- 1University of Bergen, Department of Biological Sciences, Bergen, Norway
- 2Institute of Marine Research, Tromsø, Norway
- 3Institute of Marine Research, Bergen, Norway
- 4Institute of Marine Research, Matre, Matredal, Norway
Precision feeding aims to provide the correct amount of feed to farmed animals for optimal growth and performance and to avoid feed waste. However, knowledge underlying the meal-to-meal variability in voluntary feed intake of farmed species is still limited. This study examined the relationship between meals, feed deprivation time and the feed (pellets) consumed by Atlantic salmon post smolts. The data was collected from individual fish handfed to satiety without social interaction in three independent short-term (6-12 days) experiments. The fixed variables of our model (feed deprivation time (i.e., time between meals), number of pellets provided, day, previous meal size, and fish growth) explained most of the feed intake (number of pellets ingested) (R2 0.68). Results show that fish ingested more pellets over the course of the trials as they grew, resulting in a positive correlation between feed intake and fish growth (final minus initial fish weight). The time between meals and prior meal size (the number of pellets ingested in the previous meal) significantly affected feed intake in the following meal. Our results suggest that it is possible to optimise meal size by considering the size of the previous meal and the time since it was given.
1 Introduction
Overfeeding, i.e., providing animals more feed than they consume, is a common practice in the aquaculture industry. The main reason is the concern of underfeeding fish, causing slow growth, low feed efficiency and increased stress levels and aggression (Cañon Jones et al., 2010; Martins et al., 2012). However, overfeeding results in release of uneaten feed into the environment, representing a significant source of pollution (Taranger et al., 2014; Ahmed et al., 2019). Furthermore, overfeeding wastes valuable resources and undermines the sustainability of the aquaculture industry. Because a substantial amount of the total production cost (40-50%) in salmon farming is related to feed (Asche & Oglend, 2016; Iversen et al., 2020) overfeeding also reduces profitability of aquaculture companies. Optimizing the feeding levels and thereby increasing feed efficiency and reducing nutrient waste has been a priority of the aquaculture industry, prompting the development of feeding support technologies such as echo sounders (Juell et al., 1993) and pellet detection-sensors to address this issue (Brijs et al., 2021). However, basic research and data collection is still needed to develop suitable models that can predict fish appetite and feed intake related to each meal based on a deep understanding of these physiological processes.
Feed intake is a complex process, driven by several external factors such as light, season, temperature, feed availability, feed composition, stress, predators, etc., and internal factors such as life stage, gut filling, stored energy, activity levels, etc. (Volkoff et al., 2010; Rønnestad et al., 2017). Therefore, it is very challenging to predict the best feed delivery rate and amount, which ideally correspond to the number of pellets that the fish can capture before they go to waste (Bailey & Alanärä, 2001). For farmed fish, the amount eaten during a meal (defined as “any of the regular occasions in a day when a reasonably large amount of feed is eaten” (Blundell et al., 1987)) depends on the feeding schedule combined with the appetite or motivation to eat at the time (Volkoff, 2016). Additionally, predictable feeding practices have been shown to have a positive impact on fish welfare compared to unpredictable feeding times (Cañon Jones et al., 2012). Normally, a meal may be defined by the amount of feed offered and its duration. Here, meal consumption is defined as number of eaten feed (pellets).
Defining the factors which determine meal initiation (process that leads the fish to start the meal) and meal termination (satiation; a process that leads the fish to stop the meal) are crucial in order to understand and predict feed intake (Chudtong and De Gaetano, 2021). Meal initiation is affected by appetite, defined as the desire to eat and/or hunger, that combines hedonistic signalling pathways with metabolic need to ingest feed to acquire the energy and nutrients necessary to satisfy the demands of homeostasis and growth (Berthoud, 2011; Soengas et al., 2018). On the other hand, meal termination may be mostly dictated by satiation, which is the feeling of fullness after ingesting feed. The next meal ingestion is then constrained by satiety, a multi-factorial and time related process that inhibits further feed intake and represents decline in hunger after the end of an eating episode.
For a better understanding of voluntary feed intake in Atlantic salmon, we have collected data from three short-term independent experiments, in which individual feed intake was monitored for 6 to 12 days. Two main hypotheses were generated: 1) the amount of feed consumed in the previous meal, interacts with time between meals (feed deprivation) to affect the amount of feed intake in the next meal and 2) the average feed intake rate increases with fish size. To minimise variability in stress and energy requirements outside routine metabolic demands, all analysis was conducted on individual fish without social interactions with conspecifics, excluding competition and aggression.
