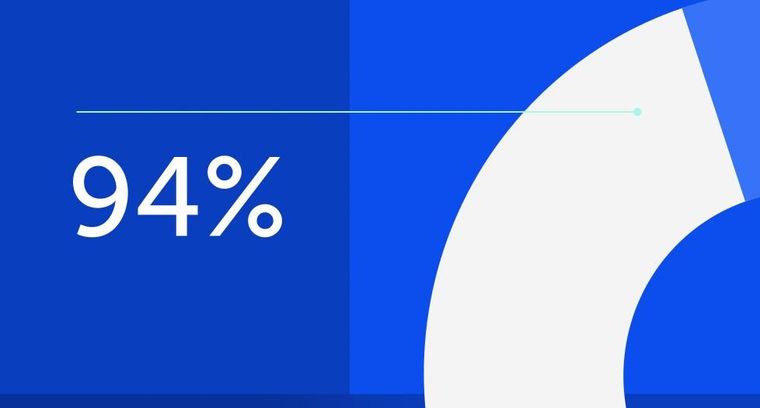
94% of researchers rate our articles as excellent or good
Learn more about the work of our research integrity team to safeguard the quality of each article we publish.
Find out more
REVIEW article
Front. Mar. Sci., 01 August 2023
Sec. Ocean Observation
Volume 10 - 2023 | https://doi.org/10.3389/fmars.2023.1176814
This article is part of the Research TopicRecent Developments in Hydrodynamic Measurement, Simulation and Forecast in the Coastal OceanView all 5 articles
Ocean observations are the foundation of our understanding of ocean processes. Improving these observations has critical implications for our ability to sustainably derive food from the ocean, predict extreme weather events that take a toll on human life, and produce the goods and services that are needed to meet the needs of a vast and growing population. While there have been great leaps forward in sustained operational monitoring of our oceans there are still key data gaps which result in sub-optimal ocean management and policy decisions. The global fishing industry represents a vast opportunity to create a paradigm shift in how ocean data are collected: the spatio-temporal extent of ocean data gaps overlaps significantly with fishers’ activities; fishing vessels are suitable platforms of opportunity to host communications and sensor equipment; and many fishing vessels effectively conduct a depth-profile through the water column in the course of normal fishing activities, representing a powerful subsurface data collection opportunity. Fishing vessel-collected ocean data can complement existing ocean observing networks by enabling the cost-effective collection of vast amounts of subsurface ocean information in data-sparse regions. There is an emerging global network of fishing vessels participating in collaborative efforts to collect oceanographic data accelerated by innovations in enabling technologies. While there are clear opportunities that arise from partnering with fishing vessels, there are also challenges ranging from geographic and cultural differences in fleets, fishing methods and practices, data processing and management for heterogeneous data, as well as long term engagement of the fishers. To advance fishing vessel-based ocean observation on a global scale, the Fishing Vessel Ocean Observing Network (FVON) aims to maximize data value, establish best practices around data collection and management, and facilitate observation uptake. FVON’s ultimate goals are to foster collaborative fishing vessel-based observations, democratize ocean observation, improve ocean predictions and forecasts, promote sustainable fishing, and power a data-driven blue economy.
The ocean (blue) economy “the sustainable use of ocean resources for economic growth, improved livelihoods, and jobs while preserving the health of the ocean ecosystem”; (United Nations, 2022) encompasses a wide range of industries including fisheries, aquaculture, shipping, transportation, manufacturing, tourism, marine biotechnology, and renewable energy (Choudhary et al., 2021). The value of the goods and services produced on, in, and for the ocean is currently between 1.5 and 2 trillion USD annually (OECD, 2016; United Nations, 2022) and is expected to reach 3 trillion USD by 2030 (Lago and Spinrad, 2022). Ocean information is the key underpinning of growth in the blue economy; governments and industries need data to expand knowledge of how marine ecosystems are changing due to anthropogenic and natural factors (Pörtner et al., 2022), increase operational efficiencies such as ship routing decisions; (Spalding et al., 2021), improve maritime security (Voyer et al., 2018), create ocean plans that balance a multitude of interests and that provide a framework for growth (Lombard et al., 2019), and effectively manage natural marine resources such as fisheries (Schmidt et al., 2019).
Ocean observations, including measurements on variables such as salinity, currents, temperature, wind speed, wave height and length, atmospheric pressure and other ocean conditions (Stammer et al., 2019), are currently collected via increasingly sophisticated sensors mounted on a growing array of mobile and fixed platforms, including autonomous underwater vehicles (AUVs), floats, buoys, drones, ships of opportunity, marine animals, and satellites (Whitt et al., 2020; Marcelli et al., 2021).
Sustained observations in the coastal and shelf seas are among the most directly societally relevant ocean observations: marine activity is focused on the ocean shelf and upper slope and nearly 2.4 billion people live within 100km of the ocean (United Nations, 2017). Ocean observations in these regions are especially important for predicting and mitigating the impacts of climate change, managing ocean ecosystems, and providing warnings of impending short-term severe weather events like storms and flooding, as well as marine heatwaves, sea level rise, and drought (Taylor and Feng, 2022). Yet the challenges of collecting data in dynamic regions means that there is often less data coverage in these regions than in the open ocean (Van Vranken et al., 2020).
Shelf seas, boundary currents, frontal mixing zones, and areas with sea ice are therefore less monitored despite their outsize influence on maritime industries, aquatic ecosystems, and our climate.
Fisheries are a critical component of the blue economy: the fisheries and aquaculture sectors contributed USD 406 billion to global Gross Domestic Product (GDP) in 2020 and directly employed over 58 million people, with approximately 200 million people employed in the value-added sectors (FAO, 2022b). The contribution of fisheries to human livelihoods is significantly higher in least-developed countries (LDCs) and Small Island Developing states (SIDs) than in other countries (Tambutti and Gómez, 2020; FAO, 2022a; FAO, 2022b).
Globally, 4.1 million fishing vessels employ a wide variety of fishing gear including trawl nets, traps, hook and line, longline, gill nets, purse-seine, and dredges. Approximately 62% of these vessels are motorized and while there only ~64,000 vessels which exceed 24 meters in length, there are a large number of vessels in the 10-24 meter length range that utilize hydraulic and electrical equipment for fishing operations and sophisticated electronics for navigation and fish-finding (IMO, 2019; FAO, 2022b). Importantly, most fishing activity occurs in these shelf and coastal regions at depths of less than 1000 meters, potentially complementing existing ocean observation networks to provide data coverage in data sparse dynamic ocean regions (Van Vranken et al., 2020).
Beginning in the early 2000s, three key pioneering programs began collecting oceanographic data with fishing vessels: Fishery and Oceanography Observing System (FOOS), Environmental Monitoring on Lobster Traps and Large Trawlers (eMOLT), and Recopesca, founded respectively in Italy, the United States, and France (Falco et al., 2007; Manning and Pelletier, 2009; Leblond et al., 2010; Martinelli et al., 2012; Patti et al., 2016). Some of the original fishing vessels are still participating today. Data from these programs are currently being used in an array of ocean modeling applications from model validation to operational assimilation (Carpi et al., 2015; Aydoğdu et al., 2016; Lamouroux et al., 2016; Grémare et al., 2019; Ito et al., 2021; Wilkin et al., 2022). These pioneer programs established systems and workflows for instrumenting and maintaining fishing vessel instrumentation, setting the course for long lasting collaborations and instigating the development of fit-for-purpose instrument and vessel integration systems.
