- 1Centre for Ecological Genomics and Wildlife Research, Department of Zoology, University of Johannesburg, Auckland Park, South Africa
- 2National Research Foundation—South African Institute for Aquatic Biodiversity, Makhanda, South Africa
Understanding the dietary preferences of endangered species can be useful in implementing conservation strategies, including habitat restoration, translocation, and captive breeding. Environmental DNA (eDNA) from feces provides a non-invasive method for analysing animal diets. Currently, metabarcoding, a PCR-based approach, is the method of choice for analysing such data. However, this method has limitations, specifically PCR bias, which can result in the overestimation of the importance of certain taxa and failure to detect other taxa because they do not amplify. The present study compared metabarcoding with metagenomics, a PCR-free method, to assess the diversity of prey items in the feces of a critically endangered South African estuarine pipefish, Syngnathus watermeyeri, and its widely distributed congener S. temminckii to investigate potential dietary competition. The metabarcoding results showed a distinct difference between the diets of S. watermeyeri and S. temminckii, with the former mainly consuming calanoid copepods and the latter preferring caridean shrimp. In each case, a single species dominated the sequences generated by metabarcoding. Metagenomics produced more species identifications, and although the same trend was found regarding the preference of S. watermeyeri for copepods and that of S. temminckii for shrimp, this approach identified additional, albeit yet unidentified, copepod species as being important in the diet of S. watermeyeri. We conclude that the lower number of species identified using metabarcoding was most likely a result of amplification bias, resulting in key copepod species missing from the dietary analysis. These findings suggest that metagenomics is not only a useful complementary method for molecular dietary analysis, but may in some cases outperform metabarcoding. However, metagenomics is even more strongly affected by the lack of reference sequences than is metabarcoding, as the majority of sequences originate from genomic regions that have not yet been sequenced for the putative prey species in question.
Introduction
Estuaries are amongst the most threatened aquatic habitats in the world, many of which have become functionally degraded due to anthropogenic pressures (Edgar et al., 2000; Turpie et al., 2002; Kaselowski and Adams, 2013; Kajee et al., 2018). These include water abstraction for agricultural and industrial activities, pollution, and urban development (Orth et al., 2006). As such, some storage reservoirs in water-scarce countries such as South Africa now have the potential to retain more than 50% of the freshwater that the estuaries would receive under normal conditions (Wooldridge and Callahan, 2000). Several endemic estuarine species in South Africa are threatened, including the Endangered Knysna seahorse, Hippocampus capensis (Lockyear et al., 2006; Mkare et al., 2017), the Critically Endangered limpet Siphonaria compressa (Allanson and Herbert, 2005) and the Critically Endangered estuarine pipefish, Syngnathus watermeyeri (Whitfield, 1995). All three species are associated with submerged macrophyte beds mainly dominated by the eelgrass Zostera capensis, which is itself listed as vulnerable by the IUCN because it is sensitive to the current level of anthropogenic pressure and experiences widespread degradation as a result of increased coastal development (Payne et al., 1998; Adams, 2016). With declines in their natural habitat, ecosystem restoration, translocation, and captive breeding need to be considered as a means to conserve the remaining populations of endangered species (Strum, 2005; Gumm et al., 2011; Landa et al., 2017). As such, a thorough knowledge of what these species consume in the wild is required to provide ecosystem managers with the information necessary to manage these populations better.
Reconstruction of diet in wild populations is critical in ecology because it reveals important details about a species’ feeding habits (Pompanon et al., 2012), how a species uses its surroundings, and if there is resource competition with other members of the same community (Klare et al., 2011; Mumma et al., 2016). Animal diets have traditionally been determined by morphological examination of the gastrointestinal and fecal contents or by direct observation of their feeding habits (Pompanon et al., 2012; Sousa et al., 2019; Harper et al., 2020). However, the hard-part remains of some prey items can be difficult to identify using morphological analysis since they are usually damaged beyond recognition (Hawlitschek et al., 2018; Harper et al., 2020). In addition, studying the diet of endangered species can be challenging, particularly when the species of interest is rare and elusive, making field studies especially difficult (Ang et al., 2010).
A recently developed alternative method of assessing animal diets is eDNA metabarcoding (Kartzinel et al., 2015; Srivathsan et al., 2015; Emami-Khoyi et al., 2016; Boukhdoud et al., 2021). This PCR-based approach amplifies short DNA fragments of specified genetic markers that can then be identified using known reference sequences (Shehzad et al., 2012). However, metabarcoding has shortcomings, including PCR bias (Ferravante et al., 2021); this may occur due to irregular primer binding, thus resulting in some species amplifying less readily than others, or not at all (Alberdi et al., 2018; Mata et al., 2019).
Metagenomics is an alternative approach that involves the direct random sequencing of the entire genomic DNA rather than a small number of genetic markers (Bohmann et al., 2014; Bovo et al., 2018; Piñol, 2021). One of its drawbacks is that the bulk of the DNA that has been sequenced cannot be reliably assigned taxonomic rank due to a lack of comprehensive reference sequences since, for most species, only small portions of the genome have so far been sequenced (Bovo et al., 2018; Piñol, 2021).
Here, we compared the use of metagenomics and metabarcoding in identifying the prey species found in the feces of the estuarine pipefish and compared the prey items identified with corresponding data from its sister species, the longsnout pipefish S. temminckii, which is more abundant and widely distributed. Since the two pipefish share the same habitats and both capture small prey items by expanding their buccal cavity and suctioning prey through their tubular snouts (van Wassenbergh et al., 2008), it was hypothesised that dietary competition might exist between the two (Whitfield et al., 2017).
Materials and methods
Study sites and sample collection
Collection of Syngnathus watermeyeri and S. temminckii was approved by the Department of Agriculture, Forestry and Fisheries of South Africa (permit: RES2018/107), and ethical clearance was granted by the SAIAB Animal Ethics Committee (reference number: 25/4/1/5_2018-07) and the University of Johannesburg Faculty of Science Ethics Committee (reference number: 2021-10-05/Serite_Teske). Samples were collected from the only two South African estuaries where the estuarine pipefish still occurs, the Bushmans and Kariega (Claassens et al., 2022; Weiss et al., 2022). Pipefishes were collected at three sites; two in the Bushmans Estuary (site 1: 33°40’43.9 “S 26°39’12.2 "E; site 2: 33°40'21.6"S 26°38'46.1"E; data from these sites was subsequently pooled) and the third site in the Kariega Estuary (33°39'10.4"S 26°39'04.6"E). Both estuaries are permanently open, freshwater deprived, and have extensive macrophyte beds that constitute ideal habitat for pipefishes (Grange et al., 2000; Whitfield et al., 2017).
