitoring and to provide essential data for developing early warning frameworks, forecasting models, and species- and location-specific adaptive management plans (Burek et al., 2008; Burge et al., 2014; Sanderson and Alexander, 2020). For our case, the harbor porpoises in the southern North Sea, data and samples of just over a decade have now been collected and stored, reflecting at least one generation of porpoises. The current data and analyses can thus be seen as a baseline or be used in comparison to future cases sampled under changing environments or health conditions.
The original contributions presented in the study are included in the article/Supplementary Material. Further inquiries can be directed to the corresponding author.
Ethical approval was not required for the study involving animals in accordance with the local legislation and institutional requirements because the animals described in this study were free-living harbor porpoises which died of natural causes or were euthanized on welfare grounds and not for the purpose of this, or other, studies. No consent from an Animal Use Committee is required, as the animals described in this study were not used for scientific or commercial testing. Consequently, animal ethics committee approval was not applicable to this work.
LI: Conceptualization, Data curation, Formal analysis, Investigation, Methodology, Visualization, Writing – original draft. JB: Conceptualization, Formal analysis, Investigation, Methodology, Writing – review & editing. MK: Data curation, Investigation, Writing – review & editing. ML: Data curation, Writing – review & editing. ER: Data curation, Writing – review & editing. AG: Data curation, Investigation, Supervision, Writing – review & editing. HH: Conceptualization, Methodology, Supervision, Writing – review & editing.
The author(s) declare financial support was received for the research, authorship, and/or publication of this article. Necropsies of harbor porpoises in The Netherlands are commissioned by the Ministry of Agriculture, Nature and Food Quality, and since 2016 embedded under the Legal Research Tasks Nature & Environment unit of Wageningen UR (project reference numbers 140000353, WOT-04-009-045).
We sincerely thank the stranding network volunteers and organizations for reporting and retrieving deceased harbor porpoises for our research. The post-mortem investigations and stomach analyses were conducted with the help of many colleagues, students and volunteers, for which we specifically want to acknowledge Johan van Amerongen, Lineke Begeman, Louis van den Boom, Kees Camphuysen, Natashja Ennen-Buijs, Sjoukje Hiemstra, Thierry Jauniaux, Guido Keijl, Darryl Leydekkers, Manon Lock, Liliane Solé, Mariel ten Doeschate, Linde van Schalkwijk, Ruby Wagensveld and Lidewij Wiersma. In addition, we are thankful for the help of Folchert van Dijken, Jeroen Vis, Anne-Marie Svoboda and Steve Geelhoed in funding acquisition and project management.
The authors declare that the research was conducted in the absence of any commercial or financial relationships that could be construed as a potential conflict of interest.
All claims expressed in this article are solely those of the authors and do not necessarily represent those of their affiliated organizations, or those of the publisher, the editors and the reviewers. Any product that may be evaluated in this article, or claim that may be made by its manufacturer, is not guaranteed or endorsed by the publisher.
The Supplementary Material for this article can be found online at: https://www.frontiersin.org/articles/10.3389/fmars.2023.1306294/full#supplementary-material
Abdi H., Williams L. J. (2010). Principal component analysis. Wiley Interdiscip. Reviews: Comput. Stat 2, 433–459. doi: 10.1002/wics.101
Aguirre A. A., Tabor G. (2004). Introduction: Marine vertebrates as sentinels of marine ecosystem health. Ecohealth 1, 236–238. doi: 10.1007/s10393-004-0091-9
Akoglu H. (2018). User's guide to correlation coefficients. Turkish J. Emergency Med. 18 (3), 91–93. doi: 10.1016/j.tjem.2018.08.001
Arbelo M., de Los Monteros A. E., Herráez P., Andrada M., Sierra E., Rodríguez F., et al. (2013). Pathology and causes of death of stranded cetaceans in the Canary Islands, (1999– 2005). Dis. Aquat. Organisms 103 (2), 87–99. doi: 10.3354/dao02558
Artois M., Bengis R., Delahay R. J., Duchêne M. J., Duff J. P., Ferroglio E., et al. (2009). Wildlife disease surveillance and monitoring. Manage. Dis. Wild Mammals, 187–213. doi: 10.1007/978-4-431-77134-0_10
ASCOBANS (1992) Agreement on the conservation of small cetaceans of the Baltic, North-East Atlantic, Irish and North Seas. Available at: https://www.ascobans.org/en/documents/agreement-text.
