- 1Key Laboratory of Marine Ranching, Ministry of Agriculture and Rural Affairs, Guangzhou, China
- 2Frontiers Science Center for Deep Ocean Multispheres and Earth System and Key Laboratory of Mariculture, Ministry of Education, Ocean University of China, Qingdao, China
- 3South China Sea Fisheries Research Institute, Chinese Academy of Fisheries Sciences, Guangzhou, China
- 4Laboratory for Marine Fisheries Science and Food Production Processes, Pilot National Laboratory for Marine Science and Technology, Qingdao, China
Chub mackerel (Scomber japonicus) is widely distributed in the northwestern Pacific Ocean and is utilized by China, Korea, and Japan in different seasons and areas. Generally, chub mackerel is divided into Tsushima Warm Current (TWC) and Pacific stocks based on its different distribution, migration, and life-history traits. The two stocks showed obvious interannual to decadal variability in abundance with evident different variation patterns. Chub mackerel has extensive spawning areas in the northwestern Pacific Ocean, with the main spawning period from March to June, and the suitable spawning SST (sea surface temperature) ranging from 15°C to 22°C. To explore the temperature effects on different variations of the two stocks, we estimated the average temperature suitability index (TSI) for chub mackerel in main spawning areas during the spawning period from March to June based on the relationship between the probability of spawning and the specific SST. Results showed that the TSIs in the shelf waters of China seas and the waters of the Tsushima Strait displayed simultaneous abrupt changes in the late 1990s for the TWC stock, whereas the TSI in the Pacific coast of Japan had a regime shift in the late 1970s for the Pacific stock. The spatio-temporal variation in spawning temperature suitability may have had an important impact on the recovery of the Pacific stock after the 2000s. We also found that there is a non-stationary relationship between chub mackerel abundance and TSI, which is driven by climate variabilities such as the Siberian High and the Aleutian Low. Moreover, TWC stock showed non-stationary relationship with TSI, and the threshold years was identified in the 1990s, and the non-stationary relationship between TSI and Pacific stock, with thresholds occurring in the late 1970s. Our research provides a reasonable explanation for the variations in the two stocks and their responses to TSI variability and offers an improved understanding of the climate-induced non-stationary relationships between chub mackerel abundance and physical drivers.
Introduction
Chub mackerel (Scomber japonicus) is a circumglobal pelagic fish (Hernández and Ortega, 2000) that is divided into two stocks (i.e., Tsushima Warm Current (TWC) (Table S1) stock and Pacific stock) in the northwestern Pacific Ocean (Shiraishi et al., 2008; Shiraishi et al., 2009; Yasuda et al., 2014). The TWC stock is mainly distributed in the Yellow Sea, East China Sea, and the Sea of Japan, and the Pacific stock inhabits the Pacific coast of Japan and the high seas of northwestern Pacific Ocean (Shiraishi et al., 2008; Kurota et al., 2018; Yukami et al., 2018). Chub mackerel is mainly utilized by China, Korea, and Japan in the northwestern Pacific Ocean, and these countries harvest it in different seasons and areas (Chen et al., 2009; Kurota et al., 2018; Lee et al., 2018; Yu et al., 2018). China mainly fish chub mackerel in the East China Sea and Yellow Sea, and it belongs to the TWC stock that spawns in the southern part of the East China Sea and the surrounding waters of Tsushima Strait (Shiraishi et al., 2008; Kurota et al., 2018). Similarly, the species captured in the South Korean seas by Korea also belongs to TWC stock (Lee et al., 2018). Japan has a long history of fishing both stocks of chub mackerel, and which is harvested in the East China Sea, the Sea of Japan, and the Pacific coast off central and northern Japan (Kurota et al., 2018; Yukami et al., 2018). Therefore, chub mackerel catches by Korea and Japan were divided into TWC and Pacific catches in the Japanese stock assessment report by the Fisheries Agency of Japan, but it did not include the Chinese catch (Kurota et al., 2018; Yukami et al., 2018). The catches of different countries or stocks had evident interannual to decadal variability but showed different patterns of variation (Figure 1). Mechanisms for variations in chub mackerel abundance of different stocks have not been suitably addressed since the 1970s.
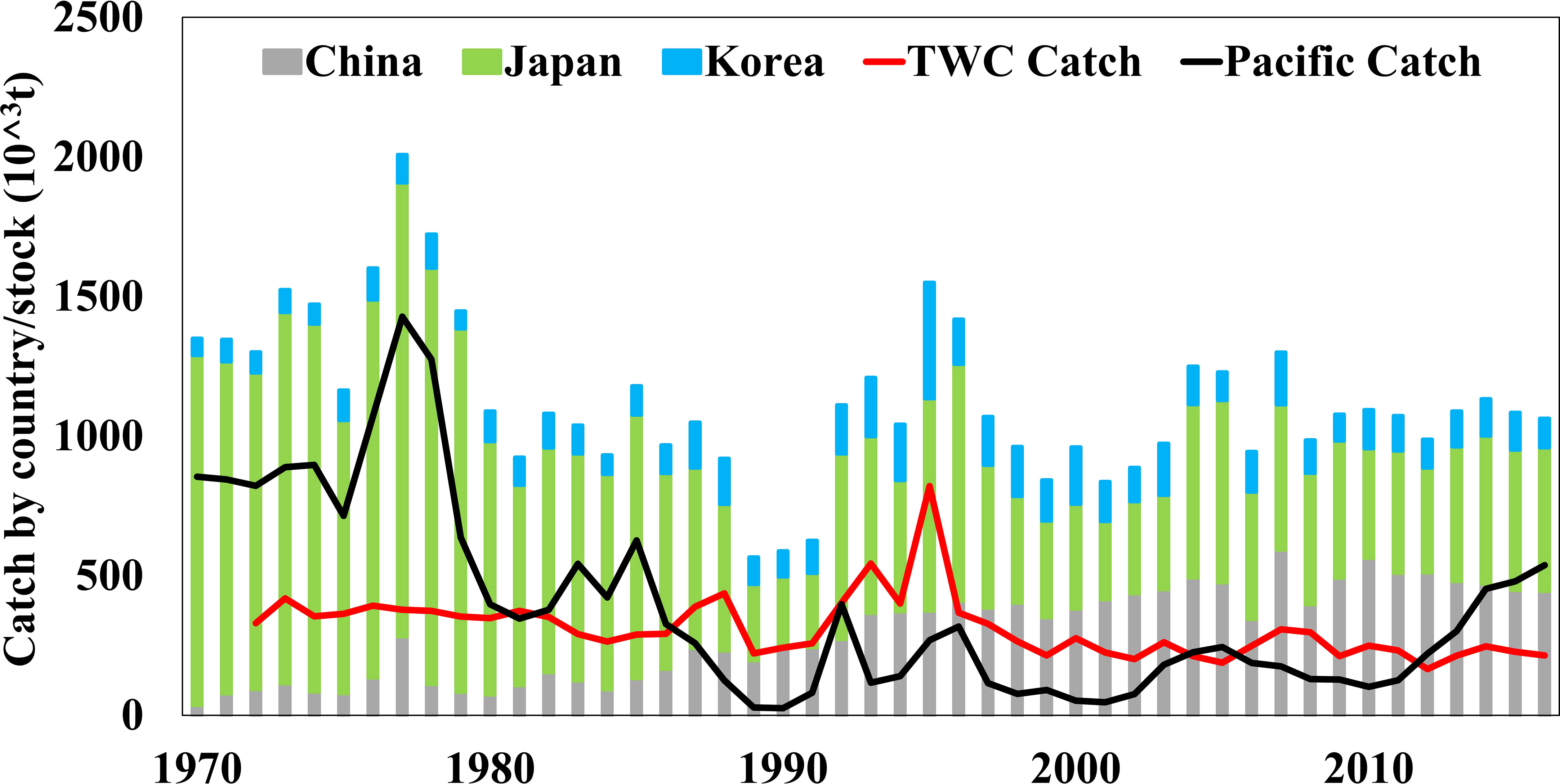
Figure 1 Variations in annual catches of chub mackerel by countries/stocks from 1970 to 2017 (FAO-FIGIS, 2018; Kurota et al., 2018; Yukami et al., 2018).
In the northwestern Pacific Ocean, the division of stocks is primarily based on differences in patterns of migratory route and spawning grounds. Main spawning areas of chub mackerel are located in the coastal waters of east Asian marginal seas and the Pacific coast of Japan (Li J. S. et al., 2014; Li Y. S. et al., 2014; Li et al., 2015a; Li et al., 2015b; Kurota et al., 2018; Yukami et al., 2018; Sogawa et al., 2019) (Figure 2). Previous studies have shown that a warmer SST in spring are conducive to earlier migratory of marine fish to spawning grounds (Sims et al., 2004). In other words, temperature is a determinant of spawning migrations (Sims et al., 2004; Wang et al., 2021). For chub mackerel, laboratory experiments have suggested that there is a significant effect of temperature on metabolic costs, reproduction, and mortality (Schaefer, 1986; Dickson et al., 2002), and water temperature during the spawning period can affect recruitment (the biomass of adult, eggs, and larvae) (Yatsu et al., 2005; Kurota et al., 2018; Yukami et al., 2018), further affecting the variation in chub mackerel abundance. Previous research demonstrated that 15°C to 22°C is the suitable spawning SST for chub mackerel, 18°C is the optimal SST, and the main period is from March to June (Takasuka et al., 2008; Yukami et al., 2009). Based on the long-term dataset of monthly egg and larval surveys on the Pacific coast of Japan, Takasuka et al. (2008) reported that the probability of egg density of chub mackerel followed a gaussian distribution with SST. This means that the variation in spawning activity or egg survival may conform to a normal distribution (Gaussian shape) when the temperature is within a certain interval. Previous studies had focused on the effect of SST on spawning, and it is difficult to quantify the specific effects of the increases/decreases in temperature on physiological mechanisms, which is a major challenge for resource forecasting or fishery management. Relatively few previous research constructs indices incorporating physiological information quantitively and evaluates the effectiveness of the incorporation. In this study, we use a temperature suitability index (TSI) that was based on the relationship between spawning probability density and SST, to explore climate-environment-biology relationships.
