- 1Stan Richards School of Advertising and Public Relations, The University of Texas at Austin, Austin, TX, United States
- 2Department of Biological Sciences, University of Bergen, Bergen, Norway
- 3Bureau of Economic Geology, The University of Texas at Austin, Austin, TX, United States
Environmental monitoring at geologic CO2 storage sites is required by regulations for the purposes of environmental protection and emissions accounting in the case of leakage to surface. However, another very important goal of environmental monitoring is to assure stakeholders that the project is monitored for safety and effectiveness. With current efforts to optimize monitoring for cost-effectiveness, the question remains: will optimization of monitoring approaches degrade stakeholder assurance, or do heavily-instrumented sites communicate higher risk to a stakeholder? We report the results of a stakeholder survey in Gulf Coast states of the US where carbon capture and storage (CCS) is developing quickly. We rely on a 2 by 2 factorial experiment in which we manipulate message complexity (complex v. simple) and social norm (support from scientists v. support from community members). Subjects were randomly assigned to one of four conditions: 1) complex message with scientist support; 2) complex message with community member support; 3) simple message with scientist support; or 4) simple message with community member support. In addition to the experimental stimuli, subjects were also asked about their need for cognition, attitudes toward science and scientists, attitudes about climate change and support for carbon capture and storage (CCS). Our sample is drawn from residents in states bordering the western Gulf of Mexico (Texas, Louisiana, Florida) where CO2 geologic storage is being planned both onshore and offshore. The results offer important implications for public outreach efforts to key stakeholders.
1 Introduction
Carbon capture and storage (CCS) is a technology that can slow global climate change by drastically and permanently keeping industrial CO2 emissions from reaching the atmosphere. In CCS, CO2 is scrubbed out of the flue emissions of large industrial smoke stacks, transported to a suitable site, and then injected into deep geological formations for permanent storage and disposal. It is an effective and proven technology but will require upscaling by two orders of magnitude to meet global emissions reduction targets (IPCC, 2023). Until recently, the technology had not expanded rapidly enough, due in part, to a lack of policy needed to accelerate its deployment. However, at the writing of this manuscript, policy drivers are newly in place and accelerating CCS projects forward at an unprecedented rate in the United States. The Biden administration’s Infrastructure Investment and Jobs Act (IIJA), also known as the Bipartisan Infrastructure Bill (BIL), has included significant funding (billions of $USD) for CCS demonstration and pilot projects and development of direct air capture (DAC) hubs. The bill also contains funding to support the development of CO2 transport and storage infrastructure. Additionally, the Inflation Reduction Act (IRA) passed in 2022, expanded the 45Q tax credit for CO2 storage in geological formations, providing a business case for CCS projects to move forward.
The most economical CCS projects for first-movers are those where sources are in proximity to storage, thus minimizing the need for transport. In the United States, such an area exists in the Gulf of Mexico (GoM) region, where research has shown that some of the most voluminous high-quality storage potential can be found both onshore and offshore (Meckel and Trevino, 2015; DeAngelo et al., 2019; Ringrose and Meckel, 2019). This region also hosts some of the highest CO2 emissions in the country, with Texas as the highest emitting state (663 MMT in 2021), Florida as the third highest (226 MMT) and Louisiana as the sixth highest (189 MMT) (U.S. Energy Information Administration, 2021). Thus, in the GoM region, the largest emitting states are also in proximity to some of the largest storage potentials, and projects are moving into the region, both onshore and offshore. There are currently approximately 60 large-scale projects being planned in the onshore of Texas and Louisiana (Clean Air Task Force data base). In the offshore, the Texas General Land Office (GLO) awarded its first lease for CO2 storage in the Texas State waters of the Gulf of Mexico in September 2021. By 2023, the GLO had awarded six additional leases for offshore carbon storage. Also in 2023, Louisiana agreed to the development of an offshore CO2 storage hub in the waters off Cameron Parish.
Thus, projects are moving into the region before many local stakeholders have even heard of CCS technology and the lack of familiarity and understanding is fueling opposition in some areas. Misconceptions about risk, scale, effectiveness and environmental impacts of CCS are commonplace. Many stakeholders have previously experienced the effects of environmental inequity from other industries and are leery about yet another large-scale technology in their communities. To address long-standing environmental injustices of the past, the U.S. Office of Fossil Energy and Carbon Management (2023) at the Department of Energy is therefore emphasizing concepts of 1) community engagement, 2) diversity equity and inclusion, and 3) workforce development in areas where projects are being sited. Also being planned is a “responsible carbon management initiative” with the purpose to “recognize and encourage project developers and others in industry to pursue the highest levels of safety, environmental stewardship, accountability, community engagement, and societal benefits in carbon management projects” (Office of Fossil Energy and Carbon Management, 2023).
This concept of environmental justice in the USA is also increasingly being applied by CCS project developers in Europe, within a “Responsible Research and Innovation” (RRI) framework. The RRI concept originated in Europe, influenced by the concept of “real-time technology assessment” (Guston and Sarewitz, 2002) that encourages scientific research to contextualize the impacts of technology development on society, societal values, and the environment (Stilgoe, 2013). RRI and environmental justice both encourage high ethical standards, gender equality, environmental integrity and engagement of communities directly affected by a technology such that they fully understand the implications of the application of that technology.
One of the most important aspects linking stakeholders to CO2 storage projects is monitoring. Monitoring ensures that CO2 is retained in the subsurface and indicates that the environment and health and safety are being protected (Dixon and Romanak, 2015; Romanak and Dixon, 2022). Monitoring is required throughout the stratigraphy of a storage site and in all phases of a project from characterization to post closure. Over the past decade, much of the research on geological CO2 storage has focused on developing reliable monitoring methods and approaches. After two decades of research and application of various monitoring technologies, current emphasis is on down-selecting tools and optimizing monitoring approaches for cost-effectiveness. However, one important goal of environmental monitoring is to provide assurances to stakeholders that resources (groundwater, soil, atmosphere, seawater, and human health and safety) are being protected. The safety of geological CO2 storage is of primary importance to stakeholders and environmental monitoring is a critical link between the public and the project. Given the sharp rise in CO2 storage projects being developed in the GoM region, we seek to understand; 1) how stakeholders may respond to optimization (e.g., simplification) of environmental monitoring plans and 2) under what circumstances might lay stakeholders prefer complex, data-rich approaches that are intellectually inaccessible to them and require them to trust scientists explicitly, as opposed to simple approaches that can be easily learned, understood, and even implemented by the stakeholder.
Historically, environmental monitoring has relied on comparing soil CO2 concentrations measured during injection to the baseline CO2 concentration measured pre-injection. Any soil CO2 concentration greater than baseline would indicate leakage, unless the increase in CO2 could somehow be linked to environmental conditions. Natural soil CO2 is predominantly produced by root and microbial respiration which consumes organic matter and oxygen in the production of CO2 and energy. Respiration is highly dependent on temperature, moisture, barometric pressure, wind speed, oxygen and nutrient input. In order to determine if changes in CO2 concentrations represent natural variability or leakage, all of these parameters would need to be measured and the data plugged into complex algorithms to determine if environmental conditions are the driver. Complex algorithms are needed to compute thresholds based on observed environmental fluctuations. Time would be required to assess and analyze the data. The final answer on leakage would be shrouded in scientific process, and the scientist would need to be trusted. We question if a method such as this risks stakeholder assurance because it takes time to assess and is too technical for stakeholders to understand, or if it communicates a thoroughness in approach because multiple lines of data are being collected and assessed scientifically. An example of data streams for a baseline approach is shown in Figure 1 and this illustration is used as the technical stimuli representing “complex monitoring” (see Appendix 1).
