- 1Hopkins Marine Station, Stanford University, Pacific Grove, CA, United States
- 2Cascadia Research Collective, Olympia, WA, United States
- 3Whale Research Lab, University of Victoria, Victoria, BC, Canada
Understanding the function of baleen whale acoustic signals requires the investigation of calling behaviors relative to location, timing, and behavioral state. Previous studies of gray whale (Eschrichtius robustus) vocal behavior have principally used passive recorders and been conducted primarily on breeding grounds and along migratory routes, and not areas associated with foraging. We conducted an analysis of accelerometer and acoustic data collected during 12 deployments of animal-borne tags in 2016, 2019, and 2021 on gray whales that return annually to northern Puget Sound, Washington. We identified 141 calls from gray whales in approximately 128 hours of accelerometer and/or acoustic data collected from tag deployments. The most prominent were pulsive ‘rumble-like’ calls, upsweeping tones, and moans. Using the tag’s accelerometer to identify calls produced by tagged animals enabled us to explore the behavioral context behind call production, revealing that vocalizations were made primarily at slow speeds (mean 1.03 ± 0.26 m/s-1), shallow depths (mean 7.63 ± 4.99 m), and temporally proximate to surface behavior (mean 43.3 ± 39.66 sec) most similar to non-foraging associated depth (mean 5.78 ± 1.46 m and speed (mean 1.46 ± 1.11 m/s-1) profiles. Vocalizations originating from the tagged animal occurred closer to the conclusion of a foraging event (mean 87.7 ± 119.32 min) than the beginning, with only 7% of all calls occurring during periods of feeding. This study demonstrates that gray whales remain vocal on foraging grounds and that call-associated accelerometry signals can be a valuable tool in identifying individual callers in animal-borne acoustic data.
Introduction
The use of passive acoustic monitoring (PAM) in marine mammal research has become more widespread over the past decade. Increasingly, the functional use of calls by baleen whales under various social and behavioral contexts has been examined, as has the relevance of calling to population dynamics (Küsel et al., 2011; Marques et al., 2012). Evaluating population-specific trends in call or cue rates from passively collected acoustic data has, for example, been used to estimate population densities (Mellinger et al., 2007; Küsel et al., 2011; Marques et al., 2012; Stevenson et al., 2020). However, using passively collected acoustic data to monitor populations more comprehensively than noting presence and relative abundance requires a greater understanding of the contextual underpinnings of that population’s vocal activity, as well as the temporal and geospatial trends in their acoustic behavior (Stimpert et al., 2015; Lewis et al., 2018). A functional understanding of the acoustic behavior of a given population can yield insight into how individuals and groups interact on spatiotemporal scales (Stimpert et al., 2015; Lewis et al., 2018).
Technological advances in multi-sensor animal-borne tag capabilities over the past decade, such as Digital Acoustic Recording Tags (DTAGs) and those developed by Custom Animal Tracking Solutions (CATS), have increased our capacity to collect high-resolution and fine-scale video, audio, and kinematic data on baleen whales (Rutz & Hays, 2009; Cade et al., 2021). This synchronous collection of acoustic and accelerometer data has the potential to uncover contextual or functional aspects of acoustic activity in whales and add context to studies utilizing remotely collected acoustic data only. A number of studies have used a tag’s high-resolution accelerometer to differentiate between tagged and non-tagged animal call production in fin (Balaenoptera physalus), blue (Balaenoptera musculus), and humpback whale (Megaptera novaeangliae) acoustic tag data. (Goldbogen et al., 2014; Stimpert et al., 2015; Saddler et al., 2017; Oestreich et al., 2020; Stimpert et al., 2020). These studies found that calls produced by tagged animals were synchronously detected on both the tag’s hydrophone and accelerometer, thought to be the product of active vibrating of the tagged animal’s body as it produces sound (Goldbogen et al., 2014; Stimpert et al., 2015; Oestreich et al., 2020; Stimpert et al., 2020). Saddler et al. (2017), however, concluded that a proportion of the calls identified from blue whale tag data could have originated from a non-tagged individual. As such, further exploration into the context of call occurrence in tagged animals relative to trends in call type use, diel patterns, and call rates, is warranted to better understand the application of accelerometers in identifying individual-level patterns in calling or call function across various species.
Studies on the acoustic behavior of Eastern North Pacific (ENP) gray whales (Eschrichtius robustus) have been primarily focused on breeding grounds and during migration (e.g. Cummings et al., 1968; Dahlheim, 1987; Crane & Lashkari, 1996; Charles, 2011; Ponce et al., 2012; Guazzo et al., 2017; Burnham et al., 2018; Burnham & Duffus, 2020). Little is currently known about gray whale acoustic activity, calling function, and the relevance of both to population dynamics in feeding areas (Stafford et al., 2007; Burnham et al., 2018; Frouin-Mouy et al., 2020; Burnham & Duffus, 2022). Gray whales produce several call types, initially categorized by Dahlheim (1987) into six core classes from recordings made principally in breeding lagoons. These categories included pulsive knock-like sounds (Class 1), sweeping tones (Class 2), moans (Class 3), ‘rumbles’ (Class 4) and two variations of subsurface exhalations (Classes 5 and 6) (Dahlheim, 1987). Class 1 knock calls have been found to be the most prevalent call when gray whales are in breeding/calving lagoons (Dahlheim, 1987; Ollervides, 2001; Charles, 2011); seeming to suggest social or reproductive function (Youngson and Darling, 2016), but have also been reported present during migration (Crane & Lashkari, 1996; Burnham & Duffus, 2020) and in association with feeding (Dahlheim, 1987; Burnham & Duffus, 2022)
More recently, studies have been successful in documenting gray whale calling behavior during periods of migration, showing that Class 3 moans dominate the acoustic repertoire in this context (Crane & Lashkari, 1996; Guazzo et al., 2017; Burnham et al., 2018; Rannankari et al., 2018; Burnham & Duffus, 2020). Comparatively less work has been conducted on foraging grounds (Stafford et al., 2007), though a recent study showed that gray whales may alter their vocal behavior on feeding grounds in relation to social setting and prey abundance (Burnham & Duffus, 2022). Understanding a population’s acoustic activity across the full range of its behavioral states is important in call categorization attempts, as modifications to calling may occur in response to differing social or physiological contexts.
