- Department of Oceanography, College of Natural Sciences, Chonnam National University, Gwangju, Republic of Korea
This study examined planktonic protists—microbial eukaryotes with cell sizes between 2 and 200 µm—to understand their diversity, assembly processes, and co-existence patterns in surface waters of the Jeju Strait (JS), Korea. Using small organelle-enriched metagenomics, the study identified 252 operational taxonomic units (OTUs) across three distinct oceanographic regions of the JS during multiple seasons. Interestingly, there were no significant variations in the diversity or phylogenetic structures of these planktonic protist communities (PPCs), indicating a uniform distribution of PPCs across the strait. However, a higher clustering of their phylogenetic structure was observed in November, implying that seasonal environmental factors influenced their assembly. The study also discovered that seasonal shifts had a major impact on the composition of these communities. For example, Myzozoa was more prevalent in March, while Ochrophyta dominated in November. Protist size also varied seasonally, with larger protists increasing from June to November and then decreasing by March. Neutral community model analysis provided insights into the role of stochastic processes in shaping community assembly. The majority of OTUs fell into the neutral category, while a smaller proportion displayed non-neutral patterns. For instance, certain species like Pseudo-nitzschia and Chaetoceros were frequently found but had lower abundance, while other species like Alexandrium and Protoceratium were less frequent but more abundant. The feeding patterns, or trophic modes, of these protists also showed variations. Additionally, the study unveiled a complex network of species interactions within the strait, including positive mutualistic relationships and negative competitive interactions. The intricate interaction network consisted of 61 distinct modules, suggesting the presence of specialized subgroups within the PPCs that fulfill unique ecological roles. These subgroups contribute significantly to the overall stability and resilience of the ecosystem.
Introduction
The Jeju Strait (JS) is a 90 km long stretch of water located between the Korean mainland and Jeju Island. The Kuroshio Current System influences this strait, which is divides into the Western Kuroshio Branch and the Eastern Kuroshio Branch. These branches further divided into various currents, including the Tsushima Warm Current (TWC), and the Jeju Warm Current (JWC) (Lie and Cho, 1997). The JS links the Yellow Sea and the East China Sea, forming part of the Korea Strait (also known as the South Sea of Korea). Within the JS, water masses with different temperatures and salinities flow and interact, creating a complex hydrodynamic environment (Chang et al., 2004). The temperature and salinity in the JS can be greatly affected by seasonal changes. During winter, lower current shear and a mixed layer are induced, while in summer, warmer and less saline surface water leads to stratification and higher surface current velocities (Shin et al., 2022). The unique current system of the JS also facilitates the development of thermohaline fronts (Kim et al., 2022).
The JS is useful for studying ecological phenomena, such as the annual red tides caused by both phytoplankton and seasonal environmental fluctuations. The JS also experiences human-induced disturbances and hosts blooms of various planktonic species (Lee et al., 2020; Won et al., 2021; Min and Kim, 2023). Despite being in a temperate region, the JS is a transition area between temperate and subtropical zones, based on water temperature and the presence of tropical/subtropical species (Kim et al., 2020a; Lee et al., 2023). Understanding the processes shaping the JS’s plankton communities is crucial because of its role as a biological corridor for migrating species, as well as its oceanographic dynamics, making it an ideal model for studying biogeochemical processes (Kim and Lee, 2022).
Marine protists, which are diverse organisms that significantly contribute to marine primary production and biogeochemical cycles, support food webs and influence local ecosystems through various behaviors and interactions, playing a vital role in regulating the marine carbon cycle (Caron et al., 2012; Lima-Mendez et al., 2015; Seeleuthner et al., 2018; Glibert and Mitra, 2022; Lim and Jeong, 2022). Pico- and nano-plankton, play a significant role in the global carbon cycle. However, studying these tiny protists is challenging due to their small size and non-distinctive morphology (Eom et al., 2021). The emergence of high-throughput DNA sequencing has enhanced our understanding of marine protist diversity and species abundance (Lee et al., 2021a; Ok et al., 2021; Gutiérrez-Rodríguez et al., 2022; Min and Kim, 2022; Jin et al., 2023). In this study, small organelle-enriched metagenomics (SoEM) was used to investigate the composition and distribution of planktonic protist communities (PPCs) in the JS, a largely unexplored region in the eastern part of the Korea Strait bordering the northeastern East China Sea. As climate change increasingly impacts the physical and chemical status of the oceans, gaining insights into the biogeography and distribution of such communities is becoming increasingly important (de Vargas et al., 2015; Lee et al., 2021b; Ok et al., 2022).
Ecological community assembly is influenced by niche theory, which suggests that species co-occurrence via interactions with each other and their environment (Hutchinson, 1957), and neutral theory, which posits that community composition is determined by random processes (Chase and Myers, 2011). The four main processes shaping community assembly are selection, dispersal, speciation, and ecological drift (Vellend and Agrawal, 2010; Stegen et al., 2012; Zhou and Ning, 2017). Recent studies have explored the relative importance of these processes in different ecosystems, including soil, freshwater, and marine environments (Stegen et al., 2012; Aguilar and Sommaruga, 2020; Skouroliakou et al., 2022). In PPCs, both deterministic (selection) and stochastic (neutral theory) processes influence assembly (Ramond et al., 2021; Skouroliakou et al., 2022). Diverse microbial assemblies can create spatial patterns, and varying dispersal levels can disrupt selection, thereby shaping the complex nature of PPCs (Leibold et al., 2004; Heino et al., 2015).
This study explores the diversity, distribution, and assembly processes of planktonic protists in the JS by leveraging high-resolution metagenomic sequencing data and environmental parameters. The aim is to scrutinize PPCs across different water masses and seasons to pinpoint the factors impacting protist diversity and co-occurrence. Specifically, the study will focus on the alpha diversity (phylogenetic structure) and beta diversity (phylogenetic turnover) of the smallest eukaryotic members of the JS surface ocean. The expected outcomes will shed light on the role of environmental filtering in shaping protist communities, as well as the diverse factors that influence their diversity and co-occurrence. Moreover, it is hoped that the results from the null model and neutral community model analysis will enhance comprehension of planktonic protist assembly mechanisms. This research is significant as relatively few studies have been carried out to investigate microbial eukaryotic diversity and community assembly processes in the JS, particularly in the northeastern East China Sea.
Materials and methods
Study stations and surface plankton sampling
Environmental variables in the JS were measured at eight stations during four sampling periods: June, September, and November 2017, and March 2018. In addition, surface seawater samples were collected specifically for the analysis of planktonic protists, with three of the eight stations chosen for this purpose (Figure 1A). These stations were located in three distinct regions: the shallow-water (S) region adjacent to the Korean peninsula, the middle region of the strait where a seasonal thermohaline front (F) is present, and the deep-water (D) region near Jeju Island. However, due to adverse weather conditions, we were unable to operate the research vessel (R/V) between December and February as originally planned.
At each site, approximately one ton of surface seawater was collected using a submerged pump with a flow rate of 70 liters per minute. The collected seawater was subjected to a filtration process for the harvesting of the planktonic protists. Initially, the seawater was pre-filtered through a 200 μm mesh to eliminate large organisms and debris. Then, it was further filtered through a 1 μm Nylon conical-shaped net, which retain the planktonic protists while allowing excess water to pass through. The filtrate fractions were transferred to 50 mL conical tubes and centrifuged at 2,500×g for 30 min. This centrifugation step effectively removes any residual seawater and resulted in a pellet of concentrated plankton. The pellet was immediately frozen and stored in liquid nitrogen until genomic DNA (gDNA) extraction, in order to preserve the DNA quality as much as possible.
Measurement of environmental variables
Temperature and salinity profiles were recorded using a CTD probe (Sea-Bird Electronics, WA, USA). Seawater samples from various depths were collected using Niskin bottles attached to a CTD-Rosset sampler. Nutrients analysis, including dissolved inorganic nitrogen (DIN), dissolved inorganic phosphorus (DIP), and dissolved inorganic silicate (DISi), was conducted using water samples obtained from multiple stations at depths ranging from 0 to 125 m. To assess pH levels, water samples were taken from the surface, middle, and bottom. Total chlorophyll a (TChl a) concentrations were specifically determined from water samples collected at depths of 0, 10, and 30 m. To assess the nutrient levels in seawater, a 100 mL sample was filtered through a 0.45 μm PTFE syringe filter (Advantec, Japan) and stored at -20°C. The sample was later measured using an auto-analyzer (Quattro, Seal Analytical, Norderstedt, Germany). The pH of the seawater samples was measured at 25°C using spectrophotometry with the m-cresol purple indicator, following established procedures described by Clayton and Byrne (1993). In addition, the TChl a concentration was determined using a chlorophyll a fluorometer (ECO-AFL/FL, Sea-Bird Electronics, WA, USA) attached to a CTD probe. The fluorescence measurements were converted to TChl a concentration using a regression method based on the relationship between fluorescence and TChl a, which was established using high-performance liquid chromatography (HPLC) as the reference method.
DNA extraction and sequencing
The small organelle-enriched metagenome pioneer version (SoEM-pv) method, as validated by Jin et al. (2023), was used to identify marine plankton via environmental DNA (eDNA). DNA extraction involved homogenizing the pellet sample in a buffer of 250 mM sucrose, 30 mM Tris-HCl, 10 mM EDTA, and pH 7.5, using an IKA-T10 homogenizer (IKA, Staufen, Germany). Small organelles were concentrated from the homogenate through a modified differential centrifugation method (Jo et al., 2019) and the DNA was extracted using the Qiagen DNeasy Blood/Tissue Kit (Qiagen, Hilden, Germany) following the manufacturer’s instructions.
For library construction, the TruSeq Nano DNA kit (Illumina, CA, USA) was used, fragmenting approximately 200 ng of DNA to a 550 bp insertion size with a LE220 Focused-ultrasonicator (Covaris, MA, USA). The DNA fragments underwent end repair, the addition of a single “A” base at the 3’ end, and ligation of the Illumina adapters. The libraries were purified and template size distribution analysis was performed using the Agilent Technologies 2100 Bioanalyzer and a DNA 1000 Chip (Agilent Technologies, CA, USA). Quantification used qPCR following the protocol guide for Illumina sequencing platforms. Paired-end sequencing (2 × 301 bp) was carried out on the Illumina Miseq platform by Macrogen Inc (Seoul, Korea). The raw sequencing data were deposited in the NCBI SRA database under the accession numbers from SRR24790320 to SRR24790330, and under the NCBI Bioproject accession number PRJNA974865.
Bioinformatics and data processing
We conducted sequence analyses by modifying the bioinformatics analysis pipeline of SoEM-pv (Jo et al., 2019). Raw sequences were preprocessed with Trimmomatic v.0.39 to exclude adapter sequences and low-quality reads below the Phred score average of 33 (Bolger et al., 2014). The paired-end reads were merged using FLASH v1.2.11, with a minimum overlap of 20 bp (Magoč and Salzberg, 2011).
Operational taxonomic units (OTUs) were identified from merged sequences using a BLAST search against the non-redundant nucleotide database (accessed November 11, 2021, from https://www.ncbi.nlm.nih.gov/nucleotide/) with an E-value under 1e-10 and a query sequence length of at least 400 bp. Confident OTUs were selected based on a minimum of five read counts. The taxonomic identification was obtained by assigning all taxonomic ranks from kingdom to species using the NCBI taxonomy database. Super-kingdoms other than eukaryotes were discarded as part of this process (Jin et al., 2023).