Now, an emerging global network is growing out of these initial programs, partnering with fishing fleets to employ their vessels and gear platforms to measure water column and bottom conditions. Data are collected during normal fishing operations and are being used for myriad of scientific, security and industrial applications. Oceanographic data can also be used to improve the sustainability of fisheries and the operational efficiency of fishing, thus providing a win-win for fishers. In many cases, this mutually beneficial data collection can encourage further cooperation between industry stakeholders and the scientific community. This paper aims to present an overview of the motivations, technologies, and foundational programs joining their efforts to build a global Fishing Vessel Ocean Observing Network (FVON).
Operational oceanography is one of the main fields that can benefit from this type of application; however, a host of additional interdisciplinary data uses and benefits arise from oceanographic data collected in parallel with fishing activities (Table 1).
Table 1 A selection of the key benefits of improved fishing vessel based in situ ocean observation, stakeholders who stand to benefit and the mechanism through which benefits are derived.
The inspiration for many of the pioneering programs instrumenting fishing gear was to collect the data necessary to enable the next generation of dynamic and ecosystem-based fisheries management strategies (Carpi et al., 2015; Russo et al., 2015). These advances link fisheries and fish resources distribution to ecosystem health and oceanographic conditions to increase the knowledge of environmental drivers of the spatio-temporal distribution of fish populations and thus enable better management decisions that increase the ecological and economic sustainability of fisheries (Aguzzi et al., 2022; Chiarini et al., 2022). Climate change is causing shifts in the distribution of marine species at approximately an order of magnitude faster than those on land (Poloczanska et al., 2013). As climate-driven changes push ocean ecosystems further from baseline conditions, the inclusion of environmental data in resource and ecosystem management will become ever more important (Hollowed et al., 2013; Schmidt et al., 2019). In order to account for environmental effects eMOLT bottom temperature data are currently used in the American lobster (Homarus americanus) stock assessment process (ASFMC, 2020). Lobsters and many other marine species are extremely sensitive to subtle temperature changes. The eMOLT bottom temperatures are used in a gridded bottom temperature time series which is then used as a covariate to account for catchability differences between ventless trap and bottom-trawl lobster surveys (ASFMC, 2020).
Improved ocean information is key for improving ocean sustainable development as well as reducing impacts of adverse events such as tropical cyclones, floods and marine heatwaves on the economy and human life in general. Predicting coastal flooding events from rising sea levels and increasingly frequent and intense storm surges depends mainly upon high-resolution meteorological and wave data collected from ships and buoys (OceanOPS and GOOS OCG, 2022). Currently, a lack of subsurface oceanographic data holds back forecasting of tropical cyclones which every year cause billions of dollars in damages and significant human casualties (Le Hénaff et al., 2021). Climate change is amplifying the intensity of marine heatwaves which often have devastating effects on marine ecosystems; subsurface measurements are key to improving our understanding of marine heatwaves (Viglione, 2021). And improving ocean predictions has applications beyond decreasing the severity of negative events, including providing opportunities for sustainable data-driven growth in sectors of the blue economy (Rayner et al., 2019).
Commercial fishing is one of the most hazardous occupations, largely due to maritime weather events (NIOSH, 2021). Fishing industry stakeholders therefore have a vested interest in contributing data to the oceanographic and forecasting communities. For example, In the United States, over 60% of fatalities in the commercial fishing industry are weather and wave related (NIOSH, 2021). Fishers have tremendous knowledge about the sea and have in most of the authors’ experiences supported initiatives where the data they collect is used to work to improve ocean modeling and weather forecasting, as these factors directly impact the safety and sustainability of their livelihoods.
Cooperation with fishing industry stakeholders is key to program success. In some regions there has been a historically divergent relationship between the research community and the commercial fishing industry. Collaborative fisheries ocean observation programs have encountered little resistance from industry in taking part in oceanographic data collection efforts when the data collection is focused on environmental factors, rather than catch which is more commercially sensitive data. In fact, cooperation with fishers has proven to be straightforward for many of the existing programs. Fishers are already accustomed to informally synthesizing different data streams to inform fishing tactics; therefore, oceanographic data is often seen as a tool to fish smarter. Custom ocean data-driven tools, i.e. deriving tools from the observations that allow fishers to improve operational efficiency and reduce negative ecological impacts, encourage participation. Cooperative research, starting with less contentious subjects like ocean observation, can help bridge the gaps between traditionally divergent groups leading to further collaborations and unanticipated knowledge exchanges. A case in point is the Shelf Research Fleet which is an ongoing collaboration between the Commercial Fisheries Research Foundation and Woods Hole Oceanographic Institute (Gawarkiewicz and Malek Mercer, 2019; Olsen et al., 2023).
Broadening beyond fishing, crowdsourcing ocean data from stakeholders across the spectrum from citizen scientists to maritime industries can unlock a tremendous untapped wealth of information, especially in coastal regions. Collaborative ocean observation empowers communities, democratizing ocean observation. Sharing data between communities and industries will yield compounding benefits for these user-observers and this blue planet.
On fine spatial scales, ocean features such as boundary currents, complex bathymetry, sea ice edges, and upwelling zones can complicate the operation of autonomous and drifting Lagrangian in situ observation platforms. However, these dynamic features form the basis for the oceans most productive ecosystems, subsequently coinciding with the world’s richest fishing grounds (Van Vranken et al., 2020). The very features that complicate autonomous data collection make these regions ideal for fishing vessel-based observations. Therefore, fishing vessel ocean observation presents a tremendous opportunity for future ocean observation networks, complementing the capabilities of established platforms, while collecting data for science, the blue economy, and society at large.
Many types of fishing gear are deployed fully or part of the way down through the water column, trap or actively catch fish, and are hauled back to the surface. Initial efforts relied on manual transfer of data from fishing gear-based sensors. Now, data loggers with “Internet of Things” (IoT) Bluetooth, WiFi, or other wireless connection capabilities go along for the ride collecting measurement profiles on the way down, at depth, and on the way back up (Figure 1). When the data logger surfaces with fishing gear, the IoT logger transmits its data automatically to a deck box or other vessel-based data collection hub (Leblond et al., 2010; Patti et al., 2016). Data are then transmitted from the deck box to a database via cellular data, Wi-Fi, or satellite internet depending on hub configuration, fishing region, and vessel systems. Once received into a database, data are quality controlled, standardized, and accessed by users including operational centers, such as Copernicus Marine Service (Le Traon et al., 2019; Penna et al., 2023). Vessels can also be instrumented with, or utilize, existing sea-surface or surface-meteorological instrumentation that is used in other ship of opportunity programs such as the Voluntary Observing Ship (VOS) scheme (Smith et al., 2019; Piecho-Santos et al., 2021). However, most fishing vessels have unique integrated subsurface data collection capabilities compared to other vessels of opportunity due to the profiling behavior of fishing gear during regular operations. Even vessels with no engine or electronics can be instrumented using solar-powered standalone deck hubs (examples are shown in Figure 3).
Figure 1 Because fishing gear descends through the water column, fishes and is then hauled back up, it can provide a profiling platform for sensors logging data. Loggers transmit data to a data hub on the vessel which then relays data packets to a cloud database, where it is subjected to quality control and standardization. Data can then be fed alongside other ocean observations to ocean analyses, models, and forecasts which are then transformed into useful applications for end users. End users include a wide range of science, government, and industry stakeholders. Critically, the fishers themselves are direct beneficiaries of data products, making them a combined user-observer. The example fishing type shown is trolling in which baited hooks are towed along at depth. Figure 2 (middle) shows a sensor attached to the “cannonball” weight used in trolling.
Figure 2 Left: The JFE Advantec CTD developed for the SFiN program in Japan and the data display Image source: JFE Advantech Co., Ltd. Middle: A ZebraTech Moana TD sensor deployed in the Southeast Alaska salmon troll fishery. Right: A Moana TD sensor in a lobster trap in the Gulf of Maine.
