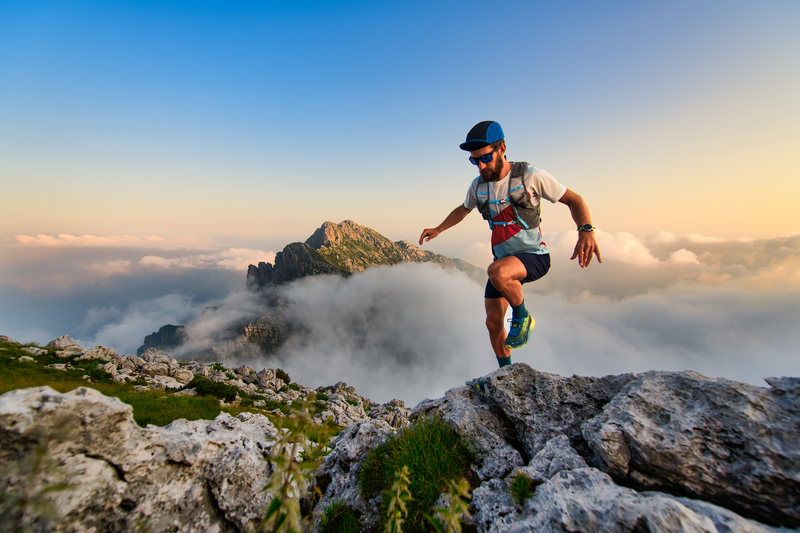
95% of researchers rate our articles as excellent or good
Learn more about the work of our research integrity team to safeguard the quality of each article we publish.
Find out more
HYPOTHESIS AND THEORY article
Front. Mar. Sci. , 18 October 2023
Sec. Ocean Solutions
Volume 10 - 2023 | https://doi.org/10.3389/fmars.2023.1269643
This article is part of the Research Topic Opportunities and Challenges of EU ETS to the Global Marine Industry View all 7 articles
In 2024, the EU intends to include the global shipping industry in the European Union Emission Trading Scheme (EU ETS). Shipping companies will have to pay for the carbon emissions of ships over 5,000 GT on routes between EU and non-EU ports. This paper selects typical shipping companies in the world. Based on the principle of fairness, historical method, baseline method and mixed method are adopted to explore their carbon emission quota allocation. The ZSG-DEA efficiency model is used to evaluate the distribution results and verify the optimal efficiency. The research results show that the mixed method has a high efficiency of allocation. The method predicts that the carbon quota of typical shipping companies in the world will reach the Pareto optimal allocation in 2024 and Maersk has the highest carbon emission quota among the eight typical shipping companies, reaching 32,431,800 tons, followed by MSC and EMC, reaching 8,542,400 tons and 6,809,500 tons, respectively. Based on the results, we can obtain a reasonable allocation of carbon allowances in the EU carbon market according to the proportion of business of shipping companies involved in EU routes. The research is still applicable to the allocation of carbon emmissions in future years. Therefore, this paper provides suggestions for the orderly allocation of carbon quota and carbon trading in the global shipping market.
It is a great significance to addressing climate change and achieving the Sustainable Development Goals in the global shipping industry. The decarbonization of shipping has become the focus of global attention. The development of carbon quota in the shipping industry and many routes will be affected (Figure 1), However, with the increasing pressure of global emission reduction, the development of carbon quota system will become an important means of emission reduction in the shipping industry.