Taxonomic classification and inhabitance (i.e., terrestrial, fresh, brackish, and marine) of each OTU were identified using the scientific name and the World Register of Marine Species (WoRMS) database with the worrms package v.0.4.2 (Chamberlain, 2020). To focus on protists, the supergroups of SAR, Excavata, and Amoebozoa were intentionally selected (Adl et al., 2012). Non-marine OTUs that were assigned by WoRMS, were removed. Functional traits of 252 OTUs were assigned to two size groups (nano-size 2–20 μm and micro-size 20–200 μm) and six trophic modes (obligate photoautotroph (OPA), heterotroph (HET), constitutive mixotroph (CM), generalist non-constitutive mixotroph (GNCM), endosymbiotic specialist (eSNCM) and plastidic specialist non-constitutive mixotroph (pSNCM)) (Flynn et al., 2019). The assignments were made using Ramond et al. (2019)’s functional traits database of marine protists. Such functional traits can give insights into the potential roles and ecological functions of the planktonic protist community (PPC) in the JS.
Statistical analysis
We determined that our sampling had sufficient sequence depth based on the rarefaction curves of each sample, but we noted significant variation in sequence depth (Figure S1). To avoid false negative error, we did not rarefy (McMurdie and Holmes, 2014), but instead normalized individual OTU read counts by relative abundance for downstream analysis.
We calculated alpha diversity indices, such as species richness and the Shannon-Wiener index of diversity, using the vegan package v.2.6.4 (Oksanen et al., 2022). Faith’s phylogenetic diversity (PD) and the net relatedness index (NRI) were computed using the picante package v.1.8.2 (Kembel et al., 2010). The phylogenetic tree was created with the phylostratr package v.0.2.1 (Arendsee et al., 2019), and all branch lengths were set to “1” using the “compute.brlen” function in the ape package v.5.7.1 (Paradis and Schliep, 2019).
To identify statistical differences among seasons and stations, we performed an analysis of variance (ANOVA) or a Kruskal-Wallis H tests, with post hoc multiple comparisons based on the Bonferroni test. Non-metric multidimensional scaling (NMDS) and analysis of similarity (ANOSIM) were used to assess differences in PPC using the Jaccard distance to estimate community dissimilarities. Principal component analysis (PCA) and ANOVA were employed to examine variations in environmental conditions across stations and seasons. We utilized several R packages, including phyloseq, dplyr, lawstat, and ggplot2, in R v.4.2.2 for data analysis and visualization (McMurdie and Holmes, 2013; Wickham et al., 2023; R Core Team, 2022).
Community assembly processes
The neutral community model (NCM) was employed to evaluate the contribution of stochastic processes to the assembly of PPCs in the JS. The MicEco package v.0.9.19 (Russel, 2023) was used to fit the NCM by predicting the relationship between the occurrence frequency and their relative abundance of PPCs. The parameter Nm is an estimate of the dispersal between communities, with N representing the metacommunity size (i.e., total number of reads), and m representing the migration rate. OTUs falling above and below the 95% confidence intervals of NCM were assumed to be non-stochastically distributed.
To assess the assembly processes of three NCM prediction groups, a framework based on phylogenetic and null model analyses was employed, as described by Stegen et al. (2013). We measured variations in the phylogenetic turnover index and taxonomic turnover index using the β-nearest taxon index (βNTI) and Bray-Curtis-based Raup-Crick (RCBray), respectively. These were calculated using the picante package v.1.8.2 (Kembel et al., 2010) and the “raup_crick” function (Stegen et al., 2013). A value of |βNTI| > 2 indicated the dominance of deterministic processes; a value of βNTI < -2 indicated homogeneous selection with significantly less phylogenetic turnover than expected; βNTI > 2 indicated heterogeneous selection with significantly more phylogenetic turnover than expected. In cases where |βNTI| < 2, RCBray > 0.95 and RCBray < -0.95 implied the relative influences of dispersal limitation and homogeneous dispersal, respectively. A value of |RCBray| < 0.95 implied the influence of an “undominated” fraction (Zhou and Ning, 2017).
Co-occurrence network analysis
To study inter-species relationships, we conducted a network analysis of the PPCs using the SparCC approach, which accurately infers correlation from compositional data, particularly with small sample sizes (Friedman and Alm, 2012; Kurtz et al., 2015). We used the “sparcc” function in the SpiecEasi package v.1.1.2 (Kurtz et al., 2015) for network inference, only retaining edges with correlation magnitudes > 0.7 and statistical significance < 0.01, drawn with the igraph package v.1.4.1 (Csardi and Nepusz, 2006). We visualized the network and calculated topological properties such as clustering coefficient, average path length, and centrality using Gephi software v.0.10.1 (Bastian et al., 2009). Individual nodes in the plankton network were categorized into a network hub, module hub, connector, and peripheral, based on their topological roles (Guimera and Nunes Amaral, 2005).
To determine module transition patterns along seasonal change, we normalized and averaged the relative abundance of OTUs in each module (Chun et al., 2019). We evaluated the interaction between modules and seven environmental variables using a partial Mantel test, with a significance level estimated by 9999 permutations using the “mantel.partial” function in the vegan v.2.6.4 software package (Oksanen et al., 2022). We measured community dissimilarity using the Bray–Curtis distance and regressed it against a pairwise Euclidean distance of environmental variables using Spearman’s correlations. In the network, a module is a group of species with similar trait sets that potentially form coevolutionary units.
Results
Variation of environmental conditions
Consistent mixing was observed throughout all seasons at the shallow-water (S) station in the JS. In contrast, strong stratification was observed in September at the thermohaline front (F) and deep-water (D) stations displayed (Figures 1B, C). There were no significant differences in the environmental variables between spatial locations (Table S1). However, in November, distinct environmental conditions were observed near the F station due to a thermohaline front (Figures 1B–D). However, the strait experienced significant changes in environmental conditions over time (Figure S2). Surface water temperature peaked in September (26.33 ± 0.86°C) and dropped to its lowest in March (13.58 ± 1.14°C). Conversely, salinity showed an opposing trend, with values ranging from 30.49 to 34.41 (Figures 1C; S2). DIP and DISi were inversely correlated with temperature and displayed significant correlations (r = -0.705, -0.727, p < 0.05); however, DIN did not show a significant correlation (r = -0.589, p = 0.057). In contrast, pH had a significant positive correlation with temperature (r = 0.909, p < 0.001).
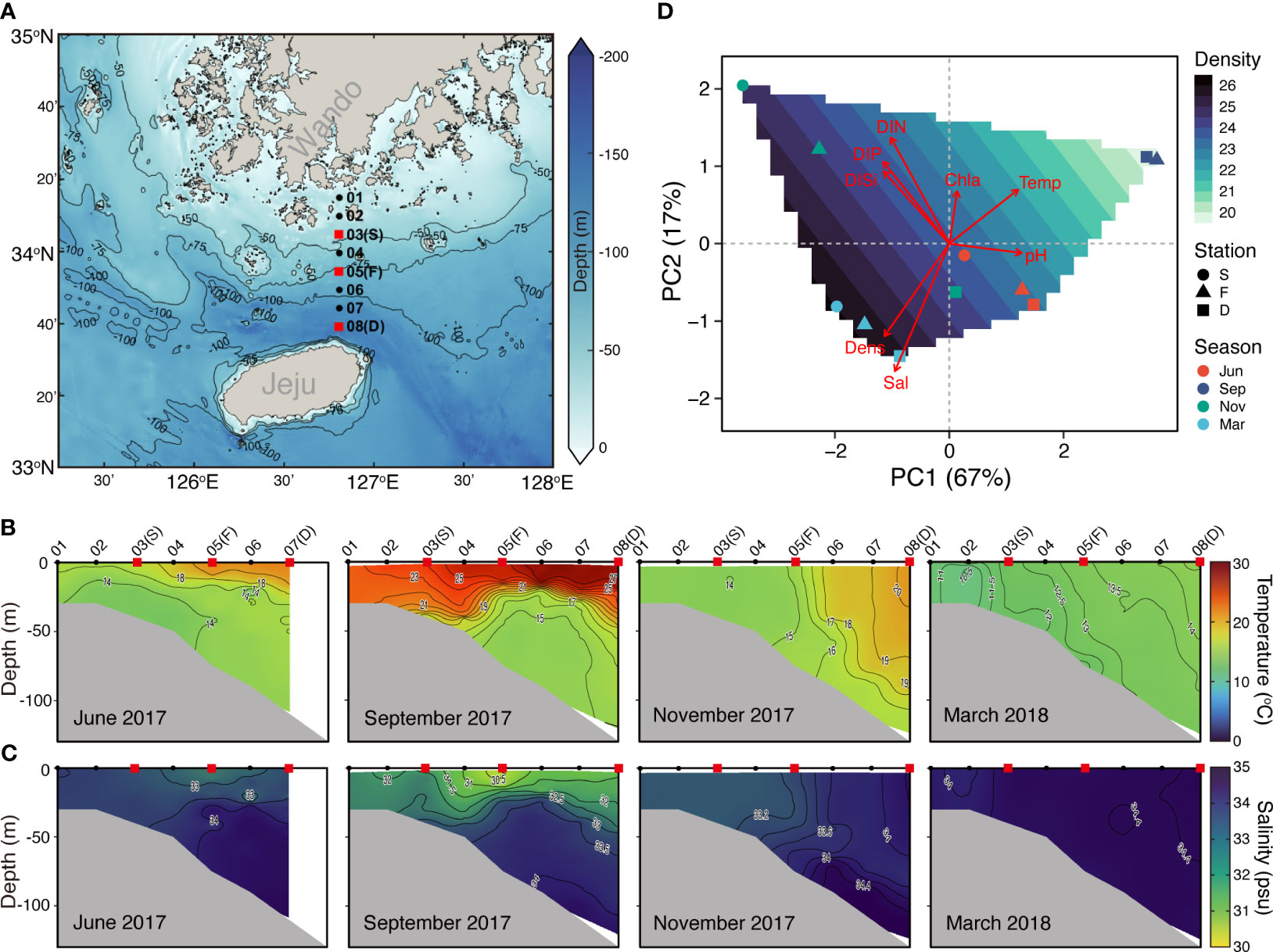
Figure 1 Overview of the Jeju Strait (JS) environmental conditions and sampling stations. (A) Map of sampling stations and depths sourced from the GEBCO gridded bathymetry data (accessed April, 5, 2023 from https://www.gebco.net/data_and_products/gridded_bathymetry_data/): The red squares represent surface water sampling stations, including shallow-water (S, average depth 44 m), thermohaline front (T, 78 m), and deep-water (D, 125 m) stations for planktonic protist community and environmental variable analysis. The black dots indicate stations solely for environmental variable measurement. (B) Vertical distribution of temperature along a transect across the JS from June 2017 to March 2018. (C) Vertical distribution of salinity along the same transect across the JS from June 2017 to March 2018. (D) Principal component analysis (PCA) plot of spatiotemporal changes: The symbols represent planktonic protist communities at JS stations across four seasons. The background contours depict water density. The red arrows indicate the contribution of environmental variables (e.g., temperature (Temp), salinity (Sal), water density (Den), dissolved inorganic nitrogen (DIN), dissolved inorganic phosphate (DIP), dissolved inorganic silicate (DISi), chlorophyll a (Chla), and pH to the first two principal components.
Spatiotemporal pattern and phylogenetic structure of PPC
Preprocessing the Illumina sequencing of small organelle-enriched DNA molecules (as per section 2.3.) revealed a total of 252 protistan OTUs from the surface water of three stations in the JS. The taxonomic and phylogenetic diversity of the PPC did not significant vary with spatial locations or temporal changes. However, the indices of phylogenetic structure differed (Figure 2). In November, NRI was higher (p = 0.052) compared with other months, and Faith’s PD was somewhat lower, indicating a more clustered phylogenetic structure and less phylogenetic diversity in the PPC. During November, the PPC demonstrated a more clustered phylogenetic structure (NRI > 0, Figure 2D), implying that environmental filtering played a significant role in PPC structuring. This suggests that specific environmental factors selected certain protist species, leading to a less diverse community (see to Table 1 in Skouroliakou et al., 2022). The NRI values showed weaker phylogenetic clustering in June and March, indicating less environmental filtering and a more diverse PPC during these seasons.
