- Shenzhen Key Laboratory of Marine Bioresource and Eco-environmental Science & Shenzhen Engineering Laboratory for Marine Algal Biotechnology, College of Life Sciences and Oceanography, Shenzhen University, Shenzhen, China
Introduction: Mangroves are a unique ecosystem, but are often affected by heavy metal pollution. Bacterial communities in mangrove sediments usually respond to heavy-metal pollution stress by expressing specific heavy-metal resistance genes (HMRGs).
Methods: Multivariate statistics were performed on Metagenomics as well as environmental data to evaluated the effects of Cu addition on the sedimentary bacterial community structure.
Results and discussion: Using metagenomics technology, we found that the Shannon andChao1 indices of natural sediments were significantly higher than in sediments with artificially added Cu. Addition of Cu significantly altered bacterial community structure and diversity at the phylum, class and order levels in sediments. The relative abundance of HMRGs in Cuadded sediments was lower than that in natural sediments. Environmental factors that affect bacterial communities also affect HMRGs. However, the contribution of heavy-metal pollution to the change in HMRG abundance was much lower than its contribution to the change in the bacterial community, indicating that the relationship between HMRG abundance and heavy-metal pollution is complex and needs to be comprehensively studied.
1 Introduction
Mangroves occur all over the world and represent a special ecosystem composed of woody plants distributed in tropical and subtropical tidal zones or transition zones between ocean and fresh water (Kathiresan and Bingham, 2001; Udechukwu et al., 2015). The unique acidic, organic-rich and hypoxic-reducing sedimentary environment of mangroves makes deposition of various pollutants from tides, rivers and rainstorms more likely than in the general intertidal zone (Alongi et al., 1993). Among these pollutants, heavy metals have attracted attention due to their high toxicity, non-degradability and bioaccumulation throughout the food chain (Dudani et al., 2017). Heavy metals, together with other environmental factors, influence the structure of sedimentary bacterial communities, which represent a vital component of the mangrove ecosystem (Hu et al., 2022). Changes in the sedimentary environment of mangroves can directly or indirectly affect the diversity, composition and metabolic activities of bacterial communities (Shannon et al., 2003; Lee et al., 2014; Echavarri-Bravo et al., 2015; Balakrishnan et al., 2017). When mangroves are polluted by excessive amounts of heavy metals, the microorganisms in sediments usually respond by expressing specific heavy-metal resistance genes (HMRGs). These genes have functions such as expelling heavy metals from cells via transporters, transforming heavy metals into non-toxic forms, heavy-metal precipitation and chelation, and biological adsorption (Bouskill et al., 2007; Gamal et al., 2019). The expression level of HMRGs in microorganisms can reflect their tolerance to heavy metals. Indeed, microbes with a more robust heavy-metal resistance system likely represent an opportunity for use in bioremediation of heavy metals in polluted environments (Roosa et al., 2014), including in mangroves.
Many studies have reported HMRGs in bacterial resistance systems, with specificity for cadmium (cadB), chromium (chrA), lead (pbrA), copper (cusA) and nickel (nikA) (Roosa et al., 2014; Chen et al., 2020), for example. However, there are few studies on the relationship between heavy metals and the representation of HMRGs in mangrove bacterial communities. Of all heavy metal pollution we chose Cu to add to natural mangrove sediments. This is because Cu was one of the most heavily polluted heavy metals in the mangrove sediment of South China (Chen et al., 2021; Hu et al., 2022), highlighting the importance of studying the response of the bacterial community to Cu. Moreover, previous studies have suggested that Hg and Cd, as well as Cu, were among the top three most widely recognized metals in heavy metal pollution studies of coastal wetlands (Li et al., 2022). However, adding Hg or Cd to the environment can cause serious pollution while Cu was less harmful and essential to some microorganisms (Zhang et al., 2020). Therefore, we chose Cu as the targeted heavy metal to explore how Cu addition to natural mangrove sediments changes bacterial community structure and the representation of HMRGs (at the DNA level) in these communities. The research results not only enrich our understanding of bacterial populations and bacterial HMRGs in mangrove sediments, but also have theoretical and practical significance for the bioremediation of pollution in mangroves.
2 Materials and methods
2.1 Cu-addition experiment
The Cu-addition experiment was carried out in the Golden Bay Mangrove Reserve in Beihai, Guangxi province (109.22˚ N, 21.42˚ E; ‘Beihai mangrove’ for short). Five sampling sites (GX1-5) in Beihai mangrove were selected in Bruguiera gymnorhiza mangrove forests. GX1, GX2, and GX3 are located at the same location, approximately 10 meters apart, while GX4 and GX5 are located at another location. Two locations were 100 meters apart with the same distance from the sea, approximately 50 meters. GX1, GX2 and GX3 were the control group with no artificial metal addition. GX4 was watered once a month with 1 L of 200 mg/L Cu solution. GX5 was watered once a month with with 1 L of 400 mg/L Cu solution. After three years of treatment, triplicate sedimentary samples were collected from all five sites; hence, a total of nine samples were collected from GX1, GX2 and GX3, and six samples from the Cu-added sites GX4 and GX5.
Surface sediments were collected in November 2020 in sealed polyethylene bags. The 15 surface sediment samples were stored in an incubator with ice packs and delivered to the laboratory within 2 h of sampling. Each sample was divided into three parts: the first part was sealed and stored at 4°C until further analysis; the second part was dried at 60°C for the determination of physical and chemical parameters; and the third part was sealed in dry ice and stored at -80°C for metagenome sequencing.
2.2 Analytical methods
2.2.1 Physicochemical properties of sediments
Following methods in Hu et al. (2022), a series of physicochemical parameters such as grain size composition, pH, total phosphorus (TP), and total nitrogen (TN) were measured. Grain size composition was measured with a Beckman Coulter LS 13320 particle size analyzer (Beckman Coulter Inc., Germany). Sediment pH was measured by pH meter, after mixing sediments with deionized water in a mass ratio of 1:5. Samples were oven dried under 55°C, grounded and desicated before TP and TN analyses. TN were determined using a PE 2400 II elemental analyzer (Perkin-Elmer, Waltham, Massachusetts, USA); TP was determined using an automatic discrete analyzer (Cleverchem 380; Dechem-Tech, Hamburg, Germany). For pH and grain size composition, all samples were measured in triplicate to check reproducibility and accuracy. For other analyses, the samples were measured in triplicate resulting in a relative deviation of less than 7%.
2.2.2 Measurements of total metals and their speciations
Contents of five heavy metals (Cr, Ni, Cu, Zn, and Pb) were analyzed in the sediment samples. These metals were selected based on their toxicity and spatiotemporal concentrations reported previously by Chen et al. (2021). Approximately 0.2 g of the dried sediment sample was digested in a microwave digestion system (Ethos One, Milestone, Italy) with a mixture of 7 mL 65% HNO3 and 1 mL 20% H2O2 (Feng et al., 2017). The volume was made up to 50 mL with Milli-Q water, and this mixture was used for the determination of the total concentration of heavy metals. Next, the speciation of heavy metals in sediments was characterized using the BCR sequential extraction method (Tessier et al., 1979; Hall et al., 1996; Hall and Pelchat, 1999; Rauret et al., 1999). The heavy metals were classified as an acid-extractable fraction (denoted CrAcid, NiAcid, CuAcid, ZnAcid and PbAcid), a reducible fraction (CrReduc, NiReduc, CuReduc, ZnReduc and PbReduc), an oxidizable fraction (CrOxid, NiOxid, CuOxid, ZnOxid and PbOxid) and a residue fraction (CrResid, NiResid, CuResid, ZnResid and PbResid) (Figure S1 shows the detailed analytical procedure). All heavy-metal solutions were tested by inductively coupled plasma optical emission spectroscopy (ICP-OES; Optima 7000DV, Perkin-Elmer, Waltham, MA, USA). All the samples were measure in triplicate resulting in a relative deviation of less than 10% and a recovery ranging of 85% - 110%.
2.2.3 Methods to evaluate heavy metal pollution
The heavy metal pollution assessment methods used in this study include a comprehensive potential ecological risk index (RI), based on the total amount of heavy metals (Hakanson, 1980), and the ratio of secondary phase to primary phase (RSP), based on the speciation of heavy metals (Wang et al., 2011; Zhang et al., 2019). The RI used both measured total concentrations and background concentrations of metals, thereby taking into account the sensitivity of biological communities and heavy-metal toxicity (Zhang et al., 2019).The RI was calculated using Equation (1):
where Ci is the measured content of metal i in sediment, and is the metal’s concentration in background sediment. We used pre-industrial metal contents as the background values (Cr=60mg/kg, Ni=30mg/kg, Cu=30mg/kg, Zn=80mg/kg, Pb=25mg/kg) (Hakanson, 1980). is the toxicity response coefficient of each metal as follows: Cr=2, Ni=2, Cu=5, Zn=1, Pb=5 (Hakanson, 1980). The RSP is generally used to distinguish natural and man-made sources of heavy metals (Wang et al., 2011). The formula for RSP is as follows:
where Msec represents the content of heavy metals in the secondary phase of soil, which is calculated from the sum of the acid-extractable fraction, reducible fraction and oxidizable fraction; and where Mprim represents the heavy-metal content in the primary phase and is calculated from the residue fraction. When RSP ≤ 1, there is no pollution; 1< RSP ≤ 2 represents slight pollution; 2< RSP ≤ 3 means moderate pollution; and RSP > 3 represents heavy pollution.
2.2.4 DNA extraction and metagenome sequencing
Sediment microbial DNA was extracted from 1 g fresh soil samples using the FastDNA spin kit for soil (MP bio, Santa Ana, CA, USA), according to the manufacturer’s instructions (Ren et al., 2016). The Qubit 2.0 fluorometer (Thermo Fisher Scientific) was used to assess the quality and purity of the DNA extracts. There were three replicates for each soil sample to obtain sufficient DNA for shotgun metagenome sequencing. An Illumina small fragment (350 bp) library was constructed and its effective concentration (>3 nM) determined using the Qubit 2.0 fluorometer, while its quality was assessed by qPCR. Paired-end sequencing was performed on an Illumina NovaSeq6000 sequencing platform at Berry Genomics Technology Co., Ltd., Beijing, China. Sequence data had been uploaded in the NCBI SRA (Sequence Read Archive) database with project number PRJNA945508.
2.2.5 Processing of sequencing data and analysis of bacterial community structure
Raw sequencing reads were filtered to enhance the reliability and quality of subsequent analysis, as described by Zhang et al. (2017). The obscure bases, adapter sequences, and reads that were below 50 bp were removed. The obtained clean reads were assembled into contigs using Megahit (https://hku-bal.github.io/megabox/) with the optimal k-mer parameter. Subsequently, the sequencing data was compared to the Gene Catalogue to identify unigenes. DIAMOND software (version 0.9.21) was used to BLAST the unigenes against the NR database (https://ftp.ncbi.nlm.nih.gov/blast/db/FASTA/), and the lowest common ancestor algorithm was used to annotate the sequences. The abundance and community structure of the samples were determined after assigning, as far as possible, kingdom, phylum, class, order, family, genus and species based on the annotation results. The alpha diversity of sediment microorganisms was assessed by calculating the Shannon (Magurran, 1988) and Chao1 indices (Chao, 1984) (using R vegan software). The Shannon index was calculated as follows:
where is the Shannon index of the microorganisms, and is the ratio of the ith species to the total number of species in the sample. The Chao1 index was calculated as follows:
where is the estimated Chao1 index, is the actual number of species, a is the number of species with an individual number of 1, and b is the number of species with an individual number of 2.
2.2.6 Annotation and abundance calculation for HMRGs of sediment microorganisms
Using DIAMOND software, the unigenes were compared with KEGG (https://www.kegg.jp/), eggNOG (http://eggnog5.embl.de/#/app/home), CAZYme (http://www.cazy.org/), CARD (https://card.mcmaster.ca/), PHI (http://www.phi-base.org/), GO (http://geneontology.org/) to obtain annotation information and gene abundance information for each sequence. Due to the large number of databases and huge quantity of annotation information, we selected data returned from the KEGG database, which integrates genomic, chemical and system function information, for subsequent analysis. To compare the abundance of each annotated gene across the various samples, the relative abundance was calculated as follows:
where G is the relative gene abundance, r is the number of reads of matched genes, and L is the length of gene fragments.
2.3 Quality assurance and quality control
Plastic utensils were mainly used for sampling; metal utensils were avoided as much as possible to avoid contamination of core sediments. All sampling instruments were cleaned with 5% nitric acid solution before use. All samples were tested in triplicate to check for reproducibility and to evaluate the precision of measurements.
