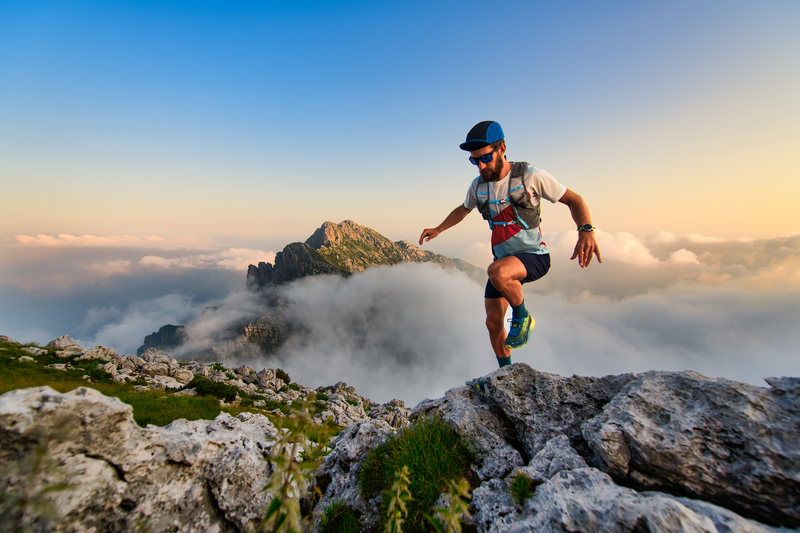
95% of researchers rate our articles as excellent or good
Learn more about the work of our research integrity team to safeguard the quality of each article we publish.
Find out more
ORIGINAL RESEARCH article
Front. Mar. Sci. , 20 December 2023
Sec. Deep-Sea Environments and Ecology
Volume 10 - 2023 | https://doi.org/10.3389/fmars.2023.1272066
Cold-water corals (CWCs) are bioengineering species that can increase habitat heterogeneity and improve the deep sea’s biological diversity and ecosystem functioning. Knowledge of their distribution provides a critical baseline for assessing the effect of natural and anthropogenic impacts on these important deep-sea habitats. The aims of this study are: i) provide new data on the spatial distribution of six CWCs species in the Strait of Sicily, ii) describe the principal environmental and anthropogenic variables that play a role in shaping their distribution, iii) identify hotspots in which individuals belonging to the various species co-occur. Presence-only data of six CWCs species, ten environmental variables (depth, slope, rugosity, aspect, flowdir, temperature, salinity, north bottom current, east bottom current, chlorophyll-a), and one variable relating to bottom trawling effort (Automatic Information System – AIS) were used to predict the suitable habitats. We used Maximum Entropy modelling (MaxEnt) approach and used the AUC (area under the receiver operating characteristic curve) and TSS (true skill statistics) to evaluate the model performance. The results showed excellent AUC, TSS and AUC’s standard deviation mean values for all six species. The validation show high predictive performance. MaxEnt identified slope, depth, and rugosity as the most important predictors, showing the highest percentage contribution for all six species considered. Throughout the study area, highlyinterspecific persistent density hotspot of CWCs co-occurrence were discovered, with a total extension of 4.05 km2 where all species co-occur. Although studies on the effect of environmental and anthropogenic factors that impact the distribution of these species of conservation interest remain scarce, the results of this study offer useful guidance for decision-makers to develop necessary conservation measures.
Cold Water Corals (CWCs) are among the most important habitat-forming and bioengineering species in the Mediterranean Sea (Chimienti et al., 2019; Barbosa et al., 2020; Chimienti et al., 2020). Due to their life-history characteristics (Huvenne et al., 2016; Bargain et al., 2017; Barbosa et al., 2020), CWCs are very sensitive to environmental modification and anthropogenic pressure (Chu et al., 2019; Sundahl et al., 2020), such as bottom trawling, ocean acidification, and pollution (Bargain et al., 2017). Considering these traits, CWCs are categorised as Vulnerable Marine Ecosystems (VMEs) (FAO (Food and Agriculture Organization), 2009; Ashford et al., 2019; Barbosa et al., 2020) and many of these species are also listed in the International Union for Conservation of Nature (IUCN) Red List as “threatened” or “endangered” (Otero et al., 2017). In addition, many commercial and non-commercial fish and invertebrate species use these habitats as nursery, feeding, and refuge areas highlighting their key ecological role as Essential Fish Habitats (EFHs) (D’Onghia et al., 2017; D’Onghia et al., 2019; Lo Iacono et al., 2018).
In the last decade, several papers reported the presence of CWCs in the deep areas of the Mediterranean. A particular region is represented by the Strait of Sicily. This area is characterised by a pronounced bathy-morphological heterogeneity, peculiar circulation patterns, and diverse seabed typology. In this region, the Atlantic waters divide into two branches, one flowing into the Tyrrhenian Sea and the other into the Sicilian Channel (Astraldi et al., 1996; Astraldi et al., 2002; Di Lorenzo et al., 2018). The latter turns branches into two ‘arms’: Atlantic Ionic water and Atlantic Tunisian water. The first one favours the formation of two vortices (one over the Adventure bank and the second off Cape Passero), with a complex circulation system that transports water between eastern and western sub-basins generating upwelling (Manzella et al., 1990; Di Lorenzo et al., 2018) and making the Strait of Sicily a highly productive area (Agostini and Bakun, 2002). Currents are considered one of the most critical variables governing CWCs dispersion since they supply food for the corals and keep them from getting buried by sediments (Thiem et al., 2006; Taviani et al., 2011; Chimienti et al., 2019).
However, scattered information about the distribution of CWC species has been reported in the Strait of Sicily as in many other regions of the Mediterranean Sea (Angiolillo et al., 2021). It is essential to increase the knowledge of deep-sea habitats (mesophotic and deep zone) to ensure adequate protection and management measures are implemented (Chimienti et al., 2020), as despite the increase in studies, very few of these species have been mapped and consequently information on these habitats in the basin remains scarce (Savini et al., 2014).
In this framework, understanding the spatial distribution of CWC ecosystems and the primary factors determining their occurrence represent the first step in applying the appropriate management and protection plans (Otero and Marin, 2019; Sundahl et al., 2020; Lauria et al., 2021).
Nowadays, modern technology, such as multi-beam echosounders and ROVs, are used for much more accurate prediction modelling (Yesson et al., 2012; Buhl-Mortensen et al., 2015; Sundahl et al., 2020; Abad-Uribarren et al., 2022). Habitat Suitability Models (HSMs) have grown significantly in resource management and conservation biology in the last few years. These models are currently used for determining the distribution of suitable habitats for VMEs in deep oceans (Stephenson et al., 2021), expanding the knowledge of their distribution at a global scale (Bargain et al., 2017).
Within this study, habitat modelling technique were used to create predictive maps of six CWC species of conservation concern in the Mediterranean Sea: Scleractinia (Madrepora oculata, Desmophyllum pertusum, Dendrophyllia cornigera), Antipatharia (Leiopathes glaberrima) and Scleralcyonacea represented by Callogorgia verticillata and Isidella elongata, and to find areas where the greatest number of these species co-occur. The species were chosen because they are among the most common arborescent VMEs found in the research area, taking into account both hard and soft bottoms.
This study aimed to: i) provide new data on the spatial distribution of six CWC species in the Strait of Sicily, ii) describe the principal environmental and anthropogenic variables that play a role in shaping their distribution, iii) identify hotspots in which individuals belonging to the various species co-occur.
The study area is located in the northern part of the Strait of Sicily (38°0,587’N; 11°19,329’E, study area’s centroid) (Figure 1), which covered 1651 km2, under MedWind project.
Figure 1 5 m resolution Multi-beam (MB) bathymetric model of the study area. MB data used for this map were obtained using a Multi-beam echosounder Kongsberg EM2040 in shallower waters (from 150 to 300 meters) and a Kongsberg EM710-MK2 in deeper waters (from 300 to 1000 meters). The lines (in red) indicate the 140 transects performed.
