- 1Institute of Analytical and Environmental Sciences, College of Nuclear Science, National Tsing Hua University, Hsinchu, Taiwan
- 2Department of Zoology, Marine Science division, College of Science, Al-Azhar University, Assiut Branch, Egypt
- 3Institute of Bioinformatics and Systems Biology, National Yang-Ming Chiao Tung University, Hsinchu, Taiwan
- 4Taiwan Ocean Research Institute, National Applied Research Laboratories, Kaohsiung, Taiwan
One of the most crucial struggles for the aquatic ecosystem and modern society is environmental pollution. New approaches, such as the environmental DNA Metagenomic approach, have become a powerful tool to investigate the abundance and diversity of sediment-associated microbiota communities. Nevertheless, information on the response of microbial populations to heavy metals (HMs) in the coastal sediments of Taiwan is scarce. 44 sediment samples were collected from the Siangshan wetland in Taiwan (Taiwan Strait) at two different depths (surface and sub-surface) to measure various environmental variables viz., heavy metals, total organic matters, and sediment composition. Moreover, the abundance and structure of sediment-associated microbiota were examined using high-throughput 16S rRNA gene (V3-V4) next-generation sequencing, to explore the response of the microbiota community to environmental variables. According to the computed pollution indices viz., sediment quality guidelines (SQGs), enrichment factor (EF), geo-accumulation index (Igeo), pollution index (PI), and pollution load index (PLI), the studied sites were classified as unpolluted, moderately polluted, and extremely polluted. Our results revealed that the majority of high-quality reads were assigned to bacteria (~ 95.05%), Archaea (~ 4.83%), and 0.13% were unclassified. Study sites were dominated largely by Proteobacteria with a total of 38.02% across all sediment samples, followed by Bacteroidota (7.18%), Desulfobacterota (6.97%), Actinobacterota (6.68%), Cyanobacteria (5.84%), Chloroflexota (4.18%), Planctomycetota (4.16%), and Firmicutes (3.69%). Beta diversity (Non-metric dimensional scaling analysis, nMDS) explained that surface and sub-surface groups had significantly different microbial community compositions (p = 0.01). Meanwhile, polluted sites exhibited more richness and diversity than unpolluted sites. Redundancy analysis (RDA) illustrated that Mn, Cu, Al, Co, Ni, Sand, and Cr had a sizable effect on the structure of microbiota communities (at the class level). This work highlights the potential responsibility of environmental variables in shaping the sedimentary microbiota of the Siangshan wetland via integrating various ecological variables with alteration of the microbiota composition.
1 Introduction
Nowadays, environmental contamination is one of the most serious problems confronting modern human society. Most near-shore marine environments around the world are under growing threat (David and Obura, 2000). Heavy metal (HM) contamination in aquatic environments is a global environmental issue that has garnered increasing attention over the last few decades because of its negative impacts (Iksandar and Keeney, 1974; Allen-Gil et al., 1997; Kim et al., 2003a; Ma et al., 2013; Wang et al., 2013). Heavy metals in the aquatic ecosystems originate from natural activities, i.e., geogenic (erosion and weathering) and anthropogenic sources (Bai et al., 2011; Wang et al., 2015b; Lee et al., 2017; Yazidi et al., 2017; Barut et al., 2018). The major sources of HMs are anthropogenic activities, such as smelting, mining, agriculture, aquaculture, and domestic or industrial discharge. (Vareda et al., 2019). Once HMs enter the aquatic ecosystem, they can be bioaccumulated by marine species and biomagnified through the food web, posing a serious menace to humans and animal hygiene (Tian et al., 2020) or adsorbed by suspended particles, then heavily accumulated in sediments (Gümgüm et al., 1994; Yi et al., 2011) while only ions persist soluble in the water column. As a result, sediments appear to be the main repository and sink for heavy metals in comparison to water, and may also serve as sources of contamination. Hence, sediments are commonly employed as geo-markers for the management and detection of likely sources of contamination in aquatic habitats (Hakanson, 1980; Ahmad et al., 2010; Bastami et al., 2012; Wang et al., 2012; Alves et al., 2014; Tavakoly Sany et al., 2014). Due to their biotoxicity, widespread origins, persistence, non-degradability, and subsequent bio-accumulation, heavy metal pollution, particularly in sediments, is one of the greatest risks to the aquatic environment (Forstner and Wittmann, 1983; Barlas et al., 2005; Gargouri et al., 2011; Fu et al., 2014).
Additionally, coastal sediments are important habitats for a wide range of microbiota such as bacteria and archaea that mediate the uptake, stashing, release, and transport of heavy metals and play a pivotal role in the biogeochemical transformations of nutrients or pollutants. (Ul-Hasan et al., 2019; Liu and Yang, 2020; Custodio et al., 2021). Since, the pollution by heavy metals not only jeopardizes the health of the native people and animals, but is also noxious to almost all the microbiomes by interfering with the growth, shape, and metabolism and inhabiting fundamental cellular activities including the synthesis of proteins and the cell membrane integrity (Kandeler et al., 2000; Walker et al., 2000) leading to shifts in structure, abundance, and distribution pattern of sediment microbiota community (Gillan et al., 2005; Deng et al., 2020; Di Cesare et al., 2020). Due to the extreme sensitivity of these communities to environmental disturbances in their natural habitats, the structure and diversity of sediment-associated microbiota communities are increasingly explored.
High-throughput sequencing (HTS) technology is improving in rate, efficiency, and cost (Di Bella et al., 2013). Consequently, it is becoming increasingly prevalent to investigate the entire population of prokaryotes in many habitats. Metagenomic approaches are culture-independent molecular tools used to explore ambient microbiota, including activity, function, diversity, and direct DNA sequencing extracted from the environment (Thies, 2015; Liao et al., 2020; Liu et al., 2020). Currently, using HTS technology to analyze microbiota in heavy metal-contaminated sediments has received more attention (Wang et al., 2010; Li et al., 2020a; Li et al., 2020b). Although the environmental DNA metagenomic approach is used to analyze microbial communities in heavy metal-contaminated sediments, this approach is rarely used in Asia, especially in Taiwan. Therefore, in our study, the microbiota communities in metal-contaminated sediments were analyzed using the eDNA technique for the first time at the Siangshan wetland in Taiwan.
In the current study, we collected the surface and sub-surface sediments from the Siangshan wetland in Hsinchu city of Taiwan. Environmental variables comprising sediment grain size, total organic matter, and heavy metals content such as Fe, Al, Mn, Zn, Cu, Ni, Co, Cr, Ga, In, Cd, and Pb were analyzed. In parallel, the composition and diversity of the microbiota community as well as how the microbiota community structure responds to environmental variables were investigated using high-throughput 16S rRNA gene (V3-V4) next-generation sequencing. The main aims of this work were to: (1) ascertain the sediment composition, total organic matter (TOM) content, and the levels of Fe, Al, Mn, Zn, Cu, Ni, Co, Cr, Ga, In, Cd, and Pb, (2) evaluate the pollution degree of HMs using stander pollution indices such as sediment quality guidelines (SQGs), geo-accumulation index (Igeo), enrichment factor (EF), pollution index (PI), and pollution load index (PLI) (Ali et al., 2015; Xu et al., 2017; Salam et al., 2019), (3) profile the diversity and structure of the sediment-associated microbiota in Siangshan wetland, and (4) explore the most important environmental variables that influence the sediment microbiota community structure in Siangshan wetland. This research can help us understand the role of heavy metals, as well as the responses and potential adaptability of microbiota communities to different metal-contaminated habitats. This will upgrade the existing understanding of the ecological risks posed by HMs deposition in the Siangshan wetland and provide a useful benchmark for future metal-microbial interaction investigations.
2 Materials and methods
2.1 Study area and sampling process
The Siangshan wetland stretches west of Hsinchu, Taiwan, from the Ke-Ya stream to the Haishan Fishing Port. The coastline is roughly 8 km and the overall study area covers about 1,600 ha and is a major muddy wetland with a variety of species and biodiversity. Siangshan Wetland was given the official name Hsinchu City Coastal Wildlife Sanctuary in 2001. The muddy intertidal region is a breeding habitat for numerous crabs, shrimp, shellfish, and benthic invertebrates, as well as a variety of threatened bird species (Young et al., 2005; Young, 2009; Young, 2019; Lee, 2020).The wetland’s southwest region has vast-scale oyster beds and mangrove forests. Historical pollution events caused by traditional electroplating factories happened from 1970 to 1990. After that, copper contamination in sediment became a major concern because of heavy metal pollution that affected oyster culture and the safety of seafood collected from the west coast of Taiwan (Han and Hung, 1990). After the 1980s, a new era of industrial development happened in Hsinchu city, Hsinchu Science Industrial Park became the top semiconductor manufacturing community in the world contemporary. Except for semiconductor production, this park also included many advanced manufacturers of optoelectronic plates, biomedical supplies, liquid crystal displayers, light-emitting diodes, etc. A new era of industrial development also brought new kinds of pollution. Based on the manufacturing process of high-tech products, many different kinds of materials are used in large quantities. Even under strict control, there is still a trace amount of contamination present in the final effluent water after the wastewater treatment plant. The consumption of water is more than 200 thousand CMD in this park, and more than 100 thousand CMD of final effluent water from the Hsinchu Science Industrial Park wastewater treatment plant is discharged into the middle course of the Ke-Ya stream. In the Siangshan wetland, the Ke-Ya stream was the major terrigenous freshwater source, more than 40% of freshwater output was effluent water from the treatment plant, about 40% was untreated domestic sewage, and less than 20% was natural water collected in the catchment area. All the freshwater from the Ke-Ya stream estuary flows into the wetland, then all pollutants from industrial use will either settle in the wetland or be carried away by the tides to the Taiwan Strait.
Total forty-four sediment samples were collected from nine main areas at Siangshan wetland, Hsinchu City, Taiwan; Ke-Ya (KY), Ke-Ya Water resource center (KW), Da-Juang (DJ), Hui-Min (HM), Fong-Cin (FC), Hai-Shan (HS), Oyster-Bed (OB), Yen-Kan (YK), Mangrove-Area (MA) in April 2021. Each area was divided into different sites that extended from the shore to the inside (perpendicular to the coastline) and were roughly 400 meters apart (Figure 1). From each site, the sediment samples were collected from the surface (0 cm) and sub-surface (~ 40 cm depth) and we collected four sub-samples (representing one sample), using a sterilized plastic spatula (to avoid cross-contamination), and mixed them thoroughly in individual sterile plastic bags, sealed, and rapidly kept in an icebox till returned to the laboratory. At our laboratory, sediment samples were divided into two groups. One group contained 200 g of each sediment sample for environmental variables assessment (grain size analysis, heavy metals, and organic matters), oven-dried at a temperature of 110°C for four hours to eliminate the moisture content, and kept at room temperature. The remainder was kept at -80°C for microbial DNA extraction and later sequencing analysis. To avoid cross-contamination, hygienic gloves were always used and replaced between collections. Each site was GPS-located (Supplementary Table 1), and all sediment samples were gathered during the low tide period.
2.2 Environmental variables assessment
2.2.1 Grain Size Analysis (GSA) and Total Organic Matter (TOM)
Sediment grain size analysis (GSA) was carried out mechanically utilizing sieve techniques (Folk, 1974). To segregate the particle-size fractions, about 100 g of dry sediments were sieved through a stainless steel mesh. (phi) was utilized to express particle size since the logarithmic scale is more practical than the equimultiple scale. Seven fractions were obtained; gravel (Φ-1 > 2.00 mm), very coarse sand (Φ0 > 1 mm), coarse sand (Φ1 > 0.5 mm), medium sand (Φ2 > 0.250 mm), fine sand (Φ3 > 0.125 mm), very fine sand (Φ4 > 0.063 mm) and silt or clay (Φ5 < 0.063 mm). The resulting sediment fractions were divided into three major categories: gravel (Φ-1), sand (Φ0 + Φ1 + Φ2 + Φ3), and mud (Φ4 + Φ5).
Total organic carbon (TOC) was analyzed via potassium dichromate oxidation-colorimetric titration (Peech, 1947; Walkley, 1947; Greweling, 1960). The organic carbon concentrations were then transformed into total organic matter (TOM) by multiplying the results by 1.8. To standardize the potassium dichromate (Cr2O7-2), a blank titration was performed in the same manner. All reagents used in the analysis were Merck PA.
2.2.2 Determination of heavy metal concentration
Heavy metals (HMs) in 44 sediment samples were measured at National Tsing Hua University following USEPA 3051A method (Element, 2007). Briefly, the samples were pre-sieved (2 mm) to remove shells, roots, and pebbles before being pulverized into a very fine powder (0.063 mm) using a porcelain mortar and pestle. Then, 0.5 g of the powdered sediment samples were digested with a 12 ml mixture of nitric and hydrochloric acids (3:1) and held inside a closed system, namely a microwave oven (MarsXpress) for 8min 40s on the temperature ramp, the period required to reach 175°C; this temperature was retained for an additional 4min 30s. Following digestion, each extract was diluted into 50 ml of ultrapure water (Millipore Direct-Q System) and then filtered through 40 mm filter paper (ADVANTEC, Japan). Twelve elements; Iron (Fe), Nickel (Ni), Zinc (Zn), Aluminum (Al), Copper (Cu), Manganese (Mn), Cobalt (Co), Chromium (Cr), Gallium (Ga), Indium (In), Cadmium (Cd), and Lead (Pb) were examined utilizing an inductively coupled plasma (ICP-OES). Calibration curves for HMs determination were established using standard 1000 ppm (ICP multi-element standard solution IV, Merck), and samples were analyzed only when the calibration curve’s r2 was greater than 0.999. After primary calibration, it was tested again after analyzing 10 samples; if there was more than 10% variance, the equipment was recalibrated. The recovery percentages for measured heavy metals were between 95.4% and 107.8%. High-purity acids were utilized in the investigation (Merck PA). All equipment was washed and sanitized for 24 hours in a 10% HNO3 solution before being rinsed with distilled water. Each sample was digested in duplicate, and the findings were represented as mg/kg.
2.3 Sediment quality assessment
2.3.1 Sediment quality guidelines (SQGs)
To more precisely portray the Siangshan wetland’s heavy metal pollution, we compared our findings to various sediment quality guidelines. These guidelines were (1) the national standard guidelines of (Taiwan, 2010) and those of (2) the Canadian Council of Ministers of the Environment (CCME, 2002), (3) the National Oceanic and Atmospheric Administration (NOAA) (Long et al., 1995), and (4) the Australian and New Zealand Environment and Conservation Council and Agriculture and Resource Management Council of Australia and New Zealand (Anzecc, 2000). Each of the four standard guidelines has lower and upper limits for various heavy metals. When the concentration of metal exceeds the lower limit, adverse effects “rarely or occasionally occur”; whenever the concentration exceeds the upper limit, adverse effects “frequently occur.” (Lin et al., 2016).