2.4 Statistical analysis
Excel was used to record and process raw data on environmental factors, the structural composition of bacterial communities, and the relative abundance of annotated genes. Based on the species annotation and abundance distribution of sediment microorganisms at the phylum, class and order levels, species distribution maps at these taxonomic levels were generated using R. Spearman correlation analysis was conducted using the R vegan package to determine the correlation between various environmental factors in sediments and the dominant bacterial communities at the phylum, class and order levels. Canonical correlation analysis was carried out using R to reveal the dynamic relationship between heavy-metal pollution and bacterial communities at the phylum, class and order levels. By reference to the KEGG database, HMRGs were selected and the distribution map of bacterial HMRGs was drawn using R. Correlations between various environmental factors of sediments and bacterial HMRGs were determined by Spearman correlation analysis using R vegan. Canonical correlation analysis was conducted using R to reveal the relationship between heavy-metal pollution and the distribution of bacterial HMRGs in sediments.
3 Results and discussion
3.1 Distribution characteristics of heavy metal pollution in mangrove sediments
3.1.1 Physicochemical parameters of mangrove sediments
It can be seen from the physicochemical parameters (Table S1) that, at all five sites, the sediments were mainly silt in most cases, with the silt content ranging from 12.40% to 96.50%, while the content of clay was in the range 2.75%-17.30%, and the sand content ranged from 3.51% to 87.60%. TP ranged from 0.13 to 0.44 mg/g, while TN content ranged from 0.03% to 0.06%. The pH values of all sediment samples were moderately acidic, ranging from 6.51 to 6.99. The addition of Cu did not change the physicochemical parameters of GX4 and GX5 significantly (tested by single factor ANOVA; for all physicochemical parameters, P>0.05). This is consistent with the report of Cai et al. (2013), which showed that the addition of metals did not significantly affect soil grain size.
3.1.2 Speciation of heavy metals in mangrove sediments
Following Cu addition, the total Cu content in samples from sites GX4 and GX5 was significantly higher than in other samples (i.e. GX1-3), as expected. Thus, the content of Cu ranged from 4.79 to 40.06 mg/kg in the order GX5>GX4>GX1≈GX3≈GX2 (F=530.186 and P<0.001, according to single-factor ANOVA). Other metals showed variable patterns. For example, the content of Cr across all sediments ranged from 7.77 to 28.66 mg/kg in the order GX5≈GX3≈GX1≈GX2>GX4 (F=9.680; P=0.002). The Ni content ranged from 2.18 to 11.59 mg/kg and showed essentially the same order, i.e. GX5≈GX1≈GX3≈GX2>GX4 (F=4.065; P=0.033). For Zn, the content ranged from 2.58 to 15.14 mg/kg, but with a different order, i.e. GX5>GX4≈GX1≈GX2>GX3 (F=66.055; P<0.001). Pb content, which ranged from 2.59 to 9.88 mg/kg, also showed a different order, i.e. GX5>GX1≈GX3≈GX4≈GX2 (F=4.241; P=0.029).
The speciation patterns of Cr, Ni, Zn, and Pb did not much differ between the Cu-addition samples and control samples (Figure 1; Table S2). For example, in all samples Cr was mainly found in the residue fraction, followed by the reducible fraction, while there was relatively little of this metal in the acid-extractable and oxidizable fractions. Ni was mostly in the residue fraction, followed by the acid-extractable fraction, the reducible fraction and the oxidizable fraction. Zn was mainly identified in the acid-extractable fraction, followed by the reducible fraction, the oxidizable fraction and the reducible fraction. Finally, Pb was present mainly in the reducible fraction, followed by the oxidizable fraction, the residue fraction and theacid-extractable fraction. However, Cu speciations differed between the Cu-addition and natural samples. In the natural samples, i.e. GX1, GX2 and GX3, Cu was mainly in the reducible and residue fractions, followed by the oxidizable fraction and the acid-extractable fraction. In the Cu-addition samples, GX4 and GX5, Cu was mainly found in the reducible fraction, followed by the acid-extractable fraction, the residue fraction and the oxidizable fraction. The differences in speciation fractions were expected since the more active Cu2+ solution CuSO4 were added in GX4 and GX5.
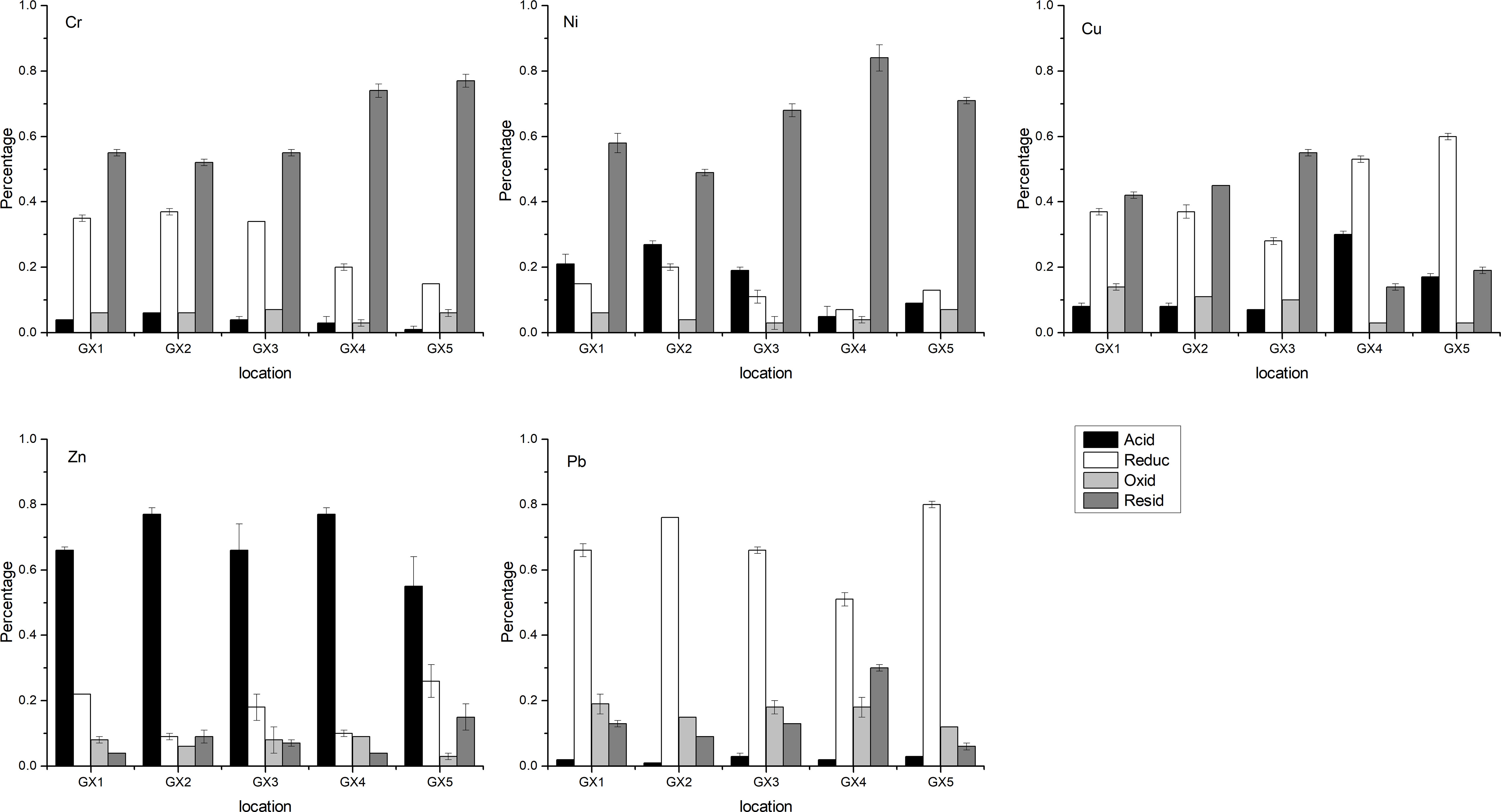
Figure 1 Distribution of the various speciations of Cr, Ni, Cu, Zn and Pb. Black represents the acid-extractable fraction, white the reducible fraction, light gray the oxidizable fraction, and grey the residue fraction.
The total heavy-metal content in the natural samples GX1, GX2, GX3 was much lower than the background levels of heavy metals in Guangdong Province (Table S3). A possible explanation for this observation is that the mangroves in the Beihai mangrove are far from urban development centers and therefore the pollution caused by human activities is not severe. In the natural sediments of Beihai mangrove, Cr and Ni are mainly in the residue fraction, while Pb and Cu are predominantly in the reducible fraction (Figure 1), which is consistent with the findings of Cordeiro et al. (2015) in Guanabara Bay, Brazil. In addition to the reducible fraction, Cu is mainly found in the residue fraction in GX1, GX2 and GX3, indicating that the artificially added Cu in GX4 and GX5 mainly exists in the reducible fraction. It can be seen that under different conditions, the relative preponderance of a particular heavy metal species may change; in this context, it should be noted that there is the possibility of mutual transformation among the various heavy metal species (Ramos et al., 1999; Jayachandran et al., 2018).
3.1.3 Assessment of heavy metal pollution in mangrove sediments
The potential ecological risk assessment results for heavy metals in the mangrove sediments of Beihai mangrove are shown in Table 1. It can be seen from the table that in GX1 RI-Pb had the highest value at 1.56, while RI-Zn had the lowest value at 0.08. In GX2, the values for RI-Ni and RI-Cu were the highest, both being 0.94; RI-Zn had the lowest value at 0.07. In GX3, RI-Cu was the highest, with a value of 1.19, while RI-Zn showed the lowest value at 0.05. In GX4, RI-Cu was the highest, with 5.00; RI-Cr returned the lowest value at 0.30. In GX5, RI-Cu had the highest value at 6.43, while RI-Zn was lowest at 0.18. It can be seen that all samples fall into the slight ecological risk category, in the following order: GX5>GX4>GX1>GX3>GX2.
RSP, the ratio of secondary phase to primary phase of the various fractions of metals, allows natural sources of heavy metals to be distinguished from anthropogenic sources (Table 2). Our results showed that all samples were heavily polluted by Zn and Pb. As expected, the Cu addition sites GX4 and GX5 were heavily polluted by Cu, while the three natural sites showed no Cu pollution. There was no Cr pollution at any of the sites tested.
All sites presented a slight ecological risk because of the heavy pollution with Zn and Pb, consistent with the observations that the residue fractions of both Zn and Pb were relatively low compared to the other three fractions (Table 2). A possible reason is that Beihai mangrove is far from urban areas, but the booming tourism industry and increased commercialization in the area in recent years may also cause man-made Zn and Pb pollution. Pb pollution mainly comes from automobile exhaust emissions and battery processing (Prosi, 1989), while Zn pollution largely results from coal combustion, automobile tire wear and manufacturing machinery (Shi et al., 2019). In summary, the relatively high RSP values for Zn and Pb observed in this study represent a significant ecological risk that cannot be ignored in Beihai mangrove.
3.2 Relationship between bacterial diversity and heavy metal pollution in mangrove sediments
3.2.1 Bacterial community structure in mangrove sediments
Metagenomic sequencing and annotation of all samples in this study revealed a total of seven kingdoms, 183 phyla, 186 classes, 443 orders, 855 families, 2,756 genera and 15,779 species (excluding unmatched and unclassified). The top 20 bacterial phyla in the mangrove sediments of Beihai mangrove are shown in Figure 2A. The average proportion of the top 10 bacterial phyla ranked as follows: Proteobacteria > Bacteroidetes > Chloroflexi > Planctomycetes > Actinobacteria > Gemmatimonadetes > Cyanobacteria > Acidobacteria > Verrucomicrobia > Ignavibacteriae; their average proportions were 64.14%, 7.89%, 4.55%, 4.28%, 4.09%, 3.41%, 3.07%, 2.46%, 1.47% and 0.67%, respectively. The top 20 bacterial classes are shown in Figure 2B and the average proportion of the top 10 bacterial classes ranked as follows: Alphaproteobacteria > Gammaproteobacteria > Deltaproteobacteria > Betaproteobacteria > Gemmatimonadetes > Flavobacteriia > Anaerolineae > Actinobacteria > Actinobacteria > Acidimicrobiia; their average proportions were 26.87%, 14.55%, 13.44%, 5.13%, 4.06%, 3.38%, 3.07%, 2.36%, 1.92% and 1.39%, respectively. The top 20 bacterial orders are shown in Figure 2C and the average proportion of the top 10 orders ranked as follows: Rhodobacterales > Sphingomonadales > Planctomycetia > Myxococcales > Gemmatimonadetes > Flavobacteriales > Rhizobiales > Desulfobacterales > Chromatiales > Desulfuromonadales > Desulfuromonadales; their average proportions were 11.42%, 5.57%, 4.95%, 4.50%, 4.30%, 3.88%, 3.52%, 3.40%, 3.20% and 2.40%, respectively.
