- 1Quantitative and Evolutionary STEM Training (QuEST) Program, University of Vermont, Burlington, VT, United States
- 2Department of Biology, University of Vermont, Burlington, VT, United States
- 3Department of Plant and Soil Science, University of Vermont, Burlington, VT, United States
- 4Department of Computer Science, University of Vermont, Burlington, VT, United States
- 5Alaska Department of Fish and Game, Douglas, AK, United States
Sea star wasting (SSW) disease, a massive and ongoing epidemic with unknown cause(s), has led to the rapid death and decimation of sea star populations with cascading ecological consequences. Changes in microbial community structure have been previously associated with SSW, however, it remains unknown if SSW-associated dysbiosis is a mechanism or artifact of disease progression, particularly in wild populations. Here, we compare the microbiomes of the sunflower sea star, Pycnopodia helianthoides, before (Naïve) and during (Exposed and Wasting) the initial outbreak in Southeast Alaska to identify changes and interactions in the microbial communities associated with sea star health and disease exposure. We found an increase in microbial diversity (both alpha and beta diversity) preceding signs of disease and an increase in abundance of facultative and obligate anaerobes (most notably Vibrio) in both Exposed (apparently healthy) and Wasting animals. Complementing these changes in microbial composition was the initial gain of metabolic functions upon disease exposure, and loss of function with signs of wasting. Using Bayesian network clustering, we found evidence of dysbiosis in the form of co-colonization of taxa appearing in large numbers among Exposed and Wasting individuals, in addition to the loss of communities associated with Naïve sea stars. These changes in community structure suggest a shared set of colonizing microbes that may be important in the initial stages of SSW. Together, these results provide several complementary perspectives in support of an early dysbiotic event preceding visible signs of SSW.
Introduction
Changes in global climate have expanded the range of many infectious diseases while the added stress from increased natural disasters, habitat loss, thermal extremes, and anthropomorphic impacts have left many species more vulnerable to infection by pathogens old and new (Daszak et al., 2001; Omazic et al., 2019; Price et al., 2019). As a result, many organisms have been experiencing an increased frequency and spread of disease outbreaks worldwide (Bartlow et al., 2019). Beginning in the summer of 2013, an outbreak of Sea Star Wasting (SSW) began decimating sea star populations along the west coast of North America. SSW disease has come to be recognized as one of the largest marine epizootics ever observed due to its wide geographic range from Mexico to Southern Alaska, and its impact on over 20 different asteroid species (Hewson et al., 2014). Cases of SSW can be traced back as early as 1896 in the eastern US (Mead et al., 1898), and small-scale wasting events have been recorded on the west coasts of North America since the 1970’s (Menge, 1979; Dungan et al., 1982; Jangoux, 1986; Eckert et al., 2000; Bates et al., 2009). However, none of these past events compare to the scale of wasting seen since 2013. SSW is of critical ecological concern as it threatens some of the most important keystone predators, resulting in large-scale impacts on biodiversity, community structure, and loss of essential habitats, such as kelp forests, which are home for many organisms and serve as nurseries for important fisheries (Menge et al., 2016). The sunflower star, Pycnopodia helianthoides, is among the most heavily impacted asteroids and has virtually disappeared from its native ranges from California to Oregon (Harvell et al., 2019). Now critically endangered, the remaining populations of P. helianthoides take refuge in northern Canada and Alaska (Harvell et al., 2019).
The etiology of SSW remains unknown. A wide array of signs commonly observed in wasting sea stars include loss of body turgor, ectodermal discoloration, puffiness, limb autonomy (twisting and curling), body wall lesions, and disintegrating tissue (Hewson et al., 2014). The disease progresses and spreads quickly, with death occurring in a matter of days and outbreaks can spread to encompass thousands of miles within months (Harvell et al., 2019). Some studies have suggested transmission through a water-borne infectious agent spreading from infected to healthy animals sharing the same aquaria (Hewson et al., 2014). In many cases, warmer ocean temperatures have coincided with wasting events (Bates et al., 2009; Staehli et al., 2009; Harvell et al., 2019), and cooler temperatures have been shown to improve asteroid survival (Kohl et al., 2016). However, cooling water temperatures coincided with increased wasting in one study off the coast of Oregon, suggesting that temperature alone is not the sole driver of SSW outbreaks (Menge et al., 2016). A controversial suggestion posits that SSW is not caused by a single causative agent, but a combination of factors including environmental change, abiotic stress (Aalto et al., 2020), undefined pathogens, and dysbiosis of the organisms’ microbiome (Lloyd and Pespeni, 2018; Aquino et al., 2020). Although early investigations implicated a densovirus as a plausible agent of the disease (Hewson et al., 2014), later studies failed to replicate these findings in other affected asteroid species in addition to the presence of the densovirus in apparently naïve individuals (Jackson et al., 2020).
Recent studies have begun investigating changes in microbial communities in association with SSW disease (Lloyd and Pespeni, 2018; Aquino et al., 2020). Host-microbe interactions play a crucial role in the development, nutrient acquisition, behavior, and pathogen resistance of most multicellular organisms (Peixoto et al., 2021). The immune system and the microbiome function synergistically to maintain homeostasis and deter pathogens; compromising one often results in the collapse of the other and may lead to immune dysregulation and increased susceptibility to infection (Peixoto et al., 2021). This disruptive process, termed microbial dysbiosis, can be broadly defined as any changes in the composition and/or function of resident microbial communities relative to those found in healthy individuals. This may result from pathobiont expansion, loss of microbial diversity, and/or loss of beneficial microbes (Petersen and Round, 2014).
Infections of microbial pathogens have been previously linked to a wide range of mass mortality events afflicting echinoderms (Scheibling, 1986; Tajima et al., 1996; Scheibling and Hennigar, 1997; Tajima et al., 2007; Becker et al., 2008; Delroisse et al., 2020). Alterations in microbial community composition have been recorded with disease onset and progression in the ochre sea star, Pisaster ochraceous (Lloyd and Pespeni, 2018). The proliferation of anaerobic bacteria at the animal-water interface, as a result of increased primary production and organic matter buildup during following warmer temperatures, has also been implicated as an early mechanism of disease onset by limiting dissolved oxygen supply to infected asteroids (Aquino et al., 2020). While the impact of SSW on the microbiome has been investigated in controlled laboratory conditions (Lloyd and Pespeni, 2018; Aquino et al., 2020), the function of the microbiome in natural marine systems remains understudied. Monitoring of natural communities and their associated microbiomes is critical to understanding the resilience of these communities in the presence and persistence of the recent wasting outbreaks. Investigations into these interactions, in both healthy and diseased organisms, will set the foundations for long-term investigations into the evolutionary and adaptive potential of the microbiome to environmental change at a scale that is difficult to replicate in laboratory settings (Leray et al., 2021).
In this study, we analyze the microbiome of the sunflower star, Pycnopodia helianthoides, as a factor of apparent health status and exposure at multiple field sites. Here, we collected tissue biopsies from wild sea stars sampled at seven field sites in Southeast Alaska when the disease was just starting to emerge in 2016 (Figure S1). At the time of collection, some impacted sites were completely overtaken by the disease, with all sea stars showing signs of wasting, while less severely impacted sites had a mixture of wasting and apparently healthy asteroids. Apparently healthy sea stars were collected from sites where wasting was observed (Exposed), alongside animals actively showing signs of wasting (Wasting). Sea stars were also collected from locations yet to show any signs of wasting (Naïve), however, there was no way to certify the absence of the disease-causing agent due to its unknown etiology. To better understand differences between a naïve, exposed, and actively wasting microbiome, we examined patterns of diversity within and between samples, identified differentially abundant taxa that may play a role in disease progression, and inferred metabolic pathways enriched or depleted between compared groups. Specific communities of samples and microbial taxa with similar patterns of abundance were then found using a Bayesian network clustering analysis based on hierarchical stochastic block models, a statistically rigorous alternative to traditional clustering approaches which were recently used to study host-microbiome interactions in the human gut (Cobo-López et al., 2022). Together these analyses provide several complementary perspectives in support of dysbiosis as a mechanism of SSW disease and its onset.
Materials and methods
Sample collection
Samples were collected in the summer of 2016 off the coast of Southeast Alaska (Figure S1). With the aid of SCUBA, a biopsy from a single ray was collected underwater from each sampled sea star, and isolated until in a wet lab aboard a research vessel (R/V Kestrel, Alaska Department of Fish and Game). Epidermal biopsy samples were collected from sea stars at both impacted and naïve sites at depths ranging from 7 to 18 meters. Seven total sites were sampled (2 Naïve and 5 impacted) with a total of 18 Wasting, 20 Exposed, and 47 Naïve individuals sampled (total N=85). Nonlethal biopsy punches (3.5mm diameter biopsy punch, Robbins Instruments, Chatham, NJ) were taken from the body wall of each individual and preserved in RNAlater (ThermoFisher Scientific, Waltham, MA) in 2ml tubes. Only epidermal body wall tissue was sampled, even when sampling wasting individuals. For individuals displaying SSW symptoms, wasting epidermal tissue at the edge of the lesion was sampled. All biopsy tissue samples were shipped to Vermont on dry ice and stored at −80°C until processing.