Barnett J., Dastjerdi A., Davison N., Deaville R., Everest D., Peake J., et al. (2015). Identification of novel cetacean poxviruses in cetaceans stranded in South West England. PloS One 10 (6), e0124315. doi: 10.1371/journal.pone.0124315
Berry M. W., Mohamed A., Yap B. W. (2020). Supervised and unsupervised learning for data science (Springer Nature Switzerland). doi: 10.1007/978-3-030-22475-2
Bossart G. D. (2011). Marine mammals as sentinel species for oceans and human health. Oceanography 19 (2), 134–137. doi: 10.1177/0300985810388525
Burek K. A., Gulland F. M., O'Hara T. M. (2008). Effects of climate change on Arctic marine mammal health. Ecol. Appl. 18 (sp2), S126–S134. doi: 10.1890/06-0553.1
Burge C. A., Mark Eakin C., Friedman C. S., Froelich B., Hershberger P. K., Hofmann E. E., et al. (2014). Climate change influences on marine infectious diseases: Implications for management and society. Annu. Rev. Mar. Sci. 6, 249–277. doi: 10.1146/annurev-marine-010213-135029
Clausen B., Andersen S. (1988). Evaluation of bycatch and health Status of the harbour porpoise (Phocoena phocoena) in Danish waters. Danish Rev. Game Biol. 13, 1–22.
Cozzi B., Huggenberger S., Oelschläger H. A. (2017). Anatomy of dolphins: insights into body structure and function (Academic Press).
Desforges J. P., Hall A., McConnell B., Rosing-Asvid A., Barber J. L., Brownlow A., et al. (2018). Predicting global killer whale population collapse from PCB pollution. Science 361 (6409), 1373–1376. doi: 10.1126/science.aat1953
Fernández A., Edwards J. F., Rodriguez F., De Los Monteros A. E., Herraez P., Castro P., et al. (2005). “Gas and fat embolic syndrome” involving a mass stranding of beaked whales (family Ziphiidae) exposed to anthropogenic sonar signals. Veterinary Pathol. 42 (4), 446–457. doi: 10.1354/vp.42-4-446
Foster G., Whatmore A. M., Dagleish M. P., Malnick H., Gilbert M. J., Begeman L., et al. (2019). Forensic microbiology reveals that Neisseria animaloris infections in harbour porpoises follow traumatic injuries by grey seals. Sci. Rep. 9 (1), 1–8. doi: 10.1038/s41598-019-50979-3
Gower J. C. (1967). A comparison of some methods of cluster analysis. Biometrics, 623–637. doi: 10.2307/2528417
Gulland F. M., Baker J. D., Howe M., LaBrecque E., Leach L., Moore S. E., et al. (2022). A review of climate change effects on marine mammals in United States waters: Past predictions, observed impacts, current research and conservation imperatives. Climate Change Ecol. 3, 100054. doi: 10.1016/j.ecochg.2022.100054
Gulland F. M., Hall A. J. (2007). Is marine mammal health deteriorating? Trends in the global reporting of marine mammal disease. EcoHealth 4 (2), 135–150. doi: 10.1007/s10393-007-0097-1
Hallanger I. G., Warner N. A., Ruus A., Evenset A., Christensen G., Herzke D., et al. (2011). Seasonality in contaminant accumulation in Arctic marine pelagic food webs using trophic magnification factor as a measure of bioaccumulation. Environ. Toxicol. Chem. 30 (5), 1026–1035. doi: 10.1002/etc.488
Halpern B. S., Walbridge S., Selkoe K. A., Kappel C. V., Micheli F., D'agrosa C., et al. (2008). A global map of human impact on marine ecosystems. Science 319 (5865), 948–952.10.1126/science.1149345
IJsseldijk L. L., Begeman L., Duim B., Gröne A., Kik M. J., Klijnstra M. D., et al. (2023). Harbor porpoise deaths associated with Erysipelothrix rhusiopathiae, the Netherlands 2021. Emerg. Infect. Dis. 29 (4), 835. doi: 10.3201/eid2904.221698