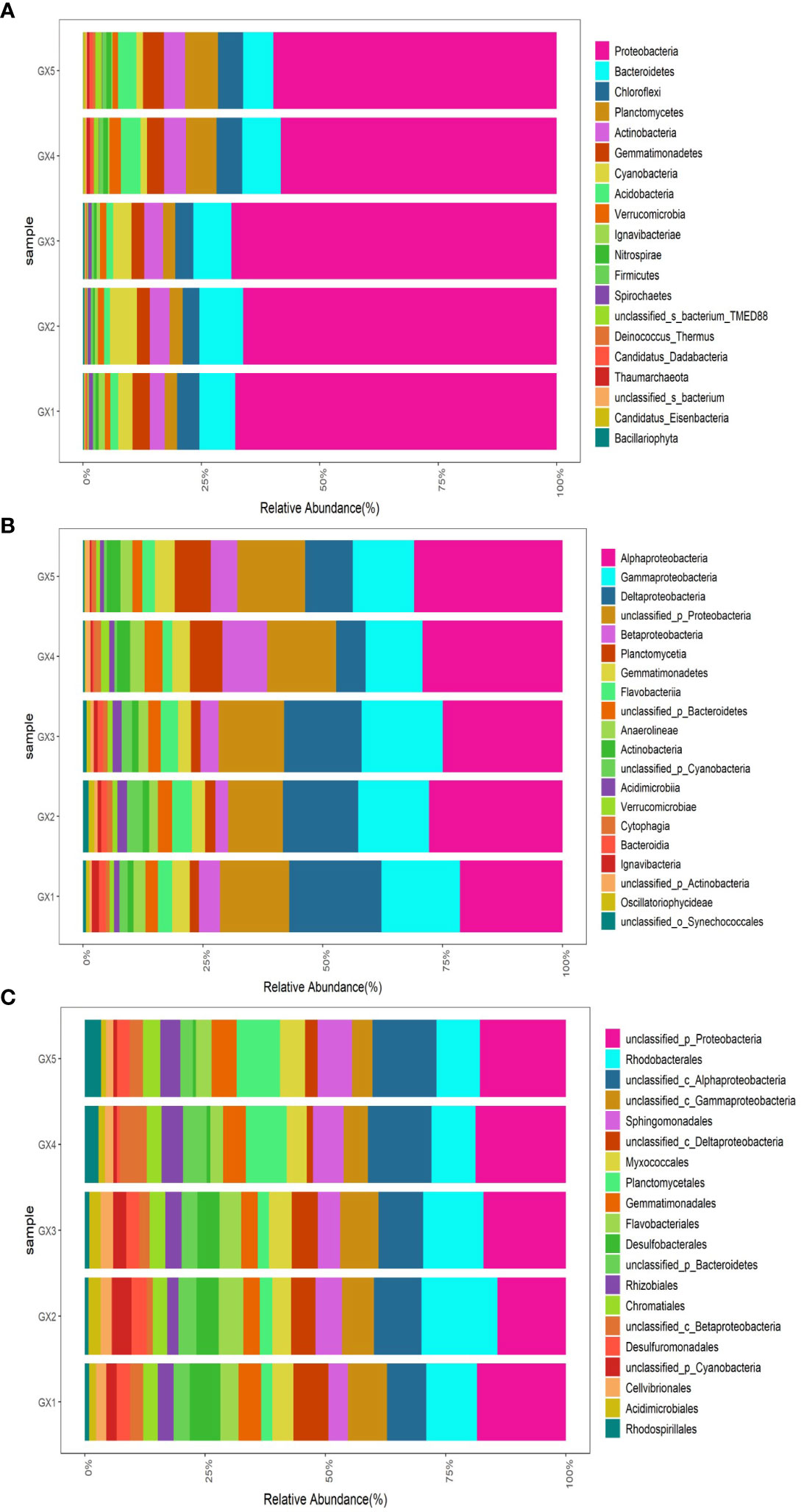
Figure 2 The proportion of top 20 microbial communities at the phylum (A), class (B) and order (C) level in the sediments of Beihai mangrove.
In the sediments of Beihai mangrove, the dominant bacterial communities at the phylum level were Proteobacteria, Bacteroidetes and Chloroflexi, which is consistent with the report of Imchen et al. (2017), who found similarly dominant bacterial communities at the phylum level at various sites in four different mangroves in India. These workers found Proteobacteria, Bacteroidetes and Actinobacteria to be the dominant groups of bacteria, although the ranking order in terms of proportion of the community differed slightly across the various sites. Nevertheless, Imchen et al. found that Proteobacteria was the most prevalent group of bacteria at multiple locations. In our study, Proteobacteria was also the predominant bacterial group in both natural and Cu-added sediments, accounting for ~64% of the total, a much larger proportion than other bacterial phyla.
The dominant bacterial communities at the class level in sediments are Gammaproteobacteria, Deltaproteobacteria, Alphaproteobacteria and Betaproteobacteria among the Proteobacteria; Flavobacteriia in phylum Bacteroidetes; and Gemmatimonadetes in Gemmatimonadetes, while the ranking order of community proportion in each sample was slightly different. Wang et al. (2020) reported similar findings when studying mangrove sediments in Longhai, China. They found that the dominant bacterial communities at the class level in all sediments were also Gammaproteobacteria, Deltaproteobacteria, Alphaproteobacteria, Anaerolineae, Gemmatimonadetes, and so on, yet the proportion of each group differed from our findings.
At the order level, the dominant bacterial community was Rhodobacterales in class Alphaproteobacteria, although the proportions were similar to those of other dominant bacterial groups, albeit different in each sample. Liu et al. (2019) also found Rhodobacterales to be the dominant microflora in sediments from different areas during a study of mangroves in Hainan.
3.2.2 Alpha diversity in mangrove sediments
The Shannon diversity of sediment microorganisms in mangroves in Beihai mangrove is shown in Figure 3. The Shannon index of GX3 was the highest among all samples at 21.92, while GX4 had the lowest value at 21.15. The Shannon index values of GX1, GX2 and GX3 (natural sediments) were all significantly higher than those of GX4 and GX5 (Cu addition). The Chao1 index calculations for sediment microorganisms are shown in Figure 3. The pattern of Chao1 index values for all samples replicated that for the Shannon index, with the Chao1 index of GX3 having the highest value and GX4 the lowest value. Similarly, the Chao1 index values for GX1, GX2 and GX3 (natural sediments) were all significantly higher than those for GX4 and GX5 (Cu addition).
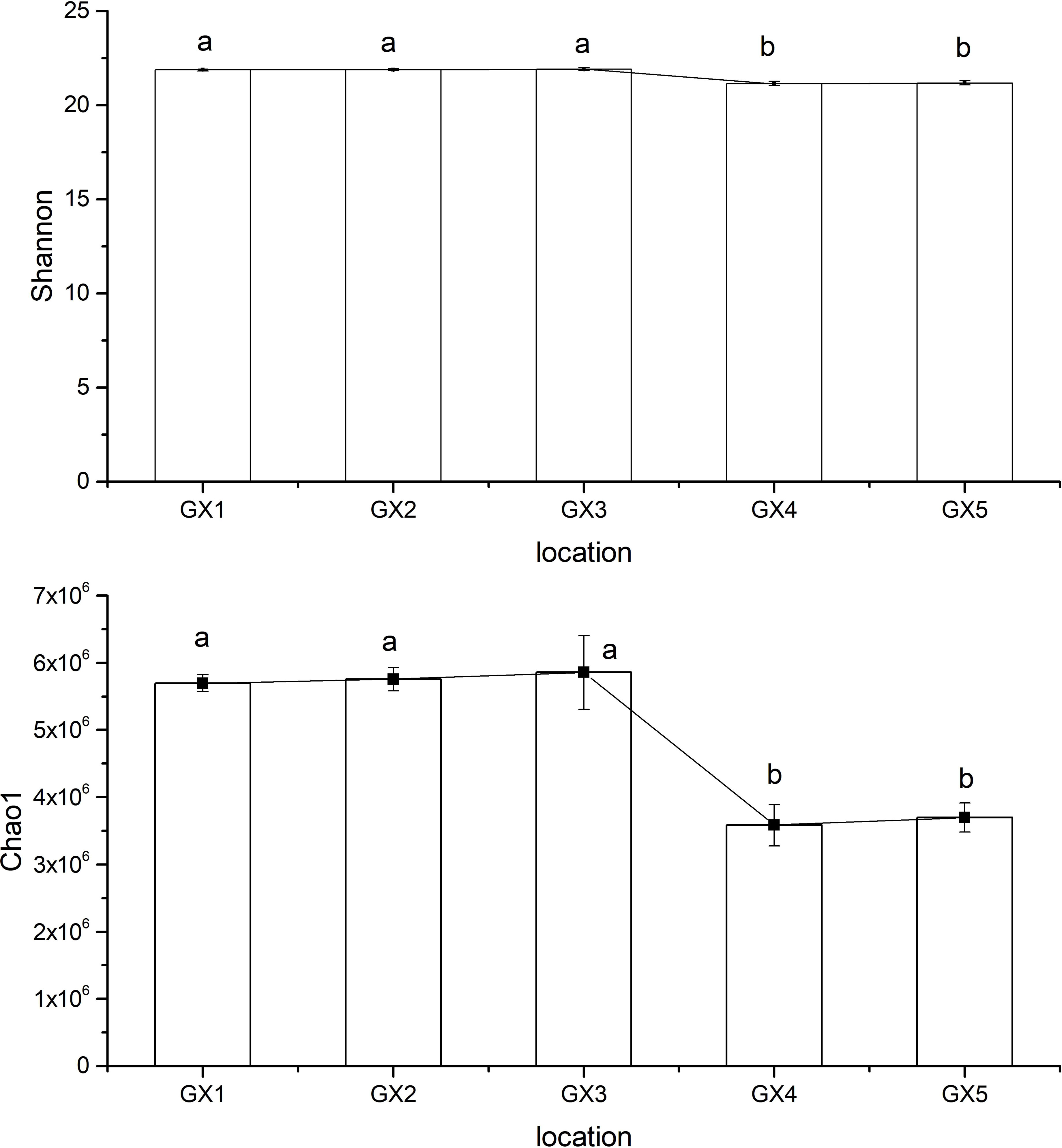
Figure 3 Shannon index (Top) and Chao1 index (Bottom) of sedimentary bacterial community in Beihai mangrove; (a, b) indicate significant differences between samples. Data were evaluated by single-factor ANOVA and were divided into two groups (a, b). Data in group (a) were significantly higher than data in group (b).
The Shannon diversity index can reflect community richness and community homogeneity, while the Chao1 index is more sensitive to the presence of rare communities within the overall bacterial population. Hence, the abundance and number of rare communities in GX1, GX2 and GX3 (natural sediments) in Beihai mangrove were much higher than those in GX4 and GX5 (Cu addition). A possible reason for this observation is that the high concentration of Cu added to the GX4 and GX5 sediments over a short period of time had a significant impact on the diversity of the bacterial community. Low heavy-metal content can stimulate bacterial growth, while high heavy-metal content is likely to inhibit bacterial growth (Wu et al., 2019). Pradhan et al. (2020) also found that a short period of heavy-metal pollution significantly reduced bacterial diversity, possibly because the bacterial community was unable to adapt to the stress induced by this pollution. On the other hand, the variation in trend of both the Shannon index and the Chao1 index across the various sediments from Beihai mangrove is consistent, indicating that the richness of the overall bacterial community is mainly determined by the number of rare species.
3.2.3 Correlation analysis of heavy metal pollution and bacterial diversity
Spearman correlation analyses were performed to determine the relationships between heavy-metal pollution and bacterial diversity (Table 3). The Shannon index of sediment microorganisms was significantly positively correlated with TN, CrAcid, NiAcid, CrReduc and CrResid and significantly negatively correlated with total Cu, total Zn, CuAcid, CuReduc, CuOxid, CuResid, ZnAcid, ZnResid, RI-Cu, RI-Zn and RI. The Chao1 index was significantly positively correlated with NiAcid, CrReduc, TN and CrResid, and significantly negatively correlated with total Cu, CuAcid, CuReduc, CuOxid, CuResid, ZnAcid, ZnResid, RI-Cu, RI, Zn and RI-Zn.