To broaden our knowledge of ENP gray whale call use on foraging grounds, and better understand the potential application of an accelerometer-based caller identification method for the vocally complex gray whale, we performed an acoustically focused analysis of multi-sensor biologging data. Our focal whales were a small group (n = 8 to 12) of known individuals, commonly referred to as the “Sounders”, that return annually to northern Puget Sound (NPS) in Washington State (Weitkamp et al., 1992; Calambokidis et al., 2015; Pruitt and Donoghue, 2016, Calambokidis, 2017; Carretta et al., 2022). While the majority of ENP gray whales migrate directly from their calving and breeding grounds in Baja California Sur to highly productive foraging areas in the Chukchi and Bering Sea from November to February, the Sounders make migratory detours to NPS to feed nearly exclusively on ghost shrimp living in intertidal zones before continuing to the Arctic (Weitkamp et al., 1992; Pruitt & Donoghue, 2016; Calambokidis, 2017; Carretta et al., 2022). The well documented life histories of the Sounders and their predictable return to NPS made them ideal test-candidates for our study. Here, we evaluate the acoustic behavior of gray whales on feeding grounds as well as the application of a new method in caller identification via tag data for this species.
Materials and methods
Tag and PAM system deployment
Twelve suction-cup attached, multi-sensor tags developed by Custom Animal Tracking Solutions (CATS), were deployed on gray whales in NPS in 2016, 2019, and 2021 (Figure 1A) following methods described by Calambokidis (2017). A total of 10 different individuals were tagged, with two individuals (CRC-ID 723 and CRC-ID 383) tagged on more than one occasion. Tag accelerometer sampling rates varied by deployment, ranging from 200 to 400 Hz, while temperature and depth sensors were sampled or down sampled to 10 Hz across all deployments (Supplemental Materials Table 1). Due to developments in tag acoustic capacities over the study period, recording methods varied by deployment. In early deployments, tags lacked a hydrophone component, so audio files were extracted from the tag’s video microphone (22 kHz sampling rate, 16-bit sampling). Tag cameras were programmed to cease recording video and audio during periods of low light overnight, resulting in non-continuous data collection that varied in its duty cycle for each deployment. Deployments in 2021 utilized CATS tags enhanced with HTI 96 hydrophones (24 kHz, 16-bit sampling). All tag deployment operations were supported by concurrent on-water observations and photo-identification efforts.
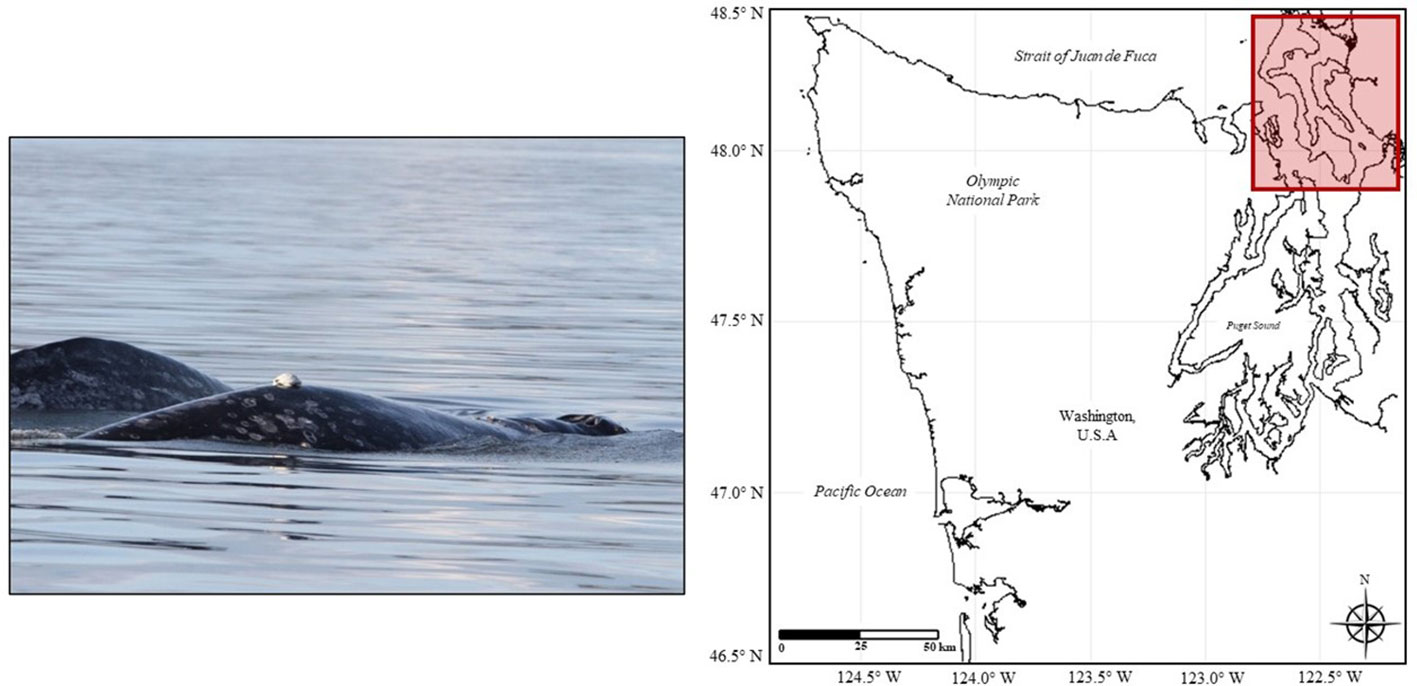
Figure 1 (Left) Deployment of a Custom Animal Tracking Solution (CATS) tag deployed on a gray whale in the study area. Photo taken under NMFS permit #16111. (Right) Map of study area (Puget Sound, Washington, USA) and surrounding areas. Latitude and longitude are shown on the x and y-axes, respectively. The study area, where tag deployments occurred, is shown within the red box boundaries.
