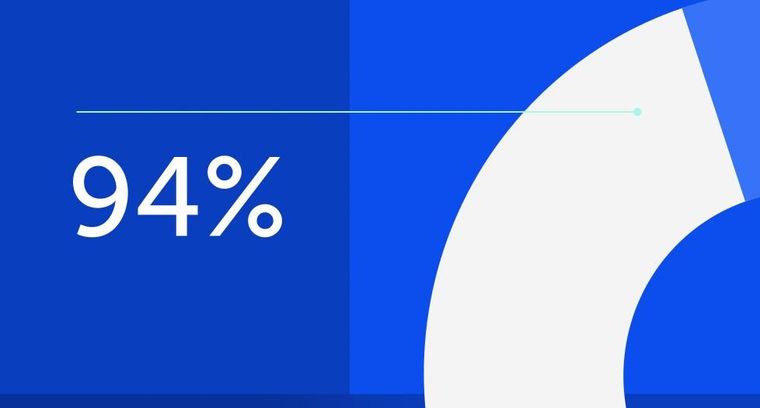
94% of researchers rate our articles as excellent or good
Learn more about the work of our research integrity team to safeguard the quality of each article we publish.
Find out more
ORIGINAL RESEARCH article
Front. Mar. Sci., 20 October 2023
Sec. Marine Megafauna
Volume 10 - 2023 | https://doi.org/10.3389/fmars.2023.1229682
This article is part of the Research TopicThe ACCOBAMS Survey Initiative (ASI): Implementing Large Scale Surveys for Marine Megafauna in the Mediterranean and Black SeasView all 13 articles
The Mediterranean sperm whale population, Physeter macrocephalus, is listed as endangered due to population decline caused by human activities. To mitigate the impact of these activities, accurate knowledge of their distribution and abundance is crucial. During their long dives, sperm whales are not available to visual observation, but since they produce sounds when they dive, they are available to acoustic detection. Therefore, we aimed to use towed acoustic data to model their habitat and fill the knowledge gap on Mediterranean sperm whale distribution. Generalised additive models were used to link the number of sperm whales detected acoustically during the ACCOBAMS Survey Initiative in 2018 with different environmental variables integrated over different depth classes, encompassing the depth range used by the species for foraging. Sperm whale distribution was influenced by water temperature at the bottom, eddy kinetic energy between 200 and 600 m, as well as gradients of sea surface temperature and chlorophyll-a concentrations. The abundance of sperm whales was estimated at 2,959 individuals [2,077 - 4,265] in the sampled areas of the Mediterranean Sea. We predicted that sperm whales were mainly distributed in summer along the continental slope of the north-western Mediterranean basin from the Balearic Islands to the Ligurian Sea and off the Algerian coast. They were present throughout the western Mediterranean Sea and in the northern Ionian Sea. In contrast, predicted densities were low in the eastern part of the Mediterranean Sea. The use of acoustic data compensated for the main difficulty in studying sperm whales, the unavailability of animals at the surface during visual observation and the paucity of visual data. We thus encourage more systematic use of passive acoustics to study sperm whale distribution. The model highlighted a higher concentration of sperm whales in the western Mediterranean basin than in the eastern basin in summer, opening up avenues to improve the conservation of this endangered Mediterranean sub-population.
The Mediterranean sperm whale population, Physeter macrocephalus (Linnaeus, 1758), genetically different from the Atlantic populations, has been listed as endangered since 1982, due to apparent population decline and habitat loss caused by human activities (Notarbartolo di Sciara et al., 2012; Rendell and Frantzis, 2016). The Mediterranean sperm whale population is particularly exposed to various anthropogenic pressures, such as activities generating high-intensity noise (e.g., military sonar, maritime works or construction), fisheries that can lead to entanglements in drift nets, ship strikes, plastic ingestion, or exposure to chemical pollution (Aguilar et al., 2002; Reeves and Notarbartolo di Sciara, 2006; Notarbartolo-Di-Sciara, 2014). To mitigate the impact of these activities, accurate knowledge of sperm whale and other cetacean distribution and abundance is crucial for planning activities in the marine environment and implementing management measures (Douvere, 2008). Previous studies have provided some information on the distribution and relative abundance of sperm whales in the Mediterranean Sea, but knowledge gaps on their distribution persist (Lewis et al., 2007; Virgili et al., 2019). More generally, heterogeneous data collection in marine environments has resulted in large gaps in our understanding of cetacean distributions in the Mediterranean Sea (Mannocci et al., 2018).
Species distribution models (SDMs) are powerful tools for identifying mechanisms influencing species distribution (Elith and Leathwick, 2009). They allow establishing relationships between the number of detected animals and different environmental variables. They also allow predicting hotspots of densities (Elith and Leathwick, 2009). Among SDMs, generalised additive models (GAMs) are commonly used to model marine mammal distributions and study their habitat preferences as they are able to capture non-linear animal-habitat relationships (Mannocci et al., 2014; Tepsich et al., 2014; Roberts et al., 2016; Virgili et al., 2022). Static variables (e.g., bathymetry, slope) and surface dynamic variables (e.g., chlorophyll-a concentration, net primary production, surface temperature, sea surface height) are commonly considered (Praca et al., 2009; Virgili et al., 2017; Virgili et al., 2019). For species that spend much of their time underwater and feed at great depths on mesopelagic and bathypelagic prey, as is the case for sperm whales, it would seem more relevant to use environmental variables integrated over the water column (Brodie et al., 2018; Virgili et al., 2022). In Virgili et al. (2022), the use of such variables increased the performance and the explanatory power of the models and improved the understanding of the distribution of sperm and beaked whales in the Bay of Biscay. Brodie et al. (2018) found that subsurface dynamic variables (isothermal layer depth, a proxy for the depth of surface mixing, and buoyancy frequency, a proxy for the stratification level of the water column) increased the explanatory power and predictive performance of the models for most species (sharks and swordfishes in the study). Therefore, environmental variables other than surface variables, such as those describing deep oceanographic processes, should also be used.
Most SDMs applied to deep-diving cetaceans are fitted to visual sightings (Roberts et al., 2016; Virgili et al., 2019). The sperm whale, however, can be difficult to observe at the surface because it spends much of its time at depth to forage. Sperm whales produce loud vocalisation, emitting series of regular echolocation clicks during dives (with a repetition rate between 0.5 and 2 clicks per second; Goold, 1995) and while foraging (Whitehead and Weilgart, 1991; Andreas et al., 2022). These clicks can be detected using passive acoustic monitoring (PAM) methods. These methods have been used since the First World War to detect underwater sounds, and it is a technology that has been developed, improved, and used by the scientific community to detect and identify marine mammals (Browning et al., 2017). Pirotta et al. (2020) used PAM methods and acoustic monitoring and showed that passive acoustic methods are valuable for studying the distribution of sperm whales. Poupard et al. (2022) were able to obtain sperm whale acoustic data over several seasons and years, showing the year-round presence of the species in the northwestern Pelagos sanctuary. They also highlight the importance of acoustic monitoring in understanding the distribution and activity of sperm whales in the region. PAM can thus be used to study species that have low availability during visual surveys.
Unlike visual sightings, animals can be detected acoustically in any light and weather conditions (e.g., day or night, or in fog; Marques et al., 2013). Hence, it seems more relevant to use acoustic data than visual data to study sperm whale distribution, as it has been done by Pirotta et al. (2020) in the Balearic Sea.
From June to September 2018, the Agreement on the Conservation of Cetaceans of the Black Sea, Mediterranean Sea and Contiguous Atlantic Area (ACCOBAMS) Survey Initiative (ASI) was conducted throughout the Mediterranean Sea. The aim of the ASI was to obtain new information on the distribution and abundance of cetaceans in the Mediterranean Sea in order to improve and strengthen measures to reduce the impacts of human activities on cetaceans. The survey had an aerial and a boat-based component. The boat-based component used towed hydrophones allowing the study of sperm whale distribution at a large-scale using PAM methods. The aerial component of the ASI also collected sightings of sperm whales but in limited numbers (Panigada et al., this special issue; Cañadas et al., this special issue).