2 Material and methods
2.1 Experimental design
All experiments were done at the Institute of Marine Research, Matre (60° N, 5° E, Western Norway) which is authorized for animal experimentation (Norwegian Food Safety Authority, facility 110), and in compliance with the Norwegian Animal Research Authority regulations. Three experiments were conducted using a solitary tank-based system for analyses of individual feed intake. Each tank was 0.5 x 0.5 x 0.4 m and contained an 85 L flow-through water volume. Fish were reared with a light/dark cycle of 18:6 hours, water temperature 12°C and oxygen above 90%. Each tank was equipped with a waste feed collector and had a small window that allowed for visual observations of the fish while feeding without disturbing them (Supplementary Figure 1).
The fish were acclimated towards a solitary tank-based condition as follows (Figure 1): Atlantic salmon post smolts from the same broodstock (NLA strain, 0+) were measured for fork length and weight and were randomly distributed into the tanks (3 fish per tank) and were handfed until a regular feed intake in all fish was observed (7 days). The 3 individuals from each tank were lightly anesthetised with 12 mgL-1 Aqui-S (New Zealand), netted and the weight and fork length registered. One of the three individuals in each tank was selected for the study based on best performance (growth) during the 7-day acclimation period. The selected individual was carefully returned to the tank for the trial. All the selected individuals resumed feeding a few hours after they were returned to their tanks.
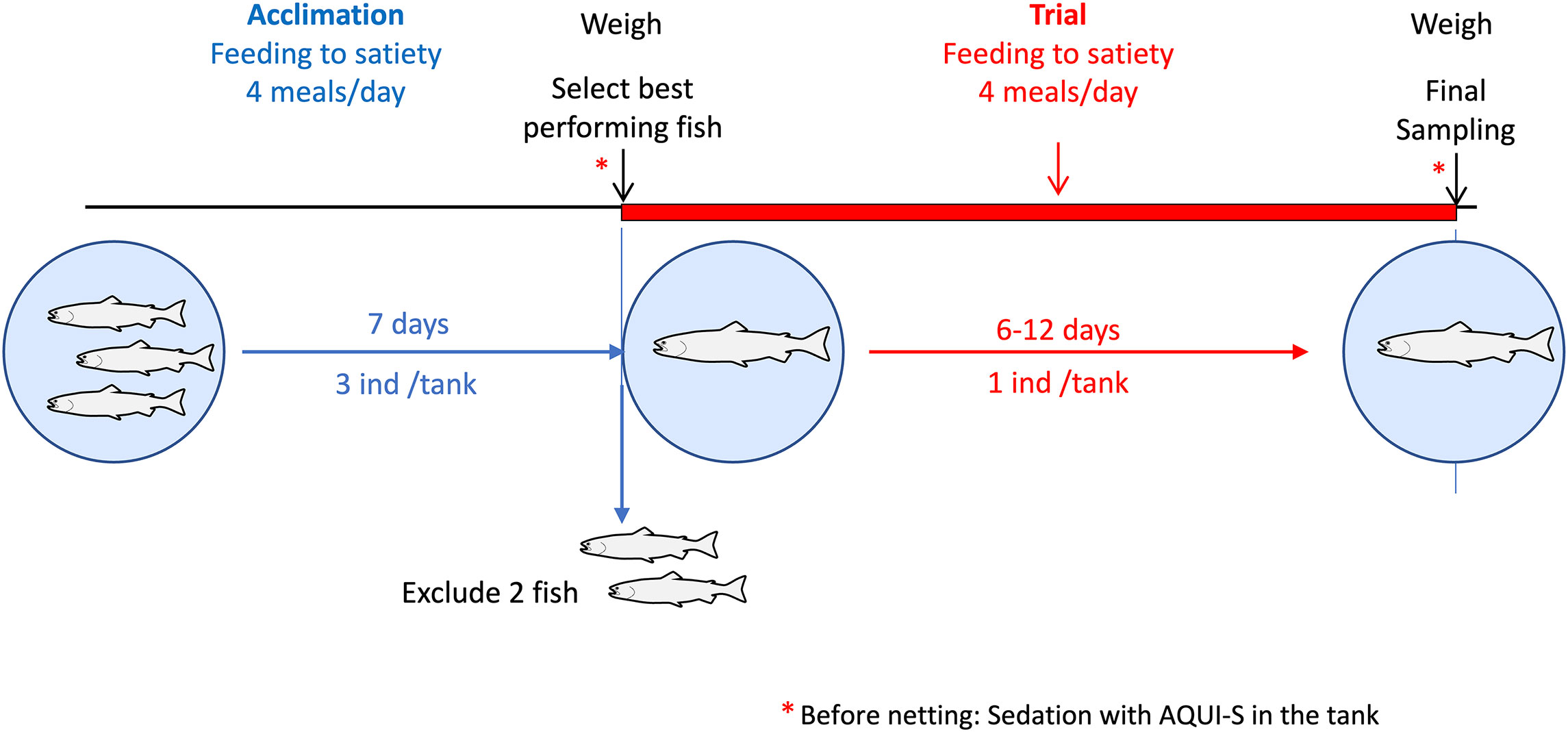
Figure 1 Experimental design of trials investigating individual feed intake in Atlantic salmon post smolts handfed to satiety. Fish (n=3 per tank) were acclimatized for 7 days. At the start of each trial fish weight was recorded and the best performing fish selected. Individual feed intake (pellets consumed per meal) was recorded for 6 (Trial 1), 12 (Trial 2) and 11 (Trial 3) days. Final fish weight was recorded at the end of each trial.