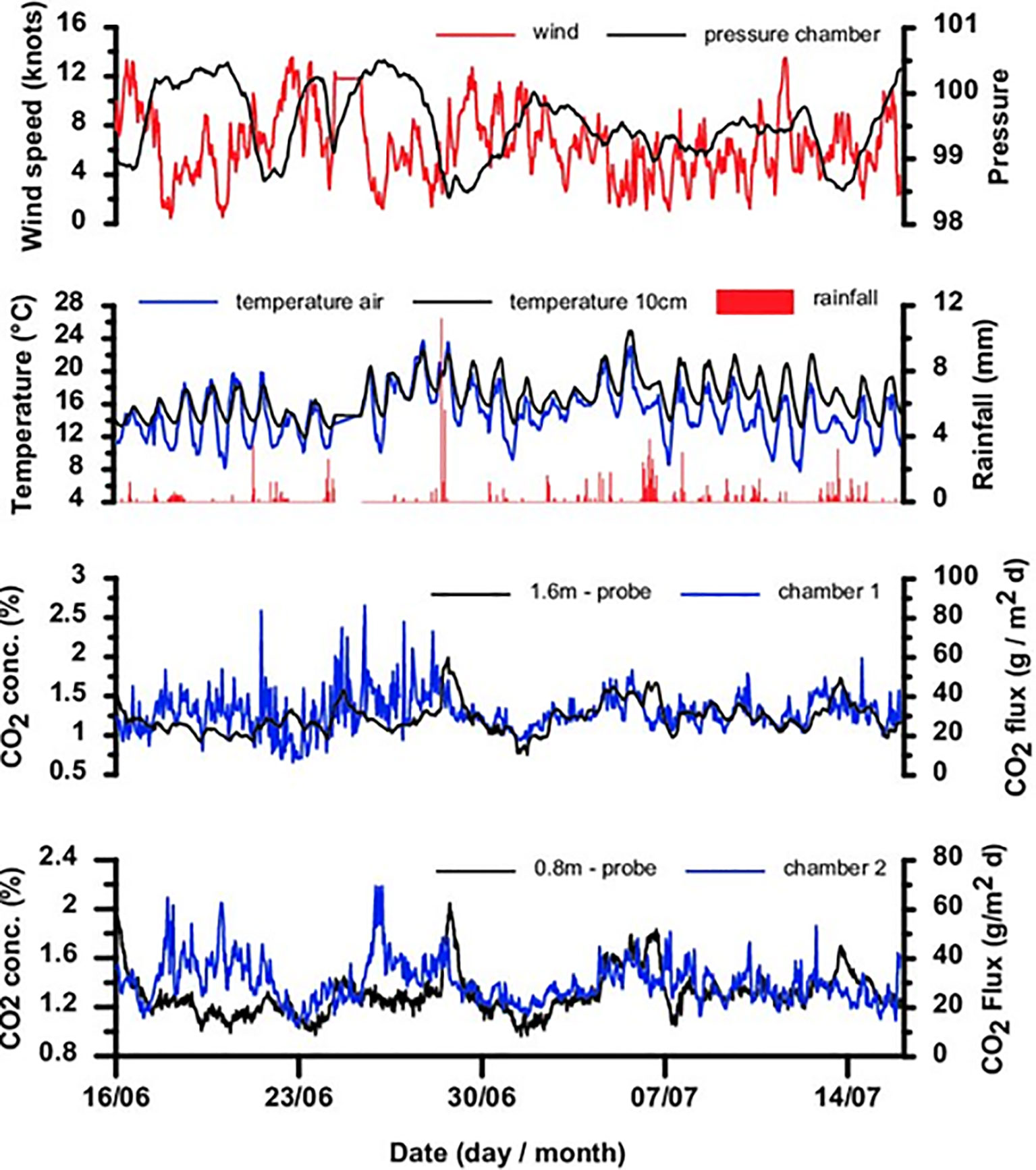
Figure 1 An example of the many data streams required to support the analysis of baseline CO2 concentrations for leakage identification.
An alternative approach to baseline methods that provides significant optimization of monitoring is the use of a stoichiometric method, known as a process-based soil gas approach (Romanak et al., 2012; Romanak et al., 2014). This approach, developed over the past decade, has been firmly adopted for terrestrial sites and is currently being amended and developed for offshore site monitoring (Uchimoto et al., 2018; Omar et al., 2021). A process-based soil gas method uses the stoichiometry of vadose zone geochemical reactions and ratios of the co-existing soil gases to determine processes affecting CO2 concentration, whether natural or from leakage (Figure 2). A trend that signifies respiration on a graph of O2 vs CO2 separates a potential leakage field (to the right of the respiration trend) from the field that represents natural processes (to the left of the respiration trend). It provides an instantaneous result using a method that is fully transparent with a graphical output that is instantaneously apparent to all. The only data that are required are from one simple gas sample per location. No supporting data are needed. Our study is motivated by question about whether local stakeholders would view this monitoring as too simple to be adequately rigorous or if they would appreciate being able to understand it and therefore take a degree of ownership in the results. The data stream for this type of approach shown in Figure 2 represents the illustration used as the technical stimuli representing “simple monitoring” (see Appendix 1).
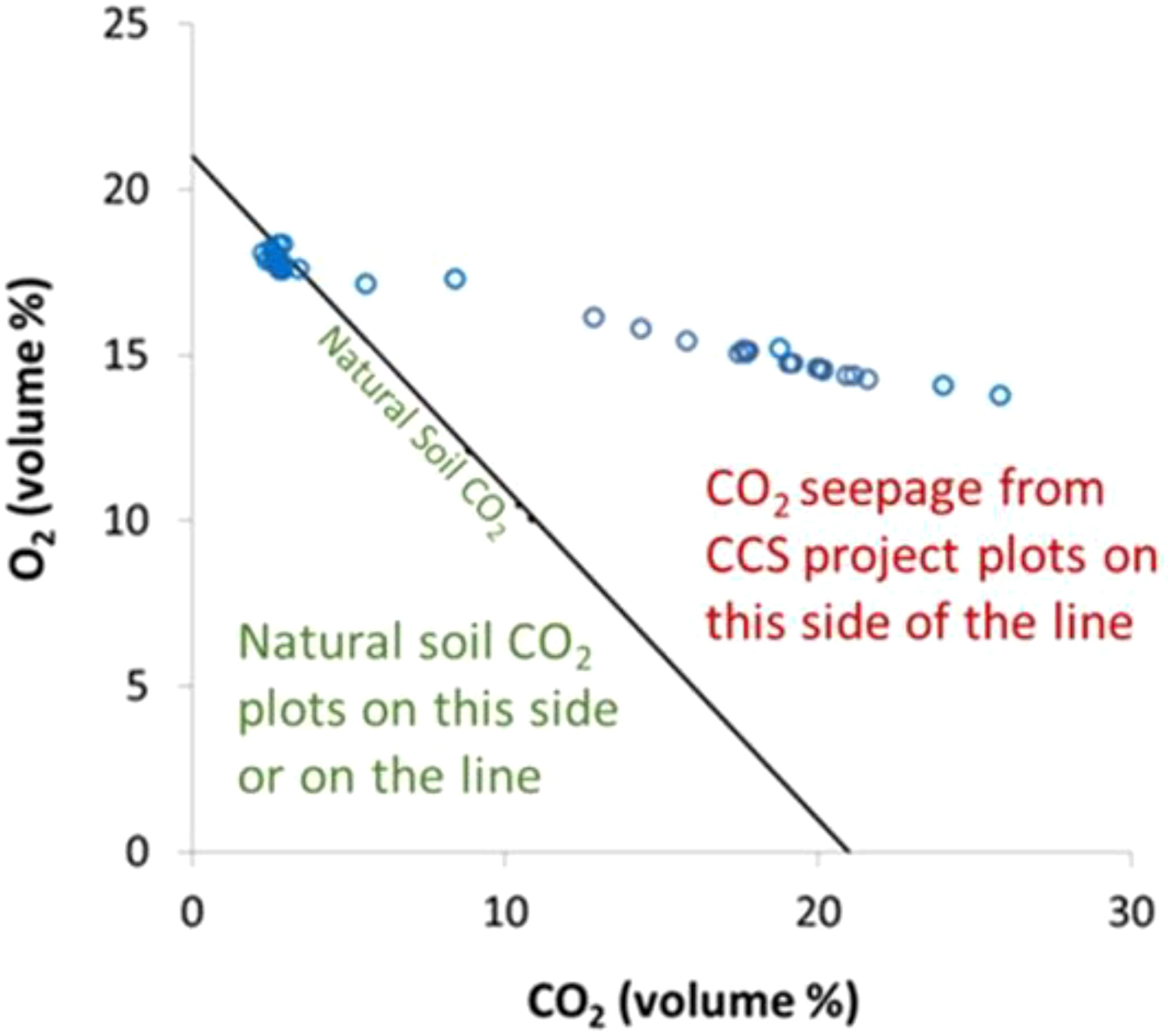
Figure 2 Example of a process-based approach to leakage identification which utilizes very little data and provides instantaneous graphical results that are easy for stakeholders to understand.
Thus as important as technological advances are to the large-scale development of CO2 storage, so is public acceptance of the technology (Leiss and Larkin, 2019). Political will, influenced by public attitudes and perceptions, must be strong to attract the necessary investments and to create new markets for CCS. Public trust in the context of CCS is a vital dimension that fuels political will. Literature in social science and cultural cognition points to the need of simple scientific messages (Kahan, 2010; Dowd et al., 2014) communicated by an individual or group that shares the same cultural values as the receiver (Cohen et al., 2007; Dowd et al., 2014). We are interested in how the design of monitoring programs for CCS are understood and perceived by stakeholders in the GoM region, and accordingly, designed a study to give insight into perceptions of two different types of environmental monitoring technologies: simple stoichiometric approaches versus more complex baseline approaches. We aim to answer the following questions: 1) are simple or more complex monitoring strategies preferred by individuals living in the gulf coast regions? 2) how do social norms and cultural cognition affect the responses, and 3) how does the group that endorses the monitoring system influence public acceptance?
2 Theoretical framework
2.1 Audience pre-dispositions
Individuals do not approach messages or communication in a uniform manner. Rather, their specific predispositions and orientations, such as prior knowledge, attitudes and beliefs, determine whether they pay attention to certain messages and how they process information. For this reason, our communication efforts do not focus on a broad or mass audience, but instead, target specific segments or groupings. This concept is substantiated by prior research on messages around CCS that has shown that audience segments evaluate CCS-related messages and their persuasiveness differently (Broecks et al., 2016).
The traditional approach to segmenting audiences has been to focus on socio-demographics, such as age, gender, race, etc. (Yankelovich and Meer, 2006). More recently, segmentation approaches have also incorporated psychographics, such as attitudes toward a phenomenon of interest (Hine et al., 2014), and behavioral data, such as communication exposure patterns (Lotenberg et al., 2011). This nuanced approach is seen as more robust and better at predicting related attitudes and behaviors (Yankelovich and Meer, 2006).