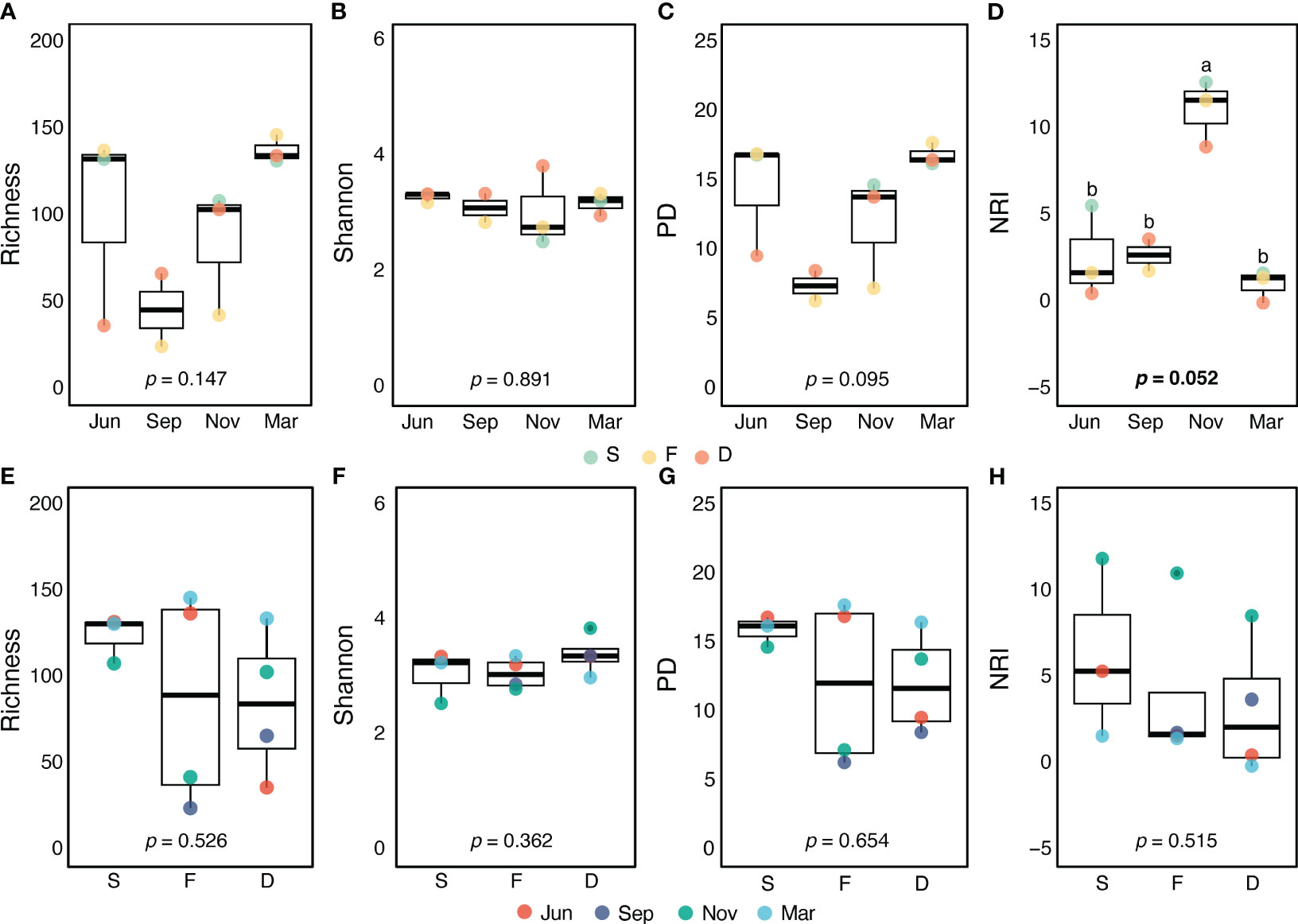
Figure 2 Comparison of alpha diversity indices across four seasons (A–D) or three stations (E–H). Statistical significance (p-value) was determined to assess differences in protistan species richness (A, E) and Shannon diversity or Shannon-Wiener index (B, F) across seasons and stations using ANOVA. Faith’s phylogenetic diversity (PD) (C, G) and the net relatedness index (NRI) (D, H) were evaluated using the Kruskal-Wallis H test. Post hoc multiple comparison results based on the Bonferroni test are indicated by small letters. Each colored dot represents the value of a sample. The solid black lines represent the median, while the black dots represent the mean.
NMDS ordination results indicated that PPCs in the JS clustered more strongly by season than by station (Figure 3A). ANOSIM also supported this finding, showing that seasonal variations significantly influenced the PPC structure (R = 0.987, p < 0.001) more than spatial variations (R = -0.133, p = 0.907). Essentially, differences in community composition were driven more by seasonal environmental changes than by variations in water masses or locations.
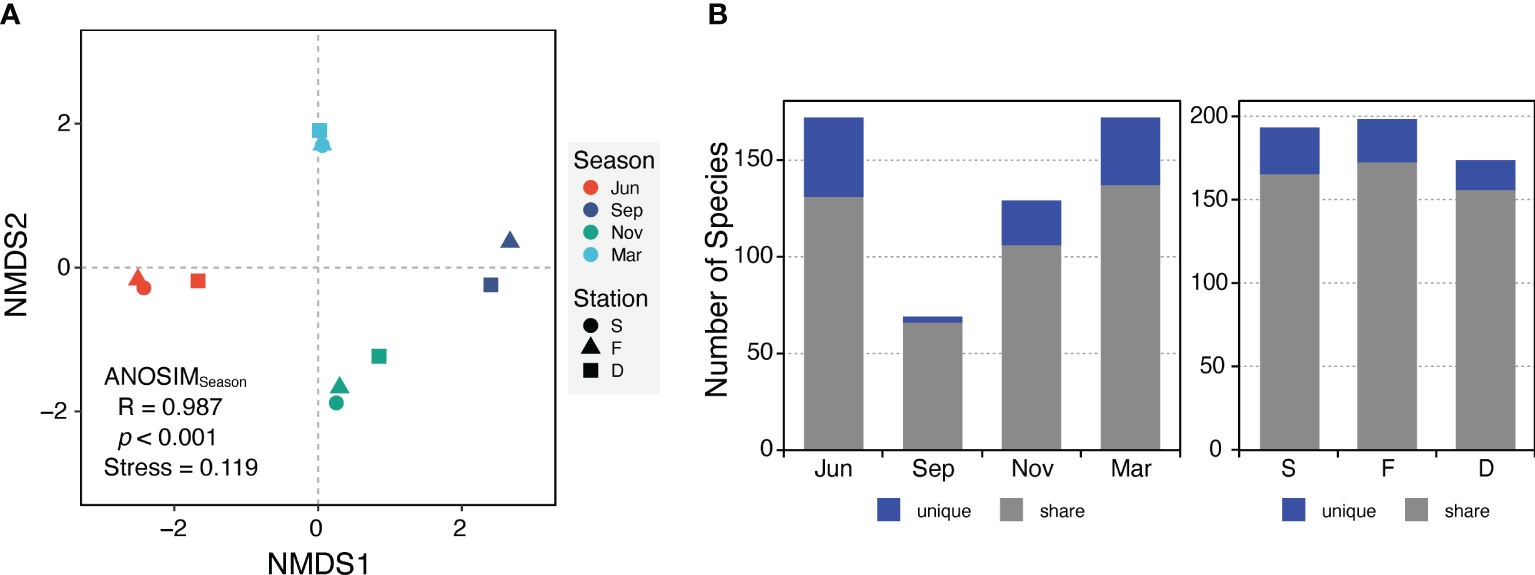
Figure 3 The dissimilarity between planktonic protist communities across four seasons and three stations were visualized using different approaches. (A) Non-metric multidimensional scaling (NMDS) ordination based on the Jaccard distance and the analysis of similarities (ANOSIM) statistic. Each colored circle, triangle, or square represents a specific sample. (B) The number of unique species (blue) and share species (gray) for each season and station.
The number of unique species and species richness varied between seasonal protist communities, while spatial communities demonstrated similar values (Figure 3B). When comparing ten different phyla, the PPCs in November were primarily composed of Ochrophyta (mostly diatoms, at 91%), constituting 95% of the community (Figures 4A, D), while PPCs displayed a more balanced composition in March, with a variety of phyla. Relative abundance of Myzozoa (averaging 49% across three stations), including Alexandrium catenella (10%), A. pacificum (12%), and A. tamarense (16%), was considerably higher in March compared with other periods. Similarly, the relative abundance of Chlorophyta Bathycoccus prasinos (7.3%) and Cryptophyta Teleaulax amphioxeia (6.8%) was also higher. Although the taxonomic composition was generally similar across size fractions, the relative abundance of micro-sized protists increased by about 50% from June to November, then decreased in March (Figures 4B, E). In the JS, Bacillariophyceae (Ochrophyta), commonly known as diatoms, and Dinophyceae (Myzozoa) were the most abundant and diverse groups within the PPCs. Bacillariophyceae accounted for 59.4% of the sequence abundance and 48.4% of the OTU richness, while Dinophyceae represented 27.4% of the sequence abundance and 35.3% of the OTU richness.
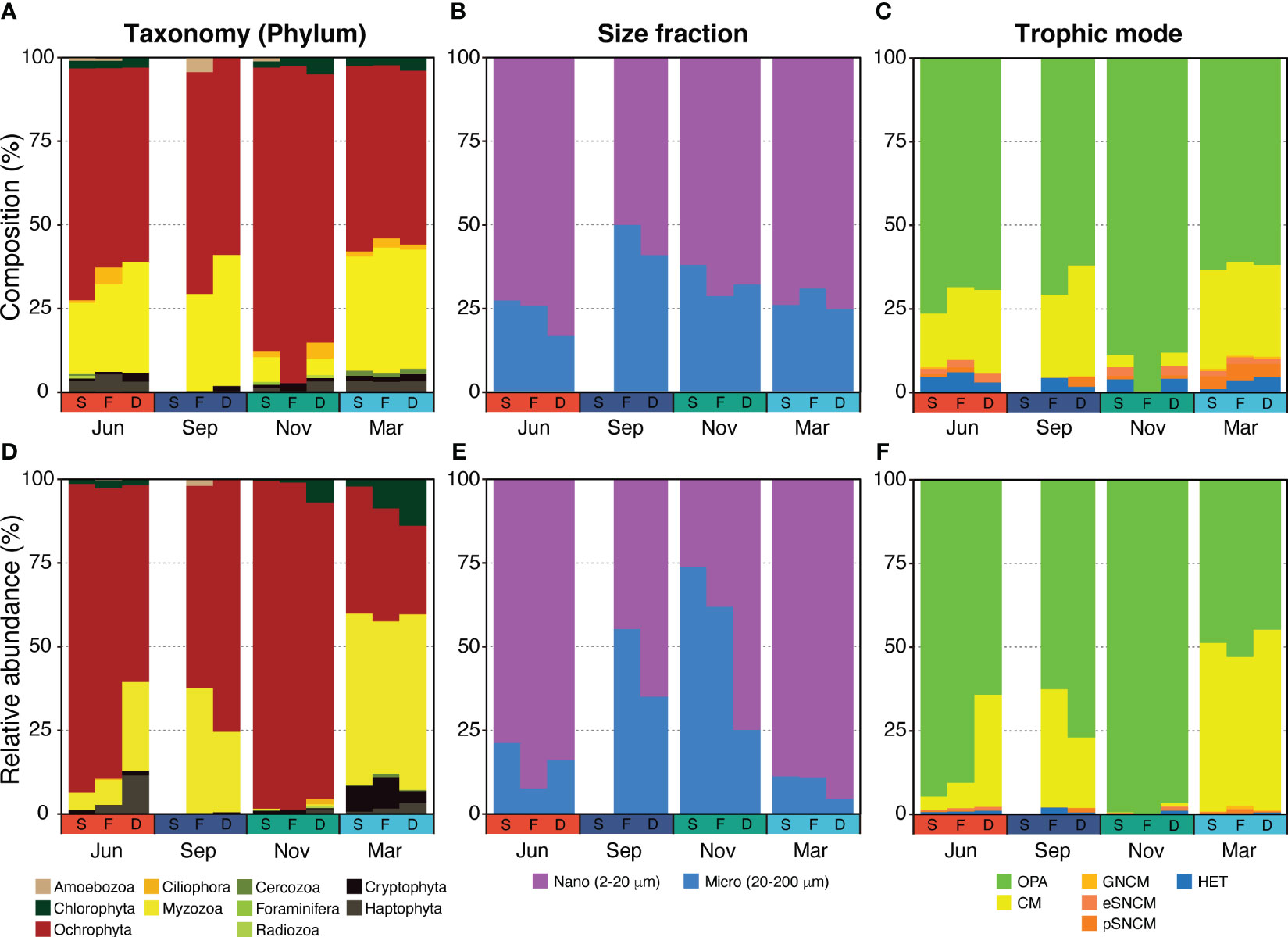
Figure 4 Spatiotemporal changes of species composition and relative abundance of the planktonic protist community at the phylum level (A, D), size fraction (B, E), and trophic mode (C, F) across the shallow-water (S), thermohaline front (F), and deep-water (D) stations.