Carbon emission quota allocation involves environmental protection, economic fairness, energy security and other aspects of consideration. Many studies on carbon emission quotas have focused on fairness or efficiency (Park et al., 2011; Atte et al., 2022; Bintang et al., 2023). The principle of fairness means that the interests and rights of all parties should be taken into account in the allocation of carbon emission quotas to ensure the fairness of carbon emission reduction (Pozo et al., 2020). Studies involving the principle of fairness mainly involve the principle of sovereignty, the principle of historical responsibility, the principle of economic activity and other allocation principles when allocating carbon emission quota. The sovereignty principle, also known as the grandfather principle. It advocates maintaining the status quo, whereby existing emitters are entitled to emit as much as they have historically emitted. The grandfather law was proposed on global climate governance, which takes historical emissions as the basis for free allocation of carbon emission quota (Rose et al., 1998). In a carbon market with emission caps and no trading, the grandfathering method is the best solution. While in a carbon market with cap-and-trading, the grandfathering method based on historical emissions and production is the second-best solution (Böhringer and Lange, et al., 2005). A study also argued that regional quota allocation should be based on the proportion of historical emissions of the emitting subjects (Schmidt and Heitzig, 2014). The principle of historical emission responsibility, also known as the polluter pays principle, means that the obligation to reduce carbon emissions should be borne more by the countries or regions that have emitted more historically. Rearch found that the historical cumulative emissions should be used as an allocation index. It required countries to undertake corresponding emission reduction obligations according to their historical responsibilities. Because the historical carbon emissions can bring high quality and welfare to these countries and it was difficult for developing countries to achieve (Wei et al., 2014). The application of the principle of economic activity is based on the GDP index. Rearch showed that carbon emission correction model can be constructed based on the per capita GDP and per capita cumulative carbon emission of each country respectively (Baer, 2013) and quota allocation should match the proportion of participants’ GDP shares (Zhou and Wang, 2016).
The efficiency principle means that in the allocation of carbon emission quotas, the maximization of economic efficiency and resource utilization efficiency should be pursued (Steenberghe, 2004; Zhou et al. , 2014). This principle advocates reducing carbon emissions through the use of market mechanisms and economic incentives. At the same time, this can also provide a flexible emission reduction scheme for companies or countries with high carbon emissions. Rearch pointed out that higher carbon efficiency means more economic output at the same level of carbon emissions (Cucchiella et al., 2018). Compared the relative performance of the initial allocation mechanism among companies, it was impossible to achieve Pareto optimality by using the historical output of companies. Moreover, external factors should be considered in the allocation to achieve social optimization (Mackenzie et al., 2008). Many models and mechanisms can be used to analyze this problem. The sub-parameter model can be established to study emission reduction in environmental cooperation. Finally it proposed that the reduction of marginal emission reduction cost would help more countries participate in emission reduction cooperation and improve emission reduction efficiency (Karp and Simon, 2013). Some research uesd the dual-mode production plans and concluded the same emission reduction standards would affect the distribution efficiency for companies producing similar products (Hong et al., 2016). Some study alos uesd a two-step optimization mechanism to solve the problem of emission quota allocation and achieve balanced efficiency and found that the allocation method considering regional heterogeneity was more logical (Shojaei and Mokhtar, 2021).
However, the pursuit of fairness while ignoring efficiency, or focusing on efficiency while ignoring fairness, may lead to inefficient or unfeasible carbon reduction measures. It also leads to the unfairness of increasing carbon emission quotas. Market mechanisms may also be subject to abuse and unfair competition. Therefore, the principle of fairness and the principle of efficiency are mutually reinforcing. Consequently, this study makes an innovative and systematic analysis of distribution in the shipping industry. Different from the traditional analysis method, it combines the historical method and the baseline method based on the principle of fairness and efficiency. Then, the ZSG-DEA efficiency model is used to evaluate the efficiency assessment of the shipping industry. The result will help us to understand the emission reduction responsibility of shipping industry and provide a new idea for the shipping industry carbon quota allocation.