As such, we also adopt this more sophisticated approach to segmentation and draw on psychographic and behavioral factors that have been shown to be important in terms of CCS: attitude toward science, need for cognition, science media consumption and climate change beliefs. Individuals with positive attitudes toward science are more open to scientific content and more willing to participate in citizen science projects (Füchslin et al., 2019). In health contexts, trust in science has been shown to predict trust in technology (Rodriguez et al., 2008). Need for cognition (NFC) is also an important differentiating factor to understand how audiences engage with messages. Those who score high on NFC scales enjoy complex messages and effortful thinking whereas those lower on the scale avoid it (Cacioppo and Petty, 1982). Given that CCS is a complex phenomenon, the degree of cognitive effort that individuals bring to processing a message is important to understand. In addition to science attitudes, exposure to science-related media has also been shown to be an important factor in understanding complex science. Past research has shown that attention to science-related content is associated with greater scientific knowledge (Su et al., 2015). Lastly, climate change attitudes are an important differentiating point when it comes to CCS, given that CCS’s potential to mitigate the effects of climate change is one of the central arguments in favor of its widespread adoption (Broecks et al., 2016). In studies of Japanese and Australian publics, individuals who are more media savvy and who are more capable of processing information are likely to develop more positive attitudes toward CCS and be more accepting of it (Ashworth et al., 2006; Itaoka, 2006).
2.2 Message complexity
Scientific information can be presented in a variety of ways, ranging from straightforward and simple, to detailed and complex. When communicating about important issues, the assumption is often that more is better – more facts, more information, more detail – yet this is not always the case (Green and Armstrong, 2015). Oftentimes, it depends on the context (Lewis and Eves, 2012) and the audience (Pogrebnyakov and Maldonado, 2018). For example, some audiences might feel more secure with extremely complex and scientific approaches versus simpler approaches. Those who enjoy effortful thinking or place a high value in science might prefer complex messages that are in-depth and detailed. Conversely, those who find cognitive effort onerous, who might not value science as much or pay as much attention to science-related media might prefer simpler approaches in which answers are immediate and the information is easily understood.
In the context of CCS, past research has shown that providing Dutch stakeholders with accurate and easily understood information about the technology leads to greater support (de Best-Waldhober et al., 2009). In the US, when publics are informed about CCS and its associated benefits and costs, they are more likely to support CCS as part of a comprehensive low-carbon energy plan (Schäfer et al., 2018). But the question becomes murkier when we take into account information about CCS monitoring activities. Although some argue that monitoring activities should be routinely shared with the public (Greenberg and Gauvreau, 2014), there is evidence to suggest that doing so can have the counter-intuitive effect of increasing perceptions of risk (Wiedemann et al., 2006). In their study of CCS monitoring activity, Seigo et al. (2011) found that individuals who were provided with more detailed information perceived CCS to be riskier and less beneficial than those who only received basic information.
We examine message complexity by manipulating the content and sophistication of information incorporated into messaging about a proposed CO2 monitoring system. The more complex message entails multiple datapoints and graphical elements whereas the simpler monitoring system message entails one graphical image.
2.3 Social norms
Social norms can also be a powerful factor in message acceptance (Maibach, 2019). Social norms signal to group members those behaviors, attitudes and beliefs that are accepted and preferred (Cialdini and Cialdini, 2007). In the context of novel or complex ideas, social norms are useful heuristics to indicate what kinds of adaptive behaviors or beliefs should be adopted in otherwise ambiguous contexts (Valente, 2012). For scientific information, potential sources run the gamut including scientists, academics, politicians, business leaders and friends. Scientists and experts are trusted sources for scientific information, but so too are people who are socially close, such as friends, or those who share in the same community, such as a place of worship or a civic group (Maibach, 2019).
Information source for CCS-related content has been shown to be an important element. Trust in the source of CCS information is a key factor influencing acceptance of CCS (Ashworth et al., 2010). Sources from environmental organizations, academia and research institutions are considered most trustworthy when communicating CCS (Ashworth et al., 2010).
Our study examines the role of social norms in the gulf coast region surveyed to see which referential group (academic and industry experts versus local officials and community leaders) holds more influence in audience processing of messages about CO2 monitoring programs.
3 A model of CO2 monitoring acceptance and CCS support
Exposure to a message does not always produce a direct effect on behaviors; rather, messages often operate via indirect or mediated paths (Shah et al., 2007). That is, a message might impact audiences indirectly as a result of related attitudes and pre-dispositions. For example, environmental concern and exposure to environmental media content work together to influence environmental behaviors (Holbert et al., 2003). In recognition of the mediated nature of communication, we draw on the Technology Acceptance Model (TAM) and the Theory of Planned Behavior (TPB) to understand how our audience segments respond to our messages about CO2 monitoring and the drivers of stakeholder adoption and support for CCS.
TAM and TPB are proven useful models to help explain public acceptance of new technologies as a result of various individual orientations, including attitudes toward the technology, perceived ease of use, perceived usefulness, self-efficacy and perceived behavioral control. In TAM, behavioral intentions to use technology are related to attitudes toward the technology as well as perceptions of how easy it is to use, termed the ease of use, and how useful it would be, labelled usefulness (Davis, 1989). For technologies that might seem complex or intimidating, attitudes toward the technology itself are strong predictors of the likelihood of adopting it (Bagozzi et al., 1992). TAM has been shown to usefully predict adoption of new technologies including solar panels (Fatoki, 2022) and sustainable transportation (Ahn and Park, 2022). Despite its robustness, TAM has been criticized for not accounting for social influence and facilitating conditions (Shachak et al., 2019). Specifically, TAM does not account for subjective norms, perceived behavioral control and self-efficacy. To broaden the robustness of TAM, it is often combined with the Theory of Planed Behavior (TPB).
TPB has been applied across numerous contexts to explain and predict human behavior (Ajzen, 1991) and adding it to TAM allows us to paint a more sophisticated understanding of those factors that predict CCS-related attitudes and behaviors. Subjective norms refers to the individual’s perception that people who are important to them believe the behavior under question is positive and should be adopted (Cheon et al., 2012). Self-efficacy describes an individuals’ confidence in their capacity to carry out a specific behavior, while perceived behavioral control reflects the individual’s belief that they actually have control over the specific behavior (Hansen et al., 2018). In the context of CCS, prior research has shown TPB is a useful model to explain various outcomes, including CCS acceptance, willingness to pay for CCS power, and information seeking about CCS (Kraeusel and Möst, 2012; Kahlor et al., 2020). In our study, we take a citizen science approach and our outcome of interest is the likelihood of stakeholders using the monitoring approach and the information it provides.
TAM and TPB have been combined effectively to predict behaviors in multiple contexts, including internet banking (Safeena et al., 2013), purchasing energy-efficient appliances (Hua and Wang, 2019), and the use of renewable energy (Alam et al., 2014). Building on this work, we also synthesize the TAM and TPB to understand and predict behaviors and attitudes related to CO2 monitoring and CCS. See Figure 3.
4 Methods
4.1 Data collection
Our sample consisted of American adults aged 18 and older living in Texas, Florida and Louisiana. These three states were chosen because they are close to existing or proposed CCS facilities. We hired the global market research firm YouGov to collect data. Compared to other market research companies, YouGov consistently ranks as the most reliable and representative (Zack and Kennedy, 2015; Clement, 2016).
An attention check was included to screen out inattentive subjects. Midway through the survey, one question asked them to select “somewhat agree” as their response. Only those who responded correctly were included in the final sample of 997 subjects (Texas = 328; Louisiana = 336; Florida = 333). Our sample was 44% male and 56% female. The average age was 47 and the modal response for education was high school graduate (40.3%). In terms of race, 56.7% were white, 18.6% were Black or African American, 20.3% were Hispanic, and the remainder were Asian, Native American or a combination of two or more races.
4.2 Procedure
To test our hypotheses, we conducted a 2 (CO2 monitor system message: simple v. complex) x 2 (social norm: academic and industry expert. community leader) factorial between-subjects experiment. Subjects received an email inviting them to complete the survey, which started with an introduction about the purpose of the study and some baseline questions about attitudes toward science, climate change beliefs, need for cognition, science media consumption, and attitudes toward CCS. Subjects were then presented with the experimental stimuli (see Appendix), which was a message depicting the output of a CO2 monitoring system that was either complex (i.e. it included a large quantity of data and graphs) or simple (i.e. it contained a simple graphical depiction) and that was endorsed by either an academic and industry expert or a community leader. Subjects were randomly assigned to one of four stimuli (simple CO2 monitor with academic and industry endorser; simple CO2 monitor with community leader endorser; complex CO2 monitor with academic and industry endorser; or complex CO2 monitor with community leader endorser). After the stimuli, subjects answered questions about ease of use, usefulness, self-efficacy, behavioral control, attitude toward the CO2 monitor, intent to use the monitor and support for CCS.
4.3 Measures
4.3.1 Manipulation check
To confirm our experimental stimuli manipulated our desired factors effectively, we carried out two manipulation checks. To check the first factor, subjects were asked on a 5-point, semantic differential scale (simple – complex; easy to understand – hard to understand; easy – difficult; straightforward – confusing; uncomplicated – complicated; direct – convoluted) to rate the monitor system they were presented with. Those in the complex condition rated the monitoring system as more complex (M = 3.53, SD = 1.12) than those in the simple condition (M = 2.9, SD = 1.14); t(995) = -8.56, p <.001. To confirm the source of the endorsement was successfully manipulated, we asked subjects whether the monitoring system was endorsed by an academic/industry expert or a community leader (X2 (1, N = 997) = 225.0, p <.001). Both manipulation checks indicate our experimental stimuli were successful at manipulating the two factors of interest.