Acoustic surveys were conducted from August 12th to September 6th, 2021. Seafloor morphology was acquired using a Multi-beam echosounder Kongsberg EM2040 in shallower waters (150 to 300 meters) and a Kongsberg EM712-MK2 in deeper waters (300 to 1000 meters). Raw acoustic data were processed to produce a 5 m cell Digital Terrain Model (DTM) of the entire area. ROV surveys were carried out from September 11th to November 17th 2021 (67 days in total) from the MainportGeo vessel, which was equipped with a Tomahawk ROV Light Work Class with two manipulators five functions, four cameras (full HD, standard colour, standard black and white and high definition 6K camera), two laser beams, sampling box, beacon, DVL system and Seabird Microcat SBE 37. A total of 140 transects were carried out in a depth range from 135 to 985 m. The length of each transect was variable, for a total of 129.5 km explored (mean 929 ± 257 dev.st). During video surveys, OFOP (Ocean Floor Observation Protocol) (van den Beld et al., 2007) data logging software was used to record each observation with its corresponding information (date, time, the ROV’s and ship’s positions, depth, substrate type and species observed). Georeferenced presence data for the six species was extrapolated from the data set. The number of organisms of each species was standardised according to the length of each transect. As a result, the final unit of measurement is n organisms x linear km. In the same period of the ROV survey, Oceanographic parameters (Temperature and Salinity) were collected in 97 random stations using a Rosette and CTD (Sea-Bird Scientific SBE 911 Plus V2). Coordinates (Latitude and Longitude) and depth for all 97 CTD points are given in Table 1 of the Supplementary Materials.
Eleven predictor variables were considered in the models, subdivided into terrain (depth, slope, roughness, aspect, flowdir), oceanographic variables (chlorophyll-a, temperature, salinity, current north, current east) and fishing effort of bottom trawling activities (AIS data) (Table 1; Figure 2). Terrain variables (slope, rugosity aspect and flowdir) were extracted from high-resolution Multi-beam Depth data (resolution 5 m) through the package “raster”, function “terrain” in the R software (Hijmans, 2023).
Table 1 Principal predictor variables used to produce the habitat suitability model for CWCs species in the Northern part of the of Strait of Sicily.
Figure 2 Spatial patterns of the environmental predictors used in the MaxEnt model. These consist of Slope (A), Depth (B), Rugosity (C), Aspect (D), Flowdir (E), Salinity (F), Temperature (G), Chlorophyll-a (H), East bottom current (I), North bottom current (J) and Fishing effort (AIS data) (K). These maps were performed in R environment.
The slope is an important variable for determining benthic habitat distribution, contributing to an increase in current flow, which benefits food supply, crucial for filter-feeding organisms (Mohn and Beckmann, 2002). Furthermore, because it restricts the use of some fishing gear, the slope can significantly lessen anthropogenic influence in some regions (Wilson et al., 2007). Its values range from 0 to 90°, with low values often corresponding to gentle slopes and high values typically corresponding to steep slopes (Wilson et al., 2007), and are not always connected to rocky substrates. Increasing slope value is related to increased terrain complexity (Bargain et al., 2018).
“Rugosity” indicates the seafloor’s complexity; in this case, low values indicate a soft seabed, while high values indicate a rocky seabed. The values were calculated as the difference in elevation between two adjacent pixels, with low values meaning no terrain variation and high values meaning terrain variation. Aspect indicates the orientation of the seabed and provides data on a certain area’s exposure to local and regional currents (Wilson et al., 2007). Flowdir indicates the direction of the substrate slope. Along with rugosity, aspect and slope provide a wider and clearer view of the substrate (Qin et al., 2007).
Regarding temperature and salinity, a continuous raster was created for both variables from the 97 CTD sampling points within the entire study area. To do this, a co-kriging analysis was carried out using temperature or salinity as respondent variables and bathymetry as a co-variable, which is important to predict the trend of the two respondent variables. The co-kriging analysis, performed in R using the ‘gstat’ package (Pebesma, 2004), provided the prediction of the target variable at unsampled points from the co-variables. north bottom current, east bottom current and chlorophyll-a (chl-a), were collected from the Copernicus Marine Service and refer to the year 2021. These three Copernicus Marine Service predictors were aggregated as an annual average. The trawling fishing effort was assessed in the study area using data acquired by Automatic Identification System (AIS) for the year 2021. The temporal frequency of vessel locations was standardised and interpolated ad 0.01 degree; fishing trips and fishing set position by trips (hauls) were detected using speed and depth filters (Russo et al., 2016). Finally, the yearly amount of effective trawling effort (ETE) was estimated, with respect to the cells of the same 1 km x 1 km grid described above, as the cumulative sum of the number of fishing hours.
Habitat suitability maps for the six species of CWCs were performed using Maximum Entropy method (MaxEnt), a modelling approach used to identify probability distribution with the highest level of entropy when only species presence data are provided (Etnoyer et al., 2018). MaxEnt was used to create a model that connected every georeferenced observation to a set of predictor variables to predict habitat distribution in terms of the probability of suitability for species distribution (Phillips et al., 2006; Elith et al., 2011; Fabri et al., 2017). Variance Inflation Factor (VIF) correlation was produced to identify the variables to be included in MaxEnt models and eliminate those with high collinearity, variables with correlation values more the 5 were removed (Supplementary Table 2).
The MaxEnt model was trained using batch files that allowed us to generate multiple models. Several default parameters were left unchanged, such as;10-5 and 500 for convergent threshold and maximum interaction value, respectively, and maximum randomly background points of 10,000 as suggested by other authors (Phillips & Dudík, 2008; Anderson et al., 2016; Bargain et al., 2017). For each species, we made four models. The predictions model were calculated on 10 replicates per species for each “Regularization Multiplier” (RM) parameter values (values of 1, 1.5, 2 and 2.5, respectively). RM is a key parameter that, at higher values, represents if the forecast will be more widespread and less localised (Phillips, 2017), reducing the ‘overfitting’ of the data (Bargain et al., 2018). Moreover, the logistic outputs were chosen since they assess the probability of existence conditional on environmental variables and are simpler to understand. A k-fold cross-validation approach was employed with the MaxEnt model to evaluate the degree of uncertainty in the model’s predictions. To compare the training and test datasets to validate the model, presence data were divided into 10 randomly generated partitions. This made it possible to acquire estimates of the predictive performance and uncertainty surrounding the fitted functions outside of the sample. The accuracy of the prediction of the models was calculated using Receiver Operating Characteristics (ROC) analysis through a comparison of the area Under the ROC Curves (AUC) and the True Skill Statistic (TSS) value (Phillips et al., 2006; Liu et al., 2016). The AUC value is related to the model’s reliability concerning the data. AUC value ranges from 0 to 1: less than 0.5 indicates that the model does not fit the data well, more than 0.7 is considered acceptable, and a value of 1 indicates an ideal model result (Bargain et al., 2017). Considering TSS value range, 0.2-0.5 indicate a poor model performance, 0.6-0.8 is useful and greater than 0.8 indicate an excellent model performance (Li et al., 2023). According to the study of Liu et al., 2016, for each model, we selected the maxSSS values (maximum sum of sensitivity and specificity) as the threshold and calculated the average TSS of the results of the 10 replicates of the MaxEnt model to evaluate model performance. Test gain (which is a measure of goodness of fit) was also applied to evaluate how close the model is to the test presence samples (if the gain is 2, it means that the average likelihood of the presence samples is exp(2)=7.4 times higher than that of a random background pixel (Phillips et al., 2017). Moreover, each variable’s contribution to the predictive model was also examined. A Jackknife test highlighted the percentage that each variable contributed to the final MaxEnt habitat suitability model. Jackknife tests examine how well the model predicts when only one of each variable is present, followed by all the variables save the one tested first. Then, habitat suitability mapping was done using the mean model of 10 replicates. The obtained probability maps of CWCs distribution have been processed using the R environment to visualise the probability of presence in the study area. From the predictive maps obtained for each species, the coverage of the species in the study area, in terms of km2, was determined. Four different ranges of occurrence probability were used.
