- 1Marine Ecology Group, National Institute of Water and Atmospheric Research, Dunedin, New Zealand
- 2Department of Marine Science, University of Otago, Dunedin, New Zealand
- 3Department of Zoology, University of Otago, Dunedin, New Zealand
Introduction: Understanding the characteristics of hotspots of species distribution provides opportunities for habitat-based management; a vital and often missing component in the conservation of mobile marine species. Correlates of species distribution derived from species distribution models (SDMs) are assumed to represent the characteristics of important habitat, which often include physical and biological (i.e., prey) components. In this study, we integrate surveys of Hector’s dolphin (Cephalorhynchus hectori) with in-situ data on environmental characteristics and prey to identify the statistical correlates of distribution and to assess ‘what makes hotspots unique’.
Methods: Between 2014 and 2017, ca. 300 surveys were carried out at hotspots and areas not routinely used by dolphins at Banks Peninsula on the east coast of New Zealand’s South Island. A broad range of prey and environmental variables were explored as drivers of dolphin distribution using generalized additive models, and principal component analysis was employed to determine a key environmental signature for hotspots.
Results: The relative abundance of dolphins was strongly correlated with prey abundance and a range of environmental variables representing habitat type and oceanographic conditions. The combination of high prey abundance and sandy, shallow, high current and low turbidity habitat was strongly represented at hotspots.
Discussion: These characteristics are also likely attributes of habitat with high ecological value generally, being related to high biodiversity, productivity, naturalness and ecosystem function. This study showcases the importance of targeted investigations into the characteristics of species hotspots to better guide the management of important areas for the conservation of both species and ecosystems.
1 Introduction
Many studies show that marine wildlife populations have ‘hotspots’ of distribution (Hastie et al., 2004; Gende and Sigler, 2006; Scott et al., 2010). What drives the occurrence of hotspots is less well known. In particular, the ecological factors that make hotspots unique are poorly understood; there are few studies that directly assess the unique characteristics of these areas. Species distribution modelling (SDM) suggests that habitat use by marine predators is shaped by physical habitat type (Torres et al., 2008b; Eierman and Connor, 2014), hydrological regime (Johnston et al., 2005; Yen et al., 2006), or a combination of both (Bailey and Thompson, 2010; Embling et al., 2012). In reality, these habitat characteristics are often proxies for the distribution of prey which is not often directly measured (see Gende and Sigler, 2006; Torres et al., 2008; Hazen et al., 2011; Barlow et al., 2020; Bennington et al., 2020b for exceptions). While SDMs are useful for determining environmental correlates of species occurrence or abundance, there is an explicit assumption that such correlates are representative of ‘good-quality’ habitat. This assumption may not necessarily be valid where environmental covariates represent temporally transient environmental conditions (e.g., seasonal temperatures), or when covariates serve as proxies for the environmental characteristics that are the true attributes of hotspots. For example, Derville et al. (2016) showed that temperature is a key factor in determining habitat use of humpback whale breeding habitat which is likely related to the physiological requirements of the species during this critical life-history stage. Thus, temperature is a true characteristic of critical habitat for this species. Temperature has also been shown to be related to the distribution patterns of a range of coastal delphinids (Bräger et al., 2003; Torres et al., 2008; Gilles et al., 2016). However, Torres et al. (2008) demonstrated that, while temperature is a strong predictor of bottlenose dolphin distribution, it is likely a proxy for the availability of prey. In this case, prey abundance, not temperature would be considered the fundamental characteristic of important habitat.
Understanding the characteristics of important habitat has obvious benefits for the protection and management of species. If the characteristics of hotspots are well understood, it will be easier for management to target the preservation of these characteristics and provide more effective protection of the biodiversity they support (Hooker et al., 2002; Hooker et al., 2011). For example, due to an appreciation of the requirement of seagrass meadows for dugong (Dugong dugon), management has been able to target stressors to this critical habitat including bottom-contact fishing practices and the impacts of sedimentation and land-derived pollutants (Marsh et al., 1999). This protection of critical seagrass habitat inevitably benefits all species that rely on it (Marsh et al., 1999). In this way, understanding the characteristics of hotspots in megafauna distribution may enable the determination of ecological features (e.g., habitat types, oceanographic conditions, species interactions) of particular importance to a certain ecosystem. The association of megafauna distribution with ecologically important habitats underpins the ‘indicator species’ concept for these taxa, i.e., hotspots of distribution are also locations with high ecological value and/or high quality habitat (Hooker and Gerber, 2004; Azzellino et al., 2014). These habitats often support a wide range of other species and thus the protection of megafauna hotspots may also benefit other taxa (i.e., the flagship/umbrella species concept (Bifolchi and Lodé, 2005; Kalinkat et al., 2017)
A broad range of environmental covariates are used to determine drivers of distribution for marine megafauna, including variables that represent physical and biological processes, or the environmental signals of those processes (Macleod et al., 2004; Johnston et al., 2005; Torres et al., 2008; Doniol-Valcroze et al., 2012; Bouchet et al., 2015; Saijo et al., 2017). There may also be physiological constraints on some taxa (e.g., diving depth, temperature tolerance) that cause environmental characteristics to have a direct influence on habitat selection (Benoit-Bird et al., 2013; Bennington et al., 2020a). Other than primary productivity, variables that directly represent ecologically important attributes (e.g., hotspots for biodiversity, habitat heterogeneity, ecosystem services) are rarely incorporated as factors influencing megafauna distribution. Knowledge of the relationships between attributes of ecological importance and megafauna distribution is useful for understanding how species are sustained by features of habitat that are often the focus of habitat-based management (Hooker and Gerber, 2004). Ultimately, this knowledge can underpin management decisions for the protection of key coastal habitat types.
Hector’s dolphin (aka New Zealand dolphin, Cephalorhynchus hectori), is a small (<1.5 m), endangered, coastal delphinid species that is endemic to New Zealand (Dawson, 2017). While populations of Hector’s dolphin have experienced substantial decline due to fisheries bycatch (Slooten et al., 2000), they remain locally abundant at Banks Peninsula on the east coast of the South Island (Figure 1) where they have been studied for over three decades (Dawson and Slooten, 1993). At Banks Peninsula, Hector’s dolphins have well documented fine scale (<5 km) hotspots in their nearshore distribution (<1 km from shore) that have been consistent for several decades (Brough et al., 2019a; Brough et al, 2020). Hector’s dolphins also have one of the smallest home-range sizes of any cetaceans (Rayment et al., 2009) and inhabit coastal habitat that is subject to a range of stressors including high vessel traffic and tourism pressure (Stone and Yoshinaga, 2000; Carome et al., 2022), sedimentation (Hart et al., 2009), habitat modification (Brough et al., 2014; Leunissen and Dawson, 2018), pollution (Stockin et al., 2010) and fisheries impacts (Dawson, 1991; Slooten and Dawson, 2008). Such habitat-use characteristics, coupled with their reliance on coastal habitat, makes Hector’s dolphins an ideal candidate for an investigation into the biophysical attributes of hotspots. In this study, we aim to identify the physical (e.g., topographical, oceanographic) and biological (e.g., prey) factors that shape the distribution of Hector’s dolphin within critical nearshore habitat. Further, we aim to determine how the perceived drivers of distribution are represented at known hotspots for the species to confirm whether such variables are representative of good quality habitat. We hypothesize that, due to high energetic requirements (Wisniewska et al., 2016), the availability of prey will be a key driver for this small, coastal delphinid. Further, alongside prey availability, we hypothesize that hotspots will be represented by a distinct combination of habitat variables – a combination that makes these locations biophysically unique. The knowledge generated by this study will provide conservation managers with opportunities for targeted interventions that limit degradation of the important ecological features for this endangered species. Furthermore, it will facilitate identification of features that may contribute to wider biodiversity values in the New Zealand coastal environment.
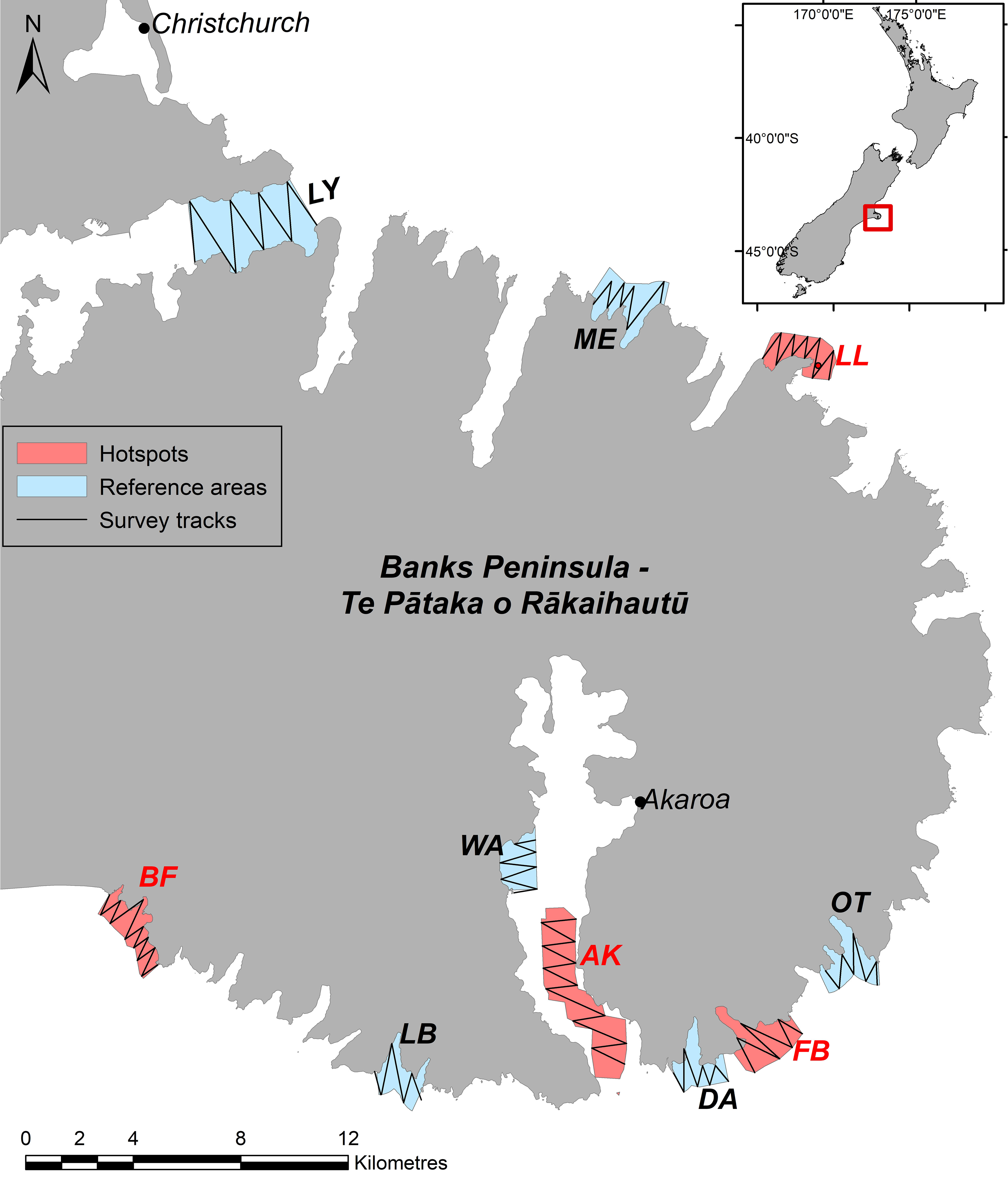
Figure 1 The location and extent of hotspots and reference areas that were the focus of targeted habitat-dolphin surveys. The survey areas are; Birdlings Flat (BF), Long Bay (LB), Wainui (WA), Akaroa (AK), Damons Bay (DA), Flea Bay (FB), Otanerito (OT), Long Lookout (LL), Menzies Bay (ME) and Lyttleton (LY). Examples of randomized survey tracks are given for each survey area, and the location of Banks Peninsula along the east coast of New Zealand’s South Island is shown (inset).