Sampling was conducted between March 30th and April 6th, 2019. In each location, all the pipefishes that could be collected within a period of 2 hours using a 5 mm stretch mesh seine net were placed into 5ℓ plastic tanks containing estuarine water for 3 h, after which they were released back into the estuaries. A total of 13 S. watermeyeri and 29 S. temminckii specimens were collected and kept in tanks in small groups of 2-3 individuals per species. The tanks were kept in the shade and aerated using portable air pumps, and the water was replaced every 30 min. Fecal pellets were dropped by the pipefishes in all these tanks throughout the 3 h period and were immediately collected using a sterile Pasteur pipette for each species, and subsequently blotted dry by placing them on paper towels before preserving them into 2 ml screw-cap microcentrifuge tubes containing RNAlater stabilization and storage reagent (QIAGEN GmbH, Hilden, Germany). Fecal pellets from all the individuals of a particular species and estuary were pooled. The tubes were kept frozen for up to two days and then stored at -70°C upon returning to the laboratory.
To control for the presence of any remaining DNA present in the estuarine water after blotting dry the fecal pellets, we collected 5 liters of surface water per estuary adjacent to the seagrass beds, using sterile plastic bottles. The water was filtered through two 100 ml Pall MicroFunnelTM filter funnels with 0.2 mm Supor® membrane per estuary, using a vacuum pump. Filtering was performed at a location where no previous DNA extractions or PCR reactions had ever been conducted, following the cleaning of all surfaces, the vacuum manifold, and all tubes with 1% bleach solution. The filters were then kept frozen until further processing.
Laboratory analysis
Prior to DNA extraction, the fecal pellets were thawed at room temperature and then transferred to new 1.5 ml microcentrifuge tubes, which were placed on a heat block for 2 h at 37°C. To ensure that ample genomic DNA was extracted from the samples, DNA extraction was done in triplicate for each fecal sample using three different extraction protocols: the CTAB procedure (Doyle, 1991), as well as two extraction kit methods, NucleoSpin and Qiagen, following manufacturers' instructions. The quality of the extractions was compared by assessing them on a NanoDrop 2000c spectrophotometer, and by running them on a 1% agarose gel containing GelRed nucleic acid gel stain. As no differences in sample quality were evident for the three extraction methods, the extractions for each fecal sample were pooled. Metabarcoding was performed at AIT Austrian Centre of Technology in a laboratory that complies with the requirements of standard ISO 9001:2015. All workbenches and equipment were cleaned with bleach, the PCR setup was performed in a physically separated laboratory and within a HEPA-filtered laminar flow chamber, and no-template controls (NTCs) were incorporated into the workflow. The mitochondrial cytochrome oxidase c subunit I (COI) gene was amplified using forward primer mlCOIintF and reverse primer jgHCO2198 (Leray et al., 2013) as described in Ntuli et al. (2020). The COI gene used for metabarcoding is a genetic marker commonly used in the sequencing of animal DNA (Hebert et al., 2003), and this marker has also been proven to be suitable for zooplankton DNA barcoding (Clarke et al., 2017). The primer combination used here has amplified more prey species from fecal DNA than any other primer combination (Leray et al., 2013), and has subsequently been used in numerous similar studies (Morrill et al., 2021; Tran et al., 2022). The PCR products were purified using the AMPure XP system (Beckman Coulter), and a NEBNext Ultra DNA Library Prep Kit (New England BioLabs, United States) was used for the preparation of genomic libraries. The resulting libraries were screened for size distribution using a 2100 Bioanalyzer (Agilent) and quantified using real-time PCR. The libraries were then sequenced on an Illumina HiSeq 4000 platform (Illumina Inc., San Diego, California, United States) at Novogene (Hong Kong), using 2×250 bp paired-end chemistry according to the manufacturer's instructions.
Metagenomic sequencing was performed at Novogene Europe, using 0.4 µg of genomic DNA for library preparations. The libraries were generated using a NEBNext DNA Library Prep Kit (New England BioLabs, United States), and indices were then added to each sample. The genomic DNA was randomly sheared into fragments of 350 bp. The fragments were end-polished, A-tailed, and ligated using the NEBNext adapter for Illumina sequencing, and the fragments were PCR enriched by P5 and indexed P7 oligos. The PCR products were purified using the AMPure XP system (Beckman Coulter), and the resulting libraries were screened for size distribution using a 2100 Bioanalyzer (Agilent) and quantified using real-time PCR. Genomic libraries were sequenced on an Illumina Novaseq6000 platform (Illumina Inc., San Diego, California, United States) at Novogene (Hong Kong), using 2 × 150 bp paired-end chemistry according to the manufacturer's instructions.
Sequence assembly and analysis
For the metabarcoding, quality control was carried out using FastQC (http://www.bioinformatics.bbsrc.ac.uk/projects/fastqc/) and sequencing adapters, all sequences with length less than 150 bp, and low-quality sequences, which were defined as those sequences with a quality Phred Score of less than 25 in a five bp sliding window, were removed using Trimmomatic v0.36 (Bolger et al., 2014). Cutadapt v4.1 (Martin, 2011) was then used to trim both forward and reverse amplification primer sequences. When only the forward or reverse read of a particular sequence passed the quality filtering step, the expected error rate for “unpaired forward” and “unpaired reverse” was estimated in VSEARCH v2.17.0 (Rognes et al., 2016), and since the forward sequences consistently showed a lower error rate compared to reverse sequences, only a subset of full length (250 bp) forward sequences were selected for downstream analysis, together with sequences produced by merging forward and reverse reads.
Metabarcoding sequences were merged using VSEARCH v2.17.0 pipeline. Briefly, all pair-end sequences were merged based on their overlaps. Chimeric amplicons were removed using a denovo method implemented in the same package, and all non-chimeric sequences with a minimum of 98% similarity were clustered into distinct groups, also known as operational taxonomic units (or OTUs). The consensus sequence for each cluster and the number of sequences that formed each cluster were extracted for the taxonomic rank assignment step.
The metagenomic sequences from each location were separately assembled into longer contigs using MEGAHIT v1.1.1 (Li et al., 2015) by selecting the "meta-large" preset, which is most appropriate for complex metagenomic assemblies (https://github.com/voutcn/megahit). When possible, assembled sequences were dereplicated using VMATCH (Kurtz, 2003), and the quality of the assemblies was assessed with QUAST v4.6.3 (Gurevich et al., 2013). To estimate the number of sequences that were assembled to form each contig, the raw sequences from each sample were mapped against the metagenomic assemblies using Bowtie2 v.2.5 (Langmead et al., 2021), and the number of mapped sequences for each contig was quantified using Samtools v.1.9 (Li et al., 2009).