Data analysis
Accelerometry data
Acoustic wav files were created from each tag’s calibrated, but undecimated, 200 or 400 Hz tri-axial accelerometry data. Behavioral information such as animal orientation, speed, and depth were discerned following methods described in Cade et al. (2021). Foraging events were identified utilizing custom written scripts in MATLAB (Cade et al., 2021; accessible via GitHub CATS Materials and Methods) to manually mark visually unique patterns of pitch, roll, and heading that are synonymous with intertidal pit feeding in this group of gray whales (J. Calambokidis, unpublished data). Specifically, foraging was defined as extended periods of roll orientation of approximately 90 degrees, at shallow depths and with negative pitch. When possible, foraging behavior was visually verified by observations of sediment disturbance and prey via tag video data. Since this feeding behavior is tidally dependent (J. Calambokidis, unpublished data) a period of 30 minutes with no observation of foraging behavior was sufficient in differentiating between foraging and non-foraging behavioral states.
Acoustic data
All acoustic recordings were examined manually in Raven Pro Interactive Sound Software via spectrograms using a 512 or 256-point Hanning-window FFT with 50% overlap, and a hop size of 256 or 128 samples, respectively. Call duration and frequency range were extracted to aid in comparison to call type descriptions from previous studies. Calls were classified in accordance with call types described by Dahlheim (1987). Additionally, calls from each deployment were ranked by their amplitude and signal to noise ratios (SNR). SNR thresholds were deployment specific due to calibration differences amongst tags. Generally, calls whose fundamental components had a SNR of at least 60 dB were considered high SNR calls and those with components under 60 dB were considered low SNR calls.
All high SNR calls and a random sample of approximately 25% of low SNR calls were cross-examined with the corresponding accelerometer acoustic files for evidence of time-synchronized call signatures as observed in previous studies (Goldbogen et al., 2014; Stimpert et al., 2015; Saddler et al., 2017; Stimpert et al., 2020; Cade et al., 2018; Oestreich et al., 2020). Those found to have a call signature on at least two of the accelerometer’s channels were considered to originate from the tagged animal, whereas all calls with no associated call signature were considered “environmental” calls and unlikely to originate from the tagged individual. All periods of accelerometry acoustic data also supported by hydrophone or camera recording were examined for visually and aurally similar signals not associated with a full-frequency call (false positives). Following comparison of concurrently recorded accelerometer and acoustic audio data, periods of accelerometer data without hydrophone recordings were evaluated for call signatures matching those identified with high SNR calls. General comments as to the structure and distribution of intensity between the accelerometer’s axes of call signatures were also made. All statistical analyses concerning general call characteristics and call correlation to kinematic data such as speed and depth were calculated using custom written scripts in MATLAB or in Excel. A one-way analysis of variance (ANOVA) was performed to assess variation in call production kinematics (depth, speed, proximity to surfacing and foraging behavior) between individual tag deployments.
Hourly calling rates were calculated on a by-deployment basis by taking the total number of tagged whale vocalizations (accelerometer call signatures) for the deployment and dividing by the total hours of associated accelerometry data available. By-deployment calling rates, including deployments with a calling rate of zero (i.e., no vocalizations identified as originating from tagged whale for the entire deployment), were then pooled, and averaged to produce a mean hourly calling rate per individual for all deployments. To take into consideration the effect of tag deployment duration on mean rates calculated, an additional weighted mean was calculated using deployment duration as a proxy for value weight.
Results
Approximately 185 hours of acoustic data were gathered over the course of the study period via CATS tag accelerometers (n = 128.4 hr), tag cameras (n = 26.6 hr), tag hydrophones (n = 17 hr). Information concerning by-deployment variation in data collected and calls identified can be found in Table 2 of the Supplementary Materials. A total of 107 calls were identified in tag hydrophone or camera acoustic recordings between all tag deployments and were classified to one of the six core call types (Dahlheim, 1987; Wisdom et al., 2001; Burnham et al., 2018; Burnham & Duffus, 2020). The dominant call type recorded (n = 37) was higher frequency pulsive Class 4 ‘rumble’ calls. Class 3 tonal calls, encompassing single or multiple-octave tonal calls, often referred to as moans, occurring primarily under 300 Hz, were also heard at relatively high densities (n = 31). Several Class 2 (n = 23) multi-harmonic tonal calls, occurring primarily under 300 Hz and with down or up-swept frequency modulation, and a few (n = 4) Class 1 knock calls were also present (see Figure 1; Supplemental Materials Table 3 for call type examples and descriptive statistics, respectively). Finally, an additional 12 calls were identified and were too variable in call structure to be reliably categorized using current cataloguing systems and were therefore considered “uncategorized”.
Forty-nine calls were classified as high SNR. Of these, 24 showed evidence of an associated accelerometer call signature matching the acoustic detection, with the remaining 25 high SNR calls not found to be associated with an accelerometry call signature. None of the low SNR calls examined (n = 32) were found to have an associated call signature on the accelerometer at the time calls were acoustically detected. Therefore, accelerometry detection was determined to be indicative of tagged animal call production, allowing the categorization of calls as originating from the tagged whale or a conspecific even if the call was categorized to have high SNR. Of the 24 high SNR calls attributed to tagged animals, most were Class 3 (n = 16) or Class 2 (n = 7) calls, with a single (n = 1) Class 4 call. Using accelerometry call signatures verified by acoustic recordings as reference, we identified an additional 34 calls during the approximately 81 hours of accelerometer recordings only (no acoustic data) likely produced by the tagged animal. Combining detection of calls via accelerometry from periods both with and without synchronous acoustic recording resulted in a cumulative total of 58 calls considered to be produced by tagged whales from all deployments. All accelerometry-based call signatures varied in intensity between accelerometer axes on an individual level, with most signatures displaying the highest intensity in the accelerometer’s x (n = 28) or z (n = 25) channel or axes (Figure 2). Lastly, all high SNR calls with accelerometer calls signatures showed structural (frequency range, harmonic count, overall duration) differences when comparing accelerometer data to the full-frequency calls in the hydrophone data (Figure 2). Examination of the data manually did not find any acoustic signals similar in parameters to calls that were not vocalisations (false positive results), confirming that all the signals included in this analysis were indeed calls.