2.3.2 Potential ecological risk indices
2.3.2.1 Enrichment factor (EF)
The enrichment factor (EF) is a pollution index that is utilized to quantify the level of anthropogenic metal contamination in sediments based on metal enrichments (Sakan et al., 2009) by standardizing the measured metal against a reference metal. The metal values in the samples were primarily standardized using the conservative element. Here, Iron (Fe) was picked as the standardization element (Daskalakis and O'Connor, 1995; Zhang et al., 2007) because it is the fourth most abundant in the earth’s crust, has a fine solid surface, it’s close to that of many metals and its natural concentration tends to be uniform. According to (Ergin et al., 1991), EF is defined by Equation 1:
Whereby and are the ratios between the concentrations of heavy metal i in the sample and its corresponding shale value, respectively, whilst Fem and Feb refer to the iron concentrations in the sample and its corresponding shale value, respectively. In this study, the background values are the average shale values obtained by Turwkian and Wedepohl (Turekian and Wedepohl, 1961) as follow; Fe: 47200, Al: 80000, Mn: 850, Zn: 95, Cu: 45, Ni: 68, Co: 19, Cr: 90, Ga: 19, In: 0.1, Cd: 0.3, and Pb: 20 mg/kg. Because there is no authorized classification system for pollution degrees based on the enrichment factor approach, a tentative five classes are proposed in Table 1 according to Sutherland (Sutherland, 2000).
2.3.2.2 Geo-accumulation index (Igeo)
The geo-accumulation index is another indicator utilized in the evaluation of HM contamination by excluding the influence of geological contributions (Müller, 1969). Igeo can be obtained by Equation 2:
Factor 1.5 is the background matrix correction factor that reduces the geogenic effects (Chen et al., 2007; Zhang et al., 2009). The sediments could be classified into six classes as shown in Table 1.
2.3.2.3 Pollution load index (PLI)
The PI and PLI were used to analyze the studied metals in order to understand heavy metal accumulation in sediments. The pollution index (PI) has been proven to be an effective method for assessing pollution over time, with a lower index value indicating less contamination (Maanan et al., 2015; Tian et al., 2017). PLI can assess overall pollution in the Siangshan wetland sites under consideration (Tomlinson et al., 1980; Tian et al., 2017). PLI was estimated for 10 elements in the present study after PI Cd and PIPb were discarded since they were below detection limits (BDL). PI and PLI are calculated by Equations 3 and 4:
PI is a single-factor pollution index worth. The PI and PLI are classified into several classes, see Table 1.
2.4 Microbiota analysis
2.4.1 Environmental DNA isolation
We recovered the DNA from all sediment samples within a month of collection in extraction sets of 10 samples at a time. Prior to DNA extraction of each sample, all laboratory instruments and surfaces were disinfected using 70% ethanol, then 10% bleach liquid, and then 70% ethanol again, before a 2 h UV light. Gloves were donned constantly and replaced between each extraction process, and sample handling from individual sites. For all surface and sub-surface samples, DNA extraction, sequence amplification, visualization, and purification were performed at National Tsing Hua University (NTHU, Taiwan). Whilst library preparation and sequencing were performed at Genomics BioSci & Tech. company, Taiwan. Before sample mashing and DNA extractions, sediment samples were defrosted at 4°C for 2 hrs.
Genomic DNA was extracted from the sediment samples utilizing the DNeasy Power Soil Pro-Kit (Qiagen). For each extraction, 250 mg of sediment was treated according to the manufacturer’s instructions (https://www.qiagen.com/us/Resources/ResourceDetail?id=9bb59b74-e493-4aeb-b6c1-f660852e8d97&lang=en). Negative controls were provided with samples to detect the presence of pollutants. Then, the DNA extracts were stored at −20°C until PCR amplification.
2.4.2 PCR amplification, visualization, and purification
The universal prokaryotic primer pair 515F (5′- GTGYCAGCMGCCGCGGTAA-3′) and 805R (5′- GACTACHVGGGTATCTAATCC-3′) were used to amplify the 16S rRNA gene (Caporaso et al., 2011; Herlemann et al., 2011), which identifies 291 bp of the V3-V4 hypervariable region (Fierer et al., 2008; Bates et al., 2011). Previous studies have demonstrated that these primers provide effective phylogenetic information and have minor biases towards any bacterial taxa (Liu et al., 2007; Bates et al., 2011; Bergmann et al., 2011).
Each sample was distinguished by a unique paired barcode or tag (Supplementary Table 2) to enable the multiplexing of PCR products in sequencing libraries (Esling et al., 2015; Steyaert et al., 2020). Each 20 μl PCR reaction contained 1 μl of DNA extract, 1 μl per primer, 10 μl of the 2x O’in 1 DNA Polymerase Premix II w/blue dye, and 7 μl PCR-grade water. Three PCR reactions were run per sample using touchdown temperature (to minimize potential PCR bias) and pooled as described in Supplementary Table 3. PCR negative controls were included in each session to ensure that no contaminations occurred. Following that, amplicons were estimated with the Quant-IT Picogreen dsDNA reagent.
An aliquot (60 μl) of each amplification process was visualized on an agarose gel (1.5%) and ran in a TBE buffer (0.5%) to check the expected size of PCRs yielded amplicons (291pb). Gels were stained with Nucleic Acid Stain (BioKit) and Every gel had a 100 base pair DNA ladder (BioKit).
Thereafter, we cut out the target DNA fragment and purified it with the PCR & Gel Clean-up kit (Enzo Life Sciences Technology Co. USA). Briefly, by melting the DNA fragment in binding DNA buffer (200 μl/100mg) and applying the wash steps by washing buffer (650 μl) and finally eluted in 25 μl of elution buffer. After cleanup, samples were visualized again by electrophoresis and quantified by the PicoGreen kit, and equivalent concentrations were mixed into one single pool for sequencing. A NanoDrop spectrophotometer was utilized to measure the pool’s cleanliness and DNA content.
2.4.3 Metagenomic sequencing
Next-generation sequencing generates a tremendous amount of possible identification data, with hundreds of thousands of reads for each sediment sample. The sequencing libraries were produced by applying the Illumina TruSeq® DNA PCR-Free Library Preparation reagent. At the Genomics BioSci & Tech. firm in Taiwan, the purified PCR amplicons were analyzed on the Illumina MiSeq technology utilizing a 2x300 bp paired-end protocol, resulting in paired-end reads that almost entirely overlap. The amplification primers are equipped with MiSeq sequencing adapters and dual-index tags that facilitate PCR product pooling and direct sequencing (multiplexing).
2.5 Bioinformatics analysis
In the present study, we used the open-source program DADA2 version 1.22.0 to quality check, filter, trim, and eliminate chimeras from the Fastaq files (raw demultiplexed sequences) following the online DADA2 workflow Tutorial 1.16 (https://benjjneb.github.io/dada2/tutorial.html) in R platform version 4.1.3 (Callahan et al., 2016). The DADA2 pipeline, which relies on clustering identical reads into distinct sequences known as amplicon sequence variants (ASVs), was more efficient and produced fewer erroneous sequences than other regularly used pipelines. Using ASVs to examine metabarcoding datasets yields more accurate information about composition and diversity than conventional operation taxonomic unit (OTU) approaches, in which cluster sequencing reads based on a pre-determined threshold of dissimilarity (Callahan et al., 2017; Needham et al., 2017). During our pipeline, the forward and reverse reads produced with next-generation sequencing were trimmed and filtered using the ‘filterAndTrim’ function. Thereafter, calculate and plot the error rates (machine-learning algorithm problems) for trimmed reads (learnErrors function), and the ASVs were figured out utilizing the dada function (describing DADA2 denoising results) depending on the trimmed reads from all samples. Merge the aligned reads by matching the denoised forward reads with the reverse-complement of the matching denoised reverse reads, to obtain the full denoised reads (mergePairs function), and chimeric sequences were removed using the removeBimeraDenovo function. For the taxonomy assignment, we aligned the resulting list of ASVs from DADA2 with the reference database available at Genomic Taxonomy Database, GTDB (Parks et al., 2022).
2.6 Statistical analysis
ASV abundance was rarified at the lowest sequencing depth (94,861) using ‘vegan’ package version 2.6.2 (Oksanen et al., 2019) in R version 4.1.3 to reduce the biases resulting from differences in sequencing depth. The rarefaction analysis was plotted employing the ‘rarecurve’ function in the ‘vegan’ package, to assess the gain in ASVs richness as we increase the number of reads for each sample. Alpha diversity indices (Sobs, Chao1, and Shannon) were computed by the ‘estimate richness’ function in the ‘phyloseq’ package (McMurdie and Holmes, 2013) and visualized using the ‘ggbetweenstats’ function in the ‘ggstatsplot’ package version 0.9.1 (Patil, 2021). Alpha diversity indices among surface and sub-surface sites were compared using Student or Welch’s t-test (Welch, 1947). Moreover, beta diversity was calculated by non-metric dimensional scaling (nMDS) depending on Bray-Curtis distances using the ‘plot_ordination’ function of the ‘vegan’ package version 1.38.0 (Oksanen et al., 2019) in R to assess the differences in the microbiota community structure among the surface and sub-surface sediment samples. The analysis of similarity (ANOSIM with 999 iterations) was conducted to test the significance of the dissimilarities in microbial community structure between sediment layers (Clarke, 1993). All alpha and beta diversity indices were calculated from rarified ASVs abundance. The distribution of top taxa (> 1% of all sequences in all sites) was visualized at phylum and class levels in both sediment layers using ‘plot_bar’ of the ‘phyloseq’ package in R. Differences were considered significant when P< 0.05. In addition, to visualize the association between environmental variables and sediment-associated microbiota community structures (most abundant classes > 1%), an interpretive method such as redundancy analysis (RDA) was conducted using CANOCO 4.5 (Lepš and Šmilauer, 2003). For RDA, all variables were log (x + 1) transformed before analysis. Also, the Monte Carlo permutation test (with 999 iterations) was used to identify the factors that had the most influence on microbiota structure. Sediment compositions (GSA), total organic matters (TOM), and pollution indices were visualized using Origin 9.
3 Results
3.1 Granulometric analysis (GSA%) and total organic matters (TOM%)
The collected surface and sub-surface sediment samples at the Siangshan wetland were subjected to GSA, TOM, and heavy metals analyses. According to our results of GSA, sediment compositions were distinguished into seven fractions with different sizes, thereafter we classified them into three main groups (gravel, sand, and mud). Surface and sub-surface sediments of KY1, KW2, HS1, HS2, and YK1 sites were predominated with sand, while HS3, YK2, and MA3 were characterized by muddy sediments at both sediment layers. However, surface sediments of the Oyster beds (OB1, OB2, OB3) were highly abundant with mud (Figure 2, Supplementary Table 4). In contrast, the gravel sediments exhibited limited spatial distribution at all studied sites.
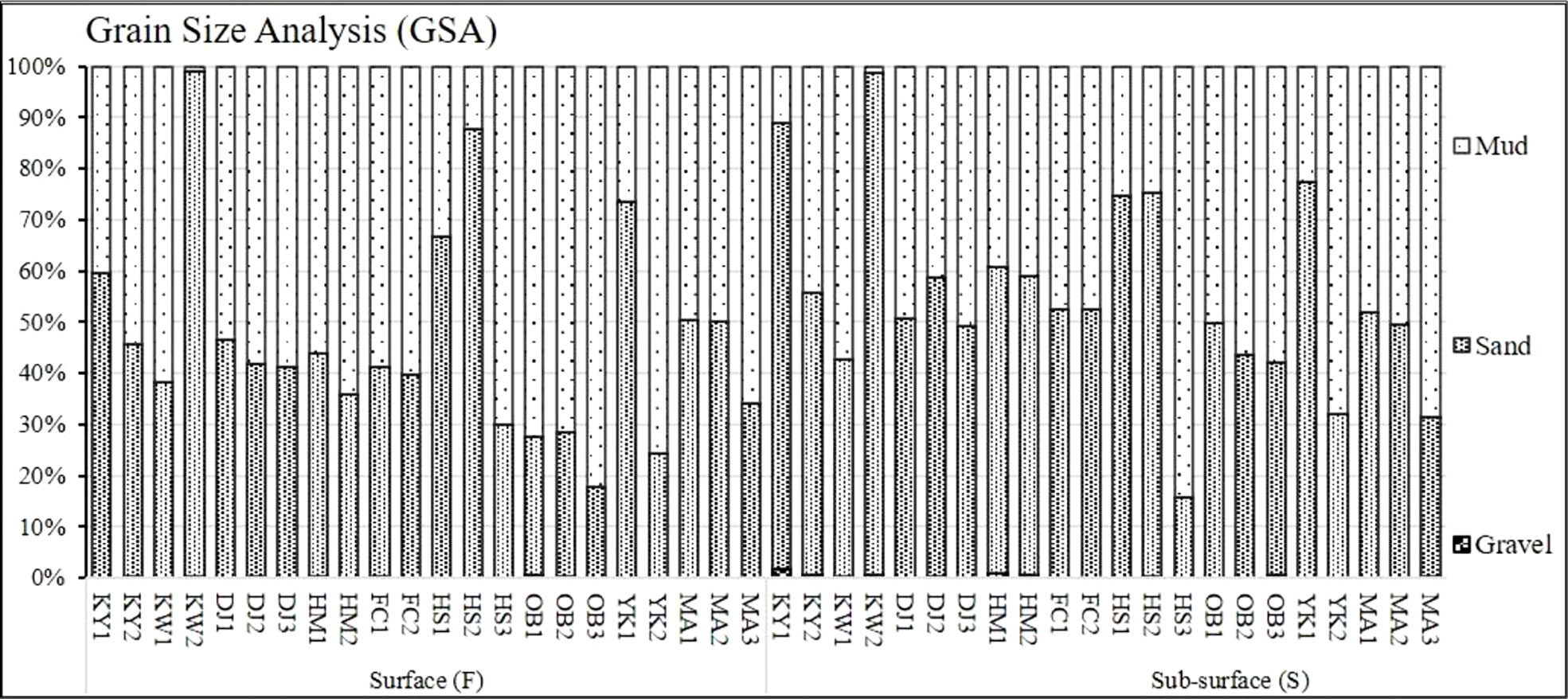
Figure 2 Granulometric analysis of surface and sub-surface sediments at Siangshan wetland, Hsinchu, Taiwan.
Figure 3 and Supplementary Table 4, illustrate the total organic matter in different layers of Siangshan wetland’s sediments. TOM ranged between 10.76% (KY1) and 0.13% (KW2) at the surface sediment, while 4.71% (MA2) and 0.07% (HS2) at the sub-surface layer. Surface sediment of the KY1 site was highly enriched with organic matter (10.76%), and TOM was slightly enriched in surface and sub-surface sediments of the Mangrove areas (MA1, MA2, MA3).