The taxonomic composition of protists, classified by their trophic mode, demonstrated a trend similar to the relative abundance of Myzozoa (Figure 4). Myzozoa, which are dinoflagellates capable of mixotrophy (a combination of phototrophy and phagotrophy), showed a positive correlation with the proportion of nano-sized protists in the community.
Community assembly processes
The NCM was applied to investigate the variability in the occurrence frequency of the entire PPCs in the JS. The model accounted for a large proportion (R2 = 0.628) of this variability (Figure 5A), suggesting that stochastic processes predominantly drove the assembly of the PPC in this strait. The null model analysis results showed that the assembly of PPCs in the JS was greatly influenced by stochastic processes (Figure 5B). Within the neutral group, stochastic processes comprised undominated processes (50.9%), homogenizing dispersal (47.3%), and dispersal limitation (1.8%).
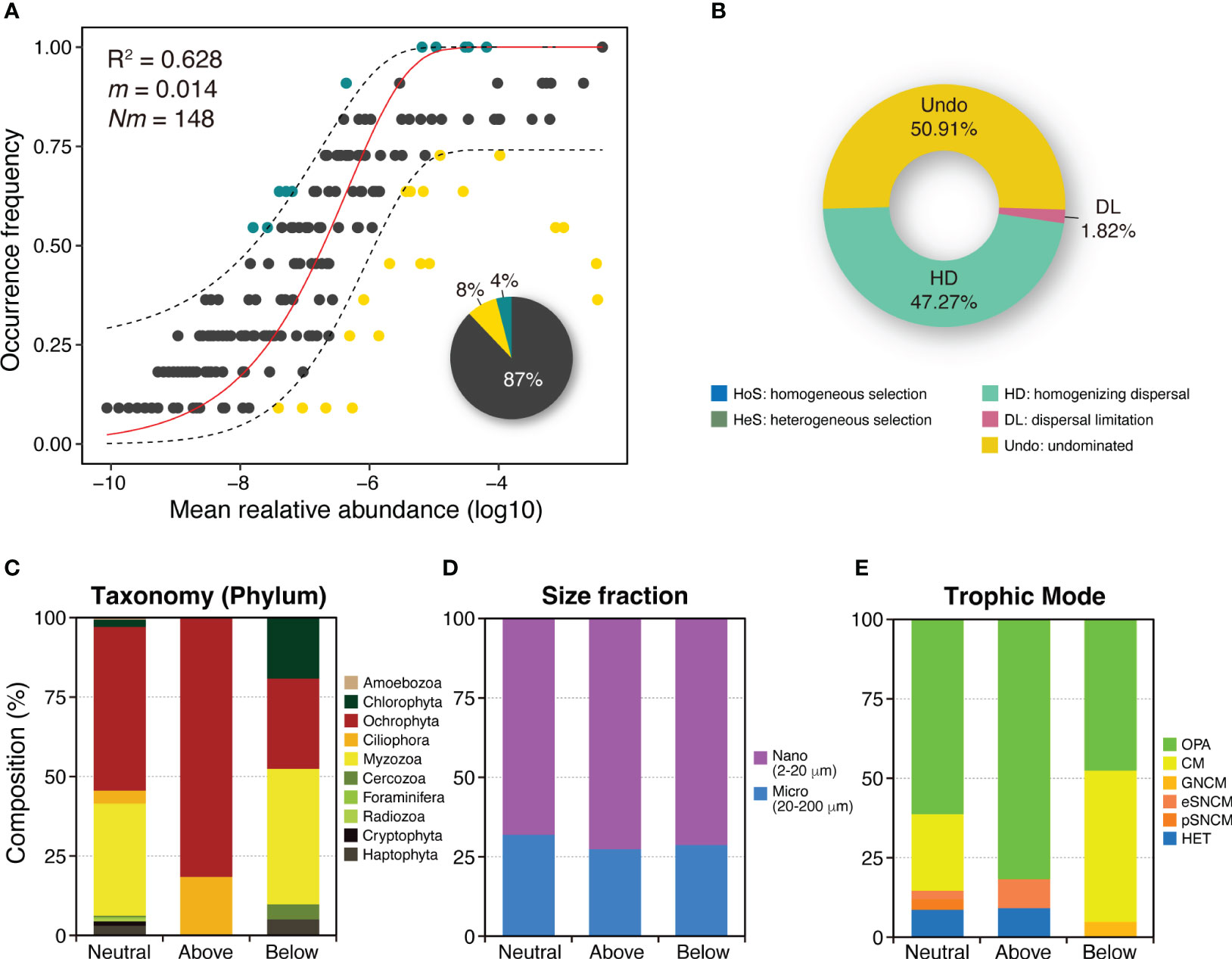
Figure 5 Analysis of the relative influences of deterministic and stochastic processes on planktonic protist community assembly. (A) Fit of the neutral community model (NCM) for community assembly. The plot shows the predicted occurrence frequencies of planktonic protist communities based on all samples collected in the Jeju Strait. The solid red line represents the best fit to the NCM described by Sloan et al. (2006), while the dashed blue lines indicate the 95% confidence interval around the model prediction. Protistan OTUs occurring more or less frequently than predicted by the NCM are depicted using different colors (dark green and yellow dots). Nm represents the metacommunity size multiplied by immigration, and R2 indicates the fit to the NCM model. The pie chart illustrates the composition of three groups (above, below, and neutral prediction based on the NCM) in the total occurrence species. (B) Representation of the proportion of ecological assembly processes as determined by the null model. The community assembly is mainly influenced by deterministic processes, including homogeneous selection (HoS) and heterogeneous selection (HeS), and by stochastic processes like homogenizing dispersal (HD), dispersal limitation (DL), and undominated processes (Undo) that include weak selection, weak dispersal, diversification, and drift. (C–E) Species composition of three prediction groups on the basis of phylum, size fraction, and trophic mode in the planktonic protist community. Each bar represents pooled communities, with OTUs divided into separate partitions based on whether they were consistent within the neutral prediction or deviated above or below it.
The OTUs were classified into three groups–above, below, and neutral–based on the NCM predictions. Most of the OTUs (87%) fell under the neutral prediction group, while a smaller portion was classified as non-neutral above or non-neutral below (4% and 8%, respectively). OTUs in the non-neutral above prediction group, which exhibited higher frequency and lower relative abundance than the neutral prediction group, comprised two Pseudo-nitszchia species, three Chaetoceros species, four Ochrophyta, and two Ciliophora (Figure 5C; Table S2). In contrast, OTUs in the non-neutral below prediction group, which demonstrated lower frequency and higher relative abundance, included five Alexandrium species, Protoceratium reticulatum, Prorocentrum triestinum, two other Myzozoa, six Ochrophyta, and four Chlorophyta and Haptophyta.
There were no differences in the size fraction of the OTUs between the neutral and non-neutral prediction groups (Figure 5D). However, trophic mode composition varied between the groups (Figure 5E). The above prediction group included a HET ciliate, Sterkiella histriomuscorum, and an eSNCM Ciliophora, in addition to mainly OPA (Table S2). By contrast, the below prediction group was predominantly composed of CM dinoflagellates and a GNCM Cercozoa species, Ebria tripartita.
Seasonal dynamics of protists in major modules
The correlation-based network of the PPC in the JS included 149 nodes representing distinct species and 209 edges indicating their interactions (Figure 6A; Table 1). The presence of 61 unique modules, many of which consisted of a single node, suggests the existence of distinct subgroups within the community with a certain level of functional differentiation. Approximately 72% of the interactions were positive (gray lines in Figure 6A), indicating symbiotic relationships, while 28% were negative (blue lines), indicating the presence of competitive interactions within the community. An average clustering coefficient of 0.39 and a path length of 3.95 suggests a propensity for species to form clusters and establish efficient relationships within the network. A network diameter of 9 indicates a well-connected community. The average betweenness centrality score of 72.5 highlights the existence of specific key species that act as bridges, facilitating interactions between different parts of the network.