The R package “geostats” (Vermeesch, 2022) was used to identify density hotspots on species distribution maps. Using the GetisOrd algorithm (Getis and Ord, 1992; Ord and Getis, 1995), it was possible to analyse the continuous raster of presence probability values for each species and delimit continuous areas where the probability of occurrence of the species is significantly high (hot spot area) and areas where it is significantly low (cold spot area). Z-core, p-value, and confidence level bin (Gi Bin) are the results of the analysis. The Gi Bin variable categorises the data into one of the three categories, ranging from -3 (cold spot – 99% confidence) to 3 (hot spot – 99% confidence). Features in the ± 3 bins have statistical significance with a confidence level of 99%; features in the ± 2 bins have a confidence level of 95%; features in the ± 1 bins have a confidence level of 90%; and clustering for features in bin 0 are not statistically significant (Milisenda et al., 2021).
Co-occurrence of species was defined as an area occupied by the largest probability of occurrence of a cell belonging to a hotspot of given species. This area was identified using R software, which extracted the area where the hot spots overlapped. The overlap rate for each grid cell was calculated using the Index of Co-occurrence (CI) (Fiorentino et al., 2003; Colloca et al., 2009; Milisenda et al., 2021) to determine the relative persistence of a cell as a potential zone for species aggregation. The cell is categorised as belonging to a hot spot of a given species. This index was calculated as the sum of the number of species categorised as a hot spot in a specific area using the formula:
When grid cell (“i”) is included in a hot spot of species “j”, ij = 1; otherwise, ij = 0; n is the total number of species. When density hotspots were not observed, the CI is reduced to 0, but when density hotspots exist for all species considered, CI increases to 6 for the cell “i”. Results were plotted in a single co-occurrence map showing a scale of different co-occurrence classes (from 0 to 6).
In addition, Spearman analysis was used to evaluate correlations between species and the R function “ggcorrplot” was used to calculate and visualise positive or negative correlations among species distribution (Kassambara and Kassambara, 2019).
ROV surveys allowed observation and mapping of the six target CWC species’ occurrence (Figure 3): M. oculata n=4342, D. pertusum n=582, D. cornigera n=192, L. glaberrima n=375, C. verticillata n=3221, I. elongata n=10378.
Figure 3 Representative images of the six target CWCs species whose distribution and co-occurrence were modelled in this study: Madrepora oculata (A), Leiopathes glaberrima (B), Callogorgia verticillata (C), Desmophyllum pertusum (D), Dendrophyllia cornigera (E) and Isidella elongata (F).
Considering all species, MaxEnt model performed well using cross-validation, with a mean AUC value greater than 0.8 and a standard deviation between 0.008 and 0.02, showing that our models were significantly better than random. Therefore, a maximum and minimum test gain value of 2.87 (for D. cornigera) and 0.69 (for I. elongata), meaning that the average likelihood of occurrence samples for these two species is 17.63 and 1.94 respectively [exp(test gain)] times higher than that of a random background pixel. Only for I. elongata, the TSS value is 0.6; for the other five species is more than 0.8. Table 2 shows the value of AUC, standard deviation, test gain with all variables, the average likelihood of the presence of samples and mean TSS for all six species. These data allow us to measure the goodness of fit.
These results suggest the effectiveness of the models and the accuracy of their predictions of habitat distribution of the six CWCs species in the Strait of Sicily.
Salinity was deleted from all models after the VIF correlation (Supplementary Table 2). Table 3 provides the percentage variable contribution retained in the final model for each species, using the jackknife contribution test. MaxEnt identified slope, depth, rugosity, temperature and current north as the four variables contributing to all six CWC species (all predictors outputs for all species are present in Supplementary Material). The slope represents the key environmental predictor explaining most of the variance. The maximum and minimum values are expressed for M. oculata (70.2%) and I. elongata (46%).
Table 3 Percentage of contribution for each predictor on the distribution of the six CWCs species included in the final MaxEnt model.
All species exhibit a curvilinear positive connection with slope, with a greater probability of occurrence in areas with high slope values. More specifically, M. oculata (Supplementary Figure 1A), D. pertusum (Supplementary Figure 2A), D. cornigera (Supplementary Figure 3A) and L. glaberrima (Supplementary Figure 4A) showed a constant positive correlation. The sea fans C. verticillata (Supplementary Figure 5A), show an optimum range of 0.49. Furthermore, the bamboo coral I. elongata (Supplementary Figure 6A), also shows a positive correlation and plateau at low slope values can be observed (around 0.3).
Depth also had a significant impact on the habitat preferences of CWC species. It showed a high probability of occurrence associated with the depth range of 430/750 m for M. oculata, (Supplementary Figure 1B) and D. pertusum (Supplementary Figure 2B). The graphs show a continuous positive correlation from 900 to 200, 700 to 200 and 900 to 150 m for D. cornigera (Supplementary Figure 3B), L. glaberrima (Supplementary Figure 4B) and C. verticillata (Supplementary Figure 5B), respectively. Instead, I. elongata (Supplementary Figure 6B) shows an optimum range between 800 and 500 m of depth.
Rugosity is an important terrain variable that explains the complexity of the substrate. The highest values were associated with M. oculata (Supplementary Figure 1C) and L. glaberrima (Supplementary Figure 4C) (300 and 250, respectively), and the lowest value was associated with I. elongata (Supplementary Figure 6C) (< 20). Dendrophyllia cornigera (Supplementary Figure 3C) showed an optimal value between 150 and 200. Callogorgia verticillata (Supplementary Figure 5C) showed values of around 50, while D. pertusum (Supplementary Figure 2C) showed an optimum of around 250 and 350.
The average response curve for Temperature shows higher probabilities of occurrence between 14°C and 15°C for M. oculata (Supplementary Figure 1G), D. pertusum (Supplementary Figure 2F), D. cornigera (Supplementary Figure 3G) and L. glaberrima (Supplementary Figure 4D). Callogorgia verticillata (Supplementary Figure 5F) show two peaks, to 14.3°C and 15°C. For I. elongata (Supplementary Figure 6E), the higher probability of occurrence is between 14.2°C and 14.7°C.
North bottom current appeared to be positively correlated with M. oculata (Supplementary Figure 1H), D. pertusum (Supplementary Figure 2G) and I. elongata (Supplementary Figure 6G) with an optimal of 0.9 and 0.10 m/s for the first two species and 0.7m/s for I. elongata, while was negatively correlated with D. cornigera (Supplementary Figure 3H), L. glaberrima (Supplementary Figure 4F) and C. verticillata (Supplementary Figure 1H) the probability of presence decreased at high current values.
East bottom current was only relevant for three species: L. glaberrima (Supplementary Figure 4E) C. verticillata (Supplementary Figure 5G) and I. elongata (Supplementary Figure 6F). The first one shows a linear positive correlation starting from low current values. For the second, two optimality ranges can be observed, one between 0.05 – 0.012 m/s and one at 0.25 m/s. Instead, I. elongata showed a negative correlation.
Focusing on the other main predictors with a significant contribution rate for the considered species, the variables were found to be: chl-a for M. oculata (Supplementary Figure 1F) D. cornigera (Supplementary Figure 3F) C. verticillata (Supplementary Figure 5E) and I. elongata (Supplementary Figure 6D). The highest suitability was found for values between 0.077 – 0.082 mg*m3 for M. oculata, and an optimal peak at the value of 0.82 mg*m3 for D. cornigera, and 0.077 mg*m3 for both C. verticillata and I. elongata.