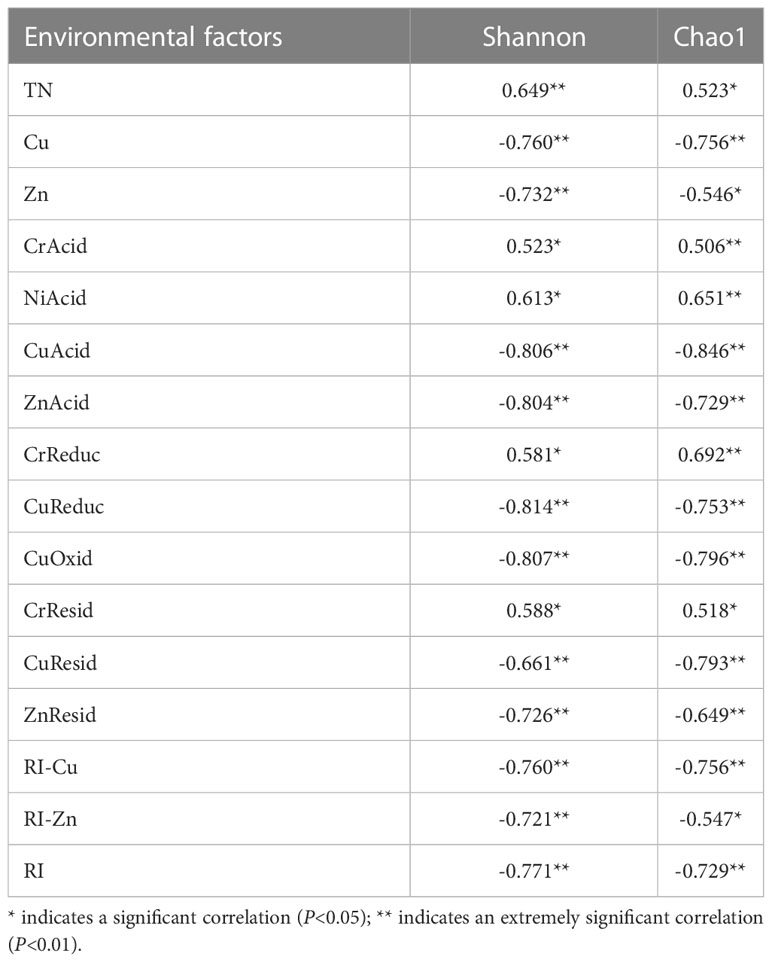
Table 3 Correlation coefficient (r) values from Spearman correlation analysis between the environmental factors and alpha microbial diversity index in Beihai mangrove.
It can be seen that the bacterial diversity index of sediments in Beihai mangrove is affected by various environmental factors. The Shannon index can represent the diversity and homogeneity of community distribution, while the Chao1 index mainly reflects the number of rare bacterial communities. We observed that both the Shannon and Chao1 indices were negatively correlated with the total amount of Cu and its speciations, the total amount of Zn, ZnAcid, ZnResid, RI-Cu, RI-Zn and RI. This is consistent with the findings of Pradhan et al. (2020), which showed that heavy-metal contamination can affect the number of rare microorganisms in soil.
Spearman correlation analyses were further performed to determine the relationships between metal pollution and the dominant bacterial communities at the phylum, class and order levels (Table 4). The dominant bacterial community at the phylum level in the mangrove sediments of Beihai mangrove is affected by various environmental factors (Table 4a). Proteobacteria, the phylum that forms the largest proportion of the overall community, is associated with pH, total amount of Cu, total amount of Zn, CrAcid, NiAcid, CuAcid, PbAcid, CrReduc, CuReduc, ZnReduc, CrResid, CuResid, ZnResid, RI- Cu, RI-Zn and RI. These results indicate that the bacterial community representing the largest proportion of the overall population at the phylum level was more obviously affected by heavy-metal pollution than the dominant taxonomic groups defined at other levels (i.e. class and order). Moreover, we found that when the total amount of heavy metals was correlated with the dominant bacterial community, some species of heavy metals were also correlated with this community. On the other hand, the metal species could be correlated with the dominant bacterial community even if the total amount of an individual heavy metal showed no such correlation (Oliveira and Pampulha, 2006). Therefore, metal speciations seemed to be more important environmental factors than total heavy-metal contents in terms of their influence on the bacterial community.
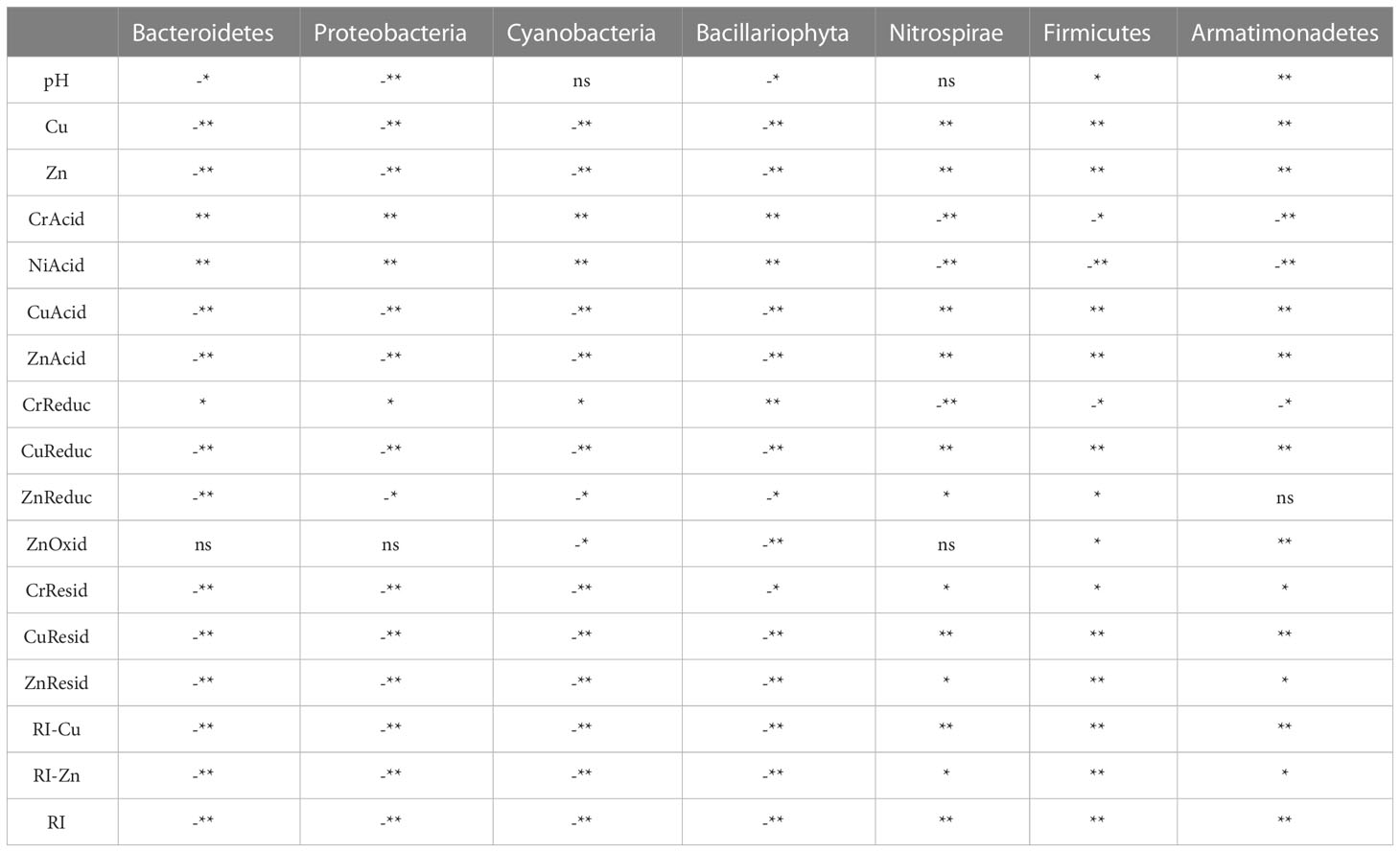
Table 4a Spearman correlation between heavy metal pollution and dominant microbial communities at the phylum level in Beihai mangrove.
We also be found that Proteobacteria and Bacteroidetes are negatively correlated with most of the environmental factors in the Beihai mangrove, while Armatimonadetes and Nitrospirae are positively correlated with most environmental factors. These observations indicate that heavy-metal pollution can have different effects on different bacterial communities at the same level (Oliveira and Pampulha, 2006; Basak et al., 2016). The diversity of some bacterial communities increased under the influence of heavy metal pollution, while conversely the diversity of other bacterial communities decreased. This might be explained by the different physiological and genetic characteristics of different bacterial communities. For example, a biotin-binding protein called bacterial avidin is found mainly in Proteobacteria and Bacteroidetes, which might be an important factor in the resistance of these taxonomic groups to environmental change (Laitinen et al., 2021). Compared with other dominant bacterial communities, the correlation in all samples between Nitrospira and environmental factors was extremely significant (P<0.01) (Table 4b). However, Nitrospira accounts for only a very small proportion of the top 20 bacterial communities. A possible reason for these correlations is that, compared with other heterotrophic dominant groups, Nitrospira species are more sensitive to toxic substances such as heavy metals (Ferhan et al., 2010). Thus, Pb inhibits bacterial growth by damaging the cell membrane and interrupting the transport of nutrients. Cu can interfere with proteins or enzymes in bacterial cells. Oxidative stress imposed on Nitrospira by redox reactions involving Cr can damage proteins and DNA (Belliveau et al., 1987; Yeager, 1991; Vaiopoulou and Gikas, 2012). At the order level, we observed the same pattern as at the phylum and class levels, i.e. that the metal speciations influence the make-up of the bacterial community more than the total amount of each heavy metal (Table 4c). Thus, taking all the above correlation analyses into account, it can be concluded that the influence of heavy metal speciation on the sediment microflora is much greater than that of the total amount of heavy metals. This is consistent with the report of Wasilkowski et al. (2014) and suggests that heavy metal speciation is a key factor affecting bacterial community structure.
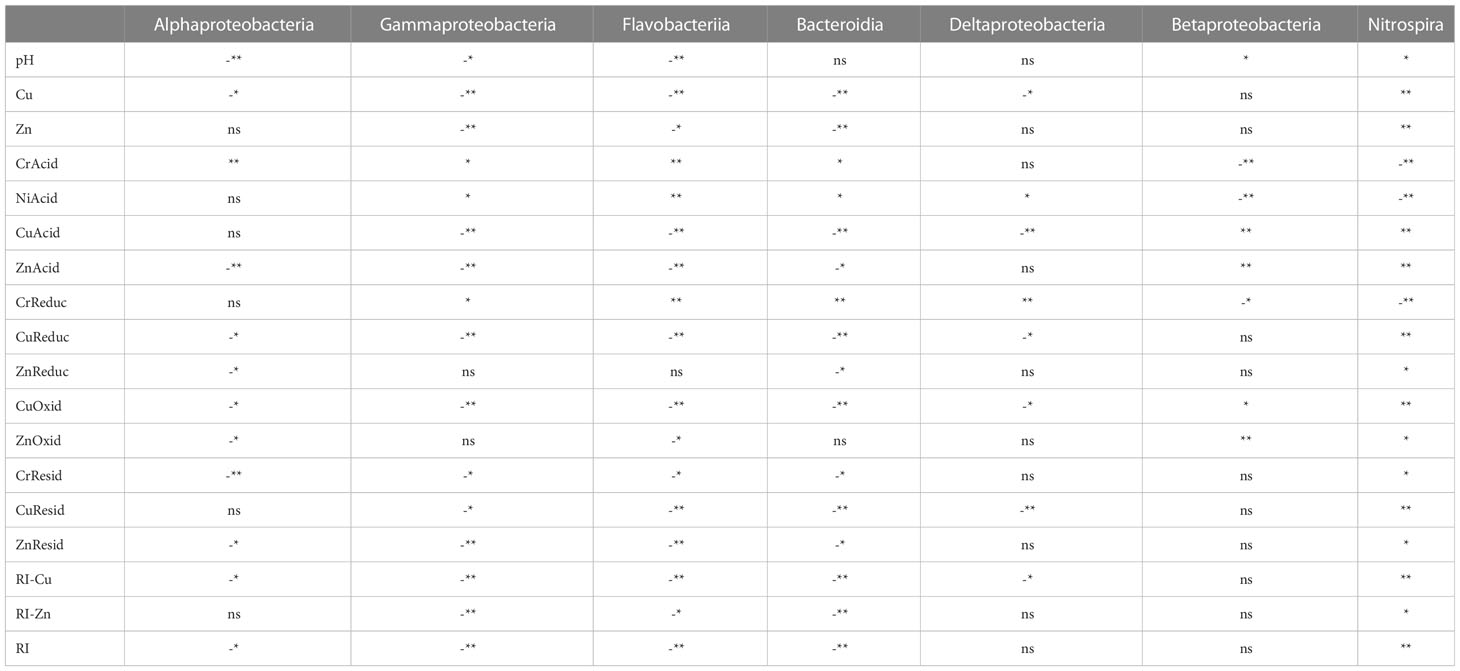
Table 4b Spearman correlation between heavy metal pollution and dominant microbial communities at the class level in Beihai mangrove.