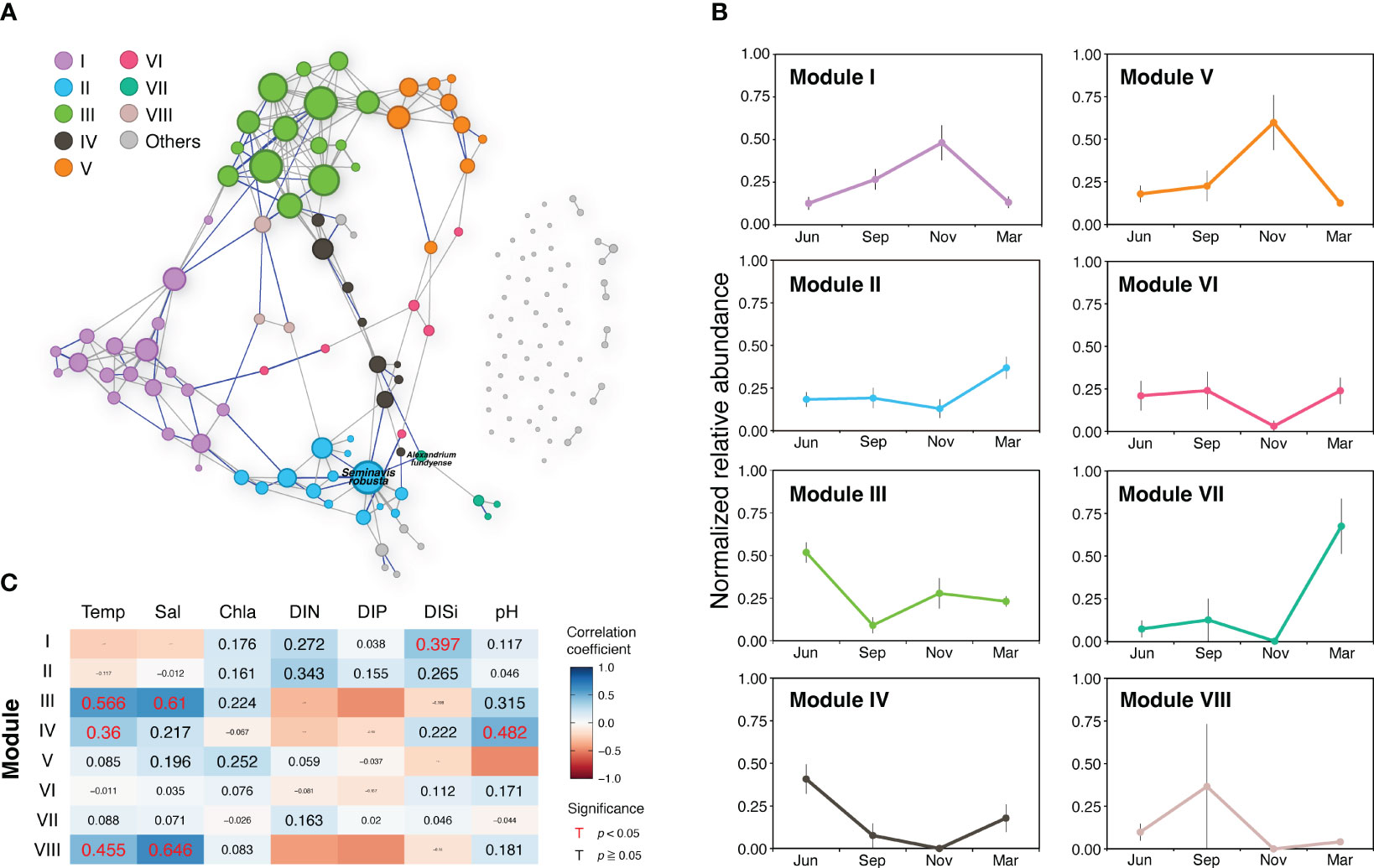
Figure 6 (A) Planktonic protist community networks based on all samples collected in the Jeju Strait. The size of nodes is proportional to the number of edges for each node. The gray and blue lines connecting paired nodes represent positive (co-occurrence) and negative (exclusion) interactions, respectively, with strong and significant correlations (|r| > 0.7, p < 0.05). Nodes are colored based on their modularity class. Two labeled nodes indicate the module hub and connector species (see Figure S3 for more details). (B) Transition pattern of major modules represented by changes in normalized relative abundance across seasons (mean ± standard error). (C) Spearman correlation between environmental variables and relative abundance of nodes belonging to major modules. The background colors and values indicate Spearman’s correlation coefficient (r). The value size reflects the significance level (p-value), with red values representing statistically significant correlations.
Within the primary network, there were 86 nodes and 202 links, primarily arranged into eight major modules, which accounted for 92% of the network. The modules and their proportions were as follows: Module I (12.8%), Module II (9.4%), Module III (10.1%), Module IV (6.0%), Module V (6.0%), Module VI (4.0%), Module VII (2.7%), and Module VIII (2.0%) (Table S3). The transition pattern of the major protist community modules was evaluated using normalized relative abundance, and the correlation between modules and environmental variables was studied via a partial Mantel test (Figures 6B, C). The results showed that in the JS, the PPC transitioned in the following sequence: Modules III and IV, Module VIII, Modules I and V, Modules II and VII (Figure 6B). Modules III, IV, and VIII exhibited a positive correlation with temperature (r = 0.360‒0.566, p < 0.05), while Modules III and VIII demonstrated a strong positive correlation with salinity (r = 0.610–0.646, p < 0.005). Module III was primarily composed of nano-sized diatoms such as Skeletonema, Thalassiosira, and Chaetoceros. Module IV, comprising Pseudonitzschia, Alexandrium minutum, and Akashiwo sanguinea, showed a positive correlation with pH (r = 0.482, p = 0.002). Modules I and V displayed the highest normalized relative abundance in November, while Module I, which comprised micro-sized diatoms such as Coscinodiscus, Rhizosolenia, and Biddulphia, was significantly associated with DISi (r = 0.397, p = 0.024). In March, Modules II and VII were abundant and had a high proportion of dinoflagellates in OTU composition. The raphid pennate diatom Seminavis robusta played a role as a hub in Module II, and the toxic dinoflagellate Alexandrium fundyense was the connector linking the primary network and Module VII (Figure 6C; Table S3). These findings were not related to any environmental variables (Figures 6A; S3).
Discussion
Seasonal dynamics: summer thermoclines and autumn thermohaline fronts in the JS
The JS is characterized by dynamic environmental conditions influenced by different water masses, including the Korea Coastal Current (KCC), the JWC, and Changjiang Dilute Water (CDW). Our study revealed distinct oceanic phenomena occurring during different seasons, manifested as thermoclines and thermohaline fronts (Figures 1B, C). In June 2017, we observed the development of a seasonal thermocline near Jeju Island in the deep-water areas of the JS. A thermocline represents a layer within the water column where there is a rapid temperature change with increasing depth. Over time, this thermocline became deeper and wider, reaching its peak extent in September. Additionally, we noticed the presence of low salinity water extending above the thermocline throughout the JS. The formation of a thermocline leads to vertical stratification within the water column, resulting in distinct phytoplankton communities with varying nutrient. This vertical stratification has a significant influence on the structure of the PPCs (Mena et al., 2019; Venkataramana et al., 2021).
In November, we observed the occurrence of thermohaline fronts in the JS (Figures 1B, C). Thermohaline fronts serve as boundaries between different water masses based on variations in temperature and salinity. They indicate significant shifts in the temperature and salinity structures of the ocean during seasonal changes, impacting the distribution of marine life and nutrient availability (Franks, 1992). In the JS, the clockwise flow of the warmer and saltier JWC around Jeju Island, combined with the well-mixed coastal water off southwestern Korea, creates a thermohaline front near station F. This front potentially contributes to the observed temperature inversion (Kim et al., 2022). The formation of a thermohaline front along the 15–18°C isotherms in November corresponds to the horizontal distribution of surface temperature (Figure 1B). Thermohaline fronts are globally recognized for promoting ocean mixing through the horizontal intrusion of water masses. They also serve as biodiversity hotspots, fostering phytoplankton growth and attracting higher trophic level organisms, which support important fisheries with abundant zooplankton (Mcginty et al., 2014; Haberlin et al., 2019; Kim et al., 2020a).
Phylogeny and taxonomy PPC diversity
In this study, we analyzed the alpha diversity indices of PPCs in the JS. Our analysis showed that seasonal changes in environmental conditions had a significant impact on Faith’s PD and NRI. Specifically, the NRI exhibited a substantial increase in November compared to other seasons (Figure 2D), which can be attributed to the presence of thermohaline fronts and the resulting distinctive environmental conditions. This finding suggests that community assembly during November was more deterministic, as indicated by the high NRI. This implies a higher likelihood of co-occurrence among closely related species than would be expected by chance. A positive NRI value signifies a clustered or converged phylogenetic structure within the community (Webb et al., 2002). The observed community assembly pattern may be influenced by environmental filtering or niche-based processes, commonly driven by recruitment and mortality dynamics affected by local environmental conditions. This suggests that environmental filtering plays a significant role in shaping the community structure (Letten et al., 2014; Chai et al., 2016).
Throughout the study period, no significant variations were observed in the alpha diversity indices across different spatial locations (Figure 3). This indicates a relatively homogeneous spatial distribution and composition of PPC in the JS. It also suggests that factors influencing the presence and abundance of planktonic protist, such as nutrient availability, water temperature, salinity, and other ecological conditions, did not exhibit significant spatial variability during the study period. However, it is important to note that other aspects of PPC, such as functional traits or specific species compositions, may still exhibit differences, even in the absence of significant variations in alpha diversity indices (Roselli and Litchman, 2017; Anderson et al., 2021).
Overall, our study highlights the influence of seasonal changes in environmental conditions on the alpha diversity of PPCs in the JS. Furthermore, it emphasizes the role of environmental filtering and deterministic community assembly processes during specific seasons, as indicated by the observed patterns in NRI. These findings will contribute to our understanding of the ecological dynamics and assembly mechanisms governing PPCs in this strait.
Representative groups of planktonic protists in the JS
In the JS, Bacillariophyceae (Ochrophyta) and Dinophyceae (Myzozoa) were found to be the most abundant and diverse groups within PPCs. Several highly abundant OTUs identified in this study were also observed in previous investigations of protistan or microbial eukaryotic communities in the northern East China Sea (Faria et al., 2014; Kim et al., 2020b; Kim et al., 2022; Sun et al., 2022).
The composition of the PPCs varies throughout the year, primarily due to nutrient conditions. For instance, in June, areas characterized by high nutrient conditions, such as shallow-water and front zones, were mainly dominated by nano- and micro-sized diatoms (Figures 4A, D). However, in September, when the JS experiences strong stratification and nutrient depletion, the community was dominated by both diatoms and dinoflagellates. With their swimming ability and mixotrophic capabilities, dinoflagellates exhibit competitive advantages under these conditions, allowing them to persist in the euphotic zone and compensate for low nutrient concentrations (Flynn et al., 2019). In November, when nutrient concentrations were high (DIN: 5–10 µM, DIP: 0.6–0.8 µM), diatoms were found to dominate both in composition and relative abundance in the shallow and frontal waters, suggesting that they are strong competitors in environments with elevated nutrient concentrations (Burson et al., 2018).
The surface waters of the deep-water area (station D) in June and all stations in September, which were characterized by limited nutrient availability, account for a significant proportion of smaller nano-sized (2-20 μm) phytoplankton, referred to as nano-sized obligate photoautotrophs (OPAs) (Figure 4). This preference for the smaller size is likely due to the higher surface area to volume ratio of smaller phytoplankton, which confers advantages in nutrient uptake in nutrient-limited environments (Harrison et al., 2015; Fernández-González et al., 2022). Additionally, interactions between predators and prey have been observed to influence the species composition of PPCs in such environments. It has been suggested that there is a stronger predator-prey coupling for smaller phytoplankton (Behrenfeld et al., 2021). Considering the seasonal changes observed in the species composition and relative abundance of the PPC in the JS, mixotrophic and heterotrophic protists showed contrasting quantitative changes compared with larger micro-sized (20-200 μm) PPC (Figure 4). This dynamic could potentially favor the survival of smaller phytoplankton species over larger ones, as they may be more efficiently consumed by their predators, such as heterotrophs or mixotrophs (Figures 4C, F). The shift toward smaller phytoplankton can also impact the overall functioning of marine ecosystems and biogeochemical cycles, as areas dominated by smaller phytoplankton often exhibit lower biodiversity levels (Henson et al., 2021).
Little dispersal limitation but high importance of neutral process for PPCs
Dispersal limitation significantly influences plankton community assembly (Lear et al., 2014). Organisms tend to colonize habitats closer to their source, with community similarities decreasing as distance increases. Cell size is also an important factor in shaping community structure through dispersal limitation (de Vargas et al., 2015). The beta diversity index, which measures community similarity, reflects the influence of dispersal limitation. A higher index indicates less similarity and a greater influence of dispersal limitation, while a lower index suggests more similarity and less influence (Zhou and Ning, 2017).