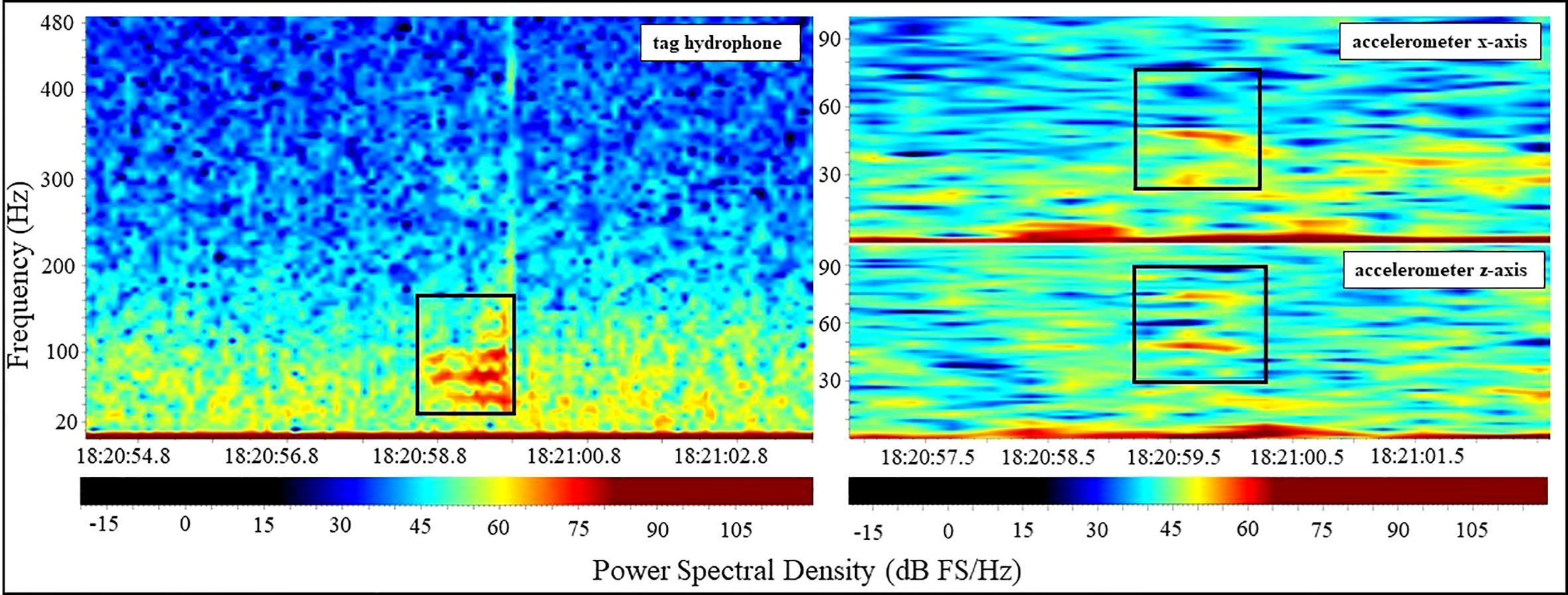
Figure 2 Cross-examination of a high SNR (PSD > 70 dB FS -20) whale call in a tag hydrophone sourced spectrogram (left) with concurrent accelerometer wav files (right) to denote presence or absence of an associated “call signature”. Both the degradation of call structure relative to the original call and the variation in call signature intensity between the two accelerometer axes is observable in this figure. Note that due to high volumes of ambient noise in tag recordings, spectral observation of potential call signatures required aural confirmation of a lower-frequency, tonal moan that was biological in nature and consistent with its associated full-frequency call to be considered to originate from the tagged individual. All spectrograms were generated with Raven Pro Interactive Sound Software, with 4096-point and 256-point Hanning window size for hydrophone (left) and accelerometer (right) sourced acoustic files, respectively.
Comparisons of tagged whale call occurrence to behavioral and kinematic tag information found vocalizations occurred primarily while travelling at slow speeds (mean 1.03 .25 m/s-1), while at shallow depths (mean 7.63 .99 m), and temporally proximate to surface behavior (mean 43.3 9.7 seconds) (Figure 3; Table 1). This was similar to overall trends in depth and speed while not actively engaged in foraging, with average speeds of 1.46 (11) m/s and depths of 5.78 (2) m. Despite tagged whales spending 44% of the time engaged in foraging behavior, a relatively small proportion (7.1%) of the calls originating from tagged whales occurred during these periods. A comparatively higher proportion (27%) of environmental calls coincided with periods of the tagged whale feeding. Calls produced by tagged whales typically occurred towards the conclusion of a feeding event rather than before the start of one, with a mean period of 87.7 ( 9.3) or 210 (1) minutes having elapsed between a tagged whale vocalization and the conclusion or initiation of a tagged whale foraging event, respectively (Figure 3; Table 1). Significant inter-deployment variation was observed in both the speed of the animal at the time of calling and the time elapsed until the next foraging event (p< 0.0001) (Table 1). However, depth at time of calling, the temporal proximity to surfacing behavior and the most recent foraging event were not found to have significant inter-deployment variation (Table 1).