Taxonomic rank assignment
To assign a taxonomic rank to consensus sequences, metabarcoding sequences were blast-searched (Altschul et al., 1990) against a local non-redundant COI database, using a minimum similarity score of 95% , a minimum query coverage of 150 bp, and an e-value of 10−5. Assembled contigs from metagenomics were then blast-searched against the complete NCBI nucleotide database ftp://ftp.ncbi.nlm.nih.gov/blast/db/nt, using the same parameters that were used for metabarcoding.
For both metabarcoding and metagenomics a consensus taxonomic rank was assigned to each sequence based on the Last Common Ancestor (LCA) of the five best matches, using BASTA (Kahlke and Ralph, 2019). In cases where no five matches exist that satisfy the requirements of 95% similarity and minimum query coverage of 150 bp, only the best matches were reported, as far as the percentage identify was not below 90% and the coverage was no less than 100 bp. Excel was then used to remove non-target taxa from the dataset (i.e., contaminants, pipefish DNA, and DNA from taxa that are too large or small to constitute prey, including mammals, bacteria, and algae). Subsets of the OTUs were then created based on those that contributed more than 1% to the overall read counts. The OTU counts for each putative species were agglomerated into the taxonomic rank of family, and visualised in Microsoft Excel with some additional annotations in Inkscape (https://inkscape.org/). A list of the potential prey items identified by metabarcoding and metagenomics was compiled. Information about the presence or absence of the identified species in South African estuaries was checked using the Global Biodiversity Information Facility (GBIF) database (https://www.gbif.org) and the World Register of Marine Organisms (WoRMS, https://marinespecies.org). In cases where the species does not occur in this region, we suggested which local species may be represented by the sequences in question based on taxonomic information. As estuaries have low diversity because few species can tolerate fluctuations in environmental conditions (Gray et al., 1997), the number of candidate species representing a particular genus or family tends to be low.
Results
The metabarcoding sequencing run generated 6 442 764 sequences from the Bushmans Estuary and 8 706 470 sequences from the Kariega Estuary for S. watermeyeri. For S. temminckii, 7 803 100 sequences and 8 540 218 sequences were recovered for the Bushmans Estuary and the Kariega Estuary, respectively. Post-quality filtering, the number of paired-end sequences kept per fecal sample ranged from 1 001 147 to 1 911 402. For the control samples, 538 422 and 93 920 sequences were generated for the Bushmans and Kariega estuaries, respectively. Of these, 32 197, and 351 were kept post-filtering (Supplementary Table 1).
Of the metabarcoding samples, the control samples from the Bushmans Estuary contained the highest numbers of unknowns (86.7%), followed by the controls from the Kariega Estuary, where 68.6% of the taxonomic assignments were unknown. The fecal samples of S. temminckii from the Kariega sample had 80 892 taxonomic assignments, and the Bushmans fecal sample had 153 925 taxonomic assignments, of which 12.5% and 48.5% of all consensus taxonomy rank assignments were classified as unknown, and therefore only the best matches were reported. The S. watermeyeri fecal samples had 26 964 and 266 754 taxonomic assignments for the Kariega and Bushmans estuaries, respectively, with 61.5% and 51.6% being reported as unknown based on the five best matches, and only best matches were reported (Supplementary Table 2).
The metagenomic assemblies comprised 611 473 (N50 = 707) and 183 631 (N50 = 2 952) singleton contigs for the S. watermeyeri samples from the Bushmans and Kariega estuaries, respectively. For S. temminckii, 467 665 (N50 = 650) singleton contigs were generated for the Kariega Estuary samples and 514 739 (N50 = 720) for the Bushmans Estuary samples. On average, 91.6 % of the raw sequences were successfully mapped against the assembled metagenomic contig. The control samples comprised 776 081 (N50 = 1 039) and 345 030 (N50 = 1 171) contigs for the Bushmans Estuary and the Kariega Estuary, respectively (Supplementary Table 3). More than 88% of the metagenomic taxonomic assignments for each fecal sample consisted of unknowns, host DNA and non-target DNA, and a similar trend was seen for the metabarcoding control samples (Supplementary Table 4).
Both the metabarcoding and the metagenomic datasets included a large proportion of pipefish DNA. For metabarcoding, this included 31% and 34% of the total number of sequences for S. watermeyeri from the Bushmans and Kariega estuaries, respectively, and 20% and 14% for the S. temminckii samples from the Bushmans and Kariega estuaries, respectively. The proportion of pipefish DNA was even greater for the metagenomic data, with 54% and 95% for S. watermeyeri from the Bushmans and Kariega, respectively, and 69% and 76% for S. temminckii from the Bushmans and Kariega estuaries, respectively.
Metabarcoding results showed a clear preference of each pipefish for a specific type of prey (Figure 1A). The estuarine pipefish data were numerically dominated by a single species of calanoid copepod, Pseudodiaptomus hessei (at both sites, this number exceeded 600 000 sequences), whereas sequences from the shrimp Palaemon peringueyi dominated the feces of S. temminckii. Other species that comprised at least 1% of the reads included two gastropods, Assiminea capensis (Assimineidae) and the Hydrobia knysnaensis (Hydrobiidae). The control samples mostly contained non-target DNA originating from bacteria, algae and larger vertebrates. A single exception was the presence of macroinvertebrate DNA from the gastropod Haminoea alfredensis in the Bushmans control sample. This species was not found in any of the fecal samples.