2 Materials and methods
Systematic visual surveys of Hector’s dolphins were used to collect data on the relationship between the distribution of dolphins and the characteristics of their habitat. ‘Zig-zag’ surveys were carried out in nearshore habitat, within four defined ‘hotspot’ and six ‘reference’ areas over summer (January-April) and winter (August-October) seasons between 2014 and 2017. The hotspots and reference areas were defined by Brough et al. (2019a), using density analyses of ca. 10,000 sightings of dolphin groups made over three decades. The start point of each survey was randomly generated to enable surveys to encounter habitat types at random within each survey area. Random start points and the different sizes of survey areas caused surveys to be of variable extents ranging between 2.2 and 7 kilometers in length, and between 15 and 45 minutes in duration. Surveys were undertaken in a 6 m powerboat, with two observers scanning port and starboard sectors of a sighting zone defined by the bow and beam of the vessel. All sightings of dolphin groups, the number of dolphins in each group, the sighting conditions, and the survey track were recorded using CyberTracker (CyberTracker Ltd) software on an Android tablet. The estimation of dolphin group size can be highly subjective and subject to significant observer bias (Gerrodette et al., 2019). As the number of dolphins encountered during surveys was the key variable used for modelling (see below), we undertook to minimize such bias as much as practicable by; 1) limiting surveys to sea conditions conducive to making accurate group-size estimates (Beaufort<3, <1m swell) and 2) using the same small number of observers (3-4) for the duration of the study and providing training to standardize group size estimation. For 2, this ensures that any error in group size estimation is similar across observers, minimizing the effect of observer bias on analyses of relative abundance. Further, Hector’s dolphins mostly occur in small groups (<10 individuals) and are highly vessel positive (DawsonE. and Slooten, 1988), likely increasing the accuracy of group-size estimation for this species. For example, Gerrodette et al. (2019) found dolphin group sizes to be underestimated by less than 1% for groups fewer than 25 individuals. The median group size estimate in our study was five. Thus, along with our methods to reduce observer bias, the behavior of the species likely ensures any error in group size estimates is minimized so as not to impact our analyses.
2.1 Prey data
Data on the relative abundance and patch characteristics of prey were collected concurrently during the dolphin-habitat surveys using hydro-acoustics (Simrad ESS7 echosounder, see Brough et al., 2019b for detailed methods). The relative abundance of Hector’s dolphins showed a strong relationship with availability of potential prey represented by epipelagic schooling species (e.g., slender sprat – Sprattus antipodum, yellow-eyed mullet – Aldrichetta forsteri) and the depth of fish schools (Brough et al., 2019b). Thus, these variables were included as candidate variables in the dolphin-habitat models used to investigate the drivers of distribution.
2.2 Environmental data
Fine scale data on seafloor habitat types for each hotspot and reference area were available from seafloor mapping studies carried out using side scan sonar and single beam echosounders (Brough et al., 2018). Physical habitat types were classified to level 2 (abiotic habitats) using the proposed NZ thematic marine habitat classification (Dohner, 2013) and were ground-truthed using a combination of Ponar sediment grabs and drop-camera deployments. Following Sanciangco et al. (2013), a modification of the Shannon-Weiner index (Shannon and Weaver, 1963) was used to represent habitat heterogeneity calculated as an index (0-1) based on the number and aerial extent of seafloor habitat types encountered during a survey. High resolution bathymetric data (5 m x 5 m) from surveys of the Banks Peninsula nearshore environment using single-beam echosounders (Brough et al., 2018) provide fine scale information on depth, slope and aspect of the seafloor for each survey area, the latter two variables being calculated using the package raster (Hijmans, 2019) in R (R-Core-Team, 2018).
Data on the velocity of tidal currents throughout the study area were available from a regional oceanographic modelling system (ROMS) constructed for Banks Peninsula (Soutelino and Beamsley, 2015). ROMS data were used to generate mean velocity curves across the semi-diurnal tidal cycle for each survey area. A single, survey-specific tidal current velocity value was extracted using the recorded tidal state at the time and location of the mid-point of each survey. Data on local oceanographic conditions at each location at the time of a survey were derived from several sources. Sea surface temperature (SST) datasets were collected alongside hydro-acoustic data in the dolphin-habitat surveys using an onboard temperature sensor within the hydro-acoustic system. The sensor was calibrated every two weeks using an RBR Concerto (RBR Ltd). Temperature at the transducer face was down sampled to 1 Hz, and gridded into 100 m x100 m bins along the survey track. From this grid, the mean, standard deviation (SD) and horizontal gradient in SST was calculated for each survey.
Remote sensing datasets on Chlorophyll a concentration and diffuse attenuation coefficient (KD490) were accessed as level 3 data products of the MODIS Aqua sensor from NASA’s ocean color website (NASA Goddard Space Flight Center et al., 2021). Both variables are regularly used in species distribution models for marine megafauna as proxies for primary productivity (e.g., Torres et al., 2008; Hazen et al., 2012) and water turbidity (e.g., Gomez and Cassini, 2015; De Wysiecki et al., 2020) respectively. Daily datasets were downloaded at 4 km resolution and were processed in SeaDas (v 7.5.1; OBPG) and in R package raster (Hijmans, 2019) to provide pixel values for the closest cell to the centroid of each dolphin-habitat survey, at the nearest possible date. Surveys were carried out with variable, but high frequency during the summer and winter seasons; often with multiple surveys of a given survey area during a week. Due to this high frequency and the fact that conditions change rapidly in this exposed, temperate habitat, remote sensing data with high temporal resolution (i.e., daily) were necessary. The date of variable availability depended largely on local weather and sea conditions but was typically within three days of the survey.
A database that summarized the physical habitat types, bathymetric variables, current velocity, oceanography, prey field characteristics, survey effort and relative abundance of dolphins for each survey was generated. Data on the physical habitat types and bathymetry were extracted from feature and raster layers in ArcMap (v. 10.3; ESRI). A digitized track-line of each survey was imported and a buffer with a 400 m (i.e., max sighting distance; Brough et al., 2019a) radius placed around the track. The resultant ‘survey polygon’ reflected the total area surveyed for each dolphin-habitat survey. The total area and percent coverage of each physical habitat type with the survey polygon was calculated. The mean and SD of the depth and slope bathymetric surfaces that overlapped a survey polygon were calculated and exported for each survey. A velocity value for the location specific tidal state was extracted from the tidal velocity models using R package raster (Hijmans, 2019). Variables derived from remote sensing datasets were imported into the database and matched against the surveys. Every survey had in-situ measures of prey relative abundance and mean school depth. The number of dolphins (n dolphins) encountered during a survey was the measure of dolphin relative abundance used for modelling. A broad range of habitat characteristics were included as candidate variables (Table 1), with multiple variables often representing single characteristics. For example, depth is represented by both mean depth and the standard deviation of depth which provides a measure of seafloor unevenness. In several instances, multiple variables from a single characteristic were correlated, which led to the removal of variables in the concurvity exercise (see below).
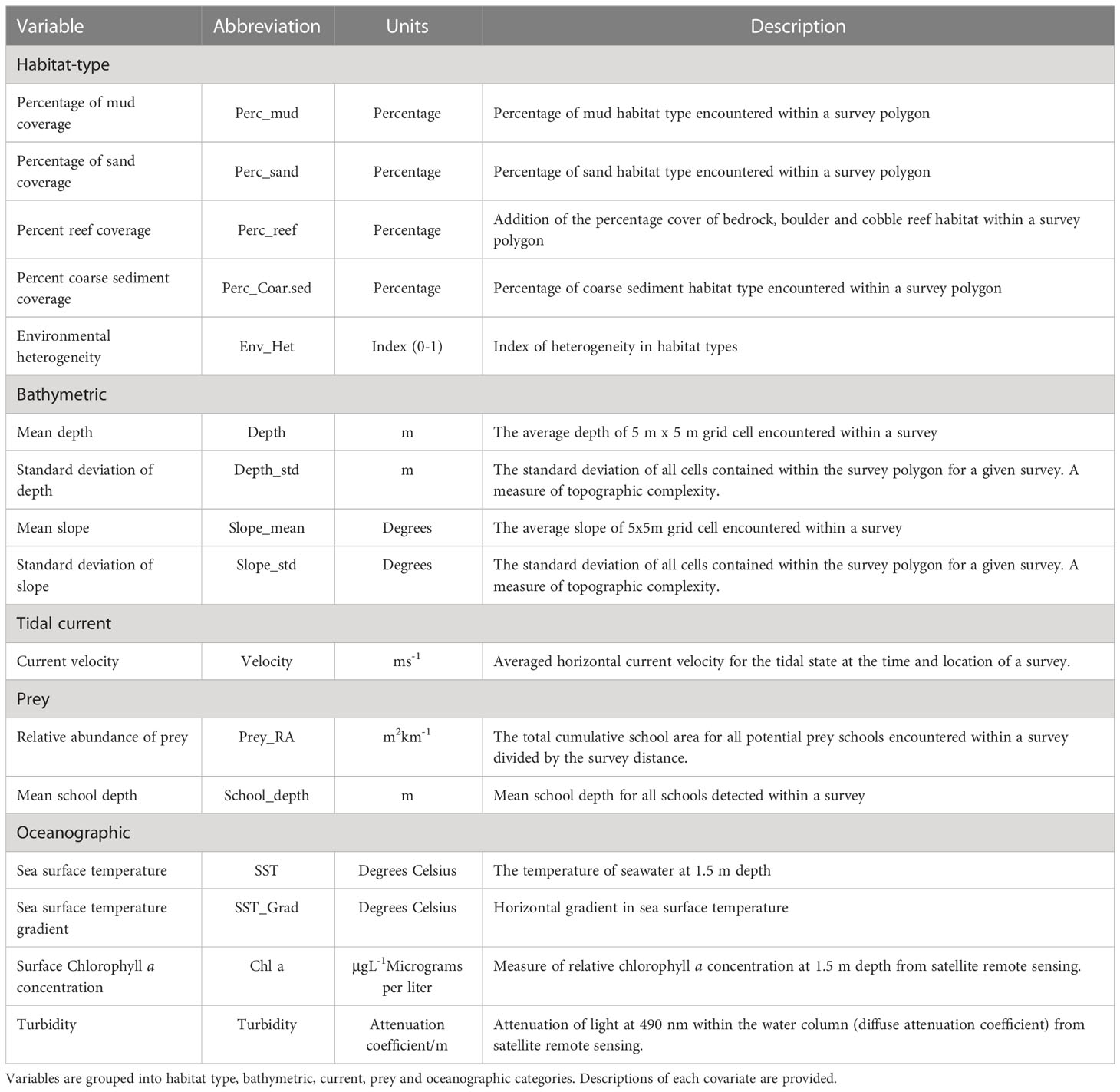
Table 1 A summary of the of environmental covariates considered in modelling dolphin-habitat relationships.
2.3 Statistical approach
To confirm differences in relative abundance between hotspots and reference areas, a generalized linear model (GLM) was fit in R with the number of dolphins encountered at each survey as a response and the survey area (Figure 1) as a single categorical predictor. The GLM was fit with a negative binomial distribution and incorporated the distance of each survey as an offset. Tukey’s post-hoc tests were used to test the difference in relative abundance between each pairwise comparison of the four hotspot areas with the six reference areas (n= 24 pairwise comparisons). Tukey’s post-hoc tests were implemented in the R package multcomp (Hothorn et al., 2008).
The relationship between the relative abundance of dolphins (n dolphins) during each survey and the physical and biological characteristics of habitat were modelled under a species distribution modelling (SDM) framework using generalized additive models (GAMs; Hastie and Tibshirani, 1987). GAMs were fit using the package mgcv (Wood, 2017) in R with a negative binomial distribution. Correlation (linear and non-linear) among environmental covariates was investigated using the concurvity function in mgcv (Wood, 2006; Wood, 2017) using a threshold of 0.3 above which terms were considered correlated (He et al., 2006; Bennington et al., 2020b). When concurvity between variables was apparent, the decision of which to retain was based on the AICc values returned by single variables GAM models that fit the variable in question against the response (n dolphins, with an offset for survey distance). The variable that produced the model with the lowest AICc value was retained. A global model containing all uncorrelated covariates was then fit in mgcv. Variables included in the global model were: Prey_RA, Sand, Perc_Reef, Velocity, Slope, SST, SST_Grad, Turbidity and Env_Het (see Table 1). Each continuous variable was fit as a smoothed function using penalized thin plate regression splines, and the degrees of freedom for each term were limited to maximum of 5 (i.e., 4 knots; (Tepsich et al., 2014; Rayment et al., 2015) in order to minimize the chances of overfitting impacting model selection and interpretation. A model set that contained a formulation for every possible combination (and inclusion/exclusion) of candidate variables contained within the global model was constructed and each model (n=2038) fit separately. Model selection was undertaken using AICc (Hurvich, 1989) and model weight scores (Burnham and Anderson, 1998), with the ‘top-model’ being the formulation with the lowest AICc and highest model weight. The importance of habitat variables was determined in a hierarchical approach using three steps; 1) Variables retained in the top-model were considered to have some effect on the relative abundance of dolphins, 2) The strength of a variable’s effect was visually identified by viewing the values of the y-axis for areas on GAM partial dependence plots where the 95% credible interval does not overlap zero. The values on the y-axis of such plots indicates the strength of the effect on the response variable (Wood, 2006). 3) As a further test, the approximate significance of each smoothed-term was extracted from the top-model. Variables with p-values < 0.05 are considered to have a significant (α=0.95) influence on the relative abundance of dolphins.