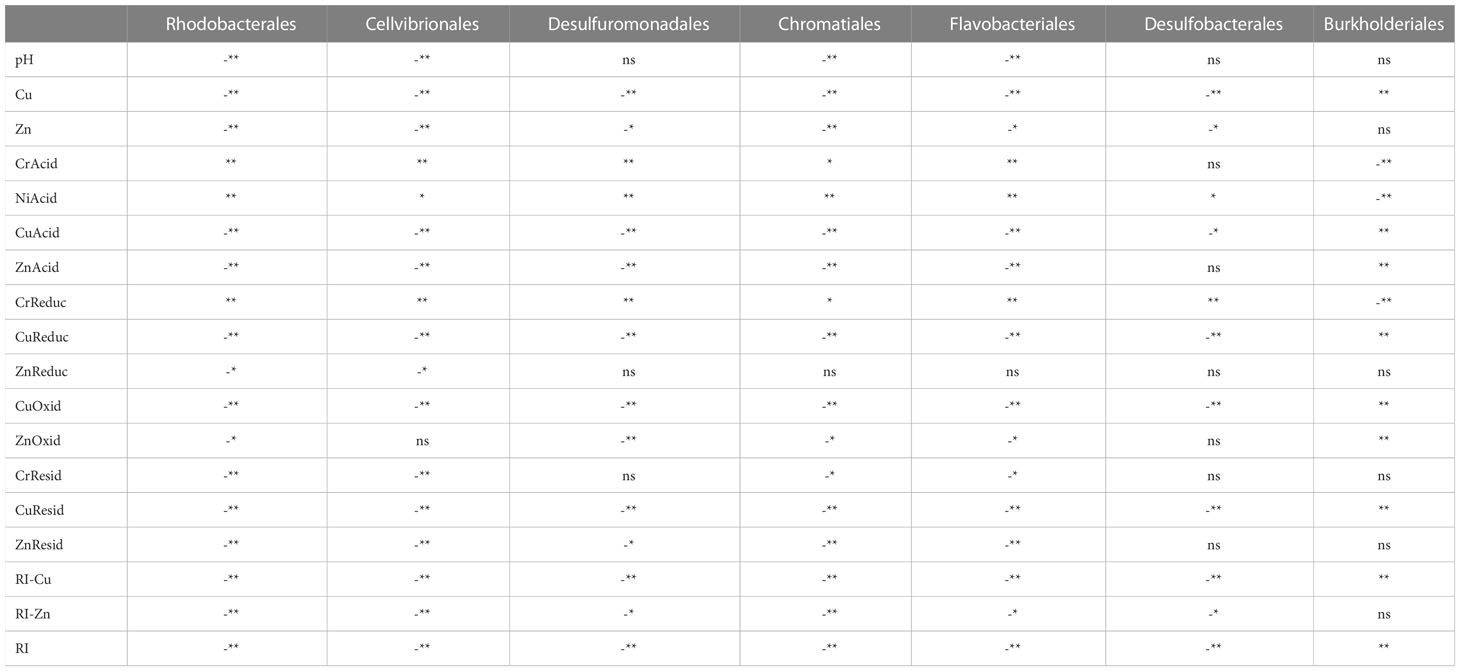
Table 4c Spearman correlation between heavy metal pollution and dominant microbial communities at the order level in Beihai mangrove.
3.2.4 Canonical correlation analysis of heavy metal pollution and bacterial diversity in mangrove sediments
Canonical correlation analysis (CCA) was used to analyze the impact of environmental factors on the bacterial community. Environmental factors with significant correlations (P<0.05) to the bacterial community variables were selected to draw the arrows in Figure 4 and the length of the arrows represents the intensity of the influence of the environmental factor on the bacterial community. CCA 1 and 2 contributed 85.57% of the bacterial community variation at the phylum level. At the phylum level, GX4 and GX5 were mainly affected by the total amount of Cu and RI-Cu, while GX1, GX2 and GX3 were mainly affected by clay, silt, sand, RI-Cr and RI-Ni (Figure 4A). At the class level, CCA 1 and 2 contributed 82.44% of the bacterial variation (Figure 4B). GX4 and GX5 were mainly affected by the total amount of Cu, RI-Cu, the total amount of Zn, and RI-Zn; GX1, GX2 and GX3 were mainly affected by sand, RI-Cr and RI-Ni. At the order level, CCA 1 and 2 contributed 82.28% of the bacterial variation (Figure 4C). GX4 and GX5 were mainly affected by the the total amount of Cu, RI-Cu, the total amount of Zn and RI-Zn; GX1, GX2 and GX3 were mainly affected by sand, RI-Cr and RI-Ni.
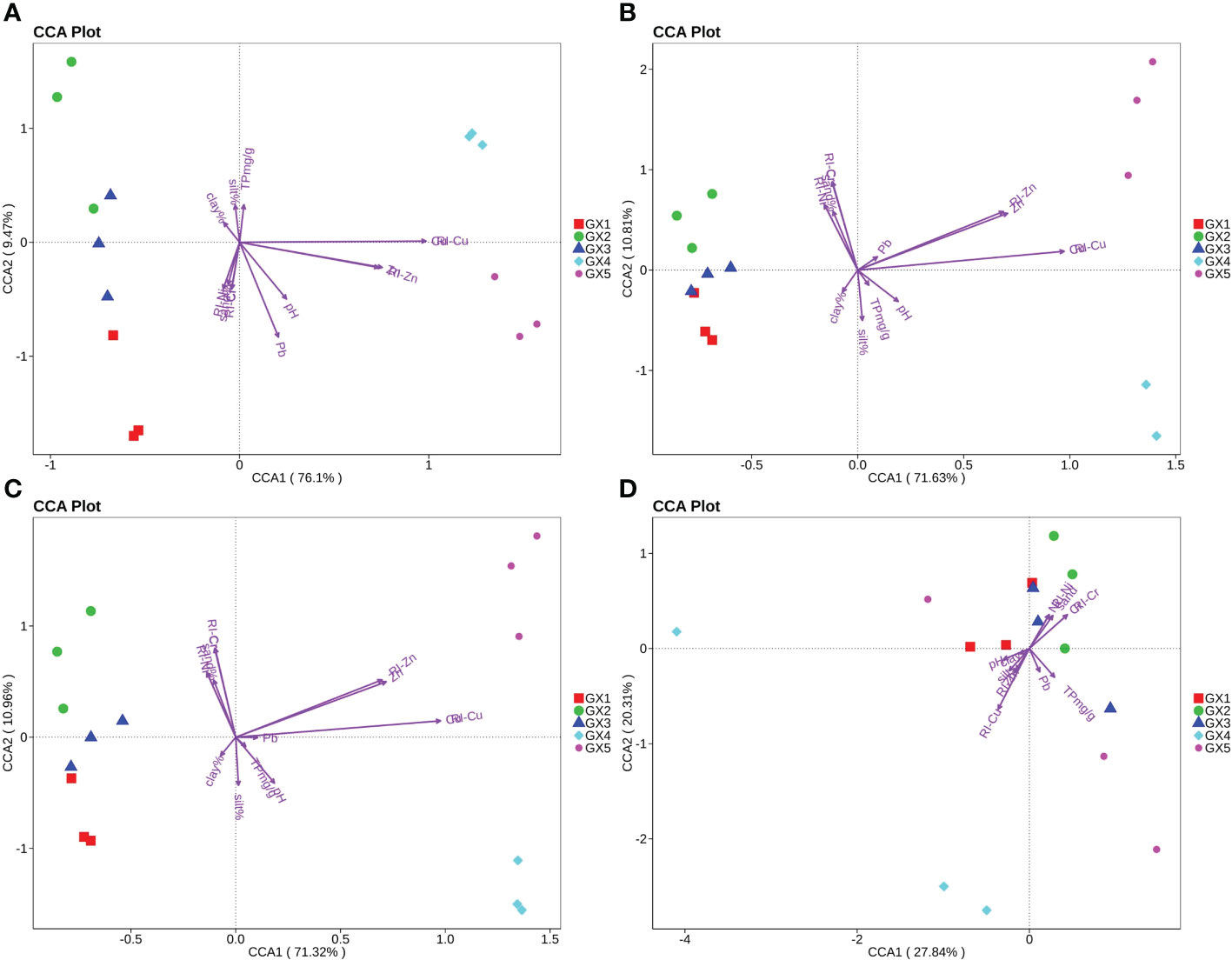
Figure 4 CCA analysis of correlation between heavy metal pollution and bacterial communities at phylum (A), class (B), and order (C) levels and bacterial HMRGs (D) in Beihai mangrove.
CCA analyses indicated that the effects of environmental factors on the bacterial community structure of Beihai mangrove sediments were different at the level of phylum, class and order. In natural sediments GX1, GX2 and GX3, the bacterial community structure was mainly affected by grain size (especially the content of sand), RI-Cr and RI-Ni. In GX4 and GX5 (Cu addition), the bacterial community structure at the level of phylum, class and order was mainly affected by the total amount of Cu, RI-Cu, the total amount of Zn and RI-Zn, indicating that the artificial addition of Cu over a short period can significantly change the bacterial community structure at various levels. The contributions of heavy-metal pollution to the bacterial community changes at phylum, class and order levels were all more than 80%, indicating that heavy-metal pollution was the key factor affecting the bacterial community structure of Beihai mangrove. On the other hand, we observed that the variations in bacterial community structure were more related to RI-metal values than metal speciations, which was not consistent with the results of the correlation analyses. One explanation might be that the correlation analysis determines the influence of a single environmental factor on the dominant bacterial flora, while the CCA analysis reflects the key factors that affect all bacterial communities. Nevertheless, our observations suggest that the RI values of metals play important roles in bacterial community structure variation. Li et al. (2021) reported similar findings when studying forest sediments, i.e. that RI index values accurately quantify the toxicity level of heavy metals and reflect the changes in bacterial community structure much more clearly than the total amount of specific metals. In addition to the effect of heavy metals, we found that grain size, the content of organic matter and pH also affect the bacterial community structure in sediments (Lee et al., 2014; Zhou et al., 2017). Indeed, grain size plays an important role in our samples, possibly because sediments with different particle sizes have different structural compositions, which affect the biogeochemical processes that microorganisms participate in, and thereby the bacterial community structure (Huang et al., 2002).
3.3 Relationship between bacterial HMRGs and heavy metal pollution in mangrove sediments
3.3.1 Relative abundance of bacterial HMRGs in sediments
In this study, the same 4,143 genes annotated by reference to the KEGG database were found across all 15 sediment samples. Of these, 13 bacterial HMRGs that were highly represented and widely distributed in all samples were selected for further study. Coincidentally, these 13 HMRGs were mentioned as the most common genes in contaminated sediments by a previous review Roosa et al. (2014). These comprised two Cr-resistance genes (chrA and chrR), three Ni-resistance genes (cnrA, ddpA and nccA), three Cu-resistance genes (copB, cusA and cusB), three Zn-resistance genes (czcB, czcD and pqqL) and two Pb-resistance genes (pbrB and pbrT). The heavy-metal resistance genes and their corresponding gene numbers in the KEGG database are shown in Table S4.