On July 2021, the European Commission formally published a package of proposals (Fit for 55). It aims to achieve the EU’s goal of reducing net carbon emissions by 55% by 2030 compared with 1990. The meeting determined that the shipping industry in or between EU ports will be included in the EU carbon market from 2024. This means that shipping companies involved in EU routes will pay for the compliance costs of their ships’ carbon emissions. The revision of the EU Carbon Emission System Directive, the revision of the EU Maritime Fuel Regulation and the revision of the Energy tax Directive will have a significant impact on the shipping industry. Since the IMO launched the preliminary strategy of greenhouse gas emission reduction in the shipping industry, emission reduction and low-carbon have become the inevitable trend of the shipping industry. In 2018, the 72nd session of the Maritime Environment Protection Committee (MEPC) adopted the Initia IMO Strategy on Reduction of GHG Emissions from Ships. This is the first time that IMO has systematically outlined its master plan for the international shipping industry to promote greenhouse gas emissions reduction. In February 2023, the Committee of Permanent Missions of the Council of the European Union and the Committee on the Environment of the European Parliament separately approved the revised ETS final compromise text. This text contains the proposal to include the maritime industry in the EU ETS. From 2024, shipowners or other organisations that assume responsibility for their ships (such as management companies or bareboat charterers will have to pay for the greenhouse gas emissions their ships generate when travelling in or between EU ports. In order to comply with this legislation, commercial operators must purchase and submit a certain number of emission allowances each year.
Based on the turnover of global shipping companies in the past five years, the following companies are selected as research objects in this paper: Maersk, Mediterranean Shipping companies. A (MSC), CMA-CGM (CMA), China Ocean Shipping Company(COSCO), Hapel-Lloyd Container Line (HPL), Evergreen(EMC), Yangming Shipping (YML) and Wanhai Lines (WHL). Limited to the availability of data, the study samples the historical data of these eight companies from 2018 to 2022, and uses the historical data to estimate the relevant data for 2024. All figures are from the company’s annual report.
The historical emission law is based on the principle of cap-and-trade. The government determines the total carbon emission quota for a certain period of time based on the historical carbon emission level of the company. It can also limit the total carbon emission of the original manufacturer. However, historical method has its limitations sucha as regardless of the emerging industries and the development of technology and leading to carbon leakage (Hu et al., 2017; Qin et al., 2017). Most carbon trading systems initially use the historical method as a free allocation method. In the first and second stages of EU ETS, most member states use the historical method for allocation. Tokyo’s carbon trading system also allocates allowances based on historical emissions.
Suppose that the carbon emission of company i is ei, i = 1,2,… 8; The output of the company is yi. The final quota for companies is Ei. When using the history method, the quota obtained by company i is the historical carbon emission of the company:
The idea of the baseline method is to calculate the quota allocation of industries based on the determined emission benchmark value of the industry and the product output. The baseline method is suitable for companies whose products are relatively single with detailed production and emission data while is not suitable for industries with complex products and production processes. The typical baseline method is based on the principle of “Best Practice”. The principle ranks the carbon emissions per unit product of the same product of different companies from small to large, selecting the top 10% as the baseline. So,each company receives a quota equal to its output multiplied by the baseline value. From the beginning of the third phase of EU ETS, a baseline allocation method based on “best practices” will be introduced for the portion of the free quota. The free allowances in California’s carbon market are also based on this baseline. This baseline is equal to 90% of the average carbon emissions per unit of product produced by different companies.
The baseline method sets the carbon emission quota for each company according to the carbon emission data of the reference year and the output of the company. When using the baseline method, the quota obtained by company i is the product of the output value of the company and the industry baseline value:
Where b is the industry base line, assuming that the carbon emission per unit product of the j company is taken as the base line value, then b=ej/yj. This paper follows the principle of “Best Practice” and selects the carbon emissions generated by the top 10% of the industry as the baseline.
Some studies have proposed the combination of several methods. One research proposed a hybrid method for free allocation based on output and emission data and outcome-based allocation was consistent with the baseline method in essence. Another research argued that baseline method should be applied to carbon market as a transitional method between historical method and auction method. It can avoid the incentive distortion of historical method (Zetterberg, 2014). In this paper, the method combining the historical method and the baseline method is called the mixed method. It means that the quota determined by the historical method and the quota determined by the baseline method are weighted according to a certain proportion. The mixed method can not only consider the historical emissions of companies, but also give companies certain incentives to reduce emissions.