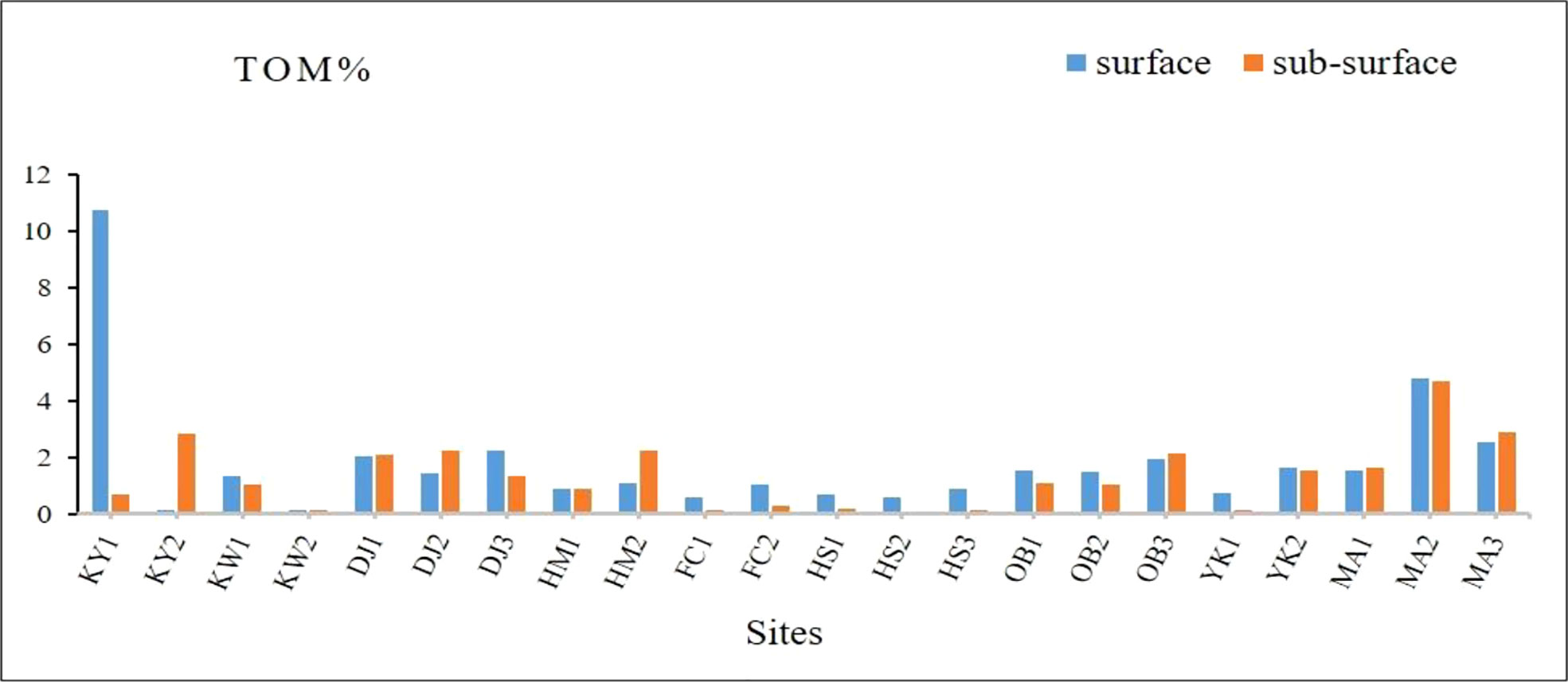
Figure 3 Total organic matter percentages of surface and sub-surface sediments at Siangshan wetland, Hsinchu, Taiwan.
3.2 Distribution of heavy metals in Siangshan wetland sediments
The concentrations of 12 heavy metals were determined in surface and sub-surface sediments and depicted in (Supplementary Tables 5, 6). At surface sediments, the concentrations ranged from 72900 to 12310 for Fe, from 64560 to 7829 for Al, from 917.40 to 184.10 for Mn, from 438.90 to 17.40 for Zn, from 210.70 to 0.10 for Cu, from 74.40 to 3.70 for Ni, from 147.30 to 34.10 for Co, from 123.70 to 24.40 for Cr, from 138 to 41.10 for Ga, from 62.40 to 5.20 for In, and finally, Cd and Pb were below detection limits (BDL). KY1 recorded the highest concentrations for Zn, Cu, and Ni (438.90, 210.70, and 74.40 mg/kg, respectively), while Fe (72900 mg/kg), Co (147.30 mg/kg), and In (62.40 mg/kg) highly accumulated in MA2. Moreover, DJ1 showed the highest values of Al (64560 mg/kg), Cr (123.70 mg/kg), and Ga (138 mg/kg), and OB3 for Mn (917.40 mg/kg). Inversely, YK1 recorded the lowest concentrations for most metals like Fe, Zn, Cu, Ni, Co, Cr, and In (Supplementary Table 5). Also, HMs concentrations varied between sub-surface sediment sites, they fluctuated between 41040 – 12750 for Fe, 65330 – 12680 for Al, 676.90 – 98.90 for Mn, 196.40 – 19.70 for Zn, 98 – BDL for Cu, 37.30 – 3.80 for Ni, 156 – 38.20 for Co, 122.40 – 27.80 for Cr, 135 – 48.70 for Ga, 57.20 – 2.10 for In, Cd, and Pb were below detection limits (BDL). Zinc (Zn), Copper (Cu), and Nickel (Ni) recorded the maximum concentrations in DJ3, while Iron (Fe), Manganese (Mn), Cobalt (Co), Chromium (Cr), and Indium (In) at DJ1. In addition, Aluminum (Al) and Gallium (Ga) were observed high in MA2. In contrast, YK1 recorded the lowest concentrations for Fe, Zn, Cu, Ni, Co, Cr, and In. Ga observed low in KY1. Cadmium (Cd) and Lead (Pb) were below the detection limits in all sites (Supplementary Table 6).
3.3 Sediment quality assessment
3.3.1 Sediment quality guidelines (SQGs)
In this study, we compared our data with reference values established in different SQGs as shown in Table 2. The overall concentration of Zn (113.41 mg/kg) at Siangshan wetlands’ sediments fell among Taiwan EPA’s lower & upper limits and below the other SQGs. Cu (40.18 mg/kg) fell between NOAA ERL & ERM and CCME ISQG & PEL, but less than Taiwan EPA’s lower & upper limits, and ANZECC & ARMCANZ’s low and high. Ni (24.70 mg/kg) was close to Taiwan EPA’s lower limit and fell among NOAA ERL & ERM, and ANZECC & ARMCANZ low and high. Moreover, Cr (80.31 mg/kg) greatly exceeded CCME ISQG and fell among Taiwan EPA’s lower and upper limits, but close to ANZECC & ARMCANZ low and lower than the others. While Cd and Pb (below detection limit) were observed below all the reference values of different SQGs.
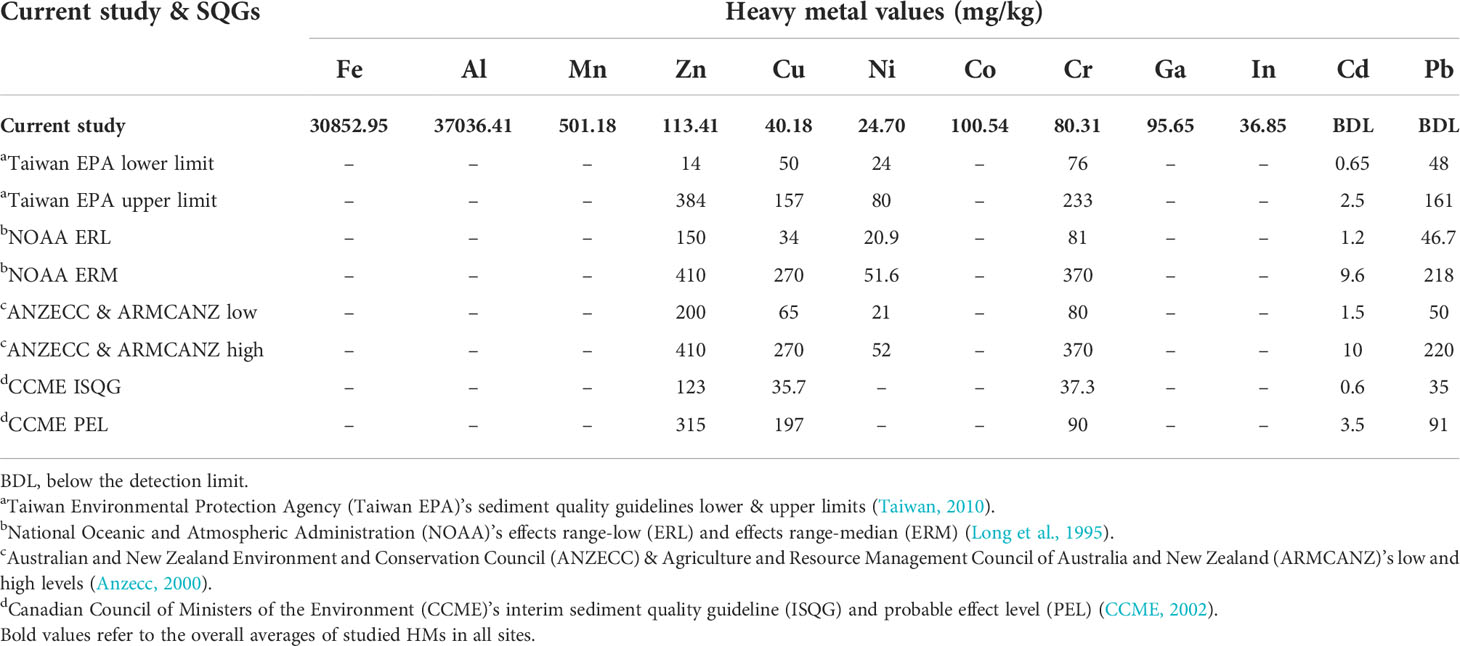
Table 2 Comparison among the overall average of heavy metal concentrations in the Siangshan wetlands’ sediments (current study) and sediment quality guidelines (SQGs) worldwide.
3.3.2 Potential ecological risk indices
3.3.2.1 Enrichment factor (EF)
The calculated heavy metal EFs are shown in Figure 4. Based on EF values, Al, Mn, Ni, Cr, Cd, and Pb in all studied sites showed minimal enrichment (class 0 < 2) (Figures 4A–D), while EF values for the elements viz. Zn, Cu, Co, and Ga ranged between classes 0 and 2 (Figures 4E–H). Interestingly, Indium (In) recorded high EF values > 40 (class 4) at all studied sites (Figure 4I).
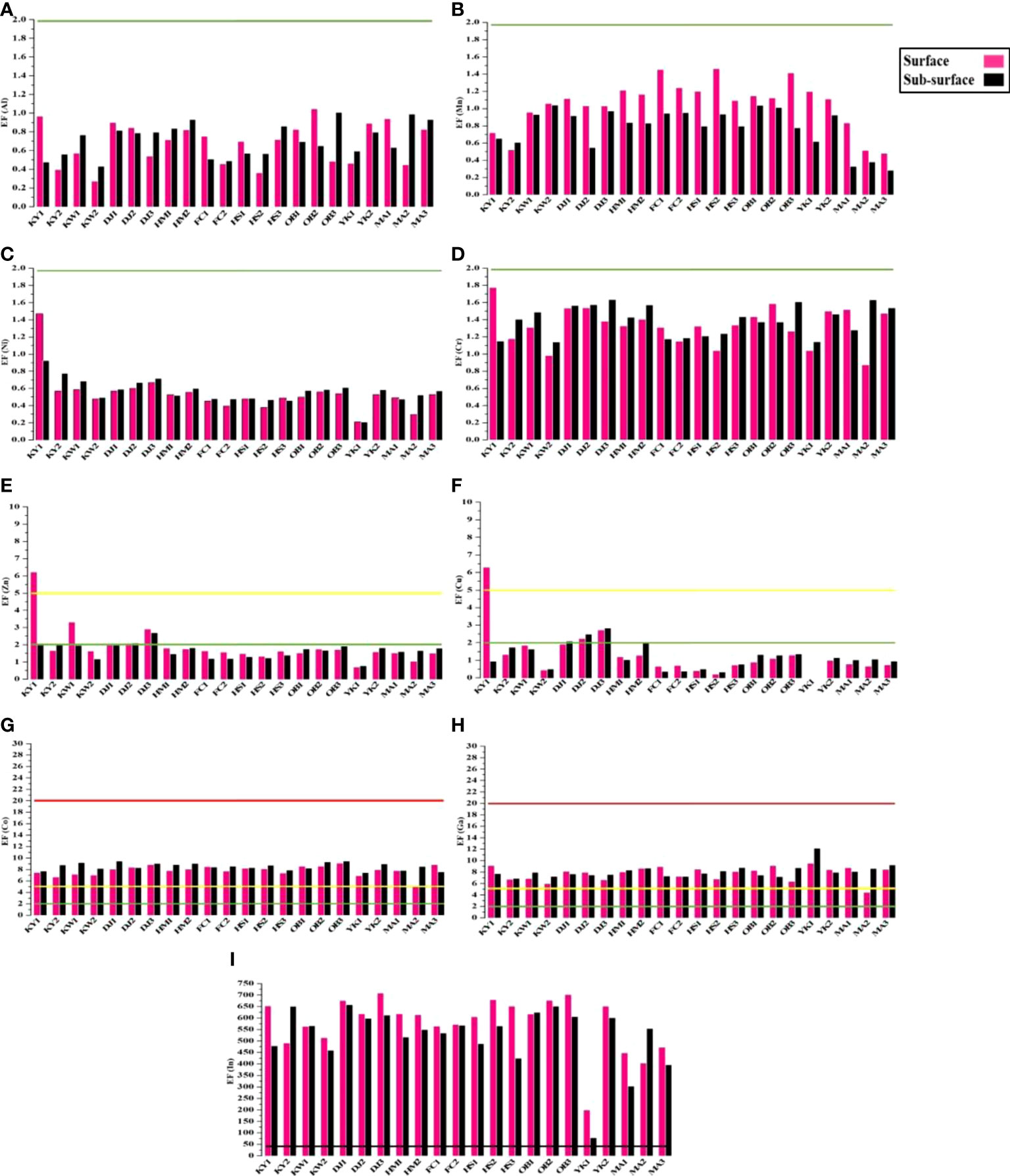
Figure 4 The values of Enrichment factor (EF) with pollution degree for studied metals in surface and sub-surface sediments of Siangshan wetland. Green, yellow, red, and black lines refer to classes 0, 1, 2, and 4, respectively (Table 1).
3.3.2.2 Geo-accumulation index (Igeo)
The geo-accumulation index (Igeo) for the sampling sites is shown in Figure 5. Igeo for Fe, Al, Mn, Zn, Cu, Ni, Cr, Cd, and Pb are falling under class 0 (unpolluted) (Figures 5A–G), while Co and Ga are marked as unpolluted to very slightly polluted (class 0, 1) at different sites (Figure 5H–I). Also, all Siangshan sites are extremely contaminated by Indium (class 5 > 5) except the YK2 sub-surface (Figure 5J).