4.3.2 Random assignment
Assuming random assignment to condition was successful, subjects in each group should not differ significantly in terms of demographic characteristics. We ran t-tests and chi-square tests to confirm the experimental groups did not differ according to age, gender, ethnicity, education, income or political ideology. Random assignment was successful.
4.4 Independent variables
Details for all measures are listed in the Appendix.
4.4.1 Attitudes toward science
This was measured on a 5-point scale using Füchslin, Schäfer and Metag’s (2019) 10-item scale (M = 3.84, SD = .80; α = .90). Sample items include “Science and research play an important role in my life”, “In general, I trust science”, and “Science should have no limits to what it is able to investigate.”
4.4.2 Climate change beliefs
This was measured with three items drawn from previous literature (Leiserowitz, 2006; Moon et al., 2020). Sample items include “Climate change is a serious problem” and “CO2 that is emitted from power plants and industrial sources has been scientifically linked to climate change” measured on a 5-point scale (M = 3.85, SD = 1.21; α = .91).
4.4.3 Need for cognition
We used six items measured on a 5-point scale from Cacioppo and Petty (1982). Sample items include “I would prefer complex to simple problems” and “Learning new ways to think doesn’t excite me very much” (M = 3.26, SD = .70; α = .66).
4.4.4 Science media consumption
We drew on previous literature (Runge et al., 2018; Schäfer et al., 2018) to develop 8 items that captured the frequency with which subjects consumed science-oriented media content. Sample items, measured on a 5-point scale, include watching science documentaries, viewing science-themed entertainment shows, or reading science blogs (M = 2.57, SD = .85; α = .85).
4.5 Key variables
4.5.1 Perceived CCS benefits
This scale relied on five previously used items (Seigo et al., 2014). Sample items include perceived benefits to oneself, to one’s community, and to one’s state (M = 3.45, SD = 1.25; α = .95).
4.5.2 CCS awareness
Three items from Milfont (2012) were used to tap awareness of CCS, including “I had heard about carbon capture and storage before today” (M = 2.30, SD = 1.37; α = .90).
4.5.3 CCS trust
To measure individual’s level of trust in institutions to safeguard CCS projects, we adapted Seigo et al. (2014) scale. It included four items, such as trust in state government agencies, trust in energy companies and trust in third parties (M = 2.86, SD = 1.07; α = .84).
4.5.4 Perceived ease of use
This was measured with one item adapted from the literature (Taylor and Todd, 1995; Chen et al., 2007): “I think the CO2 monitoring approach would be easy to use” (M = 3.18, SD = 1.06).
4.5.5 Perceived usefulness
Also adapted from previous literature (Moore and Benbasat, 1991; Chen et al., 2007), sample items include “Using the CO2 monitoring approach would improve my understanding of CCS” and “The CO2 monitoring approach would make CCS less confusing” (M = 3.37, SD = .98; α = .90).
4.5.6 Behavioral control
We used three items adapted previous scales (Taylor and Todd, 1995; Cheon et al., 2012). Sample items include “I have sufficient knowledge to use this CO2 monitoring approach” and “I am capable of using this CO2 monitoring approach” (M = 2.87, SD = 1.13; α = .90).
4.5.7 Subjective norms
This was measured with three items drawn from Cheon et al. (2012). Sample items include: “Most people who are important to me would think that the CO2 monitoring approach is fine to use.” and “Most people who are important to me would be in favor of using the CO2 monitoring approach” (M = 3.28, SD = 1.05; α = .94).
4.5.8 Self-efficacy
This was measured with three items drawn from Cheon et al. (2012). Sample items include: “I would be confident about using this CO2 monitoring approach” and “Using this CO2 monitoring approach would not challenge me” (M = 3.22, SD = 1.04; α = .87).
4.5.9 Attitude toward the monitor
This was measured using a 5-point, semantic differential scale (bad – good; negative – positive; unsatisfactory – satisfactory; unfavorable – favorable; unpleasant – pleasant) drawn from Hensel and Bruner (1992) (M = 3.41, SD = .99; α = .92).
4.5.10 Intention to use
This was measured with two items adapted from previous literature (Taylor and Todd, 1995; Yu et al., 2005; Cheon et al., 2012). Sample items include “I would like to use the monitoring approach in the future if I had the opportunity” and “There is a high likelihood that I would use the sensor and the monitoring approach within the foreseeable future” (M = 3.16, SD = 1.25; r <.001).
4.5.11 Support for CCS
This was measured with two items asking subjects how strongly they support or oppose CCS and how strongly their community would support or oppose CCS (M = 3.33, SD = 1.04; r=.60, p<.001).
5 Analysis and results
5.1 Audience segmentation
The first step in our analysis was to develop the clusters that would define our audience segments. Using TwoStep cluster analysis in SPSS (Tkaczynski, 2017), we are able to segment our sample into meaningful groups, providing more nuanced understanding of how audiences think and feel about the CO2 monitoring system than if we assumed our sample all behaved in a uniform manner. TwoStep cluster analysis, which is well suited to exploratory studies and larger sample sizes, has been used in a number of domains, including tourism, health and psychology (Hsu et al., 2006; Fillman et al., 2013; Griffin et al., 2014).
To create our audience segments, we used science values, need for cognition, science media consumption and climate change beliefs as input variables. The TwoStep cluster analysis returned two clusters (see Table 1). A contributing variable is said to be important in predicting the cluster if its average silhouette measure of cohesion and separation is greater than 0.2. For our four variables, each predictive importance (in brackets next to each variable) is greater than 0.2, meaning they are all important components of our cluster. As part of the validation process (Rundle-Thiele et al., 2015), we ran t-tests to confirm that each variable was significantly different from each other between cluster. As reported in Table 1, our four segmenting variables differed significantly across the two clusters, the difference was significant between both groups on science values, need for cognition, science media consumption, and climate change beliefs. Based on this, it was determined that the two-cluster solution was validated for this study.
Both clusters are similar in size. Based on the means of each variable, we defined the first cluster as having a Lower Science Orientation (LSO) and the second cluster as having a Higher Science Orientation (HSO). Compared to those in the LSO, those in the HSO cluster are more likely to view science as more central and important to their daily lives; to think climate change is a serious issue that needs to be resolved; to consume a greater amount of science-themed media content; and to report greater enjoyment and need for complex thinking over simple thinking.
5.2 Segment attitudes toward carbon capture and storage
To examine our two segments and their general attitudes toward CCS, we first carried out a MANOVA to determine if overall attitudes toward CCS were affected by science orientation segment. A MANOVA revealed there was a statistically significant main effect for science orientation segment on CCS awareness, CCS benefits and trust in CCS institutions F(3, 993) = 89, p <.001; Wilks’ Λ = .756.
Follow up t-tests showed the HSO segment was more aware of CCS than the LSO segment [t(995) = -8.152, p<.001], saw CCS as more beneficial than the LSO segment [t(995) = -15.58, p<.001], and was more trusting of institutions to monitor CCS projects than LSO segments [t(995) = -10.90, p<.001]. See Table 2 for mean scores. Given the different attitudes toward CCS across our two segments, it makes sense to compare these two groups in their attitude and behavioral responses related to the CO2 monitoring system.
5.3 Testing our model of CO2 monitoring acceptance and CCS support
To test the model, we ran a series of MANOVAs and linear regressions to examine the mediating role of the TAM and TPB variables on acceptance of the CO2 monitor and CCS support. This was done by first using the experimental stimuli (message: simple v. complex; and social norm: academic/industry v. community/local official) and science orientation segments to predict the TAM and TPB outcomes, which were then added to the model to predict attitude toward the CO2 monitor and intention to use it.
5.3.1 Predicting TAM and TPB outcomes of CO2 monitor type
To examine whether the independent variables predicted the five TAM and TPB variables, a MANOVA was carried out. There was a statistically significant main effect for CO2 monitor type on the combined dependent variables, F(5, 989) = 3.11, p <.01; Wilks’ Λ = .984. There was a statistically significant main effect for science orientation segment on the combined dependent variables, F(5, 989) = 37.55, p <.001; Wilks’ Λ = .84. The effect of social norms on the combined dependent variables was not significant, F(5, 989) = 0.59, p >.05; Wilks’ Λ = .997.
A series of t-tests on each of the five dependent variables was conducted as a follow-up test to the MANOVA for CO2 monitor type and science orientation segment. We ran one-tailed t-tests because, based on prior research, we expected the simple monitoring approach to be associated with more positive scores on our outcomes of interest, for example ease of use and self-efficacy. The norms condition was not included in the t-tests because the MANOVA did not indicate it was significant.
5.3.1.1 CO2 monitor type
There was a significant difference in the perceived ease of use for the simple CO2monitor (M=3.30, SD=1.05) and complex CO2 monitor (M=3.06, SD=1.06) conditions; t(995)=3.44, p <.001. For perceived behavioral control the difference was significant between the simple monitor (M=2.97, SD=1.15) and complex monitor (M=2.77, SD=1.10) conditions; t(995)=2.70, p <.01. There was also a significant difference in perceived subjective norms for the simple monitor (M=3.35, SD=1.06) and complex monitor (M=3.22, SD=1.03) conditions; t(995)=2.70, p <.05. In terms of perceived self-efficacy, there was a significant difference between the simple monitor (M=3.28, SD=1.06) and complex monitor (M=3.15, SD=1.02) conditions; t(995)=1.93, p <.05. There was no significant difference for perceived usefulness between the simple monitor (M=3.40, SD=1.03) and complex monitor (M=3.34, SD=0.94) conditions; t(995)=1.02, p >.05. See Table 3.
