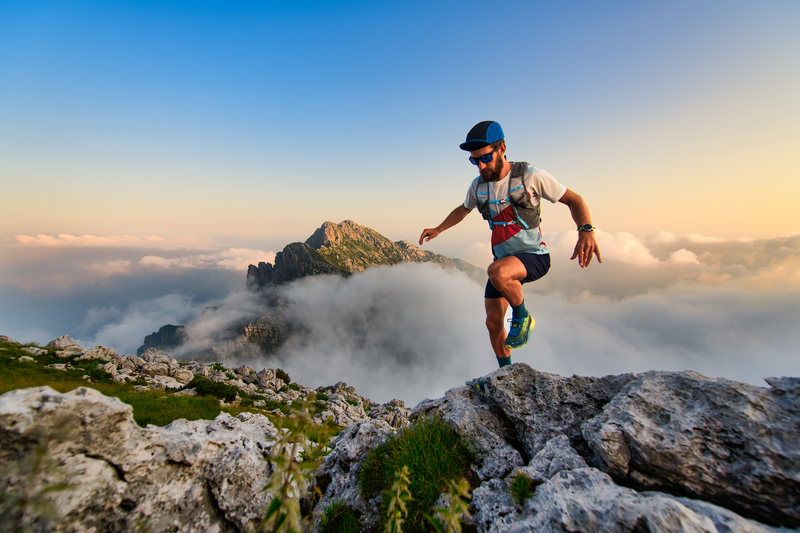
95% of researchers rate our articles as excellent or good
Learn more about the work of our research integrity team to safeguard the quality of each article we publish.
Find out more
METHODS article
Front. Mar. Sci. , 15 August 2023
Sec. Coastal Ocean Processes
Volume 10 - 2023 | https://doi.org/10.3389/fmars.2023.1167863
This article is part of the Research Topic Climate Change impacts on Mediterranean Coastal and Transitional Areas: Assessment, Projection, and Adaptation View all 10 articles
Global climate models, indispensable for projecting the human-driven climate change, have been improving for decades and are nowadays capable of reproducing multiple processes (e.g., aerosols, sea-ice, carbon cycle) at up to 25 km horizontal resolution. Meteotsunami events – tsunami waves generated by mesoscale atmospheric processes – are properly captured only by sub-kilometre-scale downscaling of these models. However, the computational cost of long-term high-resolution climate simulations providing accurate meteotsunami hazard assessments would be prohibitive. In this article, to overcome this deficiency, we present a new methodology allowing to project sub-kilometre-scale meteotsunami hazards and their climate uncertainties at any location in the world. Practically, the methodology uses (1) synoptic indices to preselect a substantial number of short-term meteotsunami episodes and (2) a suite of atmospheric and oceanic models to downscale them from an ensemble of global models to the sub-kilometre-scale. Such approach, using hundreds of events to build robust statistics, could allow for an objective assessment of the meteotsunami hazards at the climate scale which, on top of sea level rise and storm surge hazards, is crucial for building adaptation plans to protect coastal communities worldwide.
Meteorological tsunamis, or meteotsunamis, are tsunami-like ocean long waves driven by intense, fast-propagating and low-dispersive atmospheric disturbances capable of transferring their energy to the ocean (Monserrat et al., 2006). Extreme meteotsunami events can be associated with coastal flooding, structural damage and human losses, as illustrated in Figure 1 for one of the highest meteotsunami waves ever witnessed (with conservative estimate of up to 6 m in height) which took place in Vela Luka (Adriatic Sea, Croatia) on the 21st of June 1978. Two processes are preconditioning the generation of these extreme events: (1) offshore resonance (Vilibić, 2008) occurring when the speed of the atmospheric waves is equal to either the speed of the ocean long waves over a flat bathymetry (i.e., Proudman resonance; Proudman, 1929) or the speed of the edge waves along a coastline (Greenspan resonance; Greenspan, 1956), and (2) nearshore amplification, for example, through shoaling and harbour resonance (Pattiaratchi and Wijeratne, 2015; Rabinovich, 2020). Consequently, meteotsunamis mostly occur in known hotspot locations (e.g., the Balearic Islands and the Adriatic Sea in the Mediterranean region or the Great Lakes in the USA) favourable to the amplification of the long waves (Vilibić et al., 2016; Vilibić et al., 2021) and during weather events generating atmospheric disturbances travelling at speeds that trigger the Proudman or Greenspan resonances.
Figure 1 Compilation of photos and newspaper clips illustrating the catastrophic sea level oscillations and floods during the 21st of June 1978 meteotsunami event in Vela Luka, Croatia (after Vučetić and Barčot, 2008).
The most common weather conditions driving meteotsunami events are associated with mesoscale processes such as atmospheric mesoscale gravity waves (MGWs), convective storms, frontal passages/squall lines and tropical cyclones. Atmospheric MGWs may be generated by unbalanced jet streaks through the so-called spontaneous balance adjustment (Ruppert et al., 2022) and may be preserved over long distances via wave ducting in the lower troposphere (Lindzen and Tung, 1976; Monserrat and Thorpe, 1996). Consequently, MGWs can generate meteotsunamis due to rapid surface pressure oscillations up to >5 hPa in less than an hour (e.g., Sheremet et al., 2016). Individual convective storms can be generated locally in unstable atmospheric situations and are typically associated with surface pressure perturbations that, when propagating at appropriate speeds, can cause meteotsunamis (e.g., Belušić and Strelec Mahović, 2009). Squall lines, or lines of thunderstorms, often form along or ahead of cold fronts and can extend laterally for hundreds of kilometres. Long-lived strong squall lines, also called “derechos” (Spanish for ‘straight’), can travel many hundreds of kilometres and are associated with air pressure disturbances that can generate meteotsunamis through resonance (e.g., Šepić and Rabinovich, 2014; Wertman et al., 2014). Finally, meteotsunamis can also be driven by either tropical cyclone rainbands – i.e., the regions of heaviest precipitation outside of the tropical cyclone eyeball – that can develop squall-line-like characteristics or by atmospheric internal gravity waves produced by tropical cyclones (e.g., Shi et al., 2020).