IJsseldijk L. L., Brownlow A. C., Mazzariol S. (2019). Best practice on cetacean post mortem investigation and tissue sampling. Joint ACCOBAMS and ASCOBANS document.10.1007/978-3-030-22475-2
IJsseldijk L. L., Leopold M. F., Begeman L., Kik M. J., Wiersma L., Morell M., et al. (2022). Pathological findings in stranded harbor porpoises (Phocoena phocoena) with special focus on anthropogenic causes. Front. Mar. Sci. 9, 997388. doi: 10.3389/fmars.2022.997388
IJsseldijk L. L., Scheidat M., Siemensma M. L., Couperus B., Leopold M. F., Morell M., et al. (2021). Challenges in the assessment of bycatch: Postmortem findings in harbor porpoises (Phocoena phocoena) retrieved from gillnets. Veterinary Pathol. 58 (2), 405–415. doi: 10.1177/0300985820972454
IJsseldijk L. L., ten Doeschate M. T. I., Brownlow A. C., Davison N. J., Deaville R., Galatius A., et al. (2020). Spatiotemporal mortality and demographic trends in a small cetacean: Strandings to inform conservation management. Biol. Conserv. 249, 108733. doi: 10.1016/j.biocon.2020.108733
James G., Witten D., Hastie T., Tibshirani R. (2017). An Introduction to Statistical Learning with Applications in R (Nature Switzerland: Springer). doi: 10.1007/978-1-0716-1418-1
Jauniaux T., Petitjean D., Brenez C., Borrens M., Brosens L., Haelters J., et al. (2002). Post-mortem findings and causes of death of harbour porpoises (Phocoena phocoena) stranded from 1990 to 2000 along the coastlines of Belgium and Northern France. J. Comp. Pathol. 126 (4), 243–253. doi: 10.1053/jcpa.2001.0547
Jepson P. D., Baker J. R., Kuiken T., Simpson V. R., Kennedy S., Bennett P. M. (2000). Pulmonary pathology of harbour porpoises (Phocoena phocoena) stranded in England. Veterinary Rec. 146, 721–728. doi: 10.1136/vr.146.25.721
Jepson P. D., Bennett P. M., Allchin C. R., Law R. J., Kuiken T., Baker J. R., et al. (1999). Investigating potential associations between chronic exposure to polychlorinated biphenyls and infectious disease mortality in harbour porpoises from England and Wales. Sci. Total Environ. 243, 339–348. doi: 10.1016/S0048-9697(99)00417-9
Jepson P. D., Bennett P. M., Deaville R., Allchin C. R., Baker J. R., Law R. J. (2005). Relationships between polychlorinated biphenyls and health status in harbor porpoises (Phocoena phocoena) stranded in the United Kingdom. Environ. Toxicol. Chem.: Int. J. 24 (1), 238–248. doi: 10.1897/03-663.1
Jepson P. D., Deaville R., Barber J. L., Aguilar À., Borrell A., Murphy S., et al. (2016). PCB pollution continues to impact populations of orcas and other dolphins in European waters. Sci. Rep. 6, 18573. doi: 10.1038/srep18573
Kapetanou A., IJsseldijk L. L., Willems D. S., Broens E. M., Everaarts E., Buil J. B., et al. (2020). Mycotic infections in free-ranging Harbor porpoises (Phocoena phocoena). Front. Mar. Sci. 7, 344. doi: 10.3389/fmars.2020.00344
Kirkwood J. K., Bennett P. M., Jepson P. D., Kuiken T., Simpson V. R., Baker J. R. (1997). Entanglement in fishing gear and other causes of death in cetaceans stranded on the coasts of England and Wales. Veterinary Rec. 141 (4), 94–98. doi: 10.1136/vr.141.4.94
Kuiken T. (1996). “Review of the criteria for the diagnosis of by-catch in cetaceans,” in Diagnosis of by-catch in cetaceans: Proceedings of the second ECS workshop on cetacean pathology, 26, 1–7. Ed. Kuiken T. (Montpellier, France: European Cetacean Society Newsletter).