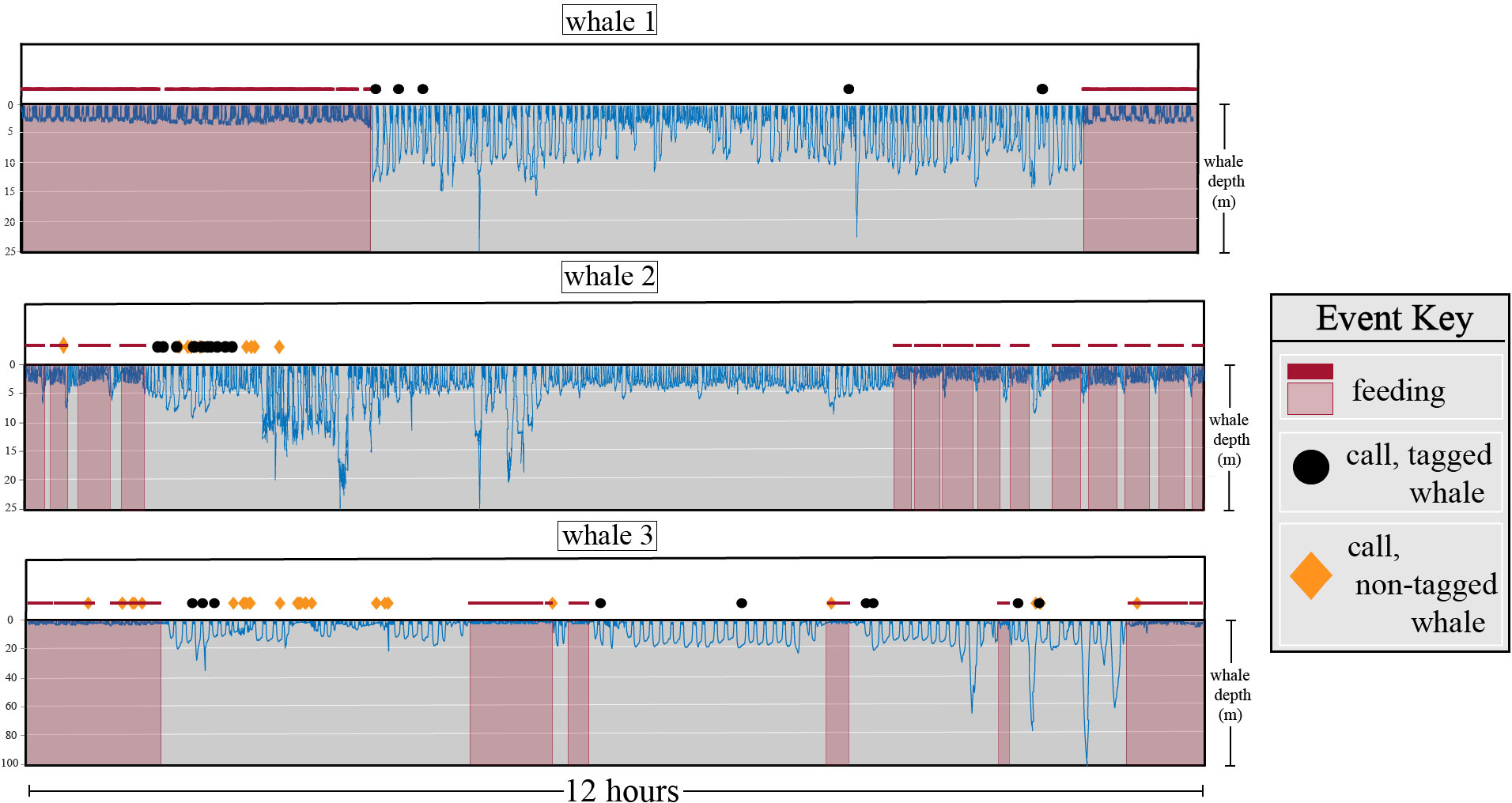
Figure 3 Behavioral context of tagged whale call production from three tag deployments on different individuals. Each deployment example consists of two subplots, with behavioral events (foraging, calling) shown as the top subplot and the depth of whale over time shown on an inverted y-axis as blue lines. Foraging periods are indicated as red-shaded areas and as solid red lines. Calls attributed to the tagged whale are shown as black circles, while calls not attributed to the tagged whale are shown as orange diamonds.
An overall mean hourly calling rate (number of calls produced by one whale per hour) of 0.67 ( 1) calls/hr/whale was calculated from all the deployments, while a weighted mean based on the respective tag’s deployment duration was 0.44 calls/hr/whale. Calling rates were compared to rates reported by relevant studies (see Table 4 in Supplemental Resources) and were generally found to be higher than previously reported.
Discussion
The work presented here is a first look at gray whale acoustic behavior on foraging grounds using animal-borne tags and adds to the few recordings of gray whales during feeding (Stafford et al., 2007; Burnham & Duffus, 2022). It is also an additional application of caller identification via accelerometry in tag-based acoustic data that, with further testing and validation, could revolutionize how individual-level acoustic activity is related to broad-scale population dynamics. Finally, the behavioral context of individual-level call production evaluated in this study has implications regarding our current understanding of the functional use of calling by gray whales, as it provides call-production specific contexts unobservable via remote or static monitoring (i.e., surface-based visual observation and passive acoustic recording).
Acoustic behavior on foraging grounds
The overall mean hourly call rate observed in this study was greater than has been previously reported during migration (Crane & Lashkari, 1996; Guazzo et al., 2017; Guazzo et al., 2019), and on breeding grounds (Dahlheim, 1987) (Supplemental Materials Table 4). This could, in part, be the product of a low sample size and high variation in tag deployment duration. It may also reflect the differences in call type use in this study area compared to those identified for migration and breeding areas. We also observed differences in call structure and usage by type, with Class 2 calls displaying less frequency modulation than previously reported from nearby recording sites (Supplemental Materials Table 3), and Class 4 calls occurring at higher proportions in this study area when compared to observations in other foraging and migration sites (Burnham et al., 2018; Burnham & Duffus, 2022). These differences could result from variation in recording technologies and/or methods used in each study. Previous work by Lopez-Urban et al. (2016) on gray whales in breeding lagoons showed differences in calls recorded via whale-borne tags compared to bottom-stationed systems, which are subject to masking or interference. However, differences in call structures and frequency of use could also be indicative of variation in the predominant behavioral states between the study areas (migration vs. feeding), and/or group-centric variation in calling behavior specific to the Sounders. Collection of additional acoustic data from both bottom-moored and animal-borne systems, complemented with observations, will help to better establish calling rates and changes in call structure over space, time and between gray whale feeding groups.
Accelerometry-based caller identification
Previous studies have demonstrated tagged animal call detection via accelerometry data primarily in fin and blue whales, both of which tend to vocalize well within typical accelerometer sampling rates. It was previously unknown if this method in caller identification could be utilized for gray whales, given their comparatively complex acoustic repertoire (i.e., frequency range utilized, structural complexity, call type usage). However, despite differences in acoustic repertoire compared to other baleen whale species this method had previously been applied to, the similarities in call signatures led us to believe it could be used to discern calls the calls originating from the tagged whale. To start, high SNR gray whale calls were not found to coincide with concurrent accelerometry call signatures in all cases. This is consistent with findings from other baleen whale species and hypotheses regarding call detection via accelerometry as a function of physical vibrations produced by calling animals and not solely SNR (Goldbogen et al., 2014; Stimpert et al., 2020). As noted in Goldbogen et al. (2014), call signatures observed on tagged fin whales were consistently anisotropic amongst the accelerometers three axes. This intra-axis variation in signal intensity is proposed to result from the tag placement relative to the location of the call source (Goldbogen et al., 2014). Exploration of the relationship between received levels of accelerometer call signals, variation of these signals amongst accelerometer axes, and correlation of both to known periods of tag slips (i.e., does a change in call intensity distribution amongst the axes change when a tag moves on the whale’s body), will help elucidate the potential causes of these variations and their utility in interpreting these signals. Future efforts to increase confidence and fully validate this method in tagged animal call identification could benefit from a study design prioritizing the tagging of multiple paired, and calling, animals, or localization via hydrophone array.