In the last decade, numerical modelling and forecast of meteotsunami events has been a pressing issue for the development of meteotsunami early warning systems (Vilibić et al., 2016). Despite the many challenges posed by accurately simulating both the localized mesoscale atmospheric processes and the nearshore amplifications driving the meteotsunami events, more and more early warning systems and high-resolution modelling suites are nowadays capable to capture these processes (e.g., Renault et al., 2011; Anderson and Mann, 2021; Angove et al., 2021; Sun and Niu, 2021; Tojčić et al., 2021; Kim et al., 2022; Rahimian et al., 2022). Historically, three complementary avenues have been explored: (1) synoptic indices, (2) high-resolution numerical models and (3) ensemble or stochastic approaches. Synoptic indices – connecting atmospheric patterns with meteotsunami events – have only been successfully used in the Balearic Islands, Spain (Ramis and Jansà, 1983; Šepić et al., 2016; Vich and Romero, 2021). They also have recently been derived worldwide and strong connections between meteotsunami events and synoptic patterns have been found along most of the world’s coastlines, but particularly at mid-latitudes (Šepić et al., 2015; Vilibić and Šepić, 2017; Zemunik et al., 2022). The advantage of the approach is that no additional numerical cost is required, as synoptic indices – identifying meteotsunami events up to a week in advance – can be derived and used with relatively coarse weather models (30-80 km in resolution). The major drawback is the impossibility to quantify the intensity of the events due to the important contribution of mesoscale processes not captured by the synoptic indices, which can be exacerbated for locally generated atmospheric disturbances, such as convective storms, that can be completely missed. Consequently, the implementation of operational coupled atmosphere-ocean modelling suites at the sub-kilometre scale is an unavoidable necessity in early warning systems. To this date, they have only been implemented in the Balearic Islands (Renault et al., 2011) and the Adriatic Sea (Denamiel et al., 2019a; Denamiel et al., 2019b). In both systems the presence of meteotsunamigenic disturbances triggering the event mode of the early warning systems are nearly always detected but their exact location and intensity is not always well simulated. The meteotsunami hazards (which can be defined both as the maximum coastal sea elevation – meteotsunami surge – and/or meteotsunami wave height) can thus be numerically quantified for the known hotspot locations. Nevertheless, even for a 3-day forecast, running sub-kilometre scale models has proven to be numerically expensive and, for the Adriatic Sea, the operational forecasting service was discontinued. Finally, deterministic forecasts often fail to predict the meteotsunami surges at hotspot locations due to the difficulty to properly simulate the exact location, speed, period, amplitude, etc., of the atmospheric disturbances driving such events. Consequently, meteotsunami hazards can only be properly quantified via ensemble or stochastic approaches. In the Balearic Islands, following Mourre et al. (2021), using multiple atmospheric model configurations improved the forecast of meteotsunami events. However, ensembles of kilometre-scale (or km-scale) atmospheric models have an enormous computational cost which can be prohibitive for their operational implementation. Stochastic methods (Geist et al., 2014) and surrogate models or ocean emulators (Denamiel et al., 2019b; Denamiel et al., 2020a; Denamiel et al., 2021a) are thus promising avenues to account for the uncertainty on simulating meteotsunami events with deterministic models. For example, such a meteotsunami surrogate model has been successfully implemented in the Adriatic Sea where it can run operationally at nearly no numerical cost (i.e., stochastic results based on 20000 samples produced in a few minutes on a laptop) despite being trained on more than 4000 simulations (i.e., running in parallel on High Performance Computers) and, hence, being numerically expensive to design (Tojčić et al., 2021).
Following these major advances, climate projection of meteotsunami hazards is the next big challenge faced by the meteotsunami community. Generally speaking, in this era of anthropogenically-induced climate changes, the climate research community needs to provide more accurate climate projections of the entire Earth system to the decision makers (Smith et al., 2014), in order to better adapt to the societal impact of future extreme events (e.g., droughts, storms, sea-level rise, etc.). Further, a great number of extreme events are localized, thus leading to an improper quantification of the hazards by state-of-the-art global and regional circulation models. This is very well illustrated in the Venice Lagoon, where underestimations of just 40 cm in storm surge height by operational forecasts (Ferrarin et al., 2021) can change the flooded area of the city of Venice from ca. 25% to 80% (Cavaleri et al., 2020), resulting in much larger flood risks and damages (estimated to up to 1.1 billion of euros just in Venice). This is also the case for meteotsunami events, which are driven by atmospheric conditions highly influenced by the on-going global warming. For example, the positive trends of temperature and maximum wind speed over the Adriatic Sea during the 1987-2017 period (Tojčić et al., 2023) might already impact the meteotsunamigenic conditions at the synoptic scale in this region. Globally, mid-troposphere jet stream intensity, meandering and latitudinal range present the highest correlations with meteotsunami occurrences (Vilibić and Šepić, 2017; Zemunik et al., 2022) and are undoubtedly affected by the ongoing climate change (Hallam et al., 2022; Moon et al., 2023). Till now, little is known about meteotsunami hazard assessments under climate change as only two studies have tried to quantify the impact of global warming on meteotsunamis. One used the synoptic index-based approach in the Balearic Islands (Vilibić et al., 2018) and found that the number of days with synoptic conditions prone to the generation of meteotsunamis could increase by one third under extreme warming conditions (Representative Concentration Pathway RCP 8.5 scenario). The other used the pseudo-global warming (PGW) downscaling approach (Schär et al., 1996; Denamiel et al., 2020b) for half a dozen meteotsunami events in the Adriatic Sea (Denamiel et al., 2022) and found a strong impact of climate warming on the spatial variability of the meteotsunami intensity under RCP 8.5 scenario. It should also be noted that both studies were carried out with a single Regional Climate Model (RCM) and for a single climate scenario, and thus do not account for the climate uncertainty.
To bridge the gap between climate modelling and accurate meteotsunami hazard assessments, this article presents a new methodology that could be used to lower the computational costs of sub-kilometre-scale meteotsunami climate modelling while keeping a fair description of the climate projection uncertainties.
We postulate that targeted downscaling of short-term meteotsunami events from global climate models (GCMs) to sub-kilometre-scale resolutions is key to assess meteotsunami hazards (i.e., maximum coastal sea levels or meteotsunami surges, and maximum meteotsunami wave heights) in future climates at a reasonable numerical cost. Hereafter, the four presented hypotheses on which our methodology is based address three scientific challenges previously highlighted by Sillmann et al. (2017): (1) What are the relevant sources of predictability of meteotsunami events that can support their attribution, prediction and projection? (2) What is the relevant definition of a meteotsunami event, as a short-term extreme? (3) What are the necessary model output requirements to analyse meteotsunami events?
This hypothesis partially addresses question 1 of Sillmann et al. (2017), and follows the early findings of Lorenz (1956; 1969), who argues that two relatively similar synoptic situations are likely to produce similar local effects. This finding has been successfully automatized and implemented for precipitation climate studies (e.g., Radanovics et al., 2013; Dayon et al., 2015; Chardon et al., 2018). For meteotsunami events, the global research is ongoing and has shown promising results along the worldwide coastlines. However, as stated in Šepić et al. (2016), the detection of real meteotsunami events with synoptic indices only works in 20% of the cases which means that 80% of false positives (i.e., flagging of non-extreme events) can be expected when using this approach. Further, Zemunik et al. (2022) show that similar synoptic patterns are common to all meteotsunami events but that the efficiency of the synoptic indices (i.e., their capability to accurately detect meteotsunami events) might strongly vary depending on the studied geographical location, as seen in Figure 2 (Hypothesis 1).