The global and top-models were checked for the validity of assumptions using a combination of diagnostic plots and the gam.check function within mgcv. The extent of spatial auto-correlation among input observations (i.e, surveys at the same location) was investigated by generating spatial correlograms and bootstrapped 95% confidence intervals (Zuur et al., 2009). To assess goodness of fit and the predictive capacity of the top-model the deviance explained was summarized and a validation procedure established. For model validation, the full dataset was randomly split into a ‘training’ set that contained two thirds of the total dataset and an ‘evaluation’ set containing the remaining third. The top-model was fit using the training data and used to predict values on the response scale given the values of the variables in the evaluation data. The validation procedure was bootstrapped 100 times with different, randomly selected training and evaluation data. The mean Spearman’s correlation coefficient was used as a measure of correlation between the predicted and observed values (Guisan and Zimmermann, 2000; Grüss et al., 2016), with a value higher than 0.50 indicating good predictive capacity (Grüss et al., 2016; Waldock et al., 2022).
To link the habitat covariates deemed important by the GAMs to the existence of hotspots, we used two complementary approaches. Firstly, a ‘GAMvelope’ (Torres et al., 2008) was used to extract the values of the environmental covariates that had a positive relationship with the abundance of dolphins. A GAMvelope describes the area on a GAM curve where values of a habitat variable (x-axis) have a positive effect on the response (y-axis) (Torres et al., 2008; Tepsich et al., 2014; Correia et al., 2015). Extracting these data and examining how they are represented spatially among survey locations allows an appraisal of the location of important habitat (Torres et al., 2008). We extracted all surveys with positive GAMvelope values and used a Chi-squared test to investigate the null hypothesis of no difference in the occurrence of positive GAMvelopes between hotspots and references areas. The test was undertaken for each variable retained in the top-model.
Secondly, principal component analysis (PCA; Jolliffe, 2002) was undertaken with each dolphin-habitat survey as an input observation and the combination of all environmental covariates retained in the top-model used to calculate principal components. The PCA was undertaken using the prcomp function in R and plotted to provide a visualization of any apparent clustering of observations among hotspots and reference areas. The alignment of each variable vector (arrows) with each PCA axis shows the correlation between a variable and the principal components, while the length of each vector is indicative of the contribution of each variable (Jolliffe, 2002). Further, correlations of clusters of observations with the magnitude and direction of vectors of environmental variables in a PCA bi-plot can be used to distinguish the unique habitat characteristics of observations in PCA space (Pitcher et al., 2011; Stephenson et al., 2018).
3 Results
Two hundred and ninety-six dolphin-habitat surveys were carried out across the ten survey areas, representing 1246 km of survey effort (Table 2). Sixty-five percent of surveys were carried out in summer seasons. The ratio of summer/winter surveys was similar across survey areas (range 1.7 – 2.5) apart from Otanerito Bay, which had 6 times more surveys in summer. The number of surveys varied across areas, but each received at least 12. In general, Hector’s dolphins were significantly more abundant at hotspot locations (AK, BF, FB, LL; Table S1) compared to the reference areas. This trend was true for all pair-wise comparison of hotspots and reference areas with the exception of Long Bay (LB) for which there were no significant differences in abundance compared to each hotspot. (Table S1)
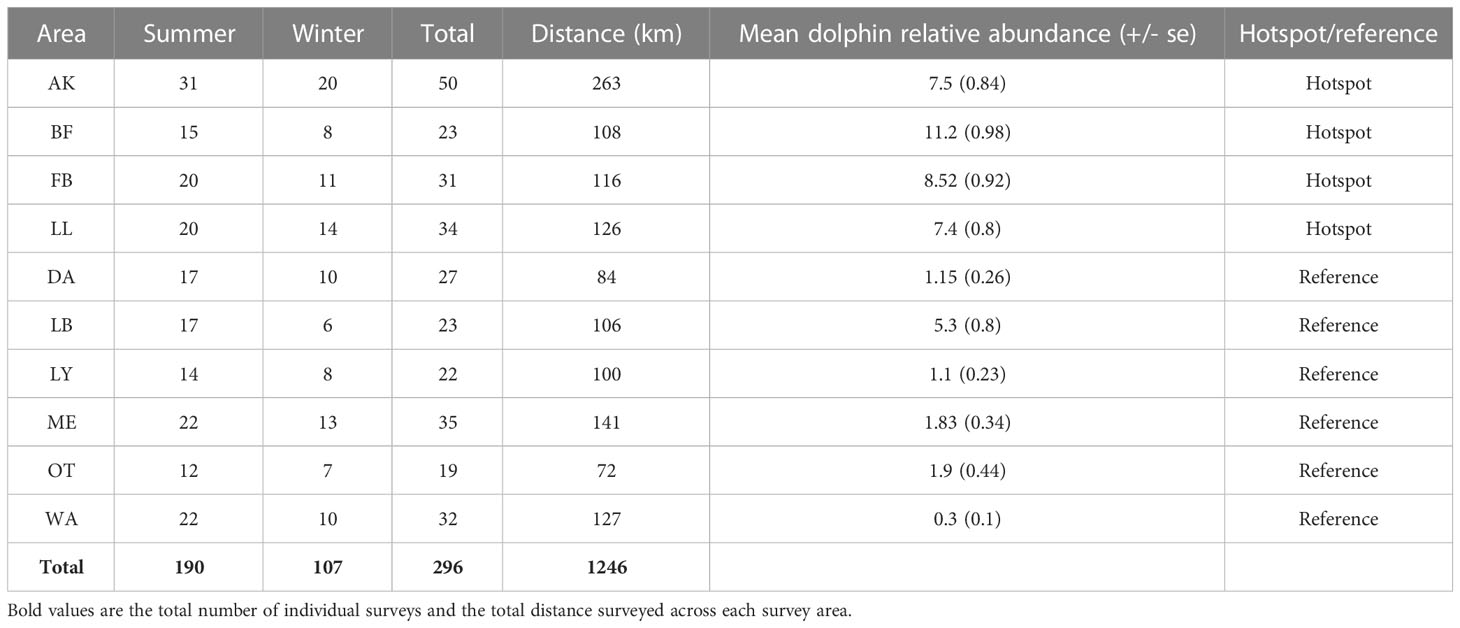
Table 2 Summary of survey effort and the relative abundance of dolphins (dolphins/km) encountered during surveys of hotspots and reference areas between 2014 and 2017.
In terms of habitat types, hard substrate was generally confined to a coastal fringe with the most common habitat types being sand and mud. Depth varied across the survey areas, ranging from 0.5 to 40 m. Steep slope gradients were rare at all locations, with the steepest values being associated with the coastal reef margin. There were substantial differences in the velocity of tidal currents among areas, the highest averaged current velocities were at Long Lookout (0.4 ms-1 just before low tide). Mean SST for surveys ranged between 5 and 19°C, while SST gradient was between 0.05 and 1.9°C. Remotely sensed chlorophyll a concentration and turbidity (KD490), were observed between 0.3 and 12 mgm-2 and 0.05 and 0.84 m respectively. The relative abundance of prey was between 0 and 2605 m2km-1 and the mean depth of schools ranged from 4 to 25 m.
After accounting for collinearity, variables that described the major physical, bathymetric, oceanographic and prey variable groups were able to be included in the global model; chlorophyll a concentration (highly correlated with turbidity) and prey school depth (highly correlated with mean depth) were omitted in preference of better performing covariates. Using the information-theoretic approach to model selection, the top-model included the same habitat covariates as the global model except for slope (Table 3). The top-model explained 47% of the deviance of the relative abundance of dolphins and had a model weight of (0.18), compared to the second-ranked model (0.14). The full top-model set (models with weight > 0.01; Burnham and Anderson, 1998) is provided in Table S2 in the supplementary material. Each of the variables retained in the top-model had a statistically significant influence on the relative abundance of dolphins, with the exception of perc_reef and depth (Table 4).
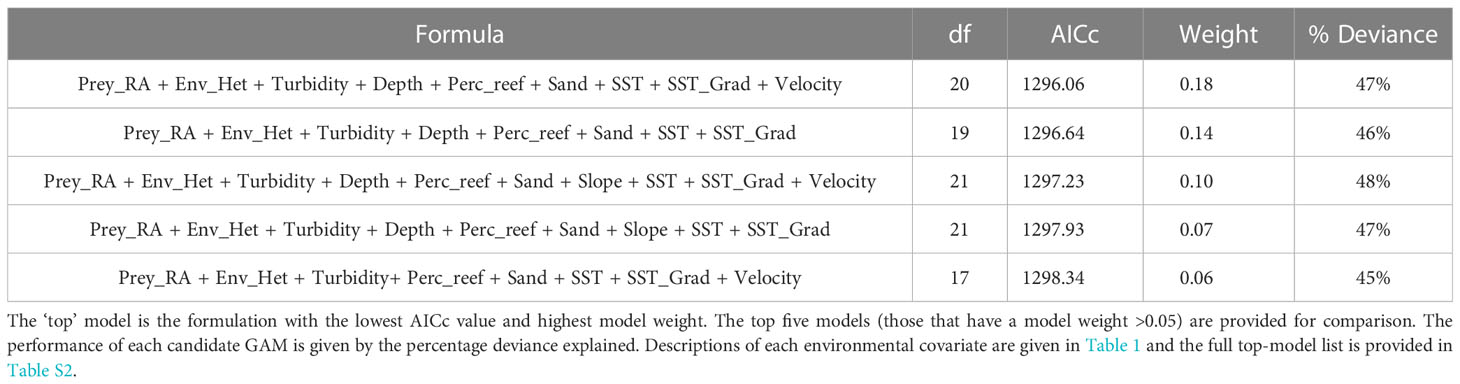
Table 3 Model selection table used to determine the most parsimonious GAM for explaining the relationship between environmental covariates and the relative abundance of Hector’s dolphins.
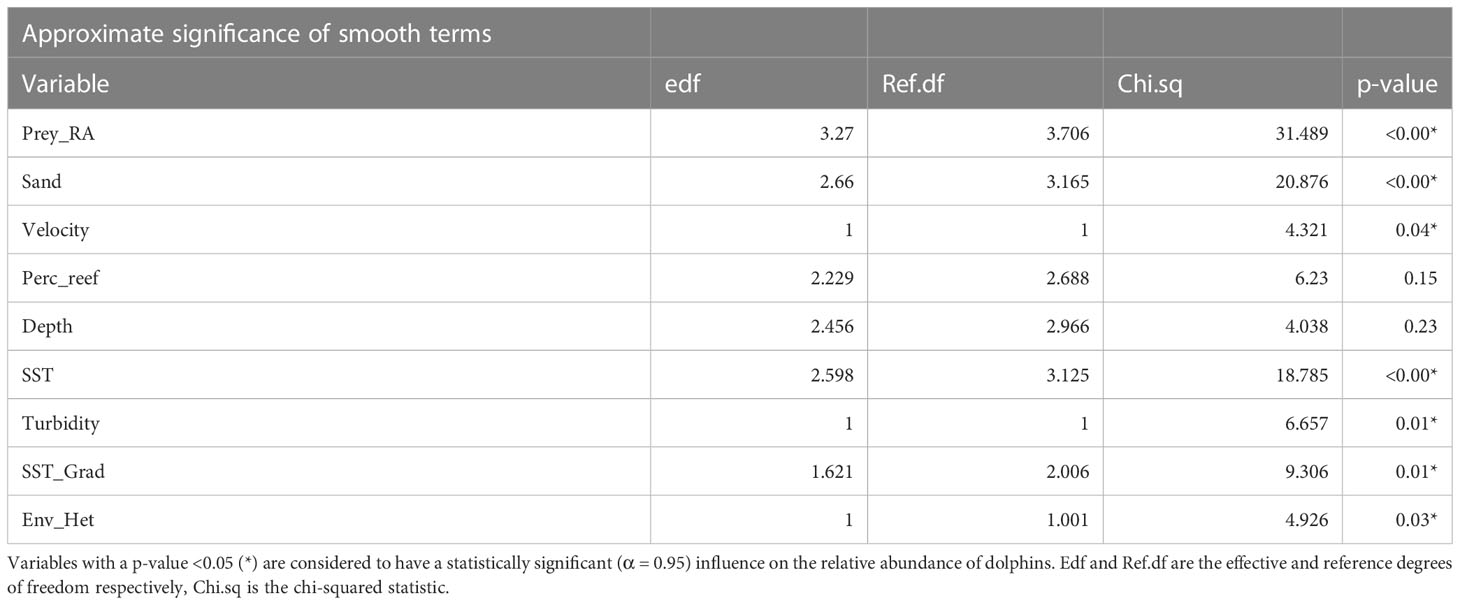
Table 4 The approximate significance of variables from the top-model used to quantify the relationship between the relative abundance of dolphins and environmental covariates.