Kuiken T., Bennett P. M., Allchin C. R., Kirkwood J. K., Baker J. R., Lockyer C. H., et al. (1994). PCBs, cause of death and body condition in harbour porpoises (Phocoena phocoena) from British waters. Aquat. Toxicol. 28 (1-2), 13–28. doi: 10.1016/0166-445X(94)90017-5
Kuiken T., García Hartmann M. (1991). Proceedings of the first European Cetacean Society workshop on cetacean pathology: dissection techniques and tissue sampling Vol. 17 (ECS Newsletter).
Lehnert K., Raga J. A., Siebert U. (2005). Macroparasites in stranded and bycaught harbour porpoises from German and Norwegian waters. Dis. Aquat. Organisms 64 (3), 265–269. doi: 10.3354/dao064265
Leopold M. F., Begeman L., Heße E., van der Hiele J., Hiemstra S., Keijl G. O., et al. (2015a). Porpoises: from predators to prey. J. Sea Res. 97, 14–23. doi: 10.1016/j.seares.2014.12.005
Leopold M. F., Begeman L., van Bleijswijk J. D., IJsseldijk L. L., Witte H. J., Gröne A. (2015b). Exposing the grey seal as a major predator of harbour porpoises. Proc. R. Soc. B: Biol. Sci. 282 (1798). doi: 10.1098/rspb.2014.2429
McAloose D., Colegrove K. M., Newton A. L. (2018). “Wildlife Necropsy,” in Pathology of Wildlife and Zoo Animals. Eds. Terio K. A., McAloose D., St. Leger J. (Academic Press Elsevier), 1–21.
Murtagh F., Contreras P. (2012). Algorithms for hierarchical clustering: An overview. Wiley Interdiscip. Reviews: Data Min. Knowledge Discovery 2, 86–97. doi: 10.1002/widm.53
Neimanis A., Stavenow J., Ågren E. O., Wikström-Lassa E., Roos A. M.. (2022). Causes of death and pathological findings in stranded harbour porpoises (Phocoena phocoena) from Swedish waters. Animals 12 (3), 369.
Orozco M. M., Argibay H. D., Minatel L., Guillemi E. C., Berra Y., Schapira A., et al. (2020). A participatory surveillance of marsh deer (Blastocerus dichotomus) morbidity and mortality in Argentina: first results. BMC Veterinary Res. 16, 1–18. doi: 10.1186/s12917-020-02533-x
Pierce G. J., Santos M. B., Murphy S., Learmonth J. A., Zuur A. F., Rogan E., et al. (2008). Bioaccumulation of persistent organic pollutants in female common dolphins (Delphinus delphis) and harbour porpoises (Phocoena phocoena) from western European seas: Geographical trends, causal factors and effects on reproduction and mortality. Environ. pollut. 153 (2), 401–415. doi: 10.1016/j.envpol.2007.08.019
R Core Team (2017). R: A language and environment for statistical computing. Available at: https://www.r-project.org/.
