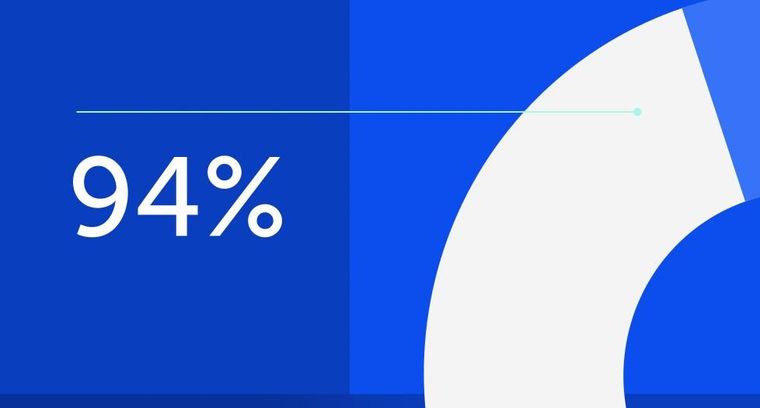
94% of researchers rate our articles as excellent or good
Learn more about the work of our research integrity team to safeguard the quality of each article we publish.
Find out more
ORIGINAL RESEARCH article
Front. Mar. Sci., 21 July 2023
Sec. Marine Ecosystem Ecology
Volume 10 - 2023 | https://doi.org/10.3389/fmars.2023.1181650
We used a one-dimensional vertical transport model, the sympagic-pelagic-benthic vertical transport model (SPBM) to explore the impact of sea-ice presence on phytoplankton phenology and biogeochemical dynamics. In the model, we introduced new parameter values for sympagic diatoms using ERSEM (European Regional Seas Ecosystem Model) in addition to the existing phytoplankton groups in the sea-ice model. We found that different groups of primary producers exhibit distinct spatial and temporal variabilities in both the sea-ice and water column depending on their physiological and biogeochemical properties. In particular, we discovered that the biomass of pelagic diatoms during the bloom season is strongly influenced by the release of sympagic algal cells during the early spring. This suggests the potential significance of sympagic algae seeding for the occurrence of pelagic diatom blooms in the Amundsen Sea. Notably, our model also indicates a potential connection between the earlier peak in particulate organic carbon flux and the release of sympagic-algae-associated particles from the sea ice, followed by their rapid sinking. Previous studies relying solely on observational data did not fully account for this mechanism. Our findings emphasize the importance of understanding the role of sympagic algae in the polar ecosystem and carbon cycle, and shed light on the complex biogeochemical dynamics associated with the sea-ice ecosystem in the polar seas.
Sea-ice, one of the largest biomes on Earth (Dieckmann and Hellmer, 2010), plays a significant role in the seasonal primary production of the polar oceans, particularly in the marginal sea-ice zone (Arrigo et al., 2010). Understanding the biology of sea-ice is essential due to the significant impact it has on these regions. Most of the regions covered by seasonal sea-ice are known to exhibit high productivity with intense, albeit brief, algal blooms (Thomas et al., 2012). Sympagic (sea-ice-associated) algae amounts to over 400 mg Chl-a m–2 and their primary productivity is above 1 g C m–2 day–1, which is comparable or even superior to other productive oceans (Arrigo et al., 1993; Arrigo et al., 1995). Arrigo et al. (2010) estimates that primary production in the sea-ice accounts for 5 – 10% of total production in the polar ocean. Recent studies have revealed the complex nature of the relationship between sympagic algae and the pelagic bloom, as the peak biomass of sympagic algae can occur at different times and with varying magnitudes across different hemispheres (Meiners et al., 2018; Lalande et al., 2020). Nonetheless, it is generally agreed that sympagic algae act as a seeding population for the pelagic bloom, reaching peak biomass before pelagic phytoplankton (Riaux-Gobin et al., 2011; Arrigo et al., 2014; Galindo et al., 2014). Furthermore, during sea-ice melt, sea-ice provides organic matters and nutrients to the pelagic community (Lannuzel et al., 2013). Sympagic algae also serve as a crucial food source for predators in winter (Bluhm et al., 2010), playing a crucial role in the reproduction and survival of the Antarctic krill (Brierley and Thomas, 2002; Smetacek and Nicol, 2005). In addition, sea-ice is involved in the production of dimethyl sulfide, the production or consumption of carbon dioxide (CO2), the accumulation of iron, and enhanced calcium carbonate precipitation (Rysgaard et al., 2007; Stefels et al., 2007; Tison et al., 2008; Lannuzel et al., 2010).
Studies have explored the physiological and biogeochemical characteristics of sympagic algae (Zhang et al., 1999; Mock and Kroon, 2002; Søgaard et al., 2011; Torstensson et al., 2015), highlighting their ability to adapt to variable physical environments through physiological and metabolic processes. Light availability is a primary factor controlling the growth of sympagic algae due to the strong attenuation of light penetrating through the sea-ice column (e.g., Kirst and Wiencke, 1995; Arrigo et al., 2010). The photophysiology of sympagic algae in the Amundsen Sea is strongly influenced by nutrient and light availability (Arrigo et al., 2014). The interaction between bacteria and sympagic algae is a critical component of the microbial loop, playing an important role in the recycling of organic matter in the sea-ice (Stewart and Fritsen, 2004; Martin et al., 2012; Cowie et al., 2014). Sympagic and pelagic biomass work together to transfer energy to both pelagic and benthic ecosystems through feeding, with any remaining biomass being sequestered into ocean sediments (Armand and Leventer, 2010).
Ducklow et al. (2015) proposed a possible connection between the ice algal bloom and the mass flux at deep depths in the water column. The authors observed that the mass fluxes collected by a sediment trap at 350 m deep of the Amundsen Sea polynya start to increase in late winter or early spring (September−November), which is two to three months earlier than the period when primary production in the pelagic surface layer reaches its maximum. This early peak in mass flux captured by the deep sediment trap has been attributed to the high primary production and grazing of sympagic algae in the sea-ice observed in this region (Fransson et al., 2011; Arrigo et al., 2014). Given that the depth-integrated Chl-a biomass in the sea-ice of the Amundsen Sea is comparable to that of the pelagic layers (Arrigo et al., 2014), it is plausible that ice algal production can significantly impact carbon export to the benthic layer and the biogeochemical dynamics in the upper ocean.
However, due to the challenging conditions of the polar oceans, field observations and sea-ice data are limited, making it difficult to quantitatively understand the role of sympagic communities in polar marine biogeochemical cycles and the interactions between sympagic and pelagic communities (Vancoppenolle and Tedesco, 2017). Therefore, modeling studies are essential for bridging these knowledge gaps in the biogeochemistry of polar oceans (Hunke et al., 2011; Meiners et al., 2012; Popova et al., 2012; Eilola et al., 2013; Vancoppenolle et al., 2013). While several biogeochemical models for the pelagic ecosystem have been developed and used to assess future changes in ecosystem dynamics under climate change scenarios (Arrigo et al., 2003; Wassmann et al., 2006; Ji et al., 2013), only a few large-scale studies (Deal et al., 2011; Sibert et al., 2011; Jin et al., 2012) have included sea-ice biogeochemical models. However, including sea-ice ecosystem processes in models can provide a more realistic simulation of the spring bloom (Tedesco et al., 2012) and the vertical flux of organic carbon as demonstrated by Ducklow et al. (2015). Therefore, developing and improving sea-ice biogeochemical models can contribute to a more comprehensive understanding of the polar ecosystem as a whole.
Motivated by the observed discrepancy in the timing of the spring bloom and the massive export of particulate matter in the Amundsen Sea reported by Ducklow et al. (2015), our study aims to investigate the impact of algal production in the sea-ice on the pelagic ecosystem using a simple sympagic model. Specifically, we sought to explore how sympagic algae may affect the intensity of primary production and organic carbon export in the pelagic system. In a previous study, Kwon et al. (2021) utilized a one-dimensional (1-D) ecosystem model, ERSEM, to identify the limiting factor governing primary production in the Amundsen Sea without considering the ecosystem in the sea-ice. By incorporating the sea-ice domain into our current study, we were able to elucidate the significant contribution of sea-ice to pelagic primary production and the pelagic ecosystem. Our findings underscore the importance of considering the role of sea-ice in biogeochemical models to achieve a more realistic representation of the polar ocean ecosystem.
To simulate primary production within the sea-ice, we utilized a vertically resolved 1-D transport ecosystem model developed by Yakubov et al. (2019) with modifications (see Section 3). This model, known as the sympagic-pelagic-benthic model (SPBM), integrates biogeochemical processes in the sea-ice, water column, and benthic domains, with a vertically resolved grid for each domain. SPBM requires inputs from hydrodynamic and ice models offline to describe the physical environments. The model employs the Framework for Aquatic Biogeochemical Models (FABM) (Bruggeman and Bolding, 2014) to provide biogeochemical source-minus-sink terms and vertical sinking velocities of particulate organic matter. SPBM does not include its own biogeochemical modules but can utilize modules from the FABM library or user-developed modules.