The fish were fed with a commercial standard diet (Nutra Parr LB 3 (3.0 mm), Skretting, Norway). The weight of 100 pellets was 1.96 g with 94% of dry matter. Feed was administered to satiation (assessed by visual observation and defined as the fish stopped ingesting pellets) four times a day: 09:00, 11:00, 13:00 and 15:00. The fish was deprived of feed in between meals. The same pellet size was used across experiments, since pellet size can affect Atlantic salmon growth (Wańkowski & Thorpe, 1979). Uneaten feed was collected from the tanks 10 min after the fish stopped feeding.
2.2 Data collection
Three separate trials with slight modifications in their design were run sequentially to create replicate data that allows for assess potential trial effects, and to create more contrast in the data. During the trials, fish were handfed in excess four times a day at the same time schedule (09:00, 11:00, 13:00 and 15:00), unless stated otherwise. No feed was given between 15:00 and 09:00, meaning they were deprived from feed for a period of 18 h every day. Feed intake was monitored for each individual for every meal. Fish weight was collected at the start and at the end of the experimental trials. Data was collected from three independent trials:
Trial 1: Atlantic salmon with an average weight 131 ± 13 g (n=14). Feed intake was monitored for 6 consecutive days.
Trial 2: Atlantic salmon with an average weight 192 ± 17 g (n=14). Feed intake was monitored for 12 consecutive days.
Trial 3: Atlantic salmon with an average weight 132 ± 13 g (n=15). Feed intake was monitored for 11 consecutive days. During the first 5 days, 4 meals were offered, while only one meal per day was provided on day 6 (at 13:00) and day 7 (at 11:00), which resulted in a feed deprivation of 22 h, followed by a period of 4 days where the fish were feed again 4 meals.
2.3 Statistical analysis
For each fish f at meal m in trial t, the total number of pellets consumed was calculated and modelled as a function of number of pellets consumed at the prior meal, number of pellets provided, day of the experiment, fish weight at the beginning of the experiment and feed deprivation time (hours between meals):
With R as the pellets consumed by fish f at meal m in trial t; b0,f ~ N(0,σI) and b1,t ~ N(0,σ2) as random intercepts for individual fish (to account for individual variation) and trial (to represent potential differences between the trials); an interaction between β1Fm,f,t as fixed effect of feed deprivation time (factor) and β2Cm-1,f,t as a first-order autocorrelation, i.e. the fixed effect of previous meal (continuous) on the current meal. This effect was added to test the assumption that observed meals are not independent, that is a meal of a fish is affected by what was previously consumed. Furthermore, the full model included β3Pm,f,t as fixed effect of pellets provided (continuous), β4Dm,f,t as fixed effect of day in trial (continuous), and β5Gf,t as fixed effect of fish growth (weight change between start and end of experiment, i.e., final fish weight minus initial fish weight; continuous). The interaction between feed deprivation time (2h and 18/22h) and previous meal was added to account for potential dependency of the effect of previous feed intake on the length of the feed deprivation period. The error distribution was assumed to be Poisson-distributed εm,f,t = Possion(λ) with log-link. All continuous fixed effects were normalized to facilitate model convergence and comparability of effect size.
The linear mixed effect model was fitted to data in R 4.2.1 (RCoreTeam, 2021) using glmmTMB (Brooks et al., 2017), and to validate the model fit, (simulated) residual distribution and model dispersion were evaluated using the DHARMa package (Hartig, 2021). A backward model selection was conducted based on Akaike information criterion with a correction for small sample sizes (AICc) (Hurvich & Tsai, 1991) to determine the most parsimonious model configuration. We calculated the reliability of observation clusters (i.e., meals observed per individual fish and per trial) and the variation explained by the clustering using the intra-class correlation coefficient (ICC).