The relative abundance of potential prey was significantly (p-value <0.001) correlated with the relative abundance of dolphins, with the strength of the effect increasing with higher prey abundance, although the effect plateaued after approximately 500 m2km-1 (Figure 2 and Table 4). At low values, the percentage of sand habitat encountered within a survey had a significant (p-value <0.001) negative influence on dolphin abundance, with a strong positive effect above approximately 20% (Figure 2). Current velocity had a significant (p-value = 0.04) linear relationship with dolphin abundance, where abundance increased with increasing velocity (Figure 2 and Table 4). The percentage of reef habitat showed a negative relationship with dolphin abundance at values higher than 15% and a positive effect between 5 and 10% (Figure 2), however this relationship was not statistically significant (p-value = 0.15). The mean depth encountered during a survey indicated an optimum depth between 12 and 22 m, however, the confidence interval of the effect overlapped 0 for the entire range of values and this relationship was not statistically significant (p-value = 0.23, Table 4). The relative abundance of dolphins was significantly (p-value <0.001) influenced by SST, with lower values (around 6°C) having a strong negative effect and a temperate range between approximately 12 and 17°C where the influence was positive. Remotely sensed values for turbidity had a significant (p-value = 0.01), linear relationship with dolphin abundance (Table 4), where low turbidity was associated with higher abundance; however, the strong relationship between very high turbidity (>0.5 m-1) and low dolphin abundance is based on two observations only (Figure 2). Similarly, the effect of the mean SST gradient was influenced by a smaller number of observations with strong gradients (>1°C), with the general trend suggesting strong gradients have a significant (p-value = 0.01) positive correlation with dolphin abundance. Environmental heterogeneity had a significant (p-value = 0.03) linear relationship with dolphin abundance (Table 4), where more homogenous habitat was correlated with higher abundance in contrast to habitats with higher heterogeneity (Figure 2).
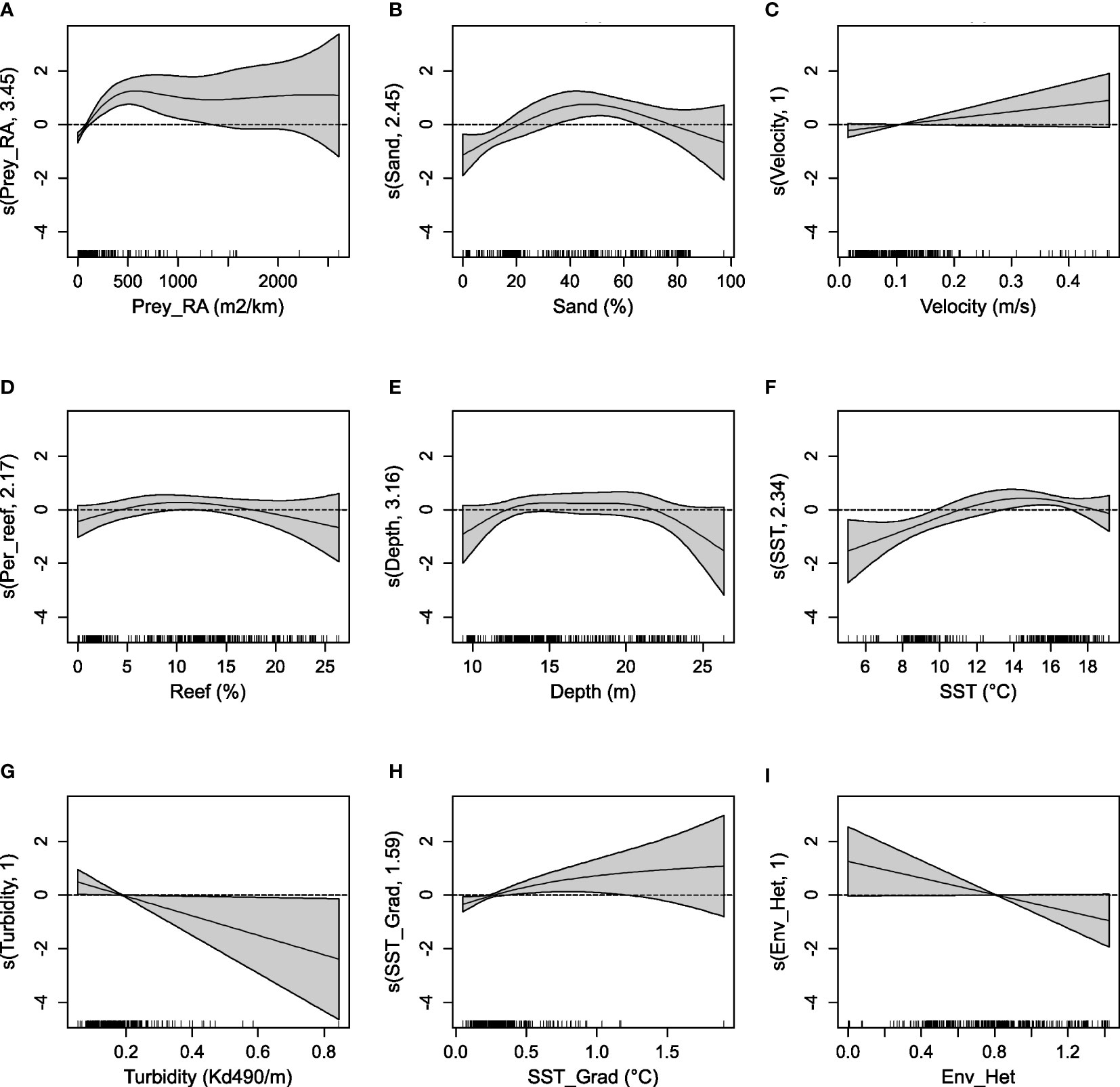
Figure 2 The influence of the environmental covariates retained in the top GAM on the relative abundance of dolphins encountered in each dolphin-habitat survey. Plots show the influence of the relative abundance of prey (A), the percent sand (B), tidal velocity (C), percent reef (D), mean depth (E), sea surface temperature (F), turbidity (G), temperature gradient (H), and habitat heterogeneity (I). Positive effects on the relative abundance of dolphins are indicated where the mean smoothed term is above 0, while negative effects are indicated where the term is below 0 and the magnitude of y-axis indicates the strength of a given effect. The shaded area represents the 95% credible interval for each smoothed term and the rug-plot above the x-axis shows the distribution of observations for each covariate. The y-axis shows the effective degrees of freedom associated with each smoothed term.
The model validation procedure using 100 bootstraps of randomly selected training and evaluation data resulted in a mean Spearman’s correlation coefficient of 0.692 (95% confidence interval 0.688 – 0.697) between predicted and observed values indicating good model performance (Grüss et al., 2016; Waldock et al., 2022).
Significant differences (Chi-squared p-value <0.05) in the proportion of surveys with positive GAMvelopes between hotspots and reference areas revealed the habitat characteristics that are more common at hotspots (Figure 3). Compared to reference areas, a higher proportion of surveys at hotspots were dominated by sandy substrate (χ2 = 34.63, p<0.001) that was positively correlated with dolphin abundance. Similarly, a greater proportion of surveys at hotspots had high prey abundance (χ2 = 3.79, p=0.04) and tidal current velocity (χ2 = 18.04, p<0.001) when compared to reference areas. Compared to reference areas, the mean depth (χ2 = 9.51, p=0.002) observed at hotspots was more commonly within the preferred depth range (ca. 12.5 – 22 m). Preferred values of environmental heterogeneity were not significantly more common at hotspots (χ2 = 0.02, p=0.8862). There was no or limited difference in the proportion of surveys containing positive GAMvelope values between hotspots and reference areas for the percentage reef (χ2 = 0.02 p=0.896), SST (χ2 = 0.50, p=0.481) and SST gradient χ2 = 0.06, p=0.683) (Figure 3). Conversely, reference areas more often had lower turbidity (χ2 = 607, p=0.014) which were associated with higher dolphin abundance.
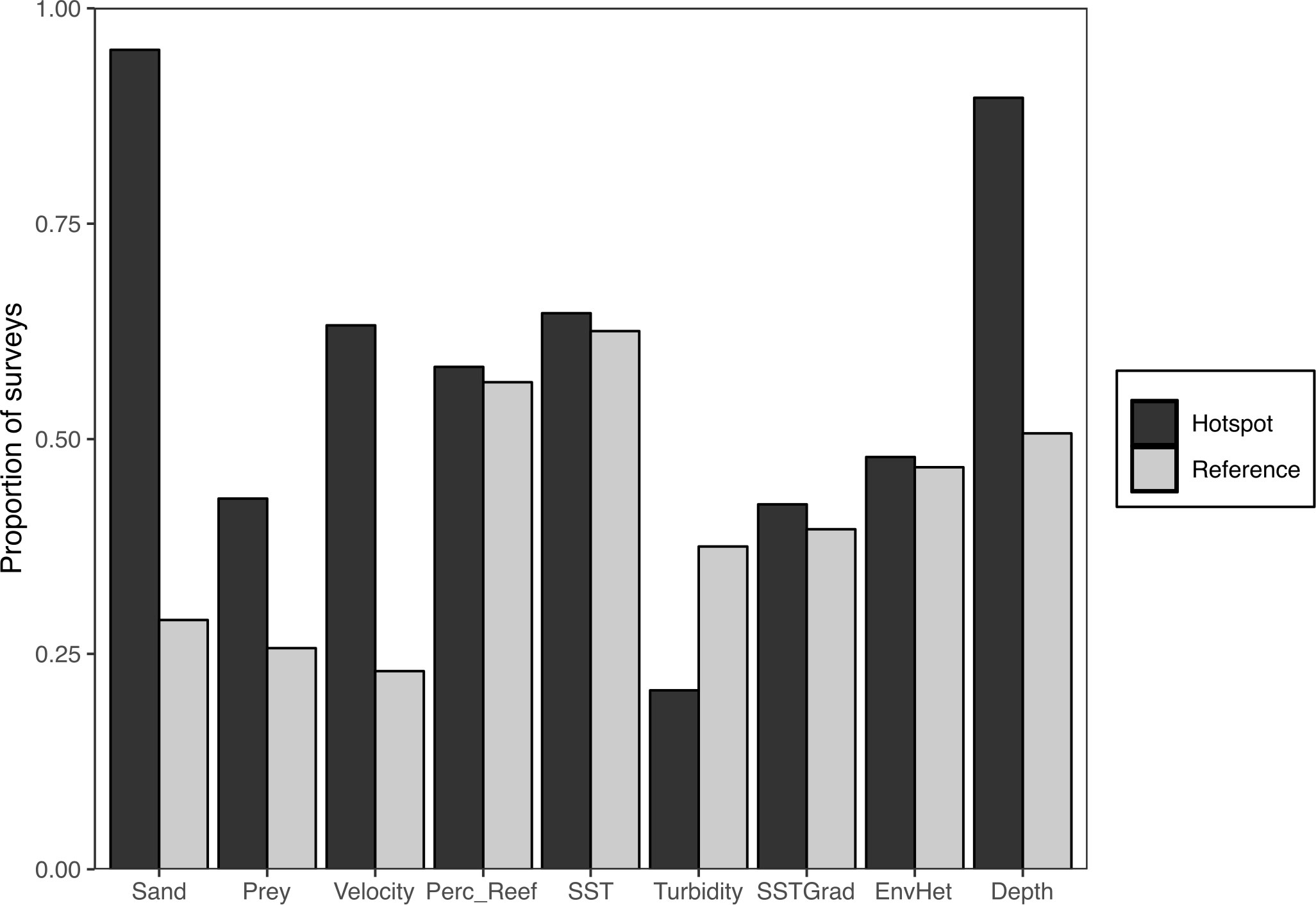
Figure 3 The proportion of surveys with positive ‘GAMvelopes’, where the observations of environmental covariates have a positive influence on dolphin abundance, among hotspots and reference areas. GAMvelopes were summarized for each of the covariates retained in the top GAM.