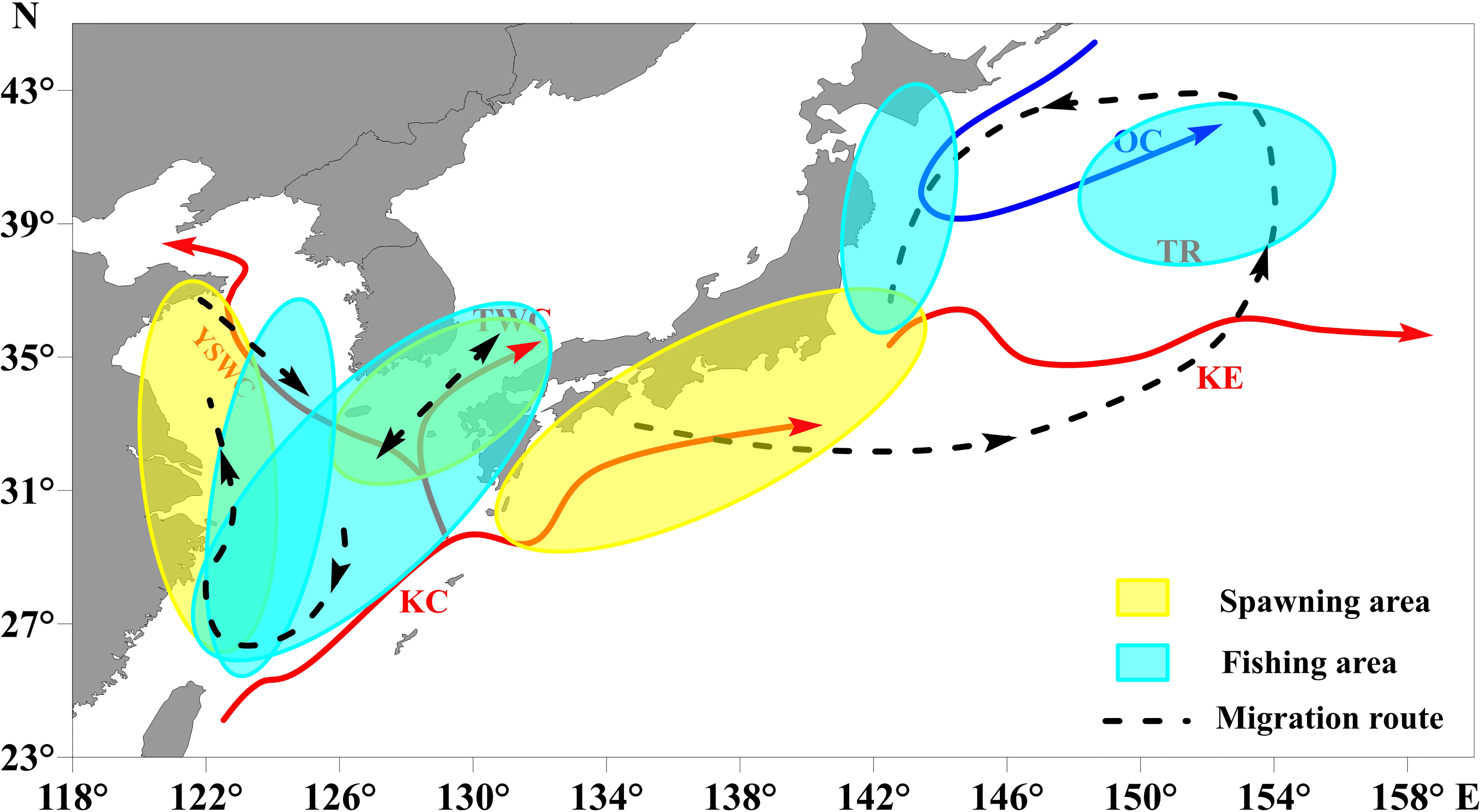
Figure 2 Schematic diagram showing main spawning areas, fishing areas, and migration route of chub mackerel and the oceanographic structure in the northwest Pacific Ocean. (YSWC, Yellow Sea Warm Current; TWC, Tsushima Warm Current; KC, Kuroshio Current; KE, Kuroshio Extension; TR, Transition Region; OC, Oyashio Current). Red and blue solid lines represent the warm and cold currents, respectively. Yellow and blue shaded areas indicate the main spawning and fishing areas of chub mackerel, respectively, and dotted lines with arrows represent their migration route.
Numerous studies have highlighted that the variation in both physical and biological time series were nonlinear. Litzow et al. (2018; 2019) presented relationships between the physical environment and biological community characterized by time-dependent non-stationarity in the northeastern North Pacific. Ma et al. (2020; 2021) revealed nonlinearity and non-stationarity in the pelagic communities and ecosystems were driven by climate variability in the northwestern North Pacific. It is widely acknowledged that climate variability can affect marine ecosystems by altering the local oceanographic environment (Overland et al., 2010; Cheung et al., 2015) but different regions can be affected by varied climate patterns (i.e. Aleutian Low, Siberian High). Relevant studies in the North Pacific indicated that the Aleutian low-pressure system was of great importance to the variability in regional climate, oceangraphic conditions and ecosystem (Di Lorenzo and Ohman, 2013). Additionally, the non-stationarity in the northeastern North Pacific has been attributed to the Aleutian Low-forced variability in the relative importance of Pacific Decadal Oscillation (PDO) and North Pacific Gyre Oscillation (NPGO) to the regional oceanographic environment variability (Mantua and Hare, 2002; Mantua, 2004; Litzow et al., 2018). In the northwestern North Pacific, the regional oceanographic conditions are to a large extent influenced by East Asian Monsoon Index (MOI), Siberian High Index (SHI), and Arctic Oscillation Index (AOI), (Jung et al., 2017; Liu et al., 2019; Ma et al., 2020). Previous studies showed that the non-stationary relationship between the pelagic community and physical drivers in the Tsushima and Pacific areas resulted from the declined variances in the Siberian High and Aleutian Low, respectively (Ma et al., 2021). However, the mechanisms of climate-induced non-stationarity on species or even stock level have not been evaluated in the northwestern Pacific Ocean.
Based on the above considerations, we conducted a study to explore the variation in the different stocks of chub mackerel, with a specific focus on the main spawning areas and seasons. We investigated their non-stationary relationships with physical drivers in the northwestern Pacific Ocean. To this end, the biological data of TWC stock (i.e., Chinese catch, TWC catch, biomass, and recruitment) and Pacific stock (i.e., Pacific catch, biomass, and recruitment), as well as SST and climatic indices, were integrated into this comprehensive research. The diverse analytical techniques were applied in our study, which have varying capacities in dealing with complex data sets. The main purpose of this study is to determine thresholds and non-stationarity in the biology-environment relationships of different chub mackerel stocks, and their responses to climate variability. In addition, we explore the variation patterns in different stocks and temperature suitability of the main spawning areas, and then analyze the main relationships between them.
Materials and methods
Catch data
Chub mackerel is mainly used by China, Korea, and Japan in the northwestern Pacific Ocean, and the Russia caught it along the northwest Pacific coast and the high seas, but the duration is short and the catch is much lower than that of China and Japan. Therefore, we apply the data mainly from China, Japan and Korea for subsequent analysis. The catch data of China, Japan, Korea, and Chinese Taipei for the period of 1950–2017 were derived from Food and Agriculture Organization of the United Nations (FAO) (FAO-FIGIS, 2018). Correlation analysis showed that there were significant correlations among the catches of China, Korea, and Chinese Taipei except for the Japanese catch (Figure S1). Additionally, chub mackerel was divided into TWC stock and Pacific stock by the Fisheries Agency of Japan (Table S2) and the catches were attributed to TWC catch (1973-2017) and Pacific catch (1970-2017). The data were extracted from the Resource Assessment Report of chub mackerel by the Japanese Fisheries Agency (Kurota et al., 2018; Yukami et al., 2018). According to the TWC catch and Pacific catch, the annual recruitment and biomass of TWC and Pacific stocks were assessed, and we also extracted the data from the Japanese stock assessment report (Kurota et al., 2018; Yukami et al., 2018). It should be noted that the Fisheries Agency of Japan only included the catch of Korea and Japan, which did not calculate the Chinese catch that also belongs to TWC stock. Therefore, in this study, we used the Chinese catch and TWC catch to represent the abundance of TWC stock, and the Pacific catch to represent the abundance of Pacific stock. The time series of all fishery data is from 1970 to 2017. The annual biomass and recruitment of TWC and Pacific stocks were also used to analyze the variations in chub mackerel abundance in the northwestern Pacific Ocean.
SST data and climatic indices
We complied monthly SST gridded data between 0°–60°N and 100°–180°E for the period of 1970–2017, which were obtained from three different sources because of a lack of any single dataset covering the entire period. The SST data for 1970–2011 with a resolution of 1° (latitude × longitude) were gained from Japan Meteorological Agency (JMA) (http://www.jma.go.jp/jma/index.html). The SST data for 2012–2015 and 2016-2017 were extracted from the Advanced Very High-Resolution Radiometer (AVHRR), Geostationary Operational Environmental Satellites (GOES), respectively. (http://oceanwatch.pifsc.noaa.gov/thredds/catalog.html). The AVHRR and GOES data were averaged into the resolution of 1° (latitude × longitude) by taking their arithmetic mean, which was to ensure that the spatial resolution of data was consistent. Then, we used the JMA data to cover the period from 1970 to 2011, the AVHRR data to cover the period from 2012 to 2015, and the GOES data for 2016 to 2017. In this study, we focused on exploring the effects of temperature on chub mackerel during the spawning period. Therefore, the average of spawning period (from March to June) in each SST grid were calculated for further analyses.
The Pacific Decadal Oscillation index (PDO), Southern Oscillation Index (SOI), Arctic Oscillation Index (AOI), Monsoon Index (MOI), North Pacific Index (NPI), Siberian High Index (SHI), and North Pacific Gyre Oscillation (NPGO) were chosen as the major mode of climate variability in the northwestern Pacific Ocean. These indices were well documented and largely associated with the variability in the northwestern Pacific Ocean ecosystem and fishery stocks (Overland et al., 2008; Tian et al., 2008; Jung et al., 2017; Ma et al., 2019; Liu et al., 2019; Ma et al., 2020). All climatic indices were derived from open-access online databases and have a monthly temporal scale for the period 1970–2017. Annual SOI is defined as the average from May to next April, which has significant correlation with winter SST in the northwestern Pacific Ocean (Tian et al., 2003). In this study, the winter PDO, AOI, MOI, NPI, SHI, and NPGO indices were the average from the previous December to February, and this period had the most significant climate change in the northwestern Pacific Ocean (Overland et al., 2008; Ma et al., 2019; Ma et al., 2020). The detailed climatic indices are provided in Supplementary Table S3.
Estimation of spawning TSI for different spawning grounds
SST is the most crucial factor for early stage (i.e. spawning and larval survival) of chub mackerel with a suitable spawning SST ranging from 15°C to 22°C and an optimal SST at 18°C (Takasuka et al., 2008; Yukami et al., 2009; Wang et al., 2021). In this study, we developed a spawning temperature suitability index (TSI) that represented the spawning probability at a specific SST. Based on the suitable and optimal spawning SST noted above, we assumed TSI at SST t to be normally distributed as (Liu et al., 2019; Wang et al., 2021; Ma et al., 2022) (Figure S2):
where μ is the mean SST equal to 18°C, σ is the variance equal to 2.0°C, and TSI has approximately 95% of the area being within 14°C to 22°C. Based on equation 1, we convert the SST to TSI for further analysis.