RNA extraction and cDNA reverse transcription
RNA was extracted from each biopsy using a modified TRIzol protocol (TRIzol reagent ThermoFisher Scientific, Waltham, MA). After lysing tissue in 250ul TRIzol, it was homogenized for 20 minutes with a plastic pestle with 750ul additional TRIzol using a Vortex Genie2 (Scientific Industries, Bohemia, NY). To extract RNA, 200 ul chloroform (ThermoFisher Scientific, Waltham, MA) was added, inverted 15 times, incubated for 3 minutes at RT, and centrifuged at 4°C for 15 minutes at 12,000×g. The RNA-containing supernatant was transferred to a new tube and the previous step was repeated. Adding 500 ul isopropanol (ThermoFisher Scientific, Waltham, MA) and 1 ul 5 mg/ml glycogen (Invitrogen, Carlsbad, CA), incubation for 10 minutes at room temperature, and centrifugation for 5minutes at 7500×g at 4°C precipitated the RNA from the supernatant. After drying for 10 minutes at RT the RNA pellet was dissolved in 50 ul nuclease-free water. A NanoDrop 2000 Spectrophotometer (ThermoFisher Scientific, Waltham, MA) and Qubit 3.0 Fluorometer (Life Technologies, Carlsbad, CA) were used to measure the quality and quantity of the RNA extractions. To check for DNA contamination, we performed negative amplification PCR (16S PCR amplification parameters below). Random hexamer primers reverse transcribed cDNA with SuperScript IV (Invitrogen, Carlsbad, CA).
16S PCR amplification and sequencing
To amplify the V3 and V4 region of the 16S bacterial gene, we used the primers: forward 5′TCGTCGGCAGCGTCAGATGTGTATAAGAGACAGCCTACGGGNGGCWGCAG and reverse 5′GTCTCGTGGGCTCGGAGATGTGTATAAGAGACAGGACTACHVGGGTATCTAATCC (Klindworth et al., 2013). 25 ul PCR reactions (1X MiFi Mix (Bioline, Toronto, Canada), 200nM each primer, and 2ul cDNA) were run with the following conditions: 95°C for 3 minutes followed by 25 cycles of 95°C for 30 seconds, 55°C for 30 seconds, and 72°C for 30 seconds, with a final extension at 72°C for 5 minutes. To clean the PCR products, AMPure XP beads (Beckman Coulter, Brea, CA) and MiSeq indexing adapters were added. The indexed PCR products were cleaned again with AMPure XP beads, following Illumina 16S metagenomic sequencing library preparation protocol. To validate band size, the cleaned, indexed PCR products were run on a 2% agarose gel. 16S rRNA Library sequencing was performed at the Cornell Biotechnology Resource Center (Ithaca, NY) using 2 × 300 base pair overlapping paired-end reads on an Illumina MiSeq platform.
Sequence data processing, taxonomic assignment, and diversity metrics
Sequences were demultiplexed and barcode sequences were removed by the core facility. QIIME2 (v.2-2021.8) was used for data cleaning and analysis (Bokulich et al., 2018). Paired end reads were imported into QIIME2 and read quality was assessed using QIIME2’s ‘qiime_demux_summarize’ function. Read quality was determined by Phred score (>30) then subsequently denoised and trimmed using DADA2 (Callahan et al., 2016), which removes errors and noise from data sequenced by Illumina, and creates information about the removed data. DADA2 parameters were set at trimming forward reads at 16bp and truncating at 289bp and reverse reads were trimmed at 0bp and truncated at 257bp. Diversity metrics were run using the ‘qiime_diversity_core-metrics-phylogenetic’ function with an Amplicon Sequence Variant (ASV) sampling depth of 13,547 to ensure maximal sample depth without omitting any samples. ASV richness (alpha diversity) of Naïve, Exposed, and Wasting asteroids was calculated using the Shannon diversity index using the qiime2 plugin and the between-group diversity (beta diversity) was calculated using the weighted_unifrac_distance_matrix to take into account the relative abundance of ASVs shared between samples (Lozupone et al., 2006). One sample (HH02_18) was responsible for all identified outliers in our beta-diversity analysis between Naïve samples, and was removed. Variation among Naïve individuals was 22.6% outlier-inclusive, and 19.9% with outliers removed (Figure 1D). Diversity plots were made using Qiime2 outputs visualized in GraphPad Prism version 9.3.0 for windows.
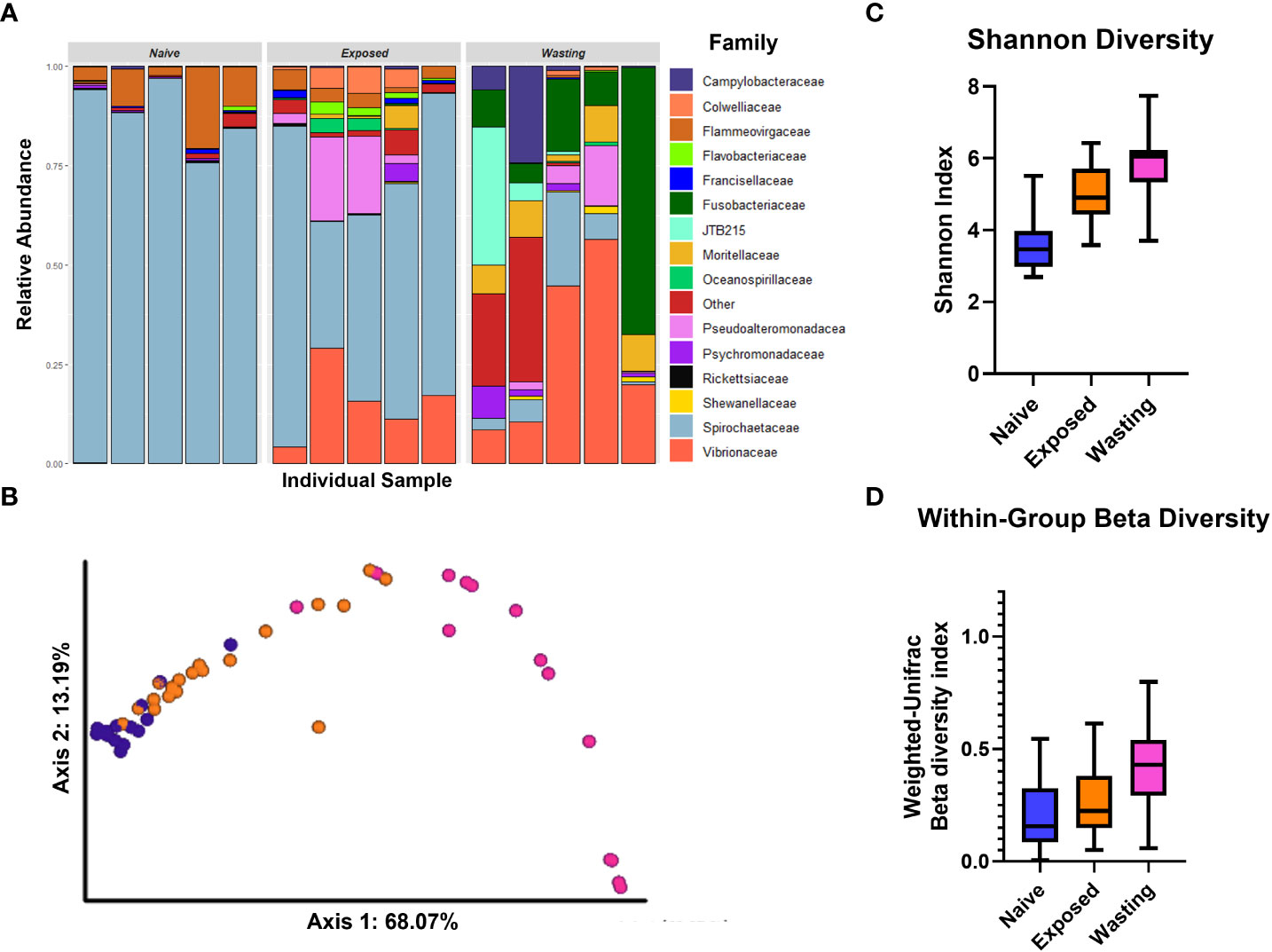
Figure 1 Divergence in microbial communities associated with site-health status. (A) Relative abundance of microbial families of five randomly selected individuals from each comparison group. (B) PCoA Emperor plots of the weighted UniFrac distance based on diversity of taxa present on samples in each site-health status; Naïve sea stars (blue), Exposed (orange) and Wasting (pink). (C) Shannon Diversity and (D) Within-Group Beta Diversity of taxa present on P. helianthoides by site-health status. The diversity of taxa differed between and within groups of Naïve and Exposed (P < 0.001) and Exposed and Wasting (P < 0.001).
Taxonomic classification
Taxonomy was assigned to each ASV by using the q2-feature-classifier (Bokulich et al., 2018) then trained and mapped to known bacterial taxa classified by the Greengenes database (McDonald et al., 2012). Greenegene reference sequences were trimmed to match our data with a minimum length of 100bp and a maximum of 500bp. ASVs mapped to the same taxonomic classification were collapsed into Observable Taxonomic Units (OTUs) at the lowest level of identification provided by Greengenes database using the “qiime_taxa_collapse”. It should be noted that not all taxa were identifiable to the species level. However, ASV’s assigned to the same class, family, or genus could still be identified as distinct OTUs based on phylogenetic mapping, even if Greengenes could not confidently assign a specific species identification. For example, there are two separate classifications assigned to the genus Vibrio with no further classification at the species level, yet retained distinct abundances tracked separately through the analyses. Respiratory profiles (e.g., aerobic, facultative anaerobic, and obligate anaerobic) of microbes of interest were inferred from literature, as cited, describing the genus and/or family.