5.3.1.2 Science orientation segment
There was a significant difference in the perceived ease of use between the HSO segment (M=3.44, SD=0.94) and LSO segment (M=2.92, SD=0.94) conditions; t(995)=-8.89, p <.001. There was a significant difference for perceived usefulness for the HSO segment (M=3.77, SD=0.91) and LSO segment (M=3.02, SD=0.93) conditions; t(995)=-13.03, p <.001. For perceived behavioral control the difference was significant between the HSO segment (M=3.13, SD=1.13) and the LSO segment (M=2.63, SD=1.08) conditions; t(995)=-7.13, p <.001. When looking at perceived subjective norms, there was a significant difference between the HSO segment (M=3.69, SD=.98) and LSO segment (M=2.92, SD=.98) conditions; t(995)=-12.41, p <.001. Lastly, there was a significant difference in perceived self-efficacy for the HSO segment (M=3.61, SD=.96) and LSO segment (M=2.86, SD=.99) conditions; t(995)=-12.14, p <.001. See Table 4.
5.3.2 Predicting CO2 monitor outcomes
The next step in the analysis was to examine how the experimental stimuli and the TAM and TPB variables influence our outcomes related to the CO2 monitor. The experimental stimuli and TAM and TPB variables were entered as predictors, first to predict attitude to the monitoring system. The next regression includes the same predictor variables but adds attitude toward the monitoring system to predict intention to use the monitor. Finally, to this block of predictors we add intention to use the monitor to predict support for CCS. We ran a series of hierarchical ordinary least squares (OLS) regressions, first on the sample as a whole, then according to our science orientation segments1. See Table 5.
5.3.2.1 Predicting attitude toward the CO2 monitor
The regression model predicting attitude toward the monitor performed well, accounting for 53.4% of the variance for the whole sample and 53.1% and 41.1% for the LSO and HSO segments, respectively. In terms of the experimental stimuli, monitor type was significant among the population as a whole (β = -.05, p<.05) and among the LSO segment (β = -.07, p<.05), with the simple monitoring system yielding more positive attitudes. Among the HSO group, sensor was not significant.
All the TAM and TPB variables were significant predictors of attitude toward the monitor. Among the whole sample, perceiving the CO2 monitor as easy to use (β = –.05, p<.05), more useful (β = .33, p<.001), believing important others valued the monitor (β = .26, p<.001), and feeling greater self-efficacy related to the monitor (β = .25, p<.001) resulted in more positive CO2 monitor attitudes. Interestingly, behavioral control was a significant predictor, but the relationship was negative (β = -.15, p<.001).
Examining our sample by individual segments, these variables all remained significant predictors (see Table 5), except for ease of use. Perceiving the monitor as easy to use (LSO: β = .10, p<.05; HSO: β = –.01, p>.05), as more useful (LSO: β = .33, p<.001; HSO: β = .27, p<.001), believing important others valued the monitor (LSO: β = .26, p<.001; HSO: β = .25, p<.001), and feeling greater self-efficacy related to the monitor (LSO: β = .22, p<.001; HSO: β = .27, p<.001), resulted in more positive monitor attitudes. As with the whole sample, behavioral control was a significant predictor among both segments, but the relationship was negative (LSO: β = –.14, p<.001; HSO: β = –.16, p<.001).
5.3.2.2 Predicting intentions to use the CO2 monitor
The regression model predicting intention to use the CO2 monitor performed well, accounting for 57.8% of the variance for the whole sample and 50.7% and 48.6% for the LSO and HSO segments, respectively. In terms of the experimental stimuli, monitor type was significant among the population as a whole (β = -.07, p<.05) with the simple monitor resulting in greater intention to use the monitor. But among the segments, after adding attitude toward the monitor as a predictor, monitor dropped out as a significant predictor among the LSO segment (β = -.01, p>.05) and the HSO segment (β = .00, p>.05).
Among the sample as a whole all the TAM and TPB variables were significant predictors of intention to use the monitor except for ease of use. Among the whole sample, perceiving the monitor as more useful (β = .23, p<.001), behavioral control (β = .09, p<.001), believing important others valued the monitor (β = .26, p<.001), and feeling more efficacious about using the monitor (β = .21, p<.001) resulted in greater likelihood of using the monitor. Adding attitude to the monitor as predictor showed a significant relationship with intention to use the monitor (β = .13, p<.001).
Examining our sample by individual segments, these variables all remained significant predictors (see Table 5), except for ease of use. Perceiving the monitor as useful (LSO: β = .24, p<.001; HSO: β = .27, p<.001), reporting greater behavioral control (LSO: β = .08, p<.05; HSO: β = .14, p<.01), believing important others valued the monitor (LSO: β = .26, p<.001; HSO: β = .25, p<.001), and feeling greater self-efficacy related to the monitor (LSO: β = .16, p<.01; HSO: β = .25, p<.001), resulted in greater likelihood of using the monitor. Adding attitude to the monitor as a predictor showed a significant relationship with intention to use the monitor (LSO: β = .17, p<.001; HSO: β = .09, p<.05).
5.3.2.3 Predicting Support for CCS
The regression model predicting support for CCS performed well, accounting for 69.2% of the variance for the whole sample and 69.8% and 60.3% for the LSO and HSO segments, respectively. In terms of the experimental stimuli, sensor type dropped out as a directly significant predictor among the sample as a whole and among the science orientation segments.
Among the sample as a whole, three of the TAM and TPB variables remained significant predictors of support for CCS. Among the whole sample, perceiving the monitor as useful (β = .09, p<.001), believing important others valued the monitor (β = .27, p<.001), and feeling more efficacious about using the monitor (β = .08, p<.01) resulted in greater support for CCS. Adding attitude to the monitor as predictor showed a significant relationship with CCS support (β = .28, p<.001), as did adding intention to use the monitor as a predictor of CCS support (β = .19, p<.001).
Examining our sample by individual segments, the patterns among both segments differed. Among the LSO segment, perceived usefulness (β = .08, p<.001), reporting greater behavioral control (β = .06, p<.05), believing important others valued the monitor (β = .23, p<.001) and feeling more efficacious about using the monitor (β = .31, p<.001) were associated with greater CCS support. Among the HSO segment, only two of these remained significant predictors of CCS support: perceived usefulness (β = .10, p<.05) and believing important others valued the monitor (β = .33, p<.001) were associated with greater CCS support. Feelings of greater behavioral control and self-efficacy were not significant predictors of CCS support. Among both segments, a more positive attitude toward the monitor (LSO: β = .31, p<.001; HSO: β = .25, p<.001) and greater intention to use the monitor (LSO: β = .22, p<.001; HSO: β = .14, p<.001) resulted in greater CCS support.
6 Discussion
Public perception is a key element of successful deployment and use of CO2 storage technologies. We set out to understand how residents in Texas, Louisiana and Florida – potential sites for geological CO2 storage – react to different environmental monitoring approaches. We focus here on three main results: 1) keep it simple: the simple, intuitive approach to CO2 monitoring is preferred for our sample as a whole and for the segment we classify as having a “low science orientation”; 2) effects are mediated: rather than operating in a direct effects model, in which the message has a direct and uniform impact on audiences, the impact of simple, intuitive messaging works via attitudes such as ease of use and self-efficacy; and 3) audience segmentation matters: segmenting stakeholders along four important constructs offers an additional layer of insight to understand stakeholder attitudes and behavioral intentions around CCS and CO2 monitoring.
First, the format of the CO2 monitor is important. Rather than providing stakeholders with complex, detailed outputs to convey data about CO2, it is better to provide simple, more direct and easily understood CO2 monitoring outputs. The simple CO2 monitoring system is seen as easier to use than the complex system, while also fostering greater feelings of self-efficacy, behavioral control and the belief that important others value the technology. These factors are, in turn, important predictors of positive attitudes toward the CO2 monitor; and, as a result of a more positive CO2 monitor attitude, individuals are more likely to use the monitor and then to support CCS.
Wallquist et al. (2010) point out the need for simple images and figures to garner public understanding and support among citizens. Further, they point out to avoid unnecessarily complex images which could do more harm than good and promote an increased perception of unwarranted risk. Our results lend support for this advice, as the simple image in our experiment yielded the more positive outcomes. Additionally, the results point to important boundary effects when it comes to providing stakeholders with information about CCS. Specifically, while prior research shows that giving audiences accurate information about CCS bolsters support for the technology, our results indicate what kind of information that should be. Rather than offering detailed, complex content that can inadvertently elicit perceptions of risk (Seigo et al., 2011), our data indicate the information should be accurate and simple. We argue that by offering stakeholders simplified information about CCS monitoring, the industry can adhere to best practices that call for routine information sharing (Greenberg and Gauvreau, 2014) without provoking related issues of risk or perceptions that the technology is not beneficial (Wiedemann et al., 2006).
These findings are especially important when we look at the two different audience segments. Those with a lower science orientation indicate a significantly stronger preference for the simple monitoring approach. Our data show this LSO segment is less aware of CCS, less likely to view it as beneficial, and less likely to trust the technology. However, presenting them with an easy to process monitoring approach offers the possibility of circumventing these concerns. As our model shows, the simple monitoring approach results in more positive attitudes toward the monitoring system, which in turn yields greater support for CCS and likelihood of using the monitor.