Regarding bottom trawling effort (AIS) being relevant for D. pertusum (Supplementary Figure 2H), L. glaberrima (Supplementary Figure 4G) and I. elongata (Supplementary Figure 6H). Fishing effort significantly negatively affects the probability of occurrence for D. pertusum and I. elongata. As fishing efforts increased, the probability of occurrence of the species decreased. In the case of L. glaberrima, however, we can observe a positive correlation. Aspect and flowdir, on the other hand, provide a low contribution for four species considered: M. oculata (Supplementary Figures 1D, E), D. pertusum (Supplementary Figures 2D, E), D. cornigera (Supplementary Figures 3D, E), and C. verticillata (Supplementary Figure 5D).
Areas with suitable conditions for the occurrence of CWC species are shown in Figure 4. Madrepora oculata shows a higher probability of occurrence in the South and South-West area, L. glaberrima in the North, West and South-West area and C. verticillata in the North and South-West area. These three species show the presence of small patches in delimitated geographical areas. Desmophyllum pertusum and D. cornigera, were more randomly distributed, while for I. elongata, suitable habitat extended throughout the entire study area, with a higher probability in the South-East area.
Figure 4 Habitat suitability maps for Madrepora oculata (A), Desmophyllum pertusum (B), Dendrophyllia cornigera (C), Leiopathes glaberrima (D), Callogorgia verticillata (E) and Isidella elongata (F) in the Northern part of the Strait of Sicily using MaxEnt model. The legend shows the probability of occurrence values (high values in green and low values in white).
The probability of highly persistent density hotspots of CWCs occurrence were found scattered throughout the entire study area, with relevance in the northern, southern, south-eastern and south-western parts (Figure 5). Areas identified as suitable (hotspots) for the six CWCs species covered an extension of 206.8 Km2, 172.4 km2 and 105 km2 for the Scleractinia M. oculata, D. pertusum and D. cornigera respectively, 119.3 km2 for the Antipatharia L. glaberrima and 168.3 km2 and 279.81 km2 for the Scleralcyonacea C. verticillata and I. elongata (Table 4). The extension of the area in which the hotspots of all six species are present covers an area of 4.05 km2 (CI 6) (CI 1 = 299.30 km2, CI 2 = 114,79 km2, CI 3 = 70.20 km2, CI 4 = 41.86 km2, CI 5 = 24 km2, CI 6 = 4.05 km2).
Figure 5 Co-occurrence Index of CWCs. The different colours express the number of species co-occurring in the same area (e.g., pink indicates 0 species, red indicates six species co-occur in the same area).
All species exhibited a positive correlation, according to Spearman’s correlation matrix (Figure 6). From the graph the highest degree of correlation was given by the pairs M. oculata - D. pertusum and D. cornigera - C. verticillata, while the lowest correlation was expressed between I. elongata - L. glaberrima.
Figure 6 Spearman correlation matrix of all CWCs species. All species present a positive correlation.
The present study modelled the distribution of six CWC species on 10 potential drivers using a MaxEnt approach in the Northern part of the Strait of Sicily. For the first time, several CWC species were studied together through high-resolution sampling, and their probability of occurrence was used to produce co-occurrence maps, which will be a powerful tool in supporting the implementation of conservation actions in the area.
Habitat suitability models generated showed an AUC and TSS value of more than 0.8 for all species except for I. elongata, for which the TSS value is 0.59 (useful), suggesting that the MaxEnt models predicting successfully the six CWC species’ distribution in the study area.
Among all the predictors, slope, depth, rugosity temperature and north bottom current were the main variables influencing the likelihood of distribution and habitat suitability of CWC species.
As reported by several other authors, slope, depth and rugosity are among the factors that most influence the habitat preferences of CWCs (García-Alegre et al., 2014; Lauria et al., 2021; Abad-Uribarren et al., 2022).
The species’ habitat preferences are strongly influenced by slope, showing that the probability of occurrence appears to be positively correlated with this variable. This factor influences current flow, and consequently influences food availability for benthic species (Mohn and Beckmann, 2002; Wilson et al., 2007; García-Alegre et al., 2014). Considering that CWCs are suspension feeders, the previously mentioned conditions (exposure to currents and increased food supply) are of fundamental importance in modelling their distribution (Portilho-Ramos et al., 2022). In addition, the slope may also be a limiting factor for fisheries (Grehan et al., 2005; García-Alegre et al., 2014) and indirectly protects the species from fishing activities providing refuge for benthic fauna (Huvenne et al., 2011; Pierdomenico et al., 2016; Pearman et al., 2020).
Slope can also influence the seabed’s exposure to currents (Bargain et al., 2018), representing one of the key environmental variables influencing deep-sea ecosystems (Hebbeln et al., 2016; Rebesco and Taviani, 2019). Hydrodynamics was among the factors affecting the distribution of some CWCs under consideration. The currents, in addition to increasing the food supply, promote the spread of coral propagules and, as a result, the species’ success while also preventing burial (White et al., 2005; Henry et al., 2014; Bargain et al., 2018; Chimienti et al., 2019; Rebesco and Taviani, 2019; Lim et al., 2020; Pearman et al., 2020). In agreement with this, many observations of CWCs have been made in association with steep walls (Davies et al., 2014; Robert et al., 2015; Pearman et al., 2020).
Depth also significantly influenced the habitat preferences of CWC species. It showed a high probability of occurrence associated with different depth ranges depending on the species considered. The ranges recorded support previous findings for these CWC species (Hebbeln et al., 2009; Mastrototaro et al., 2010; Lo Iacono et al., 2012; Gori et al., 2013; García-Alegre et al., 2014; Carbonara et al., 2022) confirming the extrapolation potential of our study and the selected model applied.
Rugosity of the seafloor is another main factor driving the distribution of CWCs, confirming that corals particularly promote substrate complexity (Bargain et al., 2018), except for some species that prefer muddy/sandy bottoms. This is the case of the bamboo coral I. elongata which presented the lowest rugosity value. This species is the only one among those analysed that is suggested to prefer muddy sediment (Mastrototaro et al., 2017; Pierdomenico et al., 2018; Chimienti et al., 2019; Carbonara et al., 2020), which was also confirmed in this present study.
Other relevant variables are temperature and fishing effort. Regarding the first one, it is widely acknowledged that the distribution of CWCs is restricted by temperature instead of depth, and they are most typically found at temperatures ranging from 4°C to 12°C (Roberts, 2009; Maier et al., 2012). They exist in the Mediterranean Sea at temperatures ranging from 12.5°C to almost 14°C, with occasional higher temperatures (Freiwald et al., 2009; Castellan et al., 2019).
Bottom trawling, negatively impact CWC communities, directly removing living benthic fauna and indirectly modifying the substrate that has a crucial role in the settlement of larvae and the possibility of natural restoration of the habitat (Hall–Spencer et al., 2002; Fanelli et al., 2017). In the present paper the models revealed an effect on the distribution of D. pertusum, L. glaberrima and I. elongata in relation with the trawling effort. The first two species are typically found on hard bottom so indirectly impacted by trawling. Infact, these species can be damaged by the resuspended sediment as demonstrated in laboratory experiments also for other CWCs (Bilan et al., 2023). In the study areas L. glaberrima frequently was observed near trawling areas but the colonies of the observed forest did not show a healthy status (V.P. personal observation). Moreover, CWCs habitats are classified as EFHs as it has a key ecological role for many commercial and non-commercial fish and invertebrate species that use this habitat as nursery, feeding, and refuge areas. This is the case of I. elongata forests that represent important areas form many species of commercial interest as for example Aristeus antennatus and Aristeomorpha foliacea (Cartes et al., 2013; Mastrototaro et al., 2017; Pierdomenico et al., 2018; STECF, 2019; Carbonara et al., 2020). Fishing trawling has a significant direct impact on I. elongata populations, removing or damaging the colonies and affecting their abundance and presence as reported in many areas (Lauria et al., 2017; Mastrototaro et al., 2017; Carbonara et al., 2020).