In the present work, we aimed to study the sperm whale summer distribution in the Mediterranean Sea. We modelled sperm whale habitats using towed passive acoustic data and static variables, such as bathymetry, along with dynamic environmental variables integrated over the water column, including inter alia temperature, salinity, currents and chlorophyll-a. First, the detection function and the Effective Strip Widths (ESW) were estimated. Then, we extracted environmental variables and associated them with the effort data. We used GAMs to describe relationships between the number of sperm whales detected acoustically and the different environmental variables as well as to explain the mechanisms leading to a concentration of individuals. We finally predicted sperm whale densities over the whole Mediterranean Sea.
The study area encompassed the entire Mediterranean Sea (from 6°W to 36°E and from 30°N to 46°N). The Mediterranean Sea is a distinctive ecosystem with a relatively small continental shelf and a mostly cyclonic circulation. The water column in the Mediterranean Sea is divided into distinct water masses: (1) the surface waters, which extend between 0 and 200 m in depth, originate from the Atlantic and enter the Mediterranean Sea via the Strait of Gibraltar; (2) underneath, the intermediate Levantine water circulates, on average between 200 and 600 m, this is the warmest and saltiest water of the Mediterranean Sea; (3) below 600 m, the deep waters originate in part from the intermediate Levantine water (Millot and Taupier-Letage, 2005). Even if dives made by sperm whales do not generally exceed 1,500–2,000 m (Amano and Yoshioka, 2003; Irvine et al., 2017; Towers et al., 2019), we chose to consider the entire water column, down to a depth of 4,100 m.
The ASI survey is the first survey carried out at the Mediterranean basin scale for all cetacean species. Acoustic surveys were conducted using hydrophone arrays towed by the R/V Song-of-the-Whale (Figure 1), a 21 m auxiliary-powered cutter-rigged sailing research vessel. A 400 m tow cable, close to the surface at a depth of less than 10 m, was used to isolate the array from any noise generated by the vessel. Acoustic data were collected 24 hours a day to maximise survey effort when water depth was appropriate (greater than 50 m). The vessel maintained a speed of 5 to 8 knots when surveying sperm whales to avoid bias due to animal movement by exceeding the speed of the target animals by 2-3 times (Buckland et al., 2015). The speed of sperm whales can vary between 2 and 6 knots (Arnbom et al., 1987), but they generally maintain an average speed of 2.1 knots (Whitehead, 2018). A pair of AQ-4 elements (Teledyne Benthos) was incorporated into the hydrophone array. They were characterised by a receiving sensitivity of -201 dB re 1V/µPa and a flat frequency response ( ± 1.5 dB) from 1 Hz to 30 kHz. Pre-amplifiers (29 dB gain) were use to prevent voltage-drop between the array and the vessel. A distance of 3 m separated each hydrophone element. A SAIL DAQ card (SA Instrumentation) digitised the array outputs at 48 kHz using a 10 Hz high pass filter and a 12 dB gain added to the signal. A click detector module was used to detect candidate sperm whale clicks in real time using PAMGuard (Gillespie et al., 2008). The click detector identified spectral properties, with most energy at or below 12 kHz (Watkins, 1980; Møhl et al., 2003).
Figure 1 Performed effort transects and acoustic detections of sperm whales collected during the ASI survey in the Mediterranean Sea in 2018. The background map represents the bathymetry (in meters; https://www.emodnet-bathymetry.eu/), black lines represent the transects and pink points the acoustic detections. The numbers are the names of the different regions in the Mediterranean Sea: 1 Alboran Sea; 2 Algerian Basin; 3 Liguro-Provençal Basin; 4 Tyrrhenian Sea; 5 Ionian Basin; 6 Levantine Basin.
A line transect distance sampling protocol was used during the survey and the perpendicular distances for each detection were estimated in PAMGuard using the target motion analysis tool. Sperm whale clicks were identified, analysed and validated by the Marine Conservation Research (MCR; Boisseau et al., this special issue).
The effort transects were linearised and segmented into 10 km segments for which the detection conditions were homogeneous. Each acoustic detection was then attributed to the effort segment on which the animals were detected (Figure 1).
During an at-sea survey, not all animals are detected acoustically; the probability of detecting an animal decreases with the distance from the transect and as detection conditions degrade. A detection function was therefore fitted to the acoustic data using Multiple Covariate Distance Sampling models (MCDS; Miller et al., 2019). The models were fitted considering two key distributions, half normal (hr) and hazard rate (hz) and different detection conditions (sea state, wave height in meters and wind speed in knots). The best model was selected according to the Kolmogorov-Smirnov and Cramer-von Mises goodness-of-fit tests and the Akaike information criterion (AIC; Buckland et al., 1993). The models were fitted using the ‘dfuncEstim’ function of the ‘Rdistance’ package (McDonald et al., 2019) and the two tests mentioned above were performed using the ‘gof_ds’ function of the ‘Distance’ package (Miller et al., 2019). Based on the detection function, ESWs were estimated for each class of the selected detection variable. An ESW is defined so that the number of objects detected beyond this distance is equal to the number of objects not detected before this limit (Buckland et al., 1993). ESWs were calculated using the ‘ESW’ function of the ‘Rdistance’ package (McDonald et al., 2019).
The number of individuals detected was determined by using a click detector module in PAMGuard (Gillespie et al., 2008) which was configured to identify potential sperm whale clicks. Field recordings were independently assessed in PAMGuard by two experienced analysts to identify potential sperm whale click sequences. Candidate clicks were identified when they formed part of a click sequence, characterised by consistent bearings and regularly spaced click intervals. Variations in bearing information were used to distinguish individual click sequences (Lewis et al., 2018). Consequently, acoustic detections were made at the individual level rather than at the group level, allowing the number of detected animals to be determined.
Knowing the ESWs and the number of individuals detected, it was possible to estimate the density of individuals along the transects. This density was then multiplied by the total area sampled during the survey (here 1,312,625 km²) to obtain the total abundance of sperm whales and the associated confidence intervals (Buckland et al., 1993).
In order to determine whether the presence of sperm whales would be related to processes at the surface or in the water column, four depth classes were delimited according to the water masses of the Mediterranean Sea: the surface, between 0 and 200 m, 200 and 600 m, 600 m and 4,100 m. Environmental variables were extracted for each depth class.
We extracted static and dynamic variables from January 1st, 2000 to December 31st, 2021 over the entire Mediterranean Sea with a spatial resolution of 0.042°x0.042° and a monthly temporal resolution (Table 1). We considered variables that can putatively affect the distribution of sperm whales by mediating food availability. Static variables remain stable over time and are independent of depth classes while dynamic variables, which vary with time, were extracted for the four depth classes previously defined.
For static variables, bathymetry was initially extracted from the Emodnet database (https://www.emodnet-bathymetry.eu/). From bathymetry, the slope (in degrees), roughness (in m), slope orientation (in m), and topographic complexity index were calculated using the ‘terrain’ function from the ‘Raster’ package (Hijmans and Van Etten, 2012).
Regarding dynamic variables, temperature, salinity, current velocity, sea surface height, mixing layer depth, chlorophyll-a concentration and net primary production were extracted from Copernicus (https://data.marine.copernicus.eu/products?facets=areas%7EMediterranean+Sea). From these variables, we computed spatial gradients of temperatures and currents, and eddy kinetic energy (EKE = 0.5*(U² + V²), where U and V are the current components). Gradients of temperatures and currents were calculated as the difference between the minimum and maximum temperature values found in the eight pixels surrounding any given pixel of the grid (function ‘detectFronts’ from the R package ‘grec’; Lau-Medrano, 2020). For each depth layer and each variable, climatological means over the 22 years of extracted data and standard deviations were calculated to assess the relevance of the variability of the variables. It means that for each month of the survey, the mean and standard deviation of the month over all years were calculated. Finally, each variable of each depth class was associated with the effort segments (Appendix 1).