Second, intentions to use the CO2 monitor and support for CCS are not directly affected by our message stimuli, but are mediated via the attitudes related to the technology’s use, its social acceptance and feelings of individual ability and self-efficacy. For intentions to use the CO2 monitor, the complexity of the monitor (simple or complex) drops out of the model as a significant predictor once attitude to the monitor is added. Instead of operating directly, the CO2 monitor operates via attitude toward the monitor. Likewise, support for CCS is not directly affected by the CO2 monitor format, which drops out of the model as significant when intention to use the CO2 monitor is added as a predictor. Again, it is through the attitude toward the monitoring system and the intention to use the monitor that greater CCS support is generated.
Our results build on previous work that highlights how communication is a mediated process (Holbert et al., 2003; Shah et al., 2007). When individuals develop attitudes and beliefs about new and emerging technologies like CCS, they do so not in isolation, but take into account subjective norms, trust, and perceived benefits (Hobman and Ashworth, 2013). Our results extend previous work showing that the decision to adopt technology, especially for technology that might seem complex or intimidating, is strongly related to attitudes toward the technology (Bagozzi et al., 1992). That is, a more positive attitude toward the technology is directly related to the likelihood of using it. Our model shows that the simpler approach yields more positive attitudes toward the monitor along with perceptions about its ease of use and usefulness, and beliefs about social norms and self-efficacy. This suggests that in addition to opting for the simple CO2 monitor system, downstream outcomes, like perceptions of ease of use, usefulness and self-efficacy ought to be emphasized and highlighted because these are important predictors of support for the CO2 monitor and CCS support.
Third, audience segment matters. By incorporating constructs that prior research has shown to be important in evaluating new technologies and complex scientific issues, our results demonstrate that audiences can be usefully and effectively segmented into two groups based on high and low orientations to science. We call these groups Higher Science Orientation (HSO) and Lower Science Orientation (LSO). The two segments differ in their attitudes toward CCS. The HSO group is more aware of CCS, sees it as more beneficial and is more trusting that CCS can be regulated and monitored than the LSO segment. As well, it is among the higher science orientation segment that we see greater ease of use, usefulness, feelings of self-efficacy, behavioral control and beliefs that important others value the CO2 monitoring technology.
These findings support prior work that calls for the inclusion of psychographic variables in audience segmentation work (Hine et al., 2014). It is important to go beyond basic demographics to understand how predispositions, like need for cognition and appreciation of science, influence attitudes and beliefs about new and emerging technologies. Our findings build on work in the context of CCS that shows audiences evaluate CCS-related messages differently, depending on the pre-existing attitudes and beliefs (Broecks et al., 2016). These insights offer important guidance on how to communicate with key stakeholder groups, which we discuss in the next section.
7 Implications for policy and practice
Given the differences in the LSO and HSO groups’ attitudes toward CO2 monitoring, it is important to tailor stakeholder engagement about CCS in ways that best speak to each segment. As a result, multiple messages that clarify CCS technology and CO2 monitoring systems should be developed with the different segments in mind. For the LSO group, messages that require less cognitive effort, that focus less on the scientific value of CCS, and that place less emphasis on climate change as a reason for adopting the technology might be more effective among this group. As well, the LSO segment’s preference for media content that is less science-focused can help guide decisions about which channels to focus on broadcasting and promoting these messages. For example, instead of newspaper articles or science documentaries, messages might be more effective if placed in non-traditional formats, such as primetime television shows or social media channels like Instagram and TikTok. Given our LSO’s lower likelihood to consumer science-oriented media content, including information about CCS technology might be better received via these less didactic channels.
Among the HSO group, given their higher scores on beliefs that people who are important to them would value the CCS monitor, outreach efforts could benefit from leveraging these social ties. Communication that emphasizes and strengthens these social norms could help further support for CO2 monitoring and CCS adoption. Relatedly, the HSO segment reported greater feelings of self-efficacy around CO2 monitoring. By harnessing this sense of strength and ability, outreach efforts that take advantage of HSO perceptions that they are capable and effective could also bolster related support attitudes.
Among LSO stakeholders, the leverage point rests with improving perceptions about the CO2 monitor’s usefulness and ease of use. Since subjective norms and self-efficacy are lower within this group, harnessing these two attitudes might not be as effective. Instead, the opportunity lies with outreach and engagement that highlights the simplicity of the technology and its utility to the community.
These results underscore that communication of CO2 storage monitoring schemes could highly benefit from an underlying social understanding of citizen values and social norms in Texas, Louisiana and Florida. For example, considering that the simple monitoring scheme is preferred in general by the entire citizen sample, this should be the preferred method of monitoring in this context, ceteris paribus. The different monitoring preferences in our results show the need for broad stakeholder engagement at the stage of designing and choosing monitoring strategies. This motivates the need for interdisciplinary teams and underlines the role that social science should play in helping to facilitate these dialogues and to contextualize and make sense of concerns and expectations of citizens.
In addition, our results reflect critical RRI and real-time technology assessment principles of anticipation and stakeholder engagement (Guston and Sarewitz, 2002; Stilgoe, 2013) and the need to dialogue with both science and industry experts and community leaders before disseminating information to citizens. This implies that radically interdisciplinary teams, where natural science integrates with social science and the humanities, thereby extending peer communities (Funtowicz and Ravetz, 1991) should collaborate with community leaders in the communication and dissemination of innovative CCS monitoring schemes. These collaborations could be pivotal to laying the groundwork for successful Carbon Capture and Storage investments and projects.
Data availability statement
The original contributions presented in the study are included in the article/supplementary material. Further inquiries can be directed to the corresponding author.
Ethics statement
The Institutional Review Board (IRB) at the University of Texas at Austin is charged with reviewing all research conducted under the auspices of UT Austin. The purpose of an IRB is to ensure research involving humans applies ethical principles and complies with federal regulatory requirements for protecting the rights and welfare of human participants. UT Austin has 2 IRBs – a Social Behavioral research IRB and a Health Science research IRB. The human research protection program and the UT Austin IRBs have been accredited by the Association for the Accreditation of Human Research Participants (AAHRPP) since 2006. The studies were conducted in accordance with the local legislation and institutional requirements. The participants provided their written informed consent to participate in this study.
Author contributions
KR devised the concept and LA, DD, and KR contributed to the study design. LA and DD designed the theoretical approach. LA performed the statistical analysis and wrote the first draft of the manuscript. DD, KR, and LA wrote sections of the manuscript. All authors contributed to the article and approved the submitted version.
Funding
The author(s) declare financial support was received for the research, authorship, and/or publication of this article. This project, ACTOM, is funded through the ACT program (Accelerating CCS Technologies, Horizon2020 Project No 294766). Financial contributions made from; The Research Council of Norway, (RCN), Norway, Ministry of Economic Affairs and Climate Policy, the Netherlands, Department for Business, Energy & Industrial Strategy (BEIS) together with extra funding from NERC and EPSRC research councils, United Kingdom, US-Department of Energy (US-DOE), USA. In-kind contributions from the University of Bergen are gratefully acknowledged. This work is also supported by the U.S. Department of Energy under Award Number DE-FE0031558.
Conflict of interest
The authors declare that the research was conducted in the absence of any commercial or financial relationships that could be construed as a potential conflict of interest.
Publisher’s note
All claims expressed in this article are solely those of the authors and do not necessarily represent those of their affiliated organizations, or those of the publisher, the editors and the reviewers. Any product that may be evaluated in this article, or claim that may be made by its manufacturer, is not guaranteed or endorsed by the publisher.
Author disclaimer
This report was prepared as an account of work sponsored by an agency of the United States Government. Neither the United States Government nor any agency thereof, nor any of their employees, makes any warranty, express or implied, or assumes any legal liability or responsibility for the accuracy, completeness, or usefulness of any information, apparatus, product, or process disclosed, or represents that its use would not infringe privately owned rights. Reference herein to any specific commercial product, process, or service by trade name, trademark, manufacturer, or otherwise does not necessarily constitute or imply its endorsement, recommendation, or favoring by the United States Government or any agency thereof. The views and opinions of authors expressed herein do not necessarily state or reflect those of the United States Government or any agency thereof.
Footnotes
- ^ There is some debate about employing parametric tests like OLS regression when using Likert-type questions. However, past research shows the risk of errors or biases in estimates are not a concern when the scale has more than four answer categories and the sample size is greater than 300 (Hagen and Pijawka, 2015; Taylor et al., 2006; Kim and Kim, 2020; Liao et al., 2022).
References
Ahn H., Park E. (2022). For sustainable development in the transportation sector: Determinants of acceptance of sustainable transportation using the innovation diffusion theory and technology acceptance model. Sustain. Dev. 30 (5), 1169–1183. doi: 10.1002/sd.2309
Ajzen I. (1991). The theory of planned behavior. Organizational Behav. Hum. Decision Processes 50 (2), 179–211. doi: 10.1016/0749-5978(91)90020-T
Alam S. S., Hashim N. H. N., Rashid M., Omar N. A., Ahsan N., Ismail M. D. (2014). Small-scale households renewable energy usage intention: Theoretical development and empirical settings. Renewable Energy 68, 255–263. doi: 10.1016/j.renene.2014.02.010
Ashworth P., Boughen N., Mayhew M., Millar F. (2010). From research to action: Now we have to move on CCS communication. Int. J. Greenhouse Gas Control 4 (2), 426–433. doi: 10.1016/j.ijggc.2009.10.012
Ashworth P., Littleboy A., Pisarski A., Beath A., Thambimuthu K. (2006). Understanding and incorporating stakeholder perspectives to low emission technologies in Australia. (Brisbane: Commonwealth Scientific and Industrial Research Organisation (CSIRO)).