Figure 2 Illustration of the research hypotheses. Hypothesis 1: Average of the synoptic conditions (wind speed at 850 hPa, temperature at 550 hPa and mean sea level pressure or mslp) for meteotsunami events at northern hemisphere stations and time series of non-seismic sea level oscillations at tsunami timescales (NSLOTT) and synoptic index ranges (after Zemunik et al., 2022). Hypothesis 2: Selection of meteotsunami events from ensemble global climate models to account for the projection uncertainty (after Jentsch et al., 2007). Hypothesis 3: atmospheric disturbances (i.e., high-pass filtered mean sea level pressure) in the Adriatic Sea extracted from 1.5-day long AdriSC model kilometre-scale simulations (after Denamiel et al., 2019a). Hypothesis 4: Adriatic meteotsunami hotspot locations (Vela Luka and Stari Grad, Croatia) represented with a 10 m resolution in the ocean mesh for precise meteotsunami hazards (i.e., maximum coastal sea levels or meteotsunami surges, and maximum meteotsunami wave heights) assessments (after Denamiel et al., 2018).
We thus propose (1) to create synoptic meteotsunami indices for specific locations where the indices are found to be efficient, and (2) to apply the indices to GCM ensembles in order to automatically pinpoint similar synoptic conditions and extract ensembles of meteotsunami events.
Although GCM ensembles do not necessarily represent the full uncertainty in climate projections (Knutti, 2010), they allow investigating uncertainty in future projections and particularly examining the maximum–minimum climate change scenarios. Due to limitations in computational resources, RCM ensembles only downscale subsets of the GCM ensembles (Ito et al., 2020). To avoid erroneous interpretations of the obtained climate impact assessments, several strategies have been derived to select subsets of GCMs (e.g., smaller biases in the historical climate simulations, coverage of the widest possible uncertainty range of future projections; Reichler and Kim, 2008; Cannon, 2015; Mendlik and Gobiet, 2016). Therefore, to fully address question 1 of Sillmann et al. (2017) and to obtain meteotsunami hazard estimates as robust as possible, we propose to downscale all events from all appropriate GCMs. Consequently, the subsampling procedure will not anymore be applied to select CGMs but to detect meteotsunami events.
Provided that the synoptic index is efficient (i.e., that enough meteotsunami events are extracted despite the known false alarm rate), the range of the climate uncertainty derived with the proposed approach will thus be similar to the one derived with the GCM ensembles as illustrated in Figure 2 (Hypothesis 2).
This hypothesis addresses question 2 of Sillmann et al. (2017) and is based on the fact that simulations of meteotsunami events mostly rely on favourable initial states and the presence of large-scale drivers, which are both seen at the synoptic scale by GCMs, as well as positive feedbacks and mesoscale processes which are well-reproduced by kilometre-scale short-term simulations. Short-term forecasts of meteotsunami events (e.g., Renault et al., 2011; Denamiel et al., 2019a; Denamiel et al., 2019b; Mourre et al., 2021; Kim et al., 2022) have demonstrated that kilometre-scale coupled atmosphere-ocean models used in 3 day-long simulations forced by global or regional model outputs realistically represent processes which are too small to be reproduced by coarser long-term simulations and are capable to simulate meteotsunami hazards (i.e., maximum coastal sea levels or meteotsunami surges, and maximum meteotsunami wave heights).
For event-based meteotsunami downscaling, we thus propose to use specialized kilometre-scale modelling suites that have already been successfully tested in operational mode but that have also been demonstrated to often fail to simulate the proper location, speed, period, amplitude, etc., of the atmospheric disturbances, as shown in Figure 2 (Hypothesis 3 & 4) for Adriatic Sea meteotsunami events. Consequently, we also recommend the use of surrogate models to account for these uncertainties.
As demonstrated by Schewe et al. (2019), state-of-the-art economic assessments of climate change impacts presently rely on global models which underestimate extreme events and associated societal risks. Additionally, in the Earth system modelling community, the accuracy of the models and thus of the climate projections during extreme events has been undeniably linked to the increase in spatial resolution of these models (Prein et al., 2015; Lucas-Picher et al., 2021). Consequently, we propose to downscale meteotsunami events to sub-kilometre-scale in order to precisely derive the impact of climate change on the meteotsunami hazards. This is particularly important for hotspot areas where the proper representation of the geomorphology but also of the human made infrastructures is strongly affecting the assessments of the climate hazards leading to the adaptation strategies – as illustrated in Figure 2 (Hypothesis 4) for the Vela Luka and Stari Grad harbours in the Adriatic Sea.
This hypothesis thus addresses question 3 of Sillmann et al. (2017) by defining the output of method and, hence, the meteotsunami hazards as distributions of maximum coastal sea levels (or meteotsunami surges) and maximum meteotsunami wave heights at precise hotspot locations depending on both the climate uncertainty (Hypothesis 2) and the atmospheric disturbance simulation uncertainty (Hypothesis 3).
The conceptual design of the methodology using synoptic indices to derive targeted meteotsunami hazards from GCM simulations is presented in Figure 3. It is based on three different modules. First, the synoptic index module will automatically extract meteotsunami events for specific regions from the available GCM ensembles (i.e., different models and climate projections). The chosen synoptic indices will optimize the extraction of true meteotsunami events from the GCMs and minimize the flagging of non-extreme events (i.e., false positive). Then, the kilometre downscaling module will consist of 3 day-long kilometre-scale simulations of all the meteotsunami events extracted from the GCMs, thus necessarily including false positives. This module will rely on a cascade of nested grids from 15-km to 1-km resolution to properly downscale the GCM results. Finally, the targeted meteotsunami hazard module, the product-oriented part of the methodology, will downscale the kilometre-scale simulations with meteotsunami event realizations (i.e., excluding false positives) to sub-kilometre-scale 1 day-long simulations and will provide targeted hazard assessments for future projections from this specific ensemble of simulations. The detailed description of the three modules is as follows.
Figure 3 Outline of the methodology using (A) synoptic indices designed with (B) global reanalysis products to derive targeted meteotsunami hazards by downscaling (C) historical and (D) future GCM simulations. For a selected region: (1) the synoptic index is used to automatically extract extreme events from GCM ensembles (blue circle), (2) the GCM results are downscaled to kilometre-scale for all chosen events (orange circle) and (3) the kilometre-scale simulations with extreme event realizations (red circle) are further downscaled to sub-kilometre-scale and targeted hazard assessment projections are derived. Artwork from Frits Ahlefeldt: https://fritsahlefeldt.com.