Reckendorf A., Everaarts E., Bunskoek P., Haulena M., Springer A., Lehnert K., et al. (2021). Lungworm infections in harbour porpoises (Phocoena phocoena) in the German Wadden Sea between 2006 and 2018, and serodiagnostic tests. Int. J. Parasitol: Parasites Wildlife 14, 53–61. doi: 10.1016/j.ijppaw.2021.01.001
Ryser-Degiorgis M. P. (2013). Wildlife health investigations: needs, challenges and recommendations. BMC Veterinary Res. 9, 1–17. doi: 10.1186/1746-6148-9-223
Sanderson C. E., Alexander K. A. (2020). Unchartered waters: Climate change likely to intensify infectious disease outbreaks causing mass mortality events in marine mammals. Global Change Biol. 26, 4284–4301. doi: 10.1111/gcb.15163
Schick L., IJsseldijk L. L., Grilo M. L., Lakemeyer J., Lehnert K., Wohlsein P., et al. (2020). Pathological findings in white-beaked dolphins (Lagenorhynchus albirostris) and Atlantic white-sided dolphins (Lagenorhynchus acutus) from the south-eastern North Sea. Front. Veterinary Sci. 7, 262. doi: 10.3389/fvets.2020.00262
Sichewo P. R., Etter E. M. C., Michel A. L. (2020). Wildlife-cattle interactions emerge as drivers of bovine tuberculosis in traditionally farmed cattle. Prev. Veterinary Med. 174, 104847. doi: 10.1016/j.prevetmed.2019.104847
Sichewo P. R., Michel A. L., Musoke J., Etter E. M. C. (2019). Risk factors for zoonotic tuberculosis at the wildlife–livestock–human interface in South Africa. Pathogens 8, 1–14. doi: 10.3390/pathogens8030101
Siebert U., Pawliczka I., Benke H., von Vietinghoff V., Wolf P., Pilāts V., et al. (2020). Health assessment of harbour porpoises (Phocoena phocoena) from Baltic area of Denmark, Germany, Poland and Latvia. Environ. Int. 143, 105904. doi: 10.1016/j.envint.2020.105904
Siebert U., Stürznickel J., Schaffeld T., Oheim R., Rolvien T., Prenger-Berninghoff E., et al. (2022). Blast injury on harbour porpoises (Phocoena phocoena) from the Baltic Sea after explosions of deposits of World War II ammunition. Environ. Int. 159, 107014. doi: 10.1016/j.envint.2021.107014
Siebert U., Tolley K., Vikingsson G. A., Olafsdottir D., Lehnert K., Weiss R., et al. (2006). Pathological findings in harbour porpoises (Phocoena phocoena) from Norwegian and Icelandic waters. J. Comp. Pathol. 134 (2-3), 134–142. doi: 10.1016/j.jcpa.2005.09.002
Siebert U., Wünschmann A., Weiss R., Frank H., Benke H., Frese K. (2001). Post-mortem findings in harbour porpoises (Phocoena phocoena) from the German North and Baltic Seas. J. Comp. Pathol. 124 (2-3), 102–114. doi: 10.1053/jcpa.2000.0436
Simmonds M. P., Isaac S. J. (2007). The impacts of climate change on marine mammals: Early signs of significant problems. Oryx 41, 19–26. doi: 10.1017/S0030605307001524
Sobek A., McLachlan M. S., Borgå K., Asplund L., Lundstedt-Enkel K., Polder A., et al. (2010). A comparison of PCB bioaccumulation factors between an arctic and a temperate marine food web. Sci. total Environ. 408 (13), 2753–2760. doi: 10.1016/j.scitotenv.2010.03.013
Sonne C., Siebert U., Gonnsen K., Desforges J. P., Eulaers I., Persson S., et al. (2020). Health effects from contaminant exposure in Baltic Sea birds and marine mammals: A review. Environ. Int. 139, 105725. doi: 10.1016/j.envint.2020.105725
St. Leger J. S., Begeman L., leetwood M., Frasca S. Jr, Garner M. M., Lair S., et al. (2009). Comparative pathology of nocardiosis in marine mammals. Vet. Pathol. 46, 299–308. doi: 10.1354/vp.46-2-299