Differential abundance
To test for differential abundance of microbial communities associated with exposure and onset of SSW, we used Analysis of Composition of Microbiomes with Bias Correction (ANCOM-BC) (Lin and Peddada, 2020) in R version 4.2.1 (Team, 2021). ANCOM-BC differential abundance analysis uses a series of pairwise comparisons to estimate the average abundance of a given microbial species through linear regression while correcting the bias induced by differences among samples. This method provides false discovery rate (FDR) corrected p-values for each taxon and confidence intervals for differentially abundant taxa. Differentially abundant taxa were defined based on FDR < 0.05 and taxa that were not present in at least 10% of the compared samples were dropped from the analysis. The W score represents the number of times the null-hypothesis (the average abundance of a given species in a group is equal to that in the other group) was rejected for a given species. Beta value represents the effect size as a log-linear (natural log) value relative between compared groups. Fold change was calculated by taking ebeta. Violin plots were constructed using log-10 transformed values from the raw abundance of each taxa using GraphPad Prism version 9.3.0 for windows.
Bipartite clustering analysis
To identify taxonomic communities and other high-level patterns of abundance, we used Bayesian stochastic blockmodeling, a principled approach to network clustering which finds statistically significant partitions in a network. First, a bipartite network was constructed by assigning sea star samples and ASVsOTUs to separate node groups. Samples and taxa were then connected with an edge if the taxa was present, with an edge weight of log(aij)+1, where aij > 0 is the abundance of taxa j in sample i. OTU abundance was aggregated to the species level, and taxa with total abundance less than 100 across all samples were removed. Samples and ASVs were assigned to hierarchical groups following the hierarchical stochastic block model (hSBM), with the maximum a posteriori estimate found using a specialized Markov chain Monte Carlo algorithm (Peixoto, 2020). We used the “degree-corrected” variant of the model (Peixoto, 2017), since it had a smaller minimum description length than the simpler non-corrected model (Figure S4). Analyses were performed using graph-tool version 2.44 (Peixoto, 2014).
Functional profiling
Phylogenetic Investigation of Communities by Reconstruction of Unobserved States version 2 (PICRUSt2) was used to predict the functional content of the microbial communities (Douglas, et al., 2020). PICRUSt2 uses extended ancestral-state reconstruction of unknown microbes and a library of reference genomes to predict which gene families [by KEGG orthology (KO) (Kanehisa et al., 2016) and EC: enzyme functions] are present. PICRUSt2 also uses these data to infer abundances of metabolic pathways using a map of gene families to pathways from the online metacyc database (Caspi et al., 2018) (https://metacyc.org/). Predicted KO abundance was predicted by Picrust2 from the corresponding metagenomes from the 16s rRNA marker gene and KEGG functional hierarchies at level 2 were mapped using the KEGGREST v1.36 (Tenenbaum and Maintainer, 2021) package in R version 4.2.1 (Team, 2021) to infer biological functional enrichment between the sample groups. Because KO mapping to KEGG orthologs provides predictions for all possible pathways (including eukaryotes), predicted eukaryotic categories were filtered from the analysis. The PICRUSt2 output was visualized with Morpheus (https://software.broadinstitute.org/morpheus). Predicted metacyc pathways (Table S3) and KEGG pathway functional categories differentially abundant between 1) Naive and Exposed, and 2) Exposed and Wasting individuals were identified by t-test with Benjamini Hochburg False Discovery Rate correction (Padj < 0.01 and Padj < 0.05 respectively).
Results
Microbial diversity increases with disease exposure and symptom onset
The relative proportions of microbes differed greatly between the three groups, Naïve, Exposed and Wasting, most notably in the progressive decrease of proportion of Spirochaetaceae and increases in Vibrionaceae and Fusobacteriaceae (Figure 1A). PCoA analysis revealed Naïve sea stars clustered strongly together and away from Wasting sea stars along PC1 accounting for 68% of the variation among samples (Figure 1B). Exposed sea stars were in between Naïve and Wasting along PC1, but clustered closer to Naïve samples in unconstrained multivariate space despite their greater geographic distance (Figure 1B). Naïve and Exposed sea stars however differed along a composite of both PC1 and PC2 (Figure 1B). The estimated number of microbial taxa (alpha diversity) differed across all three site-health groups (Shannon diversity index, P < 0.05; Figure 1C). Naïve sea stars had the lowest diversity of microbial taxa. Exposed sea stars showed an increase in microbial diversity in comparison to the Naïve group, while Wasting sea stars had the highest microbial diversity overall (Figure 1C).
Similarly, the composition of microbial taxa, beta diversity, was different across all site-health status groups (Weighted Unifrac distance; pairwise post-hoc tests: P < 0.001). Beta diversity increased with disease exposure and onset at impacted sites with the lowest diversity recorded in the Naïve group and the highest in the Wasting group (Figure 1D). The within-group beta diversity measures the differences in microbial communities between individuals of the same group, and indicates a similar trend of increasing diversity with disease exposure and symptoms. The larger the within-group beta diversity, the more dissimilar the microbial communities are between individuals of the same group. All three groups differed from each other (pairwise PERMANOVA test, P < 0.001). The Naïve and Exposed groups had a relatively low within group beta diversity with an average variation of 19.9% in the Naïve samples, 26.1% variance in the Exposed samples, suggesting that individuals within each group share relatively similar communities of microbes with other members of their group. However, the Wasting group had a variance of 41.7% indicating that individuals in the Wasting population had greater dissimilarity in their microbial composition.
Identifying differentially abundant microbial taxa associated with SSW disease
To identify microbes associated with exposure and onset of disease signs, we tested for the differential abundance of microbes identified by taxonomic assignment between samples characterized by exposure and health status. Of the 685 distinctly identified taxa, 90 were differentially abundant between Naïve and Exposed groups (50 up, 40 down in Exposed relative to Naïve) and 76 were differentially abundant between Exposed and Wasting (35 up, 41 down in Wasting relative to Exposed) (FDR < 0.05) (Figure 2A). A full list of relative fold change of each distinct taxa is available in Table S1.
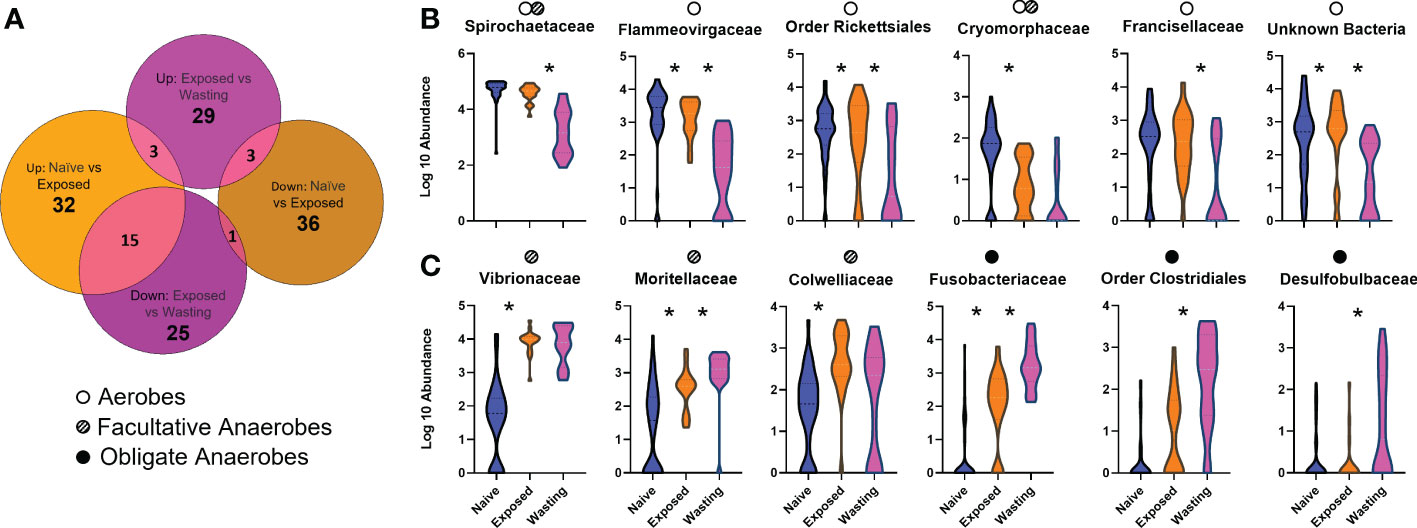
Figure 2 Differential abundances of taxa associated with site-health status (A) Number of differentially abundant OTUs identified to the species level in each comparison: Naïve vs Exposed (orange: relative to Naïve group) and Exposed vs Wasting (pink: relative to Exposed group) (Padj < 0.05). (B, C) Violin plots depicting log10-transformed abundance values of 12 differentially abundant microbial taxa, grouped by Order or Family as patterns of abundance were similar between members of the same taxonomic classification; Naïve samples (blue), Exposed (orange), and Wasting (pink). Microbial taxa were identified as aerobic (open circle), facultative anaerobes (striped circle), and obligate anaerobes (solid circle) based on respiratory function often characterized by taxa members. Multiple dots denote a family which includes species of more than one respiratory type. The "*" symbol represents statistically significant differences between the abundances of the displayed taxa between the site-health groups it lies between.