In order to determine which environmental variables best explained the distribution of sperm whales in the Mediterranean Sea, GAMs were fitted using the ‘gam’ function of the ‘mgcv’ package (Wood, 2017) with a Tweedie distribution (very close to a Poisson distribution but allowing for some over-dispersion of the data; Foster and Bravington, 2013). We removed combinations of variables with Pearson correlation coefficients higher than |0.5| and tested all models with combinations of one to four variables to avoid excessive complexity (Mannocci et al., 2014). The mean number of individuals per segment was linked to the additive predictors with a log function with four degrees of freedom. An offset equal to segment length multiplied by twice the ESW was included. For each tested model, AIC, explained deviance and Akaike weight were calculated (Burnham and Anderson, 2004; Symonds and Moussalli, 2011). The model with the lowest AIC and the highest explained deviance and Akaike weight was selected.
A prediction grid, which covered the entire Mediterranean Sea, was created for each month of the summer (May to September) with a 0.042° spatial resolution. The selected model was used to predict the density of sperm whales in the whole study area with the ‘predict’ function of the ‘mgcv’ package (Wood, 2017). Finally, the monthly predictions were averaged over the entire survey period and standard error associated with the prediction was calculated to estimate the uncertainty of our prediction.
We conducted a gap analysis on environmental space coverage to identify areas where habitat models could produce reliable predictions outside survey blocks, that is, geographical extrapolation, whilst remaining within the ranges of surveyed conditions for the combinations of covariates selected by the models, that is, areas of environmental interpolation (Mannocci et al., 2018; Virgili et al., 2019; Bouchet et al., 2020). We used the ‘map_extrapolation’ function of the ‘dsmextra’ package (Bouchet et al., 2020). The obtained map was represented according to two conditions, the interpolated data (which varied between 0 and 1) and the extrapolated data (which were lower than -1). The concept of nearest neighbour (percentage of data in close proximity) provides an additional measure of the reliability of extrapolations in a multivariate environmental space (Mannocci et al., 2018; Bouchet et al., 2020). The higher the percentage, the better the prediction.
Finally, for the selected model, the Normalised Root Mean Square Error (NRMSE) was calculated. The NRMSE is an index of the quality of the prediction, it represents the percentage of error related to the prediction (Dirwai et al., 2021).
In total, 13,806 km of transects were conducted by boat during the ASI survey, of which 97.17% were carried out in good conditions (Beaufort sea state ≤ 4). The final dataset included 284 acoustic detections of sperm whales. The majority of these detections were recorded in the western Mediterranean basin and along the Hellenic Trench.
The wind speed variable associated with a hazard rate key function best explained the distribution of sperm whale detections as a function of distance from the transect (Appendix 2, 3). The qqplot suggested a good fit.
As the distance from the transect increased, the probability of detecting sperm whales decreased more rapidly as wind speed increased (Figure 2). Consequently, as the wind speed increased, the estimated ESWs decreased (Figure 3). When the wind speed was < 5 knots, the estimated ESW was 4.88 km, while when the wind was > 25 knots the estimated ESW was 3.14 km.
Figure 2 Probability of detection as a function of the distance from the transect (in km). A hazard rate distribution was fitted to all detection data and a detection function was estimated for each class of wind speed. class 0: from 0 to 5 knots, class 1: from 5 to 10 knots, class 2: from 10 to 15 knots, class 3: from 15 to 20 knots, class 4: from 20 to 25 knots, class 5: above 25 knots.
Figure 3 Effective strip widths and associated 95% confidence intervals estimated for each class of wind speed. Class 0: from 0 to 5 knots, class 1: from 5 to 10 knots, class 2: from 10 to 15 knots, class 3: from 15 to 20 knots, class 4: from 20 to 25 knots, class 5: above 25 knots.
Based on the detection function and the ESWs, the abundance of sperm whales in the surveyed blocks was estimated at 2,959 individuals [2,077 - 4,265]. It was a global estimate produced for the sampled areas of the Mediterranean Sea (i.e., in the Western Mediterranean Sea and in the Ionian Basin).
The selected model explained 38.8% of the deviance and the NRMSE calculation resulted in an error of 5.4%. The highest relative densities were estimated for average gradients of temperature at the surface greater than 0.1°C, a standard deviation of eddy kinetic energy between 200 and 600 m around 0.004 m².s-2, a chlorophyll-a concentration around 0.06 mg.m-3 and a low variation in the standard deviation of the bottom temperature (around 0.1°C; Figure 4).
Figure 4 Relationships obtained between the relative density of sperm whales (individuals per km²) and the explanatory variables of the selected GAM. Solid lines represent the estimated smooth functions and blue shaded regions show the approximate 95% confidence intervals. Sd-BottomT: standard deviation of the bottom temperature; m-CHL-surface: mean concentration of chlorophyll-a in the surface layer; sd-EKE-200-600: standard deviation of the eddy kinetic energy between 200 and 600m; m-GrT-surface: mean temperature gradient at the surface.
The model predicted higher densities of sperm whales in the western Mediterranean basin than in the eastern basin. In the western basin, the most favourable environmental conditions for sperm whales were found in the east of the Corsican coast and in the north of the Balearic Sea, but also in the Liguro-Provençal, Ionian, and Algerian basins where sperm whale densities reached 0.05 individuals.km-². The model predicted average sperm whale densities of around 0.03 individuals.km-² in the entire western Mediterranean basin. Almost no individuals were predicted in the Alboran Sea, the Gulf of Lions and along the Spanish coast (Figure 5). In the eastern basin, the highest densities of sperm whales were predicted in the Ionian basin and along the Hellenic Trench and no individuals were predicted in the Adriatic Sea, the Aegean Sea and the south and the east of the basin. The values of the uncertainties associated with the prediction were very low, mainly around 0.0005 individuals.km-2 in the Liguro-Provençal and Algerian basins where the predictions were the highest.
Figure 5 Mean predicted summer densities of sperm whales over the entire sampling period in individuals.km-² (June to September; A) and the uncertainty associated with the predictions (B).
Predictions were environmentally extrapolated, and should therefore be taken with caution, in the Adriatic Sea, the Levantine Basin and east of Tunisia (Figure 6). They were interpolated everywhere else. The percentage of nearby data reached 25% in the whole of the western Mediterranean Sea and the Ionian Sea south of Italy (Figure 7). The model performs well in all these areas.
Figure 6 Map of extrapolation (in red; the more negative the value, the greater the extrapolation) and interpolation of predictions (in green; the closer the value is to 1, the greater the interpolation). Red areas represent the extrapolation areas where predictions are considered less reliable than in green areas because they were not sampled during the survey. The blue areas represent the absence of information on the extrapolation or interpolation of the data.
Figure 7 Map showing the percentage of nearby data. The areas with the highest percentage of nearby neighbour are the areas with the most reliable predictions because they were properly sampled during the survey. For cells outside the sampled areas, data from neighbouring cells that were sampled are used to inform predictions in the extrapolation areas.
The ASI survey filled gaps in the overall summer distribution of sperm whales in the Mediterranean Sea, as it was the first to be conducted at such a large scale (Mannocci et al., 2018). We obtained maps of the summer distribution with a low error rate.