Environmental variables can influence abundance in different ways across the geographic extent of a species’ reproductive range (Planque and Frédou, 1999). Therefore, we calculated correlations between annual catches (i.e., Chinese catch, TWC catch, and Pacific catch) and average TSI at each 1×1° grid point during the spawning period and across all years from 1970–2017. During the spawning period, the TSI near the coastal waters of China seas, the southern part of the Sea of Japan (including the Tsushima strait), and the Pacific coast of Japan were significantly correlated with catches (Figure S3). Alongside the results of the correlation mapping and previous studies about the life history of chub mackerel (Shiraishi et al, 2008; Shiraishi et al, 2009; Li J. S. et al., 2014; Li Y. S. et al., 2014; Li et al., 2015a; Li et al., 2015b; Kurota et al., 2018; Yukami et al., 2018; Sogawa et al., 2019), this suggests the main spawning areas are located in the Chinese coastal waters, surrounding waters of the Tsushima Strait, and the Pacific coast of Japan. In other words, we defined the specific areas 24°N-36°N/118°E-124°E, 31°N-36°N/126°E-132°E and 30°N-38°N/129°E-147°E as the spawning grounds, with some areas excluded (Figure 2). According to the main spawning areas that were plotted in Figure 2, we calculated the average TSI of the coastal waters of China seas, the southern part of the Sea of Japan and the Pacific coast of Japan during the period March to June (hereafter referred to as ESTSI, JSTSI, and NSTSI).
Data analyses
The empirical orthogonal function (EOF) has been widely used in atmospheric, oceanographic, and biological studies serving as an effective rank-reduction technique (Litzow et al., 2018). For any spatio-temporal field data, EOF analysis finds a set of orthogonal spatial modes and a set of uncorrelated time coefficients (Hannachi et al., 2007). Briefly, the spatial modes represent the association of each grid to the trend, and the time coefficients represent the dominant trend of the spatio-temporal field data. In this study, EOF analysis is used to identify the most important TSI variability pattern in the northwestern Pacific Ocean (Weare and Nasstrom, 1982; Ma et al., 2020). We calculated spatial modes and time coefficients (shorted as EOFs) and retained the first four principal components for each to focus on the dominant variability patterns of TSI.
Correlation analyses were used to explore the relationships between chub mackerel abundance, regional TSI, EOFs, and climatic indices. And, we conducted cross-correlation analyses with time lags of 0-2 years between abundance and physical drivers. To investigate the correlation between the TSI field and the climate indices, we mapped the distribution of their correlation coefficients. The correlation coefficient of each point of 1 (latitude × longitude) resolution was calculated by correlation analysis between the TSI at this point and the climate indices for the period 1970–2017.
We applied the sequential t-test analysis of regime shifts (STARS) to detect trends and step changes within the time series data (chub mackerel abundance, regional TSI, EOFs, climatic indices) (Rodionov, 2004). STARS results are determined by the cut-off length for proposed regimes (L), and the Huber weight parameter (H), is defined by the range of departure from the observed mean beyond which observations are considered outliers. By exploratory analyses with STARS, L was set to 10, and H to 1, with a significant level of 5%. We calculated anomalies by dividing each data point by the mean of the entire time series.
Gradient Forest (GF) analysis was employed to evaluate the contributions of climatic (i.e., PDO, SOI, AOI, MOI, NPI, SHI, and NPGO) and environmental variability (i.e., ESTSI, JSTSI, NSTSI, and EOFs), which included the time lags of 0-2 years, to chub mackerel abundance (Ellis et al., 2012; Wang et al., 2021). Gradient forest analysis was built upon random forest models to capture complex relationships between potentially correlated explanatory variables and multiple response variables by integrating individual random forest analyses over the different response variables (Ellis et al., 2012). Essentially, random forests are regression trees that partition the response variable into two groups at a specific split value for each explanatory variable to maximize homogeneity. An independent bootstrap sample of data is used to fit each tree and the data not selected in the bootstrap sample (i.e., out-of-bag OOB data) are used to provide across-validated estimate of the generalization error. Random forests provide three measures that we use to investigate response: the goodness-of-fit measure R2f for the forest for each response f, the accuracy importance Ifp for explanatory variables p within the forest, and the importance weighted by Rf2. In this study, we ran the gradient forests 1000 times to obtain the variability of Rf2. The run with the highest overall performance was then used to other statistics.
GF cannot fit clear curves between independent variables and dependent variables. Therefore, it provides some basis for further analysis using Generalized additive models (GAM) and threshold generalized additive models (TGAM), and then analyzes the characteristics of their non-stationary, as well as the characteristics of variations in different periods, and the more obvious differences in the influence mechanisms of stocks. Both GAM and TGAM were applied to identify the types of relationships (stationary or non-stationary) between chub mackerel abundance (biological responses) and regional TSI/EOFs (physical drivers), and we also considered the effect of time lags with 0-2 years. The GAM is a nonparametric generalized linear regression method, a generalization of the GLM model, in which each additive term in the model is estimated using a single smooth function (i.e. spline smoothing function, kernel function and local regression smooth function), and each additive term can explain the causal-variable relationship between the dependent and independent variables. With reference to previous studies, the spline smoothing function is usually selected (Litzow et al., 2018; Ma et al., 2020; Ma et al., 2021). Where α is the intercept term and ϵ is the random error term that obeys a normal distribution, that is the residuals, and which is independent of the explanatory variables. A “stationary” relationship is described by a single function throughout the entire period and the stationary hypothesis was formulated as follows using a GAM (Ciannelli et al., 2004):
Where Y is the response variable chub mackerel abundance, X are the predictors (regional TSI and EOFs), and s, α, and ϵ are smooth function (with k ≤ 3 to avoid over-fitting), intercept, and error terms, respectively. By contrast, a “non-stationary” relationship is described by two (or more) functions during different periods with biological responses to physical drivers changing abruptly over a threshold year (Litzow et al., 2018). The non-stationary relationship is formulated by a TGAM (Puerta et al., 2019):
The threshold year (y) that separates different periods with different responses to the driver was sought by running TGAM for all possible threshold values (years in the time series) between the 0.1 lower and the 0.9 upper quantiles (number of threshold years tested depended on the length of the time series) and selected by minimizing the generalized cross-validation score (GCV) of the model (Casini et al., 2009). To compare the fitness of stationary (GAMs) and non-stationary (TGAMs) models, the “genuine” cross-validation squared prediction error (gCV) is computed, which accounts for the estimation of the threshold line and the estimation of the degrees of freedom for the functions appearing in all stationary and non-stationary formulations (Ciannelli et al., 2004).
Results
Abundance, TSI and climate variability
For TWC stock, since the 1970s, the Chinese catch showed an increasing trend, and the catch began to stabilize after 2000, with step changes occurring in 1986/1987, 1993/1994, and 2003/2004. In addition, the TWC catch by Korea and Japan decreased around the late 1990s with a step-change in 1998/1999. Concerning Pacific stock, the Pacific catch had dramatic decreases in the late 1970s and 1980s and began to recover after the 2000s, with step changes in 1979/1980, 1988/1989, and 2014/2015 (Figure 3). The biomass (r = 0.77, p< 0.01) and recruitment (r = 0.56, p< 0.01) of TWC stocks had significant correlations with the catch. Simultaneously, the Pacific catch had significant correlation with the biomass (r = 0.84, p< 0.01) and recruitment (r = 0.56, p< 0.01). The step changes in TWC biomass and recruitment took place in 1996/1997 and 1997/1998, respectively. Pacific biomass and recruitment underwent step changes in the late 1970s, late 1980s, and 2010s, which had a similar variation pattern with Pacific catch (Figure 3).
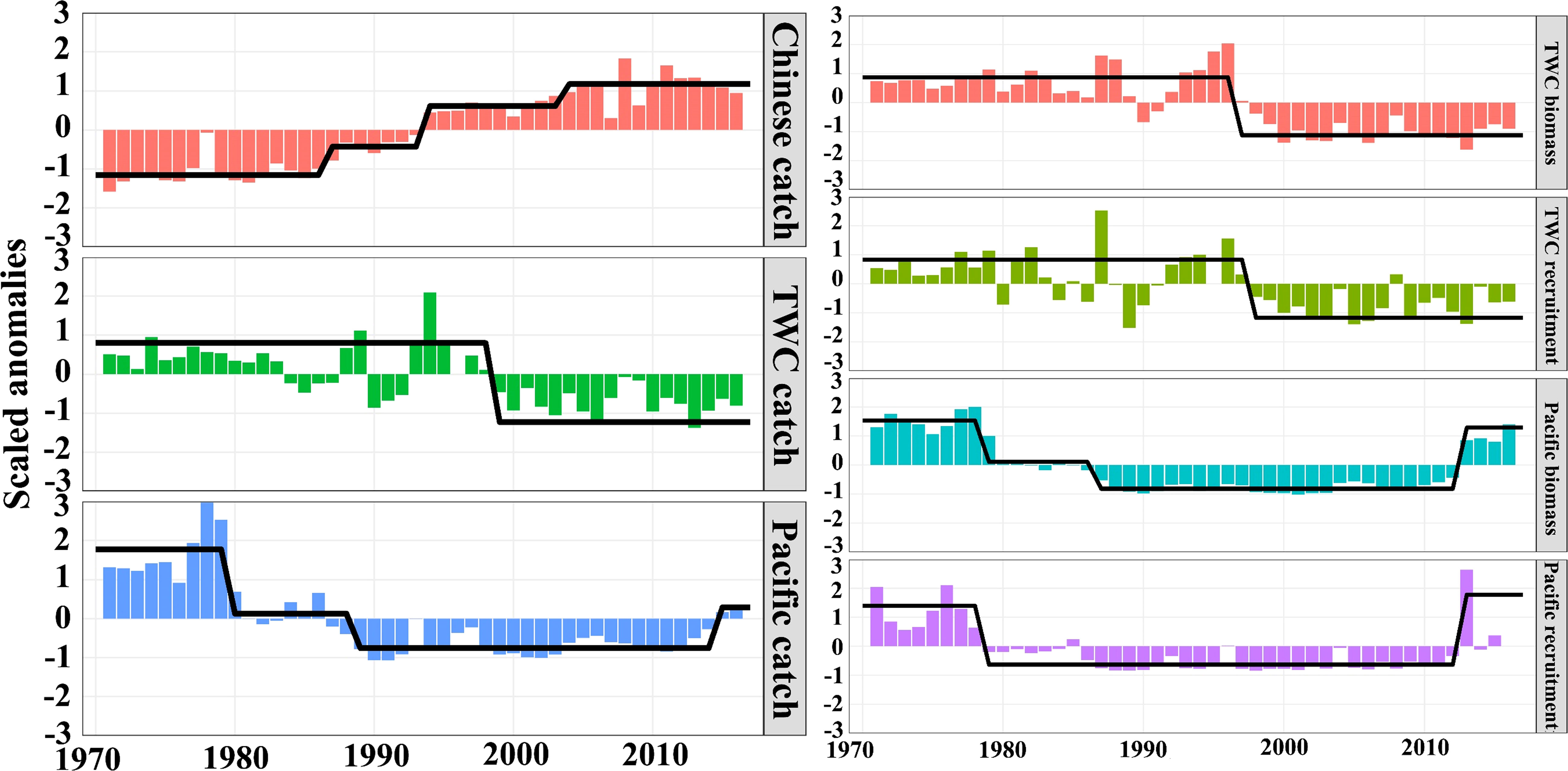
Figure 3 Left column shows the step changes of the catch (i.e., Chinese catch, TWC catch, and Pacific catch). The right column shows the step changes in biomass and recruitment. The bars indicate anomalies and the black lines represent regime means detected by the STARS.