In our study, we incorporated two biogeochemical models, the European Regional Seas Ecosystem Model (ERSEM) (Butenschön et al., 2016) and the Bottom RedOx Model (BROM) (Yakushev et al., 2017) into SPBM. ERSEM represents key processes in the pelagic water column and simulates the cycling of major elements and nutrients for primary producers in marine systems. It includes assimilation processes related to light and temperature in the euphotic zone, influencing primary production and the marine food web associated with zooplankton and benthic communities (Butenschön et al., 2016). BROM focuses on the redox dynamics driven by biogeochemical interactions between the bottom layer of the water column and the upper layer of benthic sediments. It considers biogeochemical cycles of various elements, structures in bacterial community, and detailed carbonate chemistry. These models were used to represent the biogeochemical processes including carbonate chemistry in the water column, sea-ice, and sediments.
Given the lack of available tools to model the complex ecosystem dynamics of the sympagic algae (van Leeuwe et al., 2018), we simplified the representation by adding one diatom species in the sea-ice domain to the standard ERSEM model. We assumed that the ecological functioning of sympagic algae is similar to that of pelagic diatoms in seawater acknowledging that ecological differences may exist between these groups. However, this assumption allows us to investigate the potential impacts of sympagic algae on pelagic biogeochemistry in polar seas given the limited information on the sea-ice ecosystem. Our model incorporates five groups of phytoplankton including pelagic diatoms, pico-phytoplankton, nano-phytoplankton, Phaeocystis, and sympagic diatoms, and modified the sea-ice algal growth parameters.
SPBM solves a system of 1-D transport equations for the sea-ice, water column, and benthic domains. The dynamics can be expressed as:
where denotes i-th state variable given by ERSEM or BROM, t the time step, and z the depth. is the porosity-related area restriction factor, D the total diffusivity, the porosity factor, and u the sinking velocity that includes advection and burial in sediments. represents the combined sources-minus-sinks of the state variable. Te values of , , D, and u vary across the three domains and the phase of the variables, such as solution or solid particle.
To provide inputs for SPBM, we first determined the total ice thickness (Hice) by running a hydrodynamic model. The sea-ice column is discretized into layers with constant thickness (zs) to calculate the vertical fluxes of particulate matter and solutes in the sea-ice matrix and brine channels. During the growth and melt of the sea-ice column, Hice is adjusted by varying the number of layers. The particulate matter concentrations are assumed to be the same in the brine channels and sea-ice matrix, with = 1, while the vertical flow of materials is restricted to the brine channels where particulates are mobilized, thus Af = ϕ(z), the porosity. ϕ(z) is equal to the relative volume of the brine channels to total ice volume (Arrigo et al., 1993), which can be obtained using empirical relationships (see Supplementary Material). The sea-ice matrix expels solutes into the brine channel ( = ) and their flow is restricted to the brine channels ( = ) in the model. The total diffusivity (D) in the brine channels accounts for molecular diffusivity (Dm) at the sea-ice–water interface (applied only to solutes), gravity drainage diffusivity (Dgd) at depth z, and diffusivity by convection in the bottom layer of growing sea-ice (Dgi) (Arrigo et al., 1993). The specific equations for individual diffusivities are provided by Yakubov et al. (2019).
The Photosynthetically Available Radiation (PAR) on the surface of water or ice is obtained from the hydrodynamic model. In the presence of ice, the SPBM calculate Pa, which represents PAR, by considering sea-ice and snow albedo. The PAR equations depend on the snow depth (zsnow) as follows (Light et al., 2008):
where is the fraction of radiation transmitted through the highly scattering surface of the ice, is the ice albedo for visible light, is the snow albedo for visible light, is the snow light extinction coefficient (m−1), and is the snow depth (m). We used 0.744 for (Light et al., 2008), 0.81 for (Allison et al., 1993), and 4.3 for (Perovich, 2007) in this study. The PAR at any depth in the ice, is given by:
where is the ice light extinction coefficient (0.93 m−1 in this study following Light et al. (2008)), and is the ice depth (m). The hydrodynamic model used in this study (see Section 2.2) does not simulate the temporal variation of snow depth. Instead, we assumed a constant snow depth of 0.3 m during the presence of the sea-ice, based on the mean observed snow depth in the Amundsen Sea in December (Arrigo et al., 2014) in order to consider the light attenuation caused by the snow depth.
The hydrodynamic model also calculates vertical eddy diffusivity values at each layer of the water column, which are used to calculate vertical fluxes of nutrients and solutes. The benthic flux across the sediment-water interface is computed based on the thickness of the benthic boundary layer (BBL), sediment type, and particle size. The BBL thickness is defined as the height above the sediments where the eddy diffusivity reaches a constant value (Yakubov et al., 2019). Additionally, SPBM accounts for the deposition and resuspension of particulate matter from the sediments to the water column, considering particle size, sediment composition, current velocity, and turbulence (Yakubov et al., 2019).
The model also considers the exchange of gases across the air-water interface, including the exchange of CO2 and oxygen (O2), which is calculated based on the gas transfer velocity and the concentration gradient between the air and water (Nightingale et al., 2000). The carbonate chemistry in the water column, sea-ice, and sediments is represented using a module from BROM that accounts for the dissolution and precipitation of calcium carbonate minerals and the associated changes in concentrations of carbonate ions, bicarbonate ions, and pH (Yakushev et al., 2017). This comprehensive representation of biogeochemical processes allows for a more realistic simulation of ecosystem dynamics in the polar seas.
To integrate the sea-ice and hydrodynamic models, we used the Simple Thermodynamic Ice Model (STIM) as an intermediary step. STIM isolates specific ice algorithms from the hydrodynamic model, providing a well-defined interface to test and use various ice models. We selected the General Ocean Turbulence Model (GOTM; Burchard and Petersen, 1999), a 1-D hydrodynamic model, to simulate vertical profiles of temperature and salinity. We employed MyLake (Saloranta and Andersen, 2007), one of the three thermodynamic ice models included in STIM to simulate the thermodynamic processes of sea-ice growth and melt. Although MyLake was originally developed for simulating lake ice, it can also be used for simulating sea-ice since both types of ice form when water temperature drops below the freezing point. However, it is important to note that fundamental differences exist between lake ice and sea-ice, such as the presence of salt and the impact of ocean currents on sea-ice formation. Therefore, the accuracy of using MyLake to simulate sea-ice thickness may vary depending on the specific conditions being modeled and the level of detail required in the simulation. In this study, we set the freezing point (Tf) at –1.86°C, according to Arrigo et al. (1993), which corresponds to the freezing point of seawater at approximately 34 salinity. However, we did not consider the effect of ocean currents as our model is one-dimensional and cannot account for their complex dynamics. Despite this limitation, using MyLake allowed us to efficiently generate hydrodynamic and sea-ice thickness data as input for the SPBM while simplifying the representation of the sea-ice system.
The formation of sea-ice in the STIM framework occurs when the seawater temperature reaches Tf. In the super-cooled layers with temperatures below Tf, the heat deficit is transformed into an initial sea-ice layer with a given thickness (Hice) by converting the sensible heat deficit of seawater into latent heat of sea-ice. Whenever the air temperature (Ta) falls below Tf, new sea-ice formed, and its thickness () is calculated using Stefan’s law:
where (W K−1 m−1) is the thermal conductivity of sea-ice, (kg m−3) is the density of the sea-ice, and L is the latent heat of freezing (J kg−1). The temperature of the sea-ice surface, Tice, is calculated assuming that the heat fluxes through the sea-ice and the overlying are equal to those at the interface between the sea-ice and seawater. When Ta is above Tf, sea-ice growth is stopped and melting begins. The energy for melting is calculated based on the heat flux at the sea-ice-air interface, assuming that short-wave radiation is partially absorbed on the sea-ice layer. When sea-ice is present, the seawater temperature is set at Tf. When the daily mean seawater temperature is above Tf, the bottom layer of sea-ice started melting. Please refer to aloranta and Andersen (2007) for sea-ice parameterizations in STIM. It should be noted that the present model does not consider snow accumulation and its effects on forming and melting of sea-ice. Snow can impact both sea-ice thickness and the duration of the sea-ice season (e.g., Powell et al., 2005; Sturm and Massom, 2017; Webster et al., 2018). However, recent modeling study suggests that the impact of snow over the sea-ice properties is greater for the multi-year sea-ice than for single-year seasonal sea-ice (Holland et al, 2021). Besides, modeling sea-ice deformation such as flooding, ridging, and rafting and the complex processes occurring in the snow and sea-ice system, is challenging with a 1-D framework. Since our focus is on the impact of algal production in the single-year seasonal sea-ice on the pelagic ecosystem, the absence of snow in the sympagic model does not introduce bias in representing the sea-ice ecosystem.