The analysis of differentially abundant taxa from Naïve to Exposed groups revealed an increase in taxa containing members regularly associated with facultatively anaerobic processes with disease exposure (Table 1). These taxa include seven taxa in the family Vibrionaceae (Photobacterium sp, Aliivibrio sp, Vibrio tapetis, Vibrio cyclitrophicus, Unknown sp, Vibrio sp1, Vibrio sp2; 13.4 to 1233.3 fold increase); two genera of Fusobacteriaceae (Propionigenium sp, Psychrilyobacter sp; 2.4 to 32.9 fold increase); three taxa of the family Colwelliaceae (Unknown sp, Thalassomonas sp, Colwellia austuarii; 6.4 to 21.5 fold increase); the genus Moritella (15.4 fold increase), and the family JTB215 in the Order Clostridia (6.4 fold increase) (Figure 2C, Table 1). In addition to the gain of anaerobic microbes, we observed a decrease in only a few notable taxa often characterized with aerobic function including the genus Crocinitomix in the family Cryomorphaceae (12.3 fold decrease); Psychrobacter sanguinis in the family Moraxellaceae (11.9 fold decrease); an unknown genus of Xenococcaceae (8.9 fold decrease); and Rothia nasimurium (5.7 fold decrease) of the Micrococcaceae family (Figure 2B, Table 1).
Differential abundances of taxa from Exposed to Wasting groups revealed further increases in taxa abundance with physiological signs of disease, including those with members frequently identified with obligate anaerobic processes (Table 1). These taxa include further increases of the two genera of Fusobacteriaceae (Propionigenium, Psychrilyobacter; 7.5 to 22.8 fold increase); five taxa in the order Clostridiales (family Peptostreptococcaceae, genus Clostridium, Clostridiales sp1, family JTB215, genus Fusibacter, Clostridiales sp2; 1.5 to 16.7 fold increase); a single unknown species of Spirochaeta sp (8.5), two species of the family Desulfobulbaceae (Desulfotalea sp and Unknown sp; 1.8, 4.4 fold increase); an unknown genus of Desulfobacteraceae (4.2 fold increase); and minor increases in the seven Vibrionaceae taxa mentioned above (1.0 to 3.4 fold increase) (Figure 2C, Table 1). Taxa exhibiting the greatest decreases in abundance with signs of disease included some families containing strictly aerobic species as well as some unidentifiable taxa. We noted a decreased abundance of an unknown genus of the family Flammeovirgaceae (53.9 fold decrease); an unknown genus of Spirochaetaceae spp (24.6 fold decrease) and two unknown orders in the class Spirochaetes spp (4.9 to, 9.1 fold decrease); two altogether unknown bacterial phyla (16.7 to 17.9 fold decrease); an unknown genus in the family Francisellaceae (10.4 fold decrease); and further decreases in Crocinitomix in the family Cryomorphaceae (1.7 fold decrease) (Figure 2B, Table 1).
Co-occurring microbial communities associated with healthy, exposed, and sick sea stars
To identify communities of similarly abundant microbes, and the communities of sea stars where these microbes occur, we used Bayesian network clustering analysis based on hierarchical stochastic block models. The best fit partition from the analysis, presented in Figure 3, demonstrated a highly heterogeneous community structure. At the lowest level in the hierarchy, there were 56 groups for 85 samples, and 103 groups for the 264 OTUs, which shows a high level of model complexity was required to explain the data. The group hierarchy clearly distinguished the three health conditions of the samples. At the highest level of clustering (level 2) there were three distinctive clusters for the samples, two of which comprised all but three of the Naïve samples, and one which contained all the Exposed and Wasting samples except one. The next level then tended to separate exposed and wasting samples, with 50% of Wasting individuals falling into a single group (Figure S2). The identified OTU clusters also showed a relationship with the health status of the animals on which they appear, with some occurring primarily on Naïve samples, and others primarily by Exposed and/or Wasting samples (Figure 3, right side). A full list of cluster membership is available in Table S2.
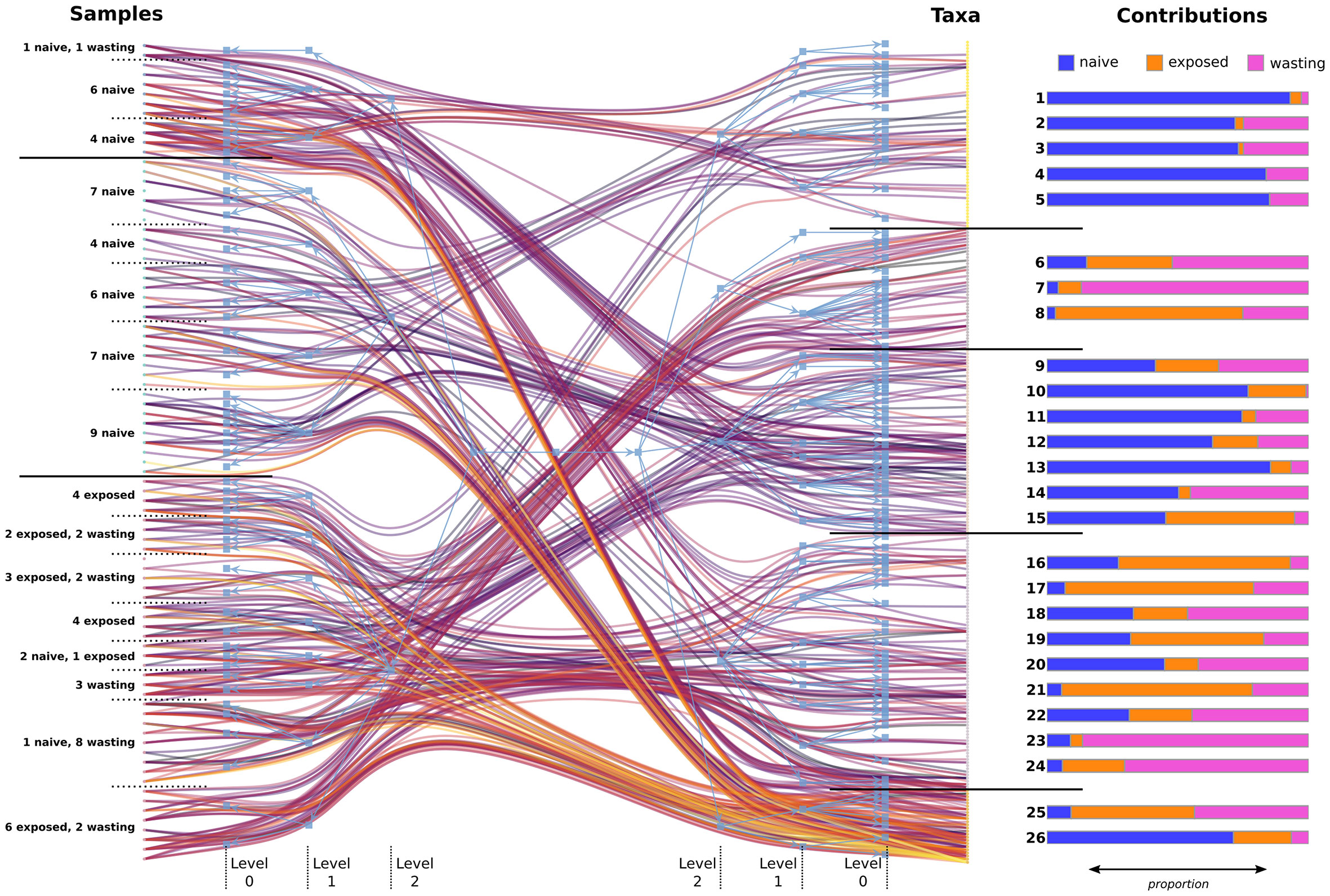
Figure 3 Statistically similar communities of samples and taxa found using hierarchical bipartite clustering. The maximum a posteriori partition is shown in blue, while a random sample of 400 links from the original abundance network is shown ranging from purple (lowest abundance) to orange (highest abundance). Levels of the partition tree have been labeled as they are referenced in the text, with horizontal black bars separating communities at the highest level of clustering (level 2). Contributions: the proportion of total abundance contributed from samples based on site-health status, for each level-1 OTU block. OTU blocks 1-24 show low abundance and uneven spread across samples whereas blocks 25 and 26 show high abundance and even spread among contributing samples (Figure S3). Level-1 OTU block membership can be found in Table S2.
The hierarchical partitions also allowed us to identify regions of high and low diversity in the data, which helps clarify communities driving the observed trends in alpha and beta diversity (Figures 1C-D). Figure S3 shows the Shannon entropy of each sample/OTU, grouped according to their level-1 block membership. We found the average diversity between blocks was significantly different than random (Welch’s one-way test; F = 17.3, p < 0.001 for sample blocks; F = 29.7, p < 0.001 for OTU blocks). Among sample blocks, those belonging to the middle level-2 block of Figure 3, which contained the majority of Naïve samples and no impacted samples, had notably lower diversity compared to the other blocks. However, the 11 Naïve and 1 Wasting sample from the top level-2 block appear to have comparable alpha diversity to the bottom level-2 block containing mostly impacted samples.