3 Results
3.1 Effect of previous meal consumption and time of feed deprivation
Model selection based on AICc showed that the full model (1) explained the data most parsimoniously (Supplementary Table 1). After correcting for all other explanatory variables, the number of pellets consumed in the previous meal significantly (p<0.001) reduced the meal after longer periods of feed deprivation (≥18 h) (Figure 2A; Supplementary Table 2), although with a moderate effect size compared to the other covariates. Specifically, the model estimates that per 10 pellets consumed in the previous meal after a longer feed deprivation period (≥18 h), the consumed number of pellets decreases by 2.75%. No significant effect was detected after short feed deprivation periods, i.e., in 2 h in between meals, (Figure 2A; Supplementary Table 2). Longer feed deprivation time (18h/22h) had a strong, significant effect on pellets consumed (Figure 2B), as did the number of pellets provided in the current meal (Supplementary Figure 2; Supplementary Table 2).
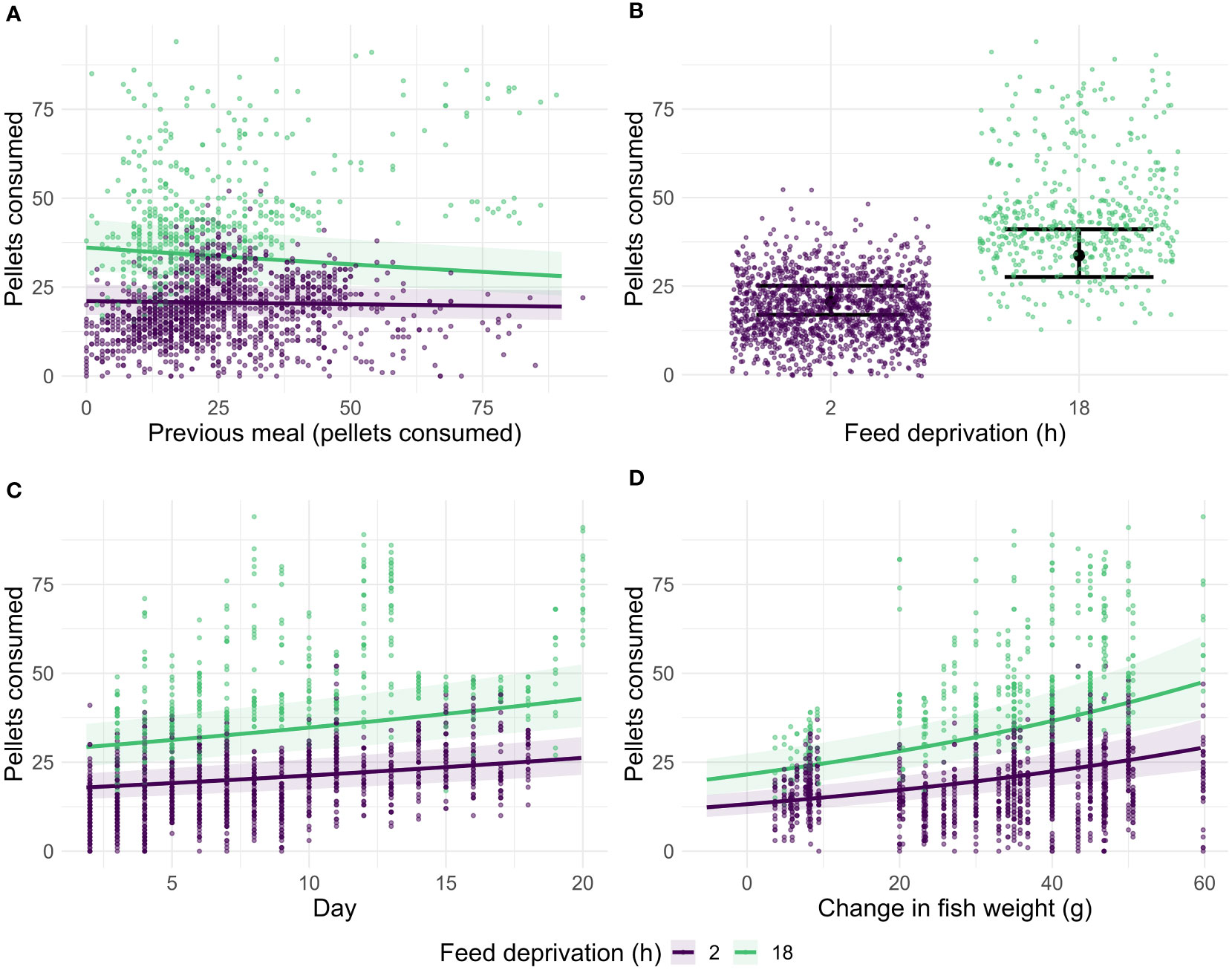
Figure 2 Number of pellets consumed in relation to pellets consumed in previous meal and the feed deprivation time, 2 or ≥18 h (A), number of pellets consumed in relation to feed deprivation time (B), number of pellets consumed in relation to the day of experiment and feed deprivation time (C), and number of pellets consumed in relation to the fish weight change (final minus initial weight) and feed deprivation time (D). Lines and shaded areas show effect and 95% confidence intervals of each variable predicted by a linear mixed effect model, dots represent measurements by fish, meal, and feed deprivation time (colours).