The implementation of SPBM required time-dependent input parameters such as turbulent diffusivity, temperature, and salinity for the water column, as well as total thickness and surface temperature of the sea-ice. Downwelling shortwave radiation and PAR were externally provided, computed using meteorological parameters from the ERA-Interim reanalysis data (Dee et al., 2011) with a resolution of 0.25°x0.25° and a 6-hour interval. The time-independent values for the water column structure were derived from the GOTM-STIM simulation.
To obtain the input values required for SPBM, we first ran the GOTM-STIM following the procedure outlined in Kwon et al. (2021). The simulation aimed to represent the vertical structure of physical properties in the western part of the Amundsen Sea continental shelf (as depicted in Figure 1 of Kwon et al. (2021)) from 1 January 1997 to 31 December 2017. The vertically averaged CTD data within the range of 74˚S to 72˚S and 110˚W to 120˚W were used as a source for the relaxation of the 1-D model. This allowed us to simulate the water column structure and provide input for the biogeochemical models (e.g., ERSEM and BROM) described earlier. To extract atmospheric forcing parameters such as zonal and meridional wind speeds, surface air temperature, dew point, and total cloud concentration, we averaged the ERA-interim data over the same area. The model was run with 10-minute time step and solved on vertical domains consisting of 40 layers in the upper 500 m of the water column. The surface layer had the highest vertical resolution (1.6 m) while the middle layer had the lowest resolution (21.7 m). A closed boundary condition was adopted at the lower boundary of the water column. The model was spun up for twenty years with the atmospheric forcing parameters set to those of 1996 to reach a quasi-equilibrium state.
Figure 1 (A) Light limitation factor (Li) against PAR for the phytoplankton species used in the model and (B) a comparison of temperature limitation factor (Lt) against the ambient temperature for sympagic and pelagic phytoplankton. Vertical dotted line in (B) indicates the freezing point of the sea-ice given in the model.
Several parameters that affect primary production in ERSEM were adjusted specifically for sympagic diatoms to better suit their environment compared to the pelagic zone (Figure 1A, Table S1). These adjustments are related to photosynthesis process in sea-ice, which is influenced by light intensity and can be expressed through the light limitation factor of functional type i, Li. This is a function of several parameters as shown in Equation (6).
A significant parameter is the Q10 value which indicates the temperature sensitivity to metabolic changes. For sympagic algae, the Q10 value was set at 1.06, while all pelagic species had a Q10 value of 2.0 (Butenschön et al., 2016), suggesting that sympagic algae thrive in low-temperature environments (Kottmeier and Sullivan, 1988). Indeed, several experiments have shown that sympagic algae grow well under suboptimal (cold) conditions (Rochet et al., 1985; Palmisano et al., 1987; Cota and Smith, 1991). Lowering the Q10 value reduced the temperature limitation of sympagic algae when the sea-ice temperature is approximately below the freezing point of seawater (Figure 1B). The temperature limitation factor (LT) is a function of Q10 and the ambient temperature, whether in sea-ice or seawater (t). It is common to all functional groups:
Equation (7) includes a second term, an inhibitory term in the traditional Q10 function added by Blackford et al. (2004), which prevents excessive reaction rates at higher temperatures encountered in certain regions. This modification yields a somewhat flatter response curve, which we believe more accurately represents the wide species range and adaptability of the functional group. The initial slope of the photosynthesis-irradiance (P–I) curve (α, code ID = alphaP1_i in Table S1) for sympagic algae was set to a high value (= 8.56 mg C m2 (mg Chl)-1 W−1 d−1) to allow them to tolerate low PAR levels inside the sea-ice. The maximum specific productivity at the reference temperature (gmax, code ID = sumP1_iX in Table S1) for sympagic algae was assigned a value, 1.45 d−1 which is the same as for pelagic diatoms. van Leeuwe et al. (2018) reported that pennate diatoms which are the dominant species living on the bottom of the pack ice prefer extremely low values of light saturation due to their low tolerance to strong light intensity. Therefore, the photo-inhibition parameter (β, code ID = betaP1_i) was set twice as large (= 0.2 mg C m2 (mg Chl)-1 W−1 d−1) as for pelagic diatoms, reflecting their weak light tolerance. However, the maximal effective ratio of Chl-a to carbon (θmax, code ID = phimP1_i in Table S1) was assumed to be the same as that for pelagic diatoms. By adjusting these parameters, the P-I can represent different light acclimations as demonstrated by Butenschön et al. (2016).
In addition to the aforementioned parameters, determining Li requires PAR and the limitation factors for silicate (LS) and iron (LFe) concentrations. PAR values were provided externally and LS and LFe were determined using Michaelis-Menten uptake kinetics (Kwon et al., 2021). Figure 1A depicts the dependence of Li for a given primary producer’s functional type against PAR, assuming no limitations in the parameter values in Equation (6) and that cellular Chl-a always has the maximal value (θ = θmax). The modified values of parameters related to light intensity, such as α, β, and gmax, are responsible for the strong photo-inhibition of sympagic diatoms compared to pelagic algae.
The half-saturation values of nitrate and phosphate were set to be the same for both sympagic and pelagic diatoms, whereas that for iron was slightly lower for sympagic diatoms (0.015 nM) compared to pelagic diatoms, to account for the replete iron concentration in sea-ice (Lannuzel et al., 2007; Lannuzel et al., 2010; Lannuzel et al., 2011; Vancoppenolle et al., 2013; Arrigo et al., 2014). Sinking rate for sympagic algae was assumed to be high (1.0 m d−1) (Mathot et al., 1991; Riebesell et al., 1991; Michel et al., 1997) compared to pelagic species (0.05 m d−1 for pelagic diatoms and Phaeocystis, and no vertical sinking for nano- and pico-phytoplankton) due to the absence of aggregation of sympagic algae in the model. Sinking rates of small, medium, and large size particulate organic matter (POM) were assumed to be 10, 25, and 50 m d−1, respectively (Table S2), based on modeling studies and observations that suggest much larger sinking rates of POM than the standard ERSEM (Asper and Smith, 2003; Stemmann et al., 2004; Trull et al., 2008; Benkort et al., 2020). All model parameters used in this study including the modified parameters for sympagic diatoms are described in Kwon et al. (2021) and Table S1 in detail.
In the SPBM model, a quasi-stationary state of the biogeochemical variables was achieved by conducting two steps of spin-up runs. During the spin-up runs, the first day was repeated 100 times, followed by repeating the first year 10 times. All the state variables in the sea-ice, water column, and sediment domains were initialized to the same conditions. The initial nutrient concentrations were chosen to reflect the high macro-nutrients and the low iron concentrations typical of the Southern Ocean, following the approach used in a previous study (Kwon et al., 2021) (see Table S3 for details). The results of the model simulation after the spin-up period were used to calculate climatological means (the “reference run”).
In Table 1, we compared our model results to the observations by Fransson et al. (2011) in the Amundsen Sea during early summer. For this study, we defined early summer as the period from the middle of November (15th Nov) to early December (10th Dec), as the sea-ice completely melted away by December 10 in the model simulation (Figure 2). Our model also tended to underestimate sea-ice thickness due probably to the absence of a snow module in the model. Additionally, deformation processes of sea-ice such as ridging and flooding which are not accounted for in our model may have contributed to this discrepancy in the representation of ice thickness representation (Maksym and Jeffries, 2000; Powell et al., 2005).
Table 1 Comparison of biogeochemical tracers in the sympagic and pelagic environments between the model simulation and the observation in the Amundsen Sea.
Figure 2 Seasonal and vertical distributions of the variables in the sea-ice (upper panels) and in the water column underneath (lower panels) by the climatological simulation. (A) PAR, (B) total Chl-a, (C) phosphate, (D) nitrate, (E) silicate, (F) DIC, (G) DOC, (H) bacterial carbon mass, (I) sympagic diatoms carbon mass, (J) sum of small phytoplankton carbon mass, (K) pelagic diatoms carbon mass, (L) Phaeocystis carbon mass and (M) microzooplankton and heterotrophicnanoflagellate (HNF) mass. Note that the color-code scales for the sea-ice and water column are different. Refer to Figure S1 for the Chl-a concentration and sympagic diatoms mass in the lowermost thin layer of the sea-ice domain.
Overall, most of our model results fall within the observed ranges although there are some exceptions. Specifically, we slightly overestimated the phosphate concentration in the seawater underneath the sea-ice, which may be due to the lower ratio of N/P in diatom consumption compared to Phaeocystis (Arrigo et al., 1999), a factor that was not considered in our model. The simulated particulate organic carbon (POC) concentration in sea-ice layer was underestimated likely due to underestimated biomasses given the low average ice Chl-a in the model (Table 1).