Since data packets are offloaded from the logger once it ascends up out of the water, often only the final upcast can be available in near real-time. Many mobile gear types have deployment times of hours or shorter, so there is less latency compared to fixed gears which can sit on the bottom for days before being hauled back up. Cellular data transmission is low-cost; however, data will only be sent when a vessel returns within cellular service close to shore. Fortunately, there are increasing options for satellite connectivity, and many vessels are getting satellite internet systems which deck hubs (Figure 3) can connect to.
Figure 3 Examples of data collection hub options on board commercial fishing vessels. LEFT: A Raspberry Pi based deck hub installation on a trawler which is the system used by eMOLT, and sometimes Ocean Data Network. RIGHT: A solar powered deck box designed by ZebraTech, Ltd (New Zealand) for the Moana Project and installed by Ocean Data Network on a crab pot fishing vessel.
A vessel-mounted data collection hub that always remains above the ocean and is less constrained by size or power considerations and enables straightforward engineering design of these systems when compared to fully autonomous or un-manned ocean observation platforms, which may instead have physical space limitations or may require more space for batteries. A challenge is the wide variety of fishing gears and fishing behavior instrumentation must be able to cope with. As an example, collecting accurate water column measurements on fishing gear can be a challenge due to the rapid descending and ascending speeds of certain types of fishing gear. Therefore, to obtain accurate water column profiles, the most appropriate combination of gear and sensor should be selected for example with respect to the response time (Martinelli et al., 2016). The most straightforward oceanographic variable to measure via fishing gear is temperature, and an increasing number of programs are adding conductivity or salinity. The sensors for these core physical Essential Ocean Variables (EOVs) are the most mature, capable, and affordable. Furthermore, instrumentation measuring core EOVs can generally be both robust and low power. However, there are programs measuring dissolved oxygen, chlorophyll fluorimetry, turbidity, current velocity and more. The costs of different instrumentation options deployed today on fishing gears can vary from $100 up to $10,000 USD. However, the costs of instrumentation are a small piece of the total costs of acquiring in situ ocean data which are traditionally dominated by platform, deployment, and servicing costs (Greenwood et al., 2014). The platform and deployment costs are at least partially mitigated by going along for the ride on fishing vessels and gears.
In the past five years there has been a proliferation of new programs, sparked by a range of factors including sensor development, knowledge of existing programs, and a recognized need for improved coastal and shelf data coverage. Growing interest in starting new programs has emerged in every large ocean basin (Figure 4). A selection of key programs from around the world are summarized in Table 2.
Table 2 A selection of the programs engaging in collaborative ocean observation with the fishing industry using vessels or gears as platforms of instrumentation.
The existing and pilot programs discussed are by no means an exhaustive list of all collaborative ocean observation efforts with fisheries (Figure 4). There are several notable programs, such as the Shelf Research Fleet (USA), which collaboratively collect oceanographic data with Commercial Fisheries Research Foundation (CFRF) fishers, but the sensors are not deployed using the fishing gear (Gawarkiewicz and Malek Mercer, 2019; Olsen et al., 2023).
Figure 4 The locations of established and pilot projects and networks collaborating with fishers to collect oceanographic data. Established programs are shown with named larger blue points, and pilots are shown in red. The scale and extent of programs varies substantially from a single vessel to hundreds of vessels. While this map shows the locations of all programs and pilots known to the authors at the time of publication of this work, it is possible that other programs exist.
Programs which are represented by authors of this publication are described in more detail below:
Beginning in 2003, a group of commercial fishing vessels operating in the Adriatic Sea, within the Mediterranean basin, were equipped with semi-autonomous systems for collecting useful oceanographic and biological data termed the Fishery Observing System FOS (Falco et al., 2007; Carpi et al., 2015; Aydoğdu et al., 2016; Sparnocchia et al., 2016; Lucchetti et al., 2018). In 2012, a modular Fishery and Oceanography Observing Systems (FOOS) was developed, capable of collecting and sending a greater number of more precise parameters in near real-time to a server and sending back useful information to vessels (Martinelli et al., 2016; Patti et al., 2016). AdriFOOS is an infrastructure composed of several FOOS systems installed on commercial fishing vessels operating in the Adriatic Sea and a data center located in the Ancona office of the Institute of Marine Biological Resources and Biotechnology of the National Research Council of Italy (Penna et al., 2023). The server receives data collected during the fishing phases along the water column and near the bottom (e.g. temperature, salinity, etc.), GPS track, catches per haul, and meteorological information. Data are then archived in a multifunctional database that allows validation, analysis processes and provision of data to European interoperable platforms (Penna et al., 2020; Penna et al., 2023). Functionality demonstrations were carried out during various European projects (Gaughan et al., 2015; Sparnocchia et al., 2017) and led to the definition of internationally recognized best practices for instrumentation pre-deployment routines (Möller et al., 2019). Adriatic fishing vessel data assimilation into ocean models has also been investigated (Aydoğdu et al., 2016; Grémare et al., 2019; Puillat, 2019) In addition, AdriFOOS is a testing and demonstration program for newly developed oceanographic, including biogeochemical, sensors designed for use on fishing gear (Pieri et al., 2021).
Environmental Monitoring On Lobster Traps and Large Trawlers (eMOLT) is a collaboration between NOAA’s Northeast Fisheries Science Center, the Gulf of Maine Lobster Foundation, and fishers in the Gulf of Maine and Mid-Atlantic Bight (Manning and Pelletier, 2009). The program started in 2001 by putting temperature loggers on lobster traps. Instrumentation and telemetry has been updated over the years and now delivers bottom temperature from approximately 40 vessels in near real-time. Bottom temperature data have been used for model validation for an assortment of local ocean models, and are assimilated into the Doppio ROMS model forecast (Wilkin et al., 2022). In addition, these bottom temperature data are used in the American lobster (Homarus americanus) stock assessment process to compensate for temperature-dependent differences in survey method catchability (ASFMC, 2020). The fishing gear-mounted sensor collaborations have expanded into further collaborations, an example of which is that hundreds of student-built drifters have been deployed by eMOLT vessels in order to resolve the surface current pathways on the US Northeast Shelf (Manning et al., 2009).
Participants in the eMOLT program directly use their own bottom temperatures to avoid unintended bycatch. Reducing regulated bycatch is beneficial both for the broader ecosystem health but also potentially allows fishers to generate more profit as they can catch more of other species before ‘choking’ out of fishing by filling their allocated quota of a ‘choke’ species.
In 2022 Australia’s Integrated Marine observing System (IMOS) and Fisheries Research Development Corporation (FRDC) co-invested in a pilot program led by the University of New South Wales and Fishwell, a private consultancy. The program is designed to pilot Moana TD sensors on a range of fishing gear types and methods across several key fisheries. Approximately 20 vessels are participating in the first phase of the program. While the technology has now advanced to a reasonable point, the goal of the Australian pilot now is to advance the development of the data pathways. Two key data pathways are identified to enhance use and uptake of the data firstly, the real time pathway to international data repositories such as the Global Telecommunications System. This will aid rapid data uptake into operational ocean forecasts. Secondly the delayed mode data pathway to deliver quality controlled C.F. complaint data formatted to the IMOS open access data portal led by the Australian Ocean Data Network (AODN). Data assimilation into pre-operational ocean models is also under development through Australian Research Council funding. The program has started with routine temperature profile measurements with the goal of broad scale high quality coverage of just a few variables with data delivered to the cloud in near real time.
The Moana project, led by the Meteorological Service of New Zealand, is the first nationwide fishing vessel-based operational observing system.