Establishes a connection between synoptic patterns and observations at a given location. It thus requires two different datasets: (1) a meteorological dataset describing the state of the atmosphere at a synoptic scale, such as a reanalysis dataset or a GCM, at different hours of the day, and (2) a dataset of quality-checked observations including high-frequency sea level data, preferably with a resolution of a minute, collected over a prolonged period (i.e., several years to decades). This module will aim to achieve the best efficiency for synoptic indices providing multivariate predictions that are (1) physically consistent (Raynaud et al., 2016), (2) spatially transferable (Radanovics et al., 2013; Chardon et al., 2018), and (3) temporally extendable to past and future climates (Dayon et al., 2015; Vilibić et al., 2018). For meteotsunami synoptic indices, points 1 and 2 have already been proven to be true as demonstrated by Zemunik et al. (2022) with averaging the synoptic conditions during meteotsunami events over the northern hemisphere stations and obtaining distinguishable characteristics (Figure 2, Hypothesis 1). The third point can only be reasonably proven by applying the presented methodology in known meteotsunami hotspot locations (e.g., Vela Luka, Croatia; Ciutadella, Spain). In practice, meteotsunami indices can be built by using either a multi-regression analysis (Šepić et al., 2016; Zemunik et al., 2022) or pattern recognition and machine learning algorithms (Cheng et al., 2014; Leuenberger and Kanevski, 2015).
Is state-of-the-art in the limited-area modelling community. It telescopically nests domains at a decreasing horizontal grid spacing with boundary conditions provided by GCMs or reanalyses, until kilometre-scales are reached (Prein et al., 2015). For meteotsunami events, we propose to downscale the atmosphere-ocean conditions to 1-km resolution from ensembles of climate projections such as CMIP6 (Coupled Model Intercomparison Project Phase 6, Eyring et al., 2016). Kilometre-scale models, such as the Weather Research and Forecasting (WRF; Skamarock et al., 2005) model in the atmosphere and the Regional Ocean Modelling System (ROMS, Shchepetkin and McWilliams, 2005; Shchepetkin and McWilliams, 2009) in the ocean, that have been successfully applied in the reproduction of meteotsunami events (Renault et al., 2011; Denamiel et al., 2019a; Mourre et al., 2021; Kim et al., 2022), can be used in this module.
Aims to further downscale the kilometre-scale meteotsunami simulations, excluding the false positive events. We propose to use sub-kilometre-scale unstructured models properly representing the geomorphology of the selected regions of interest – e.g., the ADCIRC-SWAN model (Dietrich et al., 2012) which was previously successfully applied to reproduce meteotsunami waves (Denamiel et al., 2019a). Further, when the deterministic downscaling of the atmospheric conditions will lead to the reproduction of meteotsunamigenic disturbances, but not of meteotsunami surges at hotspot locations (similarly to the operational meteotsunami forecasts), we also propose to use an ocean emulator or surrogate model approach to define the meteotsunami hazards (e.g., Denamiel et al., 2019b; Denamiel et al., 2020a; Denamiel et al., 2021a). Finally, the output of the method consists in robust statistics of targeted climate projections of meteotsunami hazards (i.e., maximum coastal sea levels or meteotsunami surges), derived from ensembles of deterministic sub-kilometre-scale simulations and stochastic surrogate models/ocean emulators for the selected regions of interest where the adaptation strategies of the coastal communities can be updated with this new information.
A practical implementation of the methodology could follow the seven steps of the workflow presented below and in Figure 4:
Figure 4 Workflow of the methodology for projecting meteotsunami hazards using synoptic indices designed with global reanalysis products (ERA5 Design) and GCM historical simulations (CMIP6 Design) to derive targeted meteotsunami hazards including the climate uncertainty (Clim. UQ) by downscaling (Km-scale Downscaling and Sub-km Down.) historical (CMIP6 Eval.) and future (CMIP6 Scen.) GCM simulations and using ocean emulators (Ocean Emul.).
In practice, meteotsunami synoptic indices are used to reconstruct the high-frequency sea level time variations at a given coastal station depending only on a combination of several wisely chosen atmospheric variables extracted from numerical models. For a selected region of interest, ERA5 atmospheric reanalysis fields (Hersbach et al., 2020) and available multi-year observations of high-frequency sea levels are used to build the synoptic index (e.g., during the 2012-2018 period) which can then be applied for any time period and any model at this station (e.g., using CMIP6 during the 2070-2100 period). To evaluate the design of the synoptic index and the capacity of the models to simulate meteotsunamis, the events extracted from ERA5 reanalysis with the synoptic index are then downscaled to sub-kilometre-scale and the surrogate model/ocean emulator is used to account for the atmospheric disturbance simulation uncertainties. The obtained results are compared with the sea level observations and the expected efficiency of the synoptic index is derived.
First, the synoptic index is calculated from the historical CMIP6 simulations for the region of interest chosen in step 1. Second, the synoptic index distributions derived from ERA5 and CMIP6 are compared. Then, if the comparison is successful – e.g., if the synoptic index distributions are significantly similar following statistical tests such as the Z-test – the extracted meteotsunami events from ERA5 reanalysis and CMIP6 historical simulations are downscaled to the kilometre-scale. Finally, the atmospheric and sea level distributions obtained at hotspot locations by downscaling ERA5 reanalysis and CMIP6 historical simulations are compared.
If the CMIP6 Evaluation Step fails (e.g., distributions significantly different following the Z-test), the synoptic index is redesigned by considering both the CMIP6 historical simulations and the ERA5 reanalysis until success of the CMIP6 Evaluation Step.
Kilometre-scale downscaling of the CMIP6 future climate scenario ensembles for the meteotsunami events extracted with the synoptic index. It is important to highlight that despite the optimization of the synoptic index presented in steps 1 to 3, many meteotsunami events might still be missed by the methodology (i.e., false negatives). Consequently, to properly account for the climate uncertainty, robust meteotsunami synoptic indices must be built to minimize the number of missed events (false negatives) and not the number of false positives.
First, false positive events are excluded from the ensemble of kilometre-scale simulations for both historical and future simulations. The selection of the events is based on the automatic detection of meteotsunamigenic disturbances from the 1 km resolution atmospheric results – e.g., as presented in Denamiel et al. (2019a) for the Adriatic Sea. Second, the historical and future ensembles of positive events are further downscaled in the ocean to sub-kilometre resolutions (up to 10 m).