The ESTSI, JSTSI, and NSTSI all had a clear long-term trend of decrease during 1970-2017 (Figure 4). Both the ESTSI and JSTSI showed step changes in the late 1990s and 2010s, and the NSTSI exhibited step-change in 1979/1980 and 2011/2012. In addition, the spatial modes and time coefficients for EOFs of the TSI were calculated in the northwestern Pacific Ocean, and the first four EOFs have an explanation of 43.10% of the total variance in TSI (Figures S4, 4). The spatial model of EOF1 indicated that the TSI in the northwestern Pacific Ocean had opposite loading on the two sides of the Kuroshio axis, the TSI of TWC and Pacific areas showed a spatial difference, and the spatial mode of EOF2 mainly represented TSI variation in the surrounding waters near Kuroshio axis. Additionally, time coefficients of EOF1 showed large interannual fluctuations with a step-like change in 2014/2015. Time coefficients of EOF2 had regime shifts in the late 1970s and 2011/2012. Time coefficients of EOF3 showed an abrupt increase in the late 1980s with a regime shift in 1987/1988, and the other regime shifts occurred in 1993/1994, 2004/2005, and 2014/2015. Time coefficients of EOF4 decreased in the early 2000s but increased in the 2010s with a step-change in 2014/2015.
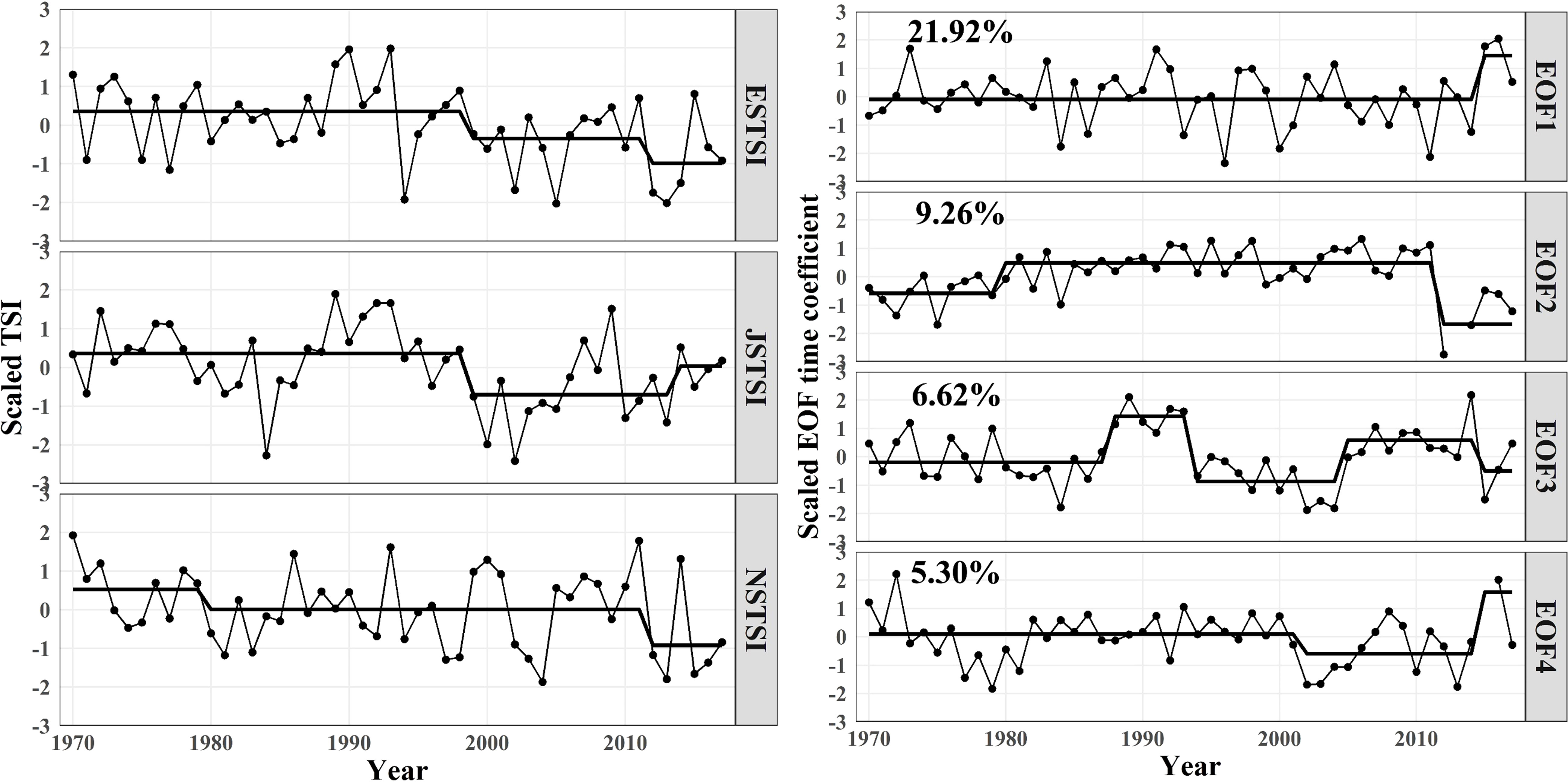
Figure 4 Trajectories in the average TSI of the coastal waters of China seas, the southern part of the Sea of Japan, and the Pacific coast of Japan during 1970-2017 (left panels) and the first four modes of the EOFs. Numbers indicate the percentage of variance explained by each EOF. Black lines represent regime means calculated by the STARS (right panels).
The climatic indices showed decadal variability (Figure S5). The PDO showed step changes in 1976/1977 and 1988/1989, while the NPI had a regime shift in 1976/1977. The SOI exhibited regime shifts in 1976/1977 and 1998/1999, and the NPGO showed a step-like change in 1997/1998. The AOI, MOI, and SHI have synchronous regime shifts in the late 1980s.
Relationships among climate, TSI and abundance
Correlation analysis showed that the SOI had a significant effect on the TSI of the Pacific coast of Japan (Figure S6B; Table S4). Both the AOI and MOI showed significant correlation with TSI in some grids within the coastal waters of China seas and southern part of Sea of Japan (Figures S6C, D; Table S4). The other climate indices were not correlated with the TSI in most of the grids within the northwestern Pacific Ocean (Figures S6A, E, F, G). In addition, the MOI was significantly correlated with EOF1 and EOF3, PDO and NPI had a significant correlation with EOF3, and AOI and NPGO were significantly correlated with EOF4 (Table S4).
Correlation and Gradient forest analysis were carried out to explore the relationship of abundance to climatic and environmental indices and their 1- and 2-year lagged time series. TWC stock (i.e., Chinese catch, TWC catch, biomass, and recruitment) showed a significant correlation with ESTSI/JSTSI and their 1- and 2-year lags, and Chinese catch had an opposite correlation pattern to TWC catch (Table S5). The Pacific stock was significantly correlated with EOF2/SHI and their 1- and 2-year lags (Table S5). Initial gradient forest analyses were carried out to explore the responses of chub mackerel abundance to regional TSI, EOFs, and climatic indices. However, the climatic indices showed negligible importance as explanatory variables while regional TSI and EOFs had higher importance. Therefore, we excluded all the climatic indices from the following gradient forest analyses to achieve better model performance. Gradient forest analysis revealed that Pacific biomass is best explained by regional TSI and EOFs followed by Pacific catch, Pacific recruitment, and Chinese catch, and the TWC catch and recruitment were poorly explained by the predictors (Figure 5A). Additionally, weighted importance indicated that EOF2lag2, EOF2lag1, EOF2, JSTSI, JSTSIlag1, and JSTSIlag2 were the first six contributors to the variations in chub mackerel abundance (Figure 5B). The TWC and Pacific stocks had different threshold responses to regional TSI and EOFs (Figure S7).
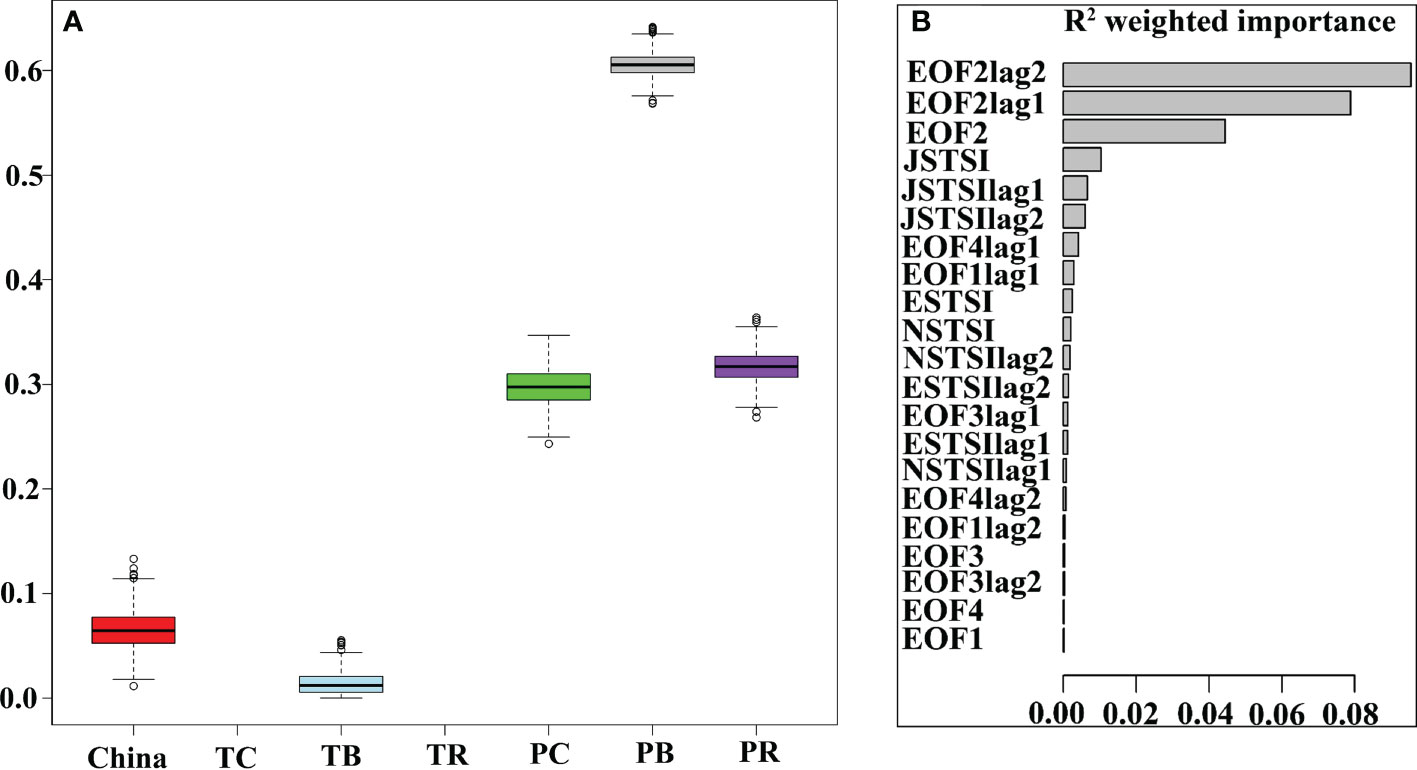
Figure 5 (A) Model performance (goodness-of-fit) for 1000 runs of gradient forest for chub mackerel abundance. (B) Gradient forest analysis shows the weighted importance of regional TSI and modes of EOFs. (CC, Chinese catch; TC, TWC catch; TB, TWC biomass; TR, TWC recruitment; PC, Pacific catch; PB, Pacific biomass; PR, Pacific recruitment). Suffixes lag1 and lag2 represent time lags of 1 and 2 years, respectively.