The Te Tiro Moana (te reo Māori for “Eyes on the Ocean”) theme of the Moana Project focuses on improving the availability of near real-time, subsurface observations within New Zealand’s maritime domain. The Mangōpare sensor program was instigated as an answer to the need for coordinated routine ocean observing within NZ. This goal required developing a temperature and depth instrument specifically designed for deployment from commercial fishing vessels. Working closely with the fishing sector and technology partner Zebra-Tech Ltd, the program developed a sensor-logger combination (Moana TD, Figure 2), deck box (Figure 3) and data pathway that is robust and fully automatic, without human intervention from the vessel crew at collection, nor data scientists at the data offload and processing phase. However, data can be returned directly to the vessel/company/fisheries manager that obtained them and to oceanographic databases for further analysis and model ingestion and/or validation. Ocean models automatically assimilate these observations to improve forecasts.
The program has seen remarkable uptake with more than 200 vessels deploying a total of 300 sensors throughout New Zealand’s Exclusive Economic Zone within the first two years of operation. Meaning that more than 1/3 of all active fishing vessels in New Zealand are instrumented with sensor hardware. Participating vessels consistently provide measurements in near real-time for assimilation into the Moana Project’s hydrodynamic modeling suite. Seafood sector engagement is high, providing an opportunity to expand the program further with additional funding. A deployment of this scale is unprecedented and is the first realization of the theoretical potential shelf and coastal data collection shown by Van Vranken et al. (2020) (Figure 5).
Figure 5 LEFT: The monthly average number of Argo temperature and salinity profiles (blue) for the one-year period from 1 January 2022 through 31 December 2022 within the Moana Project’s hydrodynamic ocean model domain. RIGHT: The same as the left panel, overlayed with the monthly average number of Mangōpare temperature sensor deployments (orange). Note: Data coverage is gridded to preserve anonymity of fishing positions for participating vessels.
Near real-time observations are now available throughout the horizontal domain of the Moana Project modeling suite by utilizing both Argo and Mangōpare measurements (Figure 5), alongside other available observations. Mangōpare coverage has increased through the prototype, testing, and rollout phases of the program (Figure 6), complementing existing near real-time datasets such as Argo and cost-effectively filling in the coastal “gap” apparent in the map on the left of Figure 5. The successes of the Moana project show that an observation network at scale, engaging a significant proportion of a country’s entire fishing fleet, is possible and can be well received. Furthermore, this network has achieved a step change in reversing the scarcity of sub-surface (mostly coastal) observations within New Zealand’s Exclusive Economic Zone (O’Callaghan et al., 2019).
Figure 6 The monthly evolution of both Argo (blue) and vessel deployments of Mangōpare sensors (orange) throughout the duration of the Moana Project within the Moana Project’s New Zealand operational model domain (Figure 5). A deployment is defined as a single Argo profile or a single fishing event measured by a Mangōpare sensor. Note that the relationship between Argo and Mangōpare is domain- dependent; and the domain shown here includes coastal and shelf areas suited to fishing vessel-based sensor deployment.
The Portuguese ocean observing system (OBSERVA.PT) is the main ocean observation program at the Portuguese Institute for the Sea and the Atmosphere (IPMA), where a core objective is the development of methodologies for automatic observations, especially on board national vessels of opportunity (e.g., cargo, fishing and cruise vessels). The OBSERVA.PT program aims to obtain information necessary to support the management of marine ecosystems and maritime meteorology in the northeastern Atlantic Ocean. The program component OBSERVA : FISH (Autonomous Observation Systems aboard Fishing Vessels to Support the Management of Marine Ecosystems) is funded by Portugal-Lisboa-Algarve2020 (Piecho-Santos et al., 2021; Rosa et al., 2021). A novel compact ferrybox developed by Portuguese startup UnderSEE is installed in a cod trawler (MV Coimbra). MV Coimbra fishes in the North Atlantic and Barents Sea and data are displayed on the EMODnet portal. Measured variables are: Temperature, pH, Dissolved Oxygen, Conductivity/Salinity, Turbidity, and Chlorophyll-a. This autonomous integrated ocean observing system on board fishing vessels will be a component of an integrated complete observing system with the European Multidisciplinary Seafloor and water column Observatory (EMSO) under EMSO.PT (Carapuço et al., 2022).
Berring Data Collective (BDC) and Ocean Data Network (ODN) are twin startups based in Denmark and the United States, respectively. The founding of these startups coincided with communication between other programs, knowledge sharing, technology transfer, and more. BDC and ODN were founded on the philosophy that a private company could support the science and scientific programs in an agile manner to bridge gaps between science and industry. BDC/ODN provide data management services for eMOLT and other programs. In addition, BDC/ODN provide data collection as a service by instrumenting vessels to meet specific spatial and temporal data needs. For example, instrumenting vessels which operate at a shelf-break or frontal mixing region with complex sub-mesoscale features that an ocean model has a difficult time resolving.
BDC and ODN are facilitating pilot projects in several regions around the world with local partners, advising on the technical implementation, and then providing turn-key data management. For example, most of pilot programs shown as red points on Figure 4 are BDC/ODN pilots with local partners.
Kyushu University leads a program initiated in the Tsushima/Korea Strait named Smart Fisheries Network (SFiN) with information and communication technology originally funded by the Japan Fisheries Agency. This program is continuing to expand and currently engages over 200 small scale vessels using compact Conductivity Temperature Depth (CTD) instruments from JFE Advantech and/or onboard Acoustic Doppler Current Profilers (ADCPs) mostly by FURUNO Electric (Ito et al., 2021). The CTD and ADCP data are currently assimilated into coastal ocean models through approximate Kalman filtering (Hirose, 2022). Improved ocean prediction and visualizations are specifically designed for fishing and are delivered via smartphone application. This program is popular with participating fishers, enabling some of them to operate both more safely and efficiently leading to a reduction of up to 15% of operating time and expenses (IDEA Co., 2021).
Collaboration between different programs has been happening longest in Europe through a series of European Union projects. Communication between the American and European programs was initiated in 2018. Then at the OceanObs’19 conference the programs in the Pacific connected with the informal group. FVON is the formalization of this emerging global network of fishing vessel ocean observation programs. The founding members of FVON are included as the authors of this manuscript and form the initial FVON steering committee. To maximize the potential of fishing vessels as platforms of opportunity, the steering group represents a range of stakeholders facilitating international engagement and development of the network. The steering group will grow and diversify as the goals and scope of this community evolve. In addition, FVON plans to expand external partnerships for facilitating wider integrations and collaborations. These efforts will provide opportunities to coordinate programs, share expertise and lessons learned, develop observing and data standards, encourage open data policies which work for all stakeholders, and connect regional observing programs. To frame the advancement of these goals, the following initial Terms of Reference for FVON are established:
1. Communicate the opportunity of fishing vessel based ocean observations with both outreach activities and scientific publications;
2. Define and update the structure, scope, and activities of the steering group starting with periodic online meetings, and moving towards hosting in-person workshops;
3. Identify stakeholders and end user requirements towards a scalable framework which is mutually beneficial for both data users and data fishers;
4. Continue the documentation of Best Practices and standardized Findability, Accessibility, Interoperability, and Reuse (FAIR) data management;
5. Expand the capabilities of fishing vessel-based ocean observation to an increasing number of Essential Ocean Variables and Essential Climate Variables;
6. Develop a strategic plan for a globally scalable network of fishing vessel and gear integrated ocean observation to contribute towards complementing components of the Global Ocean Observing System.
To achieve the goals outlined in section 3.2, the steering committee faces several immediate challenges: designing best practices for sustained quality assured sensor deployments, efficient quality control and management of ocean data collected on fishing vessels; real time data dissemination for operational outcomes, expanding current tools and approaches to encompass more types of ocean variables to provide benefits to more types of end-users, and ensuring complementarity with existing data collection systems by integrating FVON into the Global Ocean Observing System (GOOS).