Based on the experience built with operational meteotsunami forecasts, simulated meteotsunamigenic disturbances often fail to generate meteotsunami waves at hotspot locations due to a shift in space, speed, period, etc. In this case, mathematical emulators or surrogate models can be used to assess the meteotsunami hazards linked to the failed event.
The targeted meteotsunami hazards and their associated climate uncertainty are quantified for both historical and future simulations.
The anticipated results from such a procedure are the generation of datasets of historical and scenario based sub-kilometre-scale meteotsunami simulations providing targeted hazard assessments and their associated uncertainties linked to both atmospheric disturbance simulations (following the results provided by the surrogate models/ocean emulators) and climate warming (following the main trends of the CMIP6 simulations). These datasets are of critical importance for the design of climate adaption strategies at hotspot locations (e.g., Great Lakes, USA; Vela Luka, Croatia; Ciutadella, Spain; Nagasaki Bay, Japan; Freemantle, Australia) where meteotsunami events pose a threat to the population.
In contrast with the other tsunami sources (e.g. earthquakes, volcanoes, landslides), meteotsunami events are thought to be influenced by the on-going climate warning. Consequently, classical tsunami methods (e.g., Poisson rate of occurrence) cannot be applied to quantify the long-term frequency and intensity of these events, and, hence, to assess meteotsunami hazards. Instead, a climate change approach trying to extract the past and future events from climate models in order to derive statistics on their behaviour and characteristics should be used. However, running models and model ensembles at sub-kilometre-scale resolution is known to require enormous computing resources and the efficiency (in terms of improvement of the results relative to the numerical cost) of producing such climate projections for long-term simulations can be questioned. For example, despite the modern technological advances and the numerical resources available to climate scientists, the coupled atmosphere-ocean kilometre-scale climate model implemented in the Adriatic basin with nested grids ranging from 15 to 1 km resolutions takes 18 months of continuous calculation with 260 CPUs to generate 31-year long climate simulations (Denamiel et al., 2021b; Pranić et al., 2021). In other words, to produce results for only one climate scenario (e.g., RCP 8.5) during the 1950-2100 period, this model would have to be run for more than 7 years. Further, meteotsunami events would still have to be extracted and downscaled to sub-kilometre, for the entire 1950-2100 period, in order to provide accurate hazard assessments. Consequently, this numerical cost is far too prohibitive to envision running ensembles of simulations forced by multiple GCMs under multiple warming scenarios (Semenov and Stratonovitch, 2010) and to properly assess the kilometre-scale uncertainties under climate warming.
The presented method is detouring this major obstacle by efficiently using the numerical resources – previously spent to produce long-term climate simulations – to quantify the climate change uncertainty and to properly assess the meter-scale meteotsunami hazards. However, several questions concerning the validity of the approach remain unanswered at this stage. Is the degree of connection between meteotsunami events and synoptic patterns high enough along the entire world’s coastlines for the method to be generalized worldwide (or should the method only be applied in areas like the Mediterranean basin)? Synoptic indices built with the set of synoptic variables optimized for Ciutadella (Spain) have already been demonstrated to be more efficient for Mediterranean locations than for the rest of the world (Zemunik et al., 2022). Consequently, sensitivity studies should be performed, at each station individually, to define the optimal set of synoptic variables needed to build the most efficient index at a given location. Will the extraction of the meteotsunami events with the synoptic indices lead to a large number of false positive events? Currently, meteotsunami synoptic indices, even derived for well-known Mediterranean hotspot locations (e.g., Ciutadella, Spain) have been leading to up to 80% of false positives (Šepić et al., 2016). Consequently, in order to reduce the numerical cost of the method, the general efficiency of the synoptic indices should be improved by, for example, using machine learning techniques (e.g., pattern recognition) to better identify the meteotsunamigenic synoptic conditions. Will the use of surrogate models/ocean emulators to compensate the failures of the deterministic downscaling add a bias to the climate uncertainty quantification? Meteotsunami surrogate models have only been used in the Adriatic Sea to improve the forecasts of these extreme events (Denamiel et al., 2019b; Denamiel et al., 2020a) and their impact on the final hazard assessments is, for now, difficult to quantify. Meteotsunami climate modelling is at its early stage, and we believe that many of these questions could find an answer in the decade to come when more and more scientists around the world will be able to test the presented methodology.
Finally, we argue that this targeted climate modelling strategy could be adapted to other extreme climate hazards such as convective storms, whose proper reproduction is still considered as one of the greatest challenges in the atmospheric climate community (Stephens et al., 2010; Coppola et al., 2020). Consequently, the presented methodology could lead to more accurate economic assessments of the climate change impacts on extreme events with a low carbon footprint coming out of extensive numerical computations. This could result in increasing preparation and adaptation of the worldwide communities to the upcoming local risks posed by climate warming.
The original contributions presented in the study are included in the article/supplementary material. Further inquiries can be directed to the corresponding author.
CD and IV wrote the initial version of the manuscript. CD, IV and DB developed the conceptual design for assessing meteotsunami hazard in future climates. CD prepared the figures. All authors revised the manuscript, reviewed and approved the final manuscript.
We acknowledge the support of ChEESE-2P, a project funded by EuroHPC under the grant agreement No. 101093038.
The presented research is partially derived from the project proposal “Projecting climate hazards at sub-kilometre scales (REASSESS)”, submitted to the European Research Council Advanced Research projects call of 2022. The comments raised by two reviewers greatly improved the manuscript.
The authors declare that the research was conducted in the absence of any commercial or financial relationships that could be construed as a potential conflict of interest.
All claims expressed in this article are solely those of the authors and do not necessarily represent those of their affiliated organizations, or those of the publisher, the editors and the reviewers. Any product that may be evaluated in this article, or claim that may be made by its manufacturer, is not guaranteed or endorsed by the publisher.