The distribution of bacterial HMRGs in Beihai mangrove sediments is shown in Figure 5. Twelve of the selected HMRGs (with the exception of pbrT, which was not present in GX4) were found in all samples, with the relative abundance of cnrA, ddpA, copB, cusA, cusB and pqqL being higher than other genes. The relative abundance of chrA and pbrT was highest in GX1 with values of 0.0115 and 0.0015, respectively, while chrR, ddpA, nccA, cusB, czcB and pqqL showed the highest relative abundance in GX2. The relative abundance of cnrA, copB, cusA, czcD and pbrB was highest in GX3. The relative abundance of chrA, chrR, cnrA, nccA, cusA, czcB, czcD and pqqL was lowest in GX5, while the relative abundance of ddpA, copB, cusB and pbrB was lowest in GX4. It can be seen that the abundance of all HMRGs in GX1, GX2, and GX3 (natural sediments) was higher than that in GX4 and GX5 (Cu addition).
The observation that HMRGs in Cu-added sediments were less abundant than that in natural sediments seems to be contradicted by the fact that an increase in heavy metal content results in a greater representation of HMRGs (Chen et al., 2019). On the other hand, this is consistent with the bacterial diversity index of GX1, GX2 and GX3 being higher than that of GX4 and GX5 (Figure 3). We speculate that the relative paucity of HMRGs in GX4 and GX5 is likely to be related to the decrease in bacterial diversity in these samples. Sun et al. (2021) reported a similar finding that the distribution of HMRGs varies with changes in bacterial diversity. The HMRG with the highest relative abundance across all sediments was cusA, with values ranging from 0.0279 to 0.0935. This is consistent with the report of Imchen et al. (2018), who found that the abundance of HMRGs varied in mangrove sediments with different heavy metal contents (India, Brazil, and Saudi Arabia), yet cusA was always the most abundant in all sediments. This gene is involved in the dual-regulation mechanism of copper resistance in Escherichia coli, and many regulatory genes (such as cusR and cusS, etc.) control its expression (Munson et al., 2000). Moreover, cusA forms part of a complex that directly exports Cu from the cell, and is preferentially expressed compared with other Cu-resistance genes in bacterial communities (Besaury et al., 2014). At the same time, the relative abundance of ddpA and pqqL in sediments was only lower than that of cusA, which may be because ddpA encodes the binding protein of an ABC-superfamily transport pump (nickel transport system) in E. coli (Haddadin and Harcum, 2010), while pqqL encodes a periplasmic zinc metalloproteinase (Grinter et al., 2019). The relative abundance of chrA, chrR, czcB, czcD and pbrT was significantly lower than other HMRGs. It may be that chrA and chrR are present in Bacillus amyloliquefaciens and Pseudomonas putida, respectively, species that are sensitive to Cr6+ but not Cr3+ (Chihomvu et al., 2015). The czcB and czcD genes are found in Cupriavidus (Ralstonia) metallidurans and jointly encode RND heavy-metal efflux pumps; they can generate comprehensive resistance to Zn and Cd, although their sensitivity to Cd is greater than that to Zn (Legatzki et al., 2003). However, none of the samples tested in this study contained Cd and consequently there were few Cd-related HMRGs. pbrT was the least distributed HMRG in all sediments, which may be attributed to the fact that pbrT is found on an endogenous plasmid (pMOL30) in C. metallidurans CH34, which belongs to the Burkholderiaceae family in the order Burkholderiales. Burkholderiales was not one of the top 20 bacterial communities at the order level in any sediment sample, accounting for a very small proportion of the overall community.
3.3.2 Spearman correlation analysis of heavy metal pollution and bacterial HMRG representation
Spearman correlation analysis showed that there was no correlation between the abundance of individual bacterial HMRGs and the physicochemical parameters of sediments, total amount of Pb and its speciation and RSP; therefore, only correlations between HMRG levels and Cr, Ni, Cu, Zn and their speciations, and RI values are presented (Figure 6). For Cr and its speciations, chrA was negatively correlated with the total amount of Cr, pqqL was negatively correlated with CrResid, and cusA and ddpA were positively correlated with CrAcid (Figure 6A). For Ni and its speciations, cusB and ddpA were significantly positively correlated with NiAcid (Figure 6B). Many correlations were observed between Cu and its speciations and HMRGs (Figure 6C). For example, pqqL, ddpA and cusA were significantly negatively correlated with the total amount of Cu and its speciations; cnrA was significantly negatively correlated with the total amount of Cu, CuReduc, and CuOxid; cusB was significantly negatively correlated with Cu and its speciations; copB was significantly negatively correlated with Cu and all its speciations; pbrB was negatively correlated with the total amount of Cu, CuAcid, CuReduc and CuOxid; czcB was negatively correlated with the total amount of Cu, chrR was negatively correlated with CuResid, nccA was negatively correlated with CuAcid, CuReduc and CuOxid, and cnrA was negatively correlated with CuReduc; cusB was negatively correlated with the total amount of Cu, and copB was negatively correlated with the total amount of Cu, CuAcid and CuReduc. For Zn and its speciations, pqqL and ZnAcid were significantly negatively correlated; nccA was negatively correlated with ZnAcid; cusB was negatively correlated with ZnAcid and ZnOxid; cusA was negatively correlated with the total amount of Zn, ZnAcid and ZnResid; cnrA was negatively correlated with the total amount of Zn, ZnAcid, ZnReduc and ZnResid; pqqL was negatively correlated with the total amount of Zn; ddpA was negatively correlated with ZnAcid and ZnResid; and czcB was negatively correlated with ZnResid. Moreover, correlation analyses were conducted between metal RI values and HMRGs and only negative correlations were observed. For example, cusA was significantly negatively correlated with RI-Zn; pqqL, ddpA and cusA were significantly negatively correlated with RI and RI-Cu, and cnrA was significantly negatively correlated with RI. The cusB and copB genes were negatively correlated with RI and RI-Cu, while pqqL was negatively correlated with RI-Zn, cnrA was negatively correlated with RI-Cu and RI-Zn, and chrA was negatively correlated with RI-Cr. In contrast, czcD and pbrT were not associated with any environmental factors.
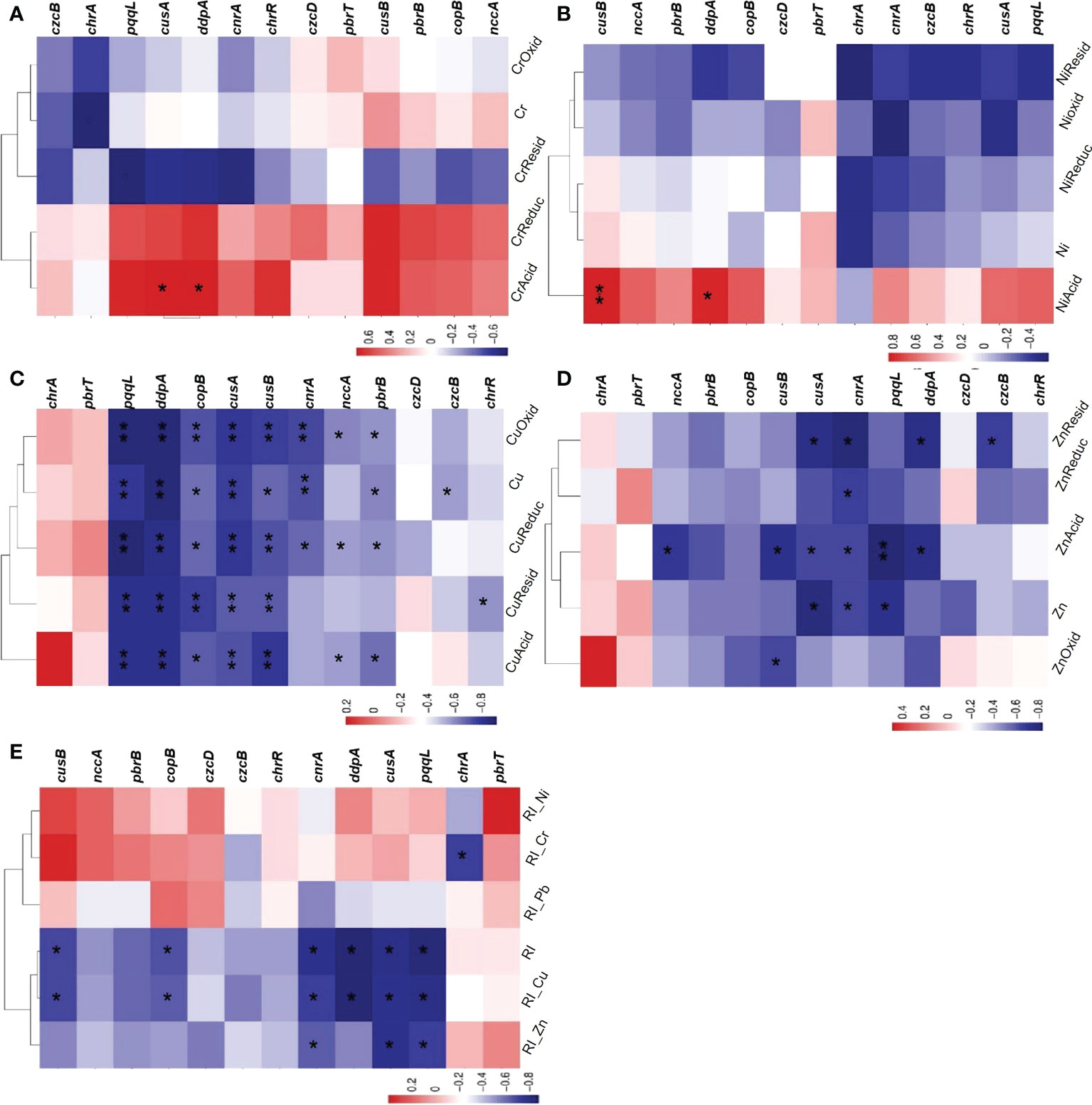
Figure 6 Spearman correlation analyses of HMRGs with Cr and its speciations (A), Ni and its speciations (B), Cu and its speciations (C), Zn and its speciations (D), and RI values of five metals (E). Asterisks represent significant correlations where * indicates P< 0.05 and ** indicates P< 0.01.
The correlations between HMRGs and the specific metals were not constant (Figure 6). For example, the Cu-resistance genes copB, cusA and cusB were negatively correlated with the total amount of Cu and its speciations, which was consistent with the report of Besaury et al. (2014), who showed that the representation of Cu-resistance genes decreases with increasing Cu concentration. It is our conjecture that the duration of three years of copper supplementation was inadequate for the bacterial community to develop a greater number of copper-resistant genes. In contrast, there was no correlation between any of the environmental factors tested and either the Ni-resistance gene nccA or the Zn-resistance gene czcB, which may be because nccA can comprehensively counteract Ni, Co and Cd accumulation, but the action of nccA has no obvious effect on the total amount of Ni (Vaiopoulou and Gikas, 2012).
Interestingly, Pb speciations showed no correlation with HMRG abundance, including pbrB and pbrT. A previous study found that pbrB and pbrT were only sensitive to Pb2+ rather than Pb4+ (Borremans et al., 2001), because pbrB is involved in the the export of Pb2+ from cells, and pbrT encodes the protein that takes up Pb2+. Given the above result, we can speculate that there was little Pb2+ in our samples. Another paradoxical finding is that the Cr-resistance gene chrR was not affected by the total amount of Cr or its speciations. A possible explanation is that Cr mainly exists as Cr3+, and chrR is only sensitive to Cr6+ (Chihomvu et al., 2015).
A further point of interest is that metal abundance can be correlated with the presence of HMRGs related to other, non-cognate metals. For example, the total amount of Cu was not only correlated with the Cu-resistance genes cusB and copB, but also with cnrA, pbrB and czcB. Sun et al. (2021) found similar correlations between the abundance of a Zn-resistance gene (czcA) and the total amount of Cu and Cr. This indicates that the bacterial community can develop cross-tolerance to several heavy metals simultaneously when subjected to the stress of a single heavy metal, which may be because the same genetic element (such as a plasmid or transposon) can carry different resistance genes, resulting in gene co-selection (Seiler and Berendonk, 2012; Andrea et al., 2016; Durrant et al., 2019). In summary, there were no clear rules governing correlation between heavy metal pollution and the abundance of particular HMRGs. Thus, the abundance of HMRGs may be influenced by various environmental factors.