FVON shall work to establish and document best practices including Data Management, instrumentation pre and post deployments routines, and the integration of data collection systems with the diversity of fishing vessels around the world. The diversity of fishing vessels and different fishing gears and techniques adds complexity to establishing best practices. Data management practices need to simultaneously work with the diversity of unique local fishing practices and facilitate interoperability so that data from a multitude of disparate sources can all be used seamlessly across basin and global scale analyses.
Data and meta-data standards are key for facilitating data usage and interoperability with respective use cases. Due to the interdisciplinary nature and use cases of integrated fishing vessel data, FVON shall strive to comply with a wide array of data standards for the multitude of use cases, and to meet the needs of relevant stakeholders. The basis for FVON data standards are the FAIR (Findable, Accessible, Interoperable, and Reusable) principles and Climate Forecasting (CF) conventions along with CARE (Collective Benefit, Authority to Control, Responsibility, and Ethics), when applicable (Wilkinson et al., 2016; Tanhua et al., 2019; Carroll et al., 2020).
Real-time QC routines have been developed in collaboration between ODN/BDC and the Moana Project. These QC routines are based upon the IOOS Quality Assurance of Real Time Ocean Data (QARTOD) and Argo routines, with additional fishing specific routines (Bushnell et al., 2019; Wong et al., 2022). Delayed mode QC are not currently implemented, but this is under development in collaboration with IMOS Australia.
Penna et al. (2023) describe how the pressure(depth)/temperature profiles dataset provided by the AdriFOOS infrastructure were subject to QA and QC according to European SeaDataNet standards (Martinelli et al., 2016; Möller et al., 2019)
Operational data delivery has been the initial focus of many programs, and progress is underway to deliver via global operational data pathways such as the World Meteorological Organization Global Telecommunication System (GTS). Delayed mode data processing for archiving is only done with AdriFOOS data, but will be an important development for increasing fishing vessel data’s utility for reanalysis and climate time scale applications. In addition, connecting to the proper repositories such as the NOAA National Center for Environmental Information, the World Ocean Database, the European Marine Observation and Data Network (EMODnet) or the European Union’s Earth observation program Copernicus will help reach data users with non-real-time data use cases.
The multitude of potential users presents opportunities but especially challenges from a data management perspective. As such, programs aim to provide observations to a range of users via bespoke tools tailored to differing needs. Key factors include: meta-data classifications to increase data utility, especially for fishing industry stakeholders so that oceanographic data can be correlated with confidential on vessel data (Buck et al., 2019). In addition, data pathways like the GTS are not the ideal for higher resolution coastal modeling so having data delivery mechanisms which are less standardized can have added value for finer scale models.
There are already a wide array of different instruments deployed on fishing vessels of differing accuracies and precision (Manning and Pelletier, 2009; Martinelli et al., 2016). Rather than coalescing to one or few instruments, FVON aims to encourage innovation both by instrument/logger suppliers and data users. To address the diversity of instrumentation, FVON aims to report sensor accuracy/precision specifications in the metadata to ensure appropriate QC and creation of derived products.
Much of the initial focus of the pioneering programs has been on subsurface physical data and the capabilities of fishing vessels as real time data collection platforms. As sensor technologies continue to improve the platforms are well placed to expand to measure other subsurface, sea surface, and surface meteorological variables. For example, there has been significant progress involving fishing vessels based European programs through the FP7 Next generation, Cost-effective, Compact, Multifunctional Web Enabled Ocean Sensor Systems Empowering Marine, Maritime and Fisheries Management project (Sparnocchia et al., 2016), in which a first generation of depth/temperature/oxygen and depth/temperature/chlorophyll-a sensors designed for fishing gears were developed and trialed. These sensor systems are under continued development; for example, through the H2020 New Approach to Underwater Technologies for Innovative, Low-cost Ocean obServation (NAUTILOS) project (Pieri et al., 2021). There is an operational program which is a collaboration lead by Oregon State University (https://fishresearch.net/) measuring bottom dissolved oxygen from instruments deployed in crab traps (Stoltz et al., 2021). In January 2023, an Ocean Carbon & Biogeochemistry scoping workshop was held at Woods Hole Oceanographic Institution focused on pH and other biogeochemical observation with fishers (https://www.us-ocb.org/coastal-bgc-obs-network-with-fishing-community/), in the context of which there was alignment between fishing industry and scientific stakeholders and a strong interest in expanding collaborative research capabilities.
In addition, fishing catch data is often already recorded which is useful both for fishing industry and fisheries science applications (Falco et al., 2007; Leblond et al., 2010; Carpi et al., 2015; Mion et al., 2015; Patti et al., 2016; Steins et al., 2022). The multi-domain co-located fishing vessel data collection opportunities would compound many of the benefits to the stakeholders discussed, such as met-ocean modelers and fisheries science. For example, co-located physical oceanographic and surface meteorological data streams can be challenging to collect with many platforms, but can enable improvements for coupled ocean-atmosphere models and resulting science and forecasting applications.
The collaborative nature of this approach to ocean observation leads to extensive dialogue on what future parameters would be most interesting to industry stakeholders. FVON shall synthesize desired data capability increases, and in turn comprise a larger cohesive market so that instrumentation makers can benefit from economies of scale.
A primary goal of FVON is to elevate fishing vessels to be an internationally accepted tool for ocean observation alongside other platforms and networks at the GOOS level. Because of the unique data collection capabilities, the complementarity with existing observation networks, and the applicability around the world, this is an approach to ocean observation which needs to be standardized and coordinated so that resulting data can be interoperable and impactful worldwide. It is FVON’s goal to advance fishing vessel based ocean observation not as a stand-alone platform, but as a component in the expanding toolbox of observing platforms. Complementing existing ocean observation networks and building towards harmonized observing technologies will have compounding benefits beyond the contribution of any single network (ETOOFS, 2022). FVON aims to accelerate and formalize the ongoing dialogues with ocean observing systems, modelers and forecasters, as well as other networks of complimentary observing approaches. Feedback from these stakeholders was a key driver in the formation of FVON, and in the future the group could be expanded with increased representation from all relevant stakeholders. Collaboration at different scales between different actors: science and industry, observing networks and technologies, as well as between nations is a key step towards unlocking the ocean observation and monitoring needed for research, forecasting, and sustainable growth in the blue economy.
Pioneering programs have demonstrated that fishing vessel-based ocean observing networks can fill key data gaps, have significant buy-in from fisheries, and can be deployed at scale. The FVON steering group’s vision is to enable a paradigm shift for ocean observation by dramatically increasing met-ocean observations around the world. The programs showcased here, particularly the Moana project, demonstrate ocean data collection at scales that have previously been unattainable due to cost and technical limitations. This cost-effective integration could contribute to solving some of the systemic issues stemming from inaccessibility of ocean data in developing nations, making ocean monitoring more equitable and accessible around the world.
Inclusive ocean observation tools will benefit fishing industry stakeholders by empowering them to contribute to climate science, sustainable fisheries management, and research on their own terms. All stakeholders should be given the opportunity to play a role in the processes and dialogues that will lead to solutions in our oceans. As this novel approach to ocean data collection evolves so will the data tools developed for industry and broader ocean community use. Over time, continued collaboration between the fishing industry and the scientific community will help to build trust and lead to further cooperative research.
Informed consent was obtained from the individual(s) for the publication of any identifiable images or data included in this article.
CV, PG, NH, JM, MM, AP-S, JS, MR, HM, and JJ contributed to the formation of the manuscript concept. CC, CV, MP, JC, HM, and JJ organized the writing efforts. All authors contributed writing and technical expertise. All authors contributed to the article and approved the submitted version.
This work was conducted with support from the Environmental Defense Fund.