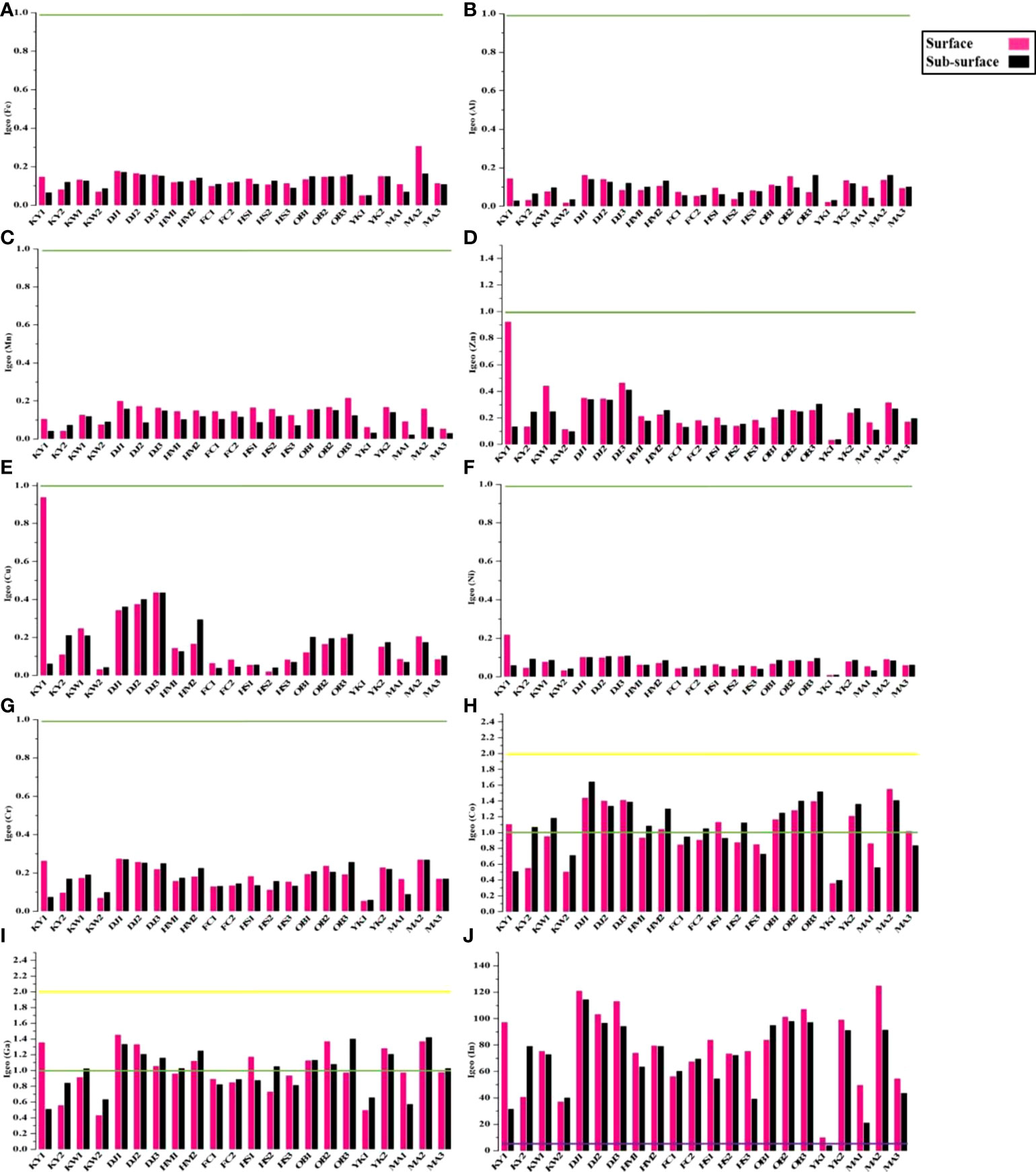
Figure 5 Geo-accumulation index (Igeo) with pollution degree for studied metals in surface and sub-surface sediments of Siangshan wetland. Green, yellow, and purple lines refer to classes 0, 1, and 5, respectively (Table 1).
3.3.2.3 Pollution index (PI)
The heavy metal PI is shown in Figure 6. PI values varied most widely among the sediment samples, where PI for Fe, Al, Mn, Ni, Cd, and Pb was less than 1 (class 0), except PI for Fe, Mn, and Ni at the surface sediments of MA2, OB3, and KY1 higher than1 (Figures 6A–C, F). There was low to high contamination of Zn, Cu, Co, Cr, and Ga in the studied sites (Figures 6D, E, G–I). In the same manner, all studied sites were highly polluted with Indium (> 6) (Figure 6J).
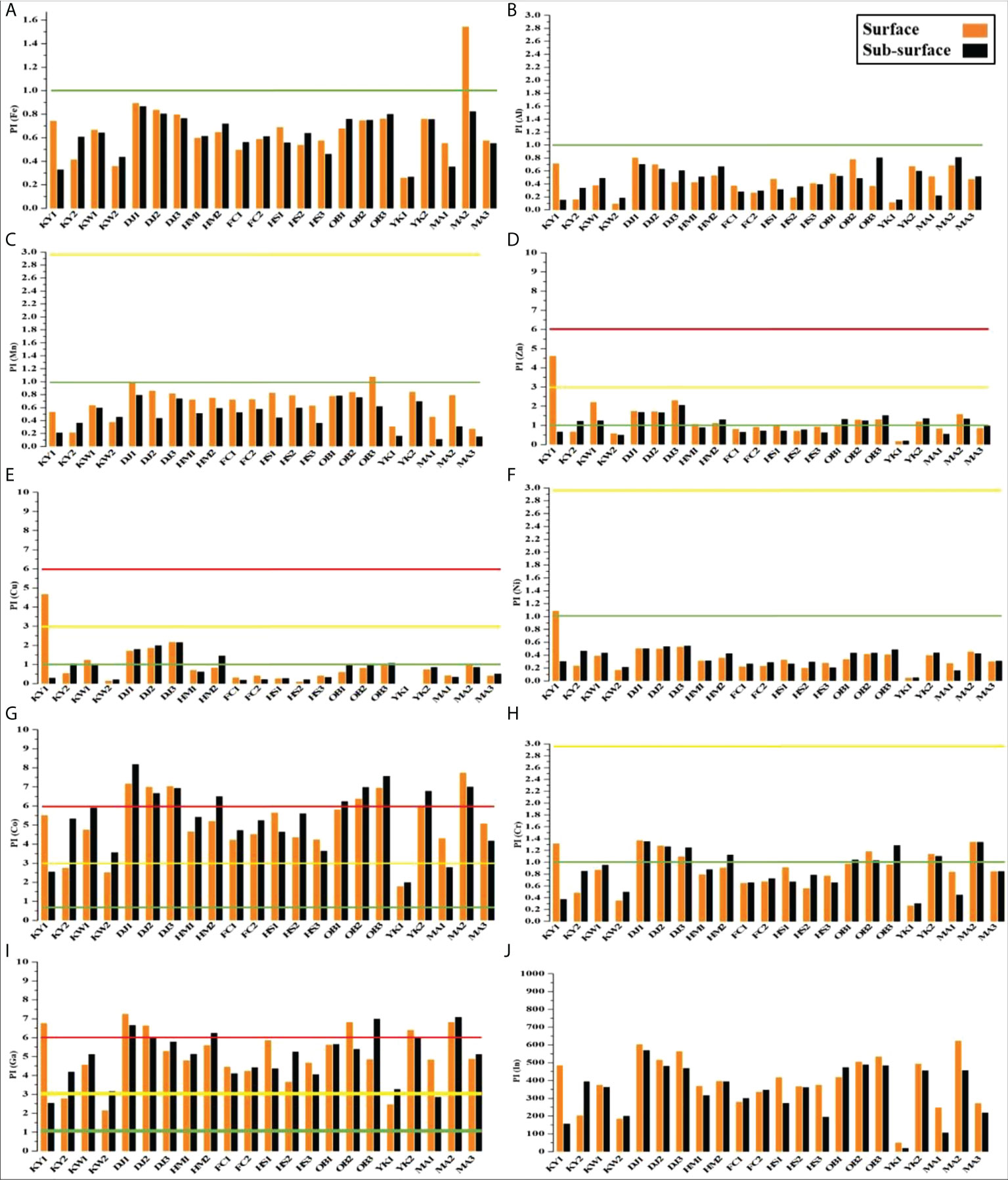
Figure 6 Pollution index (PI) with pollution degree for studied metals in surface and sub-surface sediments of Siangshan wetland. Green, yellow, and red lines refer to classes 0, 1, and 2, respectively (Table 1).
3.3.2.4 Pollution load index (PLI)
PLI was computed for the total content of heavy metals at the different sites as shown in Figure 7. High PLI values (class 2, > 2) were found in the surface sediments of KW1, DJ1, DJ2, DJ3, OB2, OB3, YK2, and MA2, but KY1 was subjected to class 3 (very strongly polluted). The remaining surface sediments were recorded as unpolluted or moderately polluted (class 0 and 1). Additionally, most sub-surface sediment sites are falling under class 1 (moderately polluted), except KW2 and YK1 are marked as unpolluted sites (class 0 < 1).
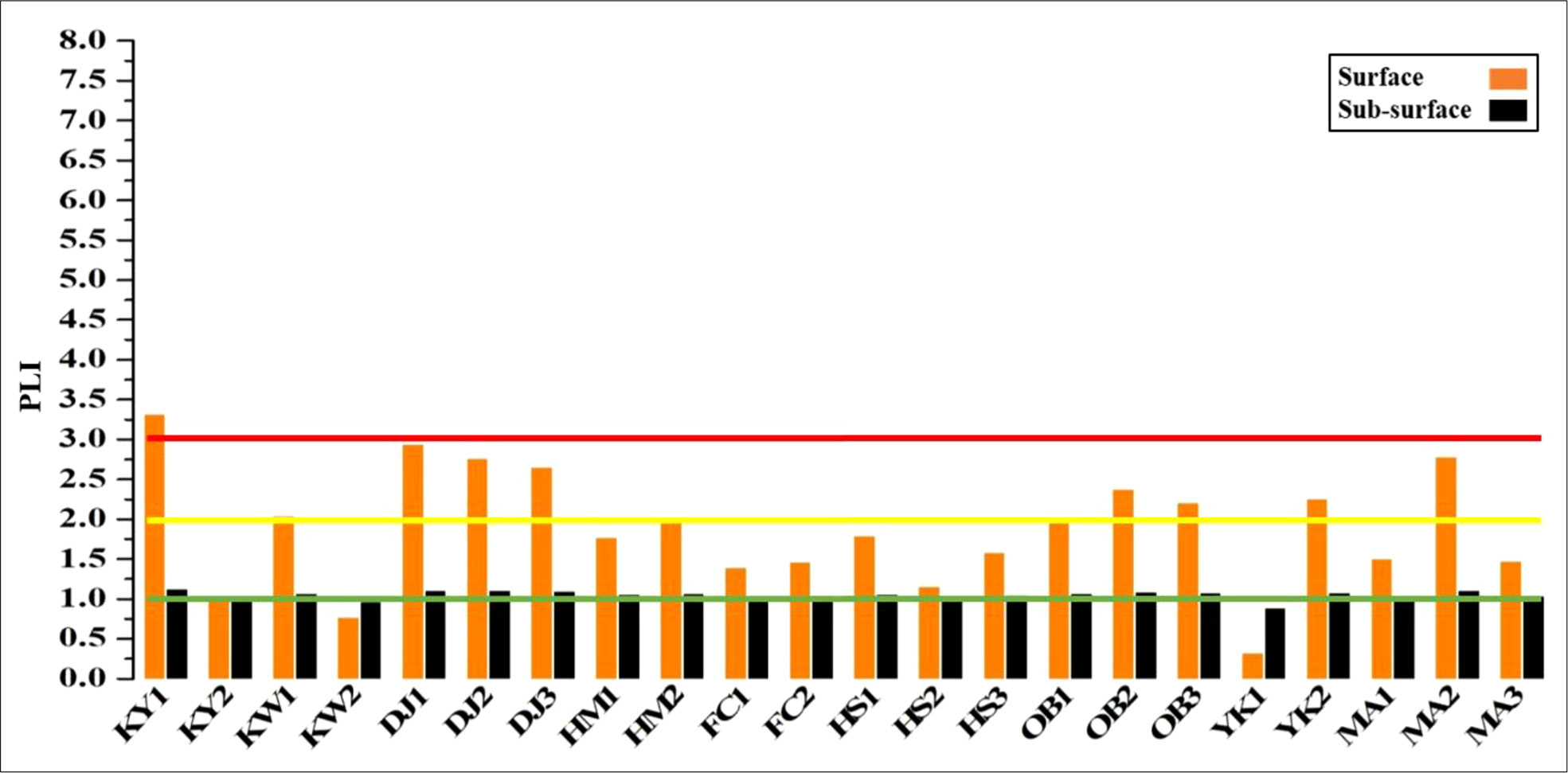
Figure 7 Pollution load index (PLI) with pollution degree for different studied sites in surface and sub-surface sediments of Siangshan wetland. Green, yellow, and red lines refer to classes 0, 1, and 2, respectively (Table 1).
3.4 Overview of high-throughput sequencing (HTS)
The PCR products of the sediment samples were sequenced by the Illumina MiSeq platform and resulted in 6,027,882 paired-end raw reads for 44 samples, out of which 5,496,315 met the quality criteria. The 971 potential chimeras were removed, and 4,075,656 (68% of the total count) non-chimeric high-quality reads were maintained after implementing rigorous quality control procedures (Table 3). The average number of qualified reads per sample was 92,629 (max = 145,870, min = 46,276). In the present study, high-quality reads that were kept after filtering requirements were clustered into 27,872 ASVs for 44 samples with an average of 633 ASVs per sample and were classified into 104 phyla, 227 classes, 573 orders, 1126 families, 2608 genera, and 3314 species. All rarefaction curves slant to reach the saturation plateau, proving that the NGS sequencing depth used in this work was adequate for characterizing microbiota community structure (Supplementary Figure 1).