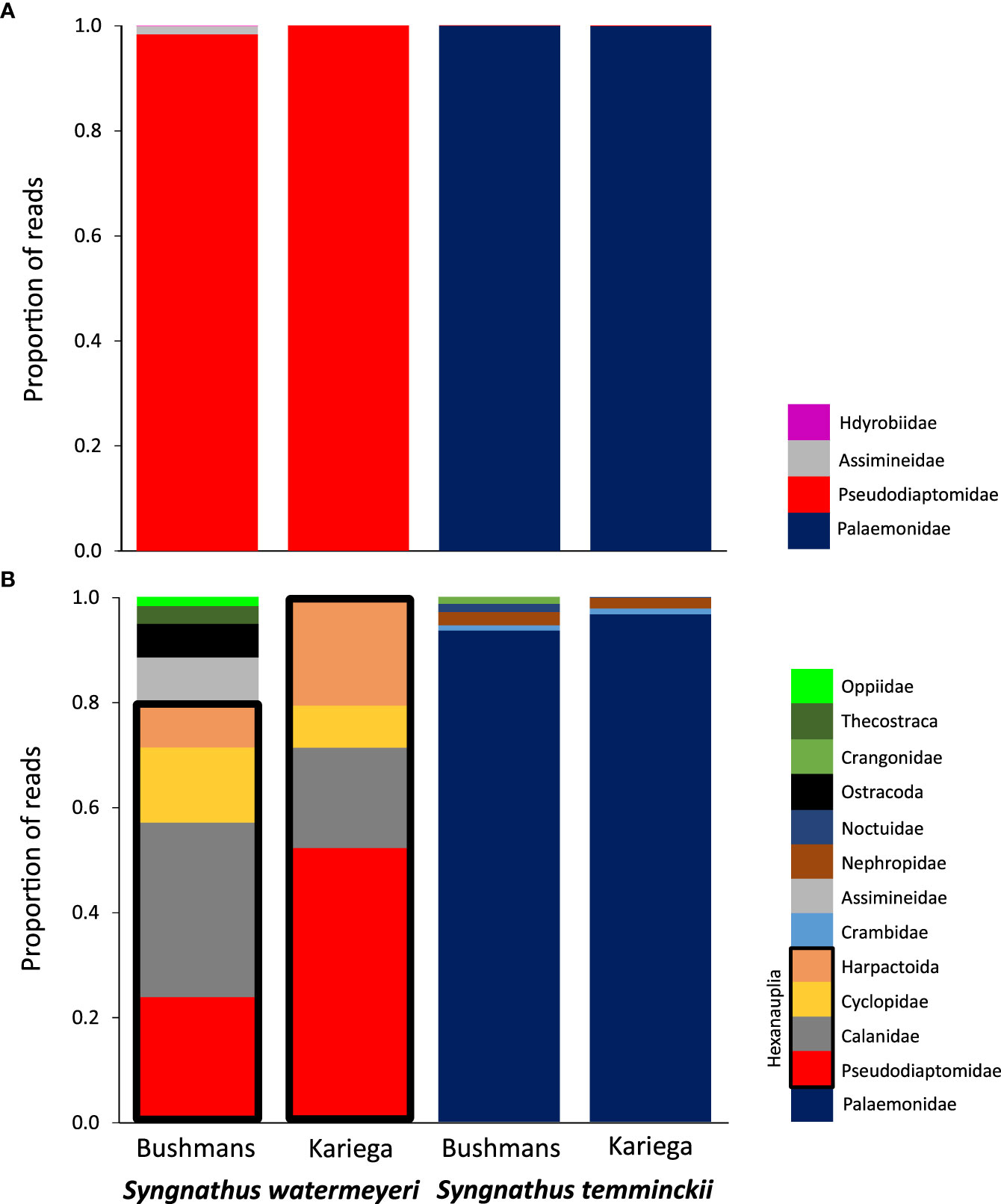
Figure 1 A comparison of the proportion of reads from putative prey families found in the feces of the two pipefish species; (A) metabarcoding data; (B) metagenomic data. In each case, species with a number of reads <1% of the total number of reads per fecal samples were excluded. The Hexanauplia (copepod) families found in the metagenomic data of S. watermeyeri are grouped together by black boxes.
Metagenomics recovered more diversity of species than metabarcoding (Table 1), although the majority of these were rare, and were excluded from Figure 1B because they were not visible (<1% of the total number of reads). In addition to the copepod Pseudodiaptomus hessei, this method also identified three additional but unidentified copepods (each in a different higher taxon, i.e. Calanidae, Cyclopidae and Harpactoida) as being important in the diet of S. watermeyeri. Similar to metabarcoding, metagenomics identified the gastropod A. capensis, and other reasonably common taxa identified included members of the Ostracoda (several distinct species were grouped here because of taxonomic uncertainty), Thecostraca and Oppiidae. The control samples included DNA from several species of gastropods that were either absent or present at very low concentrations in the fecal samples (Afrolittorina africana), and some ostracods.
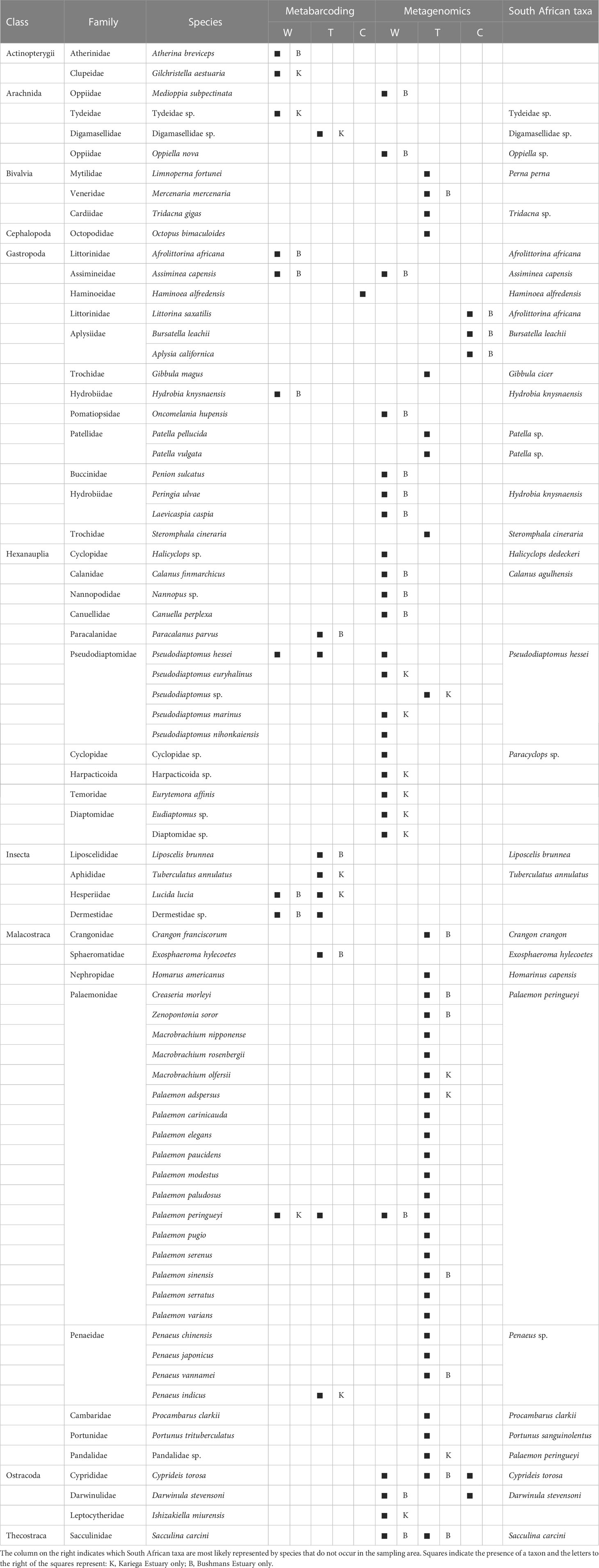
Table 1 Putative prey taxa found in the feces of Syngnathus watermeyeri (W) and S. temminckii (T), compared to species from the same classes found in the control (C) samples, based on metabarcoding and metagenomics.