Subcommunities of taxa with high entropy were also identified, particularly taxa belonging to level-1 blocks 25 and 26. These communities were also highly abundant (Figure 3, left), which shows these taxa jointly occurred at high levels across the samples they appeared in. Taxa belonging to these two blocks can be thought of as “backbone” communities that are both highly abundant and consistent across their respective groups. The Naïve-driven backbone (level-1, block 26) included taxa in the families Spirochaetaceae, Flammeovirgaceae, Francisellaceae, Rickettsiaceae, Flavobacteriaceae, Helicobacteraceae, and two altogether unknown bacterial species. Many Exposed individuals shared this Naïve backbone but gained the addition of a secondary backbone (level-1, block 25), mostly void of Naïve contribution, and which also appeared in Wasting samples. This impacted backbone of co-colonizing taxa was composed of the family Vibrionaceae (Vibrio sp1., Photobacterium sp. and Aliivibrio sp.), Moritella sp., Shewanella sp., and Pseudoalteromonas porphyrae. Conversely, blocks with consistently low entropy tended to also have lower relative abundance. Taxa in these blocks were unevenly distributed across samples, and likely represent communities of stochastically colonizing microbes which have a minor effect on overall microbiome function.
Predicted metabolic pathways and KEGG enrichment vary with exposure and onset of SSW
To test for functional divergence in the microbes between Naïve, Exposed and Wasting asteroids, we predicted metabolic metacyc pathways and KEGG Ortholog (KO) functional enrichment using PICRUSt2. Pathway enrichment revealed 106 pathways that increased from Naïve to Exposed groups and only 3 pathways that declined (Figure 4B; Padj < 0.01 by a t-test with Benjamini Hochburg correction). These pathways included NAD salvage and biosynthesis of essential amino acids, phosphates, nucleotides, and sugars such as sucrose (Table S3). Additionally, glycolysis and the TCA cycle had numerous pathways enriched from Naïve to Exposed groups as well as the enrichment of sulfate assimilation and degradation which is a strictly anaerobic pathway (Jurtshuk, 2011). The pathways found to be depleted in Exposed compared to Naïve included pathways associated with phospholipases, starch degradation III, and vitamin E biosynthesis (tocopherols). KEGG functional categories mapped to the second hierarchy level revealed increases in predicted genes involved in “Xenobiotic Biodegradation and Metabolism” and “Cellar Processing and Signaling” and no significant decreases (Padj < 0.05; Figure 4A).
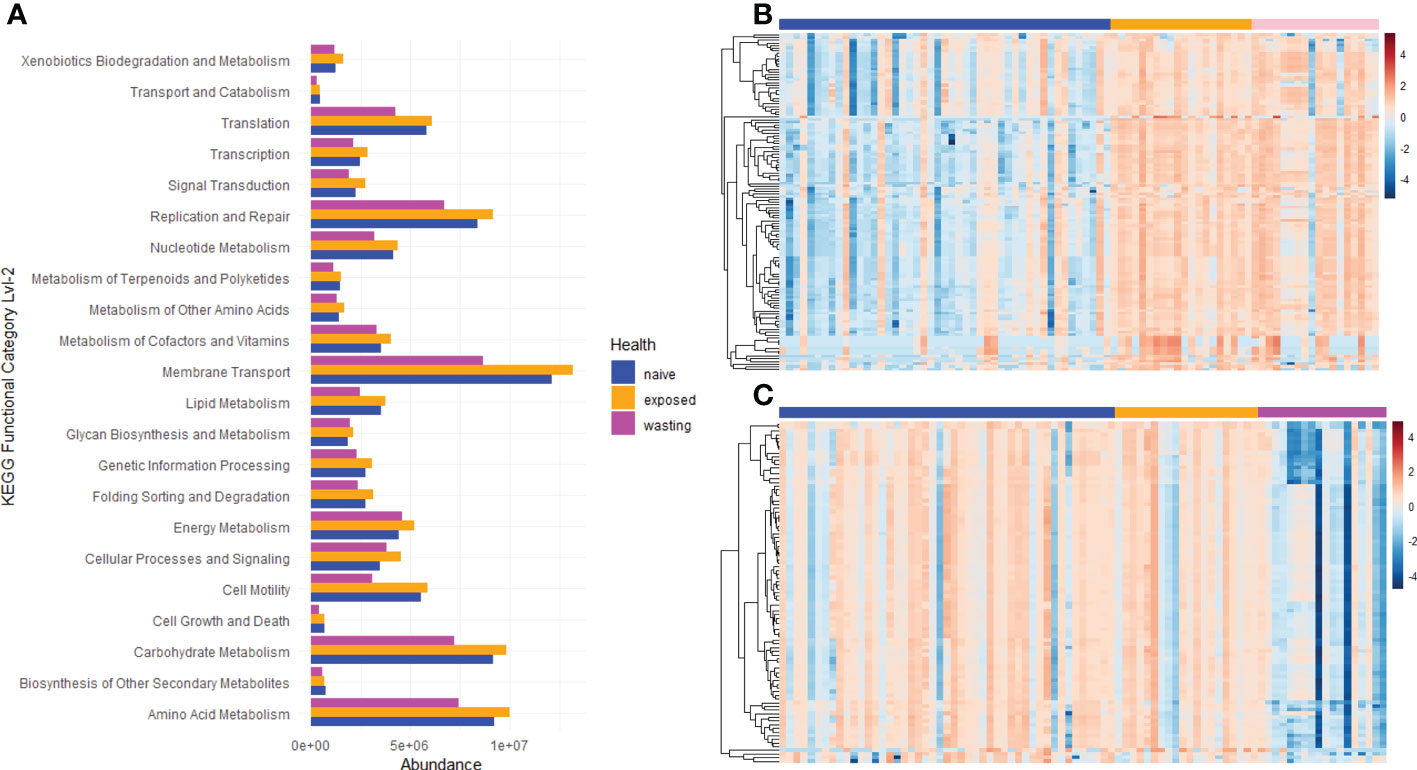
Figure 4 Metabolic Pathway and Kegg Ortholog Enrichment/Depletion associated with site-health status. (A) KEGG functional classification of predicted KEGG Orthology terms. Listed terms differ significantly between Naïve ~Exposed or Exposed~Wasting groups as determined by t-test with Benjamini Hochburg False Discovery Rate correction (Padj< 0.05). Metacyc pathway enrichment trends between (B) Naïve (blue bar) vs Exposed (orange bar) and (C) Exposed (orange bar) vs Wasting (pink bar).
In contrast, no pathways were enriched between Exposed and Wasting groups, yet 30 were found to be depleted (Figure 4C; Padj < 0.01). These depleted pathways included L-glutamate and L-glutamine biosynthesis, Salvage and biosynthesis of pyrimidine nucleosides and ribonucleosides, branch chain amino acid biosynthesis, and glycolysis (Table S3). 13 of the pathways that were enriched from Naïve to Exposed groups were then reduced from Exposed to Wasting (padj < 0.01). These included pathways associated with glycolysis, biosynthesis of nucleotide building blocks, and homolactic fermentation. KEGG functional categories mapped to the second hierarchy level revealed depletion in predicted genes involved in “Cell Growth and Death”, “Translation”, “Membrane Transport”, and various elements of metabolism with no categories increasing (Padj < 0.05; Figure 4A). In sum, there was a general gain in pathways/function from Naïve to Exposed groups, then a loss of pathways from Exposed to Wasting.
Discussion
Microbial communities play an important role in maintaining the health of their hosts by providing protection against harmful pathogens and aiding in the metabolism of organic compounds (Peixoto et al., 2021). Changes to the core microbiome, defined as any set of commonly occurring microbial taxa, or functional attributes associated with those taxa, can leave the host susceptible to disease (Neu et al., 2021; Peixoto et al., 2021). The goal of this study was to compare the microbiomes of sea stars completely naïve to SSW to those recently exposed and actively afflicted by the disease in the field. Though our analysis, we were able to identify 1) a pre-symptomatic increase in microbial diversity, 2) an increase in abundance of facultative and obligate anaerobes (most notably Vibrio) accompanied by changes in metabolic function, and 3) a consistent co-colonization of taxa in large numbers among impacted individuals, as well as a shift in the less abundant, more stochastic communities found in Naïve samples to entirely different communities in Exposed and Wasting samples. Our results reveal changes to the core microbiome of P. helianthoides preceding physiological signs characteristic of SSW and thus supports the hypothesis that an early dysbiotic event plays a key role in disease progression of SSW in the field, particularly in the proliferation of anaerobic taxa.
The initial role of host microbiomes in SSW was characterized by Lloyd and Pespeni 2018, demonstrating the progressive shifts in microbial community composition through the stages of wasting. Further studies on the mechanisms of these shifts were proposed by Aquino et al. in 2021, hypothesizing that increases in organic matter (such as algal blooms, nutrient runoff, or pollutants) could alter the interactions of microbes at the asteroid-water interface, and lead to copiotrophic proliferation, appearance of facultative anaerobes, and eventual colonization of strictly anaerobic taxa which may compromise host respiratory and immune functions. Our findings in these field samples further support these current theories in the etiology of SSW by revealing an early dysbiotic event characterized by a consistent suite of facultative anaerobes (i.e.,Vibrionaceae, Moritellaceae, Colwelliaceae) (Imhoff, 2005; Bowman, 2014; Urakawa, 2014) across Exposed individuals followed by an increase of obligate anaerobes (i.e.,Clostridiales, Fusobacteriaceae) (Olsen, 2014; Stackebrandt, 2014) and other opportunistic taxa in the Wasting asteroids (Figure 2; Figure 3). Like all previous studies on SSW, except one (Hewson et al., 2014) which was later revised (Hewson et al., 2018), we did not find evidence for a single causative agent responsible for outbreaks of SSW, however, the unknown Vibrio species showing a 1200-fold increase with exposure is worth further investigation. Our results and others suggest that the cause of SSW is likely a complex interaction between environmental stressors, undefined pathogens, and changes in the host-immune system that lead to a dysbiotic microbial community perpetuating SSW disease.