Anderson E. J., Mann G. E. (2021). A high-amplitude atmospheric inertia–gravity wave-induced meteotsunami in Lake Michigan. Nat. Hazards 106, 1489–1501. doi: 10.1007/s11069-020-04195-2
Angove M., Kozlosky L., Chu P., Dusek G., Mann G., Anderson E., et al. (2021). Addressing the meteotsunami risk in the United States. Nat. Hazards 106, 1467–1487. doi: 10.1007/s11069-020-04499-3
Belušić D., Strelec Mahović N. (2009). Detecting and following atmospheric disturbances with a potential to generate meteotsunamis in the Adriatic. Phys. Chem. Earth 34, 918–927. doi: 10.1016/j.pce.2009.08.009
Cannon A. J. (2015). Selecting GCM scenarios that span the range of changes in a multimodel ensemble: application to CMIP5 climate extremes indices. J. Clim. 28, 1260–1267. doi: 10.1175/JCLI-D-14-00636.1
Cavaleri L., Bajo M., Barbariol F., Bastianini M., Benetazzo A., Bertotti L., et al. (2020). The 2019 flooding of Venice and its implications for future predictions. Oceanography 33 (1), 42–49. doi: 10.5670/oceanog.2020.105
Chardon J., Hingray B., Favre A.-C. (2018). An adaptive two-stage analog/regression model for probabilistic prediction of small-scale precipitation in France. Hydrol. Earth Syst. Sci. 22, 265–286. doi: 10.5194/hess-22-265-2018
Cheng L., AghaKouchak A., Gilleland E., Katz R. W. (2014). Non-stationary extreme value analysis in a changing climate. Clim. Change 127, 353–369. doi: 10.1007/s10584-014-1254-5
Coppola E., Sobolowski S., Pichelli E., Raffaele F., Ahrens B., Anders I., et al. (2020). A first-of-its-kind multi-model convection permitting ensemble for investigating convective phenomena over Europe and the Mediterranean. Clim. Dyn. 55, 3–34. doi: 10.1007/s00382-018-4521-8
Dayon G., Boé J., Martin E. (2015). Transferability in the future climate of a statistical downscaling method for precipitation in France. J. Geophys. Res. Atmos. 120, 1023–1043. doi: 10.1002/2014JD022236
Denamiel C., Huan X., Šepić J., Vilibić I. (2020a). Uncertainty propagation using polynomial chaos expansions for extreme sea level hazard assessment: The case of the eastern Adriatic meteotsunamis. J. Phys. Oceanogr. 50 (4), 1005–1021. doi: 10.1175/JPO-D-19-0147.1D
Denamiel C., Huan X., Vilibić I. (2021a). Conceptual design of extreme sea-level early warning systems based on uncertainty quantification and engineering optimization methods. Front. Mar. Sci. 8. doi: 10.3389/fmars.2021.650279
Denamiel C., Pranić P., Ivanković D., Tojčić I., Vilibić I. (2021b). Performance of the Adriatic Sea and coast (AdriSC) climate component –A COAWST V3.3-based coupled atmosphere–ocean modelling suite: Atmospheric dataset. Geosci. Model. Dev. 14, 3995–4017. doi: 10.5194/gmd-14-3995-2021
Denamiel C., Pranić P., Quentin F., Mihanović H., Vilibić I. (2020b). Pseudo-global warming projections of extreme wave storms in complex coastal regions: The case of the Adriatic Sea. Clim. Dyn. 55, 2483–2509. doi: 10.1007/s00382-020-05397-x
Denamiel C., Šepić J., Huan X., Bolzer C., Vilibić I. (2019b). Stochastic surrogate model for meteotsunami early warning system in the eastern Adriatic Sea. J. Geophys. Res. Oceans 124, 8485–8499. doi: 10.1029/2019JC015574
Denamiel C., Šepić J., Ivanković D., Vilibić I. (2019a). The Adriatic Sea and coast modelling suite: Evaluation of the meteotsunami forecast component. Ocean Model. 135, 71–93. doi: 10.1016/j.ocemod.2019.02.003
Denamiel C., Šepić J., Vilibić I. (2018). Impact of geomorphological changes to harbor resonance during meteotsunamis: The Vela Luka bay test case. Pure Appl. Geophys. 175, 3839–3859. doi: 10.1007/S00024-018-1862-5
Denamiel C., Tojčić I., Vilibić I. (2022). Meteotsunamis in orography-free, flat bathymetry and warming climate conditions. J. Geophys. Res. Oceans 127, e2021JC017386. doi: 10.1029/2021JC017386
Dietrich J. C., Tanaka S., Westerink J. J., Dawson C. N., Luettich R. A. Jr., Zijlema M., et al. (2012). Performance of the Unstructured-Mesh, SWAN+ADCIRC Model in computing hurricane waves and surge. J. Sci. Comput. 52, 468–497. doi: 10.1007/s10915-011-9555-6
Eyring V., Bony S., Meehl G. A., Senior C. A., Stevens B., Stouffer R. J., et al. (2016). Overview of the Coupled Model Intercomparison Project Phase 6 (CMIP6) experimental design and organization. Geosci. Model. Dev. 9, 1937–1958. doi: 10.5194/gmd-9-1937-2016
Ferrarin C., Bajo M., Benetazzo A., Cavaleri L., Chiggiato J., Davison S., et al. (2021). Local and large-scale controls of the exceptional Venice floods of November 2019. Prog. Oceanogr. 197, 102628. doi: 10.1016/j.pocean.2021.102628
Geist E. L., ten Brink U., Gove M. (2014). A framework for the probabilistic analysis of meteotsunamis. Nat. Hazards 74, 123–142. doi: 10.1007/S11069-014-1294-1
Greenspan H. P. (1956). The generation of edge waves by moving pressure disturbances. J. Fluid Mech. 1, 574–592. doi: 10.1017/S002211205600038X
Hallam S., Josey S. A., McCarthy G. D., Hirschi J. J. M. (2022). A regional (land-ocean) comparison of the seasonal to decadal variability of the Northern Hemisphere jet stream 1871-2011. Clim. Dyn. 59, 1897–1918. doi: 10.1007/s00382-022-06185-5
Hersbach H., Bell B., Berrisford P., Hirahara S., Horányi A., Muñoz-Sabater J., et al. (2020). The ERA5 global reanalysis. Q. J. R. Meteorol. Soc. 146, 1999–2049. doi: 10.1002/qj.3803
Ito R., Shiogama H., Nakaegawa T., Takayabu I. (2020). Uncertainties in climate change projections covered by the ISIMIP and CORDEX model subsets from CMIP5. Geosci. Model. Dev. 13, 859–872. doi: 10.5194/gmd-13-859-2020
Jentsch A., Kreyling J., Beierkuhnlein C. (2007). A new generation of climate-change experiments: events, not trends. Front. Ecol. Environ. 5, 365–374. doi: 10.1890/1540-9295(2007)5[365:ANGOCE]2.0.CO;2
Kim M.-S., Woo S.-B., Eom H., You S. H., Lee H. M. (2022). Towards observation- and atmospheric model-based early warning systems for meteotsunami mitigation: A case study of Korea. Weather Clim. Extrem. 37, 100463. doi: 10.1016/j.wace.2022.100463
Knutti R. (2010). The end of model democracy? Clim. Change 102, 395–404. doi: 10.1007/s10584-010-9800-2
Leuenberger M., Kanevski M. (2015). Extreme Learning Machines for spatial environmental data. Comput. Geosci. 85, 64–73. doi: 10.1016/j.cageo.2015.06.020
Lindzen R. S., Tung K.-K. (1976). Banded convective activity and ducted gravity waves. Mon. Wea. Rev. 104, 1602–1617. doi: 10.1175/1520-0493(1976)104<1602:BCAADG>2.0.CO;2
Lorenz E. (1956). Empirical orthogonal functions and statistical weather prediction, Tech. rep (Cambridge: Massachusetts Institute of Technology, Department of Meteorology, Massachusetts Institute of Technology, Dept. of Meteorology). Available at: https://eapsweb.mit.edu/sites/default/files/Empirical_Orthogonal_Functions_1956.pdf.