3.2 Effect of growth on meal consumption
Day of trial, i.e., the time progression within the experimental trial (Figure 2C) and growth of fish (Figure 2D) had a significant (p<0.001) positive effect on the consumption, reflecting higher feed intake with larger fish size and growth. There were significant differences between the trials (Supplementary Figure 3B), which can be explained by differences in the experimental setups, i.e., variation between duration of the trials and the body weight of the fish included, as shown by an ICC of 0.2. Only a small part of the variation in the data was explained by differences between individual fish (Supplementary Figure 3A), as shown by an ICC of only 0.09. In summary, our data was mainly explained by fixed effects, with an R2 of 0.68.
4 Discussion
Return of appetite in salmonid species has been found to be correlated with the duration of feed deprivation and emptying of the stomach (Brett, 1971). Our study confirmed this by demonstrating that the feed consumed by individual Atlantic salmon handfed to satiety was positively correlated with feed deprivation time, i.e., the longer the time between the meals, the higher number of pellets were ingested (Figure 2B). These results are interconnected with how much the fish ate in the previous meal: we show that feed intake is significantly reduced by the numbers of pellets consumed in the previous meal after a longer feed deprivation period (Figure 2A). For the shorter periods between meals (2 h feed restriction), the negative effect of previous meals was not observed, which suports the hypothesis that the gastric emptying (or stomach fullness) is related with the return of appetite (Brett, 1971). Previous studies have correlated the return of appetite with gastric filling and evacuation time (Grove et al., 1978), and it is estimated that 48 h are needed to completely evacuate the Atlantic salmon stomach content (Aas et al., 2017). Also should be taken into consideration that the time to evacuate the stomach is correlated with the size of the meal ingested (Gómez-Pearanda & Clavijo-Restrepo, 2012), as well as to the physical properties of the feed (Aas et al., 2021; Bogevik et al., 2021). In this context, the use of multiple meals a day, such as in this case study, can potentially affect the gastric evacuation time and delay the return of appetite. In addition, a gastric overload reduces absorption efficiency (Jobling, 1986). Nevertheless, it has been observed that different feeding regimes (feed offered in excess for 3 h per day, during the light period of the day only, and continuously) have no effect on salmon growth (Jørgensen & Jobling, 1992).
Larger fish generally will empty a meal of a given absolute size from the stomach at a faster rate than small fish (Flowerdew & Grove, 1979). This can also be deduced from the present data if we assume that gastric evacuation time, and thus filling is correlated with a higher feed intake. It has been hypothesised that the amount consumed in the second meal is equivalent to the amount of feed already transferred to midgut (stomach content evacuated) (Huebner & Langton, 1982). Additionally, the maximum stomach volume is proportional to fish weight, and thus the positive correlation found in the present study between feed consumption and fish growth is expected. However, the interrelationship of feed intake with appetite, hunger and satiety factors is complex (reviewed in (Volkoff, 2016; Rønnestad et al., 2017)), and one factor alone such as the stomach feed content is not enough to explain the return of appetite and consequently feed intake. A range of other factors, such as dietary composition (Forsman & Ruohonen, 2009), the status of energy homeostasis in the body where also the metabolic rate including the activity are important. Also, conditioning and hedonistic factors signalled through neural and hormonal pathways (Soengas et al., 2018) affect the motivation to eat. Additionally, many external factors, such as water temperature (Volkoff & Rønnestad, 2020; Navarro-Guillén et al., 2023), hypoxia (and its combined effect with temperature (Hevrøy et al., 2012)), photoperiod and stress play important roles in this complex process (reviewed in (Volkoff et al., 2010; Conde-Sieira et al., 2018; Soengas et al., 2018; Volkoff & Rønnestad, 2020). Feeding is an important factor for the welfare of farmed fish and understanding the physiological and behavioural mechanisms controlling appetite and feeding behaviour in fish can help predict the quantity of feed that will be ingested at each feeding opportunity.