When using the mixed historical baseline method, the quota obtained by company i is weighted by the historical method and the baseline method:
Where w1 and w2 are the weights of the historical and baseline quotas respectively, and w1+w2 = 1. This paper considers the case of equal weight distribution when the importance of the two distribution methods is the same.
In the research of carbon quota allocation based on the principle of fairness and efficiency, the index method, the optimal method and the game theory are widely used. The index method is often used to reflect the principle of fairness (Duro and Padilla, 2006; Hedenus and Azar, 2006; Groot, 2010). In addition to a single indicator, research can chose multiple indicators to allocate carbon quotas (Zhang et al., 2014). Nonlinear models and game theroy are also used in carbon quota studies (Viguier et al., 2006; Xu et al., 2019; Decanio and Fremstad, 2018).
In optimization method, DEA method is widely used because it does not need to set the production frontier function in advance. To evaluate the marginal emission reduction cost of EU member states, some studies chose DEA direction distance function technology (Marklund and Samakovlis, 2007). Meanwhile,the ZSG-DEA model can be used to allocate quotas among countries to maximize efficiency. Result showed that most developed countries should increase their carbon emission quotas, while most less developed countries should reduce their carbon emission quotas (Pang et al., 2015). Based on the relaxation variable measure DEA method (SBM-DEA), reseach calculated the marginal emission reduction cost of Italian livestock farms (Cecchini et al., 2018).
The traditional DEA model can evaluate the efficiency of each DMU. The model has an assumption that different DMU in the model are independent, and each DMU will not be affected by other DMU. It is precisely because of the above assumptions in this model that the efficiency of low-efficiency DMU cannot be changed through the traditional DEA model and the optimal allocation cannot be realized. In order to make all DMU effective, the ZSG-DEA model is used in this paper.
After quota allocation based on the principle of fairness, it is necessary to measure the efficiency of various allocation methods. Then, select the allocation mechanism that takes into account the principle of fairness and efficiency. The basic principle of ZSG-DEA model is to convert the input and output indexes of multiple decision units into mathematical models. Then the model solves them by linear programming method. This model can not only evaluate the efficiency of the initial carbon quota allocation in the shipping industry, but also adjust each decision unit to reach the effective boundary. Meanwhile,it can verify the effectiveness and fairness of the allocation results. In this paper,. carbon quota (E) is taken as the non-expected input, total output value (Y) is taken as the expected output, and energy consumption (EC) is taken as the non-expected output. Build the following model:
Where, θE represents the allocation efficiency. It is under the condition that the total amount of carbon emission quota is fixed. EZGE represents the average efficiency of unit weight of all decision making units. λi represents the extent to which DUMi (company i) contributes to the predictive efficiency. ei represents the carbon quota input of company i. yi represents the total industrial output value of company i, and eci represents the energy consumption output of company i. EG, YG, ECG represents inputs, outputs and non-expected output in the evaluation of DUMG, respectively. Since the input-output based ZSG-DEA model is used in this paper, the input variables should be adjusted to make each DMU efficient. After several reallocations, almost all DMU can reach the efficiency front, and the optimal allocation of each shipping company’s carbon emission quota can be obtained. So,adjusting all non effective decision making units, until all decision making units to achieve efficient frontier, maens that efficiency is close to or equal to 1.
We need obtain historical carbon emissions and historical output data for eight companies: Maersk, MSC, CMA, COSCO,HPL, EMC, YML and WHL.
Many theories and methods can be used to calculate carbon emissions, such as life cycle theory and fuel method (Xiao et al., 2023). Considering that the shipping industry generates carbon emissions mainly from fuel emissions, in this paper, the fuel method is used to calculate the carbon emissions of ships. It can calculate the carbon emissions of ships according to the consumption of various fuels in a certain period of time. The core is to calculate the consumption of various fuels and select the corresponding carbon conversion coefficient of various fuels. In a certain period of time, the carbon emission calculated by the fuel method is equal to the sum of the product of the fuel consumption and the carbon conversion coefficient corresponding to the fuel. At present, both operational and technical carbon intensity indicators adopt the fuel method to calculate carbon emissions of ships, but the methods of obtaining fuel consumption are different. The difference is that the operational carbon intensity indicators directly calculate carbon emissions based on the reported fuel consumption of ships. But the technical carbon intensity indicators indirectly calculate fuel consumption based on the power plant power and fuel consumption rate. To calculate carbon emissions. At present, the fuel grade used by ships has been standardized, and the carbon conversion coefficient of various fuels is relatively fixed.
By collecting the energy consumption and operation data of ships and using the fuel method to calculate the carbon emissions of ships, the operational carbon intensity index can be calculated more easily. Therefore, the current operational carbon intensity index adopts the fuel method to calculate the carbon emissions of ships, which is expressed by equation (10):
Where, FCj refers to the mass of fuel j consumed by the ship in a certain period of time; CFj is the carbon conversion coefficient of fuel j.
The technical carbon intensity index is calculated under the ideal design environment and standard working conditions. The technical carbon intensity index adopts the fuel method to calculate the carbon emission of the ship. The carbon emission is equal to the sum of the product of the power, running time, fuel consumption rate, carbon conversion coefficient, etc. of various power plants, which is expressed by equation (11):
Where, pi is the power of the power plant i; ηi is the efficiency of power plant i; hi is the running time of the power plant i in the state pi; j is the fuel i uses in the state pi; CFj is the carbon conversion coefficient of fuel j; Qj is the calorific value of fuel j.
According to the above calculation method, the historical carbon emissions of eight shipping companies are calculated. The results are shown in Table 1.
The historical output value of the eight companies can be obtained from the annual reports of the companies, and the results are shown in Table 2. Affected by the COVID-19, the output value of some shipping companies changed significantly, which is because the price of TEU changed greatly.
After obtaining the historical carbon emission data and output value data of eight companies, the data of 2024 can be predicted according to the average growth rate. Further,we can obtain the initial carbon emission quota under three scenarios, in which scenario 1 corresponds to the historical method, scenario 2 corresponds to the baseline method, and scenario 3 corresponds to the mixed method. The distribution efficiency under different scenarios can be analyzed from equations (1) to (9), and the results are shown in Table 3. The main findings are as follows:
Firstly, the initial quota allocation results of companies in all cases are relatively close, and the average allocation efficiency of carbon emission quotas in scenario 3 is slightly higher. It shows that there is no significant difference between the historical method, the baseline method and the mixed method in terms of the efficiency of carbon emission quota allocation. The mixed method has a slightly higher efficiency. Secondly, the efficiency of scenario 3 is slightly higher. This scenario includes considering companies’ past carbon emission behavior, industry standards and rewarding those companies with excellent performance in emission reduction. These are the main factors affecting the allocation of carbon emission allowances. Meanwhile,these are the key factors affecting the realization of carbon emission reduction targets in the shipping industry. Finally, the allocation of carbon emission allowances under each scenario has not reached the Pareto optimal state, which indicates that there is still room for improvement in these eight companies. The efficiency of CMA in scenario 1 and scenario 3 is 1, indicating that the company’s allocation results are effective. Adjust the number of carbon emission allowances according to equation (7) until the allocation efficiency of all companies is 1. There is no further room for adjustment to reach a Pareto optimal state.
In view of the high efficiency of carbon emission quota allocation in scenario 3, the carbon emission quota of each company under Scenario 3 is adjusted according to equation (7). After two adjustments, the carbon emission quota is redistributed among eight shipping companies. The adjustment process of carbon emission quota and the final result are shown in Table 4. And according to the results, this paper draw the image of the Figures 2, 3 shows the adjustment of the efficiency of carbon and carbon quota. After two adjustment lags, the carbon emission allocation efficiency of all companies reaches 1, indicating that the carbon emission quota of all companies is effective at this time. There is no room for adjustment. At this time, the distribution scheme is the most reasonable.
Compared with the initial allocation results, the change of carbon emission quota is small, and the overall carbon emission quota decreases slightly, among which Maersk has the largest carbon emission quota, reaching almost 32 million tons. The high emission of Maersk corresponds to the large amount of carbon emission generated by its high output. the demand for emission reduction is high, so it should be allocated quite a large amount of carbon emission quota. MSC also has a high carbon emission quota. More than 8 million tons, indicating that they also have a higher demand for carbon emissions, and a higher demand for carbon emission allowances.
In summary, we can draw the following conclusions: Firstly, the historical method, the baseline method and the mixed method can all effectively allocate carbon emission quotas for shipping companies, among which the mixed method is relatively efficient. Secondly, the ZSG-DEA model can be used to determine the optimal carbon emission allocation by taking into account the principles of fairness and efficiency. In the optimal state of Pareto, Maersk has the highest carbon emission quota among the eight typical shipping companies in 2024, reaching 32,431,800 tons, followed by MSC and EMC, reaching 8,542,400 tons and 6,809,500 tons, respectively.
Based on the above conclusions, this paper puts forward the following suggestions for the allocation of carbon emission quotas for shipping companies:The carbon emission quota allocation method combining the historical method and the baseline method can make full use of the advantages of the two methods. It can achieve more effective carbon emission reduction and resource allocation. It can ensure the adaptability of allocation methods with a degree of flexibility. Also it will facilitate the participation and cooperation of different industries and companies. In addition, The shipping industry can encourage companies to adopt more environmentally friendly and low-carbon technological innovations, and obtain additional carbon credits by reducing carbon emissions. Governments can set up incentive mechanisms to support shipping companies in developing and adopting new technologies, such as ship fuel efficiency improvement and navigation path optimization. Also, the shipping industry is an international industry, and transnational cooperation and the formulation of uniform carbon emission standards are very important. International organizations and governments can jointly formulate guidelines and standards for the allocation of carbon emission allowances. Meanwhile they can ensure fair competition among shipping companies from different countries on a global scale, and jointly promote carbon emission reduction in the shipping industry. Finally, Carbon emission reduction in the shipping industry is a long-term process that requires the pursuit of progressive emission reduction targets and sustainable development. The allocation method of carbon emission allowances should be time-related. Therefore, we should gradually reduce the total amount of carbon allowances, and match the emission reduction plans of shipping companies to achieve long-term carbon reduction targets.
The original contributions presented in the study are included in the article/supplementary material. Further inquiries can be directed to the corresponding author.
ZH: Writing – original draft. YH: Writing – review & editing. LS: Writing – original draft. XQ: Writing – review & editing. XP: Writing – review & editing.
The author(s) declare financial support was received for the research, authorship, and/or publication of this article. This work was supported by National key research and development program [grant number 2022YFF0903403] .
The authors declare that the research was conducted in the absence of any commercial or financial relationships that could be construed as a potential conflict of interest.
All claims expressed in this article are solely those of the authors and do not necessarily represent those of their affiliated organizations, or those of the publisher, the editors and the reviewers. Any product that may be evaluated in this article, or claim that may be made by its manufacturer, is not guaranteed or endorsed by the publisher.
Atte Pitkänen, Tuuli. v. W., Janne. K., Helena K. (2022). Distributional fairness of personal carbon trading. Ecol. Economics 201, 1–13. doi: 10.1016/j.ecolecon.2022.107587
Baer P. (2013). The Greenhouse Development Rights framework for global burden sharing: reflection on principles and prospects. Wiley Interdiscip. Rev. Climate Change. 4 (1). doi: 10.1002/wcc.201
Bintang Y., Ping Y., Sabine F., Griscom B. W., Pete S., Florian K. (2023). Doing burden-sharing right to deliver natural climate solutions for carbon dioxide removal. Nature-Based Sol. 3.
Böhringer C., Lange A. (2005). On the design of optimal grandfathering schemes for emission allowances. Eur. Economic Rev. 49 (8), 2041–2055. doi: 10.1016/j.euroecorev.2004.06.006
Cecchini L., Venanzi S., Pierri A., Chiorri M. (2018). Environmental efficiency analysis and estimation of CO2 abatement costs in dairy cattle farms in Umbria (Italy): A SBM-DEA model with undesirable output. J. Cleaner Production 197, 895–907. doi: 10.1016/j.jclepro.2018.06.165
Cucchiella F., Adamo L. D., Gastaldi M., Miliacca M. (2018). Efficiency and allocation of emission allowances and energy consumption over more sustainable European economies. J. Cleaner Production 182, 805–817. doi: 10.1016/j.jclepro.2018.02.079
Decanio S., Fremstad A. (2018). Game theory and climate diplomacy. Ecol. Economics 85, 177–187. doi: 10.1016/j.ecolecon.2011.04.016
Duro J. A., Padilla E. (2006). International inequalities in per capita CO2 emissions: A decomposition methodology by Kaya factors. Energy Economics 28 (2), 170–187. doi: 10.1016/j.eneco.2005.12.004
Groot L. (2010). Carbon lorenz curves. Resource Energy Economics 32 (1), 0–64. doi: 10.1016/j.reseneeco.2009.07.001
Hedenus F., Azar C. (2006). Estimates of trends in global income and resource inequalities. Ecol. Economics 55 (3), 351–364. doi: 10.1016/j.ecolecon.2004.10.004
Hong Z., Chu C., Yu Y. (2016). Dual-mode production planning for manufacturing with emission constraints. Eur. J. Operational Res. 251 (1), 96–106. doi: 10.1016/j.ejor.2015.11.015
Hu Y. J., Han R., Tang B. J. (2017). Research on the initial allocation of carbon emission quotas: evidence from China. Natural Hazards 85 (2), 1189–1208. doi: 10.1007/s11069-016-2628-y
Karp L., Simon L. (2013). Participation games and international environmental agreements: A non-parametric model. J. Environ. Economics Manage. 65 (2), 326–344. doi: 10.1016/j.jeem.2012.09.002
Mackenzie I. A., Hanley N., Kornienko T. (2008). The optimal initial allocation of pollution permits: a relative performance approach. Environ. Resource Economics 39. doi: 10.1007/s10640-007-9125-4
Marklund P. O., Samakovlis E. (2007). What is driving the EU Burden-sharing Agreement: efficiency or equity? J. Environ. Manage. 85 (2), 317–329. doi: 10.1016/j.jenvman.2006.09.017
Pang R. Z., Deng Z. Q., Chiu Y. H. (2015). Pareto improvement through a reallocation of carbon emission quotas. Renewable Sustain. Energy Rev. 50 (oct. ), 419–430. doi: 10.1016/j.rser.2015.05.022
Park J. W., Kim C. U., Isard W. (2011). Permit allocation in emissions trading using the boltzmann distribution.. Physica A: Statistical Mechanics and its Applications. 391 (20), 4883–4890. doi: 10.1016/j.physa.2012.05.052
Pozo C., Galán-Martín. A., Cortés-Borda D., Sales-Pardo M., Guillén-Gosálbez G. (2020). Reducing global environmental inequality: Determining regional quotas for environmental burdens through systems optimisation. J. Cleaner Production . 270, 121828. doi: 10.1016/j.jclepro.2020.121828
Qin Q., Liu Y., Li X., Li H. (2017). A multi-criteria decision analysis model for carbon emission quota allocation in China's east coastal areas: efficiency and equity. J. Cleaner Production 168, 410–419. doi: 10.1016/j.jclepro.2017.08.220
Rose A., Stevens B., Edmonds J., Wise M. (1998). International equity and differentiation in global warming policy. Environ. Resource Economics 12 (1), 25–51. doi: 10.1023/A:1008262407777
Schmidt R. C., Heitzig J. (2014). Carbon leakage: Grandfathering as an incentive device to avert firm relocation. Acad. Press 2, 209–223. doi: 10.1016/j.jeem.2013.12.004
Shojaei T., Mokhtar A. (2021). Carbon mitigation by quota allocation. J. Environ. Manage. 304, 114097. doi: 10.1016/j.jenvman.2021.114097
Steenberghe V. V. (2004). Core-stable and equitable allocations of greenhouse gas. SSRN Electronic J. 75, 1–11. doi: 10.2139/ssrn.683162
Viguier L., Vielle M., Haurie A., Bernard A. (2006). A two-level computable equilibrium model to assess the strategic allocation of emission allowances within the European Union. Comput. Oper. Res. 3, 369–385. doi: 10.1016/j.cor.2004.06.010
Wei Y.-M., Wang L., Liao H., Wang K., Murty T., Yan J. (2014). Responsibility accounting in carbon allocation: a global perspective. Applied Energy 130, 122–133.
Xiao G., Lu Q., Ni A., Zhang C. (2023). Research on carbon emissions of public bikes based on the life cycle theory. Transportation Lett. 15, 4, 278–4, 295.
Xu Z., Yao L., Liu Q., Long Y. (2019). Policy implications for achieving the carbon emission reduction target by 2030 in Japan-Analysis based on a bilevel equilibrium model. Energy Policy 134. doi: 10.1016/j.enpol.2019.110939
Zetterberg L. (2014). Benchmarking in the european union emissions trading system: abatement incentives. Energy Economics. 43, 218–224. doi: 10.1016/j.eneco.2014.03.002
Zhang Y. J., Wang A. D., Da Y. B. (2014). Regional allocation of carbon emission quotas in China: Evidence from the Shapley value method. Energy Policy 74, 454–464. doi: 10.1016/j.enpol.2014.08.006
Zhou P., Sun Z. R., Zhou D. Q. (2014). Optimal path for controlling CO2 emissions in China: A perspective of efficiency analysis. Energy Economics 45, 99–110. doi: 10.1016/j.eneco.2014.06.019
Keywords: shipping industry, fairness and efficiency, carbon quota, ZSG-DEA model, EUETS
Citation: Hu Z, Huang Y, Sun L, Qi X and Pan X (2023) Study on international carbon emission quota allocation of shipping industry-based on fairness and efficiency. Front. Mar. Sci. 10:1269643. doi: 10.3389/fmars.2023.1269643
Received: 30 July 2023; Accepted: 05 October 2023;
Published: 18 October 2023.
Edited by:
Mingyang Zhang, Aalto University, FinlandReviewed by:
Yi Xiao, Zhejiang University, ChinaCopyright © 2023 Hu, Huang, Sun, Qi and Pan. This is an open-access article distributed under the terms of the Creative Commons Attribution License (CC BY). The use, distribution or reproduction in other forums is permitted, provided the original author(s) and the copyright owner(s) are credited and that the original publication in this journal is cited, in accordance with accepted academic practice. No use, distribution or reproduction is permitted which does not comply with these terms.
*Correspondence: Ling Sun, c3VubEBzaG10dS4gZWR1LiBjbg==
Disclaimer: All claims expressed in this article are solely those of the authors and do not necessarily represent those of their affiliated organizations, or those of the publisher, the editors and the reviewers. Any product that may be evaluated in this article or claim that may be made by its manufacturer is not guaranteed or endorsed by the publisher.
Research integrity at Frontiers
Learn more about the work of our research integrity team to safeguard the quality of each article we publish.