Lorenz E. (1969). Atmospheric predictability as revealed by naturally occurring analogues. J. Atmos. Sci. 26, 636–646. doi: 10.1175/1520-0469(1969)26<636:APARBN>2.0.CO;2
Lucas-Picher P., Argueso D., Brisson E., Tramblay Y., Berg P., Lemonsu A., et al. (2021). Convection-permitting modeling with regional climate models: Latest developments and next steps. WIREs Clim. Change 12, e731. doi: 10.1002/wcc.731
Mendlik T., Gobiet A. (2016). Selecting climate simulations for impact studies based on multivariate patterns of climate change. Clim. Change 135, 381–393. doi: 10.1007/s10584-015-1582-0
Monserrat S., Thorpe A. J. (1996). Use of ducting theory in an observed case of gravity waves. J. Atmos. Sci. 53, 1724–1736. doi: 10.1175/1520-0469(1996)053<1724:UODTIA>2.0.CO;2
Monserrat S., Vilibić I., Rabinovich A. B. (2006). Meteotsunamis: atmospherically induced destructive ocean waves in the tsunami frequency band. Nat. Hazards Earth Syst. Sci. 6, 1035–1051. doi: 10.5194/nhess-6-1035-2006
Moon W., Kim B. M., Yang G. H., Wettlaufer J. S. (2023). Wavier jet streams driven by zonally asymmetric surface thermal forcing. Proc. Natl. Acad. Sci. U.S.A. 119, e2200890119. doi: 10.1073/pnas.2200890119
Mourre B., Santana A., Buils A., Gautreau L., Ličer M., Jansà A., et al. (2021). On the potential of ensemble forecasting for the prediction of meteotsunamis in the Balearic Islands: Sensitivity to atmospheric model parameterizations. Nat. Hazards 106, 1315–1336. doi: 10.1007/s11069-020-03908-x
Pattiaratchi C. B., Wijeratne E. M. S. (2015). Are meteotsunamis an underrated hazard? Philos. Trans. R. Soc. A 373, 20140377. doi: 10.1098/rsta.2014.0377
Pranić P., Denamiel C., Vilibić I. (2021). Performance of the Adriatic Sea and coast (AdriSC) climate component—A COAWST V3.3-based one-way coupled atmosphere–ocean modelling suite: Ocean results. Geosci. Model. Dev. 14, 5927–5955. doi: 10.5194/gmd-14-5927-2021P
Prein A. F., Langhans W., Fosser G., Ferrone A., Ban N., Goergen K., et al. (2015). A review on regional convection-permitting climate modeling: Demonstrations, prospects and challenges. Rev. Geophys. 53, 323–361. doi: 10.1002/2014RG000475
Proudman J. (1929). The effects on the sea of changes in atmospheric pressure. Geophys. Suppl. Mon. Not. R. Astron. Soc. 2, 197–209. doi: 10.1111/j.1365-246X.1929.tb05408.x
Rabinovich A. B. (2020). Twenty-seven years of progress in the science of meteorological tsunamis following the 1992 Daytona Beach event. Pure Appl. Geophys. 177, 1193–1230. doi: 10.1007/s00024-019-02349-3
Radanovics S., Vidal J.-P., Sauquet E., Ben Daoud A., Bontron G. (2013). Optimising predictor domains for spatially coherent precipitation downscaling. Hydrol. Earth Syst. Sci. 17, 4189–4208. doi: 10.5194/hess-17-4189-2013
Rahimian M., Beyramzadeh M., Siadatmousavi S. M. (2022). The skill assessment of Weather and Research Forecasting and WAVEWATCH-III models during recent meteotsunami event in the Persian Gulf. Front. Mar. Sci. 9. doi: 10.3389/fmars.2022.834151
Ramis C., Jansà A. (1983). Condiciones meteorológicas simultáneas a la aparición de oscilaciones del nivel del mar de amplitud extraordinaria en el Mediterráneo occidental. Rev. Geofisica 39, 35–42.
Raynaud D., Hingray B., Zin I., Anquetin S., Debionne S., Vautard R. (2016). Atmospheric analogues for physically consistent scenarios of surface weather in Europe and Maghreb. Int. J. Clim. 37, 2160–2176. doi: 10.1002/joc.4844
Reichler T., Kim J. (2008). How well do coupled models simulate Today's climate? Bull. Am. Meteorol. Soc. 89, 303–312. doi: 10.1175/BAMS-89-3-303
Renault L., Vizoso G., Jansà A., Wilkin J., Tintoré J. (2011). Toward the predictability of meteotsunamis in the Balearic Sea using regional nested atmosphere and ocean models. Geophys. Res. Lett. 38, L10601. doi: 10.1029/2011gl047361
Ruppert J. H., Koch S. E., Chen X., Du Y., Seimon A., Sun Y. Q., et al. (2022). Mesoscale gravity waves and midlatitude weather: A tribute to Fuqing Zhang. Bull. Am. Meteorol. Soc. 103, E129–E156. doi: 10.1175/BAMS-D-20-0005.1
Schär C., Frei C., Luthi D., Davies H. C. (1996). Surrogate climate-change scenarios for regional climate models. Geophys. Res. Lett. 23, 669–672. doi: 10.1029/96GL00265
Schewe J., Gosling S. N., Reyer C., Zhao F., Ciais P., Elliott J., et al. (2019). State-of-the-art global models underestimate impacts from climate extremes. Nat. Commun. 10, 1005. doi: 10.1038/s41467-019-08745-6
Semenov M. A., Stratonovitch P. (2010). Use of multi-model ensembles from global climate models for assessment of climate change impacts. Clim. Res. 41, 1–14. doi: 10.3354/cr00836
Šepić J., Rabinovich A. B. (2014). Meteotsunami in the Great Lakes and on the Atlantic coast of the United States generated by the “derecho” of June 29–30, 2012. Nat. Hazards 74, 75–107. doi: 10.1007/s11069-014-1310-5
Šepić J., Vilibić I., Monserrat S. (2016). Quantifying the probability of meteotsunami occurrence from synoptic atmospheric patterns. Geophys. Res. Lett. 43, 10377–10384. doi: 10.1002/2016GL070754
Šepić J., Vilibić I., Rabinovich A., Monserrat S. (2015). Widespread tsunami-like waves of 23-27 June in the Mediterranean and Black Seas generated by high-altitude atmospheric forcing. Sci. Rep. 5, 11682. doi: 10.1038/srep11682
Shchepetkin A. F., McWilliams J. C. (2005). The regional oceanic modeling system: A split-explicit, free-surface, topography-following-coordinate. Ocean Model. 9, 347–404. doi: 10.1016/j.ocemod.2004.08.002