3.3.3 Canonical correlation analysis of heavy metal pollution and bacterial HMRG representation
CCA was performed on bacterial HMRGs and environmental factors and showed that clay, silt, sand, TP, pH, total amount of Cr, Ni, Zn, and Pb, RI-Cr, RI-Ni, RI-Cu and RI-Zn all affected HMRG abundance in sediments (Figure 4D). In the CCA analysis, the contribution rate of axis l and axis 2 to bacterial HMRG abundance in Beihai mangrove was 48.15%, indicating that the above environmental factors had an important impact on the representation of bacterial HMRGs. In addition, levels of sediment microorganism HMRGs in GX1, GX2 and GX3 were mainly affected by sand, total amount of Cr and Ni, RI-Cr and RI-Ni, while the HMRGs in GX4 and GX5 were mainly affected by RI-Cu. It was also noted that GX4 and GX5 mapped further from related environmental factors than GX1, GX2 and GX3 (Figure 4D), indicating that the influence of these environmental factors on HMRG representation at GX1, GX2 and GX3 in Beihai mangrove was greater than their influence on HMRG representation at sampling sites GX4 and GX5.
In combination with the results of section 3.2.4, it is clear that both environmental factors and HMRGs affect community structure at the level of phyla, class and order. These observations further demonstrate that resistance to heavy metals is a common feature of many microorganisms subjected to heavy-metal stress, and that heavy-metal pollution effects on the bacterial community also modify the diversity and abundance of individual HMRGs (Sun et al., 2021). At the same time, the speciation of heavy metals and the RSP in all samples had no effect on changes in the bacterial community and HMRG representation, indicating that RI, which includes a heavy-metal toxicity coefficient in the formula for its calculation, can accurately quantify the toxicity level of heavy metals (Li et al., 2021), and can more fully and clearly reflect the impact of heavy-metal pollution on bacterial community structure and HMRG representation. The CCA results show that the contribution rate of heavy-metal pollution to the changes in the bacterial community was always more than 80%, while the contribution to HMRG abundance was only 48.15% (Figure 4), further suggesting that HMRG representation is influenced by other factors. For example, a large number of studies on HMRGs indicate that HMRGs and antibiotic resistance genes (ARGs) are located on the same genetic element, and heavy metals can exert selective pressure not only on HMRGs, but also on ARGs. This selection pressure may lead to cross-selection of heavy metal and antibiotic resistance, potentially resulting in co-occurrence of HMRGs and ARGs (Knapp et al., 2011; Ji et al., 2012; Seiler and Berendonk, 2012; Pal et al., 2015; Andrea et al., 2016; Durrant et al., 2019).
4 Conclusion
In this study, we designed a Cu-addition experiment to explore the effect on HMRG representation and bacterial community structure in mangrove sediments experiencing heavy-metal pollution stress. With the addition of Cu, total Cu contents increased significantly, as did its reducible fraction, in comparison to natural sediments. The Cu stress affected the Chao1 index rather than the Shannon index, indicating that Cu pollution influences bacterial diversity in the Beihai mangrove mainly by affecting the number of rare species. Spearman correlation analysis indicated that the speciations of heavy metals were more suitable for the analysis of the relationship between a single dominant bacterial community and heavy-metal pollution than the total amount of metal. CCA analysis showed that heavy-metal pollution could explain most of the changes in bacterial community structure in Beihai mangrove. Thus, the addition of Cu significantly changed the bacterial community structure at the phylum level. The metal speciations and RI could better explain the changes in bacterial community. Furthermore, we compared the differences in individual HMRG levels between Cu stress and natural conditions in Beihai mangrove sediments. Whether Cu was added or not, cusA was the most abundant HMRG in all samples, while pbrT was the least abundant, indicating that HMRG representation was relatively consistent in mangrove sediments although the addition of Cu resulted in lower HMRG abundance generally. CCA analysis showed that environmental factors that affect bacterial community structure can also affect HMRG abundance, and resistance to heavy metals is a common feature of many microorganisms under heavy-metal stress. However, the effect of heavy-metal pollution on HMRG representation in Beihai mangrove was weaker than that of heavy-metal pollution on bacterial community structure, probably due to the co-occurrence of HMRGs and ARGs. In summary, during a three-year Cu-addition experiment, we found that the bacterial community structure and the abundance of particular HMRGs was affected by the addition of pollutants. More specific factors, such as metal speciations or RI, had a greater impact on bacterial characteristics than the total metal content.
Data availability statement
The original contributions presented in the study are included in the article/Supplementary Material. Further inquiries can be directed to the corresponding author.
Author contributions
JL and YX contributed to the experiment and the first draft of the manuscript. ZZ, LZ, YQ, ZG, JC, BJ, and CS contributed to the manuscript revision. SC contributed to the final draft of the design of the experiment, the statistical analysis, the final draft of the manuscript and the funding acquiring. All authors contributed to the article and approved the submitted version.
Funding
CAS Key Laboratory of Science and Technology on Operational Oceanography (No.OOST2021-07). Shenzhen Science and Technology Program (JCYJ20210324093409025). Guangdong Province Undergraduate University Teaching Quality and Teaching Reform Project([2020]19).
Acknowledgments
We would like to thank the stuff of Guangdong Neilingding Futian National Natural Reserve and Guangxi Beihai Mangrove Reserve for their support in sampling. We are also grateful for the support from the public service platform of instruments and equipment of College of Life Sciences and Oceanography in Shenzhen University.
Conflict of interest
The authors declare that the research was conducted in the absence of any commercial or financial relationships that could be construed as a potential conflict of interest.
Publisher’s note
All claims expressed in this article are solely those of the authors and do not necessarily represent those of their affiliated organizations, or those of the publisher, the editors and the reviewers. Any product that may be evaluated in this article, or claim that may be made by its manufacturer, is not guaranteed or endorsed by the publisher.
Supplementary material
The Supplementary Material for this article can be found online at: https://www.frontiersin.org/articles/10.3389/fmars.2023.1157905/full#supplementary-material
References
Alongi D. M., Christoffersen P., Tirendi F. (1993). The influence of forest type on microbial-nutrient relationships in tropical mangrove sediments. J. Exp. Mar. Biol. Ecol. 171 (2), 201–223. doi: 10.1016/0022-0981(93)90004-8
Andrea D. C., Ester M. E., Silvia D., Gianluca C. (2016). Co-Occurrence of integrase 1, antibiotic and heavy metal resistance genes in municipal wastewater treatment plants. Water Res. 94, 208–214. doi: 10.1016/j.watres.2016.02.049
Balakrishnan B., Sahu B. K., Kothilmozhian Ranishree J., Lourduraj A. V., Nithyanandam M., Packiriswamy N., et al. (2017). Assessment of heavy metal concentrations and associated resistant bacterial communities in bulk and rhizosphere soil of avicennia marina of pichavaram mangrove, India[J]. Environ. Earth ences 76 (1), 58. doi: 10.1007/s12665-016-6378-7
Basak P., Pramanik A., Sengupta S., Nag S., Bhattacharyya M. (2016). Bacterial diversity assessment of pristine mangrove microbial community from dhulibhashani, sundarbans using 16S rRNA gene tag sequencing. Genomics Data 7, 76–78. doi: 10.1016/j.gdata.2015.11.030
Belliveau B. H., Starodub M. E., Cotter C., Cotter C., Trevors J. T. (1987). Metal resistance and accumulation in bacteria. Biotechnol. Adv. 5 (1), 101–127. doi: 10.1016/0734-9750(87)90006-1
Besaury L., Pawlak B., Quillet L. (2014). Expression of copper-resistance genes in microbial communities under copper stress and oxic/anoxic conditions. Environ. Sci. pollut. Res. 23 (5), 4013–4023. doi: 10.1007/s11356-014-3254-4
Borremans B., Hobman J. L., Provoost A., Brown N. L., Van d. (2001). Cloning and functional analysis of the pbr lead resistance determinant of ralstonia metallidurans CH34. J. Bacteriology 183 (19), 5651–5658. doi: 10.1128/jb.183.19.5651-5658.2001
Bouskill N. J., Barnhart E. P., Galloway T. S., Handy R. D., Ford T. E. (2007). Quantification of changing pseudomonas aeruginosa sodA, htpX and mt gene abundance in response to trace metal toxicity: a potential in situ biomarker of environmental health. FEMS Microbiol. Ecol. 60 (2), 276–286. doi: 10.1111/j.1574-6941.2007.00296.x
Cai G. Q., Li M., Chen H. J., Sun G. H., Wu J. Q., Xu Y. H. (2013). Grain size and geochemistry of surface sediments in northwestern continental shelf of the south China Sea. Environ. Earth Sci. 70 (1), 363–380. doi: 10.1007/s12665-012-2133-x
Chao A. (1984). Non-parametric estimation of the classes in a population. Scandinavian J. Stat 11 (4), 265–270. doi: 10.2307/4615964
Chen J., Li J., Zhang H., Shi W., Liu Y. (2019). Bacterial heavy-metal and antibiotic resistance genes in a copper tailing dam area in northern China. Front. Microbiol. 10. doi: 10.3389/fmicb.2019.01916
Chen S., Liao J., Zhang Q., Ding S., He M., Huang D. (2021). Spatial variability and influencing factors of cr, Ni, Cu, zn and Pb in five mangroves reserves of south China. IOP Conf. Series: Earth Environ. Sci. 690 (1), 012017(1–17). doi: 10.1088/1755-1315/690/1/012017
Chen J. W., Zhang H., Li J. J., Liu Y., Shi W., Hu H. W. (2020). The toxic factor of copper should be adjusted during the ecological risk assessment for soil bacterial community. Ecol. Indic. 111, 106072. doi: 10.1016/j.ecolind.2020.106072
Chihomvu P., Stegmann P., Pillay M. (2015). Characterization and structure prediction of partial length protein sequences of pcoA, pcoR and chrB genes from heavy metal resistant bacteria from the klip river, south Africa. Int. J. Mol. Sci. 16 (4), 7352–7374. doi: 10.3390/ijms16047352
Cordeiro R. C., Machado W., Santelli R. E., Figueiredo A. G. J., Seoane J. C. S., Oliveira E. P., et al. (2015). Geochemical fractionation of metals and semimetals in surface sediments from tropical impacted estuary (Guanabara bay, Brazil). Envieonmental Earth Sci. 74 (2), 1363–1378. doi: 10.1007/s12665-015-4127-y
Dudani S. N., Lakhmapurkar J., Gavali D., Patel T. (2017). Heavy metal accumulation in the mangrove ecosystem of south Gujarat coast, India. Turkish J. Fisheries Aquat. Sci. 17 (4), 755–766. doi: 10.4194/1303-2712-v17_4_11
Durrant M. G., Li M. M., Siranosian B. A., Montgomery S. B., Bhatt A. S. (2019). A bioinformatic analysis of integrative mobile genetic elements highlights their role in bacterial adaptation. Cell Host Microbe 27 (1), 140–153. doi: 10.1016/j.chom.2019.10.022
Echavarri-Bravo V., Paterson L., Aspray T. J., Porter J. S., Winson M. K., Thornton B., et al. (2015). Shifts in the metabolic function of a benthic estuarine microbial community following a single pulse exposure to silver nanoparticles. Environ. pollut. 201, 91–99. doi: 10.1016/j.envpol.2015.02.033
Feng J. X., Zhu X. S., Wu H., Ning C. X., Lin G. H. (2017). Distribution and ecological risk assessment of heavy metals in surface sediments of a typical restored mangrove–aquaculture wetland in shenzhen, China. Mar. pollut. Bull. 124, 1033–1039. doi: 10.1016/j.marpolbul.2017.01.004