Non-stationary relationship between TSI and chub mackerel abundance
Model comparisons among stationary (GAM) and non-stationary (TGAM) formulations supported the non-stationary relationship between chub mackerel abundance and TSI with 1- and 2-year lags in the northwestern Pacific Ocean (Figure 6). According to variation in GCV, threshold years were selected to distinguish eras for fitting driver-response relationships separately (Figure S8). The fitted curves of the best TGAMs for the periods before and after the selected threshold years have different patterns, implying that the biology–environment relationships altered across the threshold years (Figure 7). In the case of TWC stock, the best-fitted model for Chinese catch is a non-stationary model with EOF2 as the driver and with thresholds in 1993/1994, while TWC catch, biomass, and recruitment have the best-fitted relationships with ESTSIlag2, ESTSI lag2, and EOF3 with thresholds in the late 1990s. In the case of Pacific stock, a non-stationary model with EOF4, EOF2lag1 and EOF2lag2 as the driver achieves the lowest gCV, with thresholds occurring in the late 1970s to early 1980s.
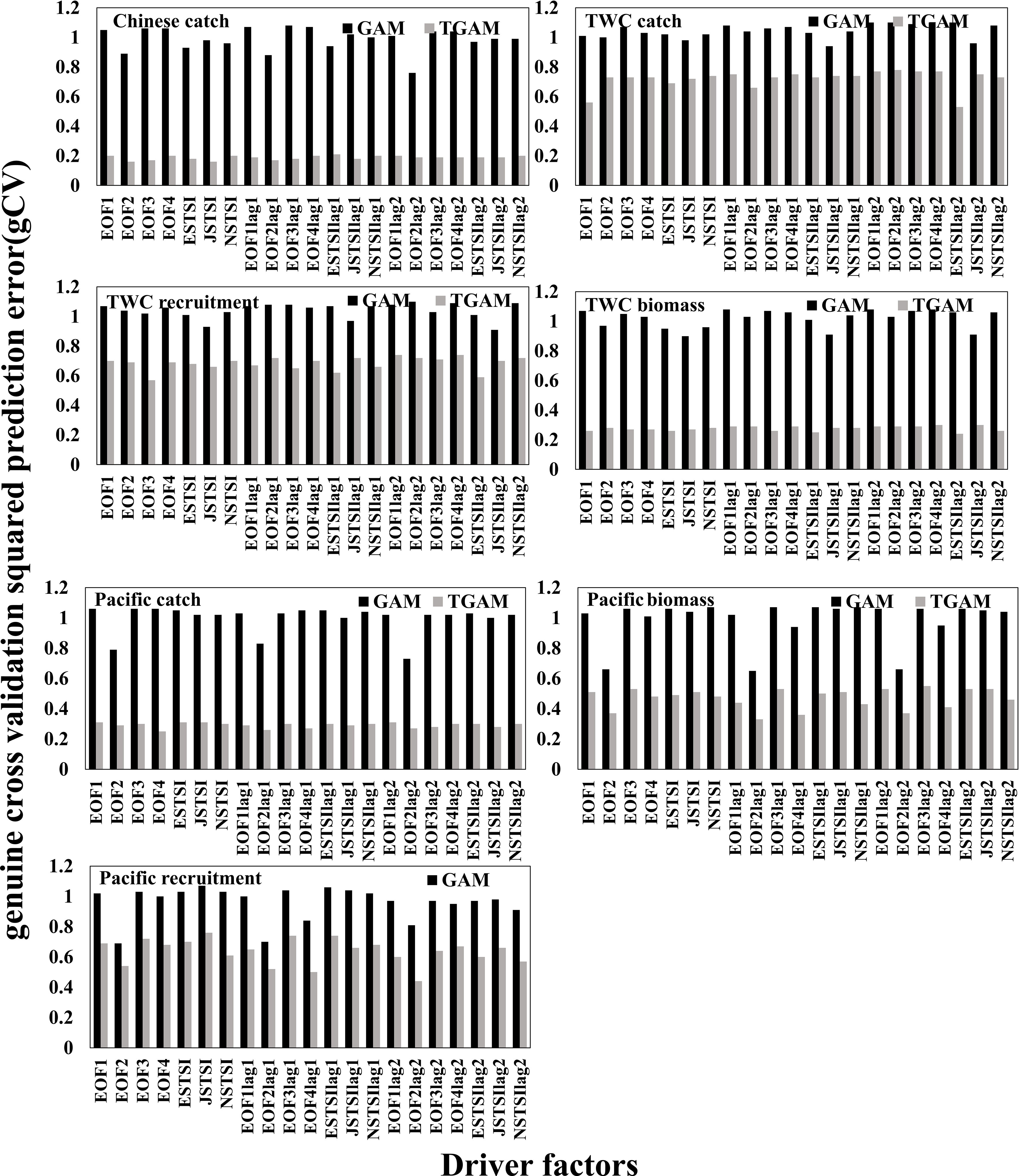
Figure 6 Model comparisons between stationary and non-stationary models. Black bars show the “genuine” cross-validation squared prediction error (gCV) of stationary models (GAMs) and gray bars show the gCV of non-stationary models (TGAMs). Suffixes lag1 and lag2 represent time lags of 1 and 2 years, respectively.
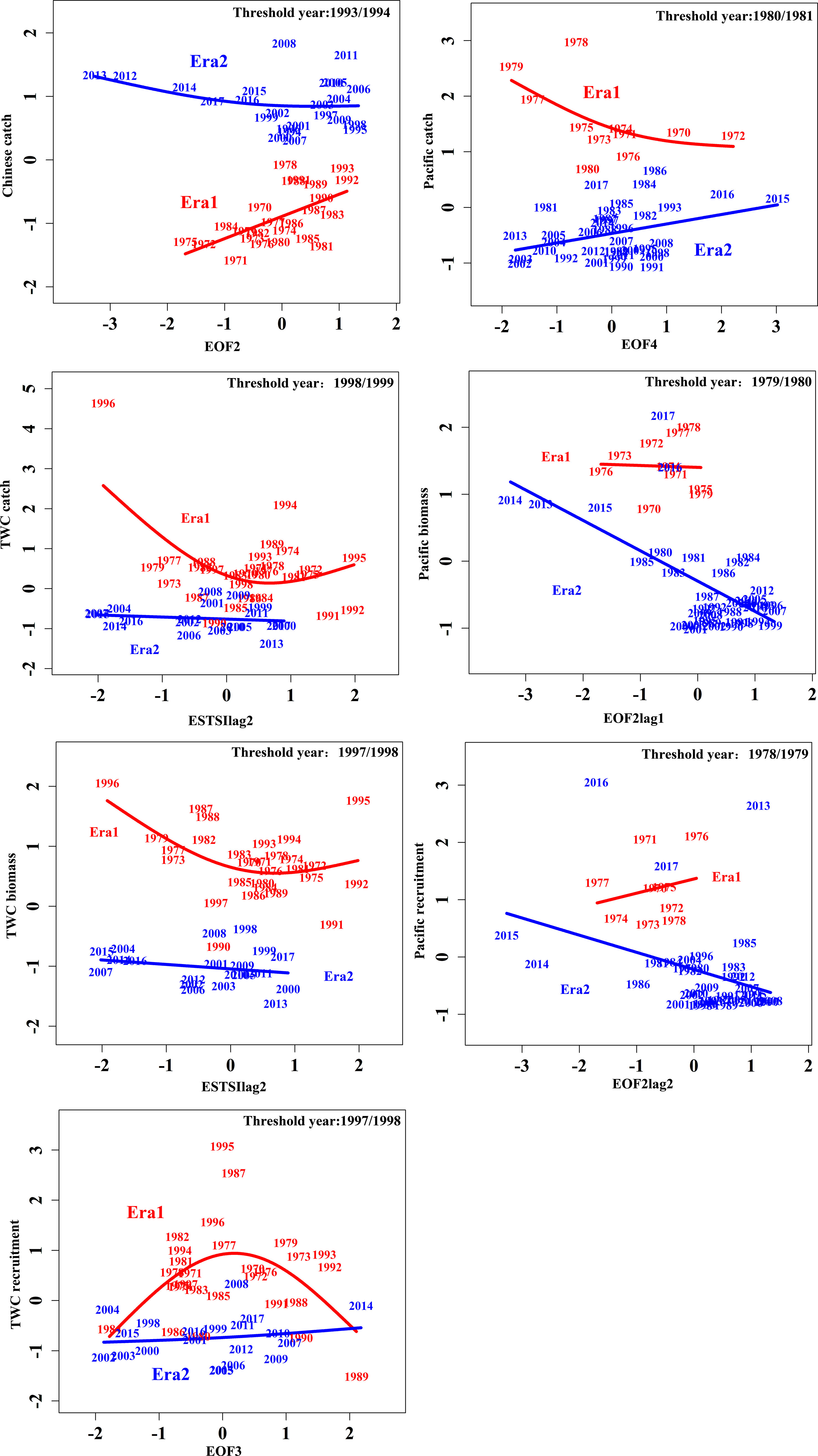
Figure 7 The relationships were fitted by the best-fitted models. Blue and red represent different eras with distinguished models, respectively. Suffixes lag1 and lag2 represent time lags of 1 and 2 years, respectively.