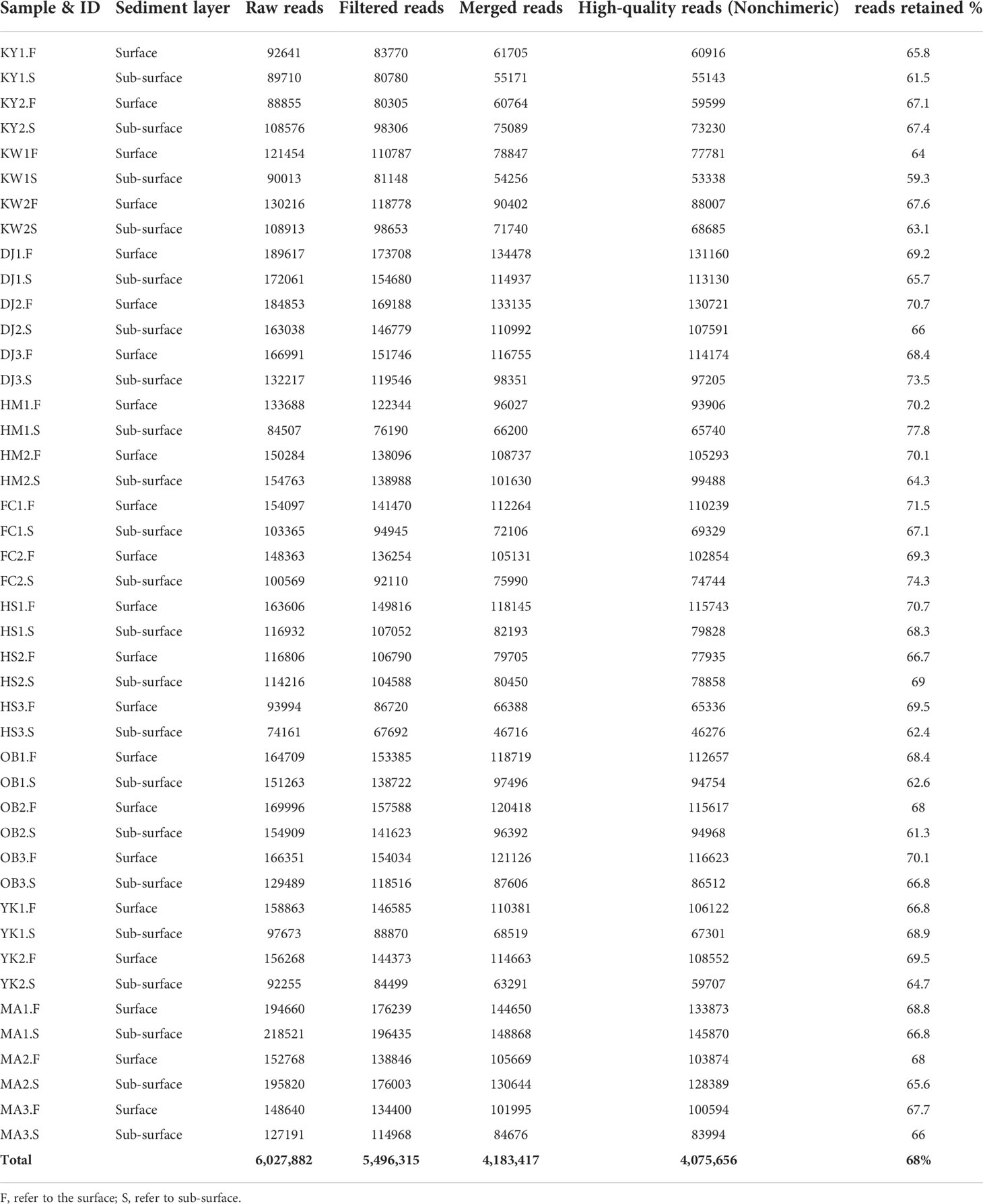
Table 3 Summary of bioinformatics analysis using DADA2 pipeline (raw reads count, filtered read count, merged, high-quality reads, and reads retained percent) at all studied sites.
3.5 Microbiota community diversity
To characterize the microbiota community structure in the Siangshan wetland’s sediments, we conducted the α-diversity and β-diversity analyses. These analyses were applied for normalized datasets using median sequencing depth (94,861). The observed ASVs (Sobs) and Chao1 indices represent microbiota richness, while the Shannon index reflects microbiota diversity. The microbiota communities within the Siangshan sediments at each site recorded relatively similar alpha diversity (Figure 8, Supplementary Table 7). Among the analyzed sediments, the highest richness (Sobs) was observed in DJ1.F (2571), followed by MA1.S (2536), DJ2.F (2459), and DJ3.F (2392), while the lowest richness showed in KW1.S (1083), KW2.S (1153), KY2.F (1214) as shown in Figure 8A. Moreover, the Chao1 index has a similar trend as the observed ASVs (Figure 8B). Results of Sobs and Chao1 were consistent with tendencies of the rarefaction curve (Supplementary Figure 1). The corresponding Shannon diversity index also displayed a narrow range, from 5.69 to 7.20 (Figure 8C). Overall, this study observed a minor difference in α-diversity among sites and lacked significance between the surface and sub-surface sediments (Student or Welch’s t-test, p > 0.05).
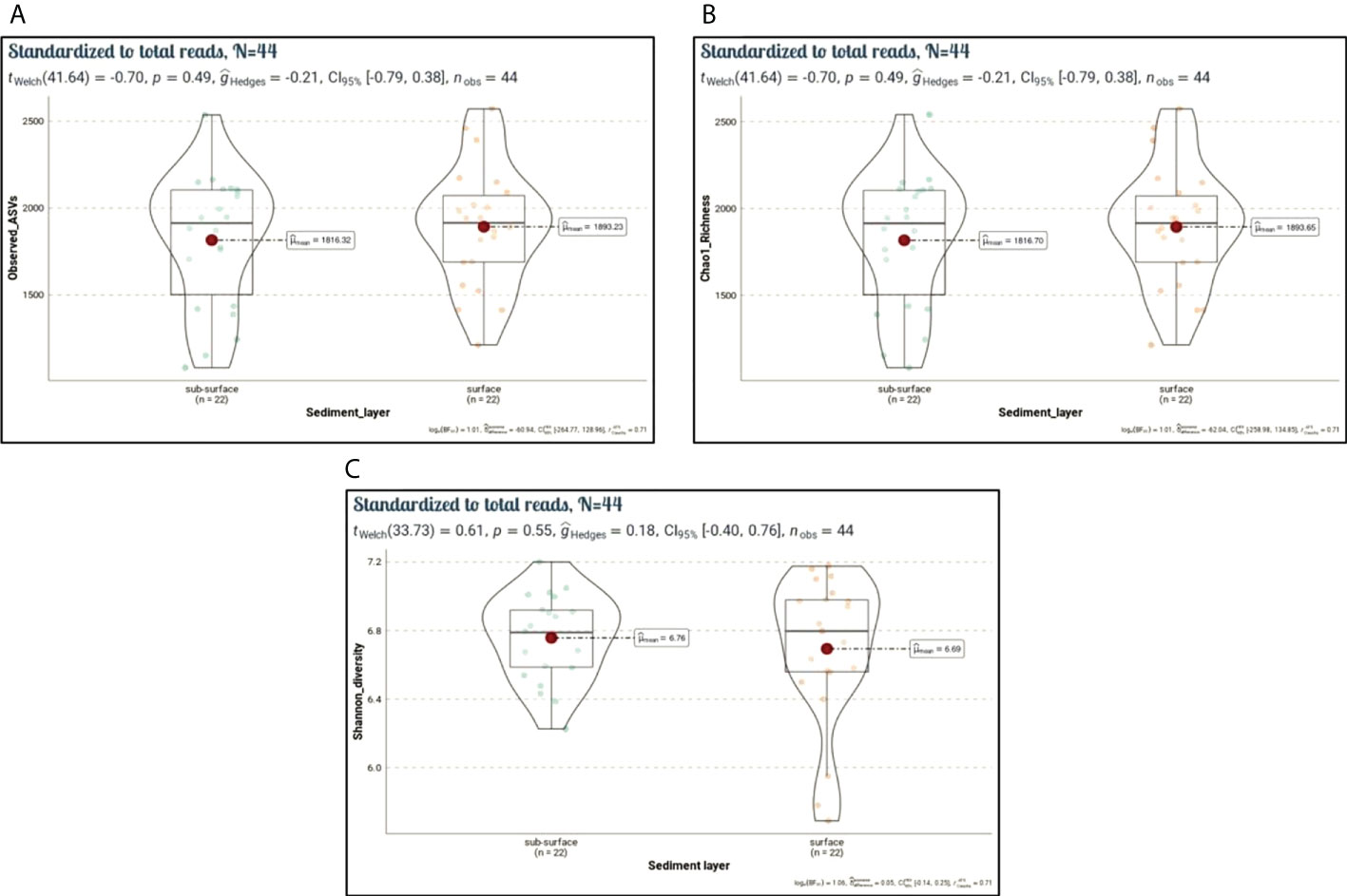
Figure 8 Alpha diversity indices (Sobs, Chao1, Shannon). Violin plots showing spatial differences in microbiota diversity among surface and sub-surface sediment sites (n = 44). (A) Number of observed species (Sobs, p = 0.49), (B) Chao1 richness (Chao1, p = 0.49), and (C) Shannon diversity index (p = 0.55). Statistical differences among surface and sub-surface sites were calculated using Student or Welch’s t-test.
In addition, β-diversity was conducted by a non-metric dimensional scale (nMDS) using Bray-Curtis distances to evaluate the dissimilarity between the microbiota communities. The nMDS ordination revealed significant differences in microbiota community composition grouping patterns between surface and sub-surface sites (Figure 9). The microbiota community at surface sites was similar and more clearly separated from the other, except for only 4 samples (KY1.F, KY2.F, MA2.F, and MA3.F). The analysis of similarity (ANOSIM) further confirmed that microbial community structure between the surface and sub-surface sediments groups was significantly dissimilar (ANOSIM with 999 iterations, R = 0.2526, p = 0.01).
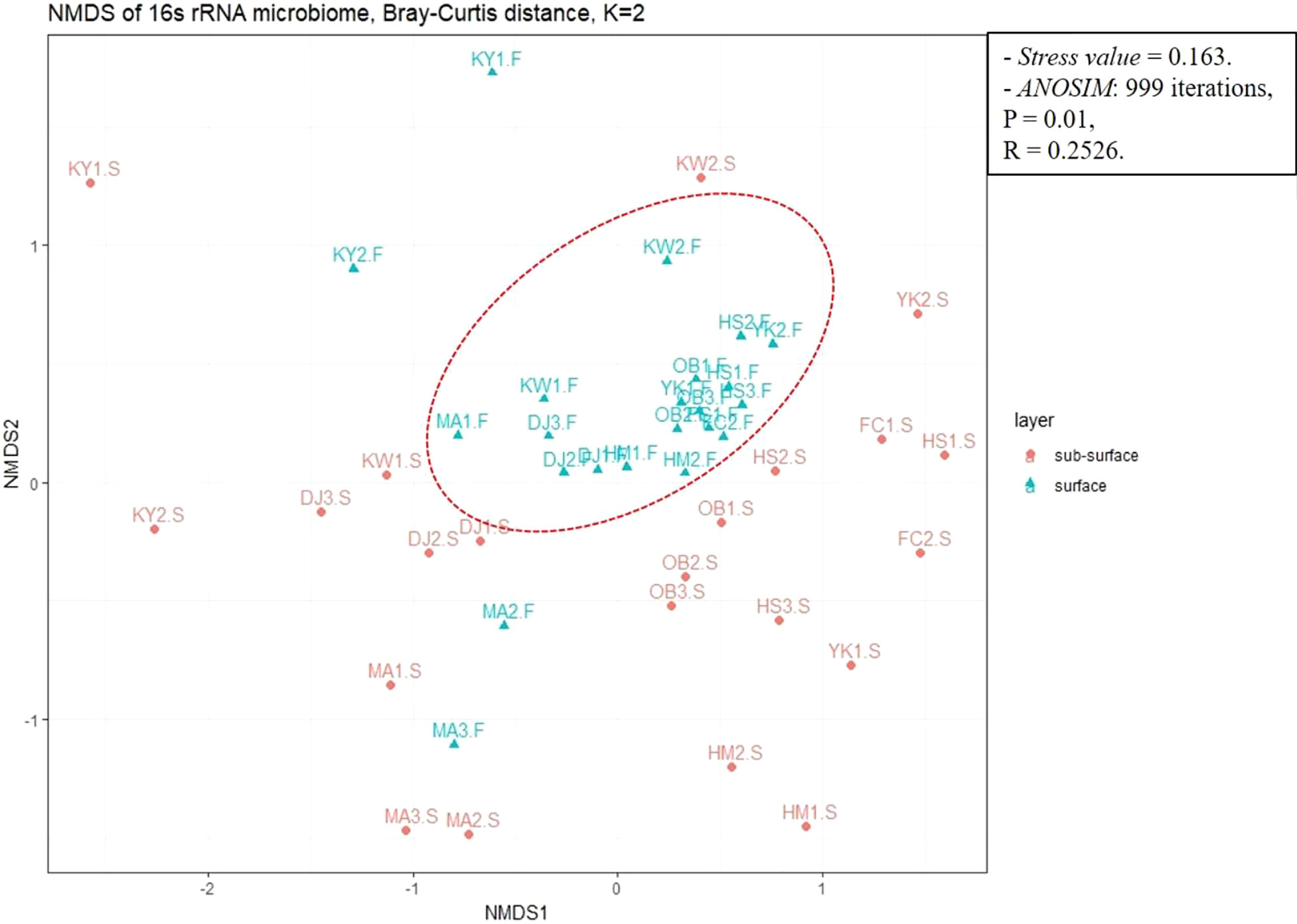
Figure 9 Beta diversity analysis showing the dissimilarity or distances between surface and sub-surface sites using non-metric dimensional scaling (nMDS) based on Bray-Curtis distance with stress value < 0.2 (0.163). Analysis of similarity (ANOSIM) was calculated to confirm the significant difference in microbiota community structure between sediment groups (p < 0.05).
3.6 Spatial distribution of microbiota community in sediments
In our study, the majority of the high-quality reads (~ 95.05%) were assigned to bacteria while about 4.83% were specified as archaea and 0.13% as unclassified. In total, 104 and 227 microbial phyla and classes, respectively were identified in the sediments. Of these phyla, 13 were considered dominant (> 1% of all sequences in all sites) (Figure 10A; Supplementary Table 8). The most abundant phylum across all sediment sites was Proteobacteria (38.02%, 1,460,037 reads). Bacteroidota was the second most abundant phylum (7.18%, 275,779 reads), followed by Desulfobacterota (6.97%, 267,806 reads), Actinobacterota (6.68%, 256,564 reads), Cyanobacteria (5.84%, 224,359 reads), Chloroflexota (4.18%, 160,703 reads), Planctomycetota (4.16%, 159,869 reads), and Firmicutes (3.69%, 141,774 reads). Other dominant phyla included Acidobacteriota, Gemmatimonadota, and Myxococcota, all of which contributed < 5% of overall community composition across all sites. Asgardarchaeota and Halobacteriota, both archaeal phyla, contributed 4.14% and 1.17%, respectively to microbiota community composition. Among the 18 most abundant classified classes, Gammaproteobacteria, Alphaproteobacteria, Bacteroidia, Cyanobacteria, Acidimicrobiia, Anaerolineae, Planctomycetes, Lokiarchaeia (Archaea), Desulfobacteria were the top 10 most abundant classes (exceeding 70% of total sequences) in all sediment sites (Figure 10B; Supplementary Table 8).