We owe a great deal of gratitude to Lisa Max and Malene Felsing for their support during the writing of this manuscript, and to Carly Rose Taft for the fantastic graphics (Figures 1, 2). Thank you to both reviewers for their constructive feedback and guidance. However, none of this would have been possible without the support, collaboration, and patience of the hundreds of fishing captains, crew members, and fishing companies who have made this work possible.
CV and JC are employed by the company Ocean Data Network.
The remaining authors declare that the research was conducted in the absence of any commercial or financial relationships that could be construed as a potential conflict of interest.
All claims expressed in this article are solely those of the authors and do not necessarily represent those of their affiliated organizations, or those of the publisher, the editors and the reviewers. Any product that may be evaluated in this article, or claim that may be made by its manufacturer, is not guaranteed or endorsed by the publisher.
Aguzzi J., Chatzievangelou D., Robinson N. J., Bahamon N., Berry A., Carreras M., et al. (2022). Advancing fishery-independent stock assessments for the Norway lobster (Nephrops norvegicus) with new monitoring technologies. Front. Mar. Sci. 9. doi: 10.3389/FMARS.2022.969071/BIBTEX
ASFMC (2020) Atlantic States marine fisheries commission: American lobster stock assessment peer review report (Woods Hole). Available at: https://asmfc.org/uploads/file/63d417a12020AmLobsterBenchmarkStockAssmt_PeerReviewReport_reduced.pdf (Accessed May 16, 2023).
Aydoğdu A., Pinardi N., Pistoia J., Martinelli M., Belardinelli A., Sparnocchia S. (2016). Assimilation experiments for the fishery observing system in the Adriatic Sea. J. Mar. Syst. 162, 126–136. doi: 10.1016/j.jmarsys.2016.03.002
Buck J. J. H., Bainbridge S. J., Burger E. F., Kraberg A. C., Casari M., Casey K. S., et al. (2019). Ocean data product integration through innovation-the next level of data interoperability. Front. Mar. Sci. 6. doi: 10.3389/fmars.2019.00032
Bushnell M., Waldmann C., Seitz S., Buckley E., Tamburri M., Hermes J., et al. (2019). Quality assurance of oceanographic observations: standards and guidance adopted by an international partnership. Front. Mar. Sci. 6. doi: 10.3389/fmars.2019.00706
Carapuço M. M., Silveira T. M., Stroynowski Z., Miranda J. M. (2022). Portuguese European Multidisciplinary seafloor and water column observatory initiative. Front. Mar. Sci. 9. doi: 10.3389/FMARS.2022.849150/BIBTEX
Carpi P., Martinelli M., Belardinelli A., Russo A., Arneri E., Coluccelli A., et al. (2015). Coupling an oceanographic model to a fishery observing system through mixed models: the importance of fronts for anchovy in the Adriatic Sea. Fish Oceanogr 24, 521–532. doi: 10.1111/fog.12126
Carroll S. R., Garba I., Figueroa-Rodríguez O. L., Holbrook J., Lovett R., Materechera S., et al. (2020). The CARE principles for indigenous data governance. Data Sci. J. 19. doi: 10.5334/dsj-2020-043
Chiarini M., Guicciardi S., Angelini S., Tuck I. D., Grilli F., Penna P., et al. (2022). Accounting for environmental and fishery management factors when standardizing CPUE data from a scientific survey: a case study for nephrops norvegicus in the pomo pits area (Central Adriatic Sea). PLoS One 17, e0270703. doi: 10.1371/journal.pone.0270703
Choudhary P., Venkata G. ,. S., Khade M., Savant S., Musale A., G R. K. K., et al. (2021). Empowering blue economy: from underrated ecosystem to sustainable industry. J. Environ. Manage 291, 112697. doi: 10.1016/J.JENVMAN.2021.112697
Domingues R., Kuwano-Yoshida A., Chardon-Maldonado P., Todd R. E., Halliwell G., Kim H.-S., et al. (2019). Ocean observations in support of studies and forecasts of tropical and extratropical cyclones. Front. Mar. Sci. 6. doi: 10.3389/fmars.2019.00446
Expert Team on Operational Ocean Forecasting Systems (ETOOFS) (2022) Guide on implementing operational ocean monitoring and forecasting systems. Available at: https://www.goosocean.org/index.php?option=com_oe&task=viewDocumentRecord&docID=30656 (Accessed February 26, 2023).
Falco P., Belardinelli A., Santojanni A., Cingolani N., Russo A., Arneri E. (2007). An observing system for the collection of fishery and oceanographic data. Ocean Sci. 3, 189–203. doi: 10.5194/OS-3-189-2007
FAO. (2022a). Sustainable development goal 14.7.1 sustainable fisheries as a percentage of GDP in small island developing states, least developed countries and all countries. Available at: https://www.fao.org/sustainable-development-goals/indicators/1471/en/ (Accessed February 22, 2023).
FAO. (2022b). The state of world fisheries and aquaculture 2022 (Rome, Italy: FAO). doi: 10.4060/CC0461EN
Gaughan P., Nolan G., Puillat I., Farcy P., Mowlem M., Seppala J., et al. (2015). Jerico WP10 deliverable 10.4 - report on potential new sensors (Fishing vessels and voluntary opportunity ships). (Brest France: JERICO).
Gawarkiewicz G., Malek Mercer A. (2019). Partnering with fishing fleets to monitor ocean conditions. Ann. Rev. Mar. Sci. 11, 391–411. doi: 10.1146/annurev-marine-010318-095201
Greenwood N., Sivyer D., Sparnocchia S., Howarth J., Hydes D., Karlson B., et al. (2014). “D4.5 –running costs of coastal observatories,” in JERICO towards a joint European research infrastructure network for coastal observatories. (Brest France: JERICO). Available at: http://www.jerico-ri.eu/download/filebase/jerico_fp7/deliverables/D4.5_Runningcosts_v1.2_withgliderannexe.pdf.
Grémare A., Puillat I., Karlson B., Artigas F., Nizzetto L., Rubio A., et al. (2019). D4.5: JRAP synthesis and contribution to the strategy for the future. (Brest France: JERICO).
Hirose N. (2022). “Coastal ocean data assimilations with fishing vessels,” in Joint workshop of the OS eval TT and CP TT and SynObs kick off (Tsukuba, Japan: OceanPredict).
Hollowed A. B., Barange M., Beamish R. J., Brander K., Cochrane K., Drinkwater K., et al. (2013). Projected impacts of climate change on marine fish and fisheries. ICES J. Mar. Sci. 70, 1023–1037. doi: 10.1093/icesjms/fst081
IDEA Co., Ltd. (2021). FY2021 smart fisheries promotion project (Ishikawa Prefecture). Available at: https:///dreams-d.riam.kyushu-u.ac.jp/report2021abstract.pdf.
IMO. (2019). Enhancing fishing vessel safety to save lives. Available at: https://www.imo.org/en/MediaCentre/HotTopics/Pages/Fishing.aspx (Accessed February 22, 2023).
Ito T., Nagamoto A., Nabuo T., Nobuteru K., Kokubo T., Takikawa T., et al. (2021). Construction of CTD hydrographic observation system by fishermen in northwest Kyushu, Japan. Bull. Jpn. Soc Fish. Oceanogr. 85, 197–203. doi: 10.34423/jsfo.85.4_197
Lago M., Spinrad R. (2022) Earth day spotlight: our ocean, our blue economy (U.S. Department of Commerce). Available at: https://www.commerce.gov/news/blog/2022/04/earth-day-spotlight-our-ocean-our-blue-economy (Accessed February 22, 2023).