Unlike previous studies with fin and blue whales, the accelerometry call signatures observed in this study were structurally dissimilar to the original calls noted on the tag hydrophone (Figure 2). For instance, very rarely (n = 1) were the harmonics of a Class 2 or 3 call observed on both the tag’s hydrophone and accelerometer. This is likely the result of a combination of factors, the first being the fundamental differences in acoustic behavior of the three species this method has been trialled. Both fin and blue whales utilize highly stereotyped call types that are generally low in frequency (<150 Hz) and high in amplitude, which enables strong propagation of these acoustic signals throughout their environment, but especially so in a whale’s near field (Watkins, 1981; Watkins et al., 1987; Stafford et al., 2001; Širović et al., 2007; Miller et al., 2021). Gray whales, in comparison, utilize call types that are more structurally complex, encompass a much larger frequency range, and are produced at lower source levels (Dahlheim, 1987; Guazzo et al., 2017; Burnham et al., 2018). Given this, it is possible that the accelerometry based call signatures observed in this study represent only the fundamental components of the associated full-frequency calls of the recording. Assuming, as Goldbogen et al. (2014) and others have proposed, that these call signatures are the result of physical vibrations produced by the tagged animal during calling, then significant degradation of the call’s structural integrity could occur given the density and viscosity of the propagation medium (body of the whale) compared to the surrounding environmental medium (water). This may mean that the thresholds used to attribute acoustic signals observed in accelerometry data to tagged whales requires refinement on a by-deployment basis, depending on tag placement and complexity of the original call observed. Furthermore, gray whales produce vocalizations, such as Class 1 knocks, which in some cases have fundamental frequencies and harmonic components above this study’s accelerometer sampling capacity. None of the Class 1 calls recorded in this study resulted in associated accelerometry signatures, which could be a product of the limited accelerometer sampling capacity.
While the use of biologging devices capable of higher-resolution accelerometry sampling could enable detection of higher-frequency calls, differences in detection probability between call types could also be accounted for by having a more comprehensive understanding of how often that call type is utilized in the study period and area, and in combination with an evaluation of intra-deployment SNR ratios. We still understand relatively little about the near field propagation of acoustic signals produced by baleen whales and how this varies relative to species morphology and signal characteristics. Also lacking is knowledge on the potential influence of geographical, social, behavioral, and physiological context on the use of calls and calling rates (Burnham & Duffus, 2022). Significantly more testing is required to better understand the considerations and applications of an accelerometry based method in complex caller identification. A well-prepared experiment in a controlled environment prioritizing individual-signal comparisons across varying acoustic collection platforms, combined with further deployments of both animal-borne and remotely placed acoustic recording systems, would greatly improve the application of this method.
Behavioral context of call production by tagged whales
Since calls produced by tagged individuals did not occur during foraging periods at high rates, but rather occurred closer to the end of a feeding event than the beginning of one, it is possible that vocalizing is not directly linked to feeding behavior, but instead may be indicative of shifts in behavioral state (i.e., from foraging to socializing). This could also suggest a behavioral hierarchy where foraging and feeding-associated behaviors (active feeding, moving between feeding areas, etc.) take precedence over social behaviors such as calling or active socializing. Additionally, the relatively shallow depths at which tagged whales were observed to call, and the surficial sediments of the study area could limit call propagation (Hamilton, 1976; Rubano, 1980), implying that the intended receiver(s) of calls emitted must be nearby. However, calling depth may simply be the product of local bathymetric characteristics rather than a product of energetic demands and morphometric characteristics. Similarly, the speeds at which a tagged whale was observed to vocalize could be indicative of a consistent “travel state” at time of calling, as calling speeds were consistent between foraging and non-foraging dives and are comparable to those observed by Guazzo et al. (2017) during migration. Further refinement of the ethogram used and comparison of the tag data to visual observations may highlight any differences, if present, between calling by gray whales in different behavioral states, and refine our understanding of call use during foraging. The characteristics defining a “travel state” or “foraging behavior” could be refined using tag sensors and concurrent surface-based observations to enhance the behavioral ethogram. To effectively investigate the potential function of acoustic activity in gray whales we need a more comprehensive understanding of the tag-measurable or observable mechanical and contextual variables associated with each behavioral state and greater baseline knowledge of ENP gray whale social dynamics within their range and the plasticity or flexibility therein.
In summary, the integration of multiple data collection methods, including animal-borne tags, static passive acoustic hydrophones, and boat-based observations, with further testing of this accelerometry based method in caller identification would significantly expand our current understanding of gray whale acoustic behavior overall, but especially regarding its potential function and role in foraging. The use of animal-borne acoustic tags will be decisive in describing call function and establishing spatiotemporal or population-based trends in acoustic use, which is one of the foremost topics of research for the acoustic community. This would also add to our understanding of the sensitivity of these whales and the potential implications of acoustic disturbance during critical feeding periods.
Data availability statement
The datasets presented in this study can be found in online repositories. The names of the repository/repositories and accession number(s) can be found below: https://purl.stanford.edu/bn098hs8476.
Ethics statement
Ethical review and approval was not required for the animal study because all operations involving tagging of animals were conducted under National Marine Fishery Service permit #16111. Tagging operations were previously reviewed and approved as required by the Animal Welfare Act by an Institutional Animal Care and Use Committee.
Author contributions
HC conceived the study with guidance from JC and DC, and conducted all data analyses and processing, with the exclusion of raw tag data processing, which was initially performed by DC. JC JG, and DC collected the data via tag deployments. Funding support developed by JC and JG. RB assisted HC in the categorization and review of calls based on previously reported call types. HC interpreted results and drafted all manuscript versions. All authors took part in reviewing and editing the manuscript draft prior to submission. All authors contributed to the article and approved the submitted version.