A total of 24 species of Palaemonidae were found in the feces of S. temminckii, compared to only one (Palaemon peringueyi) that was identified by metabarcoding. Most of these do not occur in South Africa or in the study area, suggesting that they most likely all represent the same species, Palaemon peringueyi, and were identified because no complete genome has yet been sequenced for this species. This is not only true for the species of Palaemon, but also for Macrobrachium. Although the genus occurs in subtropical South Africa, its range does not extend to the Bushmans and Kariega estuaries. The metagenomic results for this pipefish are thus largely congruent as those of based on metabarcoding in that caridean shrimp are particularly important in its diet (Figure 1B).
Discussion
This study used metabarcoding and metagenomic analysis of eDNA collected non-intrusively from fecal samples to compare the dietary preferences of the Critically Endangered estuarine pipefish, Syngnathus watermeyeri, and its more abundant congener, S. temminckii. The research aimed to provide information that contributes towards improving the conservation management of S. watermeyeri, particularly in captive breeding and releases of captive-bred progeny into estuaries within the species’ historical distribution range.
Due to the high conservation status of the estuarine pipefish, fecal samples could only be collected from a few individuals in a single season, and, fecal material from each species and estuary were pooled to minimise the cost of sequencing. Therefore, this study can only provide a snapshot of diet composition in each population rather than information on the preferences of individual pipefishes. However, the fact that all individuals from the same site were captured on the same day indicates that both species had access to the same prey species, thus rejecting the idea that the differences in diet composition could be due to small sample sizes or spatial and temporal separation of the captured individuals.
The results showed clear differences in dietary preferences between the two pipefish species. Although some species were found in the feces of both species, these were rare, and each pipefish had a preference for different types of prey. Only two invertebrate classes (Malacostraca and Hexanauplia) were consistently found in the diet of the two pipefish species. In each case, these were limited to a few species. The estuarine pipefish has a preference for copepods (class Hexanauplia). The calanoid copepod Pseudodiaptomus hessei dominated the metabarcoding sequences, while the metagnomic dataset included three additional species, each in a different family. In contrast, although the feces of S. temminckii also contained a small number of sequences from P. hessei, this pipefish showed a preference for the caridean shrimp Palaemon peringueyi. In addition to crustaceans, both metabarcoding and metagenomics confirmed the importance of the gastropod Assiminea capensis in the diet of the estuarine pipefish. As the adults of Palaemonidae and Assiminea are too large to fit through the mouths of pipefishes, it is likely that the S. watermeyeri preys upon their larvae.
Metabarcoding is presently the primary molecular method to reconstruct the diet and assess the importance of different food items based on the number of retrieved sequences. As is the case in all PCR-based approaches, our results concerning the importance of these main prey items based on metabarcoding may have been impacted by amplification bias (Tedersoo et al., 2015; Krehenwinkel et al., 2017). Metagenomics is predicted to provide superior taxonomic resolution compared to metabarcoding because of its capacity to incorporate information from multiple markers across the genome, and to assemble longer contigs for more accurate species identification (Srivathsan et al., 2016; Chua et al., 2021), and its power was clearly demonstrated by the fact that it identified several more important copepod species, in addition to P. hessei, that metabarcoding failed to identify.
The efficiency of taxonomic rank assignment in metabarcoding depends strongly on how comprehensive the available reference database is regarding the species represented and the markers used to amplify them (Chua et al., 2021), but this is even more so the case with metagenomics. In this study, most metagenomic identifications were for species that do not occur in the study area; this was particularly evident in the family Palaemonidae. In contrast to the metabarcoding sequences, most of which originated from Palaemon peringueyi, several additional caridean shrimps of the family Palaemonidae (which includes the genera Palaemon and Macrobrachium). This is likely an artifact of gene regions being represented in this dataset that have not yet been sequenced for P. peringueyi. Consequently, the taxonomic rank reported was often from species for which complete genomes have been published, including M. nipponense and P. carinicauda. Hence, both sequencing methods used in this study display bias that can be attributed to incomplete databases.
Despite these discrepancies, this study provides important information on differences in prey selection between the two pipefish species. The choice of prey items likely depends on clear differences in snout length and gape size and variations in hunting tactics. The estuarine pipefish’s shorter snout size may restrict the size of prey species on which it can feed, which would explain its preference for small copepods (and potentially also gastropod veliger larvae). It is also possible that S. watermeyeri may detect small zooplankton more effectively than S. temminckii because of the position of its eyes, which are located closer to the tip of the snout. In addition to stronger suction and greater gape size due to a larger and longer snout, S. temminckii has been observed to hunt its prey actively, whereas S. watermeyeri is more passive and waits to ambush prey animals that swim within its reach (Sven-Erick Weiss, pers. obs.). Together, these factors likely enable S. temminckii to stalk prey that might otherwise escape, such as the larvae of P. peringueyi.
The finding that the Critically Endangered S. watermeyeri relies to a large extent on relatively small zooplankters (particularly copepods) supports the hypothesis that significant reductions in zooplankton abundance in response to reduced freshwater influx (Grange et al., 2000) can result in estuarine pipefish population declines (Whitfield et al., 2017). Excessive freshwater abstraction has transformed both estuaries inhabited by S. watermeyeri from systems with well-developed salinity gradients to homogeneously marine-dominated systems. The Bushmans River, for example, has approximately 30 impoundments in its upper reaches that have significantly reduced freshwater inflow (Bornman and Klages, 2004). While this would have resulted in a decrease in phytoplankton biomass (and, by extension, zooplankton biomass) (Hilmer and Bate, 1990), the resulting increase in water clarity would also have facilitated the formation of the current extensive submerged macrophyte beds (Bornman and Klages, 2004). Because of this contradiction (reduced food availability but increased habitat availability), it cannot be ruled out that the two estuaries in their current marine-dominated state have a higher carrying capacity for S. watermeyeri than they would have had under natural conditions, at least during periods of moderate rainfall.
This study suggests that resource competition between the two pipefish species is likely negligible as they mostly consume a different diet. Thus, it is not an important factor that could explain why S. watermeyeri is such a rare species. Although the estuarine pipefish prefers copepods, results from both sequencing methods indicated that its diet could be supplemented in captivity with gastropod veliger larvae to resemble how they would eat in their natural environment. Other taxa may also be opportunistically consumed, including ostracods.
Overall, eDNA sequencing methods have offered a way of identifying soft-bodied prey that would have been difficult or even impossible to detect through traditional morphological analysis of fecal samples, such as the copepods identified here. Moreover, the identification of veliger shells in the feces would have been insufficient to identify the species from which they originated. However, the results also document how the lack of reference sequences can greatly impact the efficiency of metabarcoding and metagenomics as diet analysis methods. Our study highlights the critical need for a more comprehensive reference database of South African estuarine macroinvertebrates to improve the power of both metabarcoding and metagenomics in identifying the prey species present in fecal eDNA samples.