Figure 2 illustrates the seasonal variations of chemical and biological variables relevant to algal primary production in both the sympagic and pelagic ecosystems. As incident light increases during the sea-ice melting period from October to November (Figure 2A), phytoplankton accumulates in both the sea-ice and water column, with a concentration at the bottom layer of the sea-ice (Figures 2B, S1). The high concentration of Chl-a at the lowermost ice layer during November is not readily observable in the contour plot but is evident when specifically plotted only for the lowermost layer of ice (see also Figure S1C). In contrast, maximal concentrations of Chl-a in the surface and subsurface (~20 m) waters occur in January and February, respectively, after the sea-ice has melted away. The macro-nutrients required for primary production enrich the bottom layer of the sea-ice (Figures 2C−E) due to continuous supply from the seawater underneath. Phosphate and nitrate are almost completely depleted in the uppermost sea-ice layers due to brine porosity, which allows for the transport of these nutrients into the surrounding seawater. While the model simulation shows that nitrate and silicate were concentrated in the sea-ice bottom layer, consistent with observations, Fransson et al. (2011) found that phosphate is concentrated in the sea-ice surface, which was not reproduced in our model. This discrepancy may be due to the inaccurate representation of the elemental quota in the phytoplankton cell in our model.
The model was able to represent the observed dissolved inorganic carbon (DIC) concentrations, with lower concentrations in the sea-ice layers compared to the water column (Figure 2F). This is consistent with the process of brine rejection, which leads to a large reduction of DIC in the sea-ice (Rysgaard et al., 2007; Fransson et al., 2011).
Algal blooms in the sea-ice and in the pelagic water column result in a seasonal accumulation of dissolved organic carbon (DOC) (Figure 2G). The DOC concentration in the water column reaches its peak during mid to late summer (January to February) when primary production is most active. A fraction of the DOC is subsequently incorporated into the sea-ice, where it is gradually oxidized over time. The bacterial distribution in the sea-ice reflects the overall distribution of DOC (Figure 2H). The simulated bacterial respiration in the upper 350 m (averaging 300 mg C m-2 d-1) is significantly underestimated, as the observed respiration rate is twice as high (Ducklow et al., 2015) (= 789.1 mg C m-2 d-1 on average), which seems to stem from the inaccurate representation of bacterial physiology in this area.
The community structure within the sea-ice is primarily characterized by the dominance of sympagic diatoms in terms of biomass while Phaeocystis is the most abundant species in the water column. Sympagic diatoms exhibit a concentration in the bottom layer of the sea-ice and their biomass increases largely during the period of November-December when the sea-ice starts to melt (Figures 2I, S1). Because the photosynthetic sensitivity to the solar radiance (high α value) of the sympagic diatoms is set to be largest among those of primary producers in the model, they grow rapidly despite considerably low PAR levels below ~5 W m-2 (Figure 2A).
A substantial number of sympagic diatoms located at the bottom of the sea-ice are released into the upper pelagic layer during sea-ice melt, and then they sink rapidly relative to other large pelagic diatoms and Phaeocystis. Importantly, the sympagic diatoms continue to grow while sinking, and reach their maximum biomass in the subsurface layers (~20 m) due to their efficient acclimation to low light conditions. As shown in Figure 2B, the peak concentration of Chl-a occurs in the subsurface layers in early February because of the growth of sympagic diatoms and Phaeocystis.
In terms of biomass, pelagic diatoms are the second most abundant group in the sea-ice, although their biomass is two-orders of magnitude less than that of sympagic diatoms (Figure 2K). The pelagic diatoms biomass in the sea-ice show the gradual reduction after a sharp increase in March and April. This implies that a portion of pelagic diatom cells may be incorporated into the ice during freezing in February, but their population is likely to decrease over time due to cell mortality in the absence of incident light and the extremely low temperatures (~−6.6°C on average) within the ice during fall and winter. Chl-a concentration in the sea-ice peaked in late November when sympagic diatoms bloom on the bottom of ice (Figures 2B, S1).
In contrast, the nano- and pico-phytoplankton (small species) remain in the upper ice without migrating downward and show their maximum abundance at the onset of ice formation, indicating their entrainment into the ice during sea-ice buildup. These small algal functional types have fairly low half-saturation values for nutrients compared to larger algal functional types. Consequently, they are concentrated in the upper layers of the ice where nutrients are almost depleted due likely to avoiding competition in diatoms (Figure 2J).
While Phaeocystis dominates the pelagic ecosystem in the model, it shows negligible biomass throughout the ice layers (Figure 2L). This discrepancy arises from in the low-iron condition of the sea-ice in the model (~0.09 nM, not show here), which renders Phaeocystis less competitive due to their much higher half-saturation value (kFe) compared to other species. The model results, highlighting the dominance of sympagic diatoms in the bottom layer of the sea-ice and the prevalence of small species in the surface layer of the ice, are consistent with observations by van Leeuwe et al. (2018).
We conducted an additional simulation in our model to investigate the influence of sea-ice algal dynamics on the pelagic environment. In this simulation, we excluded biological processes within the ice domain and treated the sea ice solely as a light attenuator and a barrier to heat flux between the sea and the atmosphere. No exchange of state variables occurred between the water column and the ice domain. The functional type of sympagic diatoms was also omitted in this experiment (Figure 3I), while all other model configurations remained the same as described in Section 3.
Figure 3 Climatological simulation results of the water column under the condition devoid of sea-ice biology. (A) PAR, (B) total Chl-a, (C) phosphate, (D) nitrate, (E) silicate, (F) DIC, (G) DOC, (H) bacterial carbon mass, (I) sympagic diatoms carbon mass (no result), (J) pelagic diatoms carbon mass, (K) sum of small phytoplankton carbon mass, (L) Phaeocystis carbon mass and (M) microzooplankton and heterotrophicnanoflagellate (HNF) mass.
Our results revealed that in the absence of sea-ice biology, the Chl-a concentration in the pelagic layers was lower despite similar light conditions in the upper water column (Figures 3A, B). This decline in Chl-a concentration compared to the reference run was due to a considerably reduced biomass of diatoms (Figure 3K) which have a high cellular Chl-a to carbon ratio. The seasonal variation of silicate was negligible (Figure 3E) as diatom growth was suppressed.
The reduction in diatom biomass without sea-ice biology can be attributed to their physiological characteristics as reflected in the model. While diatoms can dominate under low-iron conditions because of their low kFe, they are less competitive against Phaeocystis under weak irradiance as they have a low α value. These properties enable diatoms to grow much faster in the ice column, leading to the release of substantial biomass of diatom cells into the pelagic layer upon ice melting in the reference simulation. Similarly, the growth of pico- and nano-phytoplankton in the water column is limited without their growth occurring prior to ice melting and subsequent release from the ice (Figure 3J). These results underscore the potentially important role of sea-ice in establishing growth conditions for diatoms and small species in the Antarctic polynya regions.
Contrary to the reference run where high biomass of Phaeocystis occurred only in January, the model without sea-ice biology exhibited sustained high Phaeocystis biomass from January to March, extending from the surface to a depth of 40 m in the water column (Figure 3L). Phaeocystis biomass was also more than twice as high as in the reference run. During the early bloom season of the pelagic layer when the growth of diatoms and small species is not yet accelerated, Phaeocystis rapidly depleted resources (iron and nutrients) in the upper layers due to their high α value, facilitating efficient nutrient uptake under dim light conditions. In an environment where the growth of sympagic diatoms and competing small species is suppressed, Phaeocystis utilized resources up to the subsurface layers by March. Consequently, nutrient and DIC depletion extended to deeper levels (Figures 3C, D, F). The distribution of zooplankton biomass also showed a similar pattern to the distribution of Phaeocystis biomass (Figure 3M). Phaeocystis, with much higher gmax and α values compared to sympagic diatoms, achieved a biomass nearly three times higher than that of diatoms in the reference simulation. As a result, larger amounts of DOC and bacterial biomass were simulated in the water column (Figures 3G, H). Figure 4 presents a comparison of Chl-a concentration and vertically integrated net primary production (NPP) in the water column between the reference model and the model without the sea-ice biology, in comparison to MODIS observations. The model without sea-ice biology exhibited a Chl-a peak occurring two weeks later and an NPP peak one month later compared to the reference run and the observations. This suggests that primary production within the sea ice from an early stage enables more rapid primary production in the pelagic environment after ice melting. In addition, the maximum Chl-a concentration and NPP in the reference model were ~2.5 times and ~20% larger, respectively, than those in the model without sea-ice biology, due to the more efficient Chl-a assimilation and photosynthesis of diatoms. As described in Section 4.2, the model without sea-ice biology had significantly lower diatom biomass compared to Phaeocystis biomass, with diatoms representing less than 1% of the Phaeocystis biomass. Considering the average biomass ratio between the two functional groups in the Amundsen Sea and the slight dominance of Phaeocystis (Lee et al., 2016; Yager et al., 2016), the model excluding sea-ice biology might challenge the potential important role of sympagic algae seeding for pelagic diatom growth in the upcoming summer season. These findings highlight the significance of the biogeochemical interplay between sea ice and the pelagic water column, which influences the community structure of lower-trophic ecosystems and, ultimately, the marine carbon cycles in polar oceans.
Figure 4 Remotely observed and simulated (A) Chl-a at the water surface and (B) vertically integrated NPP in the water column. Solid and dotted lines denote simulations with and without the sea-ice biology in the model, respectively, and bar graph for the remote observation by MODIS from Kwon et al. (2021).