Discussion
Similarities and differences in the response to TSI variability by stocks
In our study, we used Chinese catch, TWC catch by Korea and Japan, and Pacific catch by Japan to represent regional chub mackerel abundance in the northwestern Pacific Ocean and revealed the variability patterns in the different stocks that were characterized by nonlinearity at decadal scales. We found that both TWC and Pacific abundance showed obvious interannual to decadal variability, and had strong responses to TSI variability, but the responding patterns varied (Figure 3). As for the TWC stock of chub mackerel, it spawns in the East China Sea, southern part of Yellow Sea, and the surrounding waters near the Tsushima Strait, and then migrates within the East China Sea, Yellow Sea, and southern part of the Sea of Japan and the stock was jointly utilized by China, Korea and Japan (Shiraishi et al., 2009; Li J. S. et al., 2014; Li Y. S. et al., 2014; Kurota et al., 2018; Yukami et al., 2018; Sogawa et al., 2019), whose fishers mainly fish in different seasons and regions. China harvests this stock in the ESC and Yellow Sea from July to December (Chen et al., 2009; Li G. et al., 2014; Yu et al., 2018). On the other hand, Japan and Korea fish this stock mainly in the East China Sea, Tsushima Strait, and the Sea of Japan during the winter (Yasuda et al., 2014; Kurota et al., 2018; Lee et al., 2018). Concerning the Chinese catch, China mainly fishes chub mackerel in the East China Sea and Yellow Sea (Chen et al., 2009; Li G. et al., 2014; Yu et al., 2018), which belongs to the TWC stock (Kurota et al., 2018). Our study showed that Chinese catch has increased since the 1970s and remained at a stable level after the 2000s, with step-like changes in 1986/1987, 1993/1994, and 2003/2004. Correlation analyses revealed that both ESTSI and JSTSI were significantly negatively correlated with Chinese catch (Figure S3). In other words, the Chinese catch was strongly influenced by the spawning temperature suitability in the East China Sea and the southern part of the Sea of Japan. Although lacking the specific fishing effort data for chub mackerel fished in China seas, the total engine power of Chinese fishing boats could reflect the general pattern in fishing effort growth in China. We found that Chinese catch significantly regressed on the total engine powers of fishing boats in China seas (Figure S9). In other words, the annual and decadal trend and variations in Chinese catch may have been impacted by both fishing intensity and oceanographic conditions. Catches of Japan and Korea in the TWC area were attributed to the TWC catch by the Fisheries Agency of Japan (Kurota et al., 2018). Compared to China, the TWC caught by Japan and Korea were mainly fished off the coast of the East China Sea, in the Tsushima Strait, and in the southern part of the Sea of Japan (Yasuda et al., 2014; Lee et al., 2018). The step-like change of TWC stock occurred in the late 1990s with synchronous regime shifts in ESTSI and JSTSI (Figures 3, 4). Both spawning ESTSI and JSTSI had significant positive correlations with TWC catch by Japan and Korea, showing opposite correlations with Chinese catch (Table S5). Therefore, TWC stock was mainly influenced by spawning temperature suitability in the shelf waters of China seas and the waters of the Tsushima Strait. In particular, the decline in spawning temperature suitability in the late 1990s has caused a decrease in the recruitment of TWC stock, leading to a decline in the abundance of the TWC stock. These results indicated that TWC stock was widely distributed in the East Asian Marginal Seas and the catches by different countries were born in the different seasons and regions. In other words, the monthly and seasonal spawning ground conditions may have effects on catches by different countries. Therefore, cooperation in fishery management must be strengthened among countries around the northwestern Pacific Ocean. Concerning the Pacific stock of chub mackerel, it typically inhabits the Pacific coast of Japan and the Kuroshio-Oyashio transition area (Watanabe and Nishida, 2002; Yukami et al., 2018).
Compared to TWC stock, Pacific stock has exhibited more extreme fluctuations. The Pacific stock showed dramatic decrease in the late 1970s and remained at low levels until the 2000s when it began to recover. Only Japan has long-term catch data of the Pacific stock. Our analysis indicated that the Pacific stock showed a drastic change from the late 1970s to the early 1980s and the NSTSI experienced a regime shift in 1979/1980, showing deterioration in spawning temperature suitability in the Pacific coast of Japan, a crucial spawning area of the Pacific stock. Spawning, survival, and growth of egg/larvae during their early life stages have a great impact on recruitment. Oceanographic conditions during spawning directly affect the early life history and thus affect the standing stock of fisheries resources (Kodama et al., 2018; Kaneko et al., 2019). In this study, spatio-temporal variation in TSI in the northwestern Pacific Ocean was determined using EOF analysis. The spatial mode of EOF1 indicated that TSI in the northwestern Pacific Ocean had opposite loading on both sides of the Kuroshio axis, with TSI of TWC and Pacific areas showing opposite spatial patterns (Figure S4). However, variations in the abundance could not be directly related to the time coefficient of EOF1. Moreover, variation in Pacific stock was mainly influenced by EOF2 that represented TSI variation in the waters about the Kuroshio axis, and which showed synchronous regime shift in the late 1970s and early 2010s (Figures S4, 4). Alongside the results of spatial mode and time coefficient of EOF2, we found different patterns of variation in TSI along the Pacific coast of Japan. In other words, TSI around the Izu Islands and the southern part of Japan’s Pacific coast showed a decreasing trend after the 2000s, but TSI in the northern part had an increasing trend (Figures S4, 4). A previous study indicated that the spawning area of the Pacific stock has northward movement trend after the 2000s (Kanamori et al., 2019), thus the improvement of TSI there can be beneficial to the recovery of the Pacific stock. The results of this study show that the effect of spawning temperature suitability on chub mackerel abundance has a delayed effect of 1 to 2 years, and the preliminary analysis may be due to the fact that the main age of the chub mackerel stock are 0-2 years old (Kurota et al., 2018; Yukami et al., 2018), therefore, it is reasonable that the response of abundance to the environment has time lags (Wang et al., 2021).
Climate-induced non-stationarity and its effects on abundance
In the northwestern Pacific Ocean, numerous studies highlighted the effects of large-scale climatic indices on the oceanic regime shifts, and the East Asian Marginal Seas and the Pacific coast of Japan were influenced by different climatic events (Tian et al., 2004; Tian et al., 2014; Jung et al., 2017; Ma et al., 2019). Previous research indicated that the AOI, MOI, and SHI were all of great importance to the SST field in the continental shelf of the East China Sea and Sea of Japan (Tian et al., 2014; Ma et al., 2019; Ma et al., 2021). In addition, the SOI was closely related to SST in the Kuroshio Current path and Pacific coast of Japan (Tian et al., 2004; Liu et al., 2019), and Kuroshio volume transport had positive relationships with the SST field (Muhling et al., 2018; Ma et al., 2021). Other studies showed that both PDO and NPI had an obvious impact on the reverse temperature variation that occurred between winter and spring after 2000 in the spawning and nursery areas of Pacific stock of chub mackerel, which may influence the Kuroshio Current path, and thus the SST in these areas (Kaneko et al., 2019). In our study, TSI was used to analyze the effects of climate variability on chub mackerel abundance, and the TSI was calculated by the relationship between the probability of spawning and SST. Our results demonstrated that TSI in different areas can be affected by AOI, MOI, and SOI (Figure S6; Table S4), but climate indices were not correlated with chub mackerel abundance except SHI (Table S5). This indicated that climate variability has influence on biological variability through the intermediary environment variability. This provides evidence that biological variability was driven by climate in the northwestern Pacific Ocean may follow the “atmosphere-ocean–biology” process as well as the “double-integration” process (Di Lorenzo and Ohman, 2013; Ma et al., 2019). Significant differences occurred in the characteristics of the main ocean current in the TWC and Pacific areas, which had been affected by various climate modes (Figure 2) (Liu et al., 2019; Ma et al., 2019). In other words, there were significant differences in habitat environment between the TWC and Pacific stocks of chub mackerel, which ultimately resulted in significant differences in resource changes.
It is increasingly recognized that climate change may cause non-stationary mean andvariance in multivariate physical and biological time series (Ma et al., 2021). Previous studies showed clear evidence for non-stationarity in physics–biology relationships in the North Pacific (Litzow et al., 2018; Litzow et al., 2019; Puerta et al., 2019; Ma et al., 2020; Ma et al., 2021). In this study, all the relationships between chub mackerel abundance and TSI are better fitted by non-stationary TGAMs than stationary GAMs. Pelagic communities in the Tsushima and Pacific areas showed regime shifts in the late 1990s and late 1980s, respectively, and non-stationarity was identified in the Tsushima and Pacific areas as a possible result of the declined variances in the Siberian High and Aleutian Low, respectively (Ma et al., 2020; Ma et al., 2021). On one hand, our results showed that the threshold years of the biology–environment relationships in TWC stock (i.e., Chinese catch, and TWC catch, biomass, and recruitment) were in 1993/1994 and the late 1990s, which may be related to variations in the Siberian High, and this result was consistent with previous research on the non-stationarity in the pelagic community of the Tsushima area (Ma et al., 2021). The decline in Siberian high was of great impact on the temperature of YS and East China Sea in the 1990s (Jung et al., 2017; Ma et al., 2021) and the temperature variation influenced the spawning temperature suitability. This would further affect the early life history stage of chub mackerel (Wang et al., 2021). Finally, the TWC abundance of chub mackerel had evident variation in the 1990s. The Siberian High has interaction with the Arctic Oscillation, and plays a crucial role in the variations of the winter East Asian Monsoon (Gong and Ho, 2002; Wu and Wang, 2002). In the late 1980s, AOI, MOI and SHI shifted from a phase to an opposite phase. Consequently, the weakening monsoon and decreasing intrusion of cold air directly caused the increase in water temperature. This can have a great impact on the ecosystems, communities, and biology in the Tsushima area. On the other hand, the Pacific stock of chub mackerel experienced step changes in the late 1970s, late 1980s, and 2010s, and the threshold years of the non-stationary relationship were detected in the late 1970s to early 1980s, corresponding to the sharp decline of the chub mackerel stock. Litzow et al. (2018) reported that the Aleutian Low forced changes in PDO–NPGO relative importance, resulting from sharp declines in the variance of the Aleutian Low in the late 1980s as well as the associations among individual environmental variables in the Gulf of Alaska, which could induce the non-stationarity in the northeastern North Pacific. Ma et al. (2021) also indicated that the threshold years of community–climate/environment relationships in the Pacific area were in the 1980s, which is closely related to the variations in the Aleutian Low. However, the Pacific stock of chub mackerel did not show a significant step change in the late 1980s, which may be explained by the fact that the resource experienced a more dramatic decline in the late 1970s, fishing pressure remained at a higher level in the 1980s, and the resources was still at a lower level (Wang et al., 2021). Therefore, even the decline of Aleutian low pressure did not have a significant impact on it, but the climate variability in the 1970s and after 2000s had a more direct impact on its step changes. In this study, the threshold year of Pacific stock was similar to the step change of PDO, NPI, and SOI in the mid-1970s, and TSI on the Pacific coast of Japan was significantly correlated with the SOI. Therefore, the non-stationary relationship between climate/environment and biology in the Pacific area may be closely related to the influence of the Aleutian Low and ENSO on the oceanographic conditions (i.e., temperature). Previous studies had confirmed that ENSO was of great impact on the temperature during wintering and spawning seasons of chub mackerel in the Kuroshio waters (Kaneko et al., 2019; Wang et al., 2021). Hostile winter temperature conditions will affect the growth, survival, and accumulation of the energy of chub mackerel, resulting in poor spawning activity during the subsequent spawning season, and the poor spawning ground conditions are a major challenge to the survival of the larvae (Kamimura et al., 2015; Wang et al., 2021). Therefore, the step change in Pacific abundance of chub mackerel in the late 1970s may be closely related to the interannual to decadal variability in the Aleutian Low and ENSO.