Data availability statement
The data presented in the study are deposited in the NCBI Sequence Read Archive (SRA) repository BioProject ID PRJNA911940.
Ethics statement
The animal study was reviewed and approved by the SAIAB Animal Ethics Committee (reference number: 25/4/1/5_2018-07) and the University of Johannesburg Faculty of Science Ethics Committee (reference number: 2021-10-05/Serite_Teske).
Author contributions
PC, AW, and PT conceived the research. PC and PT generated funding and obtained permits. ON, AE-K, PC, NJ, TB, and PT participated in sampling and/or laboratory work. CS and AE-K analyzed the data. CS and PT created figures and tables. AE-K, BJ, and PT supervised the students. All authors contributed to the article and approved the submitted version.
Funding
This study was funded by the Mohamed bin Zayed species conservation fund (Mohamed bin Zayed Species project number 172516007) and a URC grant awarded to PT.
Acknowledgments
The Centre for High-Performance Computing (CHPC) is thanked for granting us access to the Lengau Cluster, and the University of Johannesburg IT services are acknowledged for their support. CS is grateful to the Department of Higher Education for awarding her a Nurturing Emerging Scholars Program (NESP) scholarship. ON acknowledges the National Research Foundation (NRF) for a Master’s bursary, and AE-K was partly supported through an NRF South African National Antarctic Programme (SANAP) research grant. Sven-Erick Weiss, Jody-Carynn Oliver, Laura Tensen, and Claudia Schnelle are thanked for their assistance with sampling and laboratory analysis.
Conflict of interest
The authors declare that the research was conducted in the absence of any commercial or financial relationships that could be construed as a potential conflict of interest.
Publisher’s note
All claims expressed in this article are solely those of the authors and do not necessarily represent those of their affiliated organizations, or those of the publisher, the editors and the reviewers. Any product that may be evaluated in this article, or claim that may be made by its manufacturer, is not guaranteed or endorsed by the publisher.
Supplementary material
The Supplementary Material for this article can be found online at: https://www.frontiersin.org/articles/10.3389/fmars.2023.1116741/full#supplementary-material
References
Adams J. B. (2016). Distribution and status of Zostera capensis in South African estuaries — a review. S. Afr. J. Bot. 107, 63–73. doi: 10.1016/j.sajb.2016.07.007
Alberdi A., Aizpurua O., Gilbert M. T. P., Bohmann K. (2018). Scrutinizing key steps for reliable metabarcoding of environmental samples. Methods Ecol. Evol. 9, 134–147. doi: 10.1111/2041-210X.12849
Allanson B. R., Herbert D. G. (2005). A newly discovered population of the critically endangered false limpet Siphonaria compressa Allanson, 1958 (Pulmonata: Siphonariidae), with observations on its reproductive biology. S. Afr. J. Sci. 101, 95–97. doi: 10.10520/EJC96338
Altschul S. F., Gish W., Miller W., Myers E. W., Lipman D. J. (1990). Basic local alignment search tool. J. Mol. Biol. 215, 403–410. doi: 10.1016/S0022-2836(05)80360-2
Ang A., Ismail M. R. B., Meier R. (2010). Reproduction and infant pelage colouration of the banded leaf monkey (Mammalia: Primates: Cercopithecidae) in Singapore. Raffles Bull. Zool. 58, 411–415.
Bohmann K., Evans A., Gilbert M. T. P., Carvalho G. R., Creer S., Knapp M., et al. (2014). Environmental DNA for wildlife biology and biodiversity monitoring. Trends Ecol. Evol. 29, 358–367. doi: 10.1016/j.tree.2014.04.003
Bolger A. M., Lohse M., Usadel B. (2014). Trimmomatic: a flexible trimmer for illumina sequence data. Bioinformatics 30, 2114–2120. doi: 10.1093/bioinformatics/btu170
Bornman T., Klages N. T. W. (2004). Upgrade of Kenton-on-Sea/Bushmans River Mouth by Albany Coast Water Board. IECM Report No. C84, University of Port Elizabeth, Port Elizabeth.
Boukhdoud L., Saliba C., Parker L. D., McInerney N. R., Kahale R., Saliba I., et al. (2021). Using DNA metabarcoding to decipher the diet plant component of mammals from the Eastern Mediterranean region. Metabarcoding Metagenom. 5, 219–231. doi: 10.3897/mbmg.5.70107
Bovo S., Ribani A., Utzeri V. J., Schiavo G., Bertolini F., Fontanesi L. (2018). Shotgun metagenomics of honey DNA: evaluation of a methodological approach to describe a multi-kingdom honey bee derived environmental DNA signature. PloS One 13, e0205575. doi: 10.1371/journal.pone.0205575
Chua P. Y. S., Crampton-Platt A., Lammers Y., Alsos I. G., Boessenkool S., Bohmann K. (2021). Metagenomics: a viable tool for reconstructing herbivore diet. Mol. Ecol. Resour. 21, 2249–2263. doi: 10.1111/1755-0998.13425
Claassens L., de Villiers N. M., Seath J., Wasserman J. (2022). Distribution and density of the Critically Endangered estuarine pipefish across its range – implications for conservation. Aquat. Conserv. 32, 28–41. doi: 10.1002/aqc.3742
Clarke L. J., Beard J. M., Swadling K. M., Deagle B. E. (2017). Effect of marker choice and thermal cycling protocol on zooplankton DNA metabarcoding studies. Ecol. Evol. 7, 873–883. doi: 10.1002/ECE3.2667
Doyle J. (1991). “DNA Protocols for plants”, in Molecular techniques in taxonomy (Berlin Heidelberg: Springer), 283–293. doi: 10.1007/978-3-642-83962-7_18
Edgar G. J., Barrett N. S., Graddon D. J., Last P. R. (2000). The conservation significance of estuaries: a classification of Tasmanian estuaries using ecological, physical and demographic attributes as a case study. Biol. Conserv. 92, 383–397. doi: 10.1016/S0006-3207(99)00111-1
Emami-Khoyi A., Hartley D. A., Paterson A. M., Boren L. J., Cruickshank R. H., Ross J. G., et al. (2016). Identifying prey items from New Zealand fur seal (Arctocephalus forsteri) faeces using massive parallel sequencing. Conservation Genet. Resour. 8, 343–352. doi: 10.1007/s12686-016-0560-9