The model results provided compelling evidence that the presence or absence of sea-ice biology has a significant influence on the phytoplankton community structure, with implications for mass flux and carbon export to deeper layers below the twilight zone. Different functional types of phytoplankton have varying sinking rates and cellular carbon contents, which are ultimately influenced by sea-ice ecodynamics. In the Amundsen Sea Polynya, where the sea ice retreats in late spring or early summer, the high primary productivity in the sea ice or grazing of sympagic algae have been suspected to be closely linked to the large mass flux captured at deeper layers in late winter to early spring (Fransson et al., 2011; Arrigo et al., 2014). These speculations are further supported by comparisons between sediment trap data collected in the western Antarctic Peninsula (WAP) and the Amundsen Sea (Ducklow et al., 2015). In the WAP, where the sea ice retreats as early as September, the vertical mass flux peaks in March, coinciding with the maximum sea surface Chl-a concentration. In this region, the vertical mass flux is driven by primary productivity in the water column because the sea ice completely disappears well before the irradiance increases enough to stimulate primary production, and the sea-ice season is relatively shortly (~two months). Conversely, in the Amundsen Sea, the presence of sea ice until the spring season allows sea-ice biological processes to influence POC flux before the growth of phytoplankton in the water column. Consequently, the release of particles and organisms from the sea ice during its melt likely contributes to the maximal mass flux observed in the pre-bloom season in the Amundsen Sea, as represented in our model (Figure 5).
Figure 5 Seasonal variations of (A) the modelled sea-ice thickness (blue) and the modelled Chl-a concentration (cyan) at the bottom layer of sea-ice, (B) the modelled (solid line) and observed (bar) Chl-a concentration at the water surface of the Amundsen Sea continental shelf, (C) the modelled POC concentration at the water surface, and (D) the modelled (solid line) and observed (bar) vertical POC fluxes at 350 m deep in the continental shelf of the Amundsen Sea. In (B), lime solid line denotes the SPBM simulation and lime bar represents monthly mean of the MODIS observations in Kwon et al. (2021). In (D), solid and dotted lines are with and without the sea-ice biology, respectively, and bar graph indicates the observation by Ducklow et al. (2015). Note that the vertical scales for the model simulation and the observation are different for the mass and POC fluxes.
Figure 5 presents the model results of sea-ice thickness, Chl-a concentrations at the bottom of the sea ice and water surface, water surface POC concentration, and POC flux at a depth of 350 m, compared with sediment trap data. Until December when sea ice is present, the Chl-a concentration within the sea ice increases, reaching its maximum in December, while the Chl-a concentration in the surface water peaks in January after the disappearance of sea ice. Subsequently, after approximately one month, the surface water POC concentration and POC flux at 350 m depth significantly increase (Figures 5C, D). Sediment trap data indicates that POC flux increases in February to March, likely associated with the peak of phytoplankton bloom in January (Ducklow et al., 2015). The reference model and the model without sea-ice biology show POC flux maxima in March and April, respectively, both exhibiting comparable representations for POC flux driven by phytoplankton bloom. However, the model without sea-ice biology fails to reproduce the primary peak of POC flux observed in the pre-bloom season while the reference model successfully captures this primary peak albeit occurring one month earlier (October) than observed (November).
As the sea ice thickness decreases due to melting in October (Figure 5A), the POC concentration in the uppermost layer of the water column reaches its maximum (Figure 5C) as a result of POC released from the sea ice. These POC particles are predominantly originate from sympagic diatom cells or large-sized POC that were entrained during the previous productive season. Due to their characteristics, they exhibit relatively high sinking velocities (25 and 50 m day-1 for medium and large-sized particles, respectively). Consequently, the POC flux at 350 m depth reaches its peak within two weeks after the POC peak in the surface water. The sinking POC during this period primarily consists of medium- or large- sized particles in the model results (Figure S2), which are mainly produced through diatom and zooplankton cell lysis, both in the sympagic and pelagic regions. Notably, the zooplankton biomass is concentrated only in March, with lower values in the water column, and is distributed solely in the upper ice layer after March (Figure 2M). These findings strongly suggest that the primary peak in POC flux, observed before the pelagic phytoplankton bloom period, originates from sympagic-diatom-associated particles (cell lysis) released during ice melting or transported through brine channels. Entrapping of phytoplankton onto the growing sea ice and/or blooming of sympagic algae in late fall have been reported (De Jong et al., 2018), which is associated with the massive POC flux in early spring. Furthermore, during this early season, the pelagic remineralization processes are likely suppressed by the still-cold seawater, leading to a more efficient export of POC to the deep layers. In contrast, the POC derived from the pelagic phytoplankton bloom during the summer season is expected to undergo more rapid remineralization, resulting in a reduction in the secondary peak observed after January, which is consistent with both the model and the data.
We conducted model experiments to investigate processes that could potentially lead to a decoupling between surface primary production and vertical POC flux aiming to gain a better understanding of the sea-ice ecosystem’s role in the polar marine carbon cycle. Despite the imperfect match between the model and observed POC flux, the general conclusion regarding the significant contribution of sea ice to the deep-sea POC flux, as proposed by Arrigo et al. (2012), remains valid. They suggested that the algal production occurring in the bottom layer of the sea ice and the underlying pelagic water column result in a notable flux of POC and other particulate matter. However, in order to establish the true significance of sea ice in the carbon cycle, it is crucial to address the discrepancies in magnitude and timing between observed and simulated POC flux maxima.
The model exhibited an earlier peak and larger magnitude of POC flux peak compared to the observation (Figure 5C). The earlier peak can be attributed to the model simulating sea-ice retreat earlier than observed. To align the model with observed timing, future improvements to the STIM model should consider representing the timing of sea-ice retreat one month later, in accordance with observations. Additionally, our model represented a tenfold larger POC flux peak in the pre-bloom season compared to the observed data, indicating an underestimation of bacterial respiration in the model. Studies in the Amundsen Sea Polynya by Williams et al. (2016) demonstrated high activity levels of particle-associated heterotrophic microbial communities, suggesting that they likely limit the biological pump efficiency during the early season. Previous calculations by Yager et al. (2016) and Ducklow et al. (2015) reported that approximately 3% of the net community production (NCP) integrated over the upper 100 m water column is annually trapped, with only 6% being trapped during the peak period in the Amundsen Sea. In our model, the annual NCP was estimated to be 49 g m-2 yr-2, while the POC flux at a depth of 350 m was estimated to be 16 g m-2 yr-2. This indicates that approximately 33% of the NCP is being sedimented as particles, which is significantly higher (around 11 times on an annual scale) than the values reported in Ducklow et al. (2015). This discrepancy clearly indicates an overestimation of the POC flux at depth in our model, both in relative and absolute terms. Consequently, it highlights that the standard biogeochemical models adopting general Q10 function may underestimate bacterial respiration in the Antarctic regions (e.g., Cavan et al., 2019) and the crucial role of bacteria in the Antarctic marine carbon cycle cannot be disregarded (Ducklow, 1999; Ducklow et al., 2015; Williams et al., 2016; Yager et al., 2016; Kwon et al., under review).
Furthermore, we performed sensitivity analyses by adjusting the sinking rates of small, medium, and large-sized particles by ±10% from the default values in Section 3 given that uncertainties in the vertical sinking rates of particles can influence the accuracy of POC flux simulations. The magnitude of the POC flux was highly dependent on the sinking rate, while the timing of the POC flux peak remained relatively stable (Figure S3). Previous studies have demonstrated that sinking rates of particles are influenced by various environmental factors, such as temperature, depth, and particle origin, making the sinking process complex and variable (Cadée et al., 1992; Michel et al., 2006; Wassmann et al., 2006; De La Rocha and Passow, 2007; Iversen and Ploug, 2013; Tréguer et al., 2017). However, due to limited observations and the scope of our study, we did not delve into the specific mechanisms and variability of sinking rates in the Southern Ocean. Future research efforts will be also dedicated to investigating particle sinking processes in the polar regions to enhance our knowledge of the Southern Ocean’s role as a carbon sink under a changing climate.
Our study aimed to investigate the role of sea ice in the marine carbon cycle of polar oceans, with a specific focus on the Amundsen Sea, utilizing a biogeochemical model that accounts for the sea-ice ecosystem. Our findings provide important insights into the contribution of sea ice to primary production, algal community structure, and carbon export to the pelagic ecosystem (Figure 6). Our model corroborated the notion that the sympagic algae thriving within sea ice during the pre-bloom season can serve as a seed population for pelagic diatoms. Furthermore, our model simulations revealed that the presence of sea ice leads to an earlier peak in POC flux at depth compared to the algal bloom in the water column. This can be attributed to the release of medium to large particles from the sea ice during the early stages of ice melting. These particles originate from diatom cells or organic matter entrapped within the ice during the previous ice formation season. Overall, our study highlights the significant contribution of the sea-ice ecosystem to the polar marine carbon cycle. Future research should focus on addressing uncertainties in sinking rates of particles including, microbial activities, to further improve our understanding of the polar marine carbon cycle.