Unger B., Rebolledo E. L. B., Deaville R., Gröne A., IJsseldijk L. L., Leopold M. F., et al. (2016). Large amounts of marine debris found in sperm whales stranded along the North Sea coast in early 2016. Mar. pollut. Bull. 112 (1-2), 134–141. doi: 10.1016/j.marpolbul.2016.08.027
van Beurden S. J., IJsseldijk L. L., Ordonez S. R., Förster C., de Vrieze G., Gröne A., et al. (2015). Identification of a novel gammaherpesvirus associated with (muco) cutaneous lesions in harbour porpoises (Phocoena phocoena). Arch. Virol. 160, 3115–3120. doi: 10.1007/s00705-015-2607-8
van den Heuvel-Greve M. J., van den Brink A. M., Kotterman M. J., Kwadijk C. J., Geelhoed S. C., Murphy S., et al. (2021). Polluted porpoises: Generational transfer of organic contaminants in harbour porpoises from the southern North Sea. Sci. Total Environ. 796, 148936. doi: 10.1016/j.scitotenv.2021.148936
van Elk C. E., van de Bildt M. W., van Run P. R., Bunskoek P., Meerbeek J., Foster G., et al. (2019). Clinical, pathological, and laboratory diagnoses of diseases of harbour porpoises (Phocoena phocoena), live stranded on the Dutch and adjacent coasts from 2003 to 2016. Veterinary Res. 50 (1), 1–17. doi: 10.1186/s13567-019-0706-3
van Neer A., Gross S., Kesselring T., Grilo M. L., Ludes-Wehrmeister E., Roncon G., et al. (2020). Assessing harbour porpoise carcasses potentially subjected to grey seal predation. Sci. Rep. 10, 16345. doi: 10.1038/s41598-020-73258-y
Warns-Petit E., Morignat E., Artois M., Calavas D. (2010). Unsupervised clustering of wildlife necropsy data for syndromic surveillance. BMC Veterinary Res. 6 (56), 1:11. doi: 10.1186/1746-6148-6-56
Keywords: cetacean, post-mortem investigation, animal health surveillance, supervised classification methods, unsupervised classification methods, causes of death
Citation: IJsseldijk LL, van den Broek J, Kik MJL, Leopold MF, Bravo Rebolledo E, Gröne A and Heesterbeek H (2024) Using marine mammal necropsy data in animal health surveillance: the case of the harbor porpoise in the Southern North Sea. Front. Mar. Sci. 10:1306294. doi: 10.3389/fmars.2023.1306294
Received: 03 October 2023; Accepted: 26 December 2023;
Published: 15 January 2024.
Edited by:
K. David Hyrenbach, Hawaii Pacific University, United StatesReviewed by:
Frances Gulland, University of California, Davis, United StatesCopyright © 2024 IJsseldijk, van den Broek, Kik, Leopold, Bravo Rebolledo, Gröne and Heesterbeek. This is an open-access article distributed under the terms of the Creative Commons Attribution License (CC BY). The use, distribution or reproduction in other forums is permitted, provided the original author(s) and the copyright owner(s) are credited and that the original publication in this journal is cited, in accordance with accepted academic practice. No use, distribution or reproduction is permitted which does not comply with these terms.
*Correspondence: Lonneke L. IJsseldijk, TC5MLklKc3NlbGRpamtAdXUubmw=
Disclaimer: All claims expressed in this article are solely those of the authors and do not necessarily represent those of their affiliated organizations, or those of the publisher, the editors and the reviewers. Any product that may be evaluated in this article or claim that may be made by its manufacturer is not guaranteed or endorsed by the publisher.
Research integrity at Frontiers
Learn more about the work of our research integrity team to safeguard the quality of each article we publish.