The PCA of observations of habitat characteristics provided further insights into the combination of biophysical features that distinguish hotspots and reference areas. The first three principal components had eigenvalues above 1, suggesting importance for describing variation among observations under the Kaiser-Guttman rule (Jolliffe, 2002). Cumulatively, the first three components explained 67% of the variance in observations of habitat characteristics. However, the first two principal components were sufficient to distinguish obvious clustering between observations from hotpots and reference areas (Figure 4). PCA1 is associated with prey abundance, depth and turbidity, while PCA2 has strong influences from tidal current velocity and sand, and correlations with SST and SST gradient (Figure 4). Generally, observations from hotspots were correlated with prey abundance, proportion of sand and current velocity. However, the wide spread of observations from hotspots in PCA space suggests that such habitat features combine in complex ways to contribute to good quality dolphin habitat. Reference areas were represented by two discrete clusters in PCA space. One cluster was correlated with environmental heterogeneity, percentage reef cover and depth, with the other being strongly influenced by turbidity (Figure 4).
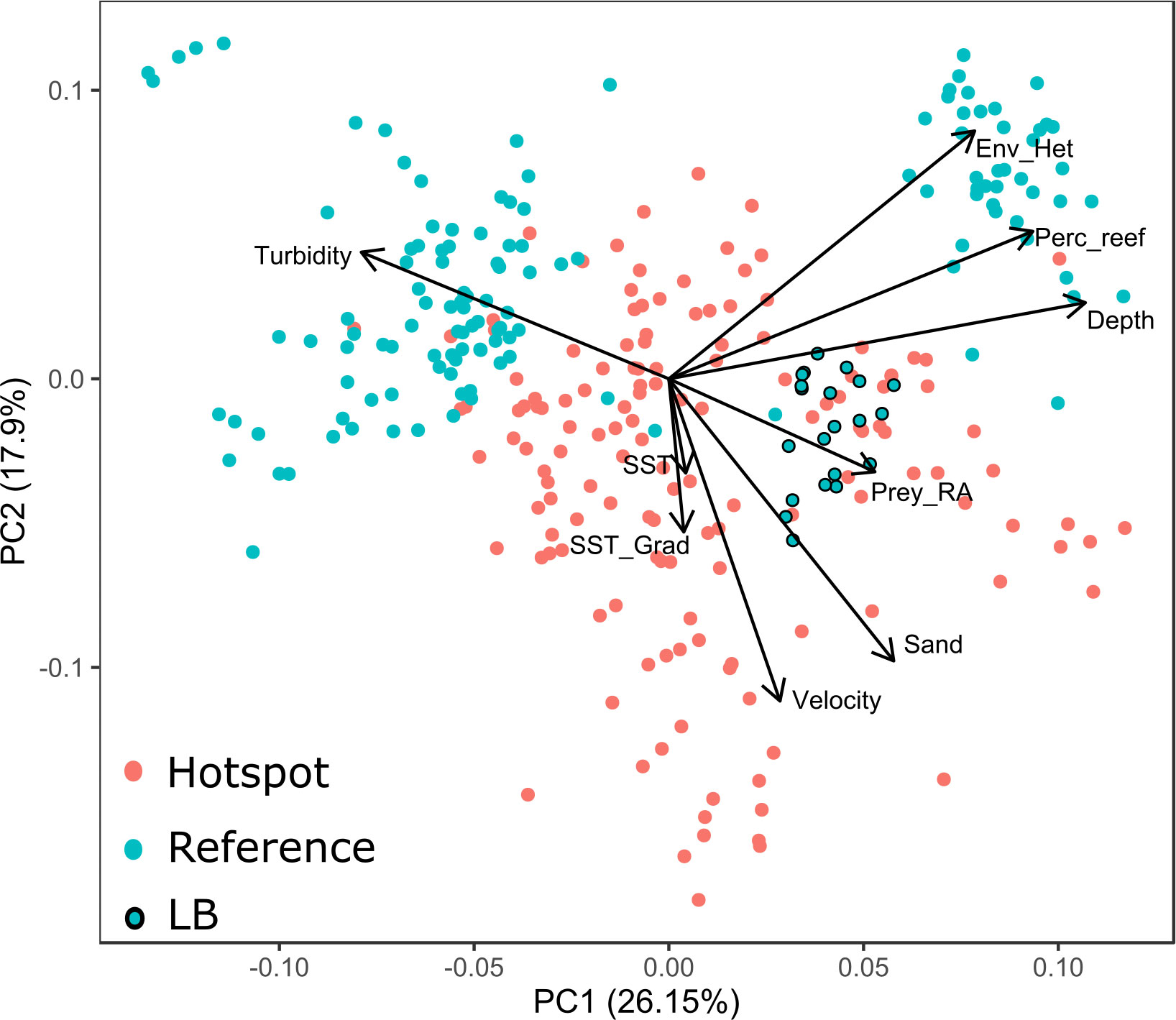
Figure 4 Biplot of a principal component analysis used to investigate the clustering of observations of environmental covariates among hotspots and reference areas. The reference area ‘LB’ is distinguished due to an environmental signature similar to hotspots. Points are given for each of the 296 dolphin-habitat surveys. The direction of an arrow relative to each PCA axis indicates how a covariate contributes to the separation of observations in PCA space, while the length of each arrow indicates the magnitude of the covariate’s contribution to each component.
4 Discussion
4.1 Influence of the environmental covariates
We integrated intensive surveys of Hector’s dolphins (Cephalorhynchus hectori) at Banks Peninsula with data on environmental characteristics and prey to identify the statistical correlates of dolphin distribution. Among all the variables tested, the relative abundance of potential prey had one of the strongest correlations with relative abundance of dolphins. When prey were more abundant, so were the dolphins. This finding confirms our hypothesis of prey being an important driver of distribution in this species. Many studies have shown the spatial and temporal overlap between marine top-predators and their prey (Baumgartner et al., 2003; Fauchald, 2009; Benoit-Bird et al., 2013; Barlow et al., 2020). Especially for small-bodied marine mammals inhabiting temperate areas, the need for frequent foraging to satisfy high energetic requirements (Harding et al., 2005; Wisniewska et al., 2016) means that these taxa are likely to exhibit particularly high overlap with prey. In studies that combine both prey and environmental variables as drivers of marine mammal habitat use, Embling et al. (2012) and Hazen et al. (2011) found that both prey and habitat covariates were important drivers of abundance, while Torres et al. (2008) found habitat models performed best with environmental covariates only. Such differences among studies are likely due to variation in the ways that habitats and prey are characterized, the foraging patterns of individual species, and the heterogeneity of the underlying community (Torres et al., 2008; Bennington et al., 2020b).
The relative abundance of dolphins had a strong positive relationship with the proportion of sandy substrate in their habitat (Figure 2). Mud and sand were the dominant habitat types in all survey areas and thus the positive association with sand is also a negative association with mud. At fine-scales, harbor (Phoca vitulina) and grey seal (Halichoerus grypus) were also less common where bottom types had a high proportion of muddy sediment (Bailey and Thompson, 2010). Preference for certain bottom types is probably related to the preference of certain prey for these habitats (Torres et al., 2008; Embling et al., 2012), though preferences of Hector’s dolphin prey for specific habitat characteristics are poorly known.
The habitat models suggest that reef habitat was not favored by Hector’s dolphins. In temperate areas, reef habitat and the macroalgae communities that it sustains, are important local sources of productivity, and provide heterogeneity that promotes high biodiversity (Smale et al., 2013; Rees et al., 2014). The strong negative relationship between Hector’s dolphins and reef shows that this species does not respond to these ecological values, at least on the scale of this study; the species rarely targets reef-associated prey (Miller et al., 2013). The abundance of dolphins showed a negative relationship with areas of high habitat heterogeneity (Figure 2), in contrast to the only other study that included heterogeneity as a factor influencing marine mammal distribution (Eierman and Connor, 2014). This finding suggests that Hector’s dolphins may not be a good indicator of habitats with high diversity of seafloor communities. Rather, they appear to favor specific habitat-types (e.g., high-current, sandy habitat) that may support high productivity and prey abundance.
The relative abundance of dolphins was positively correlated with higher tidal current velocity (Figure 2). Several studies have related tidal state to distribution or foraging in marine predators (Johnston et al., 2005; Bailey and Thompson, 2010; Lin et al., 2013; Nuuttila et al., 2017). Brough et al. (2020) found tidal state to influence the onset of foraging bouts in Hector’s dolphin. Harbor porpoise (Phocoena phocoena), an ecologically similar species to Hector’s dolphin, were also found to be more abundant at high tidal stream velocities (Marubini et al., 2009). Tidal currents are hypothesized to promote prey aggregation via the creation of hydrological features such as wakes and eddies (Johnston et al., 2005) and/or enhance catchability of prey (Bailey & Thompson, 2010).
Oceanographic covariates including surface temperature, temperature gradient and turbidity all had some influence on the relative abundance of dolphins. Of these, the influence of temperature was the most pronounced; dolphins were substantially less abundant at temperatures cooler than 12°C (i.e., winter temperatures). Thus, the influence of temperature likely describes the seasonal preference for this near-shore component of the dolphins wider habitat (Rayment et al., 2010). However, the indication of an ‘upper-limit’ for the correlation between high temperatures and higher abundance (Figure 2) should be considered in the context of warming sea conditions in this area (Shears and Bowen, 2017). The GAMs suggest the dolphins are more abundant in less turbid conditions (Figure 2). While it is generally accepted that on a broad scale, Hector’s and Maui (C. hectori maui) dolphins show a preference for nearshore habitat (that is incidentally more turbid) (Bräger et al., 2003; Derville et al., 2016), our study suggests that at fine scales the species selects locations that are less turbid – a result that raises questions about the transferability of modelled relationships between scales of analysis. Turbidity may play a role in the detection of prey, threats or conspecifics (Karczmarski et al., 2000; Heithaus and Dill, 2006). However, turbidity may also act as a proxy for correlated variables including primary productivity and swell exposure (Cloern, 1987; Dittmann et al., 2016).
4.2 Characteristics of hotspots
Several variables deemed important by the SDMs were also more common at hotspots, including habitat with a high proportion of sand, high prey abundance and current velocity (Figure 3). While not statistically significant in the GAMs, the preferred depth range was more frequent at hotspots compared to reference areas (Figure 3). In contrast, preferred values of reef habitat, temperature and temperature gradient, turbidity and heterogeneity were not more common at hotspots (Figure 3). SST, SST gradient and turbidity may be correlated with the dolphins’ broader seasonal distribution in this nearshore habitat, rather than hotspots being locations with particularly high or strong gradients in temperature, or low turbidity. Hector’s dolphins are more common inshore during summer, when waters are warmer and have lower turbidity (Bräger et al., 2003; Rayment et al., 2009), However, turbidity does distinguish a large cluster of observations from reference areas in PCA space (Figure 4) that are likely related to reference areas on the northern coast of Banks Peninsula (LY, ME) and inner harbor locations (WA) that are more turbid. While independently unrelated to hotspots (Figure 3), the combination of reef cover and environmental heterogeneity along with depth, does seem to contribute to areas that are not hotspots; as seen by a discrete cluster in the PCA analysis (Figure 4). It is likely these characteristics combine to form a habitat ‘type’ that is not routinely used by the dolphins and confirms the importance of multi-variate analyses when appraising habitat selection.
The discrepancy between the frequency of occurrence of positive GAMvelopes among hotspots/reference areas and the outputs of the models are likely attributable to the reference area ‘LB’. While this area was not designated a ‘hotspot’ by Brough et al. (2019a), our surveys indicated the area had high dolphin abundance, that was not significantly different from the abundance encountered at hotspots (Table S1). The environmental signature of this location was also more similar to hotspots than reference areas, particularly with regards to prey abundance (Figure 4). It may be that habitat at LB has improved in quality over time. A dataset spanning 30 years was used to initially designate hotspot areas, and thus any very recent preference for this area would be obscured in the analysis by Brough et al., 2019a.