Figure 6 Overview summary of the main findings regarding the role of sea-ice in the flux of POC to the deep sea. The primary peak in POC flux, observed before the pelagic phytoplankton bloom, is likely influenced by sympagic-diatom-associated particles transported from ice porosity or released during ice melting. During this early season, pelagic remineralization processes are suppressed by the still-cold seawater leading to a more efficient export of POC to the deep layers. The POC derived from the pelagic phytoplankton bloom during the summer season undergoes more rapid remineralization, resulting in a reduction in the secondary peak observed after January.
The original contributions presented in the study are included in the article/Supplementary Material. Further inquiries can be directed to the corresponding author.
YK conducted the modeling experiments, collated the observed data and wrote the manuscript with contributions from TR who designed the study. KB developed STIM model and have given approval to the final version of the manuscript. All authors contributed to the article and approved the submitted version.
This research was supported by the Korea Polar Research Institute grant (PE23140) and Korea Institute of Marine Science & Technology Promotion (KIMST) funded by the Ministry of Oceans and Fisheries (20210696, 20220033, 20210632).
We appreciate the constructive comments from the reviewers, which greatly improved the manuscript.
The authors declare that the research was conducted in the absence of any commercial or financial relationships that could be construed as a potential conflict of interest.
All claims expressed in this article are solely those of the authors and do not necessarily represent those of their affiliated organizations, or those of the publisher, the editors and the reviewers. Any product that may be evaluated in this article, or claim that may be made by its manufacturer, is not guaranteed or endorsed by the publisher.
The Supplementary Material for this article can be found online at: https://www.frontiersin.org/articles/10.3389/fmars.2023.1181650/full#supplementary-material
Allison I., Brandt R. E., Warren S. G. (1993). East Antarctic Sea-Ice - albedo, thickness distribution, and snow cover. J. Geophys Res-Oceans 98 (C7), 12417–12429. doi: 10.1029/93jc00648
Armand L. K., Leventer A. (2010). Palaeo sea-ice distribution and reconstruction derived from the geological records in Thomas D. N., Dieckmann G. S. (eds), Sea ice. 2nd edn, Wiley-Blackwell, Wiley, UK. 469–530. doi: 10.1002/9781444317145.ch13
Arrigo K. R., Lowry K. E., van Dijken G. L. (2012). Annual changes in sea ice and phytoplankton in polynyas of the Amundsen Sea, Antarctica, Deep-Sea Res Pt Ii, 71-76, 5–15. doi: 10.1016/j.dsr2.2012.03.006
Arrigo K. R., Brown Z. W., Mills M. M. (2014). Sea-Ice algal biomass and physiology in the amundsen Sea, Antarctica. Elementa: Sci. Anthropocene 2. doi: 10.12952/journal.elementa.000028
Arrigo K. R., Dieckmann G., Gosselin M., Robinson D. H., Fritsen C. H., Sullivan C. W. (1995). High resolution study of the platelet ice ecosystem in McMurdo sound, Antarctica: biomass, nutrient, and production profiles within a dense microalgal bloom. Mar. Ecol. Prog. Ser. 127, 255–268. doi: 10.3354/meps127255
Arrigo K. R., Kremer J. N., Sullivan C. W. (1993). A simulated Antarctic ast ice ecosystem. J. Geophysical Research: Oceans 98 (C4), 6929–6946. doi: 10.1029/93JC00141
Arrigo K. R., Mills M. M., Kropuenske L. R., van Dijken G. L., Alderkamp A. C., Robinson D. H. (2010). Photophysiology in two major southern ocean phytoplankton taxa: photosynthesis and growth of phaeocystis antarctica and fragilariopsis cylindrus under different irradiance levels. Integr. Comp. Biol. 50 (6), 950–966. doi: 10.1093/icb/icq021
Arrigo K. R., Robinson D. H., Worthen D. L., Dunbar R. B., DiTullio G. R., VanWoert M., et al. (1999). Phytoplankton community structure and the drawdown of nutrients and CO2 in the southern ocean. Science 283 (5400), 365–367. doi: 10.1126/science.283.5400.365
Arrigo K. R., Worthen D. L., Robinson D. H. (2003). A coupled ocean-ecosystem model of the Ross Sea: 2. iron regulation of phytoplankton taxonomic variability and primary production. J. Geophysical Res. 108 (C7). doi: 10.1029/2001jc000856
Asper V. L., Smith W. O. (2003). Abundance, distribution and sinking rates of aggregates in the Ross Sea, Antarctica. Deep Sea Res. Part I: Oceanographic Res. Papers 50 (1), 131–150. doi: 10.1016/S0967-0637(02)00146-2
Blackford J. C., Allen J. I., Gilbert F. J. (2004). Ecosystem dynamics at six contrasting sites: a generic modelling study. J Marine Syst 51 (1-4), 191–215. doi: 10.1016/j.jmarsys.2004.02.004
Benkort D., Daewel U., Heath M., Schrum C. (2020). On the role of biogeochemical coupling between sympagic and pelagic ecosystem compartments for primary and secondary production in the barents Sea. Front. Environ. Sci. 8 (217). doi: 10.3389/fenvs.2020.548013
Bluhm B. A., Gradinger R. R., Schnack-Schiel S. B. (2010). Sea-Ice meio-and macrofauna. Sea-ice 2, 357–393. doi: 10.1002/9781444317145.ch10
Brierley A. S., Thomas D. N. (2002). Ecology of southern ocean pack ice. Adv. Mar. Biol., (43), 171–IN174. doi: 10.1016/S0065-2881(02)43005-2
Bruggeman J., Bolding K. (2014). A general framework for aquatic biogeochemical models. Environ. Model. software 61, 249–265. doi: 10.1016/j.envsoft.2014.04.002
Burchard H., Petersen O. (1999). Models of turbulence in the marine environment - a comparative study of two-equation turbulence models. J. Mar. Syst. 21 (1-4), 29–53. doi: 10.1016/S0924-7963(99)00004-4
Butenschön M., Clark J., Aldridge J. N., Allen J. I., Artioli Y., Blackford J., et al. (2016). ERSEM 15.06: a generic model for marine biogeochemistry and the ecosystem dynamics of the lower trophic levels. Geoscientific Model. Dev. 9 (4), 1293–1339. doi: 10.5194/gmd-9-1293-2016
Cadée G., González H., Schnack-Schiel S. (1992). “Krill diet affects faecal string settling,” in Weddell Sea ecology (Berlin Heidelberg: Springer), 75–80.