The NCM is commonly used to assess the importance of stochastic processes, particularly neutral processes, in microbial community assembly (Chen et al., 2019; Ramond et al., 2021). The NCM has demonstrated that neutral processes play a dominant role in shaping community variations. In this study, the NCM explained a significant portion (R2 = 0.62) of the variability in the occurrence frequency of the entire PPC, highlighting the importance of neutral processes in driving PPC variations. Similar findings regarding the high contribution of neutral processes have been observed in protistan or microbial eukaryotic communities in the rivers and coastal waters of the South China Sea (Chen et al., 2017; Zou et al., 2021; Xu et al., 2020). This is due to factors such as the high and random dispersal rate of microorganisms, which is caused by the absence of distance barriers and the rapid flow rates of rivers and ocean currents, preventing the formation of distance-decay patterns. These findings underscore the importance of neutral processes in shaping the assembly and variations of PPCs, particularly in environments where dispersal rates are high and distance barriers are limited. The prevalence of neutral processes suggests that chance events and random processes, including dispersal and ecological drift, have a stronger impact on community composition in the JS than deterministic factors.
The dispersal rate of plankton can have varying effects on community structure. If the rate is high, it results in homogenization, while limited dispersal results in dispersal limitation (Vellend and Agrawal, 2010; Logares et al., 2018). The rate of immigration for PPCs in the JS was considerably lower (m = 0.014) than in other studies on microbial eukaryotic communities (m = 0.2–0.4) (Chen et al., 2017; Xu et al., 2020; Zhang et al., 2021), indicating that the PPC in the JS was highly limited by dispersal. This suggests that spatial dispersal limitation had minimal influence on the regional structure of PPC in the JS. Neutral processes, including ecological drift and homogenizing dispersal, primarily drive the variation within PPCs, overshadowing deterministic factors such as environmental conditions and species traits, as revealed by the NCM. Despite a low immigration rate, protists display a remarkable drive to disperse, facilitating a uniform distribution of species and diminishing distinct spatial patterns or distance-decay effects. These findings highlight the significant influence of stochastic processes and random events on community dynamics and protist distribution patterns.
Our results suggest that the assembly of PPCs in the JS is primarily driven by stochastic processes, particularly homogenizing dispersal and ecological drift, with minimal influence from dispersal limitation. Homogenizing dispersal, which accounts for 47% of the contribution, indicates that these plankton communities were widely dispersed and are distributed relatively evenly through the strait. This indicates a high level of similarity in community composition among different locations in the JS. In essence, there is a strong “mixing” effect, with organisms frequently moving and redistributing themselves throughout the ecosystem. Ecological drift, accounting for 51% of the contribution, suggests that random changes in species abundance over time also play a significant role in shaping these communities. The presence or absence of certain species in the community is largely influenced by chance events, such as random births and deaths or unpredictable environmental fluctuations. The very low contribution of dispersal limitation (1.82%) indicates that the movement of organisms across the strait is not significantly restricted. This could be attributed to the absence of physical barriers or the organisms’ strong dispersal abilities. Therefore, most species can reach almost all habitats across the strait, and community assemblages are not strongly influenced by the inability of species to disperse. Overall, these findings suggest that the PPCs in the JS are highly dynamic and are primarily shaped by random processes rather than deterministic environmental selection or dispersal constraints. It implies that these communities are highly resilient to changes in environmental conditions, as they are not tightly bound to specific environmental factors or locations. However, the strong influence of random processes also means that predicting the future development of these communities can be challenging.
In addition, a distinct group represented by yellow dots in Figure 5A has been characterized for several species within Chlorophyta and Myzozoa. This group exhibits a lower frequency but higher relative abundance compared to the prediction for neutrally occurring species, as shown by the NCM. Notably, species belonging to the Chloropicophyceae class are commonly found in oceanic waters, indicating their preference for such habitats (Lopes dos Santos et al., 2017). Another noteworthy genus is Bathycoccus, a cosmopolitan picophytoplankton genus classified in the Mamiellophyceae. Bathycoccus prasinos, the sole species within this genus, is a small non-motile green microalga characterized by intricate scales. It plays a vital role in the marine ecosystem by significantly contributing to primary oceanic production (Belevich et al., 2020). Interestingly, Bathycoccus prasinos has been identified as the most abundant species in the East China Sea during the Spring season (Xu et al., 2016). The below group comprises several species within the Myzozoa, specifically belonging to the Dinophyceae class, including notorious species such as A. catenella, A. pacificum, A. tamarense, Protoceratium reticulatum, and Prorocentrum triestinum, which are all known for causing harmful algal blooms (HABs) globally and in Korea specifically (Alves-de-Souza et al., 2014; Anderson et al., 2021; Min and Kim, 2023). HABs are typically episodic, occurring infrequently but in exceptionally high concentrations when they do happen. In the JS, these taxa exhibited a higher relative abundance in March compared with other periods. For example, the bloom of P. reticulatum in a Chilean fjord during the summers of 2016 and 2017 exemplified this occurrence pattern, with blooms observed at an average water temperature of 16°C (Alves-de-Souza et al., 2019).
Representative biotic interactions of microbial eukaryotes in the JS
In this study, we investigated the relationship between eight distinct modules and various environmental variables, with a particular focus on monitoring changes in their normalized relative abundance over time. We found that Modules I, III, IV, and VIII had significant associations with specific environmental factors, indicating potential influences on their biological characteristics. However, it is important to note that correlation does not imply causality, and further research is needed to validate and obtain a more comprehensive understanding of these relationships.
The plankton community network analysis of all samples in this study revealed that Module I primarily consisted of diatoms and other silicate-utilizing organisms, including micro-sized diatoms such as Coscinodiscus, Rhizosolenia, and Biddulphia genera (Table S3). The presence of Module I was found to positively correlate with DISi. This suggests that these genera can efficiently utilize dissolved inorganic silica to construct and maintain their cell walls (Martin‐Jézéquel et al, 2000).
Module III shows a clear seasonal pattern, with its normalized relative abundance peaking in June and reaching its lowest point in September. The partial Mantel test analysis revealed significant positive correlations between Module III and both temperature (r = 0.566, p = 0.002) and salinity (r = 0.610, p = 0.005). However, there were insignificant negative correlations with DIN, DIP, and DISi. These findings suggest that the species represented in Module III primarily comprised common nano-sized diatoms, including Skeletonema, Thalassiosira, and Chaetoceros, which have successfully adapted to thrive in warmer and saltier conditions. However, their activity and abundance may be curtailed during the summer months due to nutrient constraints in the JS.
Module IV, which displays a seasonal pattern similar to Module III, exhibits a significant positive correlation with both temperature and pH. This suggests that the organisms within this module may thrive under specific temperature conditions and pH levels. They could be adapted to warmer conditions and specific pH ranges that occur at certain times of the year, which explains the observed seasonal peak in June. The major species belonging to Module IV include Pseudonitzschia spp., Alexandrium minutum, and Akashiwo sanguinea, all types of plankton that play significant roles in marine ecosystems. These species are known for their ability to form blooms in coastal Korean waters, which can sometimes be harmful to other marine life because of the toxins they produce, such as domoic acid and neurotoxins, which can cause amnesic and paralytic shellfish poisoning in humans (Min and Kim, 2023).
The traits of Module VIII seem to be significantly affected by both seasonal transitions and certain environmental conditions, particularly temperature and salinity. The abundance of organisms in Module VIII follows a distinct annual pattern, peaking in September and gradually diminishing to zero by November. This pattern suggests a significant seasonal impact on the presence and prevalence of organisms within Module VIII, which may correspond to specific life cycle stages or the availability of resources. The organisms in this module might possess adaptations suited to environments with higher salinity and specific temperature ranges. However, because only four species within this module were identified, providing detailed information about their individual characteristics will be problematic.
Modules II, V, VI, and VII do not show significant correlations with the environmental variables, based on the results of partial Mantel tests, which suggests that these modules may operate independently from the tested variables and their variations. However, it is important to note that this interpretation is specific to the current context, and there may be other factors or complex interactions that could influence these modules.
This study employed SoEM sequencing methodology to investigate the seasonal and spatial dynamics of the PPC in the JS. The identification of 61 modules within the PPC network aligns with the concept of ecological niches, suggesting the presence of distinct subgroups or functional units. This modular structure indicates that different species within the PPC specialize in specific ecological roles or inhabit different habitats, contributing to the stability and resilience of the ecosystem (Forster et al., 2021). The modular organization of cellular networks, as observed in protein-interaction studies in yeast, suggests that specific biological responses may be controlled by such modules (Xue et al., 2018; Liu et al., 2019). Similarly, in the case of protists, clusters derived solely from network structure may define modules that fulfill similar biological roles (Rives and Galitski, 2003; Lewis et al., 2010). Thus, the modular structure observed in the PPC network indicates the presence of distinct ecological niches, which in turn contribute to ecosystem stability and resilience. This analysis enhances our understanding of species co-existence, resource utilization, and environmental adaptability.
Data availability statement
The data presented in the study are deposited in the NCBI Sequence Read Archive (SRA) repository (https://www.ncbi.nlm.nih.gov/bioproject/PRJNA974865).
Author contributions
JM prepared the initial draft, while all authors contributed to the conceptualization, investigation, writing, reviewing, and editing.
Funding
This research was supported by National Research Foundation (NRF) grants (NRF-2016R1A6A1A03012647, NRF-2020R1A2C3005053, NRF-2022M3I6A1085991) to KYK funded by the Korean government (MSIT).
Conflict of interest
The authors declare that the research was conducted in the absence of any commercial or financial relationships that could be construed as a potential conflict of interest.
Publisher’s note
All claims expressed in this article are solely those of the authors and do not necessarily represent those of their affiliated organizations, or those of the publisher, the editors and the reviewers. Any product that may be evaluated in this article, or claim that may be made by its manufacturer, is not guaranteed or endorsed by the publisher.
Supplementary material
The Supplementary Material for this article can be found online at: https://www.frontiersin.org/articles/10.3389/fmars.2023.1225640/full#supplementary-material
References
Adl S. M., Simpson A. G., Lane C. E., Lukeš J., Bass D., Bowser S. S., et al. (2012). The revised classification of eukaryotes. J. Eukaryot. Microbiol. 59 (5), 429–514. doi: 10.1111/j.1550-7408.2012.00644.x
Aguilar P., Sommaruga R. (2020). The balance between deterministic and stochastic processes in structuring lake bacterioplankton community over time. Mol. Ecol. 29 (16), 3117–3130. doi: 10.1111/mec.15538
Alves-de-Souza C., Iriarte J. L., Mardones J. I. (2019). Interannual Variability of Dinophysis acuminata and Protoceratium reticulatum in a Chilean Fjord: Insights from the Realized Niche Analysis. Toxins 11 (1), 19. doi: 10.3390/toxins11010019
Alves-de-Souza C., Varela D., Contreras C., de la Iglesia P., Fernández P., Hipp B., et al. (2014). Seasonal variability of Dinophysis spp. and Protoceratium reticulatum associated to lipophilic shellfish toxins in a strongly stratified Chilean fjord. Deep Sea Res. Part II: Top. Stud. Oceanogr. 101, 152–162. doi: 10.1016/j.dsr2.2013.01.014
Anderson S. I., Barton A. D., Clayton S., Dutkiewicz S., Rynearson T. A. (2021). Marine phytoplankton functional types exhibit diverse responses to thermal change. Nat. Commun. 12 (1), 6413. doi: 10.1038/s41467-021-26651-8
Arendsee Z., Li J., Singh U., Seetharam A., Dorman K., Wurtele E. S. (2019). Phylostratr: a framework for phylostratigraphy. Bioinformatics 35 (19), 3617–3627. doi: 10.1093/bioinformatics/btz171
Bastian M., Heymann S., Jacomy M. (2009). Gephi: an open source software for exploring and manipulating networks. Proc. Int. AAAI Conf. Web Soc. media 3 (1), 361–362. doi: 10.1609/icwsm.v3i1.13937
Behrenfeld M. J., Boss E. S., Halsey K. H. (2021). Phytoplankton community structuring and succession in a competition-neutral resource landscape. ISME Commun. 1 (1), 12. doi: 10.1038/s43705-021-00011-5
Belevich T. A., Milyutina I. A., Abyzova G. A., Troitsky A. V. (2020). The pico-sized Mamiellophyceae and a novel Bathycoccus clade from the summer plankton of Russian Arctic Seas and adjacent waters. FEMS Microbiol. Ecol. 97 (2), fiaa251. doi: 10.1093/femsec/fiaa251
Bolger A. M., Lohse M., Usadel B. (2014). Trimmomatic: a flexible trimmer for Illumina sequence data. Bioinformatics 30 (15), 2114–2120. doi: 10.1093/bioinformatics/btu170
Burson A., Stomp M., Greenwell E., Grosse J., Huisman J. (2018). Competition for nutrients and light: testing advances in resource competition with a natural phytoplankton community. Ecology 99 (5), 1108–1118. doi: 10.1002/ecy.2187
Caron D. A., Countway P. D., Jones A. C., Kim D. Y., Schnetzer A. (2012). Marine protistan diversity. Ann. Rev. Mar. Sci. 4 (1), 467–493. doi: 10.1146/annurev-marine-120709-142802
Chai Y., Yue M., Liu X., Guo Y., Wang M., Xu J., et al. (2016). Patterns of taxonomic, phylogenetic diversity during a long-term succession of forest on the Loess Plateau, China: insights into assembly process. Sci. Rep. 6 (1), 27087. doi: 10.1038/srep27087
Chamberlain S. (2020). worrms: World Register of Marine Species (WoRMS) Client. R package version 0.4.2. Available at: https://CRAN.R-project.org/package=worrms.