The Anna Karenina principle of animal microbiomes (Zaneveld et al., 2017) states the microbial community composition of diseased individuals varies more than healthy individuals. In the present study, comparing Naïve to Exposed to Wasting individuals, opportunistic microbiota increased with each stage in a progressively stochastic manner. For exposed and wasting sea stars, there was an increase in taxa diversity and dissimilarity between samples with SSW exposure and onset of signs. Exposed sea stars showed marked changes in community composition even before signs of the disease were present. However, what is most interesting is how these communities changed. The increase in microbial richness (alpha diversity) from Naïve to Exposed groups along with the increase in heterogeneity in microbial composition between individuals of the same group (within-group beta diversity), indicates an initial recruitment or proliferation of opportunistic taxa across Exposed individuals before symptom onset. The initial shifts in microbiota in Exposed asteroids may be a stepping-stone leading to the higher diversity observed between members of the Wasting group, indicating a more opportunistic colonization and a stochastic progression of dysbiosis coinciding with the onset of disease as anticipated by the Anna Karenina principle of dysbiotic states.
Some variation in microbiome composition may be expected from the geographic distance between Naïve and Impacted sampling sites, which were approximately 70km apart (Figure S2). However, recent studies on the structure of coral microbiomes suggest that host species have the largest influence on microbial composition, demonstrating relative stability across space and time within a host (Dunphy et al., 2019). Additionally, we found greater similarity between Naïve and Exposed samples (both apparently healthy) than we did between Exposed and Wasting (both at impacted sites) within our PCoA plot (Figure 1B), supporting the idea that disease state, not geographic distance, is driving the observed variations in microbial community structure.
Further evidence of dysbiosis was found in the form of consistent co-colonization of taxa appearing in large numbers among impacted individuals (Figure 3, level-1, block 25; Table S2), forming a backbone community unique to the impacted samples. Unsurprisingly, the taxa in this block consisted of some of the most differentially abundant facultative anaerobes to appear between Naïve and Exposed samples in our microbial abundance comparison, including Vibrionaceae (Vibrio sp1., Photobacterium sp. and Aliivibrio sp.), genus Moritella sp., Shewanella sp., and Pseudoalteromonas porphyrae (Figure 2). This suggests a shared set of microbes that may be important in the initial stages of SSW disease in exposed animals. Additionally, the community of microbes occurring both highly abundant and consistent across Naïve samples (Figure 3, level-1, block 26) seems to be less common across impacted samples. Members of this “healthy” backbone include taxa with the largest observed decreases in our differential abundance comparison including some species of Spirochaetaceae, Flammeovirgaceae, both unidentified bacteria phyla, and two members of the order Rickettsiales (Figure 2, Figure 3, Table S2). This “healthy” backbone could be thought of as the core-microbiome of Naïve sea stars, and its reduced presence across Exposed and Wasting animals further highlights the microbial imbalance caused by SSW.
Of all the taxa that increased with disease exposure and symptom onset, members of the genus Vibrio are of particular interest due to their historical role in the pathology of many marine animals, including echinoderms, strong differential abundance trends (Table 1, Figure 2), and presence of multiple species in the backbone community of Impacted samples in our clustering analysis (Figure 3, block 25 Table S2). Previous studies have demonstrated the proliferation of Vibrionaceae species throughout the progression of SSW (Lloyd and Pespeni, 2018; Aquino et al., 2020), have been regularly identified in the decaying tissue of wasting sea stars prior to the current 2013 outbreaks, and associated with many other echinoderms diseases (Eckert et al., 2000; Becker et al., 2004; Staehli et al., 2009; Kohl et al., 2016; Hira and Stensvåg, 2022). Several species of Vibrio are known to cause intestinal and extraintestinal infections in humans and animals (Farmer and Hickman-Brenner, 2006), V. coralliilyticus is known to cause tissue damage and necrosis in corals (de O Santos et al., 2011), and V. echinoideorum was recently found to facilitate the development of lesion syndrome in the green sea urchin (Hira and Stensvåg, 2022). Interestingly, NCBI Blast results placed V. echinoideorum with the highest total-score for of the top 5 ASVs with the largest fold-change when comparing Naïve to Exposed sea stars. Additionally, a 2011 study focusing on population control methods of the Crown of thorns Sea Star, Acanthaster planci, used a thiosulfate-citrate-bile-sucrose agar (TCBS) to selectively stimulate the growth of Vibrionaceae species (Rivera-Posada et al., 2011a; Rivera-Posada et al., 2011b). Not only did the TCBS stimulation of Vibrionaceae species elicit SSW-like signs and mortality, but a follow up study in 2012 demonstrated an interspecies transmissibility of the TCBS-induced disease between different species of asteroids (Caballes et al., 2012). Taken together, these results suggest an important role for Vibrio in SSW, though future work in which the abundance of Vibrio is manipulated would be needed to test this hypothesis.
While Vibrio species may be sufficient in causing disease in some cases, other opportunistic taxa may also play an important pathogenic role, taking advantage of a weakened immune system and further contributing to the anaerobic and lethal nature of SSW. A number of the differentially abundant taxa identified in the present study are linked to disease in humans and animals. Microbes in the order Clostridiales are implicated in several human intestinal diseases due to the secretion of harmful toxins (Bauer and Kuijper, 2017). Some members of Fusobacteriaceae are known to cause skin infections and topical ulcers on their hosts through the secretion of harmful metabolites (Olsen, 2014). Additionally, Moritellaceae has been characterized as a fish pathogen with severe economic impact, forming lesions on their teleost hosts (Urakawa, 2014). The mechanistic role in disease progression of the anaerobes that increase with signs of SSW remains up for debate. It is likely that the suffocating properties of these proliferating anaerobes are not the sole driver of disease. Transcriptomic profiling of affected P. helianthoides unveiled a number of immune systems, tissue remodeling, and neural genes in response to SSW, which could be more than expected from anaerobic suffocation alone (Fuess et al., 2015).
We observed a gain and loss of taxa to a similar degree in each site-health comparison, yet generally only increases in pathway and KEGG functional category enrichment upon exposure and loss of pathways and KEGG enrichment with wasting signs (Figure 2A; Figure 4). Taken together, the initial increase of pathway functions may reflect a general colonization and/or proliferation of facultative anaerobes with exposure to SSW, while the low number of depleted pathways may reflect the loss of less-impactful taxa and/or an overlap in metabolic processes between those gained and lost. The transition to wasting signs is accompanied by the loss of pathway functions which may signify the loss of putatively beneficial microbes, and the lack of pathway enrichment with the onset of wasting may signify an overlap of pathway functions that were already increased by the initial SSW exposure. This suggests that only a few of the differentially expressed taxa may be responsible for the marked changes in pathway enrichment observed in this study, most likely related to the taxa with the largest effect size addressed above. However, 16S ribosomal RNA sequencing alone is not enough to fully characterize the metabolic roles of the colonizing taxa we have observed through the progression of SSW, and thus we are hesitant to make assumptions based on specific metabolic roles predicted in this analysis. Metagenomic and metabolomic analyses will be necessary to further characterize the molecular functions of the microbes and the potential role of secondary metabolites, secreted toxins, and virulence factors in the progression of this disease.
Polymicrobial diseases are difficult to study and thus-far poorly characterized in marine systems due to the variety of yet-unidentified microbes living in the oceans. However, many of these diseases share commonalities. Black band disease in corals, for example, is caused by the proliferation of cyanobacteria, sulfide-oxidizing, and sulfate-reducing bacteria on and around coral tissues similar to the taxa identified in this study (Sato et al., 2017). Other marine diseases, such as Pacific Oyster Mortality syndrome (PCOM), Bald Urchin Disease, and SKin Ulceration Disease (SKUD) are the result of opportunistic, and non-specific, bacterial colonization (Becker et al., 2008; Delroisse et al., 2020; Petton et al., 2021). PCOM was linked to a virally induced immunocompromised state acting as the catalyst for microbial dysbiosis and the opportunistic colonization by pathogenic bacteria eventually leading to animal death (Petton et al., 2021). Nonspecific viral infection such as Sea Star Associated DensoVirus (SSWaDV), along with climate anomalies, reduced dissolved oxygen, pollutants, and other biotic/abiotic stressors could all weaken the sea star’s immune response and promote the colonization of dysbiotic microbes. The impact of one or the cumulative effect of multiple stressful factors may tip the homeostasis of the microbial communities driving them toward dysbiosis and disease. While non-specific and opportunistic colonization is supported in many studies, our findings suggest certain microbial taxa may be more likely than others to initiate these early dysbiotic stages of SSW disease, acting as a steppingstone for more opportunistic pathogens to take hold.
Conclusion
The results of this study reveal a dysbiotic event preceding visible signs of wasting disease, driven by a few key taxa, and the persistence of these communities through the progression of the disease. The proliferation of specific microbes (most notably members of the genus Vibrio) prior to signs of disease implicates changes in biotic and abiotic conditions that may underlie the SSW epidemic, particularly in the case of microbial pathogens. In the future, extending approaches to better understand the host-microbe interactions at a higher resolution will allow investigators to better identify key microbes involved in both healthy and diseased states, their related metabolic functions, and subsequent consequences of microbiome disruption as a result of biotic and abiotic stress. Additionally, the function of the core microbiome in many natural systems remains unknown. Long-term monitoring of entire communities and their associated microbiomes may provide insights into the adaptive and evolutionary potential of the microbiome in the presence and persistence of the recent wasting outbreaks.