Lamouroux J., Charria G., De Mey P., Raynaud S., Heyraud C., Craneguy P., et al. (2016). Objective assessment of the contribution of the RECOPESCA network to the monitoring of 3D coastal ocean variables in the bay of Biscay and the English channel. Ocean Dyn 66, 567–588. doi: 10.1007/s10236-016-0938-y
Leblond E., Lazure P., Laurans M., Rioual C., Woerther P., Quemener L., et al. (2010). The RECOPESCA project: a new example of participative approach to collect fisheries and in situ environmental data. Joint Coriolis-Mercator Ocean Q. Newslett. 37, 40–55. Available at: https://lsc-pagepro.mydigitalpublication.com/publication/?m=60787&i=693703&p=14&ver=html5 https://www.researchgate.net/publication/48382978_The_RECOPESCA_project_A_new_example_of_participative_approach_to_collect_fisheries_and_in_situ_environmental_data.
Le Hénaff M., Domingues R., Halliwell G., Zhang J. A., Kim H., Aristizabal M., et al. (2021). The role of the gulf of Mexico ocean conditions in the intensification of hurricane Michael, (2018). J. Geophys Res. Oceans 126. doi: 10.1029/2020JC016969
Le Traon P. Y., Reppucci A., Fanjul E. A., Aouf L., Behrens A., Belmonte M., et al. (2019). From observation to information and users: the Copernicus marine service perspective. Front. Mar. Sci. 6. doi: 10.3389/FMARS.2019.00234/BIBTEX
Lombard A. T., Ban N. C., Smith J. L., Lester S. E., Sink K. J., Wood S. A., et al. (2019). Practical approaches and advances in spatial tools to achieve multi-objective marine spatial planning. Front. Mar. Sci. 6. doi: 10.3389/FMARS.2019.00166/BIBTEX
Lucchetti A., Belardinelli A., D’Andrea L., Marčeta B., Martinelli M., Russo T., et al. (2018). Small pelagic purse seines in the Adriatic Sea: a spatial analysis and technical overview in relation to Mediterranean regulation provisions. Mar. Policy 98, 104–114. doi: 10.1016/j.marpol.2018.09.031
Manning J., Pelletier E. (2009). Environmental monitors on lobster traps (eMOLT): long-term observations of new england’s bottom-water temperatures. J. Operational Oceanogr. 2, 25–33. doi: 10.1080/1755876X.2009.11020106
Manning J. P., McGillicuddy D. J., Pettigrew N., Churchill J. H., Incze L. (2009). Drifter Observations of Maine Coastal Current Drift. Continental Shelf Res 29 (7), 835–845. doi: 10.1016/j.csr.2008.12.008
Marcelli M., Piermattei V., Gerin R., Brunetti F., Pietrosemoli E., Addo S., et al. (2021). Toward the widespread application of low-cost technologies in coastal ocean observing (Internet of things for the ocean). Mediterr Mar. Sci. 22, 255–269. doi: 10.12681/MMS.25060
Martinelli M., Falco P., Belardinelli A., Cingolani N., Arneri E., Russo A., et al. (2012). Fishery observing system (FOS): a tool for collecting oceanographic data and data on fish in the Adriatic. In: Operational Oceanography in Italy toward a sustainable management of the sea. I Quaderni di Arpa: 277–284.
Martinelli M., Guicciardi S., Penna P., Belardinelli A., Croci C., Domenichetti F., et al. (2016). Evaluation of the oceanographic measurement accuracy of different commercial sensors to be used on fishing gears. Ocean Eng. 111, 22–33. doi: 10.1016/J.OCEANENG.2015.10.037
Mateus M., Canelas R., Pinto L., Vaz N. (2020). When tragedy strikes: potential contributions from ocean observation to search and rescue operations after drowning accidents. Front. Mar. Sci. 7. doi: 10.3389/fmars.2020.00055
Mion M., Piras C., Fortibuoni T., Celić I., Franceschini G., Giovanardi O., et al. (2015). Collection and validation of self-sampled e-logbook data in a Mediterranean demersal trawl fishery. Reg. Stud. Mar. Sci. 2, 76–86. doi: 10.1016/J.RSMA.2015.08.009
Möller K. O., Petersen W., Nair R., Greenwood N., Read C., Laes-Huon A., et al. (2019). “D2.5 report on best practice in the utilization of sensors used for measuring nutrients, biology related optical properties, variables of the marine carbonate system, and for coa. in WP 2 (Harmonization of technologies and methodologies - technical strategy),” in Joint European research infrastructure network for coastal observatory – novel European eXpertise for coastal observaTories (Brest France: JERICO), 43–50. Available at: http://www.jerico-ri.eu/download/jerico-next-deliverables/JERICO_NEXT_Deliverable_2.5_Version1.0.pdf.
NIOSH. (2021). Commercial fishing safety: national overview (Washington DC, USA: United States Centers for Disease Control and Prevention, The National Institute for Occupational Safety and Health (NIOSH).
O’Callaghan J., Stevens C., Roughan M., Cornelisen C., Sutton P., Garrett S., et al. (2019). Developing an integrated ocean observing system for new Zealand. Front. Mar. Sci. 6. doi: 10.3389/fmars.2019.00143
OceanOPS, GOOS Observations Coordination Group. (2022). Ocean observing system report card 2022. Available at: https://www.ocean-ops.org/reportcard2022/ (Accessed February 22, 2023).
Olsen N. A., Bahr F., Bethoney N. D., Mercer A. M., Gawarkiewicz G. (2023). Integrating fishers’ knowledge with oceanographic observations to understand changing ocean conditions in the Northeast United States. Front. Mar. Sci. 10. doi: 10.3389/fmars.2023.1144178
Patti B., Martinelli M., Aronica S., Belardinelli A., Penna P., Bonanno A., et al. (2016). The fishery and oceanography observing system (FOOS): a tool for oceanography and fisheries science. J. Operational Oceanogr. 9, S99–S118. doi: 10.1080/1755876X.2015.1120961
Penna P., Belardinelli A., Croci S. C., Domenichetti F., Martinelli M. (2020). AdriFOOS depth / temperature profiles dataset 2012-2020. Sea Sci. Open Data Edition (SEANOE) 1–6. doi: 10.17882/73008
Penna P., Domenichetti F., Belardinelli A., Martinelli M. (2023). Dataset of Depth/Temperature profiles obtained in the period 2012-2020 using commercial fishing vessels of the AdriFOOS fleet in the Adriatic Sea (Mediterranean Sea) (In review). Earth Syst. Sci. Data 2023, 1–26. doi: 10.5194/essd-2022-458
Piecho-Santos A. M. P., Hinostroza M. A., Rosa T., Guedes Soares C. (2021). “Autonomous observing systems in fishing vessels,” in Developments in maritime technology and engineering (London: CRC Press), 805–808. doi: 10.1201/9781003216599-87
Pieri G., Ntoumas M., Martinelli M., Chatzinikolaou E., Martins F., Novellino A., et al. (2021). New technology improves our understanding of changes in the marine environment. In: Fernandez V., Lara-Lopez A., Eparkhina D., Cocquempot L., Lochet C., Lips I. (eds.). Advances in operational oceanography: expanding Europe's ocean observing and forecasting capacity. Proceedings of the 9th EuroGOOS International Conference. 3 – 5 May 2021, Online Event 2021, EuroGOOS. Brussels, Belgium. 574 pp. doi: 10.13155/83160
Poloczanska E. S., Brown C. J., Sydeman W. J., Kiessling W., Schoeman D. S., Moore P. J., et al. (2013). Global imprint of climate change on marine life. Nat. Clim Chang 3, 919–925. doi: 10.1038/nclimate1958
Pörtner H.-O., Roberts D. C., Alegría A., Nicolai M., Okem A., Petzold J., et al. (2022). The ocean and cryosphere in a changing climate special report (Geneva: Intergovernmental Panel on Climate Change). doi: 10.1017/9781009157964
Rayner R., Jolly C., Gouldman C. (2019). Ocean observing and the blue economy. Front. Mar. Sci. 6. doi: 10.3389/fmars.2019.00330
Rosa T. L., Piecho-Santos A. M., Vettor R., Guedes Soares C. (2021). Review and prospects for autonomous observing systems in vessels of opportunity. J. Mar. Sci. Eng. 9, 366. doi: 10.3390/jmse9040366
Russo T., D’Andrea L., Parisi A., Martinelli M., Belardinelli A., Boccoli F., et al. (2016). Assessing the fishing footprint using data integrated from different tracking devices: issues and opportunities. Ecol. Indic 69, 818–827. doi: 10.1016/j.ecolind.2016.04.043
Russo T., Pulcinella J., Parisi A., Martinelli M., Belardinelli A., Santojanni A., et al. (2015). Modelling the strategy of mid-water trawlers targeting small pelagic fish in the Adriatic Sea and its drivers. Ecol. Modell 300, 102–113. doi: 10.1016/j.ecolmodel.2014.12.001
Santoro F., Santin S., Scowcroft G., Fauville G., Tuddenham P. (2018) Ocean literacy for all: a toolkit (Venice: IOC/UNESCO & UNESCO Venice Office). Available at: https://aquadocs.org/handle/1834/41361 (Accessed February 25, 2023).