In this study, we focus on the effects of changes in spawning conditions on the abundance of different stocks of chub mackerel in the context of climate variability, and the results of which also show better differences in the variations of TWC and Pacific stocks. Our Study have shown that variations in climate cause changes in resources through effects on habitat and thus on ecological processes such as early recruitment. The main focus in this study is on the effect of abiotic factors on resource variability. However, previous studies have shown that biotic factors may also have a crucial impact on the variability of the abundance, for example, spawning-stock biomass (SSB) can influence the intensity of recruitment by affecting the expansion/contraction of spawning grounds (Furuichi et al., 2020). Therefore, for understand the population dynamics, it is necessary to pay attention not only to the influence of climatic and environmental factors, but also density-dependent factors (i.e. SSB) are particularly important, which provides further research ideas for future resource assessment and management among countries.
Conclusions
Chub mackerel stocks showed evident interannual and decadal variations, and patterns of variations in TWC and Pacific stocks differ significantly. Based on the relationship between the probability of spawning and specific SST, we developed the average temperature suitability index (TSI) in the main spawning areas that could explain variations in chub mackerel abundance, and the TWC and Pacific stocks corresponded well with the TSI in the coastal waters of China seas, the southern part of the Sea of Japan, and the Pacific coast of Japan. In addition, non-stationarity was identified with threshold years in the 1990s for the TWC stock and in the late 1970s for the Pacific stock, which may be closely related to variations in the Siberian High, the Aleutian Low, and ENSO. Climate-induced variations in the environment of TWC and Pacific spawning areas differ, resulting in altered marine physicochemical properties and food availability in the early life stages of chub mackerel. This affects spawning, growth, and survival, and the recruitment strengths and variations in the abundance of different stocks. Therefore, when managing chub mackerel resource, it is important to take full account of the differences of variations among stocks, as well as to strengthen national cooperation.
Data availability statement
The original contributions presented in the study are included in the article/Supplementary Material. Further inquiries can be directed to the corresponding author.
Author contributions
LW and YT conceived of the study. LW and SM conducted the data compilation and analysis. SM, YL and JL provided guidance in the methods. LW wrote the manuscript. YT and DS obtained funding for the study. All authors contributed to the article and approved the submitted version.
Funding
This work partially supported by Central Public-interest Scientific Institution Basal Research Fund, South China Sea Fisheries Research Institute, CAFS (NO. 2021TS05), and the National Natural Science Foundation of China (NSFC) (Grant No. 4193053).
Acknowledgments
We acknowledge the Food and Agriculture Organization of the United Nations, Fisheries Agency of Japan, Japan Meteorological Agency and National Oceanic and Atmospheric Administration, US Department of Commerce for providing free and open marine data.
Conflict of interest
The authors declare that the research was conducted in the absence of any commercial or financial relationships that could be construed as a potential conflict of interest.
Publisher’s note
All claims expressed in this article are solely those of the authors and do not necessarily represent those of their affiliated organizations, or those of the publisher, the editors and the reviewers. Any product that may be evaluated in this article, or claim that may be made by its manufacturer, is not guaranteed or endorsed by the publisher.
Supplementary material
The Supplementary Material for this article can be found online at: https://www.frontiersin.org/articles/10.3389/fmars.2022.996626/full#supplementary-material
References
Casini M., Hjelm J., Molinero J.-C., Lövgren J., Cardinale M., Bartolino V., et al. (2009). Trophic cascades promote threshold-like shifts in pelagic marine ecosystems. Proc. Natl. Acad. Sci. U.S.A. 106, 197–202. doi: 10.1073/pnas.0806649105
Chen X. J., Li G., Feng B., Tian S. Q. (2009). Habitat suitability index of chub mackerel (Scomber japonicus) from July to September in the East China Sea. J. Oceanogr. 65, 93–102. doi: 10.1007/s10872-009-0009-9
Cheung W. W. L., Brodeur R. D., Okey T. A., Pauly D. (2015). Projecting future changes in distributions of pelagic fish species of northeast pacific shelf seas. Prog. Oceanogr. 130, 19–31. doi: 10.1016/j.pocean.2014.09.003
Ciannelli L., Chan K.-S., Bailey K. M., Stenseth N. C. (2004). Nonadditive effects of the environment on the survival of a large marine fish population. Ecol. 85, 3418–3427. doi: 10.1890/03-0755
Dickson K. A., Donley J. M., Chugey S., Lisa B. (2002). Effects of temperature on sustained swimming performance and swimming kinematics of the chub mackerel scomber japonicus. J. Exp. Biol. 205, 969–980. doi: 10.1242/jeb.205.7.969
Di Lorenzo E., Ohman M. D. (2013). A double-integration hypothesis to explain ocean ecosystem response to climate forcing. Proc. Natl. Acad. Sci. U.S.A. 110, 2496–2499. doi: 10.1073/pnas.1218022110
Ellis N., Smith S. J., Pitcher C. R. (2012). Gradient forests: calculating importance gradients on physical predictors. Ecol. 93, 156–168. doi: 10.2307/23144030
FAO-FIGIS (2018). Fisheries global information system (FIGIS). FI institutional websites. In: FAO fisheries and aquaculture department. Available at: http://www.fao.org/fishery/statistics/global-capture-production/query/en (Accessed October 13, 2018).
Furuichi S., Yasuda T., Kurota H., Yoda M., Ma F. (2020). Disentangling the effects of climate and density-dependent factors on spatiotemporal dynamics of japanese sardine spawning. Mar. Ecol. Prog. Ser. 633, 157–168. doi: 10.3354/meps13169
Gong D. Y., Ho C. H. (2002). The Siberian high and climate change over middle to high latitude Asia. Theor. Appl. Climatol. 72, 1–9. doi: 10.1007/s007040200008
Hannachi A., Jolliffe I. T., Stephenson D. B. (2007). Empirical orthogonal functions and related techniques in atmospheric science: A review. Int. J. Climatol. 27, 1119–1152. doi: 10.1002/joc.1499
Hernández J. J. C., Ortega A. T. S. (2000). “Synopsis of biological data on the chub mackerel (Scomber japonicus houttuyn 1782),” in Fisheries synopsis no. 157 (Rome: FAO).
Jung H. K., Rahman S. M., Kang C.-K., Park S.-Y., Lee S. H., Park H. J., et al. (2017). The influence of climate regime shifts on the marine environment and ecosystems in the East Asian marginal seas and their mechanisms. Deep-Sea Res. Part II Top. Stud. Oceanogr. 143, 110–120. doi: 10.1016/j.dsr2.2017.06.010
Kamimura Y., Masanori T., Norio Y., Watanabe C., Kawabata A. (2015). Larval and juvenile growth of chub mackerel scomber japonicus in relation to recruitment in the western north pacific. Fis. Sci. 81, 1–9. doi: 10.1007/s12562-015-0869-4
Kanamori Y., Takasuka A., Nishijima S., Okamura H. (2019). Climate change shifts the spawning ground northward and extends the spawning period of chub mackerel in the western north pacific. Mar. Ecol. Prog. Ser. 624, 155–166. doi: 10.3354/meps13037
Kaneko H., Okunishi T., Seto T., Kurota H., Itoh S., Kouketsu S., et al. (2019). Dual effects of reversed winter–spring temperatures on year-to-year variation in the recruitment of chub mackerel (Scomber japonicus). Fish Oceanogr. 28, 212–227. doi: 10.1111/fog.12403
Kodama T., Wagawa T., Ohshimo S., Morimoto H., Iguchi N., Fukudome K. I., et al. (2018). Improvement in recruitment of Japanese sardine with delays of the spring phytoplankton bloom in the Sea of Japan. Fish Oceanogr. 27, 1–13. doi: 10.1111/fog.12252
Kurota T. M., Yoda K., Suzuki S., Takegaki C., Sassa, Takahashi M. (2018). “Stock assessment and evaluation for tsushima current stock of chub mackerel (fiscal year 2017/2018),” in Marine fisheries stock assessment and evaluation for Japanese waters (fiscal year 2017/2018) (Tokyo: Fisheries Agency and Fisheries Research Agency of Japan), 201–237.