Cavan E. L., Henson S. A., Boyd P. W. (2019). The sensitivity of subsurface microbes to ocean warming accentuates future declines in particulate carbon export. Front. Ecol. Evol. 6. doi: 10.3389/fevo.2018.00230
Cota G. F., Smith R. E. H. (1991). Ecology of bottomsympagic algae: III. comparative physiology. J. Mar. Syst. 2 (3), 297–315. doi: 10.1016/0924-7963(91)90038-V
Cowie R. O. M., Williams G. J., Maas E. W., Voyles K. M., Ryan K. G. (2014). Antarctic Sea-ice microbial communities show distinct patterns of zonation in response to algal-derived substrates. Aquat Microb. Ecol. 73 (2), 123–134. doi: 10.3354/ame01710
De Jong H. B., Dunbar R. B., Lyons E. A. (2018). Late Summer Frazil Ice-Associated Algal Blooms around Antarctica. Geophys Res Lett. 45 (2), 826–833. doi: 10.1002/2017GL075472
Deal C., Jin M., Elliott S., Hunke E., Maltrud M., Jeffery N. (2011). Large-Scale modeling of primary production and ice algal biomass within arctic sea-ice in 1992. J. Geophysical Research: Oceans 116 (C7), (C07004). doi: 10.1029/2010JC006409
Dee D. P., Uppala S. M., Simmons A. J., Berrisford P., Poli P., Kobayashi S., et al. (2011). The ERA-interim reanalysis: configuration and performance of the data assimilation system. Q. J. R. Meteorological Soc. 137 (656), 553–597. doi: 10.1002/qj.828
De La Rocha C. L., Passow U. (2007). Factors influencing the sinking of POC and the efficiency of the biological carbon pump. Deep Sea Res. Part II: Topical Stud. Oceanography 54 (5), 639–658. doi: 10.1016/j.dsr2.2007.01.004
Dieckmann G. S., Hellmer H. H. (2010). The importance of sea-ice: an overview. Sea-ice 2, 1–22. doi: 10.1126/science.276.5311.394
Ducklow H. W. (1999). The bacterial component of the oceanic euphotic zone. FEMS Microbiol. Ecol. 30 (1), 1–10. doi: 10.1111/j.1574-6941.1999.tb00630.x
Ducklow H. W., Wilson S. E., Post A. F., Stammerjohn S. E., Erickson M., Lee S., et al. (2015). Particle flux on the continental shelf in the amundsen Sea polynya and Western Antarctic peninsula. Elementa-Sci Anthrop 3. doi: 10.12952/journal.elementa.000046
Eilola K., Mårtensson S., Meier H. E. M. (2013). Modeling the impact of reduced sea-ice cover in future climate on the Baltic Sea biogeochemistry. Geophysical research letters 40, 1, 149–154. doi: 10.1029/2012GL054375
Fransson A., Chierici M., Yager P. L., Smith W. O. Jr. (2011). Antarctic Sea-ice carbon dioxide system and controls. J. Geophysical Research: Oceans 116 (C12), (C12035). doi: https://agupubs.onlinelibrary.wiley.com/doi/full/10.1029/2010JC006844
Galindo V., Levasseur M., Mundy C. J., Gosselin M., Tremblay J.-É., Scarratt M., et al. (2014). Biological and physical processes influencing sea-ice, under-ice algae, and dimethylsulfoniopropionate during spring in the Canadian Arctic archipelago. J. Geophysical Research: Oceans 119 (6), 3746–3766. doi: 10.1002/2013JC009497
Holland M. M., Clemens-Sewall D., Landrum L., Light B., Perovich D., Polashenski C., et al. (2021). The influence of snow on sea-ice as assessed from simulations of CESM2. Cryosphere 15 (10), 4981–4998. doi: 10.5194/tc-15-4981-2021
Hunke E. C., Notz D., Turner A. K., Vancoppenolle M. (2011). The multiphase physics of sea-ice: a review for model developers. Cryosphere 5 (4), 989–1009. doi: 10.5194/tc-5-989-2011
Iversen M. H., Ploug H. J. B. (2013). Temperature effects on carbon-specific respiration rate and sinking velocity of diatom aggregates–potential implications for deep ocean export processes. Biogeosciences 10, 6, 4073–4085. doi: 10.5194/bg-10-4073-2013
Ji R., Jin M., Varpe Ø. (2013). Sea-Ice phenology and timing of primary production pulses in the Arctic ocean. Global Change Biology 19, 3, 734–741. doi: 10.1111/gcb.12074
Jin M., Deal C., Lee S. H., Elliott S., Hunke E., Maltrud M., et al. (2012). Investigation of Arctic sea-ice and ocean primary production for the period 1992–2007 using a 3-d global ice–ocean ecosystem model. Deep Sea Res. Part II: Topical Stud. Oceanography 81-84, 28–35. doi: 10.1016/j.dsr2.2011.06.003
Kirst G. O., Wiencke C. (1995). Ecophysiology of polar algae. J. Phycol 31 (2), 181–199. doi: 10.1111/j.0022-3646.1995.00181.x
Kottmeier S. T., Sullivan C. W. (1988). Sea-Ice microbial communities (SIMCO). Polar Biol. 8 (4), 293–304. doi: 10.1007/BF00263178
Kwon Y. S., La H. S., Jung J., Lee S. H., Kim T.-W., Kang H.-W., et al. (2021). Exploring the roles of iron and irradiance in dynamics of diatoms and phaeocystis in the amundsen Sea continental shelf water. Journal of Geophysical Research: Oceans 126, 3. doi: 10.1029/2020JC016673
Lalande C., Grebmeier J. M., Hopcroft R. R., Danielson S. L. (2020). Annual cycle of export fluxes of biogenic matter near Hanna shoal in the northeast chukchi Sea. Deep Sea Res. Part II: Topical Stud. Oceanography 177, 104730. doi: 10.1016/j.dsr2.2020.104730
Lannuzel D., Bowie A. R., van der Merwe P. C., Townsend A. T., Schoemann V. (2011). Distribution of dissolved and particulate metals in Antarctic sea-ice. Mar. Chem. 124 (1), 134–146. doi: 10.1016/j.marchem.2011.01.004
Lannuzel D., Schoemann V., De Jong J., Pasquer B., van der Merwe P., Masson F., et al. (2010). Distribution of dissolved iron in Antarctic sea-ice: spatial, seasonal, and inter-annual variability. J. Geophysical Research: Biogeosciences 115 (G3), G03022. doi: https://agupubs.onlinelibrary.wiley.com/doi/full/10.1029/2009JG001031
Lannuzel D., Schoemann V., de Jong J., Tison J.-L., Chou L. (2007). Distribution and biogeochemical behaviour of iron in the East Antarctic sea-ice. Mar. Chem. 106 (1), 18–32. doi: 10.1016/j.marchem.2006.06.010
Lannuzel D., Schoemann V., Dumont I., Content M., de Jong J., Tison J. L., et al. (2013). Effect of melting Antarctic sea-ice on the fate of microbial communities studied in microcosms. Polar Biol. 36 (10), 1483–1497. doi: 10.1007/s00300-013-1368-7
Lee Y., Yang E. J., Park J., Jung J., Kim T. W., Lee S. (2016). Physical-biological coupling in the amundsen Sea, Antarctica: influence of physical factors on phytoplankton community structure and biomass. Deep Sea Res. Part I: Oceanographic Res. Papers 117, 51–60. doi: 10.1016/j.dsr.2016.10.001
Light B., Grenfell T. C., Perovich D. K. (2008). Transmission and absorption of solar radiation by Arctic sea ice during the melt season. J. Geophysical Res. 113 (C3), (C03023). doi: 10.1029/2006jc003977
Maksym T., Jeffries M. O. (2000). A one-dimensional percolation model of flooding and snow ice formation on Antarctic sea ice. J. Geophysical Research: Oceans 105 (C11), 26313–26331. doi: 10.1029/2000JC900130
Martin A., McMinn A., Davy S. K., Anderson M. J., Miller H. C., Hall J. A., et al. (2012). Preliminary evidence for the microbial loop in Antarctic sea-ice using microcosm simulations. Antarctic Sci. 24 (6), 547–553. doi: 10.1017/S0954102012000491
Mathot S., Becquevort S., Lancelot C. (1991). Microbial communities from the Sea-ice and adjacent water column at the time of ice melting in the northwestern part of the weddell Sea. Polar Res. 10 (1), 267–275. doi: 10.1111/j.1751-8369.1991.tb00652.x
Meiners K. M., Vancoppenolle M., Thanassekos S., Dieckmann G. S., Thomas D. N., Tison J.-L., et al. (2012). Chlorophyll a in Antarctic sea-ice from historical ice core data. Geophysical research letters. 39 (21), L21602. doi: 10.1029/2012GL053478
Meiners K. M., Vancoppenolle M., Carnat G., Castellani G., Delille B., Delille D., et al. (2018). Chlorophyll-a in Antarctic landfast Sea-ice: a first synthesis of historical ice core data. Journal of Geophysical Research: Oceans 123, 11, 8444–8459. doi: 10.1029/2018JC014245
Michel C., Ingram R. G., Harris L. R. (2006). Variability in oceanographic and ecological processes in the Canadian Arctic archipelago. Prog. Oceanogr 71 (2), 379–401. doi: 10.1016/j.pocean.2006.09.006
Michel C., Legendre L., Taguchi S. (1997). Coexistence of microalgal sedimentation and water column recycling in a seasonally ice-covered ecosystem (Saroma-ko lagoon, Sea of Okhotsk, Japan). J. Mar. Syst. 11 (1), 133–148. doi: 10.1016/S0924-7963(96)00034-6
Mock T., Kroon B. M. A. (2002). Photosynthetic energy conversion under extreme conditions–II: the significance of lipids under light limited growth in Antarctic sympagic algaes. Phytochemistry 61 (1), 53–60. doi: 10.1016/S0031-9422(02)00215-7
Nightingale P. D., Malin G., Law C. S., Watson A. J., Liss P. S., Liddicoat M. I., et al. (2000). In situ evaluation of air-sea gas exchange parameterizations using novel conservative and volatile tracers. Global Biogeochem Cy 14 (1), 373–387. doi: 10.1029/1999gb900091
Palmisano A. C., Beeler SooHoo J., Sullivan C. W. (1987). Effects of four environmental variables on photosynthesis-irradiance relationships in Antarctic sea-ice microalgae. Mar. Biol. 94 (2), 299–306. doi: 10.1007/BF00392944