Shchepetkin A. F., McWilliams J. C. (2009). Correction and commentary for “Ocean forecasting in terrain-following coordinates: Formulation and skill assessment of the regional ocean modeling system” by Haidvogel et al., J. Comput. Phys., 227, pp. 3595–3624. J. Comp. Phys. 228, 8985–9000. doi: 10.1016/j.jcp.2009.09.002
Sheremet A., Gravois U., Shrira V. (2016). Observations of meteotsunami on the Louisiana shelf: a lone soliton with a soliton pack. Nat. Hazards 84, 471–492. doi: 10.1007/s11069-016-2446-2
Shi L., Olabarrieta M., Nolan D. S., Warner J. C. (2020). Tropical cyclone rainbands can trigger meteotsunamis. Nat. Commun. 11, 678. doi: 10.1038/s41467-020-14423-9
Sillmann J., Thorarinsdottir T., Keenlyside N., Schaller N., Alexander L. V., Hegerl G., et al. (2017). Understanding, modeling and predicting weather and climate extremes: Challenges and opportunities. Weather Clim. Extrem. 18, 65–74. doi: 10.1016/j.wace.2017.10.003
Skamarock W. C., Klemp J. P., Dudhia J., Gill D. O., Barker D. M. (2005). A description of the advanced research WRF version 2. NCAR technical note NCAR/TN-468+STR National Center for Atmospheric Research, Boulder, 88 pp. doi: 10.5065/D6DZ069T
Smith M. J., Palmer P. I., Purves D. W., Vanderwel M. C., Lyutsarev V., Calderhead B., et al. (2014). Changing how Earth system modeling is done to provide more useful information for decision making, science, and society. Bull. Am. Meteorol. Soc. 95, 1453–1464. doi: 10.1175/BAMS-D-13-00080.1
Stephens G. L., L'Ecuyer T., Forbes R., Gettelmen A., Golaz J.-C., Bodas-Salcedo A., et al. (2010). Dreary state of precipitation in global models. J. Geophys. Res. Atmos. 115, D24211. doi: 10.1029/2010JD014532
Sun Q., Niu X. (2021). Harbor resonance triggered by atmospherically driven edge waves. Ocean Eng. 224, 108735. doi: 10.1016/j.oceaneng.2021.108735
Tojčić I., Denamiel C., Vilibić I. (2021). Performance of the Adriatic early warning system during the multi-meteotsunami event of 11–19 May 2020: an assessment using energy banners. Nat. Hazards Earth Syst. Sci. 21, 2427–2446. doi: 10.5194/nhess-21-2427-2021
Tojčić I., Denamiel C., Vilibić I. (2023). Kilometer-scale trends and variability of the Adriatic present climate, (1987–2017). Clim. Dyn. 61, 2521–2545. doi: 10.1007/s00382-023-06700-2
Vich M. D. M., Romero R. (2021). Forecasting meteotsunamis with neural networks: the case of Ciutadella harbour (Balearic Islands). Nat. Hazards 106, 1299–1314. doi: 10.1007/s11069-020-04041-5
Vilibić I. (2008). Numerical simulations of the Proudman resonance. Cont. Shelf Res. 28, 574–581. doi: 10.1016/j.csr.2007.11.005
Vilibić I., Rabinovich A. B., Anderson E. J. (2021). Special issue on the global perspective on meteotsunami science: editorial. Nat. Hazards 106, 1087–1104. doi: 10.1007/s11069-021-04679-9
Vilibić I., Šepić J. (2017). Global mapping of nonseismic sea level oscillations at tsunami timescales. Sci. Rep. 7, 40818. doi: 10.1038/srep40818
Vilibić I., Šepić J., Dunić N., Sevault F., Monserrat S., Jordà G. (2018). Proxy-based assessment of strength and frequency of meteotsunamis in future climate. Geophys. Res. Lett. 45, 10501–10508. doi: 10.1029/2018GL079566
Vilibić I., Šepić J., Rabinovich A. B., Monserrat S. (2016). Modern approaches in meteotsunami research and early warning. Front. Mar. Sci. 3, 57. doi: 10.3389/fmars.2016.00057
Vučetić T., Barčot T. (2008). Records on ‘tidal wave’ that hit Vela Luka on 21 June 1978 (Vela Luka–Split: Municipality of Vela Luka and Institute of Oceanography and Fisheries), 80.
Wertman C., Yablonsky R., Shen Y., Merrill J., Kincaid C. R., Pockalny R. A. (2014). Mesoscale convective system surface pressure anoMalies responsible for meteotsunamis along the U.S. East Coast on June 13th 2013. Sci. Rep. 4, 7143. doi: 10.1038/srep07143
Keywords: meteotsunami hazard assessments, synoptic index, downscaling, climate uncertainty, climate projections
Citation: Denamiel C, Belušić D, Zemunik P and Vilibić I (2023) Climate projections of meteotsunami hazards. Front. Mar. Sci. 10:1167863. doi: 10.3389/fmars.2023.1167863
Received: 16 February 2023; Accepted: 26 July 2023;
Published: 15 August 2023.
Edited by:
Charitha Bandula Pattiaratchi, University of Western Australia, AustraliaReviewed by:
Alexander B Rabinovich, P.P. Shirshov Institute of Oceanology (RAS), RussiaCopyright © 2023 Denamiel, Belušić, Zemunik and Vilibić. This is an open-access article distributed under the terms of the Creative Commons Attribution License (CC BY). The use, distribution or reproduction in other forums is permitted, provided the original author(s) and the copyright owner(s) are credited and that the original publication in this journal is cited, in accordance with accepted academic practice. No use, distribution or reproduction is permitted which does not comply with these terms.
*Correspondence: Ivica Vilibić, aXZpY2EudmlsaWJpY0BpcmIuaHI=
Disclaimer: All claims expressed in this article are solely those of the authors and do not necessarily represent those of their affiliated organizations, or those of the publisher, the editors and the reviewers. Any product that may be evaluated in this article or claim that may be made by its manufacturer is not guaranteed or endorsed by the publisher.
Research integrity at Frontiers
Learn more about the work of our research integrity team to safeguard the quality of each article we publish.