An important basic premise concerns the timescale of the experimental trials. In the present work we have restricted ourselves to the dynamics of feed intake taking place over a few days. However, it would be of great interest to extend these analyses over several months and at different life stages. Nevertheless, our results validate that feeding should be dynamically adjusted to fish size (growth) and clearly also to the feed deprivation time and feed consumption in previous meals. Additionally, the fact that the fish were handfed in these trials adds additional uncertainty because of potential differences between the feeding staff, i.e., in the human behaviour (“patient” vs “rush” feeder). This random factor was not accounted for in the present analysis. However, this is also relevant to the current feeding practice in salmon culture pens, where individual feeders (with guidelines but also with their own personality) assess appetite via cameras and make their own decision of meal initiation, feeding intensity and meal termination. This work shows the importance of precision and prediction of rations for each meal and supports equipment and models for autonomous feeding systems in the salmon industry. Furthermore, our study assessed only the feed intake of individual fish, removing therefore the social interaction from the equation. It has been shown that social interaction can affects the dynamic of fish feeding (Kadri et al., 1996; Cañon Jones et al., 2010), particularly during feed restriction situations (Cañon Jones et al., 2017). Thus, it would be of interest to do a similar analyses in a fish group-based trial.
Our study demonstrated that length of feed deprivation and meal size have a clear impact on feed intake and growth. It also suggests that feed waste can be reduced by optimising feed intake of fish by allowing for gastric emptying, partial digestion, and absorption of previously ingested feed, thus avoiding overfeeding. Furthermore, our results quantify the link between growth and feed intake and provide statistical modelling parameters that can be used to explore further the basic mechanisms regulating voluntary feed intake of Atlantic salmon.
Data availability statement
The raw data supporting the conclusions of this article will be made available by the authors upon request, without undue reservation.
Ethics statement
The animal study was reviewed and approved by a local representative at the Institute of Marine Research in compliance with the Norwegian Animal Research Authority regulations.
Author contributions
The experimental trials were planned and conducted by MS, TN, EH, TH and IR. The statistical study was designed by AG, FZ and IR, while AG and FZ conducted the statistical analysis and produced Figure 2. All authors contributed to the article and approved the submitted version.
Funding
The research leading to these results was supported by Research Council of Norway (grants no. 199683/S40 to EH and 172548 and 317770 to IR) and by the European Community’s Seventh Framework Programme (FP7/2007-2013) under grant agreement n 222719 – LIFECYCLE (TH, TN, AG, IR).
Acknowledgments
We thank Dr Sergey Budaev for comments on the manuscript.
Conflict of interest
The authors declare that the research was conducted in the absence of any commercial or financial relationships that could be construed as a potential conflict of interest.
Publisher’s note
All claims expressed in this article are solely those of the authors and do not necessarily represent those of their affiliated organizations, or those of the publisher, the editors and the reviewers. Any product that may be evaluated in this article, or claim that may be made by its manufacturer, is not guaranteed or endorsed by the publisher.
Supplementary material
The Supplementary Material for this article can be found online at: https://www.frontiersin.org/articles/10.3389/fmars.2023.1127519/full#supplementary-material
References
Aas T. S., Sixten H. J., Hillestad M., Sveier H., Ytrestøyl T., Hatlen B., et al. (2017). Measurement of gastrointestinal passage rate in Atlantic salmon (Salmo salar) fed dry or soaked feed. Aquac. Rep. 8, 49–57. doi: 10.1016/j.aqrep.2017.10.001
Aas T. S., Ytrestøyl T., Sixten H. J., Hillestad M., Sveier H., Åsgård T. (2021). Physical feed properties affect gastrointestinal passage rate in Atlantic salmon, Salmo salar. Aquac. Nutr. 27 (2), 386–394. doi: 10.1111/anu.13191
Ahmed N., Thompson S., Glaser M. (2019). Global aquaculture productivity, environmental sustainability, and climate change adaptability. Environ. Manage. 63 (2), 159–172. doi: 10.1007/s00267-018-1117-3
Asche F., Oglend A. (2016). The relationship between input-factor and output prices in commodity industries: The case of Norwegian salmon aquaculture. J. Commodity Markets 1 (1), 35–47. doi: 10.1016/j.jcomm.2015.11.001
Bailey J., Alanärä A. (2001). A test of a feed budget model for rainbow trout, Oncorhynchus mykiss (Walbaum). Aquaculture Research 32 (6), 465–469. doi: 10.1046/j.1365-2109.2001.00588.x
Berthoud H. R. (2011). Metabolic and hedonic drives in the neural control of appetite: who is the boss? Curr. Opin. Neurobiol. 21 (6), 888–896. doi: 10.1016/j.conb.2011.09.004
Blundell J. E., Rogers P. J., Hill A. J. (1987). “Evaluating the satiating power of foods: implications for acceptance and consumption,” in Food acceptance and nutrition. Eds. J Colms D. B., Pangborn R. M., Raunhardt O. (London: Academic Press), 205–219.