Perovich D. K. (2007). Light reflection and transmission by a temperate snow cover. J. Glaciology 53 (181), 201–210. doi: 10.3189/172756507782202919
Popova E. E., Yool A., Coward A. C., Dupont F., Deal C., Elliott S., et al. (2012). What controls primary production in the Arctic ocean? results from an intercomparison of five general circulation models with biogeochemistry. Journal of Geophysical Research: Oceans. 117, C8, C00D12. doi: 10.1029/2011JC007112
Powell D. C., Markus T., Stössel A. (2005). Effects of snow depth forcing on southern ocean sea-ice simulations. Journal of Geophysical Research: Oceans. 110, C6, C06001. doi: 10.1029/2003JC002212
Riaux-Gobin C., Poulin M., Dieckmann G., Labrune C., Vétion G. (2011). Spring phytoplankton onset after the ice break-up and sea-ice signature (Adélie land, East Antarctica). Polar Res. 0 (0). doi: 10.3402/polar.v30i0.5910
Riebesell U., Schloss I., Smetacek V. (1991). Aggregation of algae released from melting sea-ice: implications for seeding and sedimentation. Polar Biol. 11 (4), 239–248. doi: 10.1007/BF00238457
Rochet M., Legendre L., Demers S. (1985). Acclimation of sea-ice microalgae to freezing temperature. Mar. Ecol. Prog. Ser. 24 (1/2), 187–191. doi: 10.3354/meps024187
Rysgaard S., Glud R. N., Sejr M. K., Bendtsen J., Christensen P. B. (2007). Inorganic carbon transport during sea-ice growth and decay: a carbon pump in polar seas. Journal of Geophysical Research: Oceans. 112, C3, C03016. doi: 10.1029/2006JC003572
Saloranta T. M. (2000). Modeling the evolution of snow, snow ice and ice in the Baltic Sea. Tellus A: Dynamic Meteorology Oceanography 52 (1), 93–108. doi: 10.3402/tellusa.v52i1.12255
Sibert V., Zakardjian B., Gosselin M., Starr M., Senneville S., LeClainche Y. (2011). 3D bio-physical model of the sympagic and planktonic productions in the Hudson bay system. J. Mar. Syst. 88 (3), 401–422. doi: 10.1016/j.jmarsys.2011.03.014
Smetacek V., Nicol S. (2005). Polar ocean ecosystems in a changing world. Nature 437 (7057), 362–368. doi: 10.1038/nature04161
Søgaard D. H., Hansen P. J., Rysgaard S., Glud R. N. (2011). Growth limitation of three Arctic sea-ice algal species: effects of salinity, pH, and inorganic carbon availability. Polar Biol. 34 (8), 1157–1165. doi: 10.1007/s00300-011-0976-3
Stefels J., Steinke M., Turner S., Malin G., Belviso S. (2007). Environmental constraints on the production and removal of the climatically active gas dimethylsulphide (DMS) and implications for ecosystem modelling. Biogeochemistry 83 (1-3), 245–275. doi: 10.1007/s10533-007-9091-5
Stemmann L., Jackson G. A., Ianson D. (2004). A vertical model of particle size distributions and fluxes in the midwater column that includes biological and physical processes–part I: model formulation. Deep Sea Res. Part I: Oceanographic Res. Papers 51 (7), 865–884. doi: 10.1016/j.dsr.2004.03.001
Stewart F. J., Fritsen C. H. (2004). Bacteria-algae relationships in Antarctic sea-ice. Antarctic Sci. 16 (2), 143–156. doi: 10.1017/S0954102004001889
Sturm M., Massom R. A. (2017). “Snow in the sea-ice system: friend or foe?,” in Sea-Ice, (Wiley-Blackwell Publishing Ltd., U.K.) 65–109. doi: 10.1002/9781118778371.ch3
Tedesco L., Vichi M., Thomas D. N. (2012). Process studies on the ecological coupling between sympagic algae and phytoplankton. Ecol. Model. 226, 120–138. doi: 10.1016/j.ecolmodel.2011.11.011
Thomas M. K., Kremer C. T., Klausmeier C. A., Litchman E. (2012). A global pattern of thermal adaptation in marine phytoplankton. Science 338 (6110), 1085–1088. doi: 10.1126/science.1224836
Tison J. L., Worby A., Delille B., Brabant F., Papadimitriou S., Thomas D., et al. (2008). Temporal evolution of decaying summer first-year sea-ice in the Western weddell Sea, Antarctica. Deep-Sea Res. Pt Ii 55 (8-9), 975–987. doi: 10.1016/j.dsr2.2007.12.021
Torstensson A., Hedblom M., Mattsdotter Björk M., Chierici M., Wulff A. (2015). Long-term acclimation to elevated CO2 lters carbon metabolism and reduces growth in the Antarctic diatom nitzschia lecointei. Proc. R. Soc. B: Biol. Sci. 282 (1815), 20151513. doi: 10.1098/rspb.2015.1513
Tréguer P., Bowler C., Moriceau B., Dutkiewicz S., Gehlen M., Aumont O., et al. (2017). Influence of diatom diversity on the ocean biological carbon pump. Nat. Geosci 11 (1), 27–37. doi: 10.1038/s41561-017-0028-x
Trull T. W., Bray S. G., Buesseler K. O., Lamborg C. H., Manganini S., Moy C., et al. (2008). In situ measurement of mesopelagic particle sinking rates and the control of carbon transfer to the ocean interior during the vertical flux in the global ocean (VERTIGO) voyages in the north pacific. Deep Sea Res. Part II: Topical Stud. Oceanography 55 (14), 1684–1695. doi: 10.1016/j.dsr2.2008.04.021
Vancoppenolle M., Meiners K. M., Michel C., Bopp L., Brabant F., Carnat G., et al. (2013). Role of sea-ice in global biogeochemical cycles: emerging views and challenges. Quaternary Sci. Rev. 79, 207–230. doi: 10.1016/j.quascirev.2013.04.011
Vancoppenolle M., Tedesco L. (2017). Numerical models of sea-ice biogeochemistry. Sea-ice 652. doi: 10.1002/9781118778371.ch20
van Leeuwe M. A., Tedesco L., Arrigo K. R., Assmy P., Campbell K., Meiners K. M., et al. (2018). Microalgal community structure and primary production in Arctic and Antarctic sea-ice: a synthesis. Elementa-Sci Anthrop 6. doi: 10.1525/elementa.267
Wassmann P., Slagstad D., Riser C. W., Reigstad M. (2006). Modelling the ecosystem dynamics of the barents Sea including the marginal ice zone: II. carbon flux and interannual variability. J. Mar. Syst. 59 (1-2), 1–24. doi: 10.1016/j.jmarsys.2005.05.006
Webster M., Gerland S., Holland M., Hunke E., Kwok R., Lecomte O., et al. (2018). Snow in the changing sea-ice systems. Nat. Clim Change 8 (11), 946–953. doi: 10.1038/s41558-018-0286-7
Williams C. M., Dupont A. M., Loevenich J., Post A. F., Dinasquet J., Yager P. L. (2016). Pelagic microbial heterotrophy in response to a highly productive bloom of phaeocystis antarctica in the amundsen Sea polynya, Antarctica. Elementa-Sci Anthrop 4, 1–18. doi: 10.12952/journal.elementa.000102
Yager P. L., Sherrell R. M., Stammerjohn S. E., Ducklow H. W., Schofield O. M. E., Ingall E. D., et al. (2016). A carbon budget for the amundsen Sea polynya, Antarctica: estimating net community production and export in a highly productive polar ecosystem. Elementa-Sci Anthrop 4. doi: 10.12952/journal.elementa.000140
Yakubov S., Wallhead P., Protsenko E., Yakushev E., Pakhomova S., Brix H. (2019). A 1-dimensional sympagic–Pelagic–Benthic transport model (SPBM): coupled simulation of ice, water column, and sediment biogeochemistry, suitable for Arctic applications. Water 11 (8), 1582. doi: 10.3390/w11081582
Yakushev E. V., Protsenko E. A., Bruggeman J., Wallhead P., Pakhomova S. V., Yakubov S. K., et al. (2017). Bottom RedOx model (BROM v.1.1): a coupled benthic–pelagic model for simulation of water and sediment biogeochemistry. Geosci. Model. Dev. 10 (1), 453–482. doi: 10.5194/gmd-10-453-2017
Keywords: sympagic diatoms, sympagic ecosystem model, polar marine ecosystem, POC flux, ERSEM, SPBM
Citation: Kwon YS, Rhee TS and Bolding K (2023) Potential impact of the sea-ice ecosystem to the polar seas biogeochemistry. Front. Mar. Sci. 10:1181650. doi: 10.3389/fmars.2023.1181650
Received: 07 March 2023; Accepted: 03 July 2023;
Published: 21 July 2023.
Edited by:
Alberto Basset, University of Salento, ItalyReviewed by:
Michael William Lomas, Bigelow Laboratory for Ocean Sciences, United StatesCopyright © 2023 Kwon, Rhee and Bolding. This is an open-access article distributed under the terms of the Creative Commons Attribution License (CC BY). The use, distribution or reproduction in other forums is permitted, provided the original author(s) and the copyright owner(s) are credited and that the original publication in this journal is cited, in accordance with accepted academic practice. No use, distribution or reproduction is permitted which does not comply with these terms.
*Correspondence: Tae Siek Rhee, cmhlZUBrb3ByaS5yZS5rcg==
Disclaimer: All claims expressed in this article are solely those of the authors and do not necessarily represent those of their affiliated organizations, or those of the publisher, the editors and the reviewers. Any product that may be evaluated in this article or claim that may be made by its manufacturer is not guaranteed or endorsed by the publisher.
Research integrity at Frontiers
Learn more about the work of our research integrity team to safeguard the quality of each article we publish.