Lee D. B., Son S. H., Kim W., Park J. M., Joo H., Lee S. H. (2018). Spatio-temporal variability of the habitat suitability index for chub mackerel (Scomber japonicus) in the East/Japan Sea and the south Sea of south Korea. Remote Sens. 10, 938–949. doi: 10.3390/rs10060938
Li G., Chen X. J., Lei L., Guan W. J. (2014). Distribution of hotspots of chub mackerel based on remote-sensing data in coastal waters of China. Int. J. Remote Sens. 35, 4399–4421. doi: 10.1080/01431161.2014.916057
Li Y. S., Pan L. Z., Chen X. J. (2014). Effect of spawning ground location on the transport and growth of chub mackerel (Scomber japonicus) eggs and larvae in the East China Sea. Acta Ecologica Sinica. 34, 92–97. doi: 10.1016/j.chnaes.2013.06.001
Litzow M. A., Ciannelli L., Puerta P., Wettstein J. J., Rykaczewski R. R., Opiekun M. (2018). Non-stationary climate-salmon relationships in the gulf of Alaska. Proc. R. Soc B 285, 20181855. doi: 10.1098/rspb.2018.1855
Litzow M. A., Ciannelli L., Puerta P., Wettstein J. J., Rykaczewski R. R., Opiekun M. (2019). Nonstationary environmental and community relationships in the north pacific ocean. Ecol. 100, e02760. doi: 10.1002/ecy.2760
Liu S. G., Liu Y., Fu C. H., Yan L. X., Xu Y., Wan R., et al. (2019). Using novel spawning ground indices to analyze the effects of climate change on pacific saury abundance. J. Mar. Syst. 191, 13–23. doi: 10.1016/j.jmarsys.2018.12.007
Li J. S., Yan L. P., Hu F. (2014). Inter-decadal variation in the reproductive characteristics of chub mackerel scomber japonicus in the northern yellow Sea. J. Fish. Sci. China. 21, 567–573. doi: 10.3724/SP.J.1118.2014.00567
Li J. S., Yan L. P., Hu F. (2015a). Inter-decadal changes in biological characteristics of reproductive stocks of chub mackerel, scomber japonicus, in the East China Sea. J. Fish. Sci. China. 22, 1253–1259. doi: 10.3724/SP.J.1118.2015.15025
Li J. S., Yan L. P., Hu F., Zhang H. (2015b). The reproductive biology of chub mackerel (Scomber japonicus) in wen-tai fishing ground. J. Fish. Sci. China. 22, 99–105. doi: 10.3724/SP.J.1118.2015.00112
Ma S. Y., Liu Y., Li J. C., Fu C. H., Ye Z. J., Sun P., et al. (2019). Climate-induced long-term variations in ecosystem structure and atmosphere-ocean-ecosystem processes in the yellow Sea and East China Sea. Prog. Oceanogr. 175, 183–197. doi: 10.1016/j.pocean.2019.04.008
Mantua N. (2004). Methods for detecting regime shifts in large marine ecosystems: a review with approaches applied to north pacific data. Prog. Oceanogr. 60, 165–182. doi: 10.1016/j.pocean.2004.02.016
Mantua N. J., Hare S. R. (2002). The pacific decadal oscillation. J. Oceanogr. 58, 35–44. doi: 10.1023/A:1015820616384
Ma S. Y., Tian Y. J., Fu C. H., Yu H. Q., Li J. C., Liu Y., et al. (2021). Climate-induced nonlinearity in pelagic communities and nonstationary relationships with physical drivers in the kuroshio ecosystem. Fish Fish. 22, 1–17. doi: 10.1111/faf.12502
Ma S. Y., Tian Y. J., Li J. C., Ju P. L., Sun P., Ye Z. J., et al. (2022). Incorporating thermal niche to benefit understanding climate-induced biological variability in small pelagic fishes in the kuroshio ecosystem. Fish. Oceanogr. 31, 172–190. doi: 10.1111/fog.12570
Ma S. Y., Tian Y. J., Li J. C., Yu H. Q., Cheng J. H., Sun P., et al. (2020). Climate variability patterns and their ecological effects on ecosystems in the northwestern north pacific. Front. Mar. Sci. 7. doi: 10.3389/fmars.2020.546882
Muhling B., Tommasi D., Ohshimo S., Alexander M. A., Dinardo G. (2018). Regional-scale surface temperature variability allows prediction of pacific bluefin tuna recruitment. ICES. J. Mar. ci. 75, 1341–1352. doi: 10.1093/icesjms/fsy017
Overland J. E., Alheit J., Bakun A., Hurrell J. W., Mackas D. L., Miller A. J. (2010). Climate controls on marine ecosystems and fish populations. J. Mar. Syst. 79, 305–315. doi: 10.1016/j.jmarsys.2008.12.009
Overland J., Rodionov S., Minobe S., Bond N. (2008). North pacific regime shifts: definitions, issues and recent transitions. Prog. Oceanogr. 77, 92–102. doi: 10.1016/j.pocean.2008.03.016
Planque B., Frédou T. (1999). Temperature and the recruitment of Atlantic cod (Gadus morhua). Can. J. Fish. Aquat. Sci. 56, 2069–2077. doi: 10.1139/cjfas-56-11-2069
Puerta P., Ciannelli L., Rykaczewski R. R., Opiekun M., Litzow M. A. (2019). Do gulf of Alaska fish and crustacean populations show synchronous nonstationary responses to climate? Prog. Oceanogr. 175, 161–170. doi: 10.1016/j.pocean.2019.04.002
Rodionov S. N. (2004). A sequential algorithm for testing climate regime shifts. Geophys. Res. Lett. 31, 1–4. doi: 10.1029/2004GL019448
Schaefer K. M. (1986). Lethal temperatures and the effect of temperature change on volitional swimming speeds of chub mackerel, scomber japonicas. Copeia. 1, 39–44. doi: 10.2307/1444885
Shiraishi T., Ketkar S. D., Katoh Y., Nyuji M., Yamaguchi A., Matsuyama M. (2009). Spawning frequency of the tsushima current subpopulation of chub mackerel scomber japonicus off Kyushu, Japan. Fish. Sci. 75, 649–655. doi: 10.1007/s12562-009-0094-0
Shiraishi T., Okamoto K., Yoneda M., Sakai T., Ohshimo S., Onoe S., et al. (2008). Age validation, growth and annual reproductive cycle of chub mackerel scomber japonicus off the waters of northern Kyushu and in the East China Sea. Fish. Sci. 74, 947–954. doi: 10.1111/j.1444-2906.2008.01612.x
Sims D. W., Wearmouth V. J., Genner M. J., Southward A. J., Hawkins S. J. (2004). Low-temperature-driven early spawning migration of a temperate marine fish. J. Anim. Ecol. 73, 333–341. doi: 10.1111/j.0021-8790.2004.00810.x
Sogawa S., Hidaka K., Kamimura Y., Takahashi M., Saito H., Okazaki Y., et al. (2019). Environmental characteristics of spawning and nursery grounds of Japanese sardine and mackerels in the kuroshio and kuroshio extension area. Fish. Oceanogr. 28, 1–14. doi: 10.1111/fog.12423
Takasuka A., Oozeki Y., Kubota H. (2008). Multi-species regime shifts reflected in spawning temperature optima of small pelagic fish in the western north pacific. Mar. Ecol. Prog. Ser. 360, 211–217. doi: 10.3354/meps07407
Tian Y., Akamine T., Suda M. (2003). Variations in the abundance of pacific saury (Cololabis saira) from the northwestern pacific in relation to oceanic-climate changes. Fis. Res. 60, 439–454. doi: 10.1016/S0165-7836(02)00143-1
Tian Y., Kidokoro H., Watanabe T., Iguchi N. (2008). The late 1980s regime shift in the ecosystem of tsushima warm current in the Japan/East Sea: Evidence from historical data and possible mechanisms. Prog. Oceanogr. 77, 127–145. doi: 10.1016/j.pocean.2008.03.007
Tian Y., Uchikawa K., Ueda Y., Cheng J. H. (2014). Comparison of fluctuations in fish communities and trophic structures of ecosystems from three currents around Japan: Synchronies and differences. ICES. J. Mar. Sci. 71, 19–34. doi: 10.1093/icesjms/fst169
Tian Y., Ueno Y., Suda M., Akamine T. (2004). Decadal variability in the abundance of pacific saury and its response to climatic/oceanic regime shifts in the northwestern subtropical pacific during the last half century. J. Mar. Syst. 52, 235–257. doi: 10.1016/j.jmarsys.2004.04.004
Wang L., Ma S., Liu Y., Li J., Liu S., Lin L., et al. (2021). Fluctuations in the abundance of chub mackerel in relation to climatic/oceanic regime shifts in the northwest pacific ocean since the 1970s. J. Mar. Syst. 218, 103541. doi: 10.1016/j.jmarsys.2021.103541
Watanabe C., Nishida H. (2002). Development of assessment techniques for pelagic fish stocks: Applications of daily egg production method and pelagic trawl in the northwestern pacific ocean. Fish. Sci. 68, 97–100. doi: 10.2331/fishsci.68.sup1_97
Weare B. C., Nasstrom J. S. (1982). Examples of extended empirical orthogonal function analyses. Month. Weather Rev. 110, 481. doi: 10.1175/1520-0493(1982)110<0481
Wu B., Wang J. (2002). Winter Arctic oscillation, Siberian high and East Asian winter monsoon. Geophys. Res. Lett. 29, 1897. doi: 10.1029/2002GL015373
Yasuda T., Ryuji Y., Ohshimo S. (2014). Fishing ground hotspots reveal long-term variation in chub mackerel scomber japonicus habitat in the East China Sea. Mar. Ecol. Prog. Ser. 501, 239–250. doi: 10.3354/meps10679
Yatsu A., Watanabe T., Ishida M., Sugisaki H., Jacobson L. D. (2005). Environmental effects on recruitment and productivity of Japanese sardine sardinops melanostictus and chub mackerel scomber japonicus with recommendations for management. Fish. Oceanogr. 14, 263–278. doi: 10.1111/j.1365-2419.2005.00335.x
Yu W., Guo A., Zhang Y., Chen X. J., Qian W. G., Li Y. S. (2018). Climate-induced habitat suitability variations of chub mackerel scomber japonicus in the East China Sea. Fis. Res. 207, 63–73. doi: 10.1016/j.fishres.2018.06.007
Yukami R., NishidCa H., Isu S., Watanabe C., Kamimura Y., Hashimoto. M. (2018). “Stock assessment and evaluation for pacific stock of chub mackerel (fiscal year 2017/2018),” in Marine fisheries stock assessment and evaluation for Japanese waters (fiscal year 2017/2018) (Tokyo: Fisheries Agency and Fisheries Research Agency of Japan), 157–200.
Keywords: chub mackerel, Tsushima Warm Current stock, Pacific stock, temperature suitability index, climate variability, non-stationarity, northwestern Pacific Ocean
Citation: Wang L, Ma S, Liu Y, Li J, Sun D and Tian Y (2022) Climate-induced variation in a temperature suitability index of chub mackerel in the spawning season and its effect on the abundance. Front. Mar. Sci. 9:996626. doi: 10.3389/fmars.2022.996626
Received: 18 July 2022; Accepted: 09 November 2022;
Published: 25 November 2022.
Edited by:
Ibon Galparsoro, Technological Center Expert in Marine and Food Innovation (AZTI), SpainReviewed by:
Sukgeun Jung, Jeju National University, South KoreaSvein Sundby, Norwegian Institute of Marine Research (IMR), Norway
Copyright © 2022 Wang, Ma, Liu, Li, Sun and Tian. This is an open-access article distributed under the terms of the Creative Commons Attribution License (CC BY). The use, distribution or reproduction in other forums is permitted, provided the original author(s) and the copyright owner(s) are credited and that the original publication in this journal is cited, in accordance with accepted academic practice. No use, distribution or reproduction is permitted which does not comply with these terms.
*Correspondence: Yongjun Tian, eWp0aWFuQG91Yy5lZHUuY24=