Chang K. I., Teague W. J., Lyu S. J., Perkins H. T., Lee D. K., Watts D. R., et al. (2004). Circulation and currents in the southwestern East/Japan Sea: Overview and review. Prog. Oceanogr. 61 (2), 105–156. doi: 10.1016/j.pocean.2004.06.005
Chase J. M., Myers J. A. (2011). Disentangling the importance of ecological niches from stochastic processes across scales. Philos. Trans. R. Soc B: Biol. Sci. 366 (1576), 2351–2363. doi: 10.1098/rstb.2011.0063
Chen W., Pan Y., Yu L., Yang J., Zhang W. (2017). Patterns and processes in marine microeukaryotic community biogeography from xiamen coastal waters and intertidal sediments, southeast China. Front. Microbiol. 8. doi: 10.3389/fmicb.2017.01912
Chen W., Ren K., Isabwe A., Chen H., Liu M., Yang J. (2019). Stochastic processes shape microeukaryotic community assembly in a subtropical river across wet and dry seasons. Microbiome 7, 1–16. doi: 10.1186/s40168-019-0749-8
Chun S.-J., Cui Y., Lee C. S., Cho A. R., Baek K., Choi A., et al. (2019). Characterization of distinct cyanoHABs-related modules in microbial recurrent association network. Front. Microbiol. 10. doi: 10.3389/fmicb.2019.01637
Clayton T. D., Byrne R. H. (1993). Spectrophotometric seawater pH measurements: total hydrogen ion concentration scale calibration of m-cresol purple and at-sea results. Deep Sea Res. I: Oceanogr. Res. Pap. 40 (10), 2115–2129. doi: 10.1016/0967-0637(93)90048-8
Csardi G., Nepusz T. (2006). The igraph software package for complex network research. Int. J. Complex Syst. 1695 (5), 1–9. doi: 10.5281/zenodo.7682609
de Vargas C., Audic S., Henry N., Decelle J., Mahé F., Logares R., et al. (2015). Eukaryotic plankton diversity in the sunlit ocean. Science 348 (6237), 1261605. doi: 10.1126/science.1261605
Eom S. H., Jeong H. J., Ok J. H., Park S. A., Kang H. C., You J. H., et al. (2021). Interactions between common heterotrophic protists and the dinoflagellate Tripos furca: implication on the long duration of its red tides in the South Sea of Korea in 2020. Algae 36 (1), 25–36. doi: 10.4490/algae.2021.36.2.22
Faria D. G., Lee M.-D., Lee J.-B., Lee J., Chang M., Youn S. H., et al. (2014). Molecular diversity of phytoplankton in the East China Sea around Jeju Island (Korea), unraveled by pyrosequencing. J. Oceanogr. 70 (1), 11–23. doi: 10.1007/s10872-013-0208-2
Fernández-González C., Tarran G. A., Schuback N., Woodward E. M. S., Arístegui J., Marañón E. (2022). Phytoplankton responses to changing temperature and nutrient availability are consistent across the tropical and subtropical Atlantic. Commun. Biol. 5 (1), 1035. doi: 10.1038/s42003-022-03971-z
Flynn K. J., Mitra A., Anestis K., Anschütz A. A., Calbet A., Ferreira G. D., et al. (2019). Mixotrophic protists and a new paradigm for marine ecology: where does plankton research go now? J. Plankton Res. 41 (4), 375–391. doi: 10.1093/plankt/fbz026
Forster D., Qu Z., Pitsch G., Bruni E. P., Kammerlander B., Pröschold T., et al. (2021). Lake ecosystem robustness and resilience inferred from a climate-stressed protistan plankton network. Microorganisms 9 (3), 549. doi: 10.3390/microorganisms9030549
Franks P. J. S. (1992). Sink or swim: Accumulation of biomass at fronts. Mar. Ecol. Progr. Ser. 82, 1–12. doi: 10.3354/meps082001
Friedman J., Alm E. J. (2012). Inferring correlation networks from genomic survey data. PloS Comput. Biol. 8 (9), e1002687. doi: 10.1371/journal.pcbi.1002687
Glibert P. M., Mitra A. (2022). From webs, loops, shunts, and pumps to microbial multitasking: Evolving concepts of marine microbial ecology, the mixoplankton paradigm, and implications for a future ocean. Limnol. Oceanogr. 67 (3), 585–597. doi: 10.1002/lno.12018
Guimera R., Nunes Amaral L. A. (2005). Functional cartography of complex metabolic networks. Nature 433 (7028), 895–900. doi: 10.1038/nature03288
Gutiérrez-Rodríguez A., Lopes dos Santos A., Safi K., Probert I., Not F., Fernández D., et al. (2022). Planktonic protist diversity across contrasting Subtropical and Subantarctic waters of the southwest Pacific. Prog. Oceanogr. 206, 102809. doi: 10.1016/j.pocean.2022.102809
Haberlin D., Raine R., McAllen R., Doyle T. K. (2019). Distinct gelatinous zooplankton communities across a dynamic shelf sea. Limnol. Oceanogr. 64 (4), 1802–1818. doi: 10.1002/lno.11152
Harrison P. J., Zingone A., Mickelson M. J., Lehtinen S., Ramaiah N., Kraberg A. C., et al. (2015). Cell volumes of marine phytoplankton from globally distributed coastal data sets. Estuar. Coast. Shelf Sci. 162, 130–142. doi: 10.1016/j.ecss.2015.05.026
Heino J., Melo A. S., Siqueira T., Soininen J., Valanko S., Bini L. M. (2015). Metacommunity organisation, spatial extent and dispersal in aquatic systems: patterns, processes and prospects. Freshw. Biol. 60 (5), 845–869. doi: 10.1111/fwb.12533
Henson S. A., Cael B. B., Allen S. R., Dutkiewicz S. (2021). Future phytoplankton diversity in a changing climate. Nat. Commun. 12 (1), 5372. doi: 10.1038/s41467-021-25699-w
Hutchinson G. E. (1957). Concluding remarks. Cold Spring Harbor Symp. Quant. Biol. 22 (1), 415–427. doi: 10.1101/SQB.1957.022.01.039
Jin S., Lee H.-G., Park C., Kim K. Y. (2023). Small-organelle-enriched metagenomics: An improved method for environmental DNA-based identification of marine plankton. Limnol. Oceanogr.: Methods 21 (4), 178–191. doi: 10.1002/lom3.10538
Jo J., Lee H.-G., Kim K. Y., Park C. (2019). SoEM: a novel PCR-free biodiversity assessment method based on small-organelles enriched metagenomics. Algae 34 (1), 57–70. doi: 10.4490/algae.2019.34.2.26
Kembel S. W., Cowan P. D., Helmus M. R., Cornwell W. K., Morlon H., Ackerly D. D., et al. (2010). Picante: R tools for integrating phylogenies and ecology. Bioinformatics 26 (11), 1463–1464. doi: 10.1093/bioinformatics/btq166
Kim H. K., Chan B. K. K., Lee S. K., Kim W. (2020). Biogeography of intertidal and subtidal native and invasive barnacles in Korea in relation to oceanographic current ecoregions and global climatic changes. J. Mar. Biolog. Assoc. U.K. 100 (7), 1079–1091. doi: 10.1017/S0025315420001009
Kim J.-K., Choi B.-J., Kim J., Sun Y.-J. (2022a). Wind-driven retreat of cold water pool and abrupt sea temperature rise off the southwest coast of Korea in summer 2017. J. Mar. Syst. 231, 103739. doi: 10.1016/j.jmarsys.2022.103739
Kim S.-Y., Lee T. (2022). Estimation of monthly dissolved inorganic carbon inventory in the outheastern Yellow Sea. Sea 27 (4), 194–210. doi: 10.7850/JKSO.2022.27.4.194
Kim Y., Youn S.-H., Oh H. J., Kang J. J., Lee J. H., Lee D., et al. (2020b). Spatiotemporal variation in phytoplankton community driven by environmental factors in the northern East China Sea. Water 12 (10), 2695. doi: 10.3390/w12102695
Kurtz Z. D., Müller C. L., Miraldi E. R., Littman D. R., Blaser M. J., Bonneau R. A. (2015). Sparse and compositionally robust inference of microbial ecological networks. PloS Comput. Biol. 11 (5), e1004226. doi: 10.1371/journal.pcbi.1004226
Lear G., Bellamy J., Case B., Lee J., Buckley H. (2014). Fine-scale spatial patterns in bacterial community composition and function within freshwater ponds. ISME J. 8, 1715–1726. doi: 10.1038/ismej.2014.21
Lee S. Y., Jeong H. J., Kang H. C., Ok J. H., You J. H., Park S. A., et al. (2021a). Comparison of the spatial-temporal distributions of the heterotrophic dinoflagellates Gyrodinium dominans, G. jinhaense, and G. moestrupii in Korean coastal waters. Algae 36, 1, 37–50. doi: 10.4490/algae.2021.36.3.4
Lee B., Kim J. K., Kim M., Choi B.-J., Kim K. Y., Park M. G. (2023). Northward movement of the tropical dinoflagellate Ornithocercus and Triposolenia genera in Korean coastal waters is strongly associated with the inflow of the Jeju Warm Current. Front. Mar. Sci. 10. doi: 10.3389/fmars.2023.1156121
Lee H.-G., Kim H. M., Min J., Park C., Jeong H. J., Lee K., et al. (2020). Quantification of the paralytic shellfish poisoning dinoflagellate Alexandrium species using a digital PCR. Harmful Algae 92, 101726. doi: 10.1016/j.hal.2019.101726
Lee C. H., Min J., Lee H. G., Kim K. Y. (2021b). Thermal plasticity of growth and chain formation of the dinoflagellates Alexandrium affine and Alexandrium pacificum with respect to ocean acidification. Algae 36 (4), 285–298. doi: 10.4490/algae.2021.36.12.1
Leibold M. A., Holyoak M., Mouquet N., Amarasekare P., Chase J. M., Hoopes M. F., et al. (2004). The metacommunity concept: a framework for multi-scale community ecology. Ecol. Lett. 7 (7), 601–613. doi: 10.1111/j.1461-0248.2004.00608.x
Letten A. D., Keith D. A., Tozer M. G. (2014). Phylogenetic and functional dissimilarity does not increase during temporal heathland succession. Proc. R. Soc B 281 (1797), 20142102. doi: 10.1098/rspb.2014.2102
Lewis A. C. F., Jones N. S., Porter M. A., Deane C. M. (2010). The function of communities in protein interaction networks at multiple scales. BMC Syst. Biol. 4 (1), 100. doi: 10.1186/1752-0509-4-100
Lie H.-J., Cho C.-H. (1997). Surface current fields in the eastern East China Sea. J. Korean Soc Oceanogr. 32 (1), 1–7.