Ferravante C., Memoli D., Palumbo D., Ciaramella P., di Loria A., D’Agostino Y., et al. (2021). HOME-BIO (shotgun metagenomic analysis of biological entities): a specific and comprehensive pipeline for metagenomic shotgun sequencing data analysis. BMC Bioinf. 22, 106. doi: 10.1186/s12859-021-04004-y
Grange N., Whitfield A. K., De Villiers C. J., Allanson B. R. (2000). The response of two South African east coast estuaries to altered river flow regimes. Aquat. Conserv. 10, 155–177. doi: 10.1002/1099-0755(200005/06)10:3<155::AID-AQC406>3.0.CO;2-Z
Gray J. S., Poore G. C. B., Ugland K. I., Wilson R. S., Olsgard F., Johannessen O. (1997). Coastal and deep-sea benthic diversities compared. Mar. Ecol. Prog. Ser. 159, 97–103. doi: 10.3354/MEPS159097
Gumm J. M., Snekser J. L., Leese J. M., Little K. P., Leiser J. K., Imhoff V. E., et al. (2011). Management of interactions between endangered species using habitat restoration. Biol. Conserv. 144, 2171–2176. doi: 10.1016/J.biocon.2011.05.006
Gurevich A., Saveliev V., Vyahhi N., Tesler G. (2013). QUAST: quality assessment tool for genome assemblies. Bioinformatics 29, 1072–1075. doi: 10.1093/bioinformatics/btt086
Harper L. R., Watson H., Donnelly R., Hampshire R., Sayer C. D., Breithaupt T., et al. (2020). Using DNA metabarcoding to investigate diet and niche partitioning in the native European otter (Lutra lutra) and invasive American mink (Neovison vison). Metabarcoding Metagenom. 4, 113–133. doi: 10.3897/mbmg.4.56087
Hawlitschek O., Fernández-González A., Balmori-de la Puente A., Castresana J. (2018). A pipeline for metabarcoding and diet analysis from fecal samples developed for a small semi-aquatic mammal. PloS One 13, e0201763. doi: 10.1371/journal.pone.0201763
Hebert P. D. N., Ratnasingham S., DeWaard J. R. (2003). Barcoding animal life: cytochrome c oxidase subunit 1 divergences among closely related species. Proc. R. Soc. B 270, S96–S99. doi: 10.1098/RSBL.2003.0025
Hilmer T., Bate G. C. (1990). Covariance analysis of chlorophyll distribution in the Sundays River estuary, Eastern Cape. S. Afr. J. Aquat. Sci. 16, 37–59. doi: 10.1080/10183469.1990.10557366
Kahlke T., Ralph P. J. (2019). BASTA – taxonomic classification of sequences and sequence bins using last common ancestor estimations. Methods Ecol. Evol. 10, 100–103. doi: 10.1111/2041-210X.13095
Kajee M., Griffiths C. L., Lamberth S. J. (2018). Long-term physico-chemical and faunal changes in a small, rural South African estuary. Afr. Zool. 53, 127–137. doi: 10.1080/15627020.2018.1552838
Kartzinel T. R., Chen P. A., Coverdale T. C., Erickson D. L., Kress W. J., Kuzmina M. L., et al. (2015). DNA metabarcoding illuminates dietary niche partitioning by African large herbivores. Proc. Natl. Acad. Sci. U.S.A. 112, 8019–8024. doi: 10.1073/pnas.1503283112
Kaselowski T., Adams J. B. (2013). Not so pristine - characterising the physico-chemical conditions of an undescribed temporarily open/closed estuary. Water SA. 39, 627–636. doi: 10.4314/wsa.v39i5.6
Klare U., Kamler J. F., Macdonald D. (2011). A comparison and critique of different scat-analysis methods for determining carnivore diet. Mamm. Rev. 41, 294–312. doi: 10.1111/j.1365-2907.2011.00183.x
Krehenwinkel H., Wolf M., Lim J. Y., Rominger A. J., Simison W. B., Gillespie R. G. (2017). Estimating and mitigating amplification bias in qualitative and quantitative arthropod metabarcoding. Sci. Rep. 7, 1–12. doi: 10.1038/s41598-017-17333-x
Kurtz S. (2003). The Vmatch large scale sequence analysis software. Available at: http://www.vmatch.de2003 [Accessed April 15, 2023].
Landa A., Flagstad Ø., Areskoug V., Linnell J. D. C., Strand O., Ulvund K. R., et al. (2017). The endangered Arctic fox in Norway – the failure and success of captive breeding and reintroduction. Polar Res. 36, 9. doi: 10.1080/17518369.2017.1325139
Langmead B., Trapnell C., Pop M., Salzberg S. (2021). Ultrafast and memory-efficient alignment of short DNA sequences to the human genome. Genome Biol. 10, R25. doi: 10.1186/gb-2009-10-3-r25
Leray M., Yang J. Y., Meyer C. P., Mills S. C., Agudelo N., Ranwez V., et al. (2013). A new versatile primer set targeting a short fragment of the mitochondrial COI region for metabarcoding metazoan diversity: application for characterizing coral reef fish gut contents. Front. Zool. 10, 34. doi: 10.1186/1742-9994-10-34
Li H., Handsaker B., Wysoker A., Fennell T., Ruan J., Homer N., et al. (2009). The sequence Alignment/Map format and SAMtools. Bioinformatics 25, 2078–2079. doi: 10.1093/bioinformatics/btp352
Li D., Liu C.-M., Luo R., Sadakane K., Lam T.-W. (2015). MEGAHIT: an ultra-fast single-node solution for large and complex metagenomics assembly via succinct de Bruijn graph. Bioinformatics 31, 1674–1676. doi: 10.1093/bioinformatics/btv033
Lockyear J., Hecht T., Kaiser H., Teske P. (2006). The distribution and abundance of the endangered Knysna seahorse Hippocampus capensis (Pisces: Syngnathidae) in South African estuaries. Afr. J. Aquat. Sci. 31, 275–283. doi: 10.2989/16085910609503897
Mata V. A., Rebelo H., Amorim F., McCracken G. F., Jarman S., Beja P. (2019). How much is enough? effects of technical and biological replication on metabarcoding dietary analysis. Mol. Ecol. 28, 165–175. doi: 10.1111/mec.14779
Martin M. (2011). Cutadapt removes adapter sequences from high-throughput sequencing reads. EMBnet J. 17, 10–12. doi: 10.14806/ej.17.1.200
Mkare T. K., van Vuuren B. J., Teske P. R. (2017). Conservation implications of significant population differentiation in an endangered estuarine seahorse. Biodivers. Conserv. 26, 1275–1293. doi: 10.1007/s10531-017-1300-5