Funding
Support for this work provided in part by Stanford University Terman Fellowship.
Acknowledgments
A special thanks to James Fahlbusch, who performed initial and early tag deployments as well as moral support to the lead author throughout this manuscript’s creation.
Conflict of interest
The authors declare that the research was conducted in the absence of any commercial or financial relationships that could be construed as a potential conflict of interest.
Publisher’s note
All claims expressed in this article are solely those of the authors and do not necessarily represent those of their affiliated organizations, or those of the publisher, the editors and the reviewers. Any product that may be evaluated in this article, or claim that may be made by its manufacturer, is not guaranteed or endorsed by the publisher.
Supplementary material
The Supplementary Material for this article can be found online at: https://www.frontiersin.org/articles/10.3389/fmars.2023.1111666/full#supplementary-material
References
Burnham R., Duffus D. (2020). The use of passive acoustic monitoring as a census tool of gray whale (Eschrichtius robustus) migration. Ocean Coast. Manage. 188, 105070. doi: 10.1016/j.ocecoaman.2019.105070
Burnham R., Duffus D. (2022). A multi-dimensional examination of foraging habitat use by Gray whales using long time-series and acoustics data. Animals 12 (20), 2735. doi: 10.3390/ani12202735
Burnham R., Duffus D., Mouy X. (2018). Gray Whale (Eschrictius robustus) call types recorded during migration off the West coast of Vancouver island. Front. Mar. Sci. 5. doi: 10.3389/fmars.2018.00329
Cade D., Barr K., Calambokidis J., Friedlaender A., Goldbogen J. (2018). Determining forward speed from accelerometer jiggle in aquatic environments. J. Exp. Biol. 221 (2). doi: 10.1242/jeb.170449
Cade D., Gough W., Czapanskiy M., Fahlbusch J., Kahane-Rapport S., Linsky J., et al. (2021). Tools for integrating inertial sensor data with video bio-loggers, including estimation of animal orientation, motion, and position. Anim. Biotelemetry 9 (1). doi: 10.1186/s40317-021-00256-w
Calambokidis J. (2017). Contract Report: Study of seasonal resident gray whales feeding strategy in N. Puget Sound, WA. in 2016. Olympia, WA: Cascadia Research. Prepared for WA: DNR Aquatic Assessment and Monitoring Team.
Calambokidis J., Steiger G., Curtice C., Harrison J., Ferguson M., Becker E., et al. (2015). 4. biologically important areas for selected cetaceans within U.S. waters – West coast region. Aquat. Mammals 41 (1), 39–53. doi: 10.1578/am.41.1.2015.39
Carretta J., Oleson E., Forney K., Muto M., Weller D., Lang A., et al. (2022). U.S. Pacific marine mammal stock assessments: 2021. U.S. Department of Commerce, NOAA Technical Memorandum NMFS-SWFSC-663. p. 158–168. doi: 10.25923/246k-7589
Charles S. (2011). Social context of gray whale Eschrichtius robustus sound activity. Galveston, TX: Masters Thesis, Texas A and M University.
Crane N., Lashkari K. (1996). Sound production of gray whales, eschrichtius robustus, along their migration route: a new approach to signal analysis. J. Acoustical Soc. America 100 (3), 1878–1886. doi: 10.1121/1.416006
Cummings W., Thompson P., Cook R. (1968). Underwater sounds of migrating Gray whales, eschrichtius glaucus (Cope). J. Acoustical Soc. America 44 (5), 1278–1281. doi: 10.1121/1.1911259
Dahlheim M. E. (1987). Bio-acoustics of the gray whale (Eschrichtius robustus). Vancouver, BC: PhD Thesis, University of British Columbia. Available at: https://open.library.ubc.ca/soa/cIRcle/collections/ubctheses/831/items/1.0097975.
Frouin-Mouy H., Tenorio-Hallé L., Thode A., Swartz S., Urbán J. (2020). Using two drones to simultaneously monitor visual and acoustic behaviour of gray whales (Eschrichtius robustus) in Baja California, Mexico. J. Exp. Mar. Biol. Ecol. 525, 151321. doi: 10.1016/j.jembe.2020.151321
Goldbogen J., Stimpert A., DeRuiter S., Calambokidis J., Friedlaender A., Schorr G., et al. (2014). Using accelerometers to determine the calling behavior of tagged baleen whales. J. Exp. Biol. 217 (14), 2449–2455. doi: 10.1242/jeb.103259
Guazzo R., Helble T., D’Spain G., Weller D., Wiggins S., Hildebrand J. (2017). Migratory behavior of eastern north pacific gray whales tracked using a hydrophone array. PLoS One 12 (10), e0185585. doi: 10.1371/journal.pone.0185585
Guazzo R., Weller D., Europe H., Durban J., D’Spain G., Hildebrand J. (2019). Migrating eastern north pacific gray whale call and blow rates estimated from acoustic recordings, infrared camera video, and visual sightings. Sci. Rep. 9 (1), 4119–4129. doi: 10.1038/s41598-019-49115-y
Hamilton E. (1976). Variations of density and porosity with depth in deep-sea sediments. J. Sediment. Petrol. 46 (2), 280–300. doi: 10.1306/212F6F3C-2B24-11D7-8648000102C1865D
Küsel E., Mellinger D., Thomas L., Marques T., Moretti D., Ward J. (2011). Cetacean population density estimation from single fixed sensors using passive acoustics. J. Acoustical Soc. America 129 (6), 3610–3622. doi: 10.1121/1.3583504
Lewis L., Calambokidis J., Stimpert A., Fahlbusch J., Friedlaender A. S., McKenna M., et al. (2018). Context-dependent variability in blue whale acoustic behaviour. R. Soc. Open Sci. 5 (8), 180241. doi: 10.1098/rsos.180241
Lopez-Urban A., Thode A., Buzua Duran C., Urban-R J., Swartz S. (2016). Two new grey whale call types detected on bioacoustic tags. J. Mar. Biol. Assoc. United Kingdom 98 (5), 1–7. doi: 10.1017/S0025315416001697
Marques T., Thomas L., Martin S., Mellinger D., Ward J., Moretti D., et al. (2012). Estimating animal population density using passive acoustics. Biol. Rev. 88 (2), 287–309. doi: 10.1111/brv.12001
Mellinger D., Stafford K., Moore S., Dziak R., Matsumoto H. (2007). An overview of fixed passive acoustic observation methods for cetaceans. Oceanography 20 (4), 36–45. doi: 10.5670/oceanog.2007.03
Miller B., Calderan S., Leaper R., Miller E., Širović A., Stafford K., et al. (2021). Source level of antarctic blue and fin whale sounds recorded on sonobuoys deployed in the deep-ocean off antarctica. Front. Mar. Sci. 8. doi: 10.3389/fmars.2021.792651
Oestreich W., Fahlbusch J., Cade D., Calambokidis J., Margolina T., Joseph J., et al. (2020). Animal-borne metrics enable acoustic detection of blue whale migration. Curr. Biol. 30 (23), 4773–4779.e3. doi: 10.1016/j.cub.2020.08.105
Ollervides F. (2001). Gray whales and boat traffic: Movement, vocal, and behavioral responses in Bahia Magdalena, Mexico, Galveston, TX: PhD Thesis, Texas A and M University.