Schmidt J. O., Bograd S. J., Arrizabalaga H., Azevedo J. L., Barbeaux S. J., Barth J. A., et al. (2019). Future ocean observations to connect climate, fisheries and marine ecosystems. Front. Mar. Sci. 6. doi: 10.3389/fmars.2019.00550
Smith S. R., Alory G., Andersson A., Asher W., Baker A., Berry D. I., et al. (2019). Ship-based contributions to global ocean, weather, and climate observing systems. Front. Mar. Sci. 6. doi: 10.3389/fmars.2019.00434
Spalding M. J., Braestrup A. E., Refosco A. (2021). Greening the blue economy: a transdisciplinary analysis. In: Carpenter A., Johansson T. M., Skinner J. A. (eds) Sustainability in the Maritime Domain. Strategies for Sustainability. Springer, Cham. doi: 10.1007/978-3-030-69325-1_2
Sparnocchia S., Martinelli M., Belardinelli A., Penna P., Domenichetti F., Campanelli A., et al. (2017). D9.3-Mediterranean Sea demonstration report: report on demonstration of sensor systems in the Mediterranean (Ancona IT: NeXOS).
Sparnocchia S., Nair R., Petihakis G., Aydoğdu A., Dobricic S., Farcy P., et al. (2016). An interlinked coastal observatory network for Europe. J. Operational Oceanogr. 9, s193–s201. doi: 10.1080/1755876X.2015.1114808
Stammer D., Bracco A., AchutaRao K., Beal L., Bindoff N., Braconnot P., et al. (2019). Ocean climate observing requirements in support of climate research and climate information. Front. Mar. Sci. 6. doi: 10.3389/FMARS.2019.00444/BIBTEX
Steins N. A., Mackinson S., Mangi S. C., Pastoors M. A., Stephenson R. L., Ballesteros M., et al. (2022). A will-o’-the wisp? on the utility of voluntary contributions of data and knowledge from the fishing industry to marine science. Front. Mar. Sci. 9. doi: 10.3389/fmars.2022.954959
Tambutti M., Gómez J. J. (2020) The outlook for oceans, seas and marine resources in Latin America and the Caribbean: conservation, sustainable development and climate change mitigation. Available at: www.cepal.org/apps (Accessed February 22, 2023).
Tanhua T., Pouliquen S., Hausman J., O’Brien K., Bricher P., de Bruin T., et al. (2019). Ocean FAIR data services. Front. Mar. Sci. 6. doi: 10.3389/fmars.2019.00440
Taylor J., Feng M. (2022). A deep learning model for forecasting global monthly mean sea surface temperature anomalies. Front. Climate 4. doi: 10.3389/FCLIM.2022.932932/BIBTEX
United Nations. (2017). The ocean conference factsheet: people and oceans. (New York, USA: The United Nations)
United Nations. (2022). Sustainable blue economy vital for small countries and coastal populations (New York, USA: UN News).
Van Vranken C., Vastenhoud B. M. J., Manning J. P., Plet-Hansen K. S., Jakoboski J., Gorringe P., et al. (2020). Fishing gear as a data collection platform: opportunities to fill spatial and temporal gaps in operational Sub-surface observation networks. Front. Mar. Sci. 7. doi: 10.3389/fmars.2020.485512
Viglione G. (2021). Fevers are plaguing the oceans [[/amp]]mdash; and climate change is making them worse. Nature 593, 26–28. doi: 10.1038/d41586-021-01142-4
Voyer M. A., Schofield C. H., Azmi K., Warner R. M., Mcilgorm A., Voyer M., et al. (2018). Maritime security and the blue economy: intersections and interdependencies in the Indian ocean publication details. J. Indian Ocean Region 14, 1–21. doi: 10.1080/19480881.2018.1418155
Whitt C., Pearlman J., Polagye B., Caimi F., Muller-Karger F., Copping A., et al. (2020). Future vision for autonomous ocean observations. Front. Mar. Sci. 7. doi: 10.3389/FMARS.2020.00697/FULL
Wilkin J., Levin J., Moore A., Arango H., Lopez A., Hunter E. (2022). A data-assimilative model reanalysis of the U.S. mid Atlantic bight and gulf of Maine: configuration and comparison to observations and global ocean model. Prog. Oceanogr 209. doi: 10.1016/j.pocean.2022.102919
Wilkinson M. D., Dumontier M., Aalbersberg I., Appleton G., Axton M., Baak A., et al. (2016). The FAIR guiding principles for scientific data management and stewardship. Sci. Data 3, 160018. doi: 10.1038/sdata.2016.18
Keywords: in situ ocean observing systems, citizen science, industry collaboration, shelf and coastal seas, essential ocean variables (EOV), physical oceanography
Citation: Van Vranken C, Jakoboski J, Carroll JW, Cusack C, Gorringe P, Hirose N, Manning J, Martinelli M, Penna P, Pickering M, Piecho-Santos AM, Roughan M, de Souza J and Moustahfid H (2023) Towards a global Fishing Vessel Ocean Observing Network (FVON): state of the art and future directions. Front. Mar. Sci. 10:1176814. doi: 10.3389/fmars.2023.1176814
Received: 28 February 2023; Accepted: 20 June 2023;
Published: 01 August 2023.
Edited by:
Shunqi Pan, Cardiff University, United KingdomReviewed by:
Susan Kay, Plymouth Marine Laboratory, United KingdomCopyright © 2023 Van Vranken, Jakoboski, Carroll, Cusack, Gorringe, Hirose, Manning, Martinelli, Penna, Pickering, Piecho-Santos, Roughan, de Souza and Moustahfid. This is an open-access article distributed under the terms of the Creative Commons Attribution License (CC BY). The use, distribution or reproduction in other forums is permitted, provided the original author(s) and the copyright owner(s) are credited and that the original publication in this journal is cited, in accordance with accepted academic practice. No use, distribution or reproduction is permitted which does not comply with these terms.
*Correspondence: Cooper Van Vranken, Y29vcGVyQG9jZWFuZGF0YS5uZXQ=
Disclaimer: All claims expressed in this article are solely those of the authors and do not necessarily represent those of their affiliated organizations, or those of the publisher, the editors and the reviewers. Any product that may be evaluated in this article or claim that may be made by its manufacturer is not guaranteed or endorsed by the publisher.
Research integrity at Frontiers
Learn more about the work of our research integrity team to safeguard the quality of each article we publish.