Morrill A., Kaunisto K. M., Mlynarek J. J., Sippola E., Vesterinen E. J., Forbes M. R. (2021). Metabarcoding prey DNA from fecal samples of adult dragonflies shows no predicted sex differences, and substantial inter-individual variation, in diets. PeerJ 9, e12634. doi: 10.7717/peerj.12634
Mumma M. A., Adams J. R., Zieminski C., Fuller T. K., Mahoney S. P., Waits L. P. (2016). A comparison of morphological and molecular diet analyses of predator scats. J. Mammal. 97, 112–120. doi: 10.1093/jmammal/gyv160
Ntuli N. N., Nicastro K. R., Zardi G. I., Assis J., McQuaid C. D., Teske P. R. (2020). Rejection of the genetic implications of the “Abundant Centre Hypothesis” in marine mussels. Sci. Rep. 10, 604. doi: 10.1038/s41598-020-57474-0
Orth R. J., Carruthers T. J. B., Dennison W. C., Duarte C. M., Fourqurean J. W., Heck K. L., et al. (2006). A global crisis for seagrass ecosystems. Bioscience 56, 987–996. doi: 10.1641/0006-3568(2006)56[987:AGCFSE]2.0.CO;2
Payne M. F., Rippingale R. J., Longmore R. B. (1998). Growth and survival of juvenile pipefish Stigmatopora argus fed live copepods with high and low HUFA content. Aquaculture 167, 237–245. doi: 10.1016/S0044-8486(98)00318-4
Piñol J. (2021). Genotype by sequencing: an alternative new method to amplicon metabarcoding and shotgun metagenomics for the assessment of eukaryote biodiversity. Mol. Ecol. Resour. 21, 1001–1004. doi: 10.1111/1755-0998.13320
Pompanon F., Deagle B. E., Symondson W. O. C., Brown D. S., Jarman S. N., Taberlet P. (2012). Who is eating what: diet assessment using next generation sequencing. Mol. Ecol. 21, 1931–1950. doi: 10.1111/j.1365-294X.2011.05403.x
Rognes T., Flouri T., Nichols B., Quince C., Mahé F. (2016). VSEARCH: a versatile open source tool for metagenomics. PeerJ 2016, e2584. doi: 10.7717/peerj.2584
Shehzad W., Riaz T., Nawaz M. A., Miquel C., Poillot C., Shah S. A., et al. (2012). Carnivore diet analysis based on next-generation sequencing: application to the leopard cat (Prionailurus bengalensis) in Pakistan. Mol. Ecol. 21, 1951–1965. doi: 10.1111/j.1365-294X.2011.05424.x
Sousa L. L., Silva S. M., Xavier R. (2019). DNA Metabarcoding in diet studies: unveiling ecological aspects in aquatic and terrestrial ecosystems. Environ. DNA 1, 199–214. doi: 10.1002/edn3.27
Srivathsan A., Ang A., Vogler A. P., Meier R. (2016). Fecal metagenomics for the simultaneous assessment of diet, parasites, and population genetics of an understudied primate. Front. Zool. 13, 17. doi: 10.1186/s12983-016-0150-4
Srivathsan A., Sha J. C. M., Vogler A. P., Meier R. (2015). Comparing the effectiveness of metagenomics and metabarcoding for diet analysis of a leaf-feeding monkey (Pygathrix nemaeus). Mol. Ecol. Resour. 15, 250–261. doi: 10.1111/1755-0998.12302
Strum S. C. (2005). Measuring success in primate translocation: a baboon case study. Am. J. Primatol. 65, 117–140. doi: 10.1002/AJP.20103
Tedersoo L., Anslan S., Bahram M., Põlme S., Riit T., Liiv I., et al. (2015). Shotgun metagenomes and multiple primer pair-barcode combinations of amplicons reveal biases in metabarcoding analyses of fungi. MycoKeys 10, 1–43. doi: 10.3897/mycokeys.10.4852
Tran B. T., Kim K. Y., Heo J. S., Park S. J., Park H. K., Choi Y. H. (2022). Determination of the pacific oyster Magallana gigas (Crassostrea gigas) diet composition in two aquaculture farms by fecal DNA metabarcoding. Aquaculture 552, 738042. doi: 10.1016/j.aquaculture.2022.738042
Turpie J. K., Adams J. B., Joubert A., Harrison T. D., Colloty B. M., Maree R. C., et al. (2002). Assessment of the conservation priority status of South African estuaries for use in management and water allocation. Water SA. 28, 191–206. doi: 10.4314/wsa.v28i2.4885
van Wassenbergh S., Strother J. A., Flammang B. E., Ferry-Graham L. A., Aerts P. (2008). Extremely fast prey capture in pipefish is powered by elastic recoil. J. R. Soc. Interface 5, 285–296. doi: 10.1098/rsif.2007.1124
Weiss S.-E., Emami-Khoyi A., Kaiser H., Cowley P. D., James N. C., Jansen van Vuuren B., et al. (2022). The last two remaining populations of the critically endangered estuarine pipefish are inbred and not genetically distinct. Front. Mar. Sci. 8, 756595. doi: 10.3389/fmars.2021.756595
Whitfield A. K. (1995). Threatened fishes of the world: Syngnathus watermeyeri Smith, 1963 (Syngnathidae). Environ. Biol. Fishes 43, 1–2. doi: 10.1007/BF00002484
Whitfield A. K., Mkare T. K., Teske P. R., James N. C., Cowley P. D. (2017). Life-histories explain the conservation status of two estuary-associated pipefishes. Biol. Conserv. 212, 256–264. doi: 10.1016/j.biocon.2017.06.024
Keywords: DNA barcoding, environmental DNA (eDNA), fecal DNA, diet analysis, endangered species, metabarcoding, metagenomics, pipefish
Citation: Serite CP, Emami-Khoyi A, Ntshudisane OK, James NC, Jansen van Vuuren B, Bodill T, Cowley PD, Whitfield AK and Teske PR (2023) eDNA metabarcoding vs metagenomics: an assessment of dietary competition in two estuarine pipefishes. Front. Mar. Sci. 10:1116741. doi: 10.3389/fmars.2023.1116741
Received: 05 December 2022; Accepted: 10 May 2023;
Published: 12 June 2023.
Edited by:
Daria Sanna, University of Sassari, ItalyReviewed by:
Alberto Pallavicini, University of Trieste, ItalyWon Young Lee, Korea Polar Research Institute, Republic of Korea
Copyright © 2023 Serite, Emami-Khoyi, Ntshudisane, James, Jansen van Vuuren, Bodill, Cowley, Whitfield and Teske. This is an open-access article distributed under the terms of the Creative Commons Attribution License (CC BY). The use, distribution or reproduction in other forums is permitted, provided the original author(s) and the copyright owner(s) are credited and that the original publication in this journal is cited, in accordance with accepted academic practice. No use, distribution or reproduction is permitted which does not comply with these terms.
*Correspondence: Peter R. Teske, pteske101@gmail.com