Data availability statement
The datasets presented in this study can be found in online repositories. The names of the repository/repositories and accession number(s) can be found below: BioProject, PRJNA931596.
Author contributions
All authors contributed to and approved the manuscript. Conception and design: ML, MP. Sample Collection: ML, KH. Data Generation: ML. Data analysis AM, BCh, DM, BCa, MP. Visualization: AM, BCh, DM, BCa. Writing – original draft: AM, BCh. Writing – review & editing: AM, BCh, DM, BCa, MP. All authors contributed to the article and approved the submitted version.
Funding
This work was supported by the National Science Foundation grants to MP (IOS-1555058) and the Quantitative and Evolutionary STEM Traineeship supporting AM, BCh, DM, and BCa (QuEST; NRT-1735316; PI: MP) and a Vermont Space Grant Consortium and Vermont NASA EPSCoR award to MP.
Conflict of interest
The authors declare that the research was conducted in the absence of any commercial or financial relationships that could be construed as a potential conflict of interest.
Publisher’s note
All claims expressed in this article are solely those of the authors and do not necessarily represent those of their affiliated organizations, or those of the publisher, the editors and the reviewers. Any product that may be evaluated in this article, or claim that may be made by its manufacturer, is not guaranteed or endorsed by the publisher.
Supplementary material
The Supplementary Material for this article can be found online at: https://www.frontiersin.org/articles/10.3389/fmars.2023.1130912/full#supplementary-material
References
Aalto E. A., Lafferty K. D., Sokolow S. H., Grewelle R. E., Ben-Horin T., Boch C. A., et al. (2020). Models with environmental drivers offer a plausible mechanism for the rapid spread of infectious disease outbreaks in marine organisms. Sci. Rep. 10 (1), 5975. doi: 10.1038/s41598-020-62118-4
Aquino C. A., Besemer R. M., DeRito C. M., Kocian J., Porter I. R., Raimondi P. T., et al. (2020). Evidence that microorganisms at the animal-water interface drive Sea star wasting disease. Front. Microbiol. 11, 610009. doi: 10.3389/fmicb.2020.610009
Bartlow A. W., Manore C., Xu C., Kaufeld K. A., Del Valle S., Ziemann A., et al. (2019). Forecasting zoonotic infectious disease response to climate change: Mosquito vectors and a changing environment. Vet. Sci. 6, 40. doi: 10.3390/vetsci6020040
Bates A. E., Hilton B. J., Harley C. D. G. (2009). Effects of temperature, season and locality on wasting disease in the keystone predatory sea star pisaster ochraceus. Dis. Aquat. Organ. 86 (3), 245–251. doi: 10.3354/dao02125
Bauer M. P., Kuijper J. (2017). Clostridium difficile infections in hospitals and community [Internet]. Infect. Dis. 1, 351–354.e1. doi: 10.1016/b978-0-7020-6285-8.00040-x
Becker P. T., Egea E., Eeckhaut I. (2008). Characterization of the bacterial communities associated with the bald sea urchin disease of the echinoid paracentrotus lividus. J. Invertebr. Pathol. 98 (2), 136–147. doi: 10.1016/j.jip.2007.12.002
Becker P., Gillan D., Lanterbecq D., Jangoux M., Rasolofonirina R., Rakotovao J., et al. (2004). The skin ulceration disease in cultivated juveniles of holothuria scabra (Holothuroidea, Echinodermata). Aquaculture 242 (1), 13–30. doi: 10.1016/j.aquaculture.2003.11.018
Bokulich N. A., Kaehler B. D., Rideout J. R., Dillon M., Bolyen E., Knight R., et al. (2018). Optimizing taxonomic classification of marker-gene amplicon sequences with QIIME 2’s q2-feature-classifier plugin. Microbiome 6, 90. doi: 10.1186/s40168-018-0470-z
Bowman J. P. (2014). “The family colwelliaceae,” in The prokaryotes: Gammaproteobacteria. Eds. Rosenberg E., DeLong E. F., Lory S., Stackebrandt E., Thompson F. (Berlin, Heidelberg: Springer Berlin Heidelberg), 179–195.
Caballes C. F., Schupp P. J., Pratchett M. S., Rivera-Posada J. A. (2012). Interspecific transmission and recovery of TCBS-induced disease between acanthaster planci and linckia guildingi. Dis. Aquat. Organ. 100 (3), 263–267. doi: 10.3354/dao02480
Callahan B. J., McMurdie P. J., Rosen M. J., Han A. W., Johnson A. J. A., Holmes S. P. (2016). DADA2: High-resolution sample inference from illumina amplicon data. Nat. Methods 13 (7), 581–583. doi: 10.1038/nmeth.3869
Caspi R., Billington R., Fulcher C. A., Keseler I. M., Kothari A., Krummenacker M., et al. (2018). The MetaCyc database of metabolic pathways and enzymes. Nucleic Acids Res. 46, D633–D639. doi: 10.1093/nar/gkx935
Cobo-López S., Gupta V. K., Sung J., Guimerà R., Sales-Pardo M. (2022). Stochastic block models reveal a robust nested pattern in healthy human gut microbiomes. PNAS. Nexus. 1 (3), gac055. doi: 10.1093/pnasnexus/pgac055
Daszak P., Cunningham A. A., Hyatt A. D. (2001). Anthropogenic environmental change and the emergence of infectious diseases in wildlife. Acta Trop. 78 (2), 103–116. doi: 10.1016/S0001-706X(00)00179-0
Delroisse J., Van Wayneberghe K., Flammang P., Gillan D., Gerbaux P., Opina N., et al. (2020). Epidemiology of a SKin ulceration disease (SKUD) in the sea cucumber holothuria scabra with a review on the SKUDs in holothuroidea (Echinodermata). Sci. Rep. 10 (1), 22150. doi: 10.1038/s41598-020-78876-0
de O Santos E., Alves N., Dias G. M., Mazotto A. M., Vermelho A., Vora G. J., et al. (2011). Genomic and proteomic analyses of the coral pathogen vibrio coralliilyticus reveal a diverse virulence repertoire. ISME. J. 5 (9), 1471–1483. doi: 10.1038/ismej.2011.19
Dungan M. L., Miller T. E., Thomson D. A. (1982). Catastrophic decline of a top carnivore in the gulf of california rocky intertidal zone. Science 216 (4549), 989–991. doi: 10.1126/science.216.4549.989
Dunphy C. M., Gouhier T. C., Chu N. D., Vollmer S. V. (2019). Structure and stability of the coral microbiome in space and time. Sci. Rep. 9 (1), 6785.
Eckert G. L., Engle J. M., Kushner D. J. (2000). Sea Star disease and population declines at the channel islands. Proc. fifth. California. Islands. Symposium., 390–393.
Farmer J. J., Hickman-Brenner F. W. (2006). The genera vibrio and photobacterium. Prokaryotes. 1, 508–563. doi: 10.1007/0-387-30746-x_18
Fuess L. E., Eisenlord M. E., Closek C. J., Tracy A. M., Mauntz R., Gignoux-Wolfsohn S., et al. (2015). Up in arms: Immune and nervous system response to Sea star wasting disease. PloS One 10 (7), e0133053. doi: 10.1371/journal.pone.0133053
Harvell C. D., Montecino-Latorre D., Caldwell J. M., Burt J. M., Bosley K., Keller A., et al. (2019). Disease epidemic and a marine heat wave are associated with the continental-scale collapse of a pivotal predator (Pycnopodia helianthoides). Sci. Adv. 5 (1), eaau7042. doi: 10.1126/sciadv.aau7042
Hewson I., Bistolas K. S. I., Quijano Cardé E. M., Button J. B., Foster P. J., Flanzenbaum J. M., et al. (2018). Investigating the complex association between viral ecology, environment, and northeast pacific Sea star wasting. Front. Mar. Sci. 5. doi: 10.3389/fmars.2018.00077
Hewson I., Button J. B., Gudenkauf B. M., Miner B., Newton A. L., Gaydos J. K., et al. (2014). Densovirus associated with sea-star wasting disease and mass mortality. Proc. Natl. Acad. Sci. U. S. A. 111 (48), 17278–17283. doi: 10.1073/pnas.1416625111
Hira J., Stensvåg K. (2022). Evidence for association of vibrio echinoideorum with tissue necrosis on test of the green sea urchin strongylocentrotus droebachiensis. Sci. Rep. 12, 4859. doi: 10.1038/s41598-022-08772-2
Imhoff J. F. (2005). Vibrionales. Bergey’s Manual®® of Systematic Bacteriology (Boston, MA: Springer). doi: 10.1007/0-387-28022-7_11
Jackson E. W., Wilhelm R. C., Johnson M. R., Lutz H. L., Danforth I., Gaydos J. K., et al (2020). Diversity of sea star-associated densoviruses and transcribed endogenized viral elements of densovirus origin. doi: 10.1101/2020.08.05.239004
Jangoux M. (1986). Diseases of echinodermata. i. agents microorganisms and protistans. Dis. Aquat. Organisms. 2, 147–162. doi: 10.3354/dao002147
Jurtshuk P. Jr. (2011). “Bacterial metabolism,” in Medical microbiology. Ed. Baron S. (Galveston (TX: University of Texas Medical Branch at Galveston).