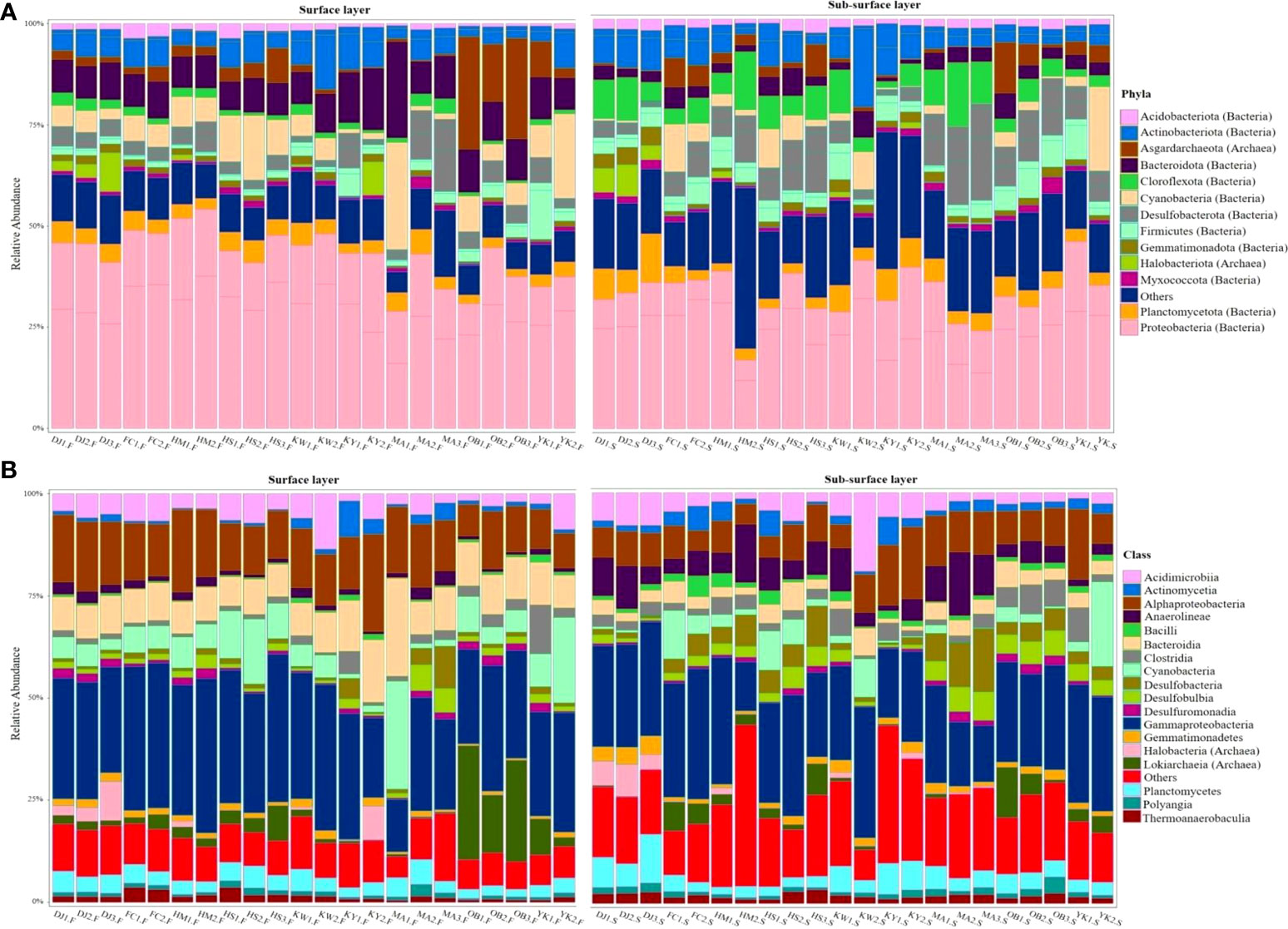
Figure 10 Stack bars illustrate the structure or composition of sediment-associated microbiota community with relative abundance > 1% across all sites at phylum (A) and class (B) levels.
Comparing the surface and sub-surface layer sediments in the Siangshan wetland, microbial phyla Proteobacteria, 42.31% (class Gammaproteobacteria and class Alphaproteobacteria), Cyanobacteria, 7.90% (class, Cyanobacteria), Bacteroidota, 10.12% (class Bacteroidia), and Asgardarchaeota, 5.52% (class Lokiarchaeia) were more abundant in most surface sediments, while Chloroflexota, 7.20% (class Anaerolineae), Desulfobacterota, 9.36% (class Desulfobacteria and class Desulfobulbia), Actinobacterota, 7.12% (class Acidimicrobiia and Actinomycetia) were more dominant in most sub-surface sediments (Supplementary Figure 2).
3.7 Influences of environmental variables on microbiota community structure
To elucidate the influences of environmental variables including heavy metals, grain particles, and organic matters on sediment-associated microbiota structure, redundancy analysis (RDA) was performed (Figure 11). 14 measured environmental variables and the most dominant microbiota (top 18 classes > 1% of all sequences across all sites) were subjected to RDA. The RDA showed two components of the graph jointly explained 47.9% (axis 1: 36.6% and axis 2: 11.3%) of the total variances of sediment microbiota community structure at the class level and the species-environment correlations were as high as 0.898 for the first axis and 0.801 for the second axis (Supplementary Table 10). Forward selection with the Monte Carlo permutation test revealed that 7 environmental variables including Mn, Cu, Al, Co, Ni, Sand, and Cr significantly influenced the structure of microbiota communities in Siangshan sediment sites (p < 0.05). Our results showed that Manganese (Mn) and Copper (Cu) with 14% and 13% contributions to the total explained distribution significantly (p < 0.05) were the most important variables that shaped the microbiota community composition. The remaining variables (Al, Co, Ni, Sand, and Cr) had relatively small contributions (Supplementary Table 9).
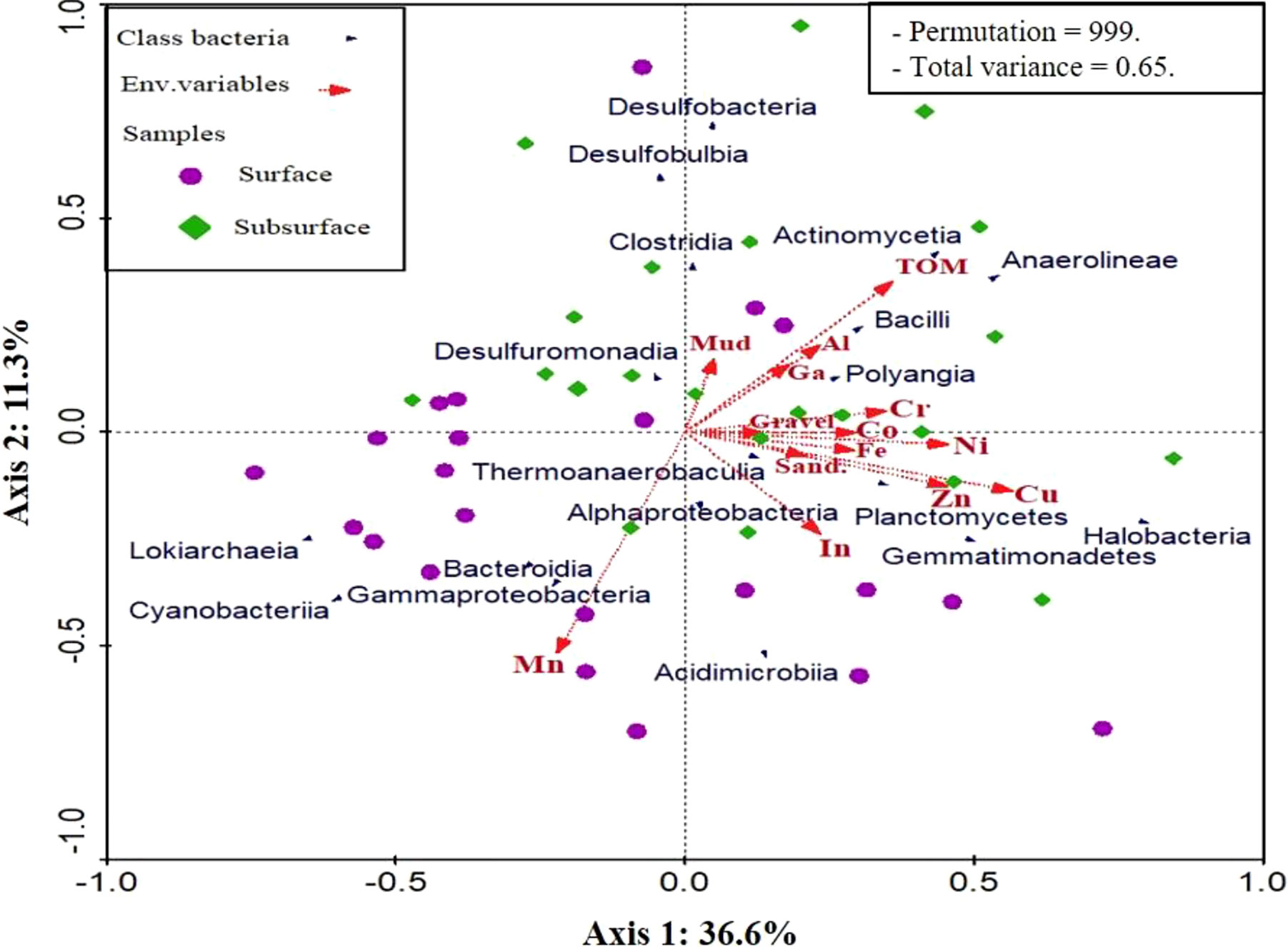
Figure 11 Redundancy analysis of the association among environmental variables (red arrows) and microbiota class distribution (blue arrows) across the surface and sub-surface. Statistical significances were determined by the Monte Carlo permutation test (p < 0.05).
4 Discussion
In this work, we explored the diversity and structural shifts in sediment-associated prokaryotic communities to elucidate the potential impacts of environmental variables including grain size, organic matter, and HMs in shaping microbiota community structure.
Generally, the Siangshan wetland was characterized by sandy or muddy sediments, but the gravel sediments recorded limited percentages at all studied sites. Based on the results of a grain size measurement, sand granules predominated in KY1, KW2, HS1, HS2, and YK1, whereas mud sediments were most plentiful in KW1, HS3, YK2, and MA3. Furthermore, the OB, DJ, HM, and FC areas’ surface sediments contained a high percentage of sand particles > 50%. The flow rate and velocity have a considerable effect on grain size (Kim et al., 2017). In fact, variations in the river channel direction and riverbed slope caused by manmade structures are related to changes in flow velocity and temporary stoppage of flowing streams (Kim et al., 2003b). The content of TOM was different between the sites we looked at in our research, where TOM was observed high percentage in the KY1 site’s surface sediments (10.76%). It could be ascribed to the presence of freshwater seepage from the Ke-Ya river, which has a high composition rate of organic elements. Furthermore, MA sites (MA1, MA2, MA3) had relatively significant concentrations at two sediment levels. Mangrove forests are regarded as a very productive ecosystem (Odum and Heald, 1975), with significant rates of organic carbon storage (Huc, 1980).
We then determined the heavy metal concentrations (Fe, Al, Mn, Zn, Cu, Ni, Cr, Co, Ga, In, Cd, and Pb) in Siangshan wetland’s sediments, and comprehensively assessed the pollution degree using different indices like; Enrichment factor (EF), Geo-accumulation index (Igeo), Pollution index (PI), and Pollution load index (PLI). The results indicated that the surface sediments of the KY1 site recorded the highest values of Zn, Cu, and Ni, and the maximum concentrations of Fe, Co, and In at MA2. Moreover, DJ1 showed the highest values of Al, Cr, and Ga, and OB3 for Mn. Inversely, YK1 recorded the lowest concentrations for most metals; Zn, Cu, Ni, Co, Cr, and In. While the DJ3 site’s sub-surface sediment recorded the highest concentrations for Zn, Cu, and Ni, as well as Fe, Mn, Co, Cr, and In recorded significant values in DJ1; Al and Ga in MA2. On the other hand, the YK1 site recorded the lowest concentrations for Fe, Zn, Cu, Ni, Co, Cr, and In; while Ga was observed at the KY1 site. The accumulation of heavy metals at these sites is due to the presence of a terrigenous freshwater supply, untreated residential sewage, and a large proportion of fine sediments that deposit with high organic matter content, which tend to be associated with heavy metals (Irvine and Birch, 1998; Yu et al., 2012; El-Metwally et al., 2017). Additionally, Siangshan sites are characterized by sandy and muddy sediments, hence the heavy metals were accumulated in high content at these sites due to the vast surface region and the availability of organic materials (Reddy et al., 2004).
In comparison with the previous studies (Supplementary Table 11), the total average concentrations of Fe (30582.95 mg/kg), Al (37036.41 mg/kg), Mn (501.18 mg/kg), and Cr (80.31 mg/kg) in the studied sites were more than those of the previous studies, but they were lower than the average concentration in shale (Turekian and Wedepohl, 1961). Contrary, Cd and Pb (BDL) were less than those in all previous studies, upper limits for natural background concentration in Taiwan (Lai et al., 2018), and average shale value (Turekian and Wedepohl, 1961). The overall mean of Zn (113.41 mg/kg) was close to the concentrations at the Nakdong River in Korea (Kim et al., 2020) and Main River & wetlands in Hsinchu of Taiwan (Ye and Young, 2008), while it lower than the concentrations of (Lai et al., 2018; Ma et al., 2018), but higher than those of other sites and the average shale value (Turekian and Wedepohl, 1961). However, the average concentration of Cu (40.18 mg/kg) was lower than those of Love River (Ma et al., 2018), the upper limit of natural background concentration in Taiwan (Lai et al., 2018), and Shale value, but higher than other previous studies. Additionally, Ni (24.70 mg/kg) was relatively similar to coastal areas of Bohai and Yellow sea, China (Tian et al., 2020), and the results of (Ye and Young, 2008), but lower than Lake Bafa in Turkey (Algül and Beyhan, 2020), Sfax solar saltern in Tunisia (Bahloul et al., 2018), Siangshan wetland, Taiwan (Young, 2019), the upper limit in Taiwan, and Shale value. Furthermore, Co (100.54 mg/kg), Ga (95.65 mg/kg), and In (36.85 mg/kg) were shown more than all the previous studies and average Shale values.
To evaluate sediment quality, we compared the overall average of HMs in sediments to sediment quality guidelines (SQGs). This is the most traditional method (El Nemr et al., 2016) and is particularly successful in assessing HM pollution in sediments (Lin et al., 2016; Duodu et al., 2017). In general, the SQGs have lower and upper limits for the frequency and severity of ecological effects, respectively. The reference values that were implemented by the Australian and New Zealand Environment and Conservation Council and the Agriculture and Resource Management Council of Australia and New Zealand (Anzecc, 2000) are analogous to the reference values that were established by the National Oceanic and Atmospheric Administration, also known as NOAA (Long et al., 1995). Those developed by the Canadian Council of Ministers of the Environment (CCME, 2002) and the Taiwan EPA (Taiwan, 2010) are comparable. Generally, the former two guidelines have relatively higher concentrations than the last two, implying that the last two have more rigorous limits for the SQGs strategy. According to our data, the total average of Zn and Ni concentrations surpassed the Taiwan EPA lower limit, NOAA ERL, and ANZECC & ARMCANZ low levels, although Cu exceeded NOAA ERL and CCME ISQG. Furthermore, Cr exceeded the CCME ISQG and Taiwan EPA’s low limit. on the other hand, Cd and Pb were below all of the different referenced values. In conclusion, except for Pb and Cd, the sediment averages of heavy metal concentrations in our work surpassed the low reference values in the various SQGs, indicating these metals have infrequent or occasional adverse effects on the aquatic population (Sarkar, 2018).
SQGs have been frequently employed in the evaluation of HMs contamination in sediments, although they do not include the background values. To more precisely assess HMs contamination in sediment, background values (Average Shale Values) of sediments must be considered. Hence, various quantitative indices (EF, Igeo, PI, and PLI) have been employed to identify the pollution degree of HMs in sediments. Based on EF values, Al, Mn, Ni, Cr, Cd, and Pb at all studied sites showed minimally enrichment (> 2) suggestive of no or minimal contamination, indicating that they are mostly originating from lithogenic or terrigenous processes. While EF values for Zn, Cu, Co, and Ga have minimal (< 2) to significant pollution (< 20).