As sperm whales are deep divers that spend most of their time underwater foraging (dives of up to 138 minutes during foraging; Watwood et al., 2006), they are more easily detected by passive acoustics thanks to the echolocation clicks they emit almost permanently while diving. Indeed, during the vessel survey, only 26 visual detections were recorded compared to 284 acoustic detections. Although the detection rate was ten times higher using acoustic techniques, all animals were not detected because sperm whales typically do not vocalise when at the surface (Watwood et al., 2006; Fais et al., 2016) and/or individuals may have been beyond the detection range of the hydrophone array. On average, sperm whales dive for 45 minutes and emit sounds and clicks for 81% of the dive, making them easy to detect acoustically (Watwood et al., 2006; Fais et al., 2016). Furthermore, as they emit clicks at relatively low frequencies (≤12 kHz), they can be detected over long distances. The ESW was estimated to be between 0.5 and 1.7 km visually (Virgili et al., 2019), whereas acoustically it was estimated to be between 3.14 and 4.88 km. The detection capabilities of the acoustic platform are significantly higher.
The total abundance of sperm whales was estimated at 2,959 individuals [2,077 - 4,265] in the surveyed blocks (i.e., in the Western Mediterranean Sea and in the Ionian Basin). It was similar to or greater than the estimates obtained in previous studies. Lewis et al. (2018) have acoustically detected 194 sperm whales in the entire Mediterranean Sea. The various surveys took place in 2004 in the western basin and spread over several years, 2003, 2007 and 2013 in the entire eastern basin. They estimated an abundance of 1,842 sperm whales [842 – 2,842] in the entire Mediterranean Sea. Poupard et al. (2022) have acoustically detected 422 sperm whales in the Pelagos sanctuary over 147 days of recording. They estimated a density of 0.00169 individuals.km-2 in the sanctuary. Our model predicted average sperm whale densities of 0.03 individuals.km-² throughout the western Mediterranean basin. Gannier (2018) have visually (from 1988 to 2012) and acoustically (since 1994) recorded 157 detections of sperm whales in the western Mediterranean basin. They estimated a population size between 200 and 1,000 individuals in the North-Western Mediterranean Sea.
Using acoustics to detect sperm whales is therefore an effective method to estimate their abundance. In the future, it would be interesting to develop more systematic acoustic surveys when targeting deep divers (mainly sperm whales and Cuvier’s beaked whale Ziphius cavirostris), while continuing to use visual observation for other species.
However, the main limitation associated with the use of acoustic detection is the estimation of the number of individuals (Kimura et al., 2010; Marques et al., 2013). It is difficult to distinguish individuals that emit sounds at similar frequencies in an acoustic recording unless they emit a sound simultaneously. This can lead to an underestimation of the abundance.
In this study, we were able to predict the summer sperm whale distribution throughout the Mediterranean Sea. However, to highlight a change in species distribution throughout the year, the same survey should be conducted at least in winter. Lack of data is a recurrent problem in cetacean habitat modelling; there are very few surveys conducted outside the summer periods creating seasonal gaps (Mannocci et al., 2018). Although we showed that sperm whale detection was influenced by wind speed, the estimated ESW with a wind speed of 25 knots was high (3.14 km), so sperm whales would probably be well detected even in winter conditions. However, detection rates for other species, such as dolphins, may decrease in elevated sea states. Shabangu et al. (2022), for example, suggest that high wind speeds induce a decrease in cetacean acoustic detectability due to increased ambient noise. Such a large-scale survey targeting only sperm whales would therefore not be cost-effective. However, other possibilities exist, such as deploying passive acoustic hydrophones on moorings, buoys, or vessels, which would record sounds throughout the year. Fixed buoys are very effective at assessing the seasonal presence of species at local scales (Mellinger et al., 2007; Stafford et al., 2007). However, they are less suitable for studying animal habitat use, at least at a large scale. This would require the installation of extensive buoy networks, which are very costly and require regular calibration to ensure accurate results (Shabangu and Findlay, 2014). In this case, a large-scale survey using towed acoustics seems more appropriate.
Although we predicted the sperm whale distribution in the entire Mediterranean Sea, the sampling effort was not uniform throughout the area. The gap analysis identified the eastern part of the Mediterranean basin, especially the Levantine basin, as an extrapolation area. This suggests that the sampling effort was not sufficient in this area and a more intensive sampling effort could help better describe the habitat used by sperm whales in the eastern Mediterranean Sea. More research is needed in the east as anthropogenic pressures are high for sperm whales (e.g., ship strike risk in the Hellenic Trench) and therefore we need to improve our understanding of distribution and seasonal variation.
We expected Beaufort sea state to be selected in the detection function because cetacean detection, notably visual detection, is often affected by sea state. Here, the wind speed was selected as the variable most affecting the acoustic detection; the higher the wind speed the lower the detection probability. This is consistent with the fact that Beaufort sea state is a subjective measure of wind speed as perceived by a visual appreciation of the effect of wind on the water surface (Barlow, 2015). Ambient noise, generated by the boat, but also by all processes other than cetacean sounds, would probably be a more important factor to consider when assessing the acoustic detectability of cetaceans. We encourage the systematic recording of ambient noise levels in future acoustic surveys.
We choose to use GAMs in this study because of their ability to handle non-linear and non-monotonic relationships; GAMs are relevant for modelling ecological relationships (Booth and Hammond, 2014). In GAMS, the explained deviance is an indicator of model quality. Deviance is rarely high for cetaceans because the variables used do not fully explain the distribution of animals, as they are proxies of prey distribution. In contrast, a high deviance would have indicated an over-fitting of the relationships to the data. The value of the selected model was 38.8%, which is a good result, similar to other studies (Bailey and Thompson, 2009; Tepsich et al., 2014; Virgili et al., 2017; Virgili, 2018; Virgili et al., 2019). We were rather confident in the model as the explained deviance was quite high but not too high and the NRMSE calculation gave a result of 5.4% error, indicating a low prediction error rate.
The good performance of the model may be related to the use of proxies that are probably more direct than the commonly used surface variables. Environmental variables were separated according to the water masses of the Mediterranean Sea, which were assumed to be homogeneous. However, as sperm whales can dive much deeper than 1,000 m (Whitehead, 2018), we might have considered other depth classes. Particularly, the 600-4,100 m layer may not have been sufficiently precise for a deep-diver that feeds in this layer depth. We might consider splitting the deepest layer to be consistent with the depths used by the sperm whales. However, this would lead to a considerable increase in the number of variables and calculation time, as well as a possible lack of variation between depth classes, as water masses tend to be more homogeneous at greater depths.
In addition, other environmental and biological variables could have been considered. Some studies use other variables to study cetacean distribution such as the distribution and concentration of prey species (Pendleton et al., 2020; Virgili et al., 2021) or canyon areas, because submarine canyons are widely recognised as hotspots in cetacean distribution (Tepsich et al., 2014). Pendleton et al. (2020) suggested that using modelled prey availability, rather than oceanographic proxies, could be important to forecast species distributions. In contrast, in Virgili et al. (2021), the model that used prey distributions obtained from the SEAPODYM model did not accurately model the prey of deep-diving cetaceans, the simulated prey mostly corresponded to the prey of the prey targeted by deep-divers. The SEAPODYM model is a tool for simulating the three-dimensional distributions of prey species in marine ecosystems. It considers various factors such as physical oceanography, prey behaviour, and predator-prey interactions to estimate the spatial distribution of prey. To study the distribution of deep divers, it therefore seems more relevant to use proxies that characterise the water column rather than the distribution of their prey, which is not modelled well enough.
Similarly, it might have been relevant to study anthropogenic pressures such as the impact of noise pollution on sperm whale distribution in order to find out whether these have a direct negative effect on sperm whale distribution. Poupard et al. (2022) analysed the ambient noise recorded by two hydrophones on a 25 m depth buoy to identify the impact of noise pollution (high or low ambient noise) on sperm whales. They showed that sperm whales were present all year round in the Mediterranean Sea, but their abundance decreased when the ambient noise was high due to the presence of ships such as ferries. This is particularly true near the coast where the anthropogenic noise is very high (Buscaino et al., 2016; Pieretti et al., 2020). Poupard et al. (2022) suggested that sperm whales do not come close to ships because ambient noise masks their echolocation when foraging. In addition, many studies have shown that cetaceans would modify their vocalisations in the vicinity of vessels and increase their sound level to maintain acoustic contact with other individuals (Castellote et al., 2012; Melcón et al., 2012; Shabangu et al., 2022).