Analysis of Spearman’s matrix suggests that all the species showed a positive correlation in terms of co-occurrence. Species that prefer more complex substrate types, mainly rocky bottoms, such as M. oculata, D. pertusum, D. cornigera, L. glaberrima, and C. verticillata are more associated and presented a high value of correlation (Spearman correlation: 0.4 - 0.7). On the other hand, the bamboo coral I. elongata, showed low correlation values with other CWC species (Spearman correlation value: 0.2 – 0.4). Regarding the correlation between I. elongata and M. oculata (0.4), this could be due to the presence of many living colonies of M. oculata being able to colonise other types of substrates (e.g., small rocky, tanatocenosis, wrecks) observed during ROV surveys in areas characterised by muddy bottoms. In addition, many colonies of M. oculata and D. cornigera were observed on stretches of thanatocoenosis. As reported by other authors, CWCs can grow and live over dead coral structures (Hebbeln et al., 2016). This study provides accurate predictive models for six CWCs in the Strait of Sicily and gives also, for the first time, the identification of suitable habitat for the co-occurrence of different CWCs. This last analysis allowed the identification of a total area of 4.05 km2 where suitable environmental characteristics for the co-occurring of different CWC species are potentially present. Moreover, the use of high-resolution bathymetry data, like in this study, is demonstrated to be of fundamental importance in habitat suitability modelling because they allow to obtain a more accurate predictive model (Ross et al., 2015). Having more accurate distribution models and also maps with a probability of co-occurrence of these vulnerable habitats provides valuable information from a management perspective and is a useful tool for decision-makers in order to adopt the best conservation and management actions (Kinlan et al., 2020).
The present study enhances our knowledge of the spatial distribution of some important CWC species (M. oculata, D. pertusum, D. cornigera, L. glaberrima, C. verticillata and I. elongata) using a fine spatial scale (5m). Our results support the idea that anthropogenic and environmental variables play a significant role in deciding species distribution. Considering this and that these ecosystems are crucial for many other species (with commercial and non-commercial value), it is critical to implement effective management strategies to ensure the protection and conservation of these populations and their associated biodiversity.
Despite the advancement of technologies (e.g., ROVs, Multi-beam echosounder, high-resolution data), data on the distribution of deep-sea species are often difficult to obtain. Predicting their distribution using suitable habitat models is crucial because mapping vulnerable environments of conservation concern is considered the first step in the environmental protection framework. It is important to emphasise that environmental factors driving species distribution can vary even at small scales, altering the probability of species presence. For this reason, this study highlights the importance of continuing to use high-resolution (5 m) spatial scales to accurately estimate the habitat suitability for benthic communities.
This paper also reports, for the first time, the co-occurrence analysis between CWC species, showing that in some zones within the study area, there is a partially overlapping distribution among some or all the species considered.
The valuable and new information obtained from this study can be useful for fisheries management and used to guide the establishment of new Fisheries Restricted Areas (FRAs) to preserve and increase the area’s potential conservation and natural restoration. In addition, the modelling approach used in this study may be extended to the entire Mediterranean, providing a large-scale view of these species’ distribution.
The datasets presented in this article are not readily available because datasets are part of an ongoing project. Requests to access the datasets should be directed to TR,dGVyZXNhLnJvbWVvQHN6bi5pdA==.
VP: Data curation, Investigation, Software, Validation, Writing – original draft. GM: Data curation, Formal analysis, Investigation, Validation, Writing – review & editing, Software. SC: Investigation, Project administration, Writing – review & editing, Software. ES: Investigation, Writing – review & editing, Software. DP: Investigation, Writing – review & editing, Software. AP: Data curation, Investigation, Software, Writing – review & editing. NS: Project administration, Supervision, Writing – review & editing. TR: Funding acquisition, Project administration, Writing – review & editing. SG: Funding acquisition, Project administration, Writing – review & editing.
The author(s) declare that no financial support was received for the research, authorship, and/or publication of this article.
The authors sincerely thank the AALEA and MainportGeo teams for the logistical help in this project and all our colleagues who participated in the survey.
The authors declare that the research was conducted in the absence of any commercial or financial relationships that could be construed as a potential conflict of interest.
All claims expressed in this article are solely those of the authors and do not necessarily represent those of their affiliated organizations, or those of the publisher, the editors and the reviewers. Any product that may be evaluated in this article, or claim that may be made by its manufacturer, is not guaranteed or endorsed by the publisher.
The Supplementary Material for this article can be found online at: https://www.frontiersin.org/articles/10.3389/fmars.2023.1272066/full#supplementary-material
Abad-Uribarren A., Prado E., Sierra S., Cobo A., Rodríguez-Basalo A., Gómez-Ballesteros M., et al. (2022). Deep learning-assisted high resolution mapping of vulnerable habitats within the Capbreton Canyon System, Bay of Biscay. Estuar. Coast. Shelf Sci. 275, 107957. doi: 10.1016/j.ecss.2022.107957
Agostini V. N., Bakun A. (2002). `Ocean triads' in the Mediterranean Sea: physical mechanisms potentially structuring reproductive habitat suitability (with example application to European anchovy, Engraulis encrasicolus ): "Ocean triads" in the Mediterranean Sea. Fish. Oceanogr. 11, 129–142. doi: 10.1046/j.1365-2419.2002.00201.x
Anderson O. F., Guinotte J. M., Rowden A. A., Clark M. R., Mormede S., Davies A. J., et al. (2016). Field validation of habitat suitability models for vulnerable marine ecosystems in the South Pacific Ocean: Implications for the use of broad-scale models in fisheries management. Ocean Coast. Manage 120, 110–126. doi: 10.1016/j.ocecoaman.2015.11.025
Angiolillo M., La Mesa G., Giusti M., Salvati E., Di Lorenzo B., Rossi L., et al. (2021). New records of scleractinian cold-water coral (CWC) assemblages in the southern Tyrrhenian Sea (western Mediterranean Sea): Human impacts and conservation prospects. Prog. Oceanography 197, 102656. doi: 10.1016/j.pocean.2021.102656
Ashford O. S., Kenny A. J., Barrio Froján C. R. S., Downie A.-L., Horton T., Rogers A. D. (2019). On the influence of vulnerable marine ecosystem habitats on peracarid crustacean assemblages in the northwest atlantic fisheries organisation regulatory area. Front. Mar. Sci. 6. doi: 10.3389/fmars.2019.00401
Astraldi M., Gasparini G. P., Sparnocchia S., Moretti M., Sansone E. (1996). The characteristics of the water masses and the water transport in the Sicily Strait at long time scales. Bull. l’Institut oceanographique Monaco n special 17 CIESM Sci. Ser. n 2, 95–115.