Lim A. S., Jeong H. J. (2022). Primary production by phytoplankton in the territorial seas of the Republic of Korea. Algae 37 (4), 265–279. doi: 10.4490/algae.2022.37.11.28
Lima-Mendez G., Faust K., Henry N., Decelle J., Colin S., Carcillo F., et al. (2015). Determinants of community structure in the global plankton interactome. Science 348 (6237), 1262073. doi: 10.1126/science.1262073
Liu L., Chen H., Liu M., Yang J. R., Xiao P., Wilkinson D. M., et al. (2019). Response of the eukaryotic plankton community to the cyanobacterial biomass cycle over 6 years in two subtropical reservoirs. ISME J. 13 (9), 2196–2208. doi: 10.1038/s41396-019-0417-9
Logares R., Tesson S. V. M., Canbäck B., Pontarp M., Hedlund K., Rengefors K. (2018). Contrasting prevalence of selection and drift in the community structuring of bacteria and microbial eukaryotes. Environ. Microbiol. 20, 2231–2240. doi: 10.1111/1462-2920.14265
Lopes dos Santos A., Pollina T., Gourvil P., Corre E., Marie D., Garrido J. L., et al. (2017). Chloropicophyceae, a new class of picophytoplanktonic prasinophytes. Sci. Rep. 7 (1), 14019. doi: 10.1038/s41598-017-12412-5
Magoč T., Salzberg S. L. (2011). FLASH: fast length adjustment of short reads to improve genome assemblies. Bioinformatics 27 (21), 2957–2963. doi: 10.1093/bioinformatics/btr507
Martin-Jézéquel V., Hildebrand M., Brzezinski M. A. (2000). Silicon metabolism in diatoms: implications for growth. J. Phycol. 36 (5), 821–840. doi: 10.1046/j.1529-8817.2000.00019.x
Mcginty N., Johnson M. P., Power A. M. (2014). Spatial mismatch between phytoplankton and zooplankton biomass at the Celtic Boundary Front. J. Plankton Res. 36 (6), 1446–1460. doi: 10.1093/plankt/fbu058
McMurdie P. J., Holmes S. (2013). phyloseq: an R package for reproducible interactive analysis and graphics of microbiome census data. PloS One 8 (4), e61217. doi: 10.1371/journal.pone.0061217
McMurdie P. J., Holmes S. (2014). Waste not, want not: why rarefying microbiome data is inadmissible. PloS Comput. Biol. 10 (4), e1003531. doi: 10.1371/journal.pcbi.1003531
Mena C., Reglero P., Hidalgo M., Sintes E., Santiago R., Martín M., et al. (2019). Phytoplankton community structure is driven by stratification in the oligotrophic mediterranean sea. Front. Microbiol. 10. doi: 10.3389/fmicb.2019.01698
Min J., Kim K. Y. (2022). Quantification of the ichthyotoxic raphidophyte Chattonella marina complex by applying a droplet digital PCR. Algae 37 (4), 281–291. doi: 10.4490/algae.2022.37.11.30
Min J., Kim K. Y. (2023). Seasonal change and subniche dynamics of three Alexandrium species in the Korea Strait. Harmful Algae 125, 102420. doi: 10.1016/j.hal.2023.102420
Ok J. H., Jeong H. J., Kang H. C., Park S. A. (2021). Ecophysiology of the kleptoplastidic dinoflagellate Shimiella gracilenta: I. spatiotemporal distribution in Korean coastal waters and growth and ingestion rates. Algae 36 (4), 263–283. doi: 10.4490/algae.2021.36.11.28
Ok J. H., Jeong H. J., Kang H. C., Park S. A. (2022). Ecophysiology of the kleptoplastidic dinoflagellate Shimiella gracilenta: II. Effects of temperature and global warming. Algae 37 (1), 49–62. doi: 10.4490/algae.2022.37.3.2
Oksanen J., Simpson G., Blanchet F., Kindt R., Legendre P., Minchin P., et al. (2022). vegan: Community Ecology Package. R package version 2.6-4. Available at: https://CRAN.R-project.org/package=vegan.
Paradis E., Schliep K. (2019). ape 5.0: an environment for modern phylogenetics and evolutionary analyses in R. Bioinformatics 35 (3), 526–528. doi: 10.1093/bioinformatics/bty633
R Core Team (2022). R: A language and environment for statistical computing. R Foundation for Statistical Computing, Vienna, Austria. Available at: http://www.R-project.org/.
Ramond P., Siano R., Schmitt S., de Vargas C., Marié L., Memery L., et al. (2021). Phytoplankton taxonomic and functional diversity patterns across a coastal tidal front. Sci. Rep. 11 (1), 2682. doi: 10.1038/s41598-021-82071-0
Ramond P., Sourisseau M., Simon N., Romac S., Schmitt S., Rigaut-Jalabert F., et al. (2019). Coupling between taxonomic and functional diversity in protistan coastal communities. Environ. Microbiol. 21 (2), 730–749. doi: 10.1111/1462-2920.14537
Rives A. W., Galitski T. (2003). Modular organization of cellular networks. PNAS 100 (3), 1128–1133. doi: 10.1073/pnas.0237338100
Roselli L., Litchman E. (2017). Phytoplankton traits, functional groups and community organization. J. Plankton Res. 39 (3), 491–493. doi: 10.1093/plankt/fbx019
Russel J. (2023). MicEco: Various functions for microbial community data. R package version 0.9.19. Available at: https://github.com/Russel88/MicEco/.
Seeleuthner Y., Mondy S., Lombard V., Carradec Q., Pelletier E., Wessner M., et al. (2018). Single-cell genomics of multiple uncultured stramenopiles reveals underestimated functional diversity across oceans. Nat. Commun. 9 (1), 310. doi: 10.1038/s41467-017-02235-3
Shin C.-W., Min H. S., Lee S., Kang H.-W., Ku B., Kim D. G., et al. (2022). Current structure and volume transport in the jeju strait observed for a year with multiple ADCP moorings. Ocean Sci. J. 57 (3), 365–380. doi: 10.1007/s12601-022-00079-7
Skouroliakou D.-I., Breton E., Irion S., Artigas L. F., Christaki U. (2022). Stochastic and deterministic processes regulate phytoplankton assemblages in a temperate coastal ecosystem. Microbiol. Spectr. 10 (6), e02427–e02422. doi: 10.1128/spectrum.02427-22
Stegen J. C., Lin X., Fredrickson J. K., Chen X., Kennedy D. W., Murray C. J., et al. (2013). Quantifying community assembly processes and identifying features that impose them. ISME J. 7 (11), 2069–2079. doi: 10.1038/ismej.2013.93
Stegen J. C., Lin X., Konopka A. E., Fredrickson J. K. (2012). Stochastic and deterministic assembly processes in subsurface microbial communities. ISME J. 6 (9), 1653–1664. doi: 10.1038/ismej.2012.22
Sun Y., Youn S.-H., Kim Y., Kang J. J., Lee D., Kim K., et al. (2022). Interannual variation in phytoplankton community driven by environmental factors in the northern East China Sea. Front. Mar. Sci. 9. doi: 10.3389/fmars.2022.769497
Vellend M., Agrawal A. (2010). Conceptual synthesis in community ecology. Q. Rev. Biol. 85 (2), 183–206. doi: 10.1086/652373
Venkataramana V., Mishra R. K., Sabu P., Anilkumar N., Sarkar A., Naik R. K., et al. (2021). Stratification governs the plankton community structure and trophic interaction in the Southwestern tropical Indian Ocean during boreal summer. Reg. Stud. Mar. Sci. 48, 101987. doi: 10.1016/j.rsma.2021.101987
Webb C. O., Ackerly D. D., McPeek M. A., Donoghue M. J. (2002). Phylogenies and community ecology. Ann. Rev. Ecol. Syst. 33 (1), 475–505. doi: 10.1146/annurev.ecolsys.33.010802.150448
Wickham H., François R., Henry L., Müller K., Vaughan D. (2023). dplyr: A grammar of data manipulation. R package version 1.1.2. Available at: https://CRAN.R-project.org/package=dplyr.
Won J., Lee Y., Lee H., Noh J. H. (2021). Effect of marine environment changes on the abundance and community composition of cyanobacteria in the south sea of korea. Ocean Polar Res. 43 (4), 279–293. doi: 10.4217/OPR.2021.43.4.279
Xu Z., Chen Y., Meng X., Wang F., Zheng Z. (2016). Phytoplankton community diversity is influenced by environmental factors in the coastal East China Sea. Eur. J. Phycol. 51 (1), 107–118. doi: 10.1080/09670262.2015.1107138
Xu Z., Li Y., Lu Y., Li Y., Yuan Z., Dai M., et al. (2020). Impacts of the Zhe-Min Coastal Current on the biogeographic pattern of microbial eukaryotic communities. Prog. Oceanogr. 183, 102309. doi: 10.1016/j.pocean.2020.102309
Xue Y., Chen H., Yang J. R., Liu M., Huang B., Yang J. (2018). Distinct patterns and processes of abundant and rare eukaryotic plankton communities following a reservoir cyanobacterial bloom. ISME J. 12, 2263–2277. doi: 10.1038/s41396-018-0159-0
Zhang J., Hu A., Sun Y., Yang Q., Dong J., Long L., et al. (2021). ). Dispersal limitation expands the diversity of coral microbiome metacommunity in the south China sea. Front. Mar. Sci. 8. doi: 10.3389/fmars.2021.658708
Zhou J., Ning D. (2017). Stochastic community assembly: does it matter in microbial ecology? Microbiol. Mol. Biol. Rev. 81 (4), e00002–e00017. doi: 10.1128/MMBR.00002-17
Keywords: assembly processes, co-existence patterns, diversity, modules, neutral community model (NCM), operational taxonomic units (OTUs), planktonic protist community (PPC), small organelle-enriched metagenomics (SoEM)
Citation: Min J and Kim KY (2023) Diversity and assembly of planktonic protist communities in the Jeju Strait, Korea. Front. Mar. Sci. 10:1225640. doi: 10.3389/fmars.2023.1225640
Received: 19 May 2023; Accepted: 18 July 2023;
Published: 17 August 2023.
Edited by:
Zhaohe Luo, Third Institute of Oceanography, Ministry of Natural Resources, ChinaReviewed by:
Abdullah Al Mamun, Chinese Academy of Sciences (CAS), ChinaZhangxi Hu, Guangdong Ocean University, China
Copyright © 2023 Min and Kim. This is an open-access article distributed under the terms of the Creative Commons Attribution License (CC BY). The use, distribution or reproduction in other forums is permitted, provided the original author(s) and the copyright owner(s) are credited and that the original publication in this journal is cited, in accordance with accepted academic practice. No use, distribution or reproduction is permitted which does not comply with these terms.
*Correspondence: Kwang Young Kim, a3lraW1AY2hvbm5hbS5hYy5rcg==