The PCA analysis suggests that not all hotspots and reference areas have the same characteristics. Observations at some reference areas were defined by reef cover, heterogeneity and depth, and others by turbidity (Figure 4). The large spread of the observations from hotspots suggests that these important areas do not have a well-defined ‘recipe’ that describes their constituent characteristics, rejecting our initial hypothesis for a unique environmental signature of these important areas. However, there are some clear contributors to good quality habitat for this species, with high sand cover, prey abundance and tidal velocity clearly separating observations among hotspots and reference areas (Figure 4). While foraging behavior is more common at these hotspots (Brough et al., 2020), it is possible that different prey with different habitat requirements are targeted in different locations. Additionally, other behaviors known to influence habitat selection (e.g., nursing young) may contribute unique habitat preference signals to this appraisal (Weir et al., 2008; Webster et al., 2009). In any case, the complexity in distinguishing a unique environmental signal for hotspots confirms the importance of targeted investigations into hotspot characteristics when habitat-based management is required.
4.3 Ecologically important habitat and threats
There is good reason to believe that the contributors to hotspot occurrence for Hector’s dolphins may also reflect habitat of high ecological value more generally. The characteristics of seafloor sediment are known to have a significant influence on benthic communities (Thrush et al., 2004). Mud content has been shown to impact benthic invertebrate abundance (Thrush et al., 2003), diversity (Dauer et al., 2000; Ellingsen, 2002) and ecosystem structure and function (Thrush et al., 2003; Jones et al., 2011; Pratt et al., 2013). At Banks Peninsula, mud content has a strong influence on benthic invertebrate fauna, with sand dominated habitat having higher richness and biomass (Fenwick, 2004). The avoidance of areas with high mud content by this apex predator may be due to the impacts of sedimentation of seafloor communities (Thrush et al., 2004) altering the availability of Hector’s dolphin prey (e.g., through benthic foraging fish – red cod, Pseudophycis bachus). If this hypothesis holds true, Hector’s dolphins may be useful indicators of seafloor community health, however further sampling at lower trophic levels is required to confirm such a claim. Sedimentation is a known threat to this nearshore habitat, with some areas experiencing up to 1 m of deposition of fine, muddy sediment over the last 70 years (Hart et al., 2009). If sedimentation is adversely impacting habitat of high ecological value that support Hector’s dolphins, management should target a reduction in this stressor.
High and dynamic tidal flow can promote localized productivity increases due to enhanced mixing and the creation of features such eddies, wakes and fronts, that in turn influence the abundance of mid-trophic species and their catchability to predators (Johnston et al., 2005; Bailey and Thompson, 2010; Embling et al., 2012; Pirotta et al., 2013). Thus, high tidal flow may be a key component of ecologically important habitat in this area. The flow of tidal currents can be impacted by coastal development such as land reclamation and wharf construction (Jefferson et al., 2009), aquaculture (Grant and Bacher, 2001) and dredging (van Maren et al., 2015). These stressors occur at Banks Peninsula and are prevalent throughout coastal New Zealand. Resource management should consider the importance of tidal current velocities for Hector’s dolphins and for contributing to habitat of high ecological value, especially due to the possible increases in coastal development over coming decades.
Mid-trophic level, epipelagic fishes perform a critical role in ecosystems by connecting primary consumers with top predators in so-called wasp-waist ecosystem structuring (Cury et al., 2000). Variability in the abundance of these taxa has been related to distinct changes in ecosystem function and health (Cury et al., 2000; Griffiths et al., 2013). Thus, locations that are routinely used by epipelagic fish, such as the hotspots for Hector’s dolphins investigated in this study, may be considered ecologically important habitat due to their perceived role in supporting high biomass of these taxa. Threats to mid-trophic level fishes are well known and include overfishing, habitat modification, disease, and climate change (Ward, 2001; Bertrand et al., 2004; Roux et al., 2013). There is limited commercial harvest of the small epipelagic species in New Zealand, however changes in oceanographic conditions, via terrestrial impacts (e.g., suspended sediment) or climate change are a distinct possibility.
Increased turbidity from suspended sediments derived from terrestrial runoff is a common feature of coastal habitat internationally (Galy et al., 2015; Halpern et al., 2015). High turbidity can alter rates of primary productivity in phytoplankton (Cloern, 1987; May et al., 2003) and macroalgae (Desmond et al., 2015; Blain et al., 2021), and can influence the health, abundance and survival of juvenile fish (Wilber and Clarke, 2001; Lowe et al., 2015). Thus, similar to the previous environmental variables, the effects of turbidity on marine mammals are likely indirect via prey, though further analysis is required to confirm relationships between prey abundance and variable turbidity.
5 Conclusions
We have confirmed that the availability of prey and sandy, shallow, high current and low turbidity habitat types contribute to the occurrence of hotspots in distribution of Hector’s dolphin. The identification of the key attributes of hotspots for this endangered dolphin provides management with the first opportunities to limit habitat-related impacts on this species by targeted interventions against stressors. These interventions may include the limitation/prevention of human activities that cause degradation to key hotspot characteristics (e.g., overfishing, sedimentation, change in tidal currents). The characteristics of hotspots for Hector’s dolphin are likely indicative of habitat of high ecological value across a range of significance criteria (e.g., Dunstan et al., 2016). Thus, protection of these characteristics would provide benefits for this endangered, endemic species and the ecosystem upon which it depends in true, ecosystem-based management.
Data availability statement
The datasets presented in this study can be found in online repositories. The names of the repository/repositories and accession number(s) can be found in the article/Supplementary Material.
Ethics statement
The animal study was reviewed and approved by University of Otago animal ethic committee.
Author contributions
TB, WR and SD contributed equally to conceptualization of this study. TB led fieldwork, analysis, and draft preparation with contributions from WR, LS and SD. All authors provided critical review on the draft manuscript. All authors contributed to the article and approved the submitted version.
Funding
Funding for this study was generously provided by the NZ Whale and Dolphin Trust, Wools NZ and Camira Fabrics, the NZ Department of Conservation, BlackCat and Akaroa Dolphins. TB was the grateful recipient of University of Otago doctoral scholarship.
Acknowledgments
We would like to thank members of the Marine Mammal Research Group of the University of Otago for assistance in the field; particularly Jesu Valdes, Lindsay Wickman and Eva Leunissen. Sincere thanks to staff of the NZ Department of Conservation at Banks Peninsula, especially Tom MacTavish, Black Cat tours Ltd and staff of the Department of Marine Science at the University of Otago. We also acknowledge funding to support revisions of prior drafts of the manuscript and publication costs from the NIWA Coasts and Estuaries Programme, Strategic Science Investment Fund (New Zealand Ministry for Business, Innovation and Employment), Project #CEME2303 “Scenarios, models and frameworks for ecosystem management”. We thank Carolyn Lundquist and XX reviewers for comments that improved the manuscript.
Conflict of interest
The authors declare that the research was conducted in the absence of any commercial or financial relationships that could be construed as a potential conflict of interest.
Publisher’s note
All claims expressed in this article are solely those of the authors and do not necessarily represent those of their affiliated organizations, or those of the publisher, the editors and the reviewers. Any product that may be evaluated in this article, or claim that may be made by its manufacturer, is not guaranteed or endorsed by the publisher.
Supplementary material
The Supplementary Material for this article can be found online at: https://www.frontiersin.org/articles/10.3389/fmars.2023.1204943/full#supplementary-material
References
Azzellino A., Fossi M. C., Gaspari S., Lanfredi C., Lauriano G., Marsili L., et al. (2014). An index based on the biodiversity of cetacean species to assess the environmental status of marine ecosystems. Mar. Environ. Res. 100, 94–111. doi: 10.1016/j.marenvres.2014.06.003
Bailey H., Thompson P. (2010). Effect of oceanographic features on fine-scale foraging movements of bottlenose dolphins. Mar. Ecol. Prog. Ser. 418, 223–233. doi: 10.3354/meps08789
Barlow D. R., Bernard K. S., Escobar-Flores P., Palacios D. M., Torres L. G. (2020). Links in the trophic chain: modeling functional relationships between in situ oceanography, krill, and blue whale distribution under different oceanographic regimes. Mar. Ecol. Prog. Ser. 642, 207–225. doi: 10.3354/meps13339
Baumgartner M. F., Cole T. V. N., Campbell R. G., Teegarden G. J., Durbin E. G. (2003). Associations between North Atlantic right whales and their prey, Calanus finmarchicus, over diel and tidal time scales. Mar. Ecol. Prog. Ser. 264, 155–166. doi: 10.3354/meps264155
Bennington S., Rayment W., Currey R., Oldridge L., Henderson S., Guerra M., et al. (2020a). Long-term stability in core habitat of an endangered population of bottlenose dolphins (Tursiops truncatus): Implications for spatial management. Aquat. Conserv.: Mar. Freshwat. Ecosyst. 31, 665–676. doi: 10.1002/aqc.3460
Bennington S., Rayment W., Dawson S. (2020b). ). Putting prey into the picture: improvements to species distribution models for bottlenose dolphins in Doubtful Sound, New Zealand. Mar. Ecol. Prog. Ser. 653, 191–204. doi: 10.3354/meps13492
Benoit-Bird K. J., Battaile B. C., Heppell S. A., Hoover B., Irons D., Jones N., et al. (2013). Prey patch patterns predict habitat use by top marine predators with diverse foraging strategies. PloS One 8, e53348. doi: 10.1371/journal.pone.0053348
Bertrand A., Segura M., Gutiérrez M., Vásquez L. (2004). From small-scale habitat loopholes to decadal cycles: a habitat-based hypothesis explaining fluctuation in pelagic fish populations off Peru. Fish. 5, 296–316. doi: 10.1111/j.1467-2679.2004.00165.x
Bifolchi A., Lodé T. (2005). Efficiency of conservation shortcuts: an investigation with otters as umbrella species. Biol. Conserv. 126, 523–527. doi: 10.1016/j.biocon.2005.07.002
Blain C. O., Hansen S. C., Shears N. T. (2021). Coastal darkening substantially limits the contribution of kelp to coastal carbon cycles. Glob. Change Biol. 27, 5547–5563. doi: 10.1111/gcb.15837
Bouchet P. J., Meeuwig J. J., Salgado Kent C. P., Letessier T. B., Jenner C. K. (2015). Topographic determinants of mobile vertebrate predator hotspots: Current knowledge and future directions. Biol. Rev. 90, 699–728. doi: 10.1111/brv.12130
Bräger S., Harraway J. A., Manly B. F. J. (2003). Habitat selection in a coastal dolphin species (Cephalorhynchus hectori). Mar. Biol. 143, 233–244. doi: 10.1007/s00227-003-1068-x
Brough T. E., MacTavish T., Bolton-Ritchie L. (2018). Mapping physical habitat types and bathymetry on the south coast of Banks Peninsula. Department of Conservation Research Report (Christchurch, NZ: Mahaanui District Office). Available at: https://api.ecan.govt.nz/TrimPublicAPI/documents/download/3544463.
Brough T., Rayment W., Dawson S. (2019b). ). Using a recreational grade echosounder to quantify the potential prey field of coastal predators. . PloS One 14, e0217013. doi: 10.1371/journal.pone.0217013
Brough T., Rayment W., Slooten E., Dawson S. (2019a). Fine scale distribution for a population of New Zealand’s only endemic dolphin (Cephalorhynchus hectori) shows long-term stability of coastal hotspots. Mar. Mamm. Sci. 35, 140–163. doi: 10.1111/mms.12528
Brough T., Rayment W., Slooten E., Dawson S. (2020). Spatiotemporal distribution of foraging in a marine predator: behavioural drivers of hotspot formation. Mar. Ecol. Prog. Ser. 635, 187–202. doi: 10.3354/meps13198
Brough T., Slooten E., Dawson S. (2014). Marine mammals and the Port Lyttelton development: An environmental impact assessment. Technical report (Dunedin, NZ: Lyttelton Port of Christchurch. University of Otago).
Burnham K. P., Anderson D. R. (1998). Model selection and inference: A practical information-theoretic approach (USA: Springer).