Bagozzi R. P., Davis F. D., Warshaw P. R. (1992). Development and test of a theory of technological learning and usage. Hum. Relations 45 (7), 659–686. doi: 10.1177/001872679204500702
Broecks K. P., van Egmond S., van Rijnsoever F. J., Verlinde-van den Berg M., Hekkert M. P. (2016). Persuasiveness, importance and novelty of arguments about Carbon Capture and Storage. Environ. Sci. Policy 59, 58–66. doi: 10.1016/j.envsci.2016.02.004
Cacioppo J. T., Petty R. E. (1982). The need for cognition. J. Pers. Soc. Psychol. 42 (1), 116. doi: 10.1037/0022-3514.42.1.116
Chen C.-D., Fan Y.-W., Farn C.-K. (2007). Predicting electronic toll collection service adoption: An integration of the technology acceptance model and the theory of planned behavior. Transportation Res. Part C: Emerging Technol. 15 (5), 300–311. doi: 10.1016/j.trc.2007.04.004
Cheon J., Lee S., Crooks S. M., Song J. (2012). An investigation of mobile learning readiness in higher education based on the theory of planned behavior. Comput. Educ. 59 (3), 1054–1064. doi: 10.1016/j.compedu.2012.04.015
Cialdini R. B., Cialdini R. B. (2007). Influence: The psychology of persuasion Vol. 55 (Collins New York: Harper Collins).
Clement S. (2016). Has someone cracked the code on making Internet polls more accurate? Washington Post. Available at: https://www.washingtonpost.com/news/the-fix/wp/2016/05/02/has-someone-cracked-the-code-on-making-internet-polls-more-accurate/.
Cohen G. L., Sherman D. K., Bastardi A., Hsu L., McGoey M., Ross L. (2007). Bridging the partisan divide: Self-affirmation reduces ideological closed-mindedness and inflexibility in negotiation. J. Pers. Soc. Psychol. 93 (3), 415. doi: 10.1037/0022-3514.93.3.415
Davis F. D. (1989). Perceived usefulness, perceived ease of use, and user acceptance of information technology. MIS Q., 319–340. doi: 10.2307/249008
DeAngelo M. V., Fifariz R., Meckel T., Treviño R. H. (2019). A seismic-based CO2-sequestration regional assessment of the Miocene section, northern Gulf of Mexico, Texas and Louisiana. Int. J. Greenhouse Gas Control 81, 29–37. doi: 10.1016/j.ijggc.2018.12.009
de Best-Waldhober M., Daamen D., Faaij A. (2009). Informed and uninformed public opinions on CO2 capture and storage technologies in the Netherlands. Int. J. Greenhouse Gas Control 3 (3), 322–332. doi: 10.1016/j.ijggc.2008.09.001
Dixon T., Romanak K. D. (2015). Improving monitoring protocols for CO2 geological storage with technical advances in CO2 attribution monitoring. Int. J. Greenhouse Gas Control 41, 29–40. doi: 10.1016/j.ijggc.2015.05.029
Dowd A.-M., Itaoka K., Ashworth P., Saito A., de Best-Waldhober M. (2014). Investigating the link between knowledge and perception of CO2 and CCS: An international study. Int. J. Greenhouse Gas Control 28, 79–87. doi: 10.1016/j.ijggc.2014.06.009
Fatoki O. (2022). Determinants of intention to purchase photovoltaic panel system: An integration of technology acceptance model and theory of planned behaviour. Int. J. Energy Economics Policy 12 (3), 432–440. doi: 10.32479/ijeep.12931
Fillman S., Cloonan N., Catts V., Miller L., Wong J., McCrossin T., et al. (2013). Increased inflammatory markers identified in the dorsolateral prefrontal cortex of individuals with schizophrenia. Mol. Psychiatry 18 (2), 206–214. doi: 10.1038/mp.2012.110
Füchslin T., Schäfer M. S., Metag J. (2019). Who wants to be a citizen scientist? Identifying the potential of citizen science and target segments in Switzerland. Public Understanding Sci. 28 (6), 652–668. doi: 10.1177/0963662519852020
Funtowicz S. O., Ravetz J. R. (1991). A new scientific methodology for global environmental issues. Ecol. Economics: Sci. Manage. Sustainability 10, 137. doi: 10.1016/0016-3287(93)90022-L
Green K. C., Armstrong J. S. (2015). Simple versus complex forecasting: The evidence. J. Business Res. 68 (8), 1678–1685. doi: 10.1016/j.jbusres.2015.03.026
Greenberg S. E., Gauvreau L. M. (2014). Communicating science and technology while engaging the public at the Illinois Basin–Decatur Project. Greenhouse Gases: Sci. Technol. 4 (5), 596–603. doi: 10.1002/ghg.1435
Griffin B., Sherman K. A., Jones M., Bayl-Smith P. (2014). The clustering of health behaviours in older Australians and its association with physical and psychological status, and sociodemographic indicators. Ann. Behav. Med. 48 (2), 205–214. doi: 10.1007/s12160-014-9589-8
Guston D. H., Sarewitz D. (2002). Real-time technology assessment. Technol. Soc. 24 (1–2), 93–109. doi: 10.1016/S0160-791X(01)00047-1
Hagen B., Pijawka D. (2015). Public perceptions and support of renewable energy in North America in the context of global climate change. Int. J. Disaster Risk Sci. 6, 385–398. doi: 10.1007/s13753-015-0068-z
Hansen J. M., Saridakis G., Benson V. (2018). Risk, trust, and the interaction of perceived ease of use and behavioral control in predicting consumers’ use of social media for transactions. Comput. Hum. Behav. 80, 197–206. doi: 10.1016/j.chb.2017.11.010
Hensel P. J., Bruner G. C. (1992). Scaling and measurement: Multi-item scaled measures in sales related research. J. Pers. Selling Sales Manage. 12 (3), 77–82.
Hine D. W., Reser J. P., Morrison M., Phillips W. J., Nunn P., Cooksey R. (2014). Audience segmentation and climate change communication: Conceptual and methodological considerations. Wiley Interdiscip. Reviews: Climate Change 5 (4), 441–459. doi: 10.1002/wcc.279
Hobman E. V., Ashworth P. (2013). Public support for energy sources and related technologies: The impact of simple information provision. Energy Policy 63, 862–869. doi: 10.1016/j.enpol.2013.09.011
Holbert R. L., Kwak N., Shah D. V. (2003). Environmental concern, patterns of television viewing, and pro-environmental behaviors: Integrating models of media consumption and effects. J. Broadcasting Electronic Media 47 (2), 177–196. doi: 10.1207/s15506878jobem4702_2
Hsu C. H., Kang S. K., Lam T. (2006). Reference group influences among Chinese travelers. J. Travel Res. 44 (4), 474–484. doi: 10.1177/0047287505282951
Hua L., Wang S. (2019). Antecedents of consumers’ intention to purchase energy-efficient appliances: An empirical study based on the technology acceptance model and theory of planned behavior. Sustainability 11 (10), 2994. doi: 10.3390/su11102994
IPCC (2023). “IPC: summary for policymakers. In: climate change 2023: synthesis report.,” in Contribution of working groups I, II and III to the sixth assessment report of the intergovernmental panel on climate change. (Geneva, Switzerland: IPCC). Available at: https://doi.org/10.59327/IPCC/AR6-9789291691647.001.
Itaoka K. (2006). “A path analysis for public survey data on social acceptance of CO_2 capture and storage technology,” in 8th International Conference on Greenhouse Gas Control Technologie (Trondheim, Norway).
Kahan D. (2010). Fixing the communications failure. Nature 463 (7279), 296–297. doi: 10.1038/463296a
Kahlor L. A., Yang J., Li X., Wang W., Olson H. C., Atkinson L. (2020). Environmental risk (and benefit) information seeking intentions: The case of carbon capture and storage in Southeast Texas. Environ. Communication 14 (4), 555–572. doi: 10.1080/17524032.2019.1699136
Kim S., Kim S. (2020). Analysis of the impact of health beliefs and resource factors on preventive behaviors against the COVID-19 pandemic. Int. J. Environ. Res. Public Health 17 (22), 8666. doi: 10.3390/ijerph17228666
Kraeusel J., Möst D. (2012). Carbon Capture and Storage on its way to large-scale deployment: Social acceptance and willingness to pay in Germany. Energy Policy. 49, 642–651. doi: 10.1016/j.enpol.2012.07.006
Leiserowitz A. (2006). Climate change risk perception and policy preferences: The role of affect, imagery, and values. Climatic Change 77 (1), 45–72. doi: 10.1007/s10584-006-9059-9
Leiss W., Larkin P. (2019). Risk communication and public engagement in CCS projects: The foundations of public acceptability. Int. J. Risk Assess. Manage. 22 (3–4), 384–403. doi: 10.1504/IJRAM.2019.103339
Lewis A. L., Eves F. F. (2012). Prompts to increase stair climbing in stations: The effect of message complexity. J. Phys. Activity Health 9 (7), 954–961. doi: 10.1123/jpah.9.7.954
Liao Q. V., Zhang Y., Luss R., Doshi-Velez F., Dhurandhar A. (2022). Connecting algorithmic research and usage contexts: A perspective of contextualized evaluation for explainable AI. Proc. AAAI Conf. Hum. Comput. Crowdsourcing 10 (1), 147–159. doi: 10.1609/hcomp.v10i1.21995
Lotenberg L. D., Schechter C., Strand J. (2011). “Segmentation and targeting,” in The SAGE handbook of social marketing. Eds. Hastings G., Angus K., Bryant &C.A. (Sage Thousand Oaks, CA: Publisher), 125–135.