Astraldi M., Gasparini G. P., Vetrano A., Vigudelli S. (2002). Hydrographic characteristics and interannual variability of water masses in the central Mediterranean: a sensitivity test for long-term changes in the Mediterranean Sea. Deep Sea Res. Part I: Oceanog. Res. Papers 49, 661–680. doi: 10.1016/S0967-0637(01)00059-0
Barbosa R. V., Davies A. J., Sumida P. Y. G. (2020). Habitat suitability and environmental niche comparison of cold-water coral species along the Brazilian continental margin. Deep Sea Res. Part I: Oceanographic Res. Papers 155, 103147. doi: 10.1016/j.dsr.2019.103147
Bargain A., Foglini F., Pairaud I., Bonaldo D., Carniel S., Angeletti L., et al. (2018). Predictive habitat modeling in two Mediterranean canyons including hydrodynamic variables. Prog. Oceanogr. 169, 151–168. doi: 10.1016/j.pocean.2018.02.015
Bargain A., Marchese F., Savini A., Taviani M., Fabri M.-C. (2017). Santa maria di leuca province (Mediterranean sea): identification of suitable mounds for cold-water coral settlement using geomorphometric proxies and maxent methods. Front. Mar. Sci. 4. doi: 10.3389/fmars.2017.00338
Bilan M., Gori A., Grinyó J., Biel-Cabanelas M., Puigcerver-Segarra X., Santín A., et al. (2023). Vulnerability of six cold-water corals to sediment resuspension from bottom trawling fishing. Mar. pollut. Bull. 196, 115423. doi: 10.1016/j.marpolbul.2023.115423
Buhl-Mortensen L., Olafsdottir S. H., Buhl-Mortensen P., Burgos J. M., Ragnarsson S. A. (2015). Distribution of nine cold-water coral species (Scleractinia and Gorgonacea) in the cold temperate North Atlantic: effects of bathymetry and hydrography. Hydrobiologia 759, 39–61. doi: 10.1007/s10750-014-2116-x
Carbonara P., Zupa W., Follesa M. C., Cau A., Capezzuto F., Chimienti G., et al. (2020). Exploring a deep-sea vulnerable marine ecosystem: Isidella elongata (Esper 1788) species assemblages in the Western and Central Mediterranean. Deep Sea Res. Part I: Oceanographic Res. Papers 166, 103406. doi: 10.1016/j.dsr.2020.103406
Carbonara P., Zupa W., Follesa M. C., Cau A., Donnaloia M., Alfonso S., et al. (2022). Spatio-temporal distribution of Isidella elongata, a vulnerable marine ecosystem indicator species, in the southern Adriatic Sea. Hydrobiologia 849 (21), 4837–4855. doi: 10.1007/s10750-022-05022-4
Cartes J. E., LoIacono C., Mamouridis V., López-Pérez C., Rodríguez P. (2013). Geomorphological, trophic and human influences on the bamboo coral Isidella elongata assemblages in the deep Mediterranean: To what extent does Isidella form habitat for fish and invertebrates? Deep Sea Res. Part I: Oceanographic Res. Papers 76, 52–65. doi: 10.1016/j.dsr.2013.01.006
Castellan G., Angeletti L., Taviani M., Montagna P. (2019). The yellow coral dendrophyllia cornigera in a warming ocean. Front. Mar. Sci. 6. doi: 10.3389/fmars.2019.00692
Chimienti G., Bo M., Taviani M., Mastrototaro F. (2019). “19 occurrence and biogeography of mediterranean cold-water corals,” in Mediterranean cold-water corals: Past, present and future (Cham: Springer), 213–243.
Chimienti G., Mastrototaro F., D’Onghia G. (2020). “Mesophotic and deep-sea vulnerable coral habitats of the mediterranean sea: overview and conservation perspectives,” in Advances in the Studies of the Benthic Zone, vol. 20 . Ed. Soto L. A. doi: 10.5772/intechopen.90024
Chu J. W. F., Nephin J., Georgian S., Knudby A., Rooper C., Gale K. S. P. (2019). Modelling the environmental niche space and distributions of cold-water corals and sponges in the Canadian northeast Pacific Ocean. Deep Sea Res. Part I: Oceanographic Res. Papers 151, 103063. doi: 10.1016/j.dsr.2019.06.009
Colloca F., Bartolino V., Lasinio G., Maiorano L., Sartor P., Ardizzone G. (2009). Identifying fish nurseries using density and persistence measures. Mar. Ecol. Prog. Ser. 381, 287–296. doi: 10.3354/meps07942
Davies J. S., Howell K. L., Stewart H. A., Guinan J., Golding N. (2014). Defining biological assemblages (biotopes) of conservation interest in the submarine canyons of the South West Approaches (offshore United Kingdom) for use in marine habitat mapping. Deep Sea Res. Part II: Topical Stud. Oceanography 104, 208–229. doi: 10.1016/j.dsr2.2014.02.001
Di Lorenzo M., Sinerchia M., Colloca F. (2018). The North sector of the Strait of Sicily: a priority area for conservation in the Mediterranean Sea. Hydrobiologia 821, 235–253. doi: 10.1007/s10750-017-3389-7
D’Onghia G., Calculli C., Capezzuto F., Carlucci R., Carluccio A., Grehan A., et al. (2017). Anthropogenic impact in the Santa Maria di Leuca cold-water coral province (Mediterranean Sea): Observations and conservation straits. Deep Sea Res. Part II: Topical Stud. Oceanography 145, 87–101. doi: 10.1016/j.dsr2.2016.02.012
D’Onghia G., Sion L., Capezzuto F. (2019). Cold-water coral habitats benefit adjacent fisheries along the Apulian margin (central Mediterranean). Fish. Res. 213, 172–179. doi: 10.1016/j.fishres.2019.01.021
Elith J., Phillips S. J., Hastie T., Dudík M., Chee Y. E., Yates C. J. (2011). A statistical explanation of MaxEnt for ecologists: Statistical explanation of MaxEnt. Divers. Distrib. 17, 43–57. doi: 10.1111/j.1472-4642.2010.00725.x
Etnoyer P. J., Wagner D., Fowle H. A., Poti M., Kinlan B., Georgian S. E., et al. (2018). Models of habitat suitability, size, and age-class structure for the deep-sea black coral Leiopathes glaberrima in the Gulf of Mexico. Deep Sea Res. Part II: Topical Stud. Oceanography 150, 218–228. doi: 10.1016/j.dsr2.2017.10.008
Fabri M.-C., Bargain A., Pairaud I., Pedel L., Taupier-Letage I. (2017). Cold-water coral ecosystems in Cassidaigne Canyon: An assessment of their environmental living conditions. Deep Sea Res. Part II: Topical Stud. Oceanography 137, 436–453. doi: 10.1016/j.dsr2.2016.06.006
Fanelli E., Delbono I., Ivaldi R., Pratellesi M., Cocito S., Peirano A. (2017). Cold-water coral Madrepora oculata in the eastern Ligurian Sea (NW Mediterranean): Historical and recent findings. Aquat. Conserv: Mar. Freshw. Ecosyst. 27, 965–975. doi: 10.1002/aqc.2751
FAO (Food and Agriculture Organization) (2009). International guidelines for the management of deep-sea fisheries in the high seas. FAO Rome p, 73.
Fiorentino F., Garofalo G., Santi A. D., Bono G., Giusto G. B., Norrito G. (2003). “Spatio-temporal distribution of recruits (0 group) of Merluccius merluccius and Phycis blennoides (Pisces, Gadiformes) in the Strait of Sicily (Central Mediterranean),” in Migrations and Dispersal of Marine Organisms: Proceedings of the 37 the European Marine Biology Symposium held in Reykjavík, Iceland, 5–9 August 2002 (Netherlands: Springer), 223–236.
Freiwald A., Beuck L., Rüggeberg A., Taviani M., Hebbeln D., R/V Meteor Cruise M70-1 Participants. (2009). The white coral community in the central mediterranean sea revealed by ROV surveys. Oceanography 22 (1), 58–74.