Carome W., Slooten E., Rayment W. J., Webster T. A., Wickman L., Brough T. E., et al. (2022). A long-term shift in the summer distribution of Hector’s dolphins is correlated with an increase in cruise ship tourism. Aquat. Conserv.: Mar. Freshwat. Ecosyst. 32, 1660–1674. doi: 10.1002/aqc.3881
Cloern J. E. (1987). Turbidity as a control on phytoplankton biomass and productivity in estuaries. Cont. Shelf Res. 7, 1367–1381. doi: 10.1016/0278-4343(87)90042-2
Correia A. M., Tepsich P., Rosso M., Caldeira R., Sousa-Pinto I. (2015). Cetacean occurrence and spatial distribution: Habitat modelling for offshore waters in the Portuguese EEZ (NE Atlantic). J. Mar. Syst. 143, 73–85. doi: 10.1016/j.jmarsys.2014.10.016
Cury P., Bakun A., Crawford R. J. M., Jarre A., Quiñones R. A., Shannon L. J., et al. (2000). Small pelagics in upwelling systems: Patterns of interaction and structural changes in ‘wasp-waist’ ecosystems. ICES J. Mar. Sci. 57, 603–618. doi: 10.1006/jmsc.2000.0712
Dauer D. M., Ranasinghe J. A., Weisberg S. B. (2000). Relationships between benthic community condition, water quality, sediment quality, nutrient loads, and land use patterns in Chesapeake Bay. Estuaries 23, 80–96. doi: 10.2307/1353227
Dawson S. (1991). Incidental catch of Hector’s dolphin in inshore gillnets. Mar. Mamm. Sci. 7, 283–295. doi: 10.1111/j.1748-7692.1991.tb00103.x
Dawson S. M. (2017). “Cephalorhynchus Dolphins: C. heavisidii, C. eutropia, C. hectori and C. commersonii,” in Encylopedia of marine mammals. Eds. Würsig B., Theissen J. G. M., Kovacs K. (USA: Elsevier), 166–172.
Dawson S. M., E. Slooten E. (1988). Hector’s dolphin, Cephalorhyncus hectori: Distribution and abundance. Report of the International Whaling Commission. 9, 315–324. doi: 10.1002/aqc.3270030305
Dawson S. M., Slooten E. (1993). Conservation of Hector’s dolphins: The case and process which led to establishment of the Banks Peninsula Marine Mammal Sanctuary. Aquat. Conserv.: Mar. Freshwat. Ecosyst. 3, 207–221. doi: 10.1002/aqc.3270030305
Derville S., Constantine R., Baker C. S., Oremus M., Torres L. G. (2016). Environmental correlates of nearshore habitat distribution by the Critically Endangered Maui dolphin. Mar. Ecol. Prog. Ser. 551, 261–275. doi: 10.3354/meps11736
Desmond M. J., Pritchard D. W., Hepburn C. D. (2015). Light limitation within southern new zealand kelp forest communities. PloS One 10, e0123676. doi: 10.1371/journal.pone.0123676
De Wysiecki A. M., Sánchez-Carnero N., Irigoyen A. J., Milessi A. C., Colonello J. H., Bovcon N. D., et al. (2020). Using temporally explicit habitat suitability models to infer the migratory pattern of a large mobile shark. Can. J. Fish. Aquat. Sci. 77, 1529–1539. doi: 10.1139/cjfas-2020-0036
Dittmann S., Dawson S., Rayment W., Webster T., Slooten E. (2016). Hector’s dolphin movement patterns in response to height and direction of ocean swellN. Z. J. Mar. Freshwat. Res. 50, 228–239doi: 10.1080/00288330.2015.1119165
Dohner M. (2013). Proposal for a new zealand marine habitat classification scheme. Prepared for the department of conservation (New Zealand).
Doniol-Valcroze T., Lesage V., Giard J., Michaud R. (2012). Challenges in marine mammal habitat modelling: Evidence of multiple foraging habitats from the identification of feeding events in blue whales. Endanger. Species Res. 17, 255–268. doi: 10.3354/esr00427
Dunstan P. K., Bax N. J., Dambacher J. M., Hayes K. R., Hedge P. T., Smith D. C., et al. (2016). Using ecologically or biologically significant marine areas (EBSAs) to implement marine spatial planning. Ocean Coast. Manage. 121, 116–127. doi: 10.1016/j.ocecoaman.2015.11.021
Eierman L. E., Connor R. C. (2014). Foraging behavior, prey distribution, and microhabitat use by bottlenose dolphins Tursiops truncatus in a tropical atoll. Mar. Ecol. Prog. Ser. 503, 279–288. doi: 10.3354/meps10721
Ellingsen K. E. (2002). Soft-sediment benthic biodiversity on the continental shelf in relation to environmental variability. Mar. Ecol. Prog. Ser. 232, 15–27. doi: 10.3354/meps232015
Embling C. B., Illian J., Armstrong E., Kooij J. V. D., Sharples J., Camphuysen K. C. J., et al. (2012). Investigating fine-scale spatio-temporal predator–prey patterns in dynamic marine ecosystems: a functional data analysis approach. J. Appl. Ecol. 49, 481–492. doi: 10.1111/j.1365-2664.2012.02114.x
Fauchald P. (2009). Spatial interaction between seabirds and prey: review and synthesis. Mar. Ecol. Prog. Ser. 391, 139–152. doi: 10.3354/meps07818
Fenwick G. D. (2004). Marine ecology of Akaroa Harbour: rocky shores and subtidal soft bottoms (Christchurch, New Zealand: NIWA client report prepared for Environment Canterbury).
Galy V., Peucker-Ehrenbrink B., Eglinton T. (2015). Global carbon export from the terrestrial biosphere controlled by erosion. Nature. 521, 204–207. doi: 10.1038/nature14400
Gende S. M., Sigler M. F. (2006). Persistence of forage fish ‘hot spots’ and its association with foraging Steller sea lions (Eumetopias jubatus) in southeast Alaska. Deep Sea Res. Part II. 53, 432–441. doi: 10.1016/j.dsr2.2006.01.005
Gerrodette T., Perryman W. L., Oedekoven C. S. (2019). Accuracy and precision of dolphin group size estimates. Mar. Mamm. Sci. 35, 22–39. doi: 10.1111/mms.12506
Gilles A., Viquerat S., Becker E. A., Forney K. A., Geelhoed S. C. V., Haelters J., et al. (2016). Seasonal habitat-based density models for a marine top predator, the harbor porpoise, in a dynamic environment. Ecosphere 7, e01367.
Gomez J. J., Cassini M. H. (2015). Environmental predictors of habitat suitability and biogeographical range of Franciscana dolphins (Pontoporia blainvillei). Global Ecol. Conser. 3, 90–99. doi: 10.1016/j.gecco.2014.11.007
Grant J., Bacher C. (2001). A numerical model of flow modification induced by suspended aquaculture in a Chinese bay. Can. J. Fish. Aquat. Sci. 58, 1003–1011. doi: 10.1139/cjfas-58-5-1003
Griffiths S. P., Olson R. J., Watters G. M. (2013). Complex wasp-waist regulation of pelagic ecosystems in the Pacific Ocean. Rev. Fish Biol. Fish. 23, 459–475. doi: 10.1007/s11160-012-9301-7
Grüss A., Yemane D., Fairweather T. (2016). Exploring the spatial distribution patterns of South African Cape hakes using generalized additive models. Afr. J. Mar. Sci. 38, 395–409. doi: 10.2989/1814232X.2016.1218367
Guisan A., Zimmermann N. E. (2000). Predictive habitat distribution models in ecology. Ecol. Eng. 135, 147–186. doi: 10.1016/S0304-3800(00)00354-9
Halpern B. S., Frazier M., Potapenko J., Casey K. S., Koenig K., Longo C., et al. (2015). Spatial and temporal changes in cumulative human impacts on the world’s ocean. Nat. Commun. 6, 7615. doi: 10.1038/ncomms8615
Harding K. C., Fujiwara M., Axberg Y., Härkönen T. (2005). Mass-dependant energetics and survival in harbor seal pups. Funct. Ecol. 19, 129–135. doi: 10.1111/j.0269-8463.2005.00945.x
Hart D. E., Todd D. J., Nation T. E., McWilliams Z. A. (2009). Upper Akaroa Harbour seabed bathymetry and soft sediments: A baseline mapping study (Christchurch, New Zealand: Environment Canterbury. Coastal Research Report 1. DTec Consulting Ltd). Available at: https://api.ecan.govt.nz/TrimPublicAPI/documents/download/1198242.
Hastie T., Tibshirani R. (1987). Generalized additive models: some applications. J. Am. Stat. Assoc. 82, 371–386. doi: 10.1080/01621459.1987.10478440
Hastie G. D., Wilson B., Wilson L. J., Parsons K. M., Thompson P. M. (2004). Functional mechanisms underlying cetacean distribution patterns: hotspots for bottlenose dolphins are linked to foraging. Mar. Biol. 144, 397–403. doi: 10.1007/s00227-003-1195-4
Hazen E. L., Jorgensen S., Rykaczewski R. R., Bograd S. J., Foley D. G., Jonsen I. D., et al. (2012). Predicted habitat shifts of Pacific top predators in a changing climate. Nat. Clim. Change. 3, 234–238. doi: 10.1038/nclimate1686
Hazen E. L., Nowacek D. P., Laurent L. S., Halpin P. N., Moretti D. J. (2011). The Relationship among oceanography, prey fields, and beaked whale foraging habitat in the Tongue of the Ocean. PloS One 6, e19269. doi: 10.1371/journal.pone.0019269
He S., Mazumdar S., Arena V. C. (2006). A comparative study of the use of GAM and GLM in air pollution research. Environmetrics 17, 81–93. doi: 10.1002/env.751
Heithaus M. R., Dill L. M. (2006). Does tiger shark predation risk influence foraging habitat use by bottlenose dolphins at multiple spatial scales? Oikos 114, 257–264. doi: 10.1111/j.2006.0030-1299.14443.x
Hijmans R. J. (2019) Raster: Geographic Data Analysis and Modeling. R package version 3.0-7. Available at: https://CRAN.R-project.org/package=raster.
Hooker S. K., Cañadas A., Hyrenbach K. D., Corrigan C., Polovina J. J., Reeves R. R. (2011). Making protected area networks effective for marine top predators. Endanger. Species Res. 13, 203–218. doi: 10.3354/esr00322
Hooker S. K., Gerber L. R. (2004). Marine reserves as a tool for ecosystem-based management: The potential importance of megafauna. Bioscience 54, 27–39. doi: 10.1641/0006-3568(2004)054[0027:MRAATF]2.0.CO;2
Hooker S. K., Whitehead H., Gowans S. (2002). Ecosystem consideration in conservation planning: Energy demand of foraging bottlenose whales (Hyperoodon ampullatus) in a marine protected area. Biol. Conserv. 104, 51–58. doi: 10.1016/S0006-3207(01)00153-7
Hothorn T., Bretz F., Westfall P. (2008). Simultaneous inference in general parametric models. Biom. J. 50, 346–363. doi: 10.1002/bimj.200810425
Hurvich C. M. (1989). Regression and time series model selection in small samples. Biometrika 76, 297–307. doi: 10.1093/biomet/76.2.297
Jefferson T. A., Hung S. K., Würsig B. (2009). Protecting small cetaceans from coastal development: Impact assessment and mitigation experience in Hong Kong. Mar. Policy 33, 305–311. doi: 10.1016/j.marpol.2008.07.011
Johnston D. W., Westgate A. J., Read A. J. (2005). Effects of fine-scale oceanographic features on the distribution and movements of harbor porpoises Phocoena phocoena in the Bay of Fundy. Mar. Ecol. Prog. Ser. 295, 279–293. doi: 10.3354/meps295279
Jolliffe I. T. (2002). Principal component analysis for special types of data (New York, USA: Springer New York).