Bogevik A. S., Samuelsen T. A., Aspevik T., Romarheim O. H., Aas T. S., Kalananthan T., et al. (2021). Disintegration stability of extruded fish feed affects gastric functions in Atlantic salmon (Salmo salar). Aquaculture 543, 737006. doi: 10.1016/j.aquaculture.2021.737006
Brett J. R. (1971). Satiation time, appetite, and maximum food intake of sockeye salmon (Oncorhynchus nerka). Journal of the Fisheries Board of Canada 28 (3), 409–415. doi: 10.1139/f71-053
Brijs J., Føre M., Gräns A., Clark T., Axelsson M., Johansen J. (2021). Bio-sensing technologies in aquaculture: how remote monitoring can bring us closer to our farm animals. Philos. Trans. R. Soc. B. 376 (1830), 20200218. doi: 10.1098/rstb.2020.0218
Brooks M., Kristensen K., van Benthem K., Magnusson A., Berg C. W., Nielsen A., et al. (2017). glmmTMB balances speed and flexibility among packages for zero-inflated generalized linear mixed modeling. R. J. 9, 378–400. doi: 10.32614/RJ-2017-066
Cañon Jones H. A., Hansen L. A., Noble C., Damsgård B., Broom D. M., Pearce G. P. (2010). Social network analysis of behavioural interactions influencing fin damage development in Atlantic salmon (Salmo salar) during feed-restriction. Appl. Anim. Behav. Sci. 127 (3), 139–151. doi: 10.1016/j.applanim.2010.09.004
Cañon Jones H. A., Noble C., Damsgård B., Pearce G. P. (2012). Investigating the influence of predictable and unpredictable feed delivery schedules upon the behaviour and welfare of Atlantic salmon parr (Salmo salar) using social network analysis and fin damage. Appl. Anim. Behav. Sci. 138 (1), 132–140. doi: 10.1016/j.applanim.2012.01.019
Cañon Jones H. A., Noble C., Damsgård B., Pearce G. P. (2017). Evaluating the effects of a short-term feed restriction period on the behavior and welfare of Atlantic salmon, Salmo salar, parr using social network analysis and fin damage. J. World Aquac. Soc. 48 (1), 35–45. doi: 10.1111/jwas.12322
Chudtong M., De Gaetano A. (2021). A mathematical model of food intake. Math Biosci Eng. 18 (2), 1238–1279. doi: 10.3934/mbe.2021067
Conde-Sieira M., Chivite M., Míguez J. M., Soengas J. L. (2018). Stress effects on the mechanisms regulating appetite in teleost fish. Front. Endocrinol. 9 (631). doi: 10.3389/fendo.2018.00631
Flowerdew M. W., Grove D. J. (1979). Some observations of the effects of body weight, temperature, meal size and quality on gastric emptying time in the turbot, Scophthalmus maximus (L.) using radiography. Journal of Fish Biology 14 (3), 229–238. doi: 10.1111/j.1095-8649.1979.tb03514.x
Forsman A., Ruohonen K. (2009). Dynamics of protein and lipid intake regulation of rainbow trout studied with a wide lipid range of encapsulated diets and self-feeders. Physiol. Behav. 96 (1), 85–90. doi: 10.1016/j.physbeh.2008.08.018
Gómez-Pearanda A., Clavijo-Restrepo L. C. (2012). Determination of the stomach emptying time of tilapia Oreochromis sp. using different weekly feeding frequencies and starvation. Acta Agronómica 61 (3), 239–234.
Grove D. J., Loizides L. G., Nott J. (1978). Satiation amount, frequency of feeding and gastric emptying rate in Salmo gairdneri. J. Fish Biol. 12 (5), 507–516. doi: 10.1111/j.1095-8649.1978.tb04195.x
Hartig F. (2021). DHARMa: Residual diagnostics for hierarchical (Multi-Level/Mixed) regression models. R package version 0.4.6. Available at: http://florianhartig.github.io/DHARMa/
Hevrøy E. M., Waagbø R., Torstensen B. E., Takle H., Stubhaug I., Jørgensen S. M., et al. (2012). Ghrelin is involved in voluntary anorexia in Atlantic salmon raised at elevated sea temperatures. Gen. Comp. Endocrinol. 175 (1), 118–134. doi: 10.1016/j.ygcen.2011.10.007
Huebner J. D., Langton R. W. (1982). Rate of gastric evacuation for winter flounder, Pseudopleuronectes americanus. Canadian Journal of Fisheries and Aquatic Science 39 (2), 356–360. doi: 10.1139/f82-049
Hurvich C. M., Tsai C.-L. (1991). Bias of the corrected AIC criterion for underfitted regression and time series models. Biometrika 78, 499–509. doi: 10.1093/biomet/78.3.499
Iversen A., Asche F., Hermansen Ø., Nystøyl R. (2020). Production cost and competitiveness in major salmon farming countries 2003–2018. Aquaculture 522, 735089. doi: 10.1016/j.aquaculture.2020.735089