Sperm whales were predicted in areas associated with high gradients of temperatures at the surface, strong variations in the current velocity between 200 and 600 m, low variations in sea bottom temperatures and rather low chlorophyll-a concentrations in the surface layer. In the literature, sperm whales have often been associated with bathymetric features, such as depth, continental slopes or canyons and seamounts, as well as frontal systems and other mesoscale features such as cyclonic eddies (Virgili et al., 2019; Pirotta et al., 2020). Recently, Virgili et al. (2022) have shown that other parameters influence the distribution of sperm whales at the surface and at depth, such as temperatures and eddies at depth. We also showed that two of the selected variables in the model characterised the water column and not the surface (the standard deviation of the sea floor temperature and the standard deviation of the eddy kinetic energy between 200 and 600 m). For species such as sperm whales, it is therefore relevant to consider the whole environment and not only the surface.
The model predicted average sperm whale densities around 0.03 individuals.km-² in the entire western Mediterranean basin, with maximum densities predicted in the Algerian and Ligurian-Provençal basins, more precisely in the Ligurian Sea and off the Gulf of Lion continental slope. The highest densities were predicted in the protected area of the Pelagos sanctuary (Ligurian Sea). In comparison, Lewis et al. (2018) predicted 2,12.10-3 individuals per km² in the southern part of the western Mediterranean Sea and 0,12.10-3 individuals per km² in the eastern Mediterranean Sea (with, however, high densities in the Aegean Sea, the Hellenic Trench and the northern Ionian Sea). Our predictions in the southern part of the western Mediterranean Sea coincide with their results.
In summer, sperm whales seemed to concentrate mainly in continental slope areas, whether off the Gulf of Lion, the Liguro-Provençal Sea, the Algerian Coast or around Corsica, frequenting depths above 2,000 m (Gannier, 2018; Laran et al., 2018). This suggests the existence of an east-west gradient of sperm whale densities, with higher abundances in the west. Indeed, from summer to autumn, the northern part of the western basin is a major concentration and feeding area for sperm whales (Drouot et al., 2004). Other studies indicate that the presence of sperm whales extends further east along the Hellenic Trench from south-west Kefallonia Island to central south Crete (Frantzis et al., 2014) and even to the Turkish coast as far as the western part of Antalya Bay (Öztürk et al., 2010). From a single survey performed at the scale of the Mediterranean Sea, we were able to predict hotspots observed at more local scales.
The high presence of sperm whales in the southeast of the Gulf of Lions and in the Ligurian Sea could be explained by the presence of numerous canyons (Harris et al., 2014). Submarine canyons act as a connecting corridor between continental shelf areas and deep waters. As a result, they are widely recognised as cetacean concentration areas (Tepsich et al., 2014). The high densities of sperm whales in the Ligurian Sea can be explained by the presence of numerous canyons in this geographical area. A large-scale study revealed the presence of sperm whales along the Hellenic Trench and the west coast of Greece, and in the north-western Mediterranean Sea from the Gulf of Lion to the Ligurian Sea (Lewis et al., 2018), providing support for the model results.
In addition, sperm whales are often observed in the channel between Mallorca and Ibiza, an area characterised by the presence of three seamounts. The Balearic Archipelago is one of the few areas in the Mediterranean Sea where females, juveniles and single males are regularly observed (Pirotta et al., 2020). This is due to the critical role of the area as a breeding and feeding ground for the threatened Mediterranean population (Rendell and Frantzis, 2016).
Sperm whales were predicted to occur in the Ionian Sea (0.03 individuals.km-², mainly off the Italian and Greek coasts), which was consistent with the literature. For example, Lewis et al. (2007); Lewis et al. (2018) found densities 0.24.10-3 individuals.km-² in the Ionian Sea and 0.12.10-3 individuals.km-² in the eastern Mediterranean Sea (similar results to Frantzis et al., 2014). Sperm whales appear to prefer the underwater relief of the sides of the Hellenic Trench at depths between 500 and 1,500 metres (Frantzis et al., 2003). However, densities were comparatively lower than in the western basin. There were two possible explanations for this: either there was less effort in the Ionian Sea, or, as suggested by Lewis et al. (2007), density estimates in the Ionian Sea were underestimated because sperm whales tend to congregate in large groups along the Hellenic Trench, skewing the count results. Even if densities were underestimated, the Ionian Sea appeared to be important for the species as it may be a breeding ground according to Drouot et al. (2004).
The eddy kinetic energy was one of the main variables that explained the distribution of sperm whales in our model. Biggs et al. (2000) showed that sperm whales are mainly distributed in cyclonic eddies, which are proxies for prey distribution. Cyclonic eddies are mesoscale structures that induce a locally increased concentration of plankton in response to the upwelling of nutrient-rich water. The abundance of plankton would induce the strong presence of prey for deep-diving cetaceans, through a trophic cascade mediated by vertically migrating micronekton. Sperm whales are large warm-blooded mammals not physiologically limited by water temperature or other hydrographic features; hence their spatial distribution would be primarily driven by the distribution of their prey, such as squid, supposedly concentrated in cyclonic oases (Biggs et al., 2000). The eddies present at a depth of 200 m can strongly modify the mixing and dispersion of organic matter and thus lead to a concentration of many prey species (Pingree and Le Cann, 1992; Koutsikopoulos and Le Cann, 1996).
Statistic modelling allows the prediction of the number and distribution of animals, their physiological status, demographic rates and interactions between individuals and species (Grimm and Railsback, 2013). This information allows more effective conservation measures to be taken.
The Mediterranean sperm whale population is particularly exposed to various anthropogenic pressures, such as high-intensity noise activities, fishing activities, ship strikes, plastic ingestion, or exposure to chemical pollution (Aguilar et al., 2002; Reeves and Notarbartolo di Sciara, 2006; Notarbartolo-Di-Sciara, 2014). To mitigate the impact of these activities, management measures need to be implemented, based on knowledge of the distribution of sperm whales. Our study shows that in the western basin, sperm whales are found in the east of the Corsican coast and in the north of the Balearic Sea, but also in the Liguro-Provençal, Ionian, and Algerian basins where sperm whale densities reached 0.05 individuals.km-². A high density of sperm whales has been estimated in the Pelagos sanctuary, mainly in the east of Corsica but also in the south of France in the Ligurian Sea, but a large area where sperm whales were predicted is not protected by the sanctuary, mainly south-east of Gulf of Lion. Since this species has been considered endangered by the International Union for Conservation of Nature’s Red List (IUCN; Notarbartolo di Sciara et al., 2012) for several years, it may be relevant to extend this marine protected area to provide better protection. High densities of sperm whales were also predicted in the Balearic Sea. This area became a marine protected area (MPA) in 2018, as it has been recognised as a corridor for cetaceans. In the model, about 0.06 individuals.km-² were predicted in the north of the MPA, which is higher than the average density in the whole area (0.03 individuals.km-²). It would be necessary to extend this MPA to the east to protect sperm whales more effectively.
Even though the MPAs do not fully encompass the distribution areas of sperm whales, three Important Marine Mammals areas (IMMAs) have been identified within these regions: the Northwest Mediterranean Sea IMMA, the Shelf of the Gulf of Lion IMMA, and the Western Ligurian Sea and Genoa Canyon IMMA. These protected areas represent a discrete portion of crucial marine mammal habitat and have the potential to be earmarked and administered for conservation purposes. The identification of IMMAs is intended to raise awareness among policy and decision makers regarding the urgent need to ensure the favourable conservation status of marine mammals in these specific regions through the implementation of appropriate management measures.
However, the model only predicts the summer distribution and not the annual distribution, which may be different in other seasons. It is therefore essential to conduct winter surveys and incorporate the results from these surveys into our results.
We aimed to fill the knowledge gap on sperm whale distribution in the Mediterranean Sea by modelling their habitat using towed acoustic data and static and dynamic environmental variables integrated over the water column. We predicted that sperm whales were mainly distributed in summer off the coast of the north-western Mediterranean basin from the Balearic Islands to the Ligurian Sea and off the Algerian coast. They were present throughout the western Mediterranean Sea and in the northern Ionian Sea. Predicted densities in the eastern part of the Mediterranean Sea were low. This distribution was influenced by several factors, such as the bottom water temperature, eddy kinetic energy at depth, surface temperature gradients and chlorophyll-a concentration. Comparison with the literature showed that their distribution coincides with the presence of canyons. Existing marine protected areas, such as the Pelagos sanctuary, the Balearic MPA and the Strait of Bonifacio generally coincide with predicted hotspots of sperm whale density. These protection zones could be extended to provide optimal protection for the species.
The use of acoustic data compensated for the main difficulty in studying sperm whales, the unavailability of animals at the surface during visual observation and the subsequent lack of data. We encourage more systematic use of towed acoustic data to study the distribution of sperm whales. As the Mediterranean sperm whale sub-population is considered to be closed as a whole, a new survey at the Mediterranean scale in winter could reveal possible changes in the species distribution during the year and enable more appropriate conservation measures to be implemented.
The raw data supporting the conclusions of this article will be made available by the authors, without undue reservation.
Ethical approval was not required for the study involving animals in accordance with the local legislation and institutional requirements because sperm whales were studied using acoustics only.
The present work was designed by CL, AV and VR. OB provided the data. CL and AV conducted the analyses. CL wrote the manuscript. All revision were done by OB, VR and AV. All authors contributed to the article and approved the submitted version.
We are grateful to Marine Research Conservation and the team aboard R/V Song of the Whale who participated in the ASI survey and collected all the data. We thank ACCOBAMS for having organised such a survey and for having provided, with MCR, the data used in the study. We finally thank the Direction Générale de l’Armement Techniques Navales (DGA TN), for funding CL and AV’s research grant. We thank the two reviewers for their helpful comments on the manuscript.
The authors declare that the research was conducted in the absence of any commercial or financial relationships that could be construed as a potential conflict of interest.
All claims expressed in this article are solely those of the authors and do not necessarily represent those of their affiliated organizations, or those of the publisher, the editors and the reviewers. Any product that may be evaluated in this article, or claim that may be made by its manufacturer, is not guaranteed or endorsed by the publisher.
The Supplementary Material for this article can be found online at: https://www.frontiersin.org/articles/10.3389/fmars.2023.1229682/full#supplementary-material
Aguilar A., Borrell A., Reijnders P. J. H. (2002). Geographical and temporal variation in levels of organochlorine contaminants in marine mammals. Mar. Environ. Res. 53, 425–452. doi: 10.1016/S0141-1136(01)00128-3
Amano M., Yoshioka M. (2003). Sperm whale diving behaviour monitored using a suction-cup-attached TDR tag. Mar. Ecol. Prog. Ser. 258, 291–295. doi: 10.3354/meps258291
Andreas J., Beguš G., Bronstein M. M., Diamant R., Delaney D., Gero S., et al. (2022). Toward understanding the communication in sperm whales. iScience 25, 104393. doi: 10.1016/j.isci.2022.104393
Arnbom T., Papastavrou V., Weilgart L. S., Whitehead H. (1987). Sperm whales react to an attack by killer whales. J. Mammalogy 68, 450–453. doi: 10.2307/1381497
Bailey H., Thompson P. M. (2009). Using marine mammal habitat modelling to identify priority conservation zones within a marine protected area. Mar. Ecol. Prog. Ser. 378, 279–287. doi: 10.3354/meps07887
Barlow J. (2015). Inferring trackline detection probabilities, g(0), for cetaceans from apparent densities in different survey conditions. Mar. Mamm. Sci. 31, 923–43. doi: 10.1111/mms.12205
Biggs D., Leben R., Ortega-Ortiz J. (2000). Ship and satellite studies of mesoscale circulation and sperm whale habitats in the northeast gulf of Mexico during GulfCet II. Gulf Mexico Sci. 18, 15–22. doi: 10.18785/goms.1801.02
Booth C. G., Hammond P. S. (2014). A comparison of different techniques for mapping cetacean habitats. Joint Nature Conservation Committee.
Bouchet P. J., Miller D. L., Roberts J. J., Mannocci L., Harris C. M., Thomas L. (2020). dsmextra: Extrapolation assessment tools for density surface models. Methods Ecol. Evol. 11, 1464–1469. doi: 10.1111/2041-210X.13469
Brodie S., Jacox M. G., Bograd S. J., Welch H., Dewar H., Scales K. L., et al. (2018). Integrating dynamic subsurface habitat metrics into species distribution models. Front. Mar. Sci. 5.
Browning E., Gibb R., Glover-Kapfer P., Jones K. E. (2017). Passive acoustic monitoring in ecology and conservation. (Report). WWF-UK. doi: 10.25607/OBP-876
Buckland S., Anderson D., Burnham K., Laake J. (1993). Distance sampling: estimating abundance of biological populations. Biometrics 431, 50. doi: 10.2307/2532812
Buckland S. T., Rexstad E. A., Marques T. A., Oedekoven C. S. (2015). Distance Sampling: Methods and Applications, Methods in Statistical Ecology (Cham: Springer International Publishing). doi: 10.1007/978-3-319-19219-2
Burnham K. P., Anderson D. R. (2004). Multimodel inference: understanding AIC and BIC in model selection. Sociological Methods Res. 33, 261–304. doi: 10.1177/0049124104268644
Buscaino G., Ceraulo M., Pieretti N., Corrias V., Farina A., Filiciotto F., et al. (2016). Temporal patterns in the soundscape of the shallow waters of a Mediterranean marine protected area. Sci. Rep. 6, 34230. doi: 10.1038/srep34230
Castellote M., Clark C. W., Lammers M. O. (2012). Acoustic and behavioural changes by fin whales (Balaenoptera physalus) in response to shipping and airgun noise. Biol. Conserv. 147, 115–122. doi: 10.1016/j.biocon.2011.12.021
Dirwai T. L., Senzanje A., Mabhaudhi T. (2021). Calibration and Evaluation of the FAO AquaCrop Model for Canola (Brassica napus) under Varied Moistube Irrigation Regimes. Agriculture 11, 410. doi: 10.3390/agriculture11050410
Douvere F. (2008). The importance of marine spatial planning in advancing ecosystem-based sea use management. Mar. Policy Role Mar. Spatial Plann. Implementing Ecosystem-based Sea Use Manage. 32, 762–771. doi: 10.1016/j.marpol.2008.03.021
Drouot V., Gannier A., Goold J. C. (2004). Diving and feeding behaviour of sperm whales (Physeter macrocephalus) in the northwestern Mediterranean Sea. Aquat. mammals 30, 419–426. doi: 10.1578/AM.30.3.2004.419
Elith J., Leathwick J. R. (2009). Species distribution models: ecological explanation and prediction across space and time. Annu. Rev. Ecology Evolution Systematics 40, 677–697. doi: 10.1146/annurev.ecolsys.110308.120159
Fais A., Lewis T. P., Zitterbart D. P., Álvarez O., Tejedor A., Soto N. A. (2016). Abundance and distribution of sperm whales in the Canary islands: can sperm whales in the archipelago sustain the current level of ship-strike mortalities? PloS One 11, e0150660. doi: 10.1371/journal.pone.0150660
Foster S. D., Bravington M. V. (2013). A Poisson–Gamma model for analysis of ecological non-negative continuous data. Environ. Ecol. Stat. 20, 533–552. doi: 10.1007/s10651-012-0233-0
Frantzis A., Alexiadou P., Gkikopoulou K. C. (2014). Sperm whale occurrence, site fidelity and population structure along the Hellenic Trench (Greece, Mediterranean Sea). Aquat. Conservation: Mar. Freshw. Ecosyst. 24, 83–102. doi: 10.1002/aqc.2435
Frantzis A., Alexiadou P., Paximadis G., Politi E., Gannier A., Corsini-Foka M. (2003). Current knowledge of the cetacean fauna of the Greek Seas. jcrm 5, 219–232. doi: 10.47536/jcrm.v5i3.801
Gannier A. (2018). Comparaison de l’habitat estival de quatre cétacés teuthophages préférentiels en Méditerranée nord-occidentale. Rev. d’Ecologie Terre Vie 73, 582.
Gillespie D., Mellinger D. K., Gordon J., McLaren D., Redmond P., McHugh R., et al. (2008). “PAMGUARD: semiautomated, open source software for real- time acoustic detection and localisation of Cetaceans,” in Journal of the Acoustical Society of America (Red Hook, NY, USA: Curran Associates), 54–62.
Goold J. C. (1995). Time and frequency domain characteristics of sperm whale clicks. J. Acoustical Soc. America 98 (3), 1279–. doi: 10.1121/1.413465
Grimm V., Railsback S. F. (2013). Individual-based modelling and ecology (Princeton: Princeton University Press).
Harris P. T., Macmillan-Lawler M., Rupp J., Baker E. K. (2014). Geomorphology of the oceans. Mar. Geology 50th Anniversary Special Issue 352, 4–24. doi: 10.1016/j.margeo.2014.01.011
Hijmans R. J., Van Etten J. (2012). Raster: Geographic analysis and modeling with raster data. R package version 2.0-12. Available at: https://CRAN.R-project.org/package=raster.
Irvine L., Palacios D. M., Urbán J., Mate B. (2017). Sperm whale dive behaviour characteristics derived from intermediate-duration archival tag data. Ecol. Evol. 7, 7822–7837. doi: 10.1002/ece3.3322
Kimura S., Akamatsu T., Li S., Dong S., Dong L., Wang K., et al. (2010). Density estimation of Yangtze finless porpoises using passive acoustic sensors and automated click train detection. J. Acoust. Soc Am. 128, 1435. doi: 10.1121/1.3442574
Koutsikopoulos C., Le Cann B. L. (1996). Physical processes and hydrological structures related to the Bay of Biscay anchovy. Scientia Marina 60, 9–19.
Laran S., Delacourtie F., Fulvio T. D., David L., Di-Meglio N., Monestiez P. (2018). Synthèse sur la distribution des cétacés dans le Sanctuaire PELAGOS et les eaux adjacentes, mise en relation avec leur environnement. Sci. Rep. Port-Cros Natl. Park 26, 119–147.
Lau-Medrano W. (2020) grec: Gradient-Based Recognition of Spatial Patterns in Environmental Data. R package version 1.4.1. Available at: https://CRAN.R-project.org/package=grec.
Lewis T., Boisseau O., Danbolt M., Lacey C., Leaper R., Matthews J., et al. (2018). Abundance estimates for sperm whales in the Mediterranean Sea from acoustic line-transect surveys. J. Cetacean Res. Manage. 18, 103–117. doi: 10.47536/jcrm.v18i1.437
Lewis T., Gillespie D., Lacey C., Matthews J., Danbolt M., Leaper R., et al. (2007). Sperm whale abundance estimates from acoustic surveys of the Ionian Sea and Straits of Sicily in 2003. J. Mar. Biol. Ass. 87, 353–357. doi: 10.1017/S0025315407054896
Linnaeus C. (1758). Systema naturae per regna tria naturae, secundum classes, ordines, genera, species, cum characteribus, differentiis, synonymis, locis (10th ed.). Stockholm, Sweden: Laurentius Salvius.
Mannocci L., Catalogna M., Dorémus G., Laran S., Lehodey P., Massart W., et al. (2014). Predicting cetacean and seabird habitats across a productivity gradient in the South Pacific gyre. Prog. Oceanography 120, 383–398. doi: 10.1016/j.pocean.2013.11.005
Mannocci L., Roberts J. J., Halpin P. N., Authier M., Boisseau O., Bradai M. N., et al. (2018). Assessing cetacean surveys throughout the Mediterranean Sea: a gap analysis in environmental space. Sci. Rep. 8, 3126. doi: 10.1038/s41598-018-19842-9
Marques T. A., Thomas L., Martin S. W., Mellinger D. K., Ward J. A., Moretti D. J., et al. (2013). Estimating animal population density using passive acoustics. Biol. Rev. 88, 287–309. doi: 10.1111/brv.12001
McDonald T., Carlisle J., McDonald A. (2019) Rdistance: Distance-Sampling Analyses for Density and Abundance Estimation. R package version 2.1.3. Available at: https://CRAN.R-project.org/package=Rdistance.
Melcón M. L., Cummins A. J., Kerosky S. M., Roche L. K., Wiggins S. M., Hildebrand J. A. (2012). Blue whales respond to anthropogenic noise. PloS One 7, e32681. doi: 10.1371/journal.pone.0032681
Mellinger D. K., Nieukirk S. L., Matsumoto H., Heimlich S. L., Dziak R. P., Haxel J., et al. (2007). Seasonal occurrence of north atlantic right whale (Eubalaena glacialis) vocalizations at two sites on the scotian shelf. Mar. Mammal Sci. 23, 856–867. doi: 10.1111/j.1748-7692.2007.00144.x
Miller D. L., Rexstad E., Thomas L., Marshall L., Laake J. L. (2019). Distance sampling in R. J. Stat. Soft. 89. doi: 10.18637/jss.v089.i01
Millot C., Taupier-Letage I. (2005). “Circulation in the mediterranean sea,” in The Mediterranean Sea, Handbook of Environmental Chemistry. Ed. Saliot A. (Berlin, Heidelberg: Springer Berlin Heidelberg), 29–66. doi: 10.1007/b107143
Møhl B., Wahlberg M., Madsen P. T., Heerfordt A., Lund A. (2003). The monopulsed nature of sperm whale clicks. J. Acoustical Soc. America 114, 1143–1154. doi: 10.1121/1.1586258
Notarbartolo-Di-Sciara G. (2014). Sperm whales, Physeter macrocephalus, in the Mediterranean Sea: a summary of status, threats, and conservation recommendations. Aquat. Conservation: Mar. Freshw. Ecosyst. 24, 4–10. doi: 10.1002/aqc.2409
Notarbartolo di Sciara G., Frantzis A., Bearzi G., Reeves R. (2012). Physeter macrocephalus. The IUCN red list of threatened species.
Öztürk A. A., Tonay A. M., Dede A., Öztürk A. (2010). “Sperm whale sightings in the Turkish part of the Aegean and Mediterranean Sea,” in Abstract book: 24th annual conference of the European cetacean society (Stralsund, Germany: European Cetacean Society), 175.
Pendleton D. E., Holmes E. E., Redfern J., Zhang J. (2020). Using modelled prey to predict the distribution of a highly mobile marine mammal. Diversity Distributions 26, 1612–1626. doi: 10.1111/ddi.13149
Pieretti N., Lo Martire M., Corinaldesi C., Musco L., Dell’Anno A., Danovaro R. (2020). Anthropogenic noise and biological sounds in a heavily industrialised coastal area (Gulf of Naples, Mediterranean Sea). Mar. Environ. Res. 159, 105002. doi: 10.1016/j.marenvres.2020.105002
Pingree R. D., Le Cann B. (1992). Three anticyclonic slope water oceanic eDDIES (SWODDIES) in the Southern Bay of Biscay in 1990. Deep Sea Res. Part A. Oceanographic Res. Papers 39, 1147–1175. doi: 10.1016/0198-0149(92)90062-X
Pirotta E., Brotons J. M., Cerdà M., Bakkers S., Rendell L. E. (2020). Multi-scale analysis reveals changing distribution patterns and the influence of social structure on the habitat use of an endangered marine predator, the sperm whale Physeter macrocephalus in the Western Mediterranean Sea. Deep Sea Res. Part I: Oceanographic Res. Papers 155, 103169. doi: 10.1016/j.dsr.2019.103169
Poupard M., Ferrari M., Best P., Glotin H. (2022). Passive acoustic monitoring of sperm whales and anthropogenic noise using stereophonic recordings in the Mediterranean Sea, North West Pelagos Sanctuary. Sci. Rep. 12, 2007. doi: 10.1038/s41598-022-05917-1
Praca E., Gannier A., Das K., Laran S. (2009). Modelling the habitat suitability of cetaceans: Example of the sperm whale in the northwestern Mediterranean Sea. Deep Sea Res. Part I: Oceanographic Res. Papers 56, 648–657. doi: 10.1016/j.dsr.2008.11.001
Reeves R. R., Notarbartolo di Sciara G. (2006). The status and distribution of cetaceans in the Black Sea and Mediterranean Sea.
Rendell L., Frantzis A. (2016). “Chapter two - mediterranean sperm whales, physeter macrocephalus: the precarious state of a lost tribe,” in Advances in Marine Biology, Mediterranean Marine Mammal Ecology and Conservation. Eds. Notarbartolo Di Sciara G., Podestà M., Curry B. E. (Cambridge, MA: Academic Press), 37–74. doi: 10.1016/bs.amb.2016.08.001
Roberts J. J., Best B. D., Mannocci L., Fujioka E., Halpin P. N., Palka D. L., et al. (2016). Habitat-based cetacean density models for the U.S. Atlantic Gulf Mexico. Sci. Rep. 6, 22615. doi: 10.1038/srep22615
Shabangu F., Findlay K. (2014). Passive acoustic monitoring of marine mammals in South Africa, with special reference to Antarctic blue whales. Marine and Maritime Sectors 153–173.
Shabangu F. W., Yemane D., Best G., Estabrook B. J. (2022). Acoustic detectability of whales amidst underwater noise off the west coast of South Africa. Mar. pollut. Bull. 184, 114122. doi: 10.1016/j.marpolbul.2022.114122
Stafford K. M., Mellinger D. K., Moore S. E., Fox C. G. (2007). Seasonal variability and detection range modeling of baleen whale calls in the Gulf of Alaska 1999–2002. J. Acoustical Soc. America 122, 3378–3390. doi: 10.1121/1.2799905
Symonds M. R. E., Moussalli A. (2011). A brief guide to model selection, multimodel inference and model averaging in behavioural ecology using Akaike’s information criterion. Behav. Ecol. Sociobiol. 65, 13–21. doi: 10.1007/s00265-010-1037-6
Tepsich P., Rosso M., Halpin P., Moulins A. (2014). Habitat preferences of two deep-diving cetacean species in the northern Ligurian Sea. Mar. Ecol. Prog. Ser. 508, 247–260. doi: 10.3354/meps10851
Towers J. R., Tixier P., Ross K. A., Bennett J., Arnould J. P. Y., Pitman R. L., et al. (2019). Movements and dive behaviour of a toothfish-depredating killer and sperm whale. ICES J. Mar. Sci. 76, 298–311. doi: 10.1093/icesjms/fsy118
Virgili A. (2018). Modelling distributions of rare marine species: the deep-diving cetaceans. PhD Thesis. La Rochelle, France: University of La Rochelle.
Virgili A., Authier M., Boisseau O., Cañadas A., Claridge D., Cole T., et al. (2019). Combining multiple visual surveys to model the habitat of deep-diving cetaceans at the basin scale: Large-scale modelling of deep-diving cetacean habitats. Global Ecol. Biogeogr 28, 300–314. doi: 10.1111/geb.12850
Virgili A., Hedon L., Authier M., Calmettes B., Claridge D., Cole T., et al. (2021). Towards a better characterisation of deep-diving whales’ distributions by using prey distribution model outputs? PloS One 16, e0255667. doi: 10.1371/journal.pone.0255667
Virgili A., Racine M., Authier M., Monestiez P., Ridoux V. (2017). Comparison of habitat models for scarcely detected species. Ecol. Model. 346, 88–98. doi: 10.1016/j.ecolmodel.2016.12.013
Virgili A., Teillard V., Dorémus G., Dunn T. E., Laran S., Lewis M., et al. (2022). Deep ocean drivers better explain habitat preferences of sperm whales Physeter macrocephalus than beaked whales in the Bay of Biscay. Sci. Rep. 12, 9620. doi: 10.1038/s41598-022-13546-x
Watkins W. A. (1980). “Acoustics and the behaviour of sperm whales,” in Animal Sonar Systems, NATO Advanced Study Institutes Series. Eds. Busnel R.-G., Fish J. F. (Boston, MA: Springer US), 283–290. doi: 10.1007/978-1-4684-7254-7_11
Watwood S. L., Miller P. J. O., Johnson M., Madsen P. T., Tyack P. L. (2006). Deep-diving foraging behaviour of sperm whales (Physeter macrocephalus). J. Anim. Ecol. 75, 814–825. doi: 10.1111/j.1365-2656.2006.01101.x
Whitehead H. (2018). “Sperm whale: physeter macrocephalus,” in Encyclopedia of Marine Mammals, 3rd ed. Eds. Würsig B., Thewissen J. G. M., Kovacs K. M. (Cambridge, MA: Academic Press), 919–925. doi: 10.1016/B978-0-12-804327-1.00242-9
Whitehead H., Weilgart L. (1991). Patterns of visually observable behaviour and vocalisations in groups of female sperm whales. Behaviour 118, 275–296.
Keywords: species distribution model, Mediterranean Sea, passive acoustic monitoring, sperm whale distribution, Physeter macrocephalus
Citation: Lerebourg C, Boisseau O, Ridoux V and Virgili A (2023) Summer distribution of the Mediterranean sperm whale: insights from the acoustic Accobams survey initiative. Front. Mar. Sci. 10:1229682. doi: 10.3389/fmars.2023.1229682
Received: 26 May 2023; Accepted: 09 October 2023;
Published: 20 October 2023.
Edited by:
Roberto Carlucci, University of Bari Aldo Moro, ItalyReviewed by:
Giulia Cipriano, University of Bari Aldo Moro, ItalyCopyright © 2023 Lerebourg, Boisseau, Ridoux and Virgili. This is an open-access article distributed under the terms of the Creative Commons Attribution License (CC BY). The use, distribution or reproduction in other forums is permitted, provided the original author(s) and the copyright owner(s) are credited and that the original publication in this journal is cited, in accordance with accepted academic practice. No use, distribution or reproduction is permitted which does not comply with these terms.
*Correspondence: Clara Lerebourg, Y2xhcmFhLmxlcmVib3VyZ0BnbWFpbC5jb20=
†Present address: Auriane Virgili, Share the Ocean, Larmor Baden, France
Disclaimer: All claims expressed in this article are solely those of the authors and do not necessarily represent those of their affiliated organizations, or those of the publisher, the editors and the reviewers. Any product that may be evaluated in this article or claim that may be made by its manufacturer is not guaranteed or endorsed by the publisher.
Research integrity at Frontiers
Learn more about the work of our research integrity team to safeguard the quality of each article we publish.