Ferhan Ç, Neslihan S., AyŞe G. G. (2010). Inhibitory effects of Cu, zn, Ni and Co on nitrification and relevance of speciation. J. Chem. Technol. Biotechnol. 85 (4), 520–528. doi: 10.1002/jctb.2321
Gamal E. H. O., Hussein H. A., Khaled E., Mohammed R. S., Iqbal A. (2019). Recent progress in metal-microbe interactions: prospects in bioremediation. J. Pure Appl. Microbiol. 13 (1), 13–26. doi: 10.22207/JPAM.13.1.02
Grinter R., Leung P. M., Wijeyewickrema L. C., Littler D., Lithgow T. (2019). Protease-associated import systems are widespread in gram-negative bacteria. PloS Genet. 15 (10), e1008435. doi: 10.1371/journal.pgen.1008435
Haddadin F. T., Harcum S. W. (2010). Transcriptome profiles for high-cell-density recombinant and wild-type escherichia coli. Biotechnol. Bioengineering 90 (2), 127–153. doi: 10.1002/bit.20340
Hakanson L. (1980). An ecological risk index for aquatic pollution control: a sedimentological approach. Water Res. 14 (8), 975–1001. doi: 10.1016/0043-1354(80)90143-8
Hall G. E. M., Pelchat P. (1999). Comparibility of results obtained by the use of different selective extraction schemer for the determination of element forms in soils. Water Air Soil pollut. 112, 41–53. doi: 10.1023/A:1005001131472
Hall G., Vaive J. E., Beer R., Hoashi M. (1996). Selective leaches revisited, with emphasis on the amorphous fe oxyhydroxide phase extraction. J. Geochemical Explor. 56, 59–78. doi: 10.1016/0375-6742(95)00050-X
Hu B. C., Liao J. X., Zhang Q. J., Ding S. L., He M. Y., Qiao Y., et al. (2022). Diversity and vertical distribution of sedimentary bacterial communities and its association with metal bioavailability in three distinct mangrove reserves of south China. Water 14 (6), 971–998. doi: 10.3390/w14060971
Huang P. M., Bollag J. M., Senesi N. (2002). Interactions between soil particles and microorganisms: impact on the terrestrial ecosystem. Chem. Int. – Newsmagazine IUPAC 24 (4), 26–26. doi: 10.1515/ci.2002.24.4.26a
Imchen M., Kumavath R., Barh D., Avezedo V., Wattam A. R. (2017). Searching for signatures across microbial communities: metagenomic analysis of soil samples from mangrove and other ecosystems. Sci. Rep. 7 (1), 165. doi: 10.1038/s41598-017-09254-6
Imchen M., Kumavath R., Barh D., Vaz A., Góes-Neto A., Tiwari S., et al. (2018). Comparative mangrove metagenome reveals global prevalence of heavy metals and antibiotic resistome across different ecosystems. Sci. Rep. 8, 11187. doi: 10.1038/s41598-018-29521-4
Jayachandran S., Chakraborty P., Ramteke D., Chennuri K., Chakraborty S. (2018). Effect of pH on transport and transformation of Cu-sediment complexes in mangrove systems. Mar. pollut. Bull. 133 (8), 920–929. doi: 10.1016/j.marpolbul.2018.03.054
Ji X. L., Shen Q. H., Liu F., Ma J., Xu G., Wang Y. L., et al. (2012). Antibiotic resistance gene abundances associated with antibiotics and heavy metals in animal manures and agricultural soils adjacent to feedlots in shanghai; China. J. Hazardous Materials 235-236 (20), 178–185. doi: 10.1016/j.jhazmat.2012.07.040
Kathiresan K., Bingham B. L. (2001). Biology of mangroves and mangrove ecosystems. Adv. Mar. Biol. 40 (01), 81–251. doi: 10.1016/S0065-2881(01)40003-4
Knapp C. W., Mccluskey S., Singh B. K., Campbell C. D., Graham D. W. (2011). Antibiotic resistance gene abundances correlate with metal and geochemical conditions in archived Scottish soils. PloS One 6 (11), e27300. doi: 10.1371/journal.pone.0027300
Laitinen O. H., Kuusela T. P., Kukkurainen S., Nurminen A., Hytnen V. P. (2021). Bacterial avidins are a widely distributed protein family in actinobacteria, proteobacteria and bacteroidetes. BMC Ecol. Evol. 21 (1), 53–55. doi: 10.1186/s12862-021-01784-y
Lee S. Y., Primavera J. H., Dahdouh-Guebas F., Mckee K., Bosire J. O., Cannicci S., et al. (2014). Ecological role and services of tropical mangrove ecosystems: a reassessment. Global Ecol. Biogeography 23 (7), 726–743. doi: 10.1111/geb.12155
Legatzki A., Grass G., Anton A., Rensing C., Nies D. H. (2003). Interplay of the czc system and two p-type ATPases in conferring metal resistance to ralstonia metallidurans. J. Bacteriology 185 (15), 4354–4361. doi: 10.1128/JB.185.15.4354-4361.2003
Li D. L., Chen J. W., Zhang H., Li J. J. (2021). Effects of Pb and cd on forest soil bacterial functional diversity and community structure. Acta Ecologica Sin. 41 (21), 8472–8483. doi: 10.5846/stxb202009062313
Li C. M., Wang H. C., Liao X. L., Xiao R., Liu K. H., Bai J. H., et al. (2022). Heavy metal pollution in coastal wetlands: a systematic review of studies globally over the past three decades. J. Hazardous Materials 424, 127312. doi: 10.1016/j.jhazmat.2021.127312
Liu M., Huang H., Bao S., Tong Y. H. (2019). Microbial community structure of soils in bamenwan mangrove wetland. Sci. Rep. 9 (1), 8406. doi: 10.1038/s41598-019-44788-x
Magurran A. (1988). Ecological diversity and its measurement Vol. 179 (Australia:Springer). Croon Helm Limited. doi: 10.1007/978-94-015-7358-0_2
Munson G. P., Lam D. L., Outten F. W., O"Halloran T. V. (2000). Identification of a copper-responsive two-component system on the chromosome of escherichia coli K-12. J. Bacteriology 182 (20), 5864–5871. doi: 10.1128/jb.182.20.5864-5871.2000
Oliveira A., Pampulha M. E. (2006). Effects of long-term heavy metal contamination on soil microbial characteristics. J. Bioscience Bioengineering 102 (3), 157–161. doi: 10.1263/jbb.102.157
Pal C., Bengtsson-Palme J., Kristiansson E., Larsson D.G.J. (2015). Co-Occurrence of resistance genes to antibiotics, biocides and metals reveals novel insights into their co-selection potential. BMC Genomics 16 (1), 1–14. doi: 10.1186/s12864-015-2153-5
Pradhan S. K., Singh N. R., Kumar U., Mishra S. R., Thatoi H. (2020). Illumina MiSeq based assessment of bacterial community structure and diversity along the heavy metal concentration gradient in sukinda chromite mine area soils, India. Ecol. Genet. Genomics 15, 100054. doi: 10.1016/j.egg.2020.100054
Prosi F. (1989). Factors controlling biological availability and toxic effects of lead in aquatic organisms. Sci. Total Environ. 79 (2), 157–169. doi: 10.1016/0048-9697%2889%2990359-8
Ramos L., González M. J., Hernández L. M. (1999). Sequential extraction of copper, lead, cadmium, and zinc in sediments from ebro river (Spain): relationship with levels detected in earthworms. Bull. Environ. contamination Toxicol. 62 (3), 301–308. doi: 10.1007/s001289900874
Rauret G., Lopez-Sanchez J., Sahuquillo A., Rubio R., Davidson C., Ure A. (1999). Improvement of the BCR three step sequential extraction procedure prior to the certification of new sediment and soil reference materials. J. Environ. Monit. Jem 1, 57–61. doi: 10.1039/A807854H
Ren C. J., Zhao F. Z., Kang D., Yang G. H., Han X. H., Tong X. G., et al. (2016). Linkages of C:N: p stoichiometry and bacterial community in soil following afforestation of former farmland. For. Ecol. Manage. 376, 59–66. doi: 10.1016/j.foreco.2016.06.004
Roosa S., Wattiez R., Prygiel E., Lesven L., Billon G., Gillan D. C. (2014). Bacterial metal resistance genes and metal bioavailability in contaminated sediments. Environ. pollut. 189 (12), 143–151. doi: 10.1016/j.envpol.2014.02.031
Seiler C., Berendonk T. U. (2012). Heavy metal driven co-selection of antibiotic resistance in soil and water bodies impacted by agriculture and aquaculture. Front. Microbiol. 3. doi: 10.3389/fmicb.2012.00399
Shannon P., Markiel A., Ozier O., Baliga N. S., Wang J. T., Ramage D., et al. (2003). Cytoscape: a software environment for integrated models of biomolecular interaction networks. Genome Res. 13 (11), 2498–2504. doi: 10.1101/gr.1239303
Shi C., Ding H., Zan Q. J., Li R. L. (2019). Spatial variation and ecological risk assessment of heavy metals in mangrove sediments across China. Mar. pollut. Bull. 143, 115–124. doi: 10.1016/j.marpolbul.2019.04.043
Sun F. L., Xu Z. T., Fan L. L. (2021). Response of heavy metal and antibiotic resistance genes and related microorganisms to different heavy metals in activated sludge. J. Environ. Manage. 300, 113754. doi: 10.1016/j.jenvman.2021.113754
Tessier A., Campbell P., Bisson M. (1979). Sequential extraction procedure for the speciation of pareticulate trace-metrals. Analytical Chem. 51, 844–851. doi: 10.1021/ac50043a017
Udechukwu B. E., Ismail A., Zulkifli S. Z., Omar H. (2015). Distribution, mobility, and pollution assessment of cd, Cu, Ni, Pb, zn, and fe in intertidal surface sediments of sg. puloh mangrove estuary, Malaysia. Environ. Sci. pollut. Res. 22 (6), 4242–4255. doi: 10.1007/s11356-014-3663-4
Vaiopoulou A. E., Gikas B. P. (2012). Effects of chromium on activated sludge and on the performance of wastewater treatment plants: a review. Water Res. 46 (3), 549–570. doi: 10.1016/j.watres.2011.11.024
Wang H., Tian T., Gong Y., Ma S., Diao X. (2020). Both environmental and spatial variables affect bacterial functional diversity in mangrove sediments at an island scale. Sci. Total Environ. 753 (3), 142054. doi: 10.1016/j.scitotenv.2020.142054
Wang L., Wang Y. P., Xu C. X., An Z. Y., Wang S. M. (2011). Analysis and evaluation of the source of heavy metals in water of the river changjiang. Environ. Monit. Assess. 173, 301–313. doi: 10.1007/s10661-010-1388-5
Wasilkowski D., Mrozik A., Piotrowska-Seget Z., KrzyzAk J., Pogrzeba M., PlAza G. (2014). Changes in enzyme activities and microbial community structure in heavy metal-contaminated soil under in situ aided phytostabilization. CLEAN - Soil Air Water 42 (11), 1618–1625. doi: 10.1002/clen.201300631
Wu S., Li R., Xie S., Shi C. (2019). Depth-related change of sulfate-reducing bacteria community in mangrove sediments: the influence of heavy metal contamination. Mar. pollut. Bull. 140, 443–450. doi: 10.1016/j.marpolbul.2019.01.042
Yeager C. C. (1991). “Copper and zinc preservatives,” in Disinfection, sterilization,and preservation, 4th ed. Ed. Block S. S. (Philadelphia: Lea and Febiger Press), 358–361.
Zhang P., Hu R., Zhu L., Wang P., Yin D., Zhang L. (2017). Distributions and contamination assessment of heavy metals in the surface sediments of western laizhou bay: implications for the sources and influencing factors. Mar. pollut. Bull. 119 (1), 429–438. doi: 10.1016/j.marpolbul.2017.03.046
Zhang T., Li L. J., Xu F., Chen X. Y., Du L., Li Y. X. (2020). Assessing the environmental risk, fractions, and remobilization of copper and zinc in the sediments of the jialing river-an important tributary of the Yangtze river in China. Environ. Sci. pollut. Res. 27 (31), 39283–39296. doi: 10.1007/s11356-020-09963-y
Zhang H., Walker T., Davis E., Ma G. (2019). Ecological risk assessment of metals in small craft harbour sediments in Nova Scotia, Canada. Mar. pollut. Bull. 146, 466–475. doi: 10.1016/j.marpolbul.2019.06.068
Keywords: Cu addition, heavy metal resistance genes, mangrove sediments, environmental factor, bacterial diversity
Citation: Liao J, Xu Y, Zhang Z, Zeng L, Qiao Y, Guo Z, Chen J, Jia B, Shang C and Chen S (2023) Effect of Cu addition on sedimentary bacterial community structure and heavy metal resistance gene abundance in mangrove wetlands. Front. Mar. Sci. 10:1157905. doi: 10.3389/fmars.2023.1157905
Received: 03 February 2023; Accepted: 11 April 2023;
Published: 27 April 2023.
Edited by:
Hao Cheng, Chinese Academy of Sciences (CAS), ChinaReviewed by:
Jie Jin, East China Normal University, ChinaFulin Sun, Chinese Academy of Sciences (CAS), China
Copyright © 2023 Liao, Xu, Zhang, Zeng, Qiao, Guo, Chen, Jia, Shang and Chen. This is an open-access article distributed under the terms of the Creative Commons Attribution License (CC BY). The use, distribution or reproduction in other forums is permitted, provided the original author(s) and the copyright owner(s) are credited and that the original publication in this journal is cited, in accordance with accepted academic practice. No use, distribution or reproduction is permitted which does not comply with these terms.
*Correspondence: Si Chen, c2ljaGVuQHN6dS5lZHUuY24=
†These authors share first authorship