The Igeo values for elements viz. Fe, Al, Mn, Zn, Cu, Ni, and Cr were marked as unpolluted, while Co and Ga range between class 0 and class 1 (unpolluted to very slightly polluted).
PI values for Fe, Al, and Mn were subjected to class 0 means that the studied sites were unpolluted with these metals, while PI values of Zn, Cu, Ni, Co, and Cr range from low to high polluted in different studied sites. Overall, EF, Igeo, and PI of Indium (In) recorded high values in all studied sites, indicating that the Siangshan sediments were highly or extremely polluted with this metal, this may be originating from an anthropogenic effluent like industrial seepage from Hsinchu Science Industrial Park. Gallium (Ga) and Indium (In) are critical transition metals utilized in liquid crystal displays (LCD), photovoltaic cells, semiconductors, light-emitting diodes (LED), and light extraction processes that cause deleterious effects on people (Ram et al., 2015; Rocchetti et al., 2015; Wang et al., 2015a; Lee et al., 2016; White et al., 2017). They are discharged into the intertidal zone during the production process such as epitaxy, cleaning activities, and chip preparation in the manufacturing of high-speed semiconductors and light-emitting diodes (Yang and Chen, 2018). Foregs Geochemical Atlas (2006) reported that Gallium content in stream sediments ranged from 1-36 mg/kg (Atlas, 2006). Also, Indium and Gallium have been discovered in the groundwater under the semiconductor fabrication areas of northern Taiwan (Chen, 2006; Hsu et al., 2011).
The surface sediments of KW1, DJ1, DJ2, DJ3, OB2, OB3, KY2, and MA2 were strongly polluted with studied metals based on PLI (> 2), while KY1 was very strongly polluted (PLI > 3) and the remaining sites were moderately polluted (PLI < 2). Inversely, most sub-surface sediment sites were moderately polluted with metals (PLI < 2) but KW2, and YK1 were unpolluted sites (PLI < 1).
Microbial communities play a vital role in the management of the marine environment, and the abundance or composition of these communities is largely influenced by environmental variables in the ecosystem. We determined 14 environmental variables influencing the microbiota communities in the Siangshan wetland.
High-throughput analysis of the 16S rRNA gene of microbial communities in all surface and sub-surface sites revealed that Proteobacteria (including Gammaproteobacteria and Alphaproteobacteria) are the most diverse and dominant that have a significant function in sediment decomposition and breakdown (Huang et al., 2019). Despite the studied sites being polluted with heavy metals, Proteobacteria were dominated. Compared to other phyla, Proteobacteria were highly abundant at the metal-contaminated sites may be attributed to the adaptation of its lower taxonomic groups to environmental changes (Bouskill et al., 2010). Moreover, Proteobacteria members are widely known for their capacity to tolerate high levels of metals (Guo et al., 2017; Chen et al., 2018). Proteobacteria are reported in both HMs contaminated and uncontaminated regions (Ma et al., 2015; Yan et al., 2016). Previous studies documented the response of Proteobacteria to HMs contamination in polluted habitats (Hu et al., 2021; Liu et al., 2021b; Serrana and Watanabe, 2022). Bacteroidota (class Bacteroidia) and Cyanobacteria (class Cyanobacteria) were more abundant phyla in surface sediments of MA1.F with relative abundances of 23.91% and 26.40%, respectively. Similarly, Asgardarchaeota (class Lokiarchaeia) were a more enriched phylum in surface sediments of OB1.F (27.84%) than in sub-surface sediments. Inversely, Desulfobacterota (represented in 3 classes Desulfobacteria, Desulfobulbia, Desulfuromonadia) and Chloroflexota (including class Anaerolineae) were more abundant phyla in sub-surface sediments, especially in MA3.S and MA2.S sites, respectively. Other important phyla observed in this study are, Actinobacterota, Planctomycetota, Acidobacteriota, Gemmatimonadota, Myxococcota, and Halobacteriota (Archaea) as the most abundant. All the abundant microbial phyla obtained in this study have been documented previously in other metal-contaminated environments, such as sediments (Lee et al., 2021; Rajeev et al., 2021; Yi et al., 2021; Custodio et al., 2022), iron tailing pond (Li et al., 2021), mining soils (Cao et al., 2017; Hemmat-Jou et al., 2018; Liu et al., 2021a), paddy soils (Tseng et al., 2021), arid loess region (Zeng et al., 2020), and electric waste soils (Jiang et al., 2019), this suggests that these phyla are associated with metal-polluted sediment.
Generally, the investigation of microbiota community composition revealed that there was variance among these communities, with ecological considerations responsible for a sizable proportion of the variation. This finding is corroborated by 16S rDNA gene sequencing, which observed that these environmental contaminations are also associated with substantial increases in ASVs within the phyla Proteobacteria, Bacteroidota, Actinobacterota, Chloroflexota, Planctomycetota, Acidobacteriota, and Verrucomicrobia (Beattie et al., 2018).
Human activities may cause shifts in microbiota diversity and structure (Wu et al., 2017). Based on the calculated pollution load index, most of the studied sites are polluted with heavy metals. Of these sites, DJ1.F, MA1.S, DJ2.F, and DJ3.F were moderate to strong polluted with heavy metals. Interestingly, these sites had more richness (Sobs and Chao1 indices) and diversity (Shannon diversity index) than other sites. The well-developed adaptation capacity of microbial communities and the effectiveness of metal detoxification by exploiting sequestering and precipitation processes may be the causes of higher richness in polluted sites (Rajeev et al., 2021). A pertinent study found that polluted sites have more biodiversity than unpolluted sites (Tao et al., 2017). Prolonged exposure of microorganisms to heavy metal contamination has different impacts on microbiota diversity, and over time, microorganisms progressively build up resistance to metals (Xu and Tang, 2018). The ability of the microbial community to tolerate contaminants through detoxifying mechanisms may enable it to survive in contaminated environments (Bourceret et al., 2016). Nevertheless, other studies have revealed that polluted regions by HMs had markedly lower levels of diversity and the structure or composition of sedimentary microbiota was shifted (Hemme et al., 2010; Frossard et al., 2017; Huaidong et al., 2017; Feng et al., 2018). Here, statistical analysis revealed there were no discernible variations in the microbial diversity and richness of the surface and sub-surface sediments (P > 0.05).
At the same time, the nMDS ordination exhibited short distances and similarities between the surface sites, except KY1.F, KY2.F, MA2.F, and MA3.F. The results illustrated that the sediment-associated microbiota community compositions are significantly dissimilar among surface and sub-surface sediment groups (ANOSIM with 999 iterations, R = 0.2526, p = 0.01).
RDA analysis was performed to further explore the environmental variables that may contribute to microbial community variances between sediment sites. In the present study, Mn and Cu were identified as the most crucial factors (F = 8.07 and p = 0.001; F = 6.04 and p = 0.002, respectively), followed by Al, Co, Ni, Sand, and Cr influencing the microbial community structure. This is consistent with previous studies in the Monkey Head iron tailing pond (Li et al., 2021), contaminated sediments in India (Rajeev et al., 2021), and the arid loess region (Zeng et al., 2020). Nevertheless, earlier studies contradicted our findings since heavy metals in aquatic sediment have minimal or no association with the composition of the microbial community (Gillan et al., 2005; Gough et al., 2008). Noteworthy it is critical to clarify that the majority of these works relied on traditional molecular methods like direct cell counts or denaturing gradient gel electrophoresis (DGGE) that primarily identify dominant bacterial communities, as result, have the potential to underestimate or misunderstand the structure of the community (Casamayor et al., 2000). Other studies have shown that the effluences of heavy metals on the abundance, structure, and composition of microbiota communities involve several fundamental mechanisms including (i) disrupting replication processes by destroying DNA and protein (Yan et al., 2020; Coclet et al., 2021) (ii) produce harmful reactive oxygen species by acting as redox catalysts, (iii) disrupting enzymatic processes, and (iv) disturbance of the membrane flexibility and ion regulation (Prabhakaran et al., 2016; Igiri et al., 2018). Indeed, the impacts of heavy metals on the communities of sedimentary microbiota are multifaceted. Initially, only bioavailable metals pose a threat to living things (Kunito et al., 1999). Secondly, the level of exposure determines how heavy metals affect microbial populations. Metals either cause a noticeable shift in microbial population and diversity in a short period of time (1 year) (Bouskill et al., 2010) or the diversity of the community remains steady after around a century of pollution, during which susceptible communities dwindle and the impervious population prevails (Reis et al., 2013).
It is worth noting that the current study only analyzed the structural diversity of the sediment-associated microbiota communities; thus, future research should identify the specific functional genes and associated biochemical functions in the communities of microbiota using PICRUSt or Tax4Fun functional predictions and Biolog analyses.
5 Conclusion
This is the first study that utilized eDNA metagenomic analysis to examine the microbial diversity in sediments of Taiwan’s Siangshan wetland. The obtained findings concluded that EF, Igeo, and PI of Indium (In) recorded high values in all studied sites, indicating that the Siangshan sediments were highly or extremely polluted with this metal, this may be originating from an anthropogenic effluent like industrial seepage of Hsinchu Science Industrial Park. A comparison of the metal concentrations with the SQGs of Taiwan, USA, Australia & New Zealand, and Canada revealed that pollution surpassed the low reference values indicating these metals have infrequent or occasional adverse effects on the aquatic population. Moreover, we applied the eDNA metagenomic approach to investigate the structure and composition of microbiota community. Study sites were dominated largely by Proteobacteria, followed by Bacteroidota, Desulfobacterota, Actinobacterota, Cyanobacteria, Chloroflexota, Planctomycetota, and Firmicutes. NGS sequencing results and alpha diversity indices demonstrated that the diversity of contaminated sites such as DJ1.F, MA1.S, DJ2.F, and DJ3.F was significantly higher than those of uncontaminated sites. The RDA analysis explored that Mn, Cu, Al, Co, Ni, Sand, and Cr had a sizable effect on the structure of microbiota communities (at the class level). Our findings provide a positive glimpse into the potential of eDNA metagenomic to define sediment-associated microbial communities and further the understanding of community structure and composition.
Data availability statement
The datasets presented in this study can be found in online repositories. The names of the repository/repositories and accession number(s) can be found below: https://www.ncbi.nlm.nih.gov/- PRJNA836729.
Author contributions
AS-T conceptualized the project, gathered samples, performed methodology, bioinformatics & statistical analyses, and manuscript writing. C-SC participated in the fieldwork, laboratory equipment setup, and sample sequencing. M-YL reviewed and revised the manuscript. S-SY conceptualized the study, sample gathering, review and revision of the article, supervision, and funding. All the authors contributed to the article and approved the submitted version.
Conflict of interest
The authors declare that the research was conducted in the absence of any commercial or financial relationships that could be construed as a potential conflict of interest.
Publisher’s note
All claims expressed in this article are solely those of the authors and do not necessarily represent those of their affiliated organizations, or those of the publisher, the editors and the reviewers. Any product that may be evaluated in this article, or claim that may be made by its manufacturer, is not guaranteed or endorsed by the publisher.
Supplementary material
The Supplementary Material for this article can be found online at: https://www.frontiersign.org/articles/10.3389/fmars.2022.990428/full#supplementary-material
References
Ahmad M., Islam S., Rahman S., Haque M., Islam M. (2010). Heavy metals in water, sediment and some fishes of Buriganga river, Bangladesh. Int. J. Environ. Res. 4 (2), 321–332. doi: 10.1038/s41598-020-68833-2
Algül F., Beyhan M. (2020). Concentrations and sources of heavy metals in shallow sediments in lake Bafa, Turkey. Scientific reports. Sci. Rep. 10, (1), 1–12. doi: 10.1038/s41598-020-68833-2
Ali U., Malik R. N., Syed J. H., Mehmood C., Sánchez-García L., Khalid A., et al. (2015). Mass burden and estimated flux of heavy metals in Pakistan coast: Sedimentary pollution and eco-toxicological concerns. Environ. Sci. Pollut. Res. 22 (6), 4316–4326. doi: 10.1007/s11356-014-3612-2
Allen-Gil S. M., Gubala C. P., Landers D. H., Lasorsa B. K., Crecelius E. A., Curtis L. R. (1997). Heavy metal accumulation in sediment and freshwater fish in US Arctic lakes. Environ. Toxicol. Chemistry: Int. J. 16 (4), 733–741. doi: 10.1002/etc.5620160418
Alves R. I., Sampaio C. F., Nadal M., Schuhmacher M., Domingo J. L., Segura-Muñoz S. I. (2014). Metal concentrations in surface water and sediments from Pardo river, Brazil: Human health risks. Environ. Res. 133, 149–155. doi: 10.1016/j.envres.2014.05.012
Anzecc A. (2000). Australian And new Zealand guidelines for fresh and marine water quality. Aust. New Z. Environ. Conserv. Council Agric. Resource Manage Council Aust:New Zealand Canberra 1, 1–314. Available at: https://www.environment.gov.au/system/files/resources/e080174c-b267-455e-a8dbd3f79e3b2142/files/nwqmsguidelines-4-vo13.pdf
Atlas F. G. (2006) Ga & in. Available at: http://weppi.gtk.fi/publ/foregsatlas/text.