Kanehisa M., Sato Y., Kawashima M., Furumichi M., Tanabe M. (2016). KEGG as a reference resource for gene and protein annotation. Nucleic Acids Res. 44, D457–D462. doi: 10.1093/nar/gkv1070
Klindworth A., Pruesse E., Schweer T., Peplies J., Quast C., Horn M., et al. (2013). Evaluation of general 16S ribosomal RNA gene PCR primers for classical and next-generation sequencing-based diversity studies. Nucleic Acids Res. 41 (1), e1. doi: 10.1093/nar/gks808
Kohl W. T., McClure T. I., Miner B. G. (2016). Decreased temperature facilitates short-term Sea star wasting disease survival in the keystone intertidal Sea star pisaster ochraceus. PloS One 11 (4), e0153670. doi: 10.1371/journal.pone.0153670
Leray M., Wilkins L. G. E., Apprill A., Bik H. M., Clever F., Connolly S. R., et al. (2021). Natural experiments and long-term monitoring are critical to understand and predict marine host–microbe ecology and evolution. PloS Biol. 19 (8), e3001322. doi: 10.1371/journal.pbio.3001322
Lin H., Peddada S. D. (2020). Analysis of compositions of microbiomes with bias correction. Nat. Commun. 11, 3514. doi: 10.1038/s41467-020-17041-7
Lloyd M. M., Pespeni M. H. (2018). Microbiome shifts with onset and progression of Sea star wasting disease revealed through time course sampling. Sci. Rep. 8 (1), 16476. doi: 10.1038/s41598-018-34697-w
Lozupone C., Hamady M., Knight R. (2006). UniFrac – an online tool for comparing microbial community diversity in a phylogenetic context. BMC Bioinf. 7, 371. doi: 10.1186/1471-2105-7-371
McDonald D., Price M. N., Goodrich J., Nawrocki E. P., DeSantis T. Z., Probst A., et al. (2012). An improved greengenes taxonomy with explicit ranks for ecological and evolutionary analyses of bacteria and archaea. ISME. J. 6, 610–618. doi: 10.1038/ismej.2011.139
Mead A. D., Southwick J. M. K., Root H. T., Willard C. W., Morton W. M. P., Roberts A. D., et al. (1898). Twenty-Eighth Annual Report of the Commissioners of Inland Fisheries, Made to the General Assembly at Its January Session Southwick J. M. K., Root H. T., Willard C. W., Morton W. M. P., Roberts A. D., Bumpus H. C., et al (London: Forgotten Books).
Menge B. A. (1979). Coexistence between the seastars asterias vulgaris and a. forbesi in a heterogeneous environment: A non-equilibrium explanation. Oecologia 41, 245–272. doi: 10.1007/bf00377430
Menge B. A., Cerny-Chipman E. B., Johnson A., Sullivan J., Gravem S., Chan F. (2016). Sea Star wasting disease in the keystone predator pisaster ochraceus in Oregon: Insights into differential population impacts, recovery, predation rate, and temperature effects from long-term research. PloS One 11 (5), 4, e015399. doi: 10.1371/journal.pone.0153994
Neu A. T., Allen E. E., Roy K. (2021). Defining and quantifying the core microbiome: Challenges and prospects. Proc. Natl. Acad. Sci. 118, 51. doi: 10.1073/pnas.2104429118
Olsen I. (2014). The family fusobacteriaceae. Prokaryotes. 1, 109–132. doi: 10.1007/978-3-642-30120-9_213
Omazic A., Bylund H., Boqvist S., Högberg A., Björkman C., Tryland M., et al. (2019). Identifying climate-sensitive infectious diseases in animals and humans in northern regions. Acta Vet. Scand. 61, 53. doi: 10.1186/s13028-019-0490-0
Peixoto T. P. (2014). Hierarchical block structures and high-resolution model selection in large networks. Physical Review X. doi: 10.1103/PhysRevX.4.011047. Available at: https://journals.aps.org/prx/abstract/10.1103/PhysRevX.4.011047.
Peixoto T. P. (2017). Nonparametric Bayesian inference of the microcanonical stochastic block model. Phys. Rev. E. 95 (1–1), 012317. doi: 10.1103/PhysRevE.95.012317
Peixoto T. P. (2020). Merge-split Markov chain Monte Carlo for community detection. Phys. Rev. E. 102 (1–1), 012305. doi: 10.1103/PhysRevE.102.012305
Peixoto R. S., Harkins D. M., Nelson K. E. (2021). Advances in microbiome research for animal health. Annu. Rev. Anim. Biosci. 9, 289–311. doi: 10.1146/annurev-animal-091020-075907
Petersen C., Round J. L. (2014). Defining dysbiosis and its influence on host immunity and disease. Cell Microbiol. 16 (7), 1024–1033. doi: 10.1111/cmi.12308
Petton B., Destoumieux-Garzón D., Pernet F., Toulza E., de Lorgeril J., Degremont L., et al. (2021). The pacific oyster mortality syndrome, a polymicrobial and multifactorial disease: State of knowledge and future directions. Front. Immunol. 12, 630343. doi: 10.3389/fimmu.2021.630343
Price S. J., Leung W. T. M., Owen C. J., Puschendorf R., Sergeant C., Cunningham A. A., et al. (2019). Effects of historic and projected climate change on the range and impacts of an emerging wildlife disease. Glob. Chang. Biol. 25 (8), 2648–2660. doi: 10.1111/gcb.14651
Rivera-Posada J. A., Pratchett M., Cano-Gómez A., Arango-Gómez J. D., Owens L. (2011a). Injection of acanthaster planci with thiosulfate-citrate-bile-sucrose agar (TCBS). i. disease induction. Dis. Aquat. Organ. 97 (2), 85–94. doi: 10.3354/dao02401
Rivera-Posada J. A., Pratchett M., Owens L. (2011b). Injection of acanthaster planci with thiosulfate-citrate-bile-sucrose agar (TCBS). II. histopathological changes. Dis. Aquat. Organ. 97 (2), 95–102. doi: 10.3354/dao02400
Sato Y., Ling E. Y. S., Turaev D., Laffy P., Weynberg K. D., Rattei T., et al. (2017). Unraveling the microbial processes of black band disease in corals through integrated genomics. Sci. Rep. 7, 40455. doi: 10.1038/srep40455
Scheibling R. (1986). Increased macroalgal abundance following mass mortalities of sea urchins (Strongylocentrotus droebachiensis) along the Atlantic coast of Nova Scotia. Oecologia 68 (2), 186–198. doi: 10.1007/BF00384786
Scheibling R. E., Hennigar A. W. (1997). Recurrent outbreaks of disease in sea urchins strongylocentrotus droebachiensis in Nova scotia: Evidence for a link with large-scale meteorologic and oceanographic events. Mar. Ecol. Prog. Ser. 152, 155–165. doi: 10.3354/meps152155
Stackebrandt E. (2014). The family clostridiaceae, other genera. Prokaryotes. 1, 67–73. doi: 10.1007/978-3-642-30120-9_214
Staehli A., Schaerer R., Hoelzle K., Ribi G. (2009). Temperature induced disease in the starfish astropecten jonstoni. Mar. Biodivers. Records. 2, 78. doi: 10.1017/s1755267209000633
Tajima K., Hirano T., Shimizu M., Ezura Y. (1996). Isolation and pathogenicity of the causative bacterium of spotting disease of sea urchin strongylocentrotus intermedius. Fish. Sci. 63 (2), 249–252. doi: 10.1016/S0167-9309(07)80073-1
Tajima K., Machado R., Cunha da Silva J., Lawrence J. M. (2007). “Chapter 9 disease in sea urchins,” in Developments in aquaculture and fisheries science, vol. p . Ed. Lawrence J. M. (Elsevier), 167–182.
Team R. C. (2021). R: A language and environment for statistical computing (Vienna, Austria: R Foundation for Statistical Computing).
Tenenbaum D., Maintainer B. P. (2021). KEGGREST: Client-side REST access to the Kyoto encyclopedia of genes and genomes (KEGG) (R Package Version 1.38.0).
Urakawa H. (2014). The family moritellaceae. Prokaryotes. p, 477–489. doi: 10.1007/978-3-642-38922-1_227
Keywords: microbiome, dysbiosis, sea star wasting disease, wildlife disease, Bayesian network analysis
Citation: McCracken AR, Christensen BM, Munteanu D, Case BKM, Lloyd M, Herbert KP and Pespeni MH (2023) Microbial dysbiosis precedes signs of sea star wasting disease in wild populations of Pycnopodia helianthoides. Front. Mar. Sci. 10:1130912. doi: 10.3389/fmars.2023.1130912
Received: 06 January 2023; Accepted: 23 February 2023;
Published: 16 March 2023.
Edited by:
Christopher John Grim, United States Food and Drug Administration, United StatesReviewed by:
Diogo Antonio Tschoeke, Federal University of Rio de Janeiro, BrazilAmanda Shore, Farmingdale State College, United States
Copyright © 2023 McCracken, Christensen, Munteanu, Case, Lloyd, Herbert and Pespeni. This is an open-access article distributed under the terms of the Creative Commons Attribution License (CC BY). The use, distribution or reproduction in other forums is permitted, provided the original author(s) and the copyright owner(s) are credited and that the original publication in this journal is cited, in accordance with accepted academic practice. No use, distribution or reproduction is permitted which does not comply with these terms.
*Correspondence: Andrew R. McCracken, YW5kcmV3Lm1jY3JhY2tlbkB1dm0uZWR1; Melissa H. Pespeni, TWVsaXNzYS5QZXNwZW5pQHV2bS5lZHU=