Maibach E. (2019). “Increasing public awareness and facilitating behavior change: Two guiding heuristics,” in Climate change and biodiversity (New Haven, CT: Yale University Press).
Milfont T. L. (2012). The interplay between knowledge, perceived efficacy, and concern about global warming and climate change: A one-year longitudinal study. Risk Analysis: Int. J. 32 (6), 1003–1020. doi: 10.1111/j.1539-6924.2012.01800.x
Moon W.-K., Kahlor L. A., Olson H. C. (2020). Understanding public support for carbon capture and storage policy: The roles of social capital, stakeholder perceptions, and perceived risk/benefit of technology. Energy Policy 139, 111312. doi: 10.1016/j.enpol.2020.111312
Moore G. C., Benbasat I. (1991). Development of an instrument to measure the perceptions of adopting an information technology innovation. Inf. Syst. Res. 2 (3), 192–222. doi: 10.1287/isre.2.3.192
Office of Fossil Energy and Carbon Management (2023) U.S. Department of Energy Announces its Intent to Launch a Responsible Carbon Management Initiative. Available at: https://www.energy.gov/fecm/articles/us-department-energy-announces-its-intent-launch-responsible-carbon-management.
Omar A. M., García-Ibánez M. I., Schaap A., Oleynik A., Esposito M., Jeansson E., et al. (2021). Detection and quantification of CO2 seepage in seawater using the stoichiometric Cseep method: Results from a recent subsea CO2 release experiment in the North Sea. Int. J. Greenhouse Gas Control 108, 103310. doi: 10.1016/j.ijggc.2021.103310
Pogrebnyakov N., Maldonado E. (2018). Didn’t roger that: Social media message complexity and situational awareness of emergency responders. Int. J. Inf. Manage. 40, 166–174. doi: 10.1016/j.ijinfomgt.2018.02.004
Ringrose P. S., Meckel T. A. (2019). Maturing global CO2 storage resources on offshore continental margins to achieve 2DS emissions reductions. Sci. Rep. 9 (1), 1–10. doi: 10.1038/s41598-019-54363-z
Rodriguez K. L., Gambino F. J., Butow P. N., Hagerty R. G., Arnold R. M. (2008). ‘It’s going to shorten your life’: Framing of oncologist–patient communication about prognosis. Psycho-Oncology: J. Psychological Soc. Behav. Dimensions Cancer 17 (3), 219–225. doi: 10.1002/pon.1223
Romanak K. D., Bennett P., Yang C., Hovorka S. D. (2012). Process-based approach to CO2 leakage detection by vadose zone gas monitoring at geologic CO2 storage sites. Geophysical Res. Lett. 39 (15). doi: 10.1029/2012GL052426
Romanak K. D., Dixon T. (2022). CO2 storage guidelines and the science of monitoring: Achieving project success under the California Low Carbon Fuel Standard CCS Protocol and other global regulations. Int. J. Greenhouse Gas Control 113, 103523. doi: 10.1016/j.ijggc.2021.103523
Romanak K. D., Wolaver B., Yang C., Sherk G. W., Dale J., Dobeck L. M., et al. (2014). Process-based soil gas leakage assessment at the Kerr Farm: Comparison of results to leakage proxies at ZERT and Mt. Etna. Int. J. Greenhouse Gas Control 30, 42–57. doi: 10.1016/j.ijggc.2014.08.008
Rundle-Thiele S., Kubacki K., Tkaczynski A., Parkinson J. (2015). Using two-step cluster analysis to identify homogeneous physical activity groups. Marketing Intell. Planning 33 (4), 522–537. doi: 10.1108/JSM-03-2015-0112
Runge K. K., Brossard D., Xenos M. A. (2018). Protective progressives to distrustful traditionalists: A post hoc segmentation method for science communication. Environ. Communication 12 (8), 1023–1045. doi: 10.1080/17524032.2018.1513854
Safeena R., Date H., Hundewale N., Kammani A. (2013). Combination of TAM and TPB in internet banking adoption. Int. J. Comput. Theory Eng. 5 (1), 146. doi: 10.7763/IJCTE.2013.V5.665
Schäfer M. S., Füchslin T., Metag J., Kristiansen S., Rauchfleisch A. (2018). The different audiences of science communication: A segmentation analysis of the Swiss population’s perceptions of science and their information and media use patterns. Public Understanding Sci. 27 (7), 836–856. doi: 10.1177/0963662517752886
Seigo S. L., Arvai J., Dohle S., Siegrist M. (2014). Predictors of risk and benefit perception of carbon capture and storage (CCS) in regions with different stages of deployment. Int. J. Greenhouse Gas Control 25, 23–32. doi: 10.1016/j.ijggc.2014.03.007
Seigo S. L., Wallquist L., Dohle S., Siegrist M. (2011). Communication of CCS monitoring activities may not have a reassuring effect on the public. Int. J. Greenhouse Gas Control 5 (6), 1674–1679. doi: 10.1016/j.ijggc.2011.05.040
Shachak A., Kuziemsky C., Petersen C. (2019). Beyond TAM and UTAUT: Future directions for HIT implementation research. Journal of Biomedical Informatics. 100 103315. doi: 10.1016/j.jbi.2019.103315
Shah D. V., McLeod D. M., Kim E., Lee S. Y., Gotlieb M. R., Ho S. S., et al. (2007). Political consumerism: How communication and consumption orientations drive “lifestyle politics.“. Ann. Am. Acad. Political Soc. Science 611 (1), 217–235. doi: 10.1177/0002716206298714
Stilgoe J. (2013). Developing a framework for responsible innovation.. Research Policy 42, 1568–1580. doi: 10.1016/j.respol.2013.05.008
Su L. Y.-F., Akin H., Brossard D., Scheufele D. A., Xenos M. A. (2015). Science news consumption patterns and their implications for public understanding of science. Journalism Mass Communication Q. 92 (3), 597–616. doi: 10.1177/1077699015586415
Taylor S., Todd P. A. (1995). Understanding information technology usage: A test of competing models. Inf. Syst. Res. 6 (2), 144–176. doi: 10.1287/isre.6.2.144
Taylor A. B., West S. G., Aiken L. S. (2006). Loss of power in logistic, ordinal logistic, and probit regression when an outcome variable is coarsely categorized. Educ. psychol. Measurement 66 (2), 228–239. doi: 10.1177/0013164405278580
Tkaczynski A. (2017). “Segmentation using two-step cluster analysis,” in Segmentation in social marketing (Springer: Tzaczynski), 109–125.
Uchimoto K., Nishimura M., Kita J., Xue Z. (2018). Detecting CO2 leakage at offshore storage sites using the covariance between the partial pressure of CO2 and the saturation of dissolved oxygen in seawater. Int. J. Greenhouse Gas Control 72, 130–137. doi: 10.1016/j.ijggc.2018.03.020
U.S. Energy Information Administration (2021) State carbon dioxide emissions data. Available at: https://www.eia.gov/environment/emissions/state/.
Wallquist L., Visschers V. H., Siegrist M. (2010). Impact of knowledge and misconceptions on benefit and risk perception of CCS (ACS Publications).
Wiedemann P. M., Thalmann A. T., Grutsch M. A., Schütz H. (2006). The impacts of precautionary measures and the disclosure of scientific uncertainty on EMF risk perception and trust. J. Risk Res. 9 (4), 361–372. doi: 10.1080/13669870600802111
Yankelovich D., Meer D. (2006). Rediscovering market segmentation. Harvard Business Rev. 84 (2), 122.
Yu J., Ha I., Choi M., Rho J. (2005). Extending the TAM for a t-commerce. Inf. Manage. 42 (7), 965–976. doi: 10.1016/j.im.2004.11.001
Appendix
Experimental stimuli showing complex monitoring message and scientist social norms (left panel) and simple monitoring message and community social norms (right panel).
Keywords: CCS, monitoring, environmental monitoring, technology acceptance, stakeholder acceptance, audience segmentation
Citation: Atkinson L, Dankel DJ and Romanak KD (2024) The effect of monitoring complexity on stakeholder acceptance of CO2 geological storage projects in the US gulf coast region. Front. Mar. Sci. 10:1154543. doi: 10.3389/fmars.2023.1154543
Received: 30 January 2023; Accepted: 20 December 2023;
Published: 16 January 2024.
Edited by:
Hirdan Costa, University of São Paulo, BrazilReviewed by:
Hiroki Wakamatsu, Forestry and Fisheries, JapanLeslie Mabon, The Open University, United Kingdom
Copyright © 2024 Atkinson, Dankel and Romanak. This is an open-access article distributed under the terms of the Creative Commons Attribution License (CC BY). The use, distribution or reproduction in other forums is permitted, provided the original author(s) and the copyright owner(s) are credited and that the original publication in this journal is cited, in accordance with accepted academic practice. No use, distribution or reproduction is permitted which does not comply with these terms.
*Correspondence: Katherine D. Romanak, a2F0aGVyaW5lLnJvbWFuYWtAYmVnLnV0ZXhhcy5lZHU=