Bahloul M., Baati H., Amdouni R., Azri C. (2018). Assessment of heavy metals contamination and their potential toxicity in the surface sediments of sfax solar saltern, Tunisia. Environ. Earth Sci. 77 (1), 1–22. doi: 10.1007/s12665-018-7227-7
Bai J., Cui B., Chen B., Zhang K., Deng W., Gao H., et al. (2011). Spatial distribution and ecological risk assessment of heavy metals in surface sediments from a typical plateau lake wetland, China. Ecol. Model. 222 (2), 301–306. doi: 10.1016/j.ecolmodel.2009.12.002
Barlas N., Akbulut N., Aydoğan M. (2005). Assessment of heavy metal residues in the sediment and water samples of uluabat lake, Turkey. Bull. Environ. Contamination Toxicol. 74 (2), 286–293. doi: 10.1007/s00128-004-0582-y
Barut I. F., Ergin M., Meriç E., Avşar N., Nazik A., Suner F. (2018). Contribution of natural and anthropogenic effects in the iznik lake bottom sediment: Geochemical and microfauna assemblages evidence. Quaternary Int. 486, 129–142. doi: 10.1016/j.quaint.2017.10.026
Bastami K. D., Bagheri H., Haghparast S., Soltani F., Hamzehpoor A., Bastami M. D. (2012). Geochemical and geo-statistical assessment of selected heavy metals in the surface sediments of the gorgan bay, Iran. Mar. Pollut. Bull. 64 (12), 2877–2884. doi: 10.1016/j.marpolbul.2012.08.015
Bates S. T., Berg-Lyons D., Caporaso J. G., Walters W. A., Knight R., Fierer N. (2011). Examining the global distribution of dominant archaeal populations in soil. ISME J. 5 (5), 908–917. doi: 10.1038/ismej.2010.171
Beattie R. E., Henke W., Campa M. F., Hazen T. C., McAliley L. R., Campbell J. H. (2018). Variation in microbial community structure correlates with heavy-metal contamination in soils decades after mining ceased. Soil Biol. Biochem. 126, 57–63. doi: 10.1016/j.soilbio.2018.08.011
Bergmann G. T., Bates S. T., Eilers K. G., Lauber C. L., Caporaso J. G., Walters W. A., et al. (2011). The under-recognized dominance of verrucomicrobia in soil bacterial communities. Soil Biol. Biochem. 43, 1450–1455. doi: 10.1016/j.soilbio.2011.03.012
Bourceret A., Cébron A., Tisserant E., Poupin P., Bauda P., Beguiristain T., et al. (2016). The bacterial and fungal diversity of an aged PAH-and heavy metal-contaminated soil is affected by plant cover and edaphic parameters. Microbial Ecol. 71 (3), 711–724. doi: 10.1007/s00248-015-0682-8
Bouskill N. J., Barker-Finkel J., Galloway T. S., Handy R. D., Ford T. E. (2010). Temporal bacterial diversity associated with metal-contaminated river sediments. Ecotoxicology 19 (2), 317–328. doi: 10.1007/s10646-009-0414-2
Callahan B. J., McMurdie P. J., Holmes S. P. (2017). Exact sequence variants should replace operational taxonomic units in marker-gene data analysis. ISME J. 11 (12), 2639–2643. doi: 10.1038/ismej.2017.119
Callahan B. J., McMurdie P. J., Rosen M. J., Han A. W., Johnson A. J. A., Holmes S. P. (2016). DADA2: High-resolution sample inference from illumina amplicon data. Nat. Methods 13 (7), 581–583. doi: 10.1038/nmeth.3869
Cao X., Diao M., Zhang B., Liu H., Wang S., Yang M. (2017). ). spatial distribution of vanadium and microbial community responses in surface soil of panzhihua mining and smelting area, China. Chemosphere 183, 9–17. doi: 10.1016/j.chemosphere.2017.05.092
Caporaso J. G., Lauber C. L., Walters W. A., Berg-Lyons D., Lozupone C. A., Turnbaugh P. J., et al. (2011). Global patterns of 16S rRNA diversity at a depth of millions of sequences per sample. Proc. Natl. Acad. Sci. 108 (supplement_1), 4516–4522. doi: 10.1073/pnas.1000080107
Casamayor E. O., Schaüfer H., Banãeras L., Pedroís-Alioí C., Muyzer ,. G. (2000). Identification of and spatio-temporal differences between microbial assemblages from two neighboring sulfurous lakes: Comparison by microscopy and denaturing gradient gel electrophoresis. Appl. Environ. Microbiol. 66 (2), 499–508. doi: 10.1128/AEM.66.2.499-508.2000
CCME (2002). Canadian water quality guidelines for the protection of aquatic life: Summary table. Canada:Canadian Council of Ministers of the Environment. Available at: http://www.ccme.ca/en/resources/canadian_environmental_quality_guidelines/
Chen H.-W. (2006). Gallium, indium, and arsenic pollution of groundwater from a semiconductor manufacturing area of Taiwan. Bull. Environ. Contamination Toxicol. 77 (2), 289–296. doi: 10.1007/s00128-006-1062-3
Chen Y., Jiang Y., Huang H., Mou L., Ru J., Zhao J., et al. (2018). Long-term and high-concentration heavy-metal contamination strongly influences the microbiome and functional genes in yellow river sediments. Sci. Total Environ. 637, 1400–1412. doi: 10.1016/j.scitotenv.2018.05.109
Chen C.-W., Kao C.-M., Chen C.-F., Dong C.-D. (2007). Distribution and accumulation of heavy metals in the sediments of Kaohsiung Harbor, Taiwan. Chemosphere 66 (8), 1431–1440. doi: 10.1016/j.chemosphere.2006.09.030
Clarke K. R. (1993). Non-parametric multivariate analyses of changes in community structure. Aust. J. Ecol. 18 (1), 117–143. doi: 10.1111/j.1442-9993.1993.tb00438.x
Coclet C., Garnier C., D’Onofrio S., Durrieu G., Pasero E., Le Poupon C., et al. (2021). Trace metal contamination impacts predicted functions more than structure of marine prokaryotic biofilm communities in an anthropized coastal area. Front. Microbiol. 12, 589948. doi: 10.3389/fmicb.2021.589948
Custodio M., Espinoza C., Peñaloza R., Peralta-Ortiz T., Sánchez-Suárez H., Ordinola-Zapata A., et al. (2022). Microbial diversity in intensively farmed lake sediment contaminated by heavy metals and identification of microbial taxa bioindicators of environmental quality. Sci. Rep. 12 (1), 1–12. doi: 10.1038/s41598-021-03949-7
Custodio M., Fow A., Peñaloza R., Chanamé F., Cano D. (2021). Evaluation of surface sediment quality in rivers with fish farming potential (Peru) using indicators of contamination, accumulation and ecological risk of heavy metals and arsenic. J. Ecol. Eng. 22 (5), 78–87. doi: 10.12911/22998993/135870
Daskalakis K. D., O'Connor T. P. (1995). Normalization and elemental sediment contamination in the coastal United States. Environ. Sci. Technol. 29 (2), 470–477. doi: 10.1021/es00002a024
David T.M.C.R.S., Obura O.. (2000). Coral reefs of the Indian Ocean: Their ecology and conservation New York, USA:Oxford University Press on Demand.
Deng W., Zhang A., Chen S., He X., Jin L., Yu X., et al. (2020). Heavy metals, antibiotics and nutrients affect the bacterial community and resistance genes in chicken manure composting and fertilized soil. J. Environ. Manage. 257, 109980. doi: 10.1016/j.jenvman.2019.109980
Di Bella J. M., Bao Y., Gloor G. B., Burton J. P., Reid G. (2013). High throughput sequencing methods and analysis for microbiome research. J. microbiological Methods 95 (3), 401–414. doi: 10.1016/j.mimet.2013.08.011
Di Cesare A., Pjevac P., Eckert E., Curkov N., Šparica M. M., Corno G., et al. (2020). The role of metal contamination in shaping microbial communities in heavily polluted marine sediments. Environ. Pollut. 265, 114823. doi: 10.1016/j.envpol.2020.114823
Duodu G. O., Ogogo K. N., Mummullage S., Harden F., Goonetilleke A., Ayoko G. A. (2017). Source apportionment and risk assessment of PAHs in Brisbane river sediment, Australia. Ecol. Indic. 73, 784–799. doi: 10.1016/j.ecolind.2016.10.038
Element C. (2007). Method 3051A microwave assisted acid digestion of sediments, sludges, soils, and oils. Z. Für Anal. Chem. 111, 362–366. Available at: https://www.jonesenv.com/PDF/3051a.pdf
El-Metwally M.E., Madkour A.G., Fouad R.R., Mohamedein L.I., Eldine H.A.N., Dar M.A, et al (2017). Assessment the leachable heavy metals and ecological risk in the surface sediments inside the Red Sea ports of Egypt. Int J of Mar Sci 7 (23), 214–228. doi: 10.5376/ijms.2017.07.0023.
El Nemr A., El-Said G. F., Ragab S., Khaled A., El-Sikaily A. (2016). The distribution, contamination and risk assessment of heavy metals in sediment and shellfish from the red Sea coast, Egypt. Chemosphere 165, 369–380. doi: 10.1016/j.chemosphere.2016.09.048
Ergin M., Saydam C., Baştürk Ö., Erdem E., Yörük R. (1991). Heavy metal concentrations in surface sediments from the two coastal inlets (Golden horn estuary and izmit bay) of the northeastern Sea of marmara. Chem. Geology 91 (3), 269–285. doi: 10.1016/0009-2541(91)90004-B
Esling P., Lejzerowicz F., Pawlowski J. (2015). Accurate multiplexing and filtering for high-throughput amplicon-sequencing. Nucleic Acids Res. 43 (5), 2513–2524. doi: 10.1093/nar/gkv107
Feng G., Xie T., Wang X., Bai J., Tang L., Zhao H., et al. (2018). Metagenomic analysis of microbial community and function involved in cd-contaminated soil. BMC Microbiol. 18 (1), 1–13. doi: 10.1186/s12866-018-1152-5
Fierer N., Hamady M., Lauber C. L., Knight R. (2008). The influence of sex, handedness, and washing on the diversity of hand surface bacteria. Proc. Natl. Acad. Sci. 105 (46), 17994–17999. doi: 10.1073/pnas.0807920105
Förstner U., Ahlf W., Calmano W., Kersten M. (1990). “Sediment criteria development,” in Sediments and environmental geochemistry (Berlin, Heidelberg, Germany:Springer), 311–338. doi: 10.1007/978-3-642-75097-7_18
Forstner U., Wittmann G. (1983). Toxic metal. metal pollution in aquatic environment (Jerman: Springer Verlag), 3–68.
Frossard A., Hartmann M., Frey B. (2017). Tolerance of the forest soil microbiome to increasing mercury concentrations. Soil Biol. Biochem. 105, 162–176. doi: 10.1016/j.soilbio.2016.11.016
Fu J., Zhao C., Luo Y., Liu C., Kyzas G. Z., Luo Y., et al. (2014). Heavy metals in surface sediments of the jialu river, China: Their relations to environmental factors. J. Hazardous Materials 270, 102–109. doi: 10.1016/j.jhazmat.2014.01.044
Gargouri D., Azri C., Serbaji M. M., Jedoui Y., Montacer M. (2011). Heavy metal concentrations in the surface marine sediments of sfax coast, Tunisia. Environ. Monit. Assess. 175 (1), 519–530. doi: 10.1007/s10661-010-1548-7
Gillan D. C., Danis B., Pernet P., Joly G., Dubois P. (2005). Structure of sediment-associated microbial communities along a heavy-metal contamination gradient in the marine environment. Appl. Environ. Microbiol. 71 (2), 679–690. doi: 10.1128/AEM.71.2.679-690.2005
Gough H. L., Dahl A. L., Nolan M. A., Gaillard J. F., Stahl D. A. (2008). Metal impacts on microbial biomass in the anoxic sediments of a contaminated lake. J. Geophysical Research: Biogeosciences 113 (G2), 1–10. doi: 10.1029/2007JG000566
Greweling T. (1960). Chemical soil tests (New York State College: Cornell University Agricultural Experiment Station).
Gümgüm B., Tez Z., Gülsün Z. (1994). Heavy metal pollution in water, sediment and fish from the Tigris river in Turkey. Chemosphere 29 (1), 111–116. doi: 10.1016/0045-6535(94)90094-9
Guo H., Nasir M., Lv J., Dai Y., Gao J. (2017). Understanding the variation of microbial community in heavy metals contaminated soil using high throughput sequencing. Ecotoxicology Environ. Saf. 144, 300–306. doi: 10.1016/j.ecoenv.2017.06.048
Hakanson L. (1980). An ecological risk index for aquatic pollution control. A sedimentological approach. Water Res. 14 (8), 975–1001. doi: 10.1016/0043-1354(80)90143-8
Han B.-C., Hung T.-C. (1990). Green oysters caused by copper pollution on the Taiwan coast. Environ. Pollut. 65 (4), 347–362. doi: 10.1016/0269-7491(90)90126-W
Hemmat-Jou M., Safari-Sinegani A., Mirzaie-Asl A., Tahmourespour (2018). Analysis of microbial communities in heavy metals-contaminated soils using the metagenomic approach. Ecotoxicology 27 (9), 1281–1291. doi: 10.1007/s10646-018-1981-x
Hemme C. L., Deng Y., Gentry T. J., Fields M. W., Wu L., Barua S., et al. (2010). Metagenomic insights into evolution of a heavy metal-contaminated groundwater microbial community. ISME J. 4 (5), 660–672. doi: 10.1038/ismej.2009.154
Herlemann D. P., Labrenz M., Jürgens K., Bertilsson S., Waniek J. J., Andersson A. F. (2011). Transitions in bacterial communities along the 2000 km salinity gradient of the Baltic Sea. ISME J. 5 (10), 1571–1579. doi: 10.1038/ismej.2011.41
Hsu S.-C., Hsieh H.-L., Chen C.-P., Tseng C.-M., Huang S.-C., Huang C.-H., et al. (2011). Tungsten and other heavy metal contamination in aquatic environments receiving wastewater from semiconductor manufacturing. J. Hazardous Materials 189 (1-2), 193–202. doi: 10.1016/j.jhazmat.2011.02.020
Huaidong H., Waichin L., Riqing Y., Zhihong Y. (2017). Illumina-based analysis of bulk and rhizosphere soil bacterial communities in paddy fields under mixed heavy metal contamination. Pedosphere 27 (3), 569–578. doi: 10.1016/S1002-0160(17)60352-7
Huang W., Chen X., Wang K., Chen J., Zheng B., Jiang X. (2019). Comparison among the microbial communities in the lake, lake wetland, and estuary sediments of a plain river network. Microbiologyopen 8 (2), e00644. doi: 10.1002/mbo3.644
Huc A. (1980). Origin and formation of organic matter in recent sediments and its relation to kerogen (Kerogen Insoluble Organic matter from sedimentary rocks), (pp.445–472).
Hu X., Wang J., Lv Y., Liu X., Zhong J., Cui X., et al. (2021). Effects of heavy metals/metalloids and soil properties on microbial communities in farmland in the vicinity of a metals smelter. Front. Microbiol. 2347. doi: 10.3389/fmicb.2021.707786
Igiri B. E., Okoduwa S. I., Idoko G. O., Akabuogu E. P., Adeyi A. O., Ejiogu I. K. (2018). Toxicity and bioremediation of heavy metals contaminated ecosystem from tannery wastewater: A review. J. Toxicol. 2018, 1–16. doi: 10.1155/2018/2568038
Iksandar I. K., Keeney D. R. (1974). Concentration of heavy metals in sediment cores from selected Wisconsin lakes. Environ. Sci. Technol. 8 (2), 165–170. doi: 10.1021/es60087a001
Irvine I., Birch G. (1998). Distribution of heavy metals in surficial sediments of Port Jackson, Sydney, New South Wales. Australian Journal of Earth Sciences 45 (2), 297–304
Jiang B., Adebayo A., Jia J., Xing Y., Deng S., Guo L., et al. (2019). Impacts of heavy metals and soil properties at a Nigerian e-waste site on soil microbial community. J. Hazardous Materials 362, 187–195. doi: 10.1016/j.jhazmat.2018.08.060
Kandeler E., Tscherko D., Bruce K., Stemmer M., Hobbs P. J., Bardgett R. D., et al. (2000). Structure and function of the soil microbial community in microhabitats of a heavy metal polluted soil. Biol. Fertility Soils 32 (5), 390–400. doi: 10.1007/s003740000268
Kim I. S., Kang K. H., Johnson-Green P., Lee E. J. (2003a). Investigation of heavy metal accumulation in polygonum thunbergii for phytoextraction. Environ. Pollut. 126 (2), 235–243. doi: 10.1016/S0269-7491(03)00190-8
Kim J.-Y., Koh Y.-K., Youn S.-T., Shin S.-E., Park B.-Y., Moon B.-C., et