Jørgensen E. H., Jobling M. (1992). Feeding behaviour and effect of feeding regime on growth of Atlantic salmon, Salmo salar. Aquaculture 101 (1), 135–146. doi: 10.1016/0044-8486(92)90238-G
Jobling M. (1986). Gastrointestinal overload — a problem with formulated feeds? Aquaculture 51 (3), 257–263. doi: 10.1016/0044-8486(86)90317-0
Juell J. E., Furevik D. M., Bjordal Å. (1993). Demand feeding in salmon farming by hydroacoustic food detection. Aquac. Eng. 12 (3), 155–167. doi: 10.1016/0144-8609(93)90008-Y
Kadri S., Huntingford F. A., Metcalfe N. B., Thorpe J. E. (1996). Social interactions and the distribution of food among one-sea-winter Atlantic salmon (Salmo salar) in a sea-cage. Aquaculture 139 (1), 1–10. doi: 10.1016/0044-8486(95)01163-3
Martins C. I. M., Galhardo L., Noble C., Damsgård B., Spedicato M. T., Zupa W., et al. (2012). Behavioural indicators of welfare in farmed fish. Fish Physiol. Biochem. 38 (1), 17–41. doi: 10.1007/s10695-011-9518-8
Navarro-Guillén C., Gilannejad N., Pérez-Hilario D., Martínez-Rodríguez G., Yúfera M. (2023). Gut transit of daily consecutive meals in greater amberjack juveniles reared at different temperatures. Aquaculture 567, 739244. doi: 10.1016/j.aquaculture.2023.739244
Rønnestad I., Gomes A. S., Murashita K., Angotzi R., Jönsson E., Volkoff H. (2017). Appetite-controlling endocrine systems in teleosts. Front. Endocrinol. 8, 73–73. doi: 10.3389/fendo.2017.00073
RCoreTeam (2021). R: A language and environment for statistical computing (Vienna: R Foundation for Statistical Computing).
Soengas J. L., Cerdá-Reverter J. M., Delgado M. J. (2018). Central regulation of food intake in fish: an evolutionary perspective. J. Mol. Endocrinol 60 (4), R171–R199. doi: 10.1530/JME-17-0320
Taranger G. L., Karlsen Ø., Bannister R. J., Glover K. A., Husa V., Karlsbakk E., et al. (2014). Risk assessment of the environmental impact of Norwegian Atlantic salmon farming. ICES J. Mar. Sci. 72 (3), 997–1021. doi: 10.1093/icesjms/fsu132
Volkoff H. (2016). The neuroendocrine regulation of food intake in fish: A review of current knowledge. Front. Neurosci. 10 (540). doi: 10.3389/fnins.2016.00540
Volkoff H., Hoskins L. J., Tuziak S. M. (2010). Influence of intrinsic signals and environmental cues on the endocrine control of feeding in fish: Potential application in aquaculture. Gen Comp Endocrinol. 167 (3), 352–359. doi: 10.1016/j.ygcen.2009.09.001
Volkoff H., Rønnestad I. (2020). Effects of temperature on feeding and digestive processes in fish. Temp. (Austin) 7 (4), 307–320. doi: 10.1080/23328940.2020.1765950
Keywords: meal size, consumption, feed deprivation, growth, aquaculture, precision feeding, salmon, feed intake
Citation: Gomes AS, Zimmermann F, Hevrøy EM, Søyland MAL, Hansen TJ, Nilsen TO and Rønnestad I (2023) Statistical modelling of voluntary feed intake in individual Atlantic salmon (Salmo salar L.). Front. Mar. Sci. 10:1127519. doi: 10.3389/fmars.2023.1127519
Received: 19 December 2022; Accepted: 06 February 2023;
Published: 16 February 2023.
Edited by:
Enric Gisbert, Institute of Agrifood Research and Technology (IRTA), SpainReviewed by:
Juan Antonio Martos-Sitcha, University of Cádiz, SpainBrett Glencross, International Fishmeal and Oil Manufacturers Association, United Kingdom
Xu-Fang Liang, Huazhong Agricultural University, China
Copyright © 2023 Gomes, Zimmermann, Hevrøy, Søyland, Hansen, Nilsen and Rønnestad. This is an open-access article distributed under the terms of the Creative Commons Attribution License (CC BY). The use, distribution or reproduction in other forums is permitted, provided the original author(s) and the copyright owner(s) are credited and that the original publication in this journal is cited, in accordance with accepted academic practice. No use, distribution or reproduction is permitted which does not comply with these terms.
*Correspondence: Ivar Rønnestad, SXZhci5Sb25uZXN0YWRAdWliLm5v
†Present addresses: Ernst M. Hevrøy, Research and Development Department, MOWI Feed AS, Bergen, Norway
Marcus A. L. Søyland, Skretting AS., Stavanger, Norway