Jones H. F., Pilditch C. A., Bruesewitz D. A., Lohrer A. M. (2011). Sedimentary environment influences the effect of an infaunal suspension feeding bivalve on estuarine ecosystem function. PloS One 6, e27065. doi: 10.1371/journal.pone.0027065
Kalinkat G., Cabral J. S., Darwall W., Ficetola G. F., Fisher J. L., Giling D. P., et al. (2017). Flagship umbrella species needed for the conservation of overlooked aquatic biodiversity. Conserv. Biol. 31, 481–485. doi: 10.1111/cobi.12813
Karczmarski L., Cockcroft V. G., Mclachlan A. (2000). Habitat use and preferences of Indo-Pacific humpback dolphins Sousa chinensis in Algoa Bay, South Africa. Mar. Mamm. Sci. 16, 65–79. doi: 10.1111/j.1748-7692.2000.tb00904.x
Leunissen E. M., Dawson S. M. (2018). Underwater noise levels of pile-driving in a New Zealand harbour, and the potential impacts on endangered Hector’s dolphins. Mar. pollut. Bull. 135, 195–204. doi: 10.1016/j.marpolbul.2018.07.024
Lin T.-H., Akamatsu T., Lien-Siang C. (2013). Tidal influences on the habitat use of Indo-Pacific humpback dolphins in an estuary. Mar. Biol. 160, 1353–1363. doi: 10.1007/s00227-013-2187-7
Lowe M. L., Morrison M. A., Taylor R. B. (2015). Harmful effects of sediment-induced turbidity on juvenile fish in estuaries. Mar. Ecol. Prog. Ser. 539, 241–254. doi: 10.3354/meps11496
Macleod K., Fairbairns R., Gill A., Fairbairns B., Gordon J., Blair-myers C., et al. (2004). Seasonal distribution of minke whales Balaenoptera acutorostrata in relation to physiography and prey off the Isle of Mull, Scotland. Mar. Ecol. Prog. Ser. 277, 263–274. doi: 10.3354/meps277263
Marsh H., Eros C., Corkeron P. J., Breen B. (1999). A conservation strategy for dugongs: implications of Australian research. Mar. Freshw. Res. 50, 979–990. doi: 10.1071/MF99080
Marubini F., Gimona A., Evans P. G. H., Wright P. J., Pierce G. J. (2009). Habitat preferences and interannual variability in occurrence of the harbor porpoise Phocoena phocoena off northwest Scotland. Mar. Ecol. Prog. Ser. 381, 297–310. doi: 10.3354/meps07893
May C. L., Koseff J. R., Lucas L. V., Cloern J. E., Schoellhamer D. H. (2003). Effects of spatial and temporal variability of turbidity on phytoplankton blooms. Mar. Ecol. Prog. Ser. 254, 111–128. doi: 10.3354/meps254111
Miller E., Lalas C., Dawson S., Ratz H., Slooten E. (2013). Hector’s dolphin diet: The species, sizes and relative importance of prey eaten by Cephalorhynchus hectori, investigated using stomach content analysis. Mar. Mamm. Sci. 29, 606–628. doi: 10.1111/j.1748-7692.2012.00594.x
NASA Goddard Space Flight Center, Ocean Ecology Laboratory, Ocean Biology Processing Group. (2021). Moderate-resolution imaging spectroradiometer (MODIS) aqua CHLa/KD490 data; NASA OB.DAAC (USA: Greenbelt, MD).
Nuuttila H. K., Courtene W., Sarah J., Simon M., Evans P. G. H. (2017). Don’t forget the porpoise: acoustic monitoring reveals fine scale temporal variation between bottlenose dolphin and harbor porpoise in Cardigan Bay SAC. Mar. Biol. 164, 1–16. doi: 10.1007/s00227-017-3081-5
Pirotta E., Thompson P. M., Miller P. I., Brookes K. L., Cheney B., Barton T. R., et al. (2013). Scale-dependent foraging ecology of a marine top predator modelled using passive acoustic data. Funct. Ecol. 28, 206–217. doi: 10.1111/1365-2435.12146
Pitcher C., Ellis N., Smith S. (2011) Example Analysis of Biodiversity Survey Data With R Package GradientForest. R vignette. Available at: http://gradientforest.r-forge.r-project.org/biodiversity-survey.pdf.
Pratt D. R., Lohrer A. M., Pilditch C. A., Thrush S. F. (2013). Changes in ecosystem function across sedimentary gradients in estuaries. Ecosystems 17, 182–194. doi: 10.1007/s10021-013-9716-6
Rayment W., Dawson S., Slooten E. (2010). Seasonal changes in distribution of Hector’s dolphin at Banks Peninsula, New Zealand: implications for protected area design. Aquat. Conserv.: Mar. Freshwat. Ecosyst. 20, 106–116. doi: 10.1002/aqc
Rayment W., Dawson S., Slooten E., Bräger S., du Fresne S., Webster T. (2009). Kernel density estimates of alongshore home range of Hector’s dolphins at Banks Peninsula, New Zealand. Mar. Mamm. Sci. 25, 537–556. doi: 10.1111/j.1748-7692.2008.00271.x
Rayment W., Dawson S., Webster T. (2015). Breeding status affects fine-scale habitat selection of southern right whales on their wintering grounds. J. Biogeogr. 42, 463–474. doi: 10.1111/jbi.12443
R-Core-Team (2018). “R: A language and environment for statistical computing,” in R foundation for statistical computing (Vienna, Austria). Available at: https://www.R-project.org/.
Rees M. J., Jordan A., Price O. F., Coleman M. A., Davis A. R. (2014). Abiotic surrogates for temperate rocky reef biodiversity: Implications for marine protected areas. Divers. Distrib. 20, 284–296. doi: 10.1111/ddi.12134
Roux J.-P., van der Lingen C. D., Gibbons M. J., Moroff N., Shannon L. J., Smith A. D., et al. (2013). Jellyfication of marine ecosystems as a consequence of overfishing small pelagic fish: lessons from the benguela. Bull. Mar. Sci. 89, 249–284. doi: 10.5343/bms.2011.1145
Saijo D., Mitani Y., Abe T., Sasaki H., Goetsch C., Costa D. P., et al. (2017). Linking mesopelagic prey abundance and distribution to the foraging behavior of a deep-diving predator, the northern elephant seal. Deep Sea Res. Part II. 140, 163–170. doi: 10.1016/j.dsr2.2016.11.007
Sanciangco J. C., Carpenter K. E., Etnoyer P. J., Moretzsohn F. (2013). Habitat availability and heterogeneity and the indo-pacific warm pool as predictors of marine species richness in the tropical Indo-Pacific. PloS One 8, e56245. doi: 10.1371/journal.pone.0056245
Scott B. E., Sharples J., Ross O. N., Wang J., Pierce G. J., Camphuysen C. J. (2010). Sub-surface hotspots in shallow seas: fine-scale limited locations of top predator foraging habitat indicated by tidal mixing and sub-surface chlorophyll. Mar. Ecol. Prog. Ser. 408, 207–226. doi: 10.3354/meps08552
Shannon C., Weaver W. (1963). The mathematical theory of communication (Urbana: University of Illinois Press).
Shears N. T., Bowen M. M. (2017). Half a century of coastal temperature records reveal complex warming trends in western boundary currents. Sci. Rep. 7, 1–9. doi: 10.1038/s41598-017-14944-2
Slooten E., Dawson S. M. (2008). Sustainable levels of human impact for Hector’s Dolphin. Open Conserv. Biol. J. 2 (1), 37–43. doi: 10.2174/1874839200802010037
Slooten E., Fletcher D., Taylor B. L. (2000). Accounting for uncertainty in risk assessment: Case study with Hector’s dolphin mortality due to gillnet entanglement. Conserv. Biol. 14, 1264–1270. doi: 10.1046/j.1523-1739.2000.00099-411.x
Smale D. A., Burrows M. T., Moore P., O’Connor N., Hawkins S. J. (2013). Threats and knowledge gaps for ecosystem services provided by kelp forests: A northeast Atlantic perspective. Ecol. Evol. 3, 4016–4038. doi: 10.1002/ece3.774
Soutelino R. G., Beamsley B. (2015) The influence of the Southland Current on circulation patterns within Pegasus Bay, in: Proceedings of Coasts & Ports Conference. September. Available at: https://search.informit.com.au/documentSummary.
Stephenson F., Leathwick J. R., Geange S. W., Bulmer R. H., Hewitt J. E., Anderson O. F., et al. (2018). Using Gradient Forests to summarize patterns in species turnover across large spatial scales and inform conservation planning. Divers. Distrib. 24, 1641–1656. doi: 10.1111/ddi.12787
Stockin K. A., Law R. J., Roe W. D., Meynier L., Martinez E., Duignan P. J., et al. (2010). PCBs and organochlorine pesticides in Hector’s (Cephalorhynchus hectori hectori) and Maui’s (Cephalorhynchus hectori maui) dolphins. Mar. pollut. Bull. 60, 834–842. doi: 10.1016/j.marpolbul.2010.01.009
Stone G. S., Yoshinaga A. (2000). Hector’s Dolphin Cephalorhynchus hectori calf mortalities may indicate new risks from boat traffic and habituation. Pacific Conserv. Biol. 6, 162–170. doi: 10.1071/PC000162
Tepsich P., Rosso M., Halpin P. N., Moulins A. (2014). Habitat preferences of two deep-diving cetacean species in the northern Ligurian Sea. Mar. Ecol. Prog. Ser. 508, 247–260. doi: 10.3354/meps10851
Thrush S. F., Hewitt J. E., Cummings V. J., Ellis J. I., Hatton C., Lohrer A., et al. (2004). Muddy waters: elevating sediment input to coastal and estuarine habitats. Front. Ecol. Environ. 2, 299–306. doi: 10.1890/1540-9295(2004)002[0299:MWESIT]2.0.CO;2
Thrush S. F., Hewitt J. E., Norkko A., Nicholls P. E., Funnell G. A., Ellis J. I. (2003). Habitat change in estuaries: predicting broad-scale responses of intertidal macrofauna to sediment mud content. Mar. Ecol. Prog. Ser. 263, 101–112. doi: 10.3354/meps263101
Torres L. T., Read A. J., Haplin P. (2008). Fine-scale habitat modeling of a marine top predator: Do prey data improve predictive capacity? Ecol. Appl. 18, 1702–1717. doi: 10.1890/07-1455.1
van Maren D. S., van Kessel T., Cronin K., Sittoni L. (2015). The impact of channel deepening and dredging on estuarine sediment concentration. Cont. Shelf Res. 95, 1–14. doi: 10.1016/j.csr.2014.12.010
Waldock C., Stuart-Smith R. D., Albouy C., Cheung W. W., Edgar G. J., Mouillot D., et al. (2022). A quantitative review of abundance-based species distribution models. Ecography 2022 (1), e05694. doi: 10.1111/ecog.05694
Ward T. (2001). Effects of the 1995 and 1998 mass mortality events on the spawning biomass of sardine, Sardinops sagax, in South Australian waters. ICES J. Mar. Sci. 58 (4), 865–875. doi: 10.1006/jmsc.2001.1077
Webster T. A., Dawson S. M., Slooten E. (2009). Evidence of sex segregation in Hector’s dolphin (Cephalorhynchus hectori). Aquat. Mamm. 35, 212–219. doi: 10.1578/AM.35.2.2009.212
Weir J. S., Duprey N., Würsig B. (2008). Dusky dolphin (Lagenorhynchus obscurus) subgroup distribution: are shallow waters a refuge for nursery groups? Can. J. Zool. 86, 1225–1234. doi: 10.1139/Z08-101
Wilber D. H., Clarke D. G. (2001). Biological effects of suspended sediments: a review of suspended sediment impacts on fish and shellfish with relation to dredging activities in estuaries. N. Am. J. Fish. Manage. 21 (4), 855–875. doi: 10.1577/1548-8675(2001)021<0855:BEOSSA>2.0.CO;2
Wisniewska D. M., Teilmann J., Lee A., Siebert U., Teglberg P., Shearer J. (2016). Ultra-high foraging rates of harbor porpoises make them vulnerable to anthropogenic disturbance. Curr. Biol. 26, 1441–1446. doi: 10.1016/j.cub.2016.03.069
Wood S. N. (2017) Package ‘mgcv’. R package. Available at: https://cran.r-project.org/web/packages/mgcv.
Yen P. P. W., Sydeman W. J., Bograd S. J., Hyrenbach K. D. (2006). Spring-time distributions of migratory marine birds in the southern California Current: Oceanic eddy associations and coastal habitat hotspots over 17 years. Deep Sea Res. Part II. 53, 399–418. doi: 10.1016/j.dsr2.2006.01.013
Keywords: species hotspots, species distribution model, Hector’s dolphin, marine mammal, habitat characteristics, prey, forage fish
Citation: Brough TE, Rayment WJ, Slooten L and Dawson S (2023) Prey and habitat characteristics contribute to hotspots of distribution for an endangered coastal dolphin. Front. Mar. Sci. 10:1204943. doi: 10.3389/fmars.2023.1204943
Received: 13 April 2023; Accepted: 11 July 2023;
Published: 28 July 2023.
Edited by:
Guillermo Luna-Jorquera, Universidad Católica del Norte, ChileReviewed by:
Cristobal Anguita, University of Chile, ChileChun-Chieh Wang, Guangxi Academy of Sciences, China
Copyright © 2023 Brough, Rayment, Slooten and Dawson. This is an open-access article distributed under the terms of the Creative Commons Attribution License (CC BY). The use, distribution or reproduction in other forums is permitted, provided the original author(s) and the copyright owner(s) are credited and that the original publication in this journal is cited, in accordance with accepted academic practice. No use, distribution or reproduction is permitted which does not comply with these terms.
*Correspondence: Tom E. Brough, VG9tLkJyb3VnaEBuaXdhLmNvLm56