García-Alegre A., Sánchez F., Gómez-Ballesteros M., Hinz H., Serrano A., Parra S. (2014). Modelling and mapping the local distribution of representative species on the Le Danois Bank, El Cachucho Marine Protected Area (Cantabrian Sea). Deep Sea Res. Part II: Topical Stud. Oceanography 106, 151–164. doi: 10.1016/j.dsr2.2013.12.012
Getis A., Ord J. K. (1992). The analysis of spatial association by use of distance statistics. Geogr. Anal. 24 (3), 189–206. doi: 10.1111/j.1538-4632.1992.tb00261.x
Gori A., Orejas C., Madurell T., Bramanti L., Martins M., Quintanilla E., et al. (2013). Bathymetrical distribution and size structure of cold-water coral populations in the Cap de Creus and Lacaze-Duthiers canyons (northwestern Mediterranean). Biogeosci. 10 (3), 2049–2060. doi: 10.5194/bg-10-2049-2013
Grehan A. J., Unnithan V., Roy K. O., Opderbecke J. (2005). Fishing impacts on Irish deepwater coral reefs: making a case for coral conservation. In American Fisheries Society Symposium. Am. Fish. Soc 41, 819. doi: 10.47886/9781888569605.ch144
Hall–Spencer J., Allain V., Fosså J. H. (2002). Trawling damage to Northeast Atlantic ancient coral reefs. Proc. R. Soc Lond. B 269, 507–511. doi: 10.1098/rspb.2001.1910
Hebbeln D., Van Rooij D., Wienberg C. (2016). Good neighbours shaped by vigorous currents: Cold-water coral mounds and contourites in the North Atlantic. Mar. Geol. 378, 171–185. doi: 10.1016/j.margeo.2016.01.014
Hebbeln D., Wienberg C., Beuck L., Freiwald A. P. (2009). Wintersteller and cruise participants Report and preliminary results of RV POSEIDON Cruise POS 385 "Cold-Water Corals of the Alboran Sea (western Mediterranean Sea)", Faro - Toulon, May 29 -June;16, 2009 (Bremen: Berichte, Fachbereich Geowissenschaften, Universität Bremen), 79. No. 273.
Henry L.-A., Frank N., Hebbeln D., Wienberg C., Robinson L., de Flierdt T., et al. (2014). Global ocean conveyor lowers extinction risk in the deep sea. Deep Sea Res. Part I: Oceanographic Res. Papers 88, 8–16. doi: 10.1016/j.dsr.2014.03.004
Huvenne V. A. I., Bett B. J., Masson D. G., Le Bas T. P., Wheeler A. J. (2016). Effectiveness of a deep-sea cold-water coral Marine Protected Area, following eight years of fisheries closure. Biol. Conserv. 200, 60–69. doi: 10.1016/j.biocon.2016.05.030
Huvenne V. A. I., Tyler P. A., Masson D. G., Fisher E. H., Hauton C., Hühnerbach V., et al. (2011). A picture on the wall: innovative mapping reveals cold-water coral refuge in submarine canyon. PloS One 6, e28755. doi: 10.1371/journal.pone.0028755
Kassambara A., Kassambara M. A. (2019). Visualization of a correlation matrix using ggplot2. “ggcorrplot.”
Kinlan B. P., Poti M., Drohan A. F., Packer D. B., Dorfman D. S., Nizinski M. S. (2020). Predictive modeling of suitable habitat for deep-sea corals offshore the Northeast United States. Deep Sea Res. Part I: Oceanographic Res. Papers 158, 103229. doi: 10.1016/j.dsr.2020.103229
Lauria V., Garofalo G., Fiorentino F., Massi D., Milisenda G., Piraino S., et al. (2017). Species distribution models of two critically endangered deep-sea octocorals reveal fishing impacts on vulnerable marine ecosystems in central Mediterranean Sea. Sci. Rep. 7, 8049. doi: 10.1038/s41598-017-08386-z
Lauria V., Massi D., Fiorentino F., Milisenda G., Cillari T. (2021). Habitat suitability mapping of the black coral Leiopathes glaberrima to support conservation of vulnerable marine ecosystems. Sci. Rep. 11, 15661. doi: 10.1038/s41598-021-95256-4
Li S., Wang Z., Zhu Z., Tao Y., Xiang J. (2023). Predicting the potential suitable distribution area of Emeia pseudosauteri in Zhejiang Province based on the MaxEnt model. Sci. Rep. 13, 1806. doi: 10.1038/s41598-023-29009-w
Lim A., Wheeler A. J., Conti L. (2020). Cold-water coral habitat mapping: trends and developments in acquisition and processing methods. Geosciences 11, 9. doi: 10.3390/geosciences11010009
Liu C., Newell G., White M. (2016). On the selection of thresholds for predicting species occurrence with presence-only data. Ecol. Evol. 6, 337–348. doi: 10.1002/ece3.1878
Lo Iacono C., Orejas C., Gori A., Gili J. M., Requena S., Puig P., et al. (2012). Habitats of the Cap de Creus Continental Shelf and Cap de Creus Canyon, Northwestern Mediterranean, in: Seafloor Geomorphology as Benthic Habitat. Elsevier 457–469. doi: 10.1016/B978-0-12-385140-6.00032-3
Lo Iacono C., Robert K., Gonzalez-Villanueva R., Gori A., Gili J.-M., Orejas C. (2018). Predicting cold-water coral distribution in the Cap de Creus Canyon (NW Mediterranean): Implications for marine conservation planning. Prog. Oceanogr. 169, 169–180. doi: 10.1016/j.pocean.2018.02.012
Maier C., Watremez P., Taviani M., Weinbauer M. G., Gattuso J. P. (2012). Calcification rates and the effect of ocean acidification on Mediterranean cold-water corals. Proc. R. Soc Ser. B Biol. Sci. 279 (1734), 1716–1723. doi: 10.1098/rspb.2011.1763
Manzella G. M. R., Hopkins T. S., Minnett P. J., Nacini E. (1990). Atlantic water in the strait of Sicily. J. Geophys. Res. 95, 1569. doi: 10.1029/JC095iC02p01569
Mastrototaro F., Chimienti G., Acosta J., Blanco J., Garcia S., Rivera J., et al. (2017). Isidella elongata (Cnidaria: Alcyonacea) facies in the western Mediterranean Sea: visual surveys and descriptions of its ecological role. Eur. Zool. J. 84, 209–225. doi: 10.1080/24750263.2017.1315745
Mastrototaro F., D’Onghia G., Corriero G., Matarrese A., Maiorano P., Panetta P., et al. (2010). Biodiversity of the white coral bank off Cape Santa Maria di Leuca (Mediterranean Sea): An update. Deep Sea Res. Part II: Topical Stud. Oceanography 57, 412–430. doi: 10.1016/j.dsr2.2009.08.021
Milisenda G., Garofalo G., Fiorentino F., Colloca F., Maynou F., Ligas A., et al. (2021). Identifying persistent hot spot areas of undersized fish and crustaceans in southern european waters: implication for fishery management under the discard ban regulation. Front. Mar. Sci. 8. doi: 10.3389/fmars.2021.610241
Mohn C., Beckmann A. (2002). Numerical studies on flow amplification at an isolated shelfbreak bank, with application to Porcupine Bank. Cont. Shelf Res. 22, 1325–1338. doi: 10.1016/S0278-4343(02)00004-3
Ord J. K., Getis A. (1995). Local spatial autocorrelation statistics: distributional issues and an application. Geogr. Anal. 27 (4), 286–306. doi: 10.1111/j.1538-4632.1995.tb00912.x
Otero M. M., Numa C., Bo M., Orejas C., Garrabou J., Cerrano C., et al. (2017). Overview of the conservation status of Mediterranean anthozoans. (Malaga, Spain: IUCN), x + 73. doi: 10.2305/IUCN.CH.2017.RA.2.en
Otero M. D. M., Marin P. (2019). “46 conservation of cold-water corals in the mediterranean: current status and future prospects for improvement,” in Mediterranean cold-water corals: past, present and future (Springer) 9, 535–545. doi: 10.1007/978-3-319-91608-165978_46
Pearman T. R. R., Robert K., Callaway A., Hall R., Lo Iacono C., Huvenne V. A. I. (2020). Improving the predictive capability of benthic species distribution models by incorporating oceanographic data – Towards holistic ecological modelling of a submarine canyon. Prog. Oceanogr. 184, 102338. doi: 10.1016/j.pocean.2020.102338
Pebesma E. J. (2004). Multivariable geostatistics in S: the gstat package. Comput. Geosci. 30, 683–691. doi: 10.1016/j.cageo.2004.03.012
Phillips S. J., Anderson R. P., Dudík M., Schapire R. E., Blair M. E. (2017). Opening the black box: an open-source release of maxent. Ecography 40 (7), 887–893. doi: 10.1111/ecog.03049
Phillips S. J., Anderson R. P., Schapire R. E. (2006). Maximum entropy modeling of species geographic distributions. Ecol. Modell. 190, 231–259. doi: 10.1016/j.ecolmodel.2005.03.026
Phillips S. J., Dudík M. (2008). Modeling of species distributions with Maxent: new extensions and a comprehensive evaluation. Ecography 31, 161–175. doi: 10.1111/j.0906-7590.2008.5203.x
Phillips S. J. (2017). A brief tutorial on maxent. Available at: http://biodiversityinformatics.amnh.org/open_source/maxent/ (Accessed on October 10, 2023).