Ponce D., Thode A., Guerra M., Urbán J., Swartz S. (2012). Relationship between visual counts and call detection rates of gray whales (Eschrichtius robustus) in Laguna San ignacio, Mexico. J. Acoustical Soc. America 131 (4), 2700–2713. doi: 10.1121/1.3689851
Pruitt C., Donoghue C. (2016). Technical Report: Ghost shrimp: commercial harvest and gray whale feeding, North Puget Sound, WA. Olympia, WA: DNR Aquatic Assessment and Monitoring Team. Available at: https://www.dnr.wa.gov/publications/aqr_aamt_shrimp_whale_study_2015.pdf.
Rannankari L., Burnham R., Duffus D. (2018). Diurnal and seasonal acoustic trends in northward migrating Eastern pacific Gray whales (Eschrichtius robustus). Aquat. Mammals 44 (1), 1–6. doi: 10.1578/am.44.1.2018.1
Rubano L. (1980). Acoustic propagation in shallow water over a low-velocity bottom. J. Acoust. Soc. Am. 67 (5), 1608–1613. doi: 10.1121/1.384336
Rutz C., Hays G. (2009). New frontiers in biologging science. Biol. Lett. 5 (3), 289–292. doi: 10.1098/rsbl.2009.0089
Saddler M., Bocconcelli A., Hickmott L., Chiang G., Landea-Briones R., Bahamonde P., et al. (2017). Characterizing Chilean blue whale vocalizations with DTAGs: a test of using tag accelerometers for caller identification. J. Exp. Biol. 220 (22), 4119–4129. doi: 10.1242/jeb.151498
Širović A., Hildebrand J., Wiggins S. (2007). Blue and fin whale call source levels and propagation range in the Southern Ocean. J. Acoust. Soc. Am. 122 (2), 1208–1215. doi: 10.1121/1.2749452
Stafford K., Moore S., Spillane M., Wiggins S. (2007). Gray Whale calls recorded near Barrow, Alaska, throughout the winter of 2003–04. ARCTIC 60 (2). doi: 10.14430/arctic241
Stafford K., Nieukirk S., Fox C. (2001). Geographic and seasonal variation of blue whale calls in the north pacific. J. Cetacean Res. Manage. 3 (1), 65–76. doi: 10.47536/jcrm.v3i1.902
Stevenson B., Dam-Bates P., Young C., Measey J. (2020). A spatial capture–recapture model to estimate call rate and population density from passive acoustic surveys. Methods Ecol. Evol. 12 (3), 432–442. doi: 10.1111/2041-210x.13522
Stimpert A., DeRuiter S., Falcone E., Joseph J., Douglas A., Moretti D., et al. (2015). Sound production and associated behavior of tagged fin whales (Balaenoptera physalus) in the southern California bight. Anim. Biotelemetry 3 (1). doi: 10.1186/s40317-015-0058-3
Stimpert A., Lammers M., Pack A., Au W. (2020). Variations in received levels on a sound and movement tag on a singing humpback whale: implications for caller identification. J. Acoust. Soc. Am. 147 (5), 3684–3690. doi: 10.1121/10.0001306
Watkins W. (1981). Activities and underwater sounds of fin whales. Sci. Rep. Whales Res. Inst. 33, 83–117. doi: 10.1016/0198-0254(82)90294-1
Watkins W., Tyack P., Moore K., Bird J. (1987). The 20-Hz signals of finback whales (Balaenoptera physalus). J. Acoustical Soc. America 82 (6), 1901–1912. doi: 10.1121/1.395685
Weitkamp L., Wissmar R., Simenstad C., Fresh K., Odell J. (1992). Gray Whale foraging on ghost shrimp (Callianassa californiensis) in littoral sand flats of puget sound, U.S.A. Can. J. Zoology 70 (11), 2275–2280. doi: 10.1139/z92-304
Wisdom S., Bowles A. E., Anderson K. E. (2001). Development of behavior and sound repertoire of a rehabilitating gray whale calf. Aquat. Mamm. 27, 239–255.
Keywords: gray whale, biologging, acoustics, passive acoustic monitoring, foraging, caller identification
Citation: Clayton H, Cade DE, Burnham R, Calambokidis J and Goldbogen J (2023) Acoustic behavior of gray whales tagged with biologging devices on foraging grounds. Front. Mar. Sci. 10:1111666. doi: 10.3389/fmars.2023.1111666
Received: 29 November 2022; Accepted: 20 June 2023;
Published: 12 July 2023.
Edited by:
Mark Jessopp, University College Cork, IrelandReviewed by:
Ailbhe Kavanagh, Marine Institute, IrelandIsrael Maciel, State University of Rio de Janeiro, Brazil
Copyright © 2023 Clayton, Cade, Burnham, Calambokidis and Goldbogen. This is an open-access article distributed under the terms of the Creative Commons Attribution License (CC BY). The use, distribution or reproduction in other forums is permitted, provided the original author(s) and the copyright owner(s) are credited and that the original publication in this journal is cited, in accordance with accepted academic practice. No use, distribution or reproduction is permitted which does not comply with these terms.
*Correspondence: Hannah Clayton, aGNsYXl0b24xQHN0YW5mb3JkLmVkdQ==