- 1Ecoresolve, San Francisco, CA, United States
- 2Applied Geography and GIS Program, Department of Humanities, College of Arts and Sciences, Qatar University, Doha, Qatar
- 3BlueForests, San Francisco, CA, United States
- 4United Nations Volunteering Program, via Morobe Development Foundation, Lae, Papua New Guinea
- 5Department of Geography, Faculty of Social and Management Sciences, University of Buea, Buea, Cameroon
- 6Department of Geography, College of Arts and Social Sciences, Sultan Qaboos University, Muscat, Oman
- 7Department of Ecology and Conservation Biology, Texas A&M University, College Station, TX, United States
- 8Scion, Christchurch, New Zealand
- 9Elliott School of International Affairs, George Washington University, Washington, DC, United States
- 10Center for Interacting Urban Networks (CITIES) and Arabian Center for Climate and Environmental Sciences (ACCESS), New York University Abu Dhabi, Abu Dhabi, United Arab Emirates
- 11Faculty of Science, Medicine and Health, School of Earth, Atmospheric and Life Sciences (SEALS), Wollongong, NSW, Australia
- 12Department of Civil Engineering, College of Engineering, American University of Sharjah (AUS), Sharjah, United Arab Emirates
- 13School of Earth, Atmosphere and Environment, Monash University, Clayton, VIC, Australia
- 14Department of Biological and Agricultural Engineering, Texas A&M University, College Station, TX, United States
- 15Research Centre for Oceanography, National Research and Innovation Agency, Jakarta, Indonesia
- 16Department of Earth Sciences, Institute for Geographical Sciences, Freie Universität Berlin, Berlin, Germany
- 17Department of Agricultural and Forest Sciences and Engineering, University of Lleida, Lleida, Spain
- 18Forest Science and Technology Centre of Catalonia (CTFC), Solsona, Spain
- 19The Nature Conservancy, Maryland/DC Chapter, Cumberland, MD, United States
- 20Unit of Applied Artificial Intelligence, Eurecat, Centre Tecnològic de Catalunya, Barcelona, Spain
- 21School of Biological Sciences, University of California—Irvine, Irvine, CA, United States
- 22Department of Forest Analytics, Texas A&M Forest Service, Dallas, TX, United States
- 23Earth Observation Center, Institute of Climate Change, Universiti Kebangsaan Malaysia, Bangi Selangor, Malaysia
- 24University of Liverpool Management School, University of Liverpool, Liverpool, United Kingdom
- 25National Agricultural Research Center (NARC), Amman, Jordan
- 26Tecnosylva, León, Spain
- 27Department of Civil Engineering, Papua New Guinea University of Technology, Lae, Papua New Guinea
- 28Department of Geography, University of California - Berkeley, Berkeley, CA, United States
Mangrove forests in the Gulf Cooperation Council (GCC) countries are facing multiple threats from natural and anthropogenic-driven land use change stressors, contributing to altered ecosystem conditions. Remote sensing tools can be used to monitor mangroves, measure mangrove forest-and-tree-level attributes and vegetation indices at different spatial and temporal scales that allow a detailed and comprehensive understanding of these important ecosystems. Using a systematic literature approach, we reviewed 58 remote sensing-based mangrove assessment articles published from 2010 through 2022. The main objectives of the study were to examine the extent of mangrove distribution and cover, and the remotely sensed data sources used to assess mangrove forest/tree attributes. The key importance of and threats to mangroves that were specific to the region were also examined. Mangrove distribution and cover were mainly estimated from satellite images (75.2%), using NDVI (Normalized Difference Vegetation Index) derived from Landsat (73.3%), IKONOS (15%), Sentinel (11.7%), WorldView (10%), QuickBird (8.3%), SPOT-5 (6.7%), MODIS (5%) and others (5%) such as PlanetScope. Remotely sensed data from aerial photographs/images (6.7%), LiDAR (Light Detection and Ranging) (5%) and UAV (Unmanned Aerial Vehicles)/Drones (3.3%) were the least used. Mangrove cover decreased in Saudi Arabia, Oman, Bahrain, and Kuwait between 1996 and 2020. However, mangrove cover increased appreciably in Qatar and remained relatively stable for the United Arab Emirates (UAE) over the same period, which was attributed to government conservation initiatives toward expanding mangrove afforestation and restoration through direct seeding and seedling planting. The reported country-level mangrove distribution and cover change results varied between studies due to the lack of a standardized methodology, differences in satellite imagery resolution and classification approaches used. There is a need for UAV-LiDAR ground truthing to validate country-and-local-level satellite data. Urban development-driven coastal land reclamation and pollution, climate change-driven temperature and sea level rise, drought and hypersalinity from extreme evaporation are serious threats to mangrove ecosystems. Thus, we encourage the prioritization of mangrove conservation and restoration schemes to support the achievement of related UN Sustainable Development Goals (13 climate action, 14 life below water, and 15 life on land) in the GCC countries.
Highlights
● Few remote sensing studies have focused on mangrove forests in GCC countries.
● A survey of 58 studies found that mangrove forest parameters were mainly estimated from Landsat images (75.9%).
● LiDAR (5.2%) and UAV (3.4%) imagery were the least used compared to other high resolution satellite remote sensing data sources.
● Mangrove cover in GCC countries decreased, remained stable or increased from 1996 and 2020, attributed to reforestation and afforestation efforts.
● Visual digitization and interpretation (46.6%) and machine learning (25.9%) techniques were the most commonly used mangrove classification approaches.
1 Introduction
Global mangrove forests provide important ecosystem services that are critical for the environment and human well-being. These include climate regulation through carbon sequestration, water purification through filtering and pollutant retention, nutrient cycling, provision of livelihoods, soil erosion control, coastal protection, and habitat provision (Bunting et al., 2022; Hagger et al., 2022). Global mangroves are recognized as carbon-rich tropical ecosystems (Donato et al., 2011), estimated to mitigate more than 25.5 million carbon tonnes annually and supply 10% of the vital liquefied carbon in the world’s oceans (Khader, 2023). In general, healthy mangroves can store about 21 gigatons of carbon, the equivalent to three years of greenhouse gas (GHG) emissions from a nation like Australia, while restored degraded mangroves could lead to the sequestration of an additional 1.3 gigatons on a global scale (Spalding and Leal, 2021). Thus, mangrove ecosystems are now considered a high-priority conservation target for large scale international conservation initiatives such as the International Blue Carbon Initiative and the Global Mangrove Alliance. These ecosystems are increasingly incorporated into the Nationally Determined Contributions of countries to meet their pledges to the Paris Agreement of the United Nations Framework Convention on Climate Change (Friess et al., 2020).
Mangroves exist in complex social-ecological systems around the world, and local economic pressures and biophysical drivers impact the condition of these mangrove forests (Goldberg et al., 2020; Hagger et al., 2022). Mangroves are disappearing three to five times faster than other forests, which is resulting in severe ecological and socio-economic challenges (Friess et al., 2020). From 1996 to 2020, more than 5, 245 km2 (about 3.4%) of mangrove cover has been lost due to expansion of urban development, agriculture, and aquaculture in coastal environments (Bunting et al., 2022). Advances in remote sensing technologies have allowed us to quantify losses in mangrove cover due to anthropogenic stressors and characterize land use changes with high accuracy and precision (Goldberg et al., 2020; Bunting et al., 2022).
Mangroves in the Gulf Cooperation Council (GCC) countries are resilient evergreen forest ecosystems that are well adapted to the extreme environmental conditions of high temperatures and hypersalinity found in this region that make it hard for other forest ecosystems to thrive (Friis and Burt, 2020; Getzner and Islam, 2020). As a consequence of these environmental extremes, only two mangrove species - namely Avicennia marina and Rhizophora mucronata - commonly called gray and red mangrove, respectively, have been reported to flourish in the GCC countries (Almahasheer, 2018). They represent the dominant tree species resilient enough to grow and endure the extremely dry climates of the GCC countries, leading to the development of a unique vegetation habitat (Elsebaie et al., 2013; Almahasheer, 2018). Mangroves in the GCC countries tolerate temperatures of up to 47°C, water temperatures of up to 22-34°C, and water salinity of up to 44% (Elsebaie et al., 2013; Monsef et al., 2013). It was reported that A. marina grows naturally in all of the GCC countries, while R. mucronata was reported to grow over the Red Sea coast in Farasan Kabir and Kamaran Islands of Saudi Arabia (Kumar et al., 2010; Alwhibi, 2017). R. mucronata also grows in the waters of Ras Ghanada Island in the UAE, following a successful reintroduction effort by the Environment Agency-Abu Dhabi (Milani, 2018). In Qatar, mangrove height and tree density have been found to range between 0.6-6 meters and 1,000 to 2,600 trees/ha, respectively (Al-Khayat and Balakrishnan, 2014). Measurements obtained from the field and analyzes of leaves or sediments can complement remote sensing data in characterizing the status of mangrove communities within the GCC as shown by multiple studies (Al-Ali et al., 2015; Moore et al., 2015; Alwhibi, 2017).
Mangroves are the only naturally-occurring evergreen forest in the GCC countries and hold great importance because of the ecosystem services they provide (Friis and Burt, 2020). Mangroves in the GCC countries represent only a small fraction (0.11%, 147,359 km2) of the total global mangrove area in 2020 (Bunting et al., 2022). They are found along the coastal shorelines of the Red Sea, Arabian Sea, Arabian Gulf, and Gulf of Oman, and these areas are located in Saudi Arabia, UAE, Qatar, Oman, Bahrain, and Kuwait. Among these countries, Saudi Arabia and the UAE have the largest mangrove forest area, which in combination constitute more than 95% of the total mangrove cover in the GCC countries (Bunting et al., 2022).
The importance of mangrove ecosystems for climate change mitigation is well documented as they sequester carbon more efficiently than other terrestrial forest ecosystems and consequently are considered important long-term carbon sinks (Alongi, 2020; Al-Nadabi and Sulaiman, 2021; Abd El-Hamid et al., 2022a; Aljenaid et al., 2022). Mangroves represent one of the most important ‘blue carbon’ ecosystems in this arid region (Schile et al., 2017; Cusack et al., 2018; Bukoski et al., 2020; Macreadie et al., 2021) and at the global level for climate change mitigation and adaptation (Duarte et al., 2013; Alongi, 2020). The carbon sequestration potential of mangroves is of particular importance for many countries within the GCC countries, which include some of the highest per capita greenhouse gas emitters in the world. During 2019, carbon emissions in metric tons (tCO2e) per capita were very high for Qatar (32.47 tCO2e), Kuwait (22.02 tCO2e), Bahrain (20.26 tCO2e), Saudi Arabia (15.28 tCO2e), Oman (15.29 tCO2e), and UAE (19.33 tCO2e) (Climate Watch, 2022). This is tied to the socio-economic landscape of the GCC countries that has been progressively characterized by the development of the oil and gas industry and urban development at the detriment of mangrove conservation (Burt and Bartholomew, 2019; Vaughan et al., 2019).
Consequently, protection, conservation and restoration initiatives are of high interest for these countries as mangrove forests can be used to offset, for example, part of national oil and gas company emissions through carbon market mechanisms (Macreadie et al., 2021). Thus, progressive efforts have been made by the governments of Oman, United Arab Emirates (UAE), Qatar, Bahrain, and Saudi Arabia, especially post-2000 to expand and implement mangrove restoration and conservation programs (Milani, 2018). Most recently, the UAE through Abu Dhabi’s Environment Agency and Abu Dhabi National Oil Company (ADNOC) began plans to plant up to 10 million mangrove seedlings in Abu Dhabi by 2030 (ADNOC, 2023). However, long-term sustenance and adaptive management of these restoration initiatives is important to ensure climate-related outcomes are realized.
Despite ongoing restoration since the 1980s, and more extensively in recent years, A. marina along the Qatar coastline (at Simaisma, Al Thakhira, Al Khor, and Fuwairit) are threatened due to the escalating scale of coastal development and an expanding tourism industry (Al-Khayat and Balakrishnan, 2014; Burt et al., 2017). Environmental hazards are exacerbated by climate change, including, but not limited to, sea-level rise, recurrent hypoxia, a shortage of fresh water, extreme storm surges, increasing wave energy, drought, flooding, inundation and erosion (Lincoln et al., 2021; Melville-Rea et al., 2021; Lachkar et al., 2022). It is important to assess the potential impacts of these threats so that management efforts can be undertaken to adapt and minimize their impacts (Babu et al., 2012; Bahrawi and Elhag, 2016; Hereher, 2016; Hereher and Al-Awadhi, 2019). Thus, developing a conceptual understanding of the extent and the severity of the impacts of the direct and indirect threats that lead to loss of mangrove cover, is important to develop strategies and actions (Rittenhouse, 2017). Because this mangrove ecosystem is very unique, there is a need to characterize its current status and the additional biophysical and anthropogenic pressures it endures.
Efforts to map, monitor and model mangrove restoration opportunities using remote sensing techniques can greatly improve the success of restoration programs (Monsef et al., 2013). Previous studies in the GCC region have used remote sensing data to quantify mangrove distribution and cover, temporal gain or loss of cover, ecological state of mangrove ecosystems (Elsebaie et al., 2013; Alsumaiti et al., 2019; Butler et al., 2020; Butler et al., 2021; Aljenaid et al., 2022), and the influence of physical and chemical properties of seawater, soil and geomorphology on the site suitability for mangrove restoration projects (Elsebaie et al., 2013; Alsumaiti, 2017; Butler et al., 2020; Butler et al., 2021; Aljenaid et al., 2022).
The Global Mangrove Watch (GMW) provides useful baseline data around current mangrove extent within the GCC. During 2020, GMW detected mangrove cover within five of the six GCC countries, but mangroves were not detected in Kuwait (Figure 1). Between these countries, Saudi Arabia (77.1 km²) and the UAE (74.4 km²) account for the largest areas, while Qatar (4.5 km²), Bahrain (0.6 km²) and Oman (1.4 km²) have smaller mangrove areas (Bunting et al., 2022). Kuwait is the only country in the region that has no natural mangrove forest. Plantation establishment experiments have been undertaken in Kuwait since 1968 using A. marina and have reportedly been successful since 2001 (Milani, 2018), evidenced by a reported area of 0.58 km² of mangrove cover in 2017 (Almahasheer (2018). The discrepancy between mangrove cover in Kuwait, reported in various sources, is highlighted by (Guo et al., 2021) as their method provides results for 1990, 2000, 2010 and 2015 while FAO, GMW and WCMC (the Global Distribution of Mangroves study executed by USGS in 2011) do not.
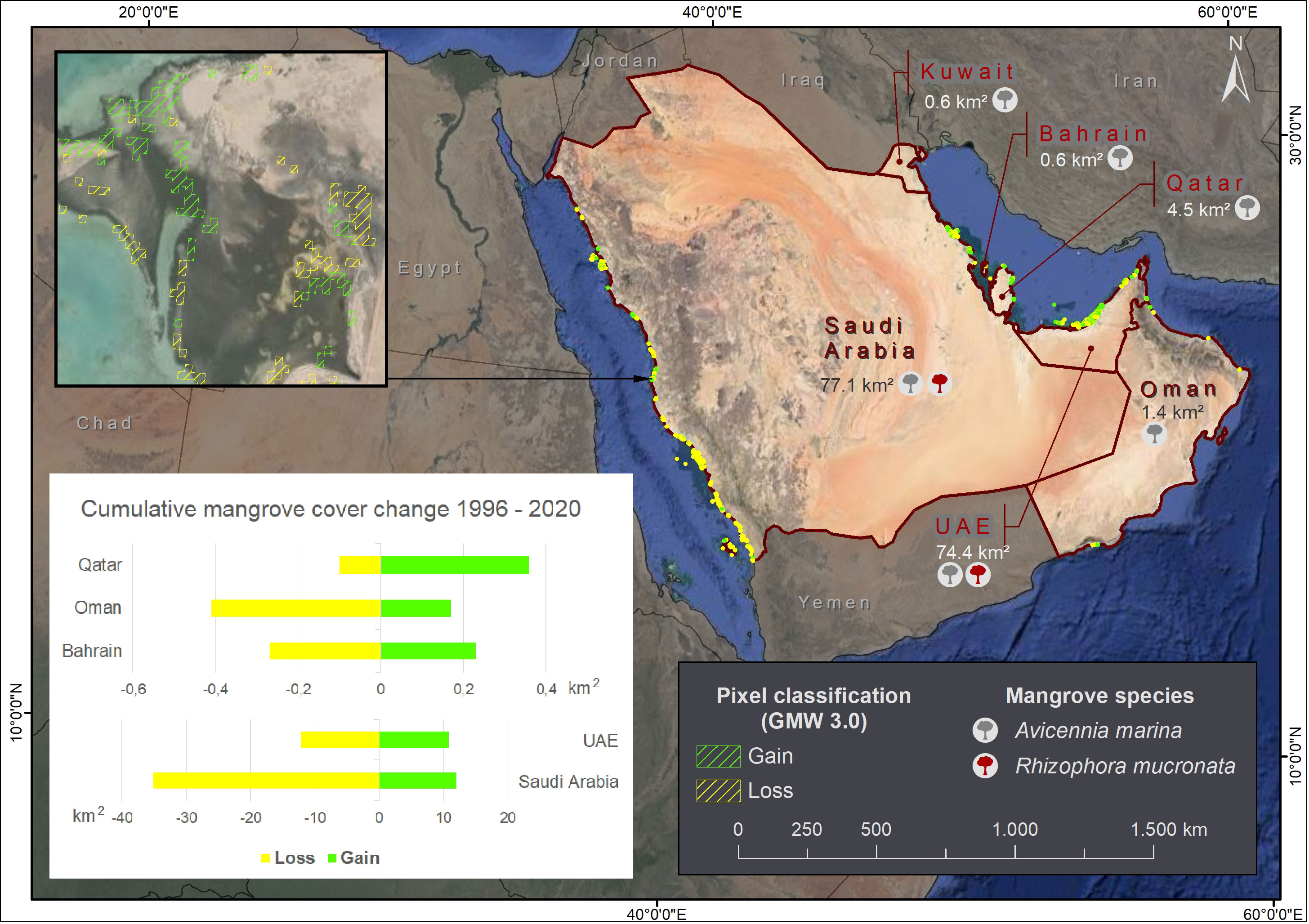
Figure 1 Location of mangrove forest and area determined by GMW 3.0. Green pixels represent gain in mangrove cover and yellow is used for loss in a comparison between 1996 and 2020. The cumulative change for Qatar, Oman and Bahrain is below 1 km2 while UAE and Saudi Arabia are over 10 km2. A. marina is present in all GCC countries while R. mucronata can only be found in Saudi Arabia and UAE.
Based on the data released by GMW (Bunting et al., 2022), Qatar is the only GCC country in which the mangrove forest extent increased between 1996 and 2020 (by 0.26 km²). For Bahrain and Oman, estimated losses were 0.04 km² and 0.24 km², respectively, over this time period. Area changes in Saudi Arabia and UAE were similar, with area peaking in 2008, declining until 2016, and then slightly increasing until 2020. Over the period from 1996 to 2020, there were losses in mangrove extent in Saudi Arabia and UAE of 23 km² and a near-negligible 1.38 km², respectively (Figure 1).
While remote sensing has been increasingly used as the primary data source for delineating mangrove distribution, cover and habitat in the GCC countries, there are often variations in characteristics of these data (e.g. spectral, spatial and temporal resolution) that can influence estimates. Thus, the importance of fieldwork that provides ground truth data to validate satellite data and the opportunity to consult local and community experts cannot be overlooked, particularly for local scale studies (Mateos-Molina et al., 2020). Importantly, studies that combine field and remote sensing data are important to accurately represent mangrove ecosystems and their dynamics (Howari et al., 2009), including, for example, the sensitivity of range limits to climate variability, deforestation or regeneration rates and assessments of biomass and carbon stocks (Ximenes et al., 2023). However, no study has reviewed remote sensing-based assessments of mangrove ecosystems for the entire GCC region, advantages and limitations of different remotely sensed data types, and the measured mangrove characteristics that are important for climate change mitigation.
In this study, we undertook a comprehensive analysis of the remote sensing-based mangrove studies published from 2010 to 2022 in the GCC countries. Our objectives were to 1) examine the temporal and spatial distribution of the reviewed articles, 2) the most widely estimated mangrove forest parameters, 3) the vegetation indices/metrics that are used to classify mangrove forests, and 4) the remote sensing data types and classification methods used to assess and characterize mangrove forests in the GCC countries. We discuss the mangrove conservation initiatives implemented in the GCC countries, and how the fusion of remote sensing data can be used to increase the feasibility and accuracy of mapping and monitoring mangroves with reasonable cost and agility. Because data from UAV-LiDAR are very useful for detailed small scale studies that require high accuracy and ground validation of satellite data, we further discuss the UAV-based rules and regulations for operation in each GCC country. Lastly, we also highlight how high resolution LiDAR, UAVs and Google Earth Engine (GEE) data can be used to improve mangrove conservation and restoration planning in the GCC countries and describe current trends and progress in this area.
2 Methods
2.1 Data collection
This systematic review included only peer-reviewed scientific research papers with focus on mangroves in the GCC countries. The Preferred Reporting Items for Systematic Reviews and Meta-analysis statement (PRISMA) (Moher et al., 2009) was adopted for this purpose. The systematic literature review process also followed the population, intervention, comparators, outcomes and study type/design (PICOS) (Badzmierowski et al., 2021). Our review process used three different databases: Google Scholar, Scopus, and Web of Science. The search expression and workflow are presented in Figure 2. We used a combination of Python-based automated literature review and manual review to identify as many relevant articles as possible. Initially, the SerpAPI Google Scholar API (https://serpapi.com/search?engine=google_scholar) in Python was utilized to perform the Google Scholar search. Using this method, we examined the first ten Google Scholar pages of 100 results per page giving a total of 1000 articles. We limited the search to the first 1000 articles in the Google Scholar search after a pre-test screening of the literature search results. This screening showed only five articles were included after reviewing 1000 articles, but none were retrieved through examining 500 more articles (Ewane et al., 2023a). Similarly, in the case of the manual literature review, we examined the first 1000 results in Google Scholar and all the obtained results for Scopus and Web of Science for the publication period from January 2010 through December 2022. Relevant articles were identified by reading the title, abstract, and methods section first and these articles were later verified by reading the entire paper.
The primary list of returned articles - from the combination of automated and manual review - included 807 results, of which 79 were removed after filtering for duplicates. We also excluded non-English articles. Subsequently, we applied a secondary filtering phase, where we excluded multiple versions of blog posts and global articles that were not directly related to the GCC countries. We excluded gray literature from blogs, online newspaper articles, press releases, etc. We included published articles in journals and gray literature from conference proceedings, book chapters and institutional reports. The eligibility criteria for inclusion or exclusion of the searched articles is included under the search criteria in Figure 2. We continued with supplementary searches by identifying relevant articles in the reference list of included articles in a backward and forward snowballing approach (Badzmierowski et al., 2021). The final list included 58 peer-reviewed articles from different journals, conference proceedings, book chapters and institutional reports (Figure 2).
2.2 Data analysis
We extracted information on the country and location of the studies in the GCC region, the journal and year of publications, sensors/platforms, classification approaches, vegetation indices, and mangrove parameters estimated. We developed frequencies for the extracted data, analyzed and presented as maps, figures, and tables. We analyzed the remote sensing data used to derive the vegetation indices to estimate mangrove cover, distribution, and related mangrove forest characteristics to provide an understanding on spatiotemporal dynamics of mangrove ecosystems. We compiled the provided ecosystem services, threats, and ongoing mangrove conservation initiatives to guide future research endeavors and inform policy and practice on mangrove conservation in the region.
The final list of 58 included papers were published across 49 different journals, conference proceedings, book chapters and institutional reports. The five main journals where more than one article was published include Marine Pollution Bulletin (4), Environmental Monitoring and Assessment (3), Arabian Journal of Geosciences (3), Remote Sensing (MDPI) (2), and Journal of Ecosystem and Ecography (2). All the other journals and publication outlets published just one article (See Supplementary Table 1).
3 Results
3.1 Temporal and spatial distribution of the reviewed articles
A clear trend in the number of papers (remote sensing-based) published from 2010 to 2022 was not established. The number of papers published was highly variable over time, with publications peaking in 2022 (17.2%), followed by 2013/2020 (12.1%), and 2016/2021 (8.6%), as shown in Figure 3. The remote sensing-based mangrove papers varied in geographic scale of focus with some global (e.g., Bunting et al., 2022) and regional (e.g., Kumar, 2011) studies assessing mangroves in more than one GCC country. This increased the total number of times that a particular GCC country was mentioned in the 58 articles to 74 times. Most of the remote sensing-based papers focused on mangroves in Saudi Arabia (38.7%), followed by UAE (26.7%), Qatar (16%), Oman (8%), Bahrain (8%) and Kuwait (2.7%) as shown in Figure 4A. Figure 4B shows the proportion of studies we categorized as local, national, regional and global for each GCC country.
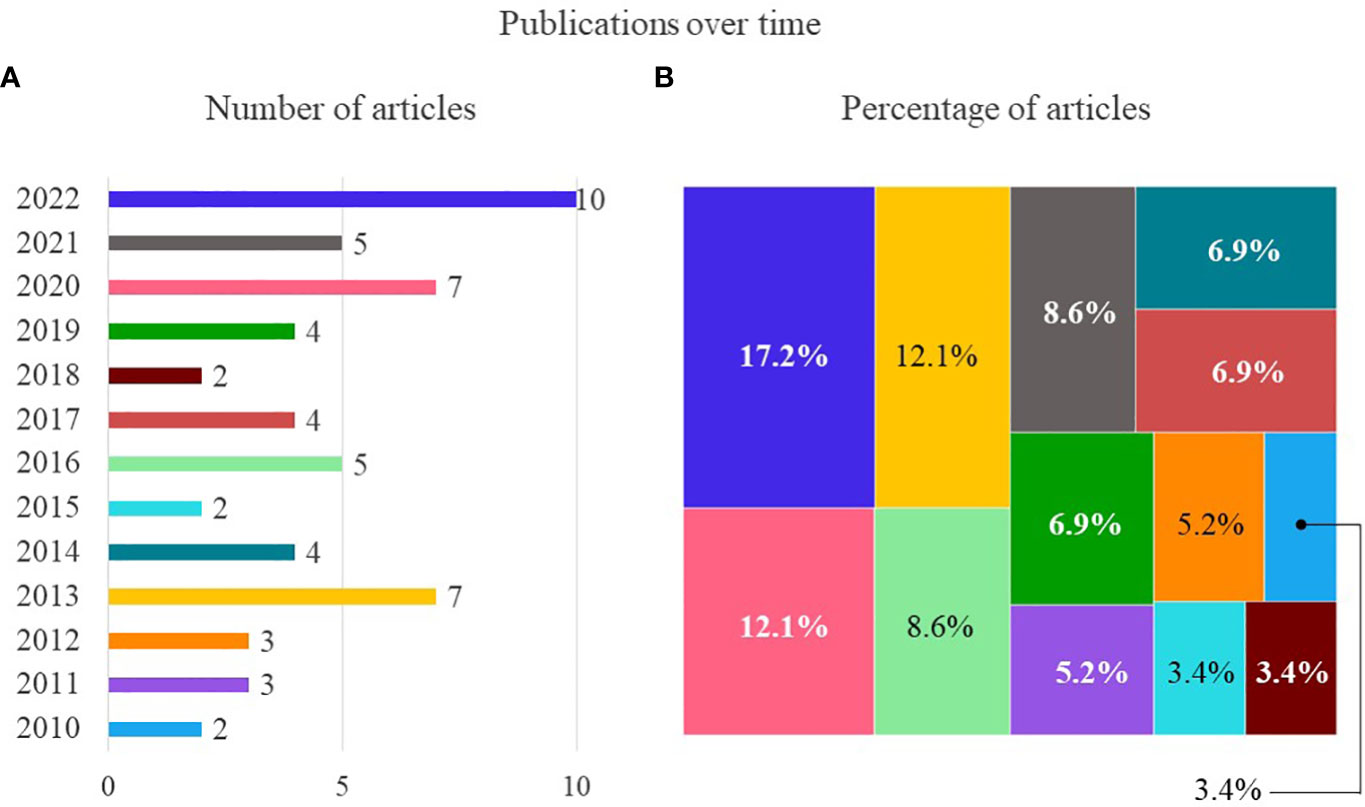
Figure 3 Number (A) and percentage (B) of articles published over time (January 2010 to December 2022).
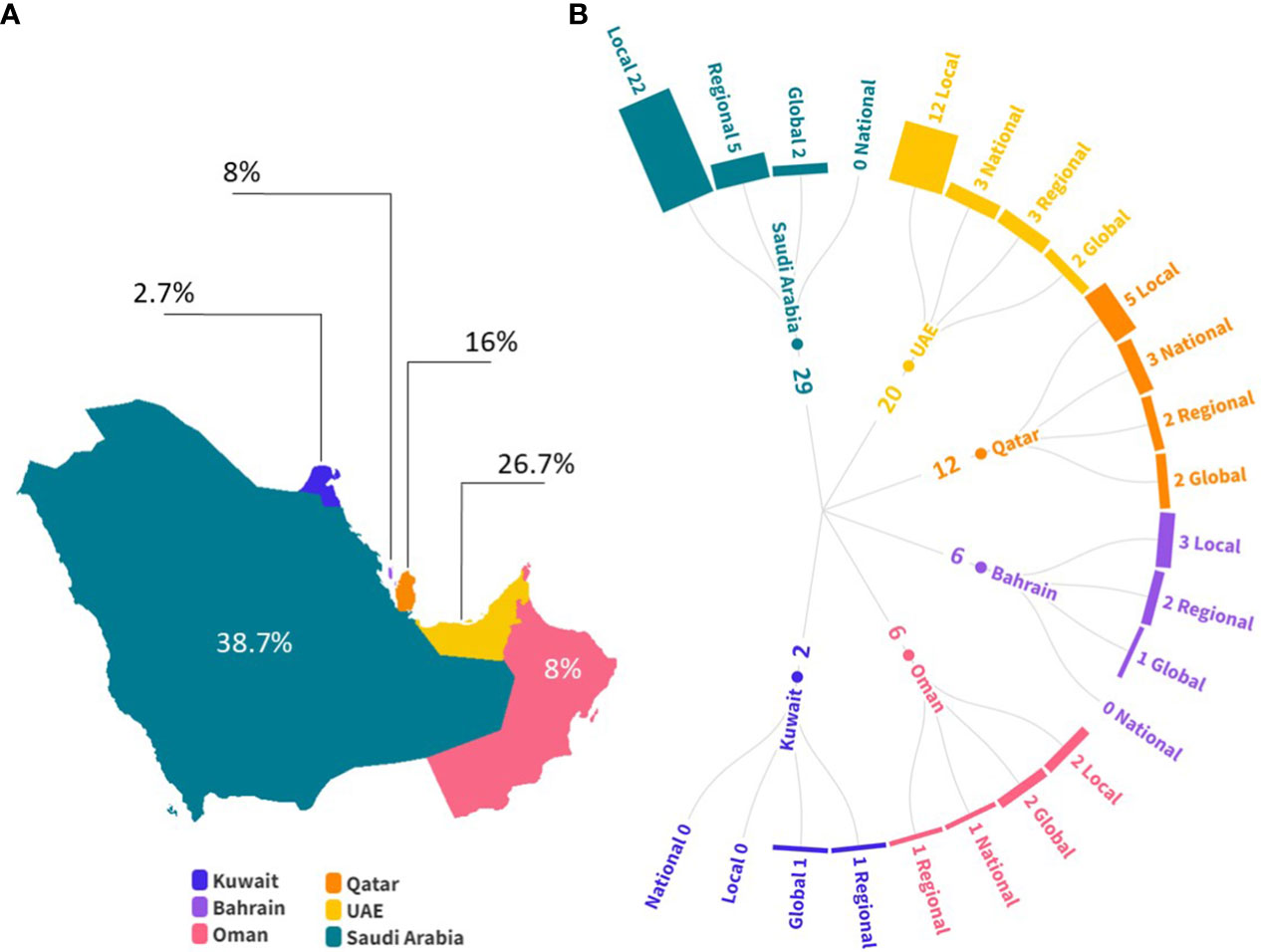
Figure 4 Location and scale of the studies that illustrate (A) percentage of articles that mentioned each GCC country, and (B) number and scale of the articles that included each GCC country. Studies that included countries in multiple continents were considered global, the studies that included multiple neighboring countries were considered regional, and the studies covering more than 70% of a country’s shoreline were considered national. Regional and global studies were taken into account for each country included.
The spatial distribution of the study areas in each paper showed that some locations have multiple overlapping local studies (yellow polygons) while others were only included in the broader scale of national, regional or global studies (white polygons) (Figure 5). It was found that the 12 studies for Saudi Arabia include large extensions over the Red Sea but there are also five studies covering the mangroves over the Arabian Gulf in Al-Khair, Jubail and Tarut Bay. The UAE has ten overlapping local studies that include the mangroves near Abu Dhabi, while only one targeted the mangroves located toward the northern coast. Qatar has four studies and we categorize them as national and the other five studies are in the vicinity of AI-Dhakira, northwest near Khasooma and moving southeast near Hamad Container Terminal Port. For Oman, the studies were at the national level and included the shoreline over the Gulf of Oman and the Arabian Sea. In the case of Bahrain, the local studies overlapped over Muharraq Island and Tubli Bay while Kuwait was only included in the regional and global studies. Since the GMW is a global study and the spatial data is available to the general public, it can be used as a base for regional analysis. The mangrove cover for the GCC countries (excluding Kuwait) determined by Bunting et al. (2022) for the year 2020 is presented as a spatial reference in relation to the study areas.
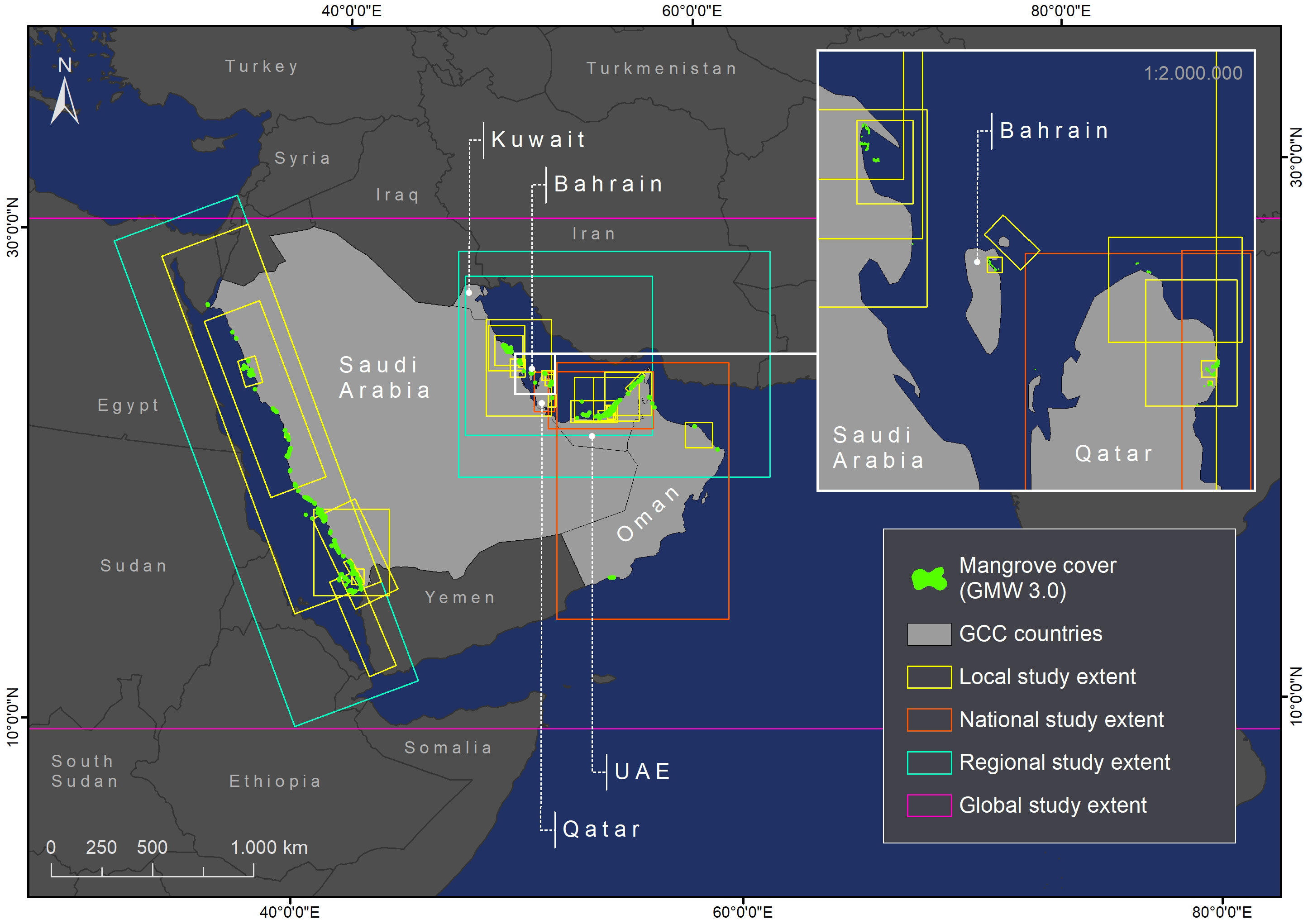
Figure 5 Study area extent for the reviewed papers in relation to the mangrove cover determined by GMW 3.0.
3.2 Measured mangrove characteristics and vegetation indices using remote sensing data
All the reviewed studies measured mangrove cover change (100%) and mangrove distribution mapping (100%) as the main mangrove characteristics using remote sensing data. Studies also included the estimation of mangrove health/greenness (41.4%), biomass (10.3%), height (8.6%), density (8.6%), carbon stock (8.6%), species type (5.2%), restoration assessment (5.2%), sensitivity mapping (5.2%), and chlorophyll content (1.7%). A total of 33.9% of the studies examined more than one parameter, that is, mangrove cover and one or more other mangrove-related parameters (Figure 6).
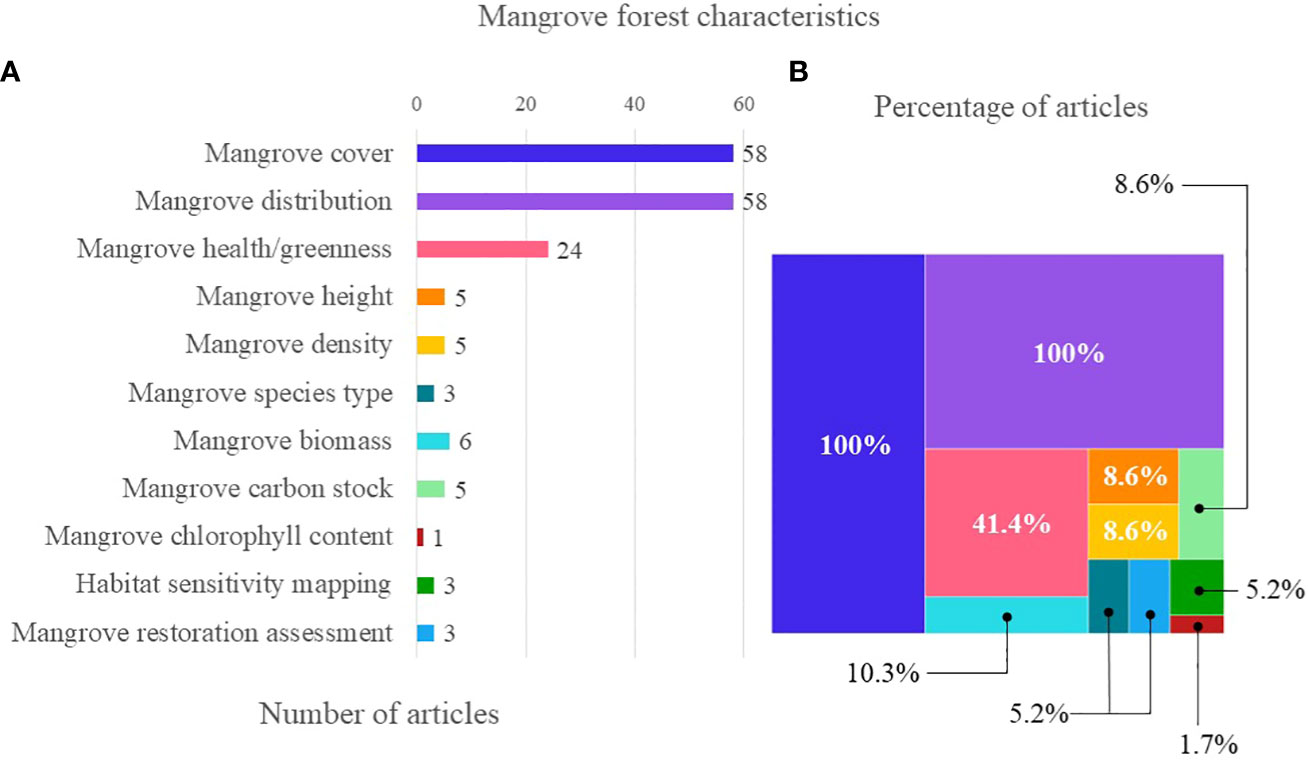
Figure 6 Mangrove forest characteristics estimated using remote sensing data in relation to (A) number and (B) percentage of articles.
The main vegetation index used to classify mangrove forest/tree stands in the reviewed papers was the Normalized Difference Vegetation Index (NDVI) (24 papers, 41%). Some of the reviewed papers used the multi-indices method to detect and classify mangrove cover, distribution and health. Five of the reviewed remote sensing-based papers (8.6%) used NDVI in combination with other vegetation indices such as 1) Leaf Area Index (LAI) and Optimized Soil Adjusted Vegetation Index (OSAVI) to study mangrove health (Arshad et al., 2020); 2) Enhanced Vegetation Index (EVI), Modified Soil-Adjusted Vegetation Index (MSAVI) and Normalized Difference Moisture Index (NDMI) to study mangrove forest degradation and regeneration (Aljahdali et al., 2021); 3) Normalized Difference Built-up Index (NDBI) and Urban Thermal Field Variance Index (UTFVI) to study the effects of coastal development on mangrove ecosystems (Abd El-Hamid et al., 2022b); 4) Modified Normalized Difference Water Index (MNDWI), Normalized Difference Water Index (NDWI) and Ratio Index for Bright Soil (RIBS) for mangrove cover and distribution mapping (Hereher and Al-Awadhi, 2019); and 5) Submerged Mangrove Recognition Index (SMRI) to quantify mangrove changes during tidal inundation (Li et al., 2019) (Figure 7). A total of 34 (59%) out of the 58 reviewed papers did not explicitly indicate that NDVI or other vegetation indices were used for the detection and delineation of mangrove cover.
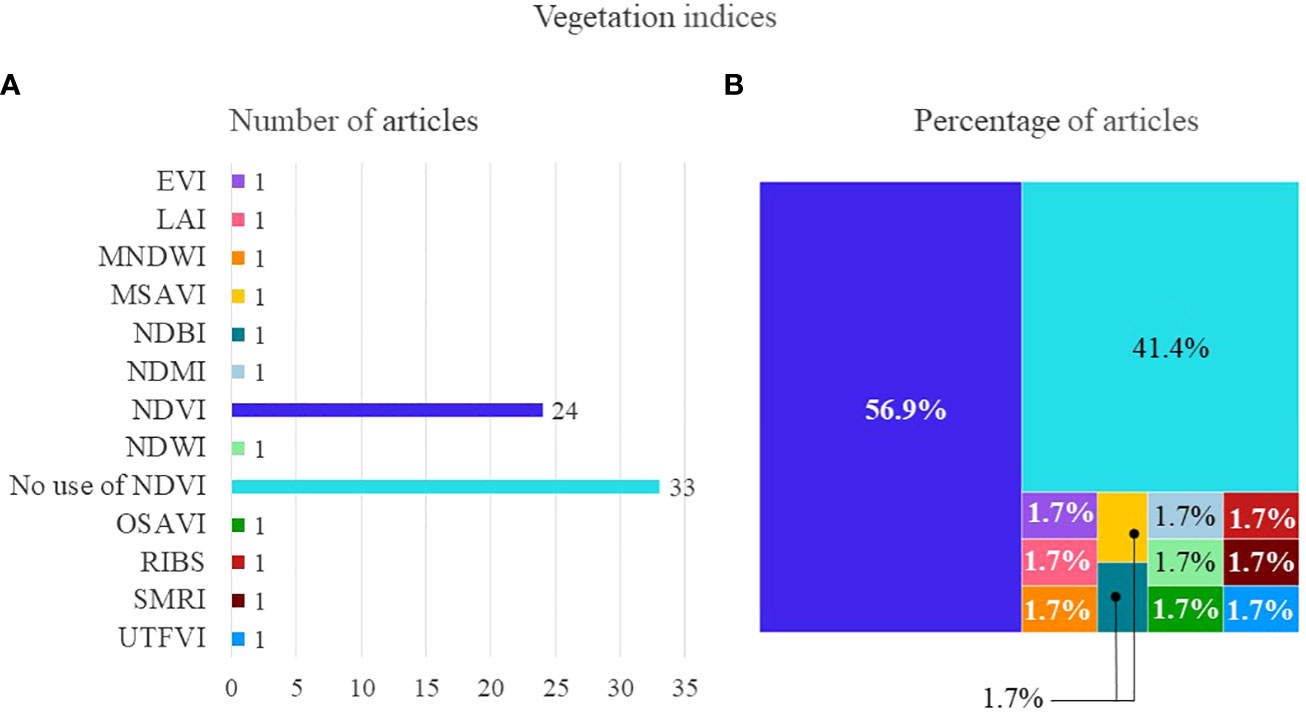
Figure 7 Vegetation indices used for mangrove classifications in the reviewed remote sensing studies focused on GCC region (2010-2022) in relation to (A) number and (B) percentage of articles: NDVI, Normalized Difference Vegetation Index; SMRI, Submerged Mangrove Recognition Index; EVI, Enhanced Vegetation Index; MSAVI, Modified Soil-Adjusted Vegetation Index; NDMI, Normalized Difference Moisture Index; NDBI, Normalized Difference Built-up Index; UTFVI, Urban Thermal Field Variance Index; MNDWI, Modified Normalized Difference Water Index; NDWI, Normalized Difference Water Index; RIBS, Ratio Index for Bright Soil; LAI, Leaf Area Index; OSAVI, Optimized Soil Adjusted Vegetation Index.
The multi-indices approach to map mangrove cover change was important to overcome the challenge of detecting submerged mangroves and differentiating mangrove forests from water during tidal inundation. NDVI and SMRI values showed agreement for predicting mangrove cover and distribution and in the differentiating submerged mangroves from water in tidal flats during conditions of low and high tides (Li et al., 2019). In particular, the SMRI was reported as an effective indicator to detect submerged mangroves in both high and medium spatial resolution WorldView-2 and Landsat satellite images, respectively, over the western Arabian Gulf along the Saudi Arabian coastline. Thus, values of SMRI obtained from high resolution satellite imageries efficiently differentiates spectral signatures of mangrove forests under high and low tides with high accuracy (94%), and is usually preferred for mangrove cover classifications and distribution mapping during tidal inundation (Li et al., 2019; Xia et al., 2018; Xia et al., 2020).
3.3 Overview of remote sensing-based studies data sources
Remote sensing data derived from the instruments onboard Landsat satellites (multispectral scanner (MSS) thematic mapper (TM), enhanced thematic mapper plus (ETM+), operational land imager (OLI), and thermal infrared sensor (TIRS)) were the most widely used to map and monitor mangroves. These data featured in three-quarters (75.9%) of the reviewed papers that measured mangrove forest characteristics such as distribution and cover. This datasource was used solely or in combination with other satellite imageries from IKONOS (12.1%), Sentinel (8.6%), Worldview (8.6%), GeoEye (6.9%), SPOT-5 (6.9%), QuickBird (5.2%), MODIS (5.2%), SAR - JERS-1 SAR, ALOS PALSAR and ALOS-2 PALSAR-2 (3.4%) and IRISS LISS-3 (3.4%). Remote sensing data from Google Earth Pro imagery (10.3%), SRTM/ASTER - DEM imagery (13.8%), aerial photographs/imagery (6.9%), airborne terrestrial LIDAR (5.2%) and UAV imagery (3.4%) were also used. The satellite imageries from Pleiades-1A, PlanetScope, Mapper (ALTM) 3100 EA system, Earth Observing-1 (EO-1), DubaiSat-2 and AVHRR, and JAI AD-080GE multi-spectral 2-channel CCD scan camera imagery from close-range sensing of mangroves are also featured in some studies and presented as Others (12.1%) in Figure 8A.
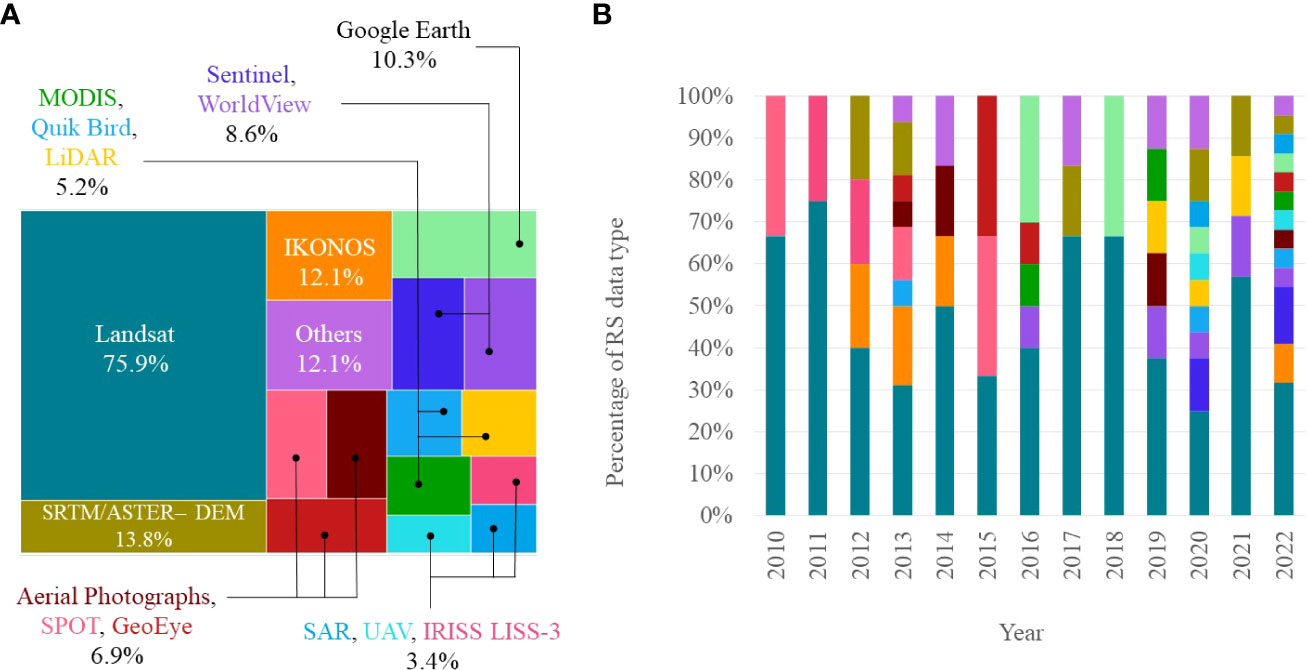
Figure 8 Remote sensing data types used in the reviewed papers as an overall percentage and for each year of the considered timeframe. (A) Remote sensing data type usage (percentage) between January 2010 and December 2022. Percentages do not sum to 100% as more than one remote sensing data source was used in a single reviewed article. (B) Percentage of remote sensing data type used for each year over the time period.
One third (27.9%) of the reviewed papers used data fusion methods, integrating one or two medium-resolution Landsat, Sentinel or SPOT-5 imageries and one or two high-resolution data from Worldview, Quick Bird, aerial photographs and LiDAR. Satellite imagery of various types were documented 82 times in the 58 remote sensing-based mangrove papers, accounting for over three-quarters (75.9%) of the data types. From 2019, high spatial resolution imageries from UAVs and LiDAR and medium to high-resolution remote sensing data from Sentinel, Worldview, Quickbird, PlanetScope, SAR (JERS-1 SAR, ALOS PALSAR and ALOS-2 PALSAR-2) were increasingly used in the remote sensing-based mangrove studies (Figure 8B).
3.4 Classification methods used to detect mangrove forests cover and their accuracies
The reviewed studies used a variety of classification approaches to detect mangrove forest cover and changes over time from the remote sensing imagery. These included manual identification of changes based on visual digitization and interpretation - onscreen vector-based digitization methods (27 studies, 46.6%), machine learning algorithms (15 studies, 25.9%), and statistical modeling techniques (2 studies, 3.4%). A total of 7 studies (12.1%) used supervised and unsupervised classification approaches while the remaining 7 studies (12.1%) did not provide any specific information on the classification approaches used (Figure 9A). The reviewed remote sensing studies mostly used ENVI, ArcGIS and ERDAS for image processing and segmentation. Google Earth Engine was less used, mainly for global and regional studies, using multi-temporal and multi-sensor satellite imageries.
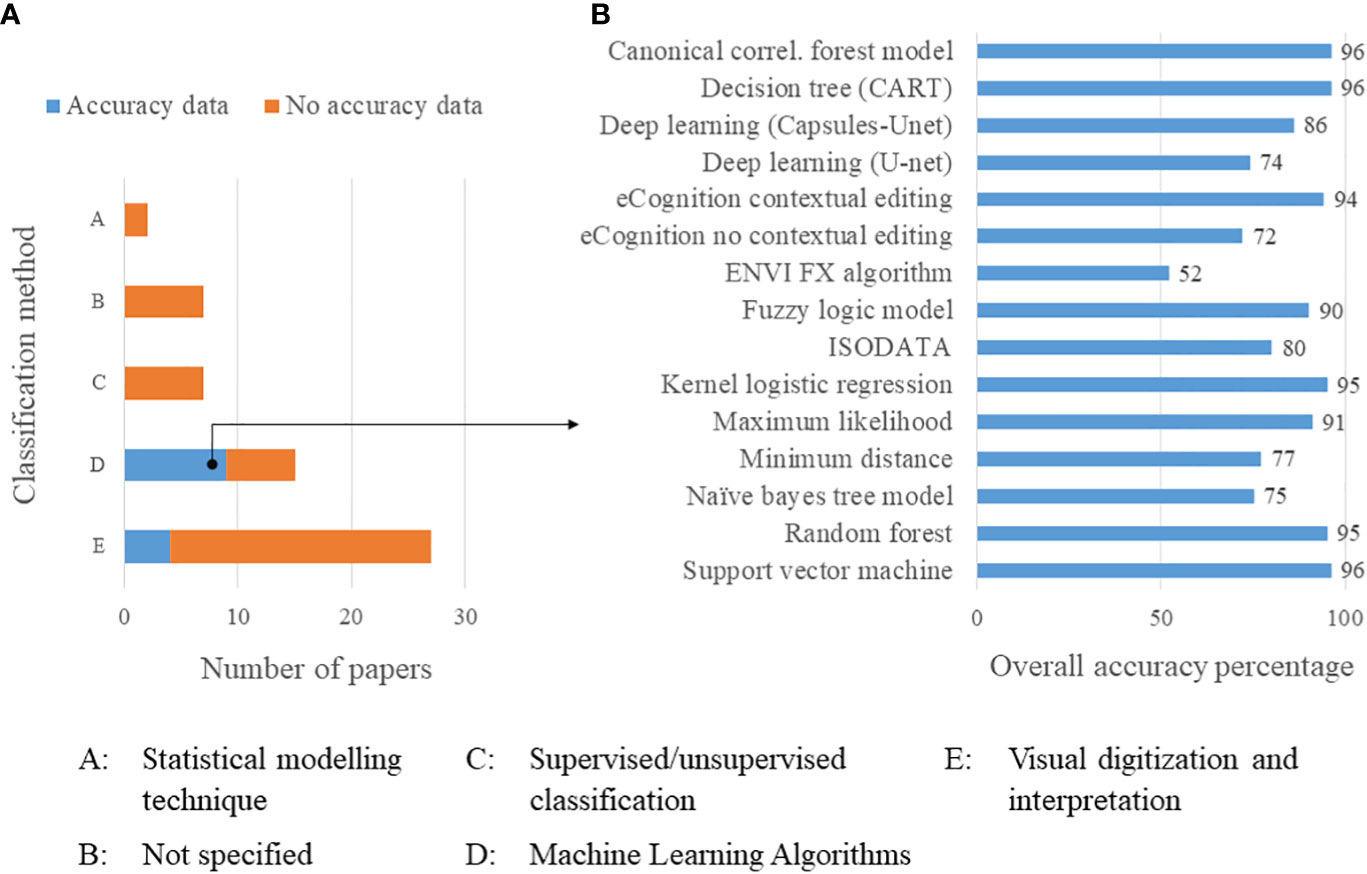
Figure 9 (A) Frequency of papers by mangrove classification methods, further broken down into those that included or did not have accuracy data, and (B) overall accuracy of the various machine learning algorithm (Classification method D) used in the studies.
Data on the accuracy of the classification methods used to detect mangrove cover and distribution in the GCC countries was provided only in 13 (22.4%) of the reviewed studies. The most common machine learning techniques (accuracy given in brackets) used in 9 reviewed studies to detect and classify mangrove forest cover and changes over time included: random forest (95%), super vector machine (96%), decision tree or classification and regression tree (CART) algorithm (>95%), iterative self-organizing data analysis technique algorithm (ISODATA) (80%) and maximum likelihood (91%). Others included the canonical correlation forest models (96.2%), kernel logistic regression (95%), eCognition with contextual editing (94%), fuzzy logic model (90%) and deep learning (Capsules-Unet) (86%). Minimum distance (77%), naive bayes tree model (75%), deep learning (U-net) (74%), eCognition no contextual editing (72%) and ENVI FX algorithm (52%) classifiers were less accurate (Figure 9B). The 4 reviewed studies that used visual digitisation and interpretation techniques also reported classification accuracies of greater than 90%. Overall, the classification accuracies and estimation of vegetation greenness and health, based on NDVI, was relatively invariant to the classification method and remote sensing data used.
The specific classification method and accuracies used in the detection of mangrove forest cover were described more in the reviewed studies that used machine learning algorithm techniques than those that used visual digitisation and interpretation and other classification techniques. Details of the classification methods and accuracies of the models used to predict key attributes are provided in Supplementary Tables 2, 3.
4 Discussion
4.1 Contribution of remote sensing studies
For mangrove studies based in GCC countries over the past 30 years, satellite remote sensing data sources were particularly useful in determining mangrove cover, distribution, density, tree height, biomass and carbon stock, and potential areas for future afforestation initiatives (Elsebaie et al., 2013; Monsef et al., 2013; Alwhibi, 2017; Blanco-Sacristán et al., 2022). The majority of the studies used medium-resolution Landsat imageries from MSS, TM, ETM, ETM+, OLI and TIRS instruments/sensors solely or in combination with high-resolution satellite data from WorldView, QuickBird, Sentinel, Ikonos and SPOT to study the spatiotemporal dynamics of mangrove cover and distribution in the GCC countries. Data fusion methods involving the combination and integration of satellite imageries, LiDAR, and UAV (for ground truthing) images provided significant progress in efficiently and accurately detecting and mapping mangrove habitat and their sensitivity to natural and anthropogenic stressors (Butler et al., 2020; Jiang et al., 2022).
Estimates of mangrove cover and distribution for the GCC countries showed an appreciable decrease in dense mangrove cover, particularly post-2007, except for Qatar and the UAE (Bunting et al., 2022). For example, Bahrain and Saudi Arabia lost about 25.9% (21 ha) and 30.6% (3,396 ha) of their natural mangrove cover from 2007 to 2020, respectively. Similarly, Oman lost 14.3% (24 ha) of natural mangroves from 1996 to 2020 (Bunting et al., 2022). This was mainly due to the growth of urban development activities related to coastal infrastructure and industrial expansion (Milani, 2018; Arshad et al., 2020; Aljenaid et al., 2022; AlQahtany et al., 2022), in addition to climate change impacts (Al-Naimi et al., 2016; Almahasheer, 2018; Blanco-Sacristán et al., 2022). However, some studies reported stable to slight increases in mangrove cover over the same period in the GCC countries (Almahasheer et al., 2016; Almahasheer, 2018; Milani, 2018). This was particularly evident by appreciable increases and relatively stable mangrove cover in the post-2000s in Qatar and UAE, respectively, where government efforts toward mangrove reforestation and afforestation projects had likely played a significant role in increasing mangrove cover post-2000. This is compared to pre-2000 records when most loss in mangrove extent generally occurred (Almahasheer et al., 2016; Alsumaiti, 2017; Almahasheer, 2018; Bunting et al., 2022).
The limitations of the remote sensing-based mangrove studies that were identified mainly focused on disparities in mangrove distribution and cover data within each GCC country. The observed disparity in the statistics in mangrove distribution and cover change (loss/decrease or gain/increase) was attributed to the differences in mapping methods, classification approaches, and spatial resolution of the satellite remotely sensed data used between different studies (See Supplementary Table 2). For example, the remote sensing method and data (JERS-1 SAR, ALOS PALSAR and ALOS-2 PALSAR-2) used in the GMW (Bunting et al., 2022) were different from most of the other studies, where Landsat and WorldView, QuickBird, Sentinel, Ikonos and SPOT satellite imageries were mainly used (See Supplementary Table 3). Guo et al. (2021) found that factors such as the spatial resolution of the remote sensing images, tidal inundation, and information extraction strategy can account for inconsistencies in mangrove cover estimates.
The classification methods used in the various remote sensing studies in the GCC countries vary widely - for instance, supervised/unsupervised, visual interpretation by experts and/or machine learning algorithms - and the scope of the studies can range from global to local. The scale of a study influences some of the decisions regarding sources of remote sensing data and the classification approach used. For example, the scope of the study, such as the question the mapping is intended to address, will also modify the type of data and classification approach used. As a result, studies could use high temporal resolution data to track dynamics, versus low temporal resolution data to quantify changes at specific time steps such as pre- and post-restoration. Global scale studies such as the World Atlas of Mangroves (WAM-1, WAM-2), the Global Distribution of Mangroves (GDM) and the GMW rely on the use of larger scale mostly lower temporal resolution remote sensing data. Local scale studies mostly used a combination of high and low temporal resolution remote sensing and field data, with less use of LiDAR and UAV data.
4.2 Major findings
Mangrove ecosystems in the GCC countries provide diverse ecosystem services that are of critical importance to the ecological, social and economic well-being of the region (Vaughan et al., 2019). However, the main mangrove ecosystem service that was reported to be directly measured using remote sensing data sources was carbon sequestration and storage (Al-Nadabi and Sulaiman, 2021; Abd El-Hamid et al., 2022a; Aljenaid et al., 2022). Globally, mangroves are recognized as an important asset for blue carbon sequestration (Friess et al., 2019), thus its conservation provides optimism for climate change mitigation and adaptation efforts (Friess et al., 2020). The reviewed studies did not explicitly use remote sensing data or technology to investigate other important benefits of mangrove ecosystems to the environment and society of the GCC countries.
Despite the well-known importance of mangroves in the GCC region, these highly complex, vulnerable and fragile forest ecosystems are facing a wide variety of threats, including stressors driven by climate change and anthropogenic land use change activities (Burt, 2014; Al-Naimi et al., 2016; Almahasheer, 2018; Blanco-Sacristán et al., 2022). Specific threats to mangrove ecosystems are important to understand and can be identified through the use of remotely sensed data. In particular, climate change-induced sea-level rise, erosion and flooding, changes in precipitation, increases in temperature and salinity, and decreases in dissolved oxygen in submerged mangrove aerial roots are expected to significantly increase mortality of mangrove trees in the GCC countries (Al-Khayat and Balakrishnan, 2014; Al-Naimi et al., 2016; Almahasheer, 2018; Samara et al., 2020; Blanco-Sacristán et al., 2022; Subraelu et al., 2022). Rapid urbanization, land reclamation and dredging operations for infrastructural and industrial development and sewage disposal constitute important threats to the sustainability of mangrove ecosystems (Al-Naimi et al., 2016; Almahasheer, 2018; Milani, 2018; Aljenaid et al., 2022; Blanco-Sacristán et al., 2022).
Increased timber exploitation, camel grazing associated with local livelihood sustenance activities, and unsustainable tourism development are also threatening the conditions of mangroves in the GCC countries (Kumar et al., 2010; Al-Ali et al., 2015; Alsumaiti, 2017; Alwhibi, 2017; Milani, 2018). A lack of awareness about the importance of mangroves among local people was reported to have negatively impacted the conservation of mangrove ecosystems in Saudi Arabia (Al-Ali et al., 2015; Alwhibi, 2017). However, anthropogenic pressures are not always considered entirely negative for mangrove ecosystem health. Evidence suggested that treated sewage could enhance mangrove growth due to its low salinity and high nutrient concentrations. Similarly, dredging of channels, particularly in lagoon areas characterized by hypersalinity/extreme temperatures, can result in increased mixing with less extreme coastal waters, enhancing mangrove growth (Burt et al., 2021).
Most of the reviewed studies used open source (free access) and time series Landsat imageries to map mangrove distribution and cover. The low spatial (30 m) and temporal (16 days) resolutions of Landsat imageries limited the ability to extract detailed tree- and forest-level characteristics, which are important for monitoring biomass and estimating carbon sequestration. Only a few of the reviewed studies used moderate to high spatial (10 m or less) and temporal (4 days or less) resolution remote sensing data to study mangrove ecosystems in the GCC region. High spatial resolution sensors offer the opportunity to obtain more detailed ecological information of forest ecosystems and are increasingly being used for monitoring mangroves although they can be costly (Goldblatt et al., 2017; Psomiadis et al., 2017; Xian et al., 2019).
Field-based measurements of mangrove forest characteristics are usually expensive, time-consuming and difficult to undertake due to the challenging physical conditions and remoteness of mangrove habitats. As an alternative to field-based assessments, LiDAR data were used in three of the reviewed studies to measure mangrove aboveground biomass (AGB) and tree height inventory in UAE (Alsumaiti et al., 2019), and for benthic habitat (including mangrove forests) mapping and benthic habitat sensitivity analysis of mangrove forests in Qatar (Butler et al., 2020). The inclusion of LiDAR data improved the accuracy and efficiency of mangrove detection when compared to pixel-based classifiers alone (Butler et al., 2021). A high-resolution centimeter-scale UAV-mounted MicaSense RedEdge-MX sensor was used for validating data from satellite imageries in Saudi Arabia (Jiang et al., 2022) and to obtain georeferenced photographs of mangroves for interpretation in UAE (Mateos-Molina et al., 2020). UAVs allow the collection of high-resolution, time series imagery over small spatial scales, with the advantages of lowering costs and the risks of human involvement, which can be considerable in mangrove ecosystems. Such UAV-derived high-resolution data improves the accuracy of mangrove forest classification and the estimation of tree-level growth characteristics, and early detection of any ecological changes (Meyer et al., 2019; Ewane et al., 2023b).
4.3 Integration of UAVs in mangrove forests monitoring and assessment
Satellite imagery has been the primary resource used to map mangrove forests in GCC countries. In contrast, UAVs are yet to be widely used due to issues related to accessibility, local laws and regulations, logistics, privacy and ethics in GCC countries. Despite the technical and logistic limitations associated with UAV-based endeavors, there is a potential for greater use of UAVs in forest resource management schemes, as the use of UAVs is becoming increasingly cost-competitive to bridge the data limitation gap between field measurements and satellite remote sensing (Ewane et al., 2023b). High-resolution UAV imagery provides detailed data with high accuracy that can be sufficient to validate satellite data. As UAVs can collect a wide array of useful data including LiDAR, multi and hyperspectral, infrared, accelerometers, pressure gauges and temperature sensors (Nitoslawski et al., 2021), they can be used for high-accuracy mangrove mapping and present opportunities for monitoring, evaluation, and reporting (Jiang et al., 2022).
UAVs have been used to monitor mangrove ecosystems and provide detailed tree-level information over small to large areas in a fast, repeatable, cost-effective, and accurate way (Ruwaimana et al., 2018; Castellanos-Galindo et al., 2019; Jones et al., 2020; Navarro et al., 2020). This highly detailed tree-level information of mangrove ecosystems can be integrated as a complementary data source to cover low data frequency gap from field surveys. UAV based imagery makes it possible to map mangrove cover with higher accuracy than satellite-based imagery since the latter has been found to overestimate mangrove cover in semi-arid dwarf mangroves in Mexico (Hsu et al., 2020). UAVs provide one of the best remote sensing options to rapidly acquire accurate monitoring data to evaluate progress and validate satellite data to support mangrove restoration and conservation initiatives for increased carbon sequestration.
UAVs offer the best option to monitor and assess mangrove ecosystems in GCC countries since mangrove extent are relatively small and are mostly discontinuous in their distribution. Because the operational costs of using UAVs reduces over time, its use becomes advantageous over satellite data and field surveys for long-term monitoring over small to large areas (Ewane et al., 2023b). Although the application of UAVs in mangrove monitoring and assessment is highly feasible in the GCC countries, their deployment does require in-depth knowledge of the regulations governing their use and required authorisations in the various GCC countries. In addition, the processing time for UAV imageries can be ten times greater than that of satellite images due to the larger data size of UAV images particularly when revisit times are very frequent (Ruwaimana et al., 2018).
4.4 UAV-based rules and regulations initiatives in the GCC countries
The rules and regulations for the use of UAVs are generally strict in the GCC countries, and differ in their requirements among the countries. In particular, the use of UAVs in most of the GCC countries (Bahrain, Oman, Saudi Arabia, Qatar and UAE) is subject to a prior acquisition of an authorization or permit. In Qatar, UAE and Oman, obtaining this permit can be quite challenging and time consuming (OZYRPAS, 2022; UAV Coach, 2023).
In the UAE, for instance, a two-step application process must be followed. Firstly, the operator (person or group) needs to register for, and obtain, a drone operator’s license which usually takes from one to three weeks. Secondly, an application for a permit to fly over a specific area is required in order to be able to capture images, and this permit is only valid for 14 days (General Civil Aviation Authority - GCAA of UAE, 2023). Even after gaining the permit, there may still be specific rules that surround the flight. These include awareness of safety measures such as - not flying UAVs in the vicinity of people, airports, or governmental facilities, - flying UAVs only in daylight and good weather - and limiting flight height to a maximum of 120 meters, among other practices (General Civil Aviation Authority - GCAA of UAE, 2023; UAV Systems International, 2023). These restrictions and time delays also apply for research-related flight permits.
Similarly in Bahrain, the UAV type needs to be specified based on its weight in order to register at Bahrain Civil Aviation Affairs (BCAA) where the submission should take place prior to its importation by the owner or the intended owner (Ministry of Transportation and Telecommunication, 2023). The user/owner may be requested to prove a certain level of skills and proficiency to the authority to fly UAVs.
In Saudi Arabia, the government has launched an awareness and outreach initiative to facilitate the registration and issuing of licenses to operate UAVs. The initiative will also promote the issuance of operating licenses to practitioners by the General Authority of Civil Aviation (GACA) as a fundamental prerequisite for their activities. This is part of the country’s efforts to develop a more efficient and organized drone operation in line with Saudi Arabia’s Vision 2030 (Drone Laws, 2022). The application and status tracking is fully online via the governmental website (https://eaviation.gaca.gov.sa/uas/login.xvw).
In Kuwait, the user only needs a permit if the UAV is being used for commercial purposes (Markert, 2020; UAV Systems International, 2023), and it must also have insurance and registration (Markert, 2020). Once the permit is obtained, there are restrictions regarding proximity to crowds or airports and only daylight flights are allowed (Markert, 2020; UAV Systems International, 2023).
4.5 Importance of mangroves
The reviewed studies implicitly and explicitly evaluated and documented five important mangrove ecosystem services, which included habitat for fish and fauna species, carbon storage and sequestration, coastal protection, provision of livelihood opportunities, and water purification and filtration closely related to nutrient cycling (Figure 10). Based on the reviewed remote sensing studies, mangroves in the GCC countries filter and trap sediments that further improve anchorage to their roots (Butler et al., 2020), and sequester and store high quantities of carbon in soil and trees (Alwhibi, 2017; Butler et al., 2020; Aljenaid et al., 2022; Blanco-Sacristán et al., 2022). They filter pollutants and purify water (Al-Naimi et al., 2016; Alwhibi, 2017; Alsumaiti et al., 2019), and help protect coastal shorelines from tsunamis, tropical cyclones, storm surges, erosion and flooding (Elmahdy and Mohamed, 2013; Al-Ali et al., 2015; Alwhibi, 2017; Milani, 2018; Subraelu et al., 2022). In addition, mangrove ecosystems provide habitats for diverse fisheries and fauna species (Alwhibi, 2017; Milani, 2018; Mateos-Molina et al., 2020; Elmahdy and Ali, 2022). Mangrove ecosystems are important recreation areas for tourists (Alsumaiti, 2017). Their leaves, stems and roots are important medicinal resources and fodder for domestic animals (Alwhibi, 2017). Lastly, mangrove trees also offer livelihood benefits to some communities such as seafood, fuelwood, timber and wood for housing materials (Al-Ali et al., 2015; Alsumaiti, 2017; Alwhibi, 2017).
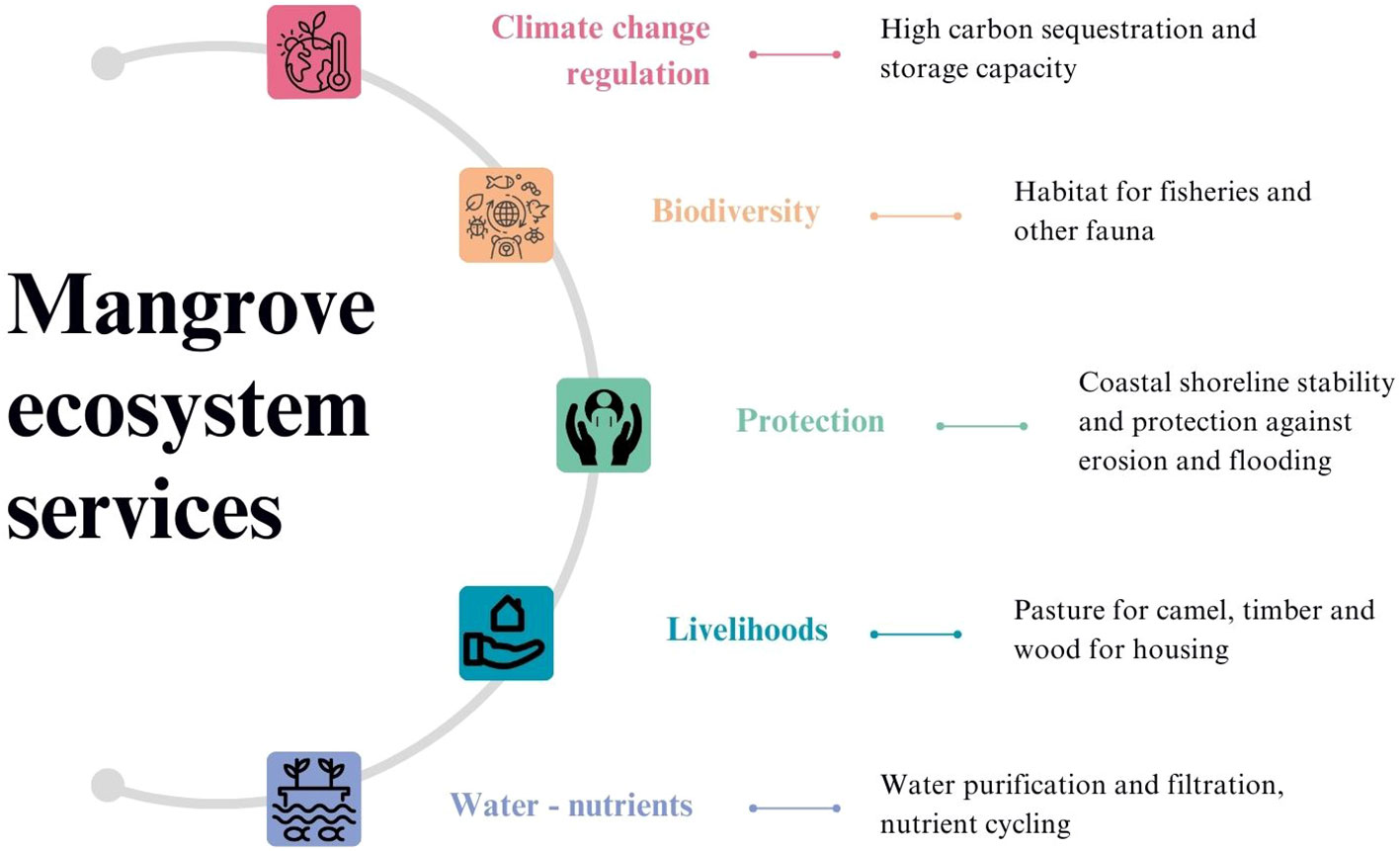
Figure 10 Important ecosystem services of mangroves in the GCC countries identified in the reviewed papers.
Mangrove carbon stocks and sequestration capacity was estimated using remote sensing data in previous studies. For example, an average above-ground carbon sequestration of 6.3 Mg C/m2 was estimated using NDVI derived from above ground biomass using Landsat OLI imagery. Higher above ground and below ground biomass and carbon stocks were reported in landward than seaward areas (Al-Nadabi and Sulaiman, 2021). Mangrove forests of about 328 ha and 48 ha were estimated to store up to 34,932 Mg C ha−1 and 5,112 Mg C ha−1 of carbon, respectively, in 1967 and 2020, with the decrease due to land reclamation associated with coastal development. This was estimated using multi-sensor high resolution satellite images from Worldview-3, Worldview-2, IKONOS, and QuickBird, coupled with true-color orthorectified aerial photographs, and GIS-based spatial analysis of mangroves in Bahrain from 1967 to 2020 (Aljenaid et al., 2022). Using the Carbon Sequestration Storage Model in the InVEST software program, above ground biomass estimated using NDVI from moderate resolution Landsat TM, ETM and OLI stored a total carbon stock of 3,772,968 Mg C along 13,500 km2 of the Jazan biome coastline in Saudi Arabia partly covered by mangroves in 2021 (Abd El-Hamid et al., 2022a).
4.6 Major threats to mangroves
Mangrove forest ecosystems in the GCC countries are concurrently stressed by the combination of five natural and anthropogenic threats/pressures, which include coastal industrial and infrastructural development, pollution, climate change, erosion and flooding, and unsustainable extraction of timber/wood (Figure 11). Mangrove loss associated with rapid urban development activities such as development of crude oil refinery and petroleum industries, road and housing, seaports and harbors infrastructures are by far the primary threats to mangrove forest ecosystems in all GCC countries (Al-Khayat and Balakrishnan, 2014; Arshad et al., 2020; Abd El-Hamid et al., 2022b). The loss in mangrove cover reduces its carbon stock and sequestration and storage capacity due to a reduction in above ground biomass and loss of sediments (Al-Nadabi and Sulaiman, 2021; Aljenaid et al., 2022; Abd El-Hamid et al., 2022a).
Pollution due to oil spills from pipeline leakage and heavy metal are threatening mangrove ecosystem health and species survival in the GCC (Aljamali et al., 2014; Al-Khayat and Balakrishnan, 2014; Al-Ali et al., 2015; Warren et al., 2016). Mangrove ecosystems are also threatened by climate change-driven sea level rise and associated flash flooding and inundation risks and coastal shoreline erosion (Aljamali et al., 2014; Hereher, 2016; Alsumaiti, 2017; Subraelu et al., 2022). Sea-level rise driven flooding and inundation is expected to submerge and destroy mangrove trees and modify the supply of propagules as mangroves reach a maximum of 6 m tall (Al-Khayat and Balakrishnan, 2014; Al-Awadhi et al., 2020). Climate change driven rising temperatures and excessive evaporation causes droughts and hypersalinity in mangrove lagoons. Uncontrolled cattle grazing by camels and wood exploitation are also important threats to mangroves in Saudi Arabia (Alwhibi, 2017).
4.7 Some examples of hotspots of mangrove loss in the GCC countries
Spatial and temporal changes that characterize loss and gain in mangrove forest cover in the GCC countries are provided in Figure 1 and specific locations of high mangrove cover loss and gain are provided in Supplementary Tables 2, 3. In Bahrain hotspots of mangrove loss were detected mainly in Tubli bay in the northeast region at locations in Ras Tubli, Ras Sanad and Sitra Island, where mangrove cover decreased from 170.78 ha to 4.17 ha, 96.75 ha to 33.90 ha and 60.47 ha to 9.93 ha, respectively, from 1967 to 2020. In total, mangrove cover decreased from 328 ha to 48 ha in the three locations from 1967 to 2020, with these changes derived from aerial photographs and multi-sensor satellite imagery (Aljenaid et al., 2022).
In Qatar hotspots of mangrove loss were detected in six locations, including planted mangrove areas at Al Mafjar and Fuwairit in the north, natural mangrove areas at Al Thakira and Al Khor, and planted mangroves at Simaisma in the east, and planted mangroves at Zekreet in the west using Landsat imagery. Zekreet is the main hotspot of declining and stunted plantations of A.marina with just 1.11 ha remaining, with losses caused by climate change-driven rising seawater temperature, hyper salinity and lowered dissolved oxygen while Al Thakira (65.2%) and Al Khor (38.8%) coast boost luxuriant stands of natural mangroves (Al-Khayat and Balakrishnan, 2014).
In Saudi Arabia hotspots of mangrove loss were originally detected at locations in the Tarut Bay in the eastern province (Almahasheer, 2018) and along the Jazan shoreline in the Red Sea (Abd El-Hamid et al., 2022b) of Saudi Arabia. Mangroves in these areas were exposed to many environmental and human pressures such as urban encroachment, pollutants, and land reclamation (Almahasheer, 2018).
In the UAE hotspots of mangrove loss were detected in locations in Umm AnNar and Mosaffah, Khor Ra’s al-Khaimah mangal and Khor Kalba communities due to urban development in the coastal shoreline of Abu Dhabi (Almahasheer, 2018). Mangroves decreased slightly at locations in Khor Kalba natural reserve, RAK natural reserve, Umm Al Quwain estuarine, Ajman-Hammriah and Ras Al Khor in Dubai, from 1990 to 2019, especially in the RAK and Umm Al Quwain areas facing the Arabian Gulf (Elmahdy et al., 2020).
Kuwait does not have any natural mangrove forests (FAO, 2005), while planted mangrove areas of about 0.58 km2 were detected at 29° N in 2017 (Almahasheer, 2018; Guo et al., 2021). It is thought that mangroves in Kuwait were completely destroyed during the Kuwait war. There is increasing afforestation of mangroves from nursery reared propagules of A. marina that were successfully transplanted in the artificial islands of Sabah Al-Ahmad Sea City of Kuwait (Loughland et al., 2020).
4.8 Ongoing mangrove conservation initiatives
Most governments in the GCC countries have implemented coastal environmental management and planning initiatives that include mangrove protection and restoration (Milani, 2018). Restoration of A. marina along the coast of Al-Sharifa Island, Al-lith Red Sea in Saudi Arabia was carried out in the mid-2000s, and these mangrove saplings had a 39% survival rate (Chithambaran, 2019). Mangrove conservation has been studied in Oman (Al-Afifi, 2018). Since 2000, Oman has partnered with the government of Japan to work on conservation and restoration of mangroves, planting saplings in 7 of the 11 governorates (UNEP, 2018). In 2022, “the Environment Authority of Oman launched an action plan to plant 1,500,000 mangrove seeds in Khor Ghawi in the Wilayat of Al Jazir and the Wetland Reserve in the Wilayat of Mahout in the Al Wusta Governorate during the months of July and August of 2022, as part of the national initiative to plant 10 million trees (EA, 2022).” Also, in the UAE, 12% of the country’s marine and coastal areas are designated as protected areas, and the government is continuing to expand marine protected areas to conserve important habitats such as that of mangroves (Lamine et al., 2020). Recent efforts are underway in the UAE to restore marine ecosystems, and the Aquaculture and Marine Studies (AMSC) has planted 5,026 hectares of A. marina in Abu Dhabi Emirate (Yosef et al., 2022). Research is underway in the UAE to utilize treated sewage effluent as a water-source to enhance the growth of mangrove seedlings in nurseries (Erftemeijer et al., 2021). This research was linked to the growing evidence that treated sewage enhances mangrove growth due to the lower salinity and the high nutrient concentration of this media (Burt et al., 2021).
There are a few current and ongoing conservation initiatives in the GCC region. Saudi Arabia plans to plant over 300 million mangrove trees by 2030 (Al-Sinan et al., 2023). The UAE has prioritized the importance of mangrove conservation and preservation, citing it as a defining and important characteristic of their ecosystems during public events. On July 26th, 2022, the UAE Pavilion celebrated “International Day for Conservation of Mangrove Ecosystems” at the Floriade expo 2022 by highlighting mangroves in their exhibition (Ismail and Alghoul, 2022). An alliance between the UAE and Indonesia was announced at United Nations Climate Change Conference or Conference of the Parties (COP27) of the UNFCCC in 2022 in Egypt, in which the two nations plan to accelerate mangrove restoration and support mangrove conservation globally (Ministry of Climate Change and Environment, 2022; The National, 2022). Also in 2020, 1,000 mangrove saplings were planted in the Al Wusta governorate of Oman (Oman Observer, 2020; Times News Service, 2022).
Overall, there is a lack of literature on community-based mangrove conservation and restoration initiatives in the GCC countries. The increases in mangrove cover that have occurred post-2000, for example, in UAE and Qatar are attributable to government efforts toward promoting mangrove plantation reforestation and afforestation projects for improved and sustainable coastal environmental management and planning. This played a significant role in increasing mangrove cover over this century, compared to pre-2000 records when much of the loss in mangrove extent generally occurred (Almahasheer et al., 2016; Alsumaiti, 2017; Almahasheer, 2018; Bunting et al., 2022).
Kuwait engaged in the planting of A. marina in the artificial islands of Sabah Al- Ahmad Sea City along the southern coast of Kuwait over the last two decades and reports suggest that the germination of the nursery reared propagules and transplantation of the mangrove seedlings was successful during 2006-2008. A decade after transplantation, successful seed production and self-germination indicated establishment of a sustainable A. marina population, which enhances biodiversity and offers valuable ecosystem goods and services (Loughland et al., 2020).
4.9 Mangrove conservation in relation to United Nations Sustainable Development Goals (UN SDGs)
Mangrove forests in the GCC countries, though comparatively smaller in spatial extent and shorter in height (mostly less than 6 meters tall), provide a range of environmental, social and economic benefits in the form of ecosystem services (Figure 10). For example, mangrove ecosystems foster rich biodiversity and nurturing flora and fauna of diverse microorganisms indispensable for basic chemical and biological processes. These benefits, including carbon sequestration, water purification, nutrient cycling, erosion and flooding control are critical to enhancing the region’s effort toward achieving progress with several of the UN Sustainable Development Goals (SDGs), particularly related to climate action (SDG 13), life below water (SDG 14) and life on land (SDG15). Considering that the GCC countries are among the highest carbon emitters per capita from oil and gas industries and the mangrove cover has generally declined over the past three decades, expanding mangrove conservation and restoration initiatives is very important for the well-being of the environment and society.
Mangrove restoration can be enhanced with the use of specifically designed UAVs through direct seeding to expand mangrove cover as recently demonstrated in the UAE (Mohan et al., 2021; ADNOC Uses Innovative Drone Technology to Plant Mangroves, 2023). Mangroves will play a key role (as the only evergreen forest and the most important carbon sink in this arid region) in achieving the recent climate change mitigation initiative in the UAE (Net Zero 2050 and COP-28) (ADNOC Uses Innovative Drone Technology to Plant Mangroves, 2023). Such mangrove restoration initiatives are invaluable for the GCC countries to increase mangrove carbon sequestration for climate change mitigation, and storm, flooding, erosion, and marine habitat and biodiversity protection. This is important for achieving sustainable development and sustainability in coastal environmental management in the region.
4.10 Existing research gaps
This review of remote sensing-based studies enabled the identification of considerable research, management and policy gaps on remote sensing-based mangrove data collection, use and interpretation, and data management and availability to the public. In particular, these largely focus on the use and integration of LiDAR and UAV data, and the application of emerging classification approaches that rely on machine learning and artificial intelligence.
● There is a need to explore further the integration of multiple remote sensing data sources with different resolutions and scales. This should include the integration of high-resolution long-term data from satellite sources and large scale LiDAR and UAV data that is frequently acquired. Adoption of this data fusion approach will improve accuracy and efficiency for long-term area-based monitoring of mangrove forests over large spatial scales and tree-level characteristics over small spatial scales.
● Similarly, generation of more remotely sensed data from LiDAR and UAVs and development of new applications that can use small-scale data from this platform and data source is required. These data should be compiled into a user-friendly interface that incorporates rigorous quality assurance and quality control protocols that support automation and standardization.
● Tree-level data acquisition is almost non-existent due to the minimal usage of UAVs and LiDAR data. Thus, enhanced LiDAR and UAV applications could facilitate the detection and measurement of individual tree-level growth characteristics and conditions. These acquisition techniques can provide useful data on tree stress allowing mortality to be identified and be associated with the various threats to mangrove ecosystems in the GCC countries for upscaling and validation of satellite-derived data.
● Standard protocols for data measurement, reporting, analysis, validation, etc. are required for comparison and quality assessment purposes. Thus, increased accessibility to high-resolution satellite, LiDAR and UAV data at lower cost using standardized methodologies that produce comparable results for different periods of time and locations is needed. Results from these analyses should be freely available to the public.
● Baseline estimation of mangrove cover change needs to be robust and different kinds of machine learning algorithms should be explored and compared to locate the best option. This application would capitalize on the high level of detail that can be extracted from fine-resolution images and 3D point clouds.
● Long-term data monitoring and collection should be prioritized, and more attention is especially needed for newly planted sites.
● Remote sensing-based studies that can estimate mangrove forest greenness and moisture content using different combinations of vegetation indices obtained from multispectral or hyperspectral imagery would be invaluable to accurately detect and classify mangrove forest.
● The need for hardware/software for processing data can be mitigated by using platforms such as GEE or similar data cube platforms such as Digital Earth Australia that provide remotely sensed data. The GEE platform offers opportunities for coding, neural networks, deep learning, and machine learning that would help to improve data and geographic accuracy and streamline predictions within mangrove ecosystems.
4.11 Recommendations for future research
● GCC-wide LiDAR and UAV data collection campaigns are recommended for the provision of long-term data for estimating mangrove forests and tree-level characteristics and valuation of mangrove ecosystem services in real time for inclusion in various models to improve model reliability. Analysis of such data could be used to guide coastal planning and development policy reforms and practices, which support sustainable mangrove management and protection.
● In particular, GCC countries such as Kuwait, Bahrain and Oman with limited studies and spatial data and discrepancy in mangrove cover and distribution results would benefit immensely from the use of advanced UAV and LiDAR remote sensing technologies to gather high resolution data to provide validation options for moderate and high resolution satellite data. This will help bridge the observed regional gap in remote sensing-based mangrove assessment studies between the other GCC countries such as Saudi Arabia, UAE and Qatar.
● The moderate and high resolution remote sensing data for large and small spatial scales should be combined with machine learning algorithms to increase the accuracy and efficiency of detecting and classifying mangrove cover. Very high spatial resolution images such as QuickBird (0.6 m) and WorldView (0.3 m) or 1-m resolution DEM derived from Airborne Laser Scanning (ALS) LIDAR data integrated with machine learning approaches and validated with fieldwork are recommended for future studies to map and monitor changes in mangroves (Elmahdy and Ali, 2022). Results show that non-parametric models such as random forest provided high predictive accuracy in detecting and classifying mangrove forests in the GCC countries as has been shown previously (Elmahdy et al., 2020). Deep learning using Capsules-Unet method performed more accurately and efficiently in processing detailed information on mangrove cover change and extracting tiny features over 25 years than using the U-net method (Guo et al., 2021). The Object-based eCognition with contextual editing was more accurate and efficient than the Object-based: eCognition no contextual editing, Pixel-based ISODATA and Object-based ENVI FX in their ability to detect and classify mangrove habitats (Butler et al., 2020).
● A recommended mapping protocol is to fuse moderate to high spatial resolution time series datasets and preprocess and train models from these datasets using ensemble advanced machine learning techniques in GEE (Pham et al., 2019; Elmahdy et al., 2020). GEE provides a better web platform to model mangrove cover change and carbon stock and sequestration for global, regional and national studies using multi-temporal and multi-sensor medium and high resolution satellite imageries (Blanco-Sacristán et al., 2022). This approach could provide benchmark datasets to guide current and future mangrove afforestation and conservation initiatives as part of each GCC country’s net zero CO2 emission inventory and climate change mitigation efforts (Aljenaid et al., 2022).
● Very high spatial resolution images such as QuickBird (0.6 m) and WorldView (0.3 m) or 1-m resolution DEM derived from Airborne Laser Scanning (ALS) LIDAR data integrated with deep learning approaches and validated with fieldwork are recommended for future studies to map and monitor changes in mangroves (Elmahdy and Ali, 2022).
● Remote sensing-based mangrove forest carbon stocks estimation should integrate field measurements since model estimates can be significantly different from those obtained using comprehensive field inventories (Bukoski et al., 2020).
● In general, studies of mangroves, combining very high resolution remote sensing data with machine learning and deep learning techniques is likely to greatly advance our ability to map mangrove area, identify species and quantify biomass and carbon stocks.
4.12 Potential opportunities and policy implications
As technology advances, there are increasing opportunities for the use of UAVs for mangrove monitoring and assessment over small areas in GCC countries. The often small and dispersed distribution of mangroves is well suited to the use of terrestrial LiDAR and UAV remote sensing technologies. These emerging remote sensing technologies provide opportunities for the acquisition of high-resolution forest-level and tree-level data at small spatial scales that are often needed for the validation and upscaling of satellite data over larger spatial scales (Ewane et al., 2023b). There are also opportunities for training and evaluation of remote sensing tools and integration of machine learning algorithms to improve on the accuracy of assessment of mangrove distribution and cover and specific characteristics.
There are great opportunities for the expansion of mangrove regeneration and restoration initiatives. Government efforts to promote improved coastal environmental management and planning through expanding mangrove plantations have been laudable. This serves as part of the growing movement toward using nature-based solutions and ecological engineering approaches to respond to looming climate change challenges for coastal communities (Burt and Bartholomew, 2019; Pittman et al., 2022). These efforts can be further enhanced by involving local community stakeholders in mangrove conservation and restoration initiatives for increased success as a nature-based solution to climate change mitigation efforts in the GCC countries. There are also opportunities for involving local experts through participatory mapping exercises and the integration of local ecological knowledge to support secondary ground-truthing and post-classification improvement and accuracy assessment of mangrove data in the GCC region (Mateos-Molina et al., 2020).
Mangrove forest conservation and restoration provide viable opportunities for carbon offset programs for the industries contributing to GHG emissions in the GCC countries through compliance and/or voluntary carbon markets. Governments in the GCC countries have the opportunity to develop well-structured mechanisms and legal frameworks for compliance and voluntary carbon markets for companies in individual countries and the region. Expansion of remote sensing-based studies that estimate biomass and blue carbon for integration into carbon markets for mangrove forests ecosystem is invaluable. This nature-based carbon offset credits initiative in the compliance and voluntary carbon markets may encourage more oil and gas companies to fund mangrove restoration programmes as a nature-based solution to climate change in the GCC countries. Predictive models are useful in designing national and regional scale policy programs and strategies for mangrove forest biomass carbon estimation and monitoring, but less so at local scales.
There are opportunities to develop Mangrove Monitoring, Evaluation, and Reporting (MMER) programs and to establish an open-source benchmark mangrove distribution, cover and forest characteristics dataset for individual GCC countries, as part of a national forest inventory. These initiatives would support mangrove conservation and climate change mitigation efforts by the government, international organizations, and oil and petrochemical industries. It will also foster the movement toward using ecosystem-based approaches to coastal management that are growing in the GCC region (Fanning et al., 2021; Mateos-Molina et al., 2021).
There are opportunities for understanding the impact of climate change and environmental variables on mangrove cover and distribution. This can be achieved by automating mangrove forest monitoring using integrated high-resolution data with machine learning techniques (Alsumaiti et al., 2019; Elmahdy et al., 2020; Guo et al., 2021). This will improve on mangrove classification, biomass estimation and carbon sequestration accuracy assessment under different climate change scenarios. There are opportunities for the use of UAVs and LiDAR technologies to help support research on afforestation and reforestation best practices, including aspects such as seedling density, substrate slope and inundation and related parameters in order to enhance the growth and survivorship of seedlings in future planting efforts. Also, development of a novel mangrove-soil-climate nexus approach to improve our understanding of the interconnections and factors influencing the growth of mangroves in the region would be invaluable.
5 Conclusions
Mangrove species A. marina and R. mucronata are the two most resilient evergreen forests along the coastlines of the GCC countries that provide critical ecosystem services crucial to the environment and human-well-being. Remote sensing tools can be used to monitor and measure a wide range of mangrove forest characteristics at different spatial and temporal scales that allow for a more detailed and better understanding of mangrove ecosystems. Based on a systematic literature review, the largest expanse of mangrove forests was reported in Saudi Arabia, followed by the UAE, Qatar, Oman, Bahrain and Kuwait, respectively, with this area mainly estimated from satellite images. Mangrove cover in the GCC countries, from 1996 to 2020, decreased in Saudi Arabia, Bahrain, Oman and Kuwait, remained relatively stable in the UAE but increased appreciably in Qatar. However, reported changes in area varied highly between studies due to the lack of a standardization methodology, differences in satellite imagery resolutions and classification approaches used in different studies. Mangrove ecosystems in the GCC countries are threatened by climate change, but more so by progressive anthropogenic land use change stressors. GCC countries such as UAE and Qatar are investing tremendously toward expanding mangrove plantations through various large scale reforestation and afforestation projects.
The overall paucity of studies that used remotely sensed data in the GCC countries, particularly in Kuwait, Bahrain, Oman, and Qatar highlights the urgent need for more research in this area. In particular, the lack of LiDAR and UAV data and the relative complexities around flying UAVs in the GCC countries explains why only a few mangrove forest tree-level characteristics were measured in the reviewed remote sensing studies. Studies should capitalize on recent developments that use high-resolution remote sensing data at different spatial scales and in real-time for the sustainable management of the mangrove forests. The reforestation and conservation of mangrove forests should be prioritized to sustain key mangrove ecosystem services. Community involvement should also be encouraged to increase local awareness of mangrove conservation and support global UN Sustainable Development Goals related to climate change actions, life below water, and life on land.
Data availability statement
The raw data supporting the conclusions of this article will be made available by the authors, without undue reservation.
Author contributions
MR: Writing - original draft, Review & editing, Methodology, Data curation, Formal analysis. EBE: Writing - original draft, Review & editing, Methodology, Data curation, Formal analysis, Supervision, Project administration. MMA: Writing - original draft, Review & editing, Supervision, Project administration. MSW: Review & Editing. AB: Writing - original draft, Review & Editing. AA: Review & Editing. JAB: Review & Editing. KR: Review & Editing. TA: Review & Editing. RR: Review & Editing; RM: Review & Editing. FS: Review & Editing. MF: Writing - original draft, Review & Editing. Sd-M: Review & Editing. GAPG: Review & Editing. YARC: Review & Editing. PSPA: Review & Editing. LFV-C: Review & Editing. TA-A: Review & Editing. SK: Writing - original draft. SS: Review & Editing. WSWMJ: Review & Editing. JFM: Review & Editing. EK: Writing - original draft. JP: Writing – original draft. MJA: Review & Editing. AC: Review & Editing. WD: Review & Editing. MM: Conceptualization, Writing - original draft, Review & editing, Methodology, Supervision, Project administration, Data curation, Formal analysis. All authors contributed to the article and approved the submitted version.
Funding
This project and publication was made possible by a High-Impact Grant Award [211] from Qatar University, Qatar. The publication was also funded by the Internal Grant Award No. IG/ART/GEOG/23/01 from Sultan Qaboos University of Oman, entitled “Managing Ecosystem Services and Natural Resources for Water and Food Resilience in a Changing Climate”.
Acknowledgments
The authors would like to thank the following people for their support during the literature review: Daniel A. Friess, Esmaeel Adrah, Shaurya Bajaj, Gabby Richardson and Dhouha Elouerfelli. EBE was supported by the University of Buea Research Grants (Decision No. 2023/0546/UB/DFinA/DFSMS/FO/FIN/AA). RR would like to thank Australian Research Council Discovery Award DP180103444. JAB was supported by the NYUAD Center for Interacting Urban Networks (CITIES) and the Arabian Center for Climate and Environmental Sciences (ACCESS), funded by Tamkeen under the NYUAD Research Institute Award CG001 and CG009, respectively. TA acknowledges the support of the ORGS at the American University of Sharjah (UAE) through grant # FRG21-E77. The statements made herein are solely the responsibility of the authors.
Conflict of interest
Authors MR, EBE, JFM, JP, and MM were employed by Ecoresolve. Authors MMA, AB, GAPG, PSPA, LFV-C, SK, SS and WSWMJ were volunteers (unpaid) with Ecoresolve at the scientific research and advisory roles. Authors EBE, JFM, and MM were employed by BlueForests. Author AC was employed by Tecnosylva.
The remaining authors declare that the research was conducted in the absence of any commercial or financial relationships that could be construed as a potential conflict of interest.
Publisher’s note
All claims expressed in this article are solely those of the authors and do not necessarily represent those of their affiliated organizations, or those of the publisher, the editors and the reviewers. Any product that may be evaluated in this article, or claim that may be made by its manufacturer, is not guaranteed or endorsed by the publisher.
Supplementary material
The Supplementary Material for this article can be found online at: https://www.frontiersin.org/articles/10.3389/fmars.2023.1241928/full#supplementary-material
References
Abd El-Hamid H. T., Eid E. M., El-Morsy M. H. E., Osman H. E. M., Keshta A. E. (2022a). Benefits of blue carbon stocks in a coastal jazan ecosystem undergoing land use change. Wetlands 42, 103. doi: 10.1007/s13157-022-01597-9
Abd El-Hamid H. T., Arshad M., Eid E. M. (2022b). The effects of coastal development on the urban heat island in the mangrove ecosystem along the Jazan coast, KSA. J. Coast. Conserv. 26, 70. doi: 10.1007/s11852-022-00915-x
ADNOC Uses Innovative Drone Technology to Plant Mangroves (2003). Available at: https://www.adnoc.ae/en/news-and-media/press-releases/2023/adnoc-uses-innovative-drone-technology-to-plant-mangroves (Accessed May 25, 2023).
Al-Afifi Z. M. M. (2018). The Conservation, Sustainable use and Management of Mangrove Habitats in Oman. Available at: https://etheses.whiterose.ac.uk/23437/ (Accessed May 28, 2023).
Al-Ali A. M., Del Campo A. G., Rocha C. (2015). Environmental assessment of Mangrove communities in Tarut Bay, Eastern Arabian Peninsula, based on multidisciplinary approach. Int. Arch. Photogramm. Remote Sens. Spat. Inf. Sci. XL-7/W3, 269–276. doi: 10.5194/isprsarchives-XL-7-W3-269-2015
Al-Awadhi T., Mansour S., Hereher M. (2020). Assessment of coastal sensitivity to non-eustatic sea level rise: a case study on Muscat coast—Sultanate of Oman. Arab. J. Geosci. 13, 1–11. doi: 10.1007/s12517-020-05321-x
Aljamali F. H., Ali E. M., Ahmed M. H. (2014). Impacts of accelerated coastal developments on the eastern coast of Qatar. Case study: umm saeed coastal area. Int. J. Environ. Sci. Eng. IJESE 5, 127–137.
Aljenaid S., Abido M., Redha G. K., AlKhuzaei M., Marsan Y., Khamis A. Q., et al. (2022). Assessing the spatiotemporal changes, associated carbon stock, and potential emissions of mangroves in Bahrain using GIS and remote sensing data. Reg. Stud. Mar. Sci. 52, 102282. doi: 10.1016/j.rsma.2022.102282
Aljahdali M. O., Munawar S., Khan W. R. (2021). Monitoring Mangrove Forest Degradation and Regeneration: Landsat Time Series Analysis of Moisture and Vegetation Indices at Rabigh Lagoon, Red Sea. Forests 12 (1), 52. doi: 10.3390/f12010052
Al-Khayat J. A., Balakrishnan P. (2014). Avicennia marina around Qatar: tree, seedling and pneumatophore densities in natural and planted mangroves using remote sensing. Int. J. Sci. 3, 18–27.
Almahasheer H. (2018). Spatial coverage of mangrove communities in the Arabian Gulf. Environ. Monit. Assess. 190, 85. doi: 10.1007/s10661-018-6472-2
Almahasheer H., Aljowair A., Duarte C. M., Irigoien X. (2016). Decadal stability of Red Sea mangroves. Estuar. Coast. Shelf Sci. 169, 164–172. doi: 10.1016/j.ecss.2015.11.027
Al-Nadabi A. S., Sulaiman H. (2021). Carbon sequestration service of a ramsar site: A conservation-role model for defying developmental pressure in the middle of a rapidly expanding city. Open J. For. 11, 381–397. doi: 10.4236/ojf.2021.114023
Al-Naimi N., Al-Ghouti M. A., Balakrishnan P. (2016). Investigating chlorophyll and nitrogen levels of mangroves at Al-Khor, Qatar: an integrated chemical analysis and remote sensing approach. Environ. Monit. Assess. 14, 2546. doi: 10.1007/s10661-016-5269-4
Alongi D. M. (2020). Carbon balance in salt marsh and mangrove ecosystems: A global synthesis. J. Mar. Sci. Eng. 8, 767. doi: 10.3390/jmse8100767
AlQahtany A. M., Dano U. L., Elhadi Abdalla E. M., Mohammed W. E., Abubakar I. R., Al-Gehlani W. A. G., et al. (2022). Land reclamation in a coastal metropolis of Saudi Arabia: environmental sustainability implications. Water 14, 2546. doi: 10.3390/w14162546
Al-Sinan M. A., Bubshait A. A., Alamri F. (2023). Saudi Arabia’s journey toward net-zero emissions: progress and challenges. Energies 16, 978. doi: 10.3390/en16020978
Alsumaiti T. S. (2017). Mapping changes in mangrove forests and the future impacts of sea level rise in Abu Dhabi, United Arab Emirates. Int. J. Basic Appl. Sci. 7, 57–61.
Alsumaiti T. S., Tullis J. A., Xue B. (2019). LIDAR-derived biomass and height inventory of avicennia marina in eastern mangrove lagoon national park, Abu Dhabi. Int. J. Bus. Appl. Soc Sci. 5 (1), 137–152.
Alwhibi M. S. (2017). Density and distribution of mangrove stands between Ras – Al Turfa and Bab-Al mandab using remote sensing system. Biosci. Biotechnol. Res. Asia 14, 115–121. doi: 10.13005/bbra/2425
Arshad M., Eid E. M., Hasan M. (2020). Mangrove health along the hyper-arid southern Red Sea coast of Saudi Arabia. Environ. Monit. Assess. 192, 1–15. doi: 10.1007/s10661-020-8140-6
Babu D. S. S., Sivalingam S., MaChado T. (2012). Need for adaptation strategy against global sea level rise: an example from Saudi coast of Arabian gulf. Mitig. Adapt. Strateg. Glob. Change 17, 821–836. doi: 10.1007/s11027-011-9346-2
Badzmierowski M. J., Evanylo G. K., Daniels W. L., Kathryn C. (2021). What is the impact of human wastewater biosolids (sewage sludge) application on long-term soil carbon sequestration rates? A systematic review protocol.. Environmental Evidence 10 (6). doi: 10.1186/s13750-021-00221-3
Bahrawi J. A., Elhag M. (2016). Simulation of sea level rise and its impacts on the western coastal area of Saudi Arabia. IJMS 45 (1), 54–61.
Blanco-Sacristán J., Johansen K., Duarte C. M., Daffonchio D., Hoteit I., McCabe M. F. (2022). Mangrove distribution and afforestation potential in the Red Sea. Sci. Total Environ. 843, 157098. doi: 10.1016/j.scitotenv.2022.157098
Bukoski J. J., Elwin A., MacKenzie R. A., Sharma S., Purbopuspito J., Kopania B., et al. (2020). The role of predictive model data in designing mangrove forest carbon programs. Environ. Res. Lett. 15, 084019. doi: 10.1088/1748-9326/ab7e4e
Bunting P., Rosenqvist A., Hilarides L., Lucas R. M., Thomas N., Tadono T., et al. (2022). Global mangrove extent change 1996–2020: global mangrove watch version 3.0. Remote Sens. 14, 3657. doi: 10.3390/rs14153657
Burt J. A. (2014). The environmental costs of coastal urbanization in the Arabian Gulf. City 18, 760–770. doi: 10.1080/13604813.2014.962889
Burt J. A., Bartholomew A. (2019). Towards more sustainable coastal development in the Arabian Gulf: Opportunities for ecological engineering in an urbanized seascape. Mar. pollut. Bull. 142, 93–102. doi: 10.1016/j.marpolbul.2019.03.024
Burt J. A., Ben-Hamadou R., Abdel-Moati M. A. R., Fanning L., Kaitibie S., Al-Jamali F., et al. (2017). Improving management of future coastal development in Qatar through ecosystem-based management approaches. Ocean Coast. Manage. 148, 171–181. doi: 10.1016/j.ocecoaman.2017.08.006
Burt J. A., Killilea M., Rademacher A. (2021). Unexpected nature? Proliferating mangroves on the coast of Abu Dhabi – urban environments initiative. Urban Environ. Initiat.
Butler J. D., Purkis L. M., Purkis S. J., Yousif R., Al-Shaikh I. (2021). A benthic habitat sensitivity analysis of Qatar’s coastal zone. Mar. Pollut. Bull. 167, 112333. doi: 10.1016/j.marpolbul.2021.112333
Butler J. D., Purkis S. J., Yousif R., Al-Shaikh I., Warren C. (2020). A high-resolution remotely sensed benthic habitat map of the Qatari coastal zone. Mar. pollut. Bull. 160, 111634. doi: 10.1016/j.marpolbul.2020.111634
Castellanos-Galindo G. A., Casella E., Mejía-Rentería J. C., Rovere A. (2019). Habitat mapping of remote coasts: Evaluating the usefulness of lightweight unmanned aerial vehicles for conservation and monitoring. Biol. Conserv. 239, 108282. doi: 10.1016/j.biocon.2019.108282
Chithambaran S. (2019). Restoration of mangrove vegetation at Red Sea coast, Saudi Arabia. Available at: https://www.semanticscholar.org/paper/Restoration-of-mangrove-vegetation-at-Red-Sea-Saudi-Chithambaran/90dcd5208c2df20a4caf205f8ea850c901a76cb7 (Accessed May 28, 2023).
Climate Watch (2022). Climate Watch. Available at: https://www.climatewatchdata.org/ (Accessed May 27, 2023).
Cusack M., Saderne V., Arias-Ortiz A., Masqué P., Krishnakumar P. K., Rabaoui L., et al. (2018). Organic carbon sequestration and storage in vegetated coastal habitats along the western coast of the Arabian Gulf. Environ. Res. Lett. 13, 074007. doi: 10.1088/1748-9326/aac899
Donato D. C., Kauffman B. J., Murdiyarso D., Kurnianto S., Stidham M., Kanninen M. (2011). Mangroves among the most carbon-rich forests in the tropics. Nat. Geosci. 4, 293–297. doi: 10.1038/ngeo1123
Drone Laws (2022). Drone Laws in Saudi Arabia (Httpsdrone-Lawscom). Available at: https://drone-laws.com/drone-laws-in-saudi-arabia/ (Accessed May 28, 2023).
Duarte C. M., Losada I. J., Hendriks I. E., Mazarrasa I., Marba N. (2013). The role of coastal plant communities for climate change mitigation and adaptation. Nat. Clim. Change 3, 961–968. doi: 10.1038/nclimate1970
Elmahdy S. I., Ali T. A. (2022). Monitoring changes and soil characterization in mangrove forests of the United Arab Emirates using the canonical correlation forest model by multitemporal of landsat data. Front. Remote Sens. 3. doi: 10.3389/frsen.2022.782869
Elmahdy S. I., Ali T. A., Mohamed M. M., Howari F. M., Abouleish M., Simonet D. (2020). Spatiotemporal mapping and monitoring of mangrove forests changes from 1990 to 2019 in the Northern Emirates, UAE using random forest, Kernel logistic regression and Naive Bayes Tree models. Front. Environ. Sci. 8. doi: 10.3389/fenvs.2020.00102
Elmahdy S. I., Mohamed M. M. (2013). Change detection and mapping of mangrove using multi-temporal remote sensing data: a case study of Abu Dhabi, UAE. J. Geomat. 7, 41–45.
Elsebaie I. H., Aguib A. S. H., Garni D. A. (2013). The role of remote sensing and GIS for locating suitable mangrove plantation sites along the southern Saudi Arabian red sea coast. Int. J. Geosci. 04, 471–479. doi: 10.4236/ijg.2013.42044
Environmental Authority of Oman (2022). Oman’s Environment Authority to plant 1.5 million mangroves. Available at: https://arabiandaily.com/omans-environment-authority-to-plant-1-5-million-mangroves/ (Accessed May 25, 2023).
Erftemeijer P. L. A., Cambridge M. L., Price B. A., Ito S., Yamamoto H., Agastian T., et al. (2021). Enhancing growth of mangrove seedlings in the environmentally extreme Arabian Gulf using treated sewage sludge. Mar. Pollut. Bull. 170, 112595. doi: 10.1016/j.marpolbul.2021.112595
Ewane B. E., Shaurya Bajaj S., Velasquez-Camacho L. V., Srinivasan S., Maeng J., Singla A. (2023a). Influence of urban forests on residential property values: A systematic review of remote sensing-based studies. Heliyon, Environment. Accepted. 9, e20408. doi: 10.1016/j.heliyon.2023.e20408
Ewane B. E., Mohan M., Bajaj S., Galgamuwa G. A. P., Watt M. S., Arachchige P. P., et al. (2023b). Climate-change-driven droughts and tree mortality: assessing the potential of UAV-derived early warning metrics. Remote Sens. 15, 2627. doi: 10.3390/rs15102627
Fanning L. M., Al-Naimi M. N., Range P., Ali A.-S. M., Bouwmeester J., Al-Jamali F., et al. (2021). Applying the ecosystem services - EBM framework to sustainably manage Qatar’s coral reefs and seagrass beds. Ocean Coast. Manage. 205, 105566. doi: 10.1016/j.ocecoaman.2021.105566
FAO (2005). Global forest resources assessment. Thematic study of mangroves. Kuwait Country Profile. FAO, Food and Agriculture Organization of the United Nations. Available at: http://www.fao.org/forestry/9195-03f3d0eaaddd5c05f779ee62b2b2b78d6.pdf.
Friess D., Rogers K., Lovelock C., Krauss K. W., Hamilton S. E., Yip Lee S., et al. (2019). The state of the world’s mangrove forests: past, present, and future. Annu. Rev. Envrionment Resour. 44, 1–27. doi: 10.1146/annurev-environ-101718-033302
Friess D., Yando E., Moraes de Oliveira Abuchahla G., Adams J. B., Cannicci S., Canty S. W. J., et al. (2020). Mangroves give cause for conservation optimism, for now. Curr. Biol. 30, 153–154. doi: 10.1016/j.cub.2019.12.054
Friis G., Burt J. A. (2020). Evolution of mangrove research in an extreme environment: Historical trends and future opportunities in Arabia. Ocean Coast. Manage. 195, 105288. doi: 10.1016/j.ocecoaman.2020.105288
General Civil Aviation Authority - GCAA of UAE (2023). UAS Registration. Available at: https://www.gcaa.gov.ae/en/pages/UASRegistration.aspx (Accessed May 28, 2023).
Getzner M., Islam M. S. (2020). Ecosystem services of mangrove forests: results of a meta-analysis of economic values. Int. J. Environ. Res. Public. Health 17, 5830. doi: 10.3390/ijerph17165830
Goldberg L., Lagomasino D., Thomas N., Fatoyinbo T. (2020). Global declines in human-driven mangrove loss. Glob. Change Biol. 26, 5844–5855. doi: 10.1111/gcb.15275
Goldblatt R., Rivera Ballesteros A., Burney J. (2017). High spatial resolution visual band imagery outperforms medium resolution spectral imagery for ecosystem assessment in the semi-arid Brazilian sertão. Remote Sens. 9, 1336. doi: 10.3390/rs9121336
Guo Y., Liao J., Shen G. (2021). Mapping large-scale mangroves along the maritime silk road from 1990 to 2015 using a novel deep learning model and landsat data. Remote Sens. 13, 245. doi: 10.3390/rs13020245
Hagger V., Worthington T. A., Lovelock C. E., Adame M. F., Amano T., Brown M. B., et al. (2022). Drivers of global mangrove loss and gain in social-ecological systems. Nat. Commun. 13, 6373. doi: 10.1038/s41467-022-33962-x
Hereher M. E. (2016). Vulnerability assessment of the Saudi Arabian Red Sea coast to climate change. Environ. Earth Sci. 75, 30. doi: 10.1007/s12665-015-4835-3
Hereher M. E., Al-Awadhi T. (2019). Remote sensing of coastal ecosystems using spectral indices. Proc. 3rd Int. Conf. Vis. Image Signal Process., 1–5. doi: 10.1145/3387168.3387174
Howari F. M., Jordan B. R., Bouhouche N., Wyllie-Echeverria S. (2009). Field and remote-sensing assessment of mangrove forests and seagrass beds in the northwestern part of the United Arab Emirates. J. Coast. Res. 251, 48–56. doi: 10.2112/07-0867.1
Hsu A. J., Kumagai J., Favoretto F., Dorian J., Martinez B. G., Aburto-Oropeza O. (2020). Driven by drones: improving mangrove extent maps using high-resolution remote sensing. Remote Sens. 12 (23), 3986. doi: 10.3390/rs12233986
Ismail E., Alghoul R. (2022). UAE celebrates International Day for Conservation of Mangrove Ecosystem at Floriade Expo 2022. WAM - Emir. News Agency.
Jiang J., Johansen K., Tu Y. H., McCabe M. F. (2022). Multi-sensor and multi-platform consistency and interoperability between UAV, Planet CubeSat, Sentinel-2, and Landsat reflectance data. GIScience Remote Sens. 59, 936–958. doi: 10.1080/15481603.2022.2083791
Jones A. R., Raja Segaran R., Clarke K. D., Waycott M., Goh W. S. H., Gillanders B. M. (2020). Estimating mangrove tree biomass and carbon content: A comparison of forest inventory techniques and drone imagery. Front. Mar. Sci. 6. doi: 10.3389/fmars.2019.00784
Khader H. (2023). Mangroves in Qatar: perspectives (EcoMENA). Available at: https://www.ecomena.org/mangroves-in-Qatar/ (Accessed May 27, 2023).
Kumar A. (2011). Temporal changes in mangrove cover between 1972 and 2001 along the south coast of the Arabian Gulf. 34th Int. Symp. Remote Sens. Environ.
Kumar A., Khan M. A., Muqtadir A. (2010). Distribution of mangroves along the Red Sea coast of the Arabian Peninsula: Part-1: The northern coast of western Saudi Arabia. E-J. Earth Sci. India 3, 28–42.
Lachkar Z., Mehari M., Lévy M., Paparella F., Burt J. A. (2022). Recent expansion and intensification of hypoxia in the Arabian Gulf and its drivers. Front. Mar. Sci. 9. doi: 10.3389/fmars.2022.891378
Lamine E. B., Mateos-Molina D., Antonopoulou M., Burt J. A., Das H. S., Javed S., et al. (2020). Identifying coastal and marine priority areas for conservation in the United Arab Emirates. Biodivers. Conserv. 29, 2967–2983. doi: 10.1007/s10531-020-02007-4
Li W., El-Askary H., Qurban M. A., Li J., ManiKandan K. P., Piechota T. (2019). Using multi-indices approach to quantify mangrove changes over the Western Arabian Gulf along Saudi Arabia coast. Ecological Indicators 102, 734–745. doi: 10.1016/j.ecolind.2019.03.047
Lincoln S., Buckley P., Howes E. L., Maltby K. M., Pinnegar J. K., Ali T. S., et al. (2021). A regional review of marine and coastal impacts of climate change on the ROPME sea area. Sustainability 13, 13810. doi: 10.3390/su132413810
Loughland R., Butt S. J., Nithyanandan M. (2020). Establishment of mangrove ecosystems on man-made islands in Kuwait: Sustainable outcomes in a challenging and changing environment. Aquat. Bot. 167, 103273. doi: 10.1016/j.aquabot.2020.103273
Macreadie P. I., Costa M. D. P., Atwood T. B., Friess D. A., Kelleway J. J., Kennedy H., et al. (2021). Blue carbon as a natural climate solution. Nat. Rev. Earth Environ. 2, 826–839. doi: 10.1038/s43017-021-00224-1
Markert F. (2020)Drone Regulations worldwide. In: Drone Travel. Available at: https://drone-traveller.com/drone-regulations-worldwide/ (Accessed May 28, 2023).
Mateos-Molina D., Antonopoulou M., Baldwin R., Bejarano I., Burt J. A., Garcia-Charton J. A., et al. (2020). Applying an integrated approach to coastal marine habitat mapping in the north-western United Arab Emirates. Mar. Environ. Res. 161, 105095. doi: 10.1016/j.marenvres.2020.105095
Mateos-Molina D., Ben Lamine E., Antonopoulou M., Burt J. A., Das H. S., Javed S., et al. (2021). Synthesis and evaluation of coastal and marine biodiversity spatial information in the United Arab Emirates for ecosystem-based management. Mar. pollut. Bull. 167, 112319. doi: 10.1016/j.marpolbul.2021.112319
Melville-Rea H., Eayrs C., Anwahi N., Burt J. A., Holland D., Samara F., et al. (2021). A roadmap for policy-relevant sea-level rise research in the United Arab Emirates. Front. Mar. Sci. 8. doi: 10.3389/fmars.2021.670089
Meyer L. H., Heurich M., Beudert B., Premier J., Pflugmacher D. J. R. S. (2019). Comparison of Landsat-8 and Sentinel-2 data for estimation of leaf area index in temperate forests. Remote Sens. 11, 1160. doi: 10.3390/rs11101160
Milani A. S. (2018). Mangrove Forests of the Persian Gulf and the Gulf of Oman. In: Makowski C., Finkl C. (eds) Threats to Mangrove Forests. Coastal Research Library (Cham: Springer) 25, 53–57. doi: 10.1007/978-3-319-73016-5_3
Ministry of Climate Change and Environment (2022). MOCCAE News | Media Center | UAE Ministry of Climate Change and Environment. Available at: https://www.moccae.gov.ae/en/media-center/news/8/11/2022/uae-Indonesia-unveil-mangrove-alliance-for-climate-at-cop27.aspx#page=1 (Accessed May 28, 2023).
Ministry of Transportation and Telecommunication (2023). Laws and Regulations | Ministry of Transportation and Telecommunication. Available at: https://mtt.gov.bh/content/caa-laws-and-regulations (Accessed May 28, 2023).
Mohan M., Richardson G., Gopan G., Aghai M. M., Bajaj S., Galgamuwa G. A. P., et al. (2021). UAV-supported forest regeneration: current trends, challenges and implications. Remote Sens. 13, 2596. doi: 10.3390/rs13132596
Moher D., Liberati A., Tetzlaff J., Altman D. G. (2009). Preferred reporting items for systematic reviews and meta-analyses: the PRISMA statement. BMJ 62 (10), 1006–12. doi: 10.1136/bmj.b2535
Monsef H. A.-E., Aguib A. S. H., Smith S. E. (2013). Locating suitable mangrove plantation sites along the Saudi Arabia Red Sea Coast. J. Afr. Earth Sci. 83, 1–9. doi: 10.1016/j.jafrearsci.2013.02.005
Moore G. E., Grizzle R. E., Ward K. M., Alshihi R. M. (2015). Distribution, pore-water chemistry, and stand characteristics of the mangroves of the United Arab Emirates. J. Coast. Res. 314, 957–963. doi: 10.2112/JCOASTRES-D-14-00142.1
Navarro A., Young M., Allan B., Carnell P., Macreadie P., Ierodiaconou D. (2020). The application of Unmanned Aerial Vehicles (UAVs) to estimate above-ground biomass of mangrove ecosystems. Remote Sens. Environ. 242, 111747. doi: 10.1016/j.rse.2020.111747
Nitoslawski S. A., Wong-Stevens K., Steenberg J. W. N., Witherspoon K., Nesbitt L., Konijnendijk van den Bosch C. C. (2021). The digital forest: mapping a decade of knowledge on technological applications for forest ecosystems. Earths Future 9, e2021EF002123. doi: 10.1029/2021EF002123
Oman Observer (2020). Over 1,000 mangrove seedlings planted in Al Wusta (Oman Obs). Available at: https://www.omanobserver.om/article/9090/Main/over-1000-mangrove-seedlings-planted-in-al-wusta (Accessed May 28, 2023).
OZYRPAS (2022). Global Drone Regulations Database. Available at: https://www.droneregulations.info/index.html (Accessed May 28, 2023).
Pham T., Yokoya N., Bui D., Yoshino K., Friess D. (2019). Remote Sensing Approaches for Monitoring Mangrove Species, Structure, and Biomass: Opportunities and Challenges. Remote Sensing 11 (3), 230. doi: 10.3390/rs11030230
Pittman M., Bell P. R., Miller C. V., Enriquez N. J., Wang X., Zheng X., et al. (2022). Exceptional preservation and foot structure reveal ecological transitions and lifestyles of early theropod flyers. Nat. Commun. 13 (1), 7684. doi: 10.1038/s41467-022-35039-1
Psomiadis E., Dercas N., Dalezios N. R., Spyropoulos N. V. (2017). Evaluation and cross-comparison of vegetation indices for crop monitoring from sentinel-2 and worldview-2 images, Remote Sensing for Agriculture, Ecosystems, and Hydrology XIX. Int. Soc Opt. Photonics 10421, 1B. doi: 10.1117/12.2278217
Rittenhouse C. (2017). Conservation planning: informed decisions for a healthier planet. Landsc. Ecol. 32, 2219–2221. doi: 10.1007/s10980-017-0577-9
Ruwaimana M., Satyanarayana B., Otero V. M., Muslim A., Syafiq A. M., Ibrahim S., et al. (2018). The advantages of using drones over space-borne imagery in the mapping of mangrove forests. PloS One 13 (7), e0200288. doi: 10.1371/journal.pone.0200288
Samara F., Solovieva N., Ghalayini T., Nasrallah Z. A., Saburova M. (2020). Assessment of the environmental status of the mangrove ecosystem in the United Arab Emirates. Water 12, 1623. doi: 10.3390/w12061623
Schile L. M., Kauffman J. B., Crooks S., Fourqurean J. W., Glavan J., Megonigal J. P. (2017). Limits on carbon sequestration in arid blue carbon ecosystems. Ecol. Appl. 27, 859–874. doi: 10.1002/eap.1489
Spalding M. D., Leal M. (2021). The State of the World’s Mangroves 2021 (Mangrove Alliance). Available at: https://www.mangrovealliance.org/the-state-of-the-worlds-mangroves-2021/ (Accessed May 27, 2023).
Subraelu P., Sefelnasr A., Yagoub M. M., Sherif M., Ebraheem A. A. (2022). Global Warming Climate Change and Sea Level Rise: Impact on Land Use Land Cover Features along UAE coast through Remote Sensing and GIS. J. Ecosys Ecograph 12, 2. doi: 10.4172/2157-7625.1000329
The National (2022). Cop27: UAE and Indonesia lead alliance to protect and expand mangrove forests (The National). Available at: https://www.thenationalnews.com/climate/cop27/2022/11/08/uae-and-Indonesia-lead-international-alliance-to-protect-and-expand-mangrove-forests/ (Accessed May 28, 2023).
Times News Service (2022). Over 1 million mangrove saplings planted in Al Wusta Governorate I Times of Oman (Times Oman). Available at: https://timesofoman.com/article/119795-over-1-million-mangrove-saplings-planted-in-al-wusta-governorate (Accessed May 28, 2023).
UAV Coach (2023). Regulatory Updates Archives (UAV Coach). Available at: https://uavcoach.com/category/regulatory-updates/ (Accessed May 28, 2023).
UAV Systems International (2023). Drone Laws By Country (UAV Syst. Int). Available at: https://uavsystemsinternational.com/pages/drone-laws-by-country (Accessed May 28, 2023).
UNEP (2018). Mangrove restoration paying dividends in Oman (UNEP). Available at: http://www.unep.org/news-and-stories/story/mangrove-restoration-paying-dividends-oman (Accessed May 28, 2023).
Vaughan G. O., Al-Mansoori N., Burt J. A. (2019). The Arabian Gulf. In: Sheppard C., Ed., World Seas: An Environmental Evaluation. The Indian Ocean to the Pacific, 2nd Edition. (Cambridge: Academic Press) 1, 1–23. doi: 10.1016/B978-0-08-100853-9.00001-4
Warren C., Dupont J., Abdel-Moati M., Hobeichi S., Palandro D., Purkis S. (2016). Remote sensing of Qatar nearshore habitats with perspectives for coastal management. Mar. pollut. Bull. 105, 641–653. doi: 10.1016/j.marpolbul.2015.11.036
Xia Q., Qin C., Li H., Huang C., Su F. (2018). Mapping mangrove forests based on multi-tidal high-resolution satellite imagery. Remote Sens. 10 (9), 1343. doi: 10.3390/rs10091343
Xia Q., Qin C.-Z., Li H., Huang C., Su F.-Z., Jia M.-M. (2020). Evaluation of submerged mangrove recognition index using multi-tidal remote sensing data. Ecol. Indic. 113, 106196. doi: 10.1016/j.ecolind.2020.106196
Xian G., Shi H., Dewitz J., Wu Z. (2019). Performances of WorldView 3, Sentinel 2, and Landsat 8 data in mapping impervious surface. Remote Sens. Appl. Soc Environ. Dev. 15, 100246. doi: 10.1016/j.rsase.2019.100246
Ximenes A. C., Cavanaugh K. C., Arvor D., Murdiyarso D., Thomas N., Arcoverde G. F. B., et al. (2023). A comparison of global mangrove maps: Assessing spatial and bioclimatic discrepancies at poleward range limits. Sci. Total Environ. 860, 160380. doi: 10.1016/j.scitotenv.2022.160380
Keywords: mangrove distribution and cover, mangrove forest classification, remote sensing, mangroves and climate change, mangrove afforestation, mangrove ecosystem services, machine learning, Arabian Gulf
Citation: Rondon M, Ewane EB, Abdullah MM, Watt MS, Blanton A, Abulibdeh A, Burt JA, Rogers K, Ali T, Reef R, Mohtar R, Sidik F, Fahrenberg M, de-Miguel S, Galgamuwa GAP, Charabi YAR, Arachchige PSP, Velasquez-Camacho LF, Al-Awadhi T, King S, Srinivasan S, Jaafar WSWM, Montenegro JF, Karakasidou E, Pons J, Abbady MJ, Cardil A, Doaemo W and Mohan M (2023) Remote sensing-based assessment of mangrove ecosystems in the Gulf Cooperation Council countries: a systematic review. Front. Mar. Sci. 10:1241928. doi: 10.3389/fmars.2023.1241928
Received: 17 June 2023; Accepted: 23 October 2023;
Published: 20 November 2023.
Edited by:
Vitor H. Paiva, University of Coimbra, PortugalReviewed by:
Asmaa Hassan Mohammed, National Authority for Remote Sensing and Space Sciences, EgyptZhongyi Sun, Hainan University, China
Yuhan Zheng, Fudan University, China
Copyright © 2023 Rondon, Ewane, Abdullah, Watt, Blanton, Abulibdeh, Burt, Rogers, Ali, Reef, Mohtar, Sidik, Fahrenberg, de-Miguel, Galgamuwa, Charabi, Arachchige, Velasquez-Camacho, Al-Awadhi, King, Srinivasan, Jaafar, Montenegro, Karakasidou, Pons, Abbady, Cardil, Doaemo and Mohan. This is an open-access article distributed under the terms of the Creative Commons Attribution License (CC BY). The use, distribution or reproduction in other forums is permitted, provided the original author(s) and the copyright owner(s) are credited and that the original publication in this journal is cited, in accordance with accepted academic practice. No use, distribution or reproduction is permitted which does not comply with these terms.
*Correspondence: Ewane Basil Ewane, basil.ewane@ecoresolve.eco; Meshal M. Abdullah, m.abdullah1@squ.edu.om; Midhun Mohan, mikey@ecoresolve.eco