Pierdomenico M., Martorelli E., Dominguez-Carrió C., Gili J. M., Chiocci F. L. (2016). Seafloor characterisation and benthic megafaunal distribution of an active submarine canyon and surrounding sectors: The case of Gioia Canyon (Southern Tyrrhenian Sea). J. Mar. Syst. 157, 101–117. doi: 10.1016/j.jmarsys.2016.01.005
Pierdomenico M., Russo T., Ambroso S., Gori A., Martorelli E., D’Andrea L., et al. (2018). Effects of trawling activity on the bamboo-coral Isidella elongata and the sea pen Funiculina quadrangularis along the Gioia Canyon (Western Mediterranean, southern Tyrrhenian Sea). Prog. Oceanogr. 169, 214–226. doi: 10.1016/j.pocean.2018.02.019
Portilho-Ramos R., da C., Titschack J., Wienberg C., Siccha Rojas M. G., Yokoyama Y., et al. (2022). Major environmental drivers determining life and death of cold-water corals through time. PloS Biol. 20, e3001628. doi: 10.1371/journal.pbio.3001628
Qin C., Zhu A.-X., Pei T., Li B., Zhou C., Yang L. (2007). An adaptive approach to selecting a flow-partition exponent for a multiple-flow-direction algorithm. Int. J. Geogr. Inf. Sci. 21, 443–458. doi: 10.1080/13658810601073240
Rebesco M., Taviani M. (2019). “4 a turbulent story: mediterranean contourites and cold-water corals,” in Mediterranean cold-water corals: past, present and future: understanding the deep-sea realms of coral, vol. 9. (Cham: Springer), 35–46. doi: 10.1007/978-3-319-91608-165978_46
Robert K., Jones D. O. B., Tyler P. A., Van Rooij D., Huvenne V. A. I. (2015). Finding the hotspots within a biodiversity hotspot: fine-scale biological predictions within a submarine canyon using high-resolution acoustic mapping techniques. Mar. Ecol. 36, 1256–1276. doi: 10.1111/maec.12228
Roberts J. M. (2009). Cold-water corals: the biology and geology of deep-sea coral habitats (Cambridge, UK: Cambridge University Press).
Ross L. K., Ross R. E., Stewart H. A., Howell K. L. (2015). The influence of data resolution on predicted distribution and estimates of extent of current protection of three ‘listed’deep-sea habitats. PloS One 10 (10), e0140061. doi: 10.1371/journal.pone.0140061
Russo T., D’andrea L., Parisi A., Martinelli M., Belardinelli A., Boccoli F., et al. (2016). Assessing the fishing footprint using data integrated from different tracking devices: Issues and opportunities. Ecol. Indic. 69, 818–827. doi: 10.1016/j.ecolind.2016.04.043
Savini A., Vertino A., Marchese F., Beuck L., Freiwald A. (2014). Mapping Cold-Water Coral Habitats at Different Scales within the Northern Ionian Sea (Central Mediterranean): An Assessment of Coral Coverage and Associated Vulnerability. PloS One 9, e87108. doi: 10.1371/journal.pone.0087108
STECF (2019) Annual Economic Report on the EU Fishing Fleet (STECF 19-06). Available at: http://publications.jrc.ec.europa.eu/repository/handle/JRC117567.
Stephenson F., Rowden A. A., Anderson O. F., Pitcher C. R., Pinkerton M. H., Petersen G., et al. (2021). Presence-only habitat suitability models for vulnerable marine ecosystem indicator taxa in the South Pacific have reached their predictive limit. ICES J. Mar. Sci. 78, 2830–2843. doi: 10.1093/icesjms/fsab162
Sundahl H., Buhl-Mortensen P., Buhl-Mortensen L. (2020). Distribution and Suitable Habitat of the Cold-Water Corals Lophelia pertusa, Paragorgia arborea, and Primnoa resedaeformis on the Norwegian Continental Shelf. Front. Mar. Sci. 7. doi: 10.3389/fmars.2020.00213
Taviani M., Angeletti L., Antolini B., Ceregato A., Froglia C., Lopez Correa M., et al. (2011). Geo-biology of mediterranean deep-water coral ecosystems. Mar. Res. CNR 6, 705–719.
Thiem Ø., Ravagnan E., Fosså J. H., Berntsen J. (2006). Food supply mechanisms for cold-water corals along a continental shelf edge. J. Mar. Syst. 60, 207–219. doi: 10.1016/j.jmarsys.2005.12.004
van den Beld I., Rengstorf A., Grehan A. (2007). “Development of standard operating procedures for video analysis and classification, GIS integration, video visualization and image data archiving,” Final Report INF-08-09-GRE.. (Galway, Earth and Ocean Sciences, NUI), 1–73.
White M., Mohn C., de Stigter H., Mottram G. (2005). “Deep-water coral development as a function of hydrodynamics and surface productivity around the submarine banks of the Rockall Trough, NE Atlantic,” in Cold-water corals and ecosystems. Eds. Freiwald A., Roberts J. M. (Berlin, Heidelberg: Springer), 503–514. doi: 10.1007/3-540-27673-4_25
Wilson M. F. J., O'Connell B., Brown C., Guinan J. C., Grehan A. J. (2007). Multiscale terrain analysis of multi-beam bathymetry data for habitat mapping on the continental slope. Mar. Geod. 30, 3–35. doi: 10.1080/01490410701295962
Keywords: CWCs, Maxent, SDMs, habitat suitability, deep sea, co-occurrence
Citation: Palummo V, Milisenda G, Canese S, Salvati E, Pica D, Passarelli A, Spanò N, Romeo T and Greco S (2023) Effect of environmental and anthropogenic factors on the distribution and co-occurrence of cold-water corals. Front. Mar. Sci. 10:1272066. doi: 10.3389/fmars.2023.1272066
Received: 03 August 2023; Accepted: 04 December 2023;
Published: 20 December 2023.
Edited by:
Rhian G. Waller, University of Gothenburg, SwedenReviewed by:
Lene Buhl-Mortensen, Norwegian Institute of Marine Research (IMR), NorwayCopyright © 2023 Palummo, Milisenda, Canese, Salvati, Pica, Passarelli, Spanò, Romeo and Greco. This is an open-access article distributed under the terms of the Creative Commons Attribution License (CC BY). The use, distribution or reproduction in other forums is permitted, provided the original author(s) and the copyright owner(s) are credited and that the original publication in this journal is cited, in accordance with accepted academic practice. No use, distribution or reproduction is permitted which does not comply with these terms.
*Correspondence: Valeria Palummo, dmFsZXJpYS5wYWx1bW1vQHN6bi5pdA==; Giacomo Milisenda, Z2lhY29tby5taWxpc2VuZGFAc3puLml0
Disclaimer: All claims expressed in this article are solely those of the authors and do not necessarily represent those of their affiliated organizations, or those of the publisher, the editors and the reviewers. Any product that may be evaluated in this article or claim that may be made by its manufacturer is not guaranteed or endorsed by the publisher.
Research integrity at Frontiers
Learn more about the work of our research integrity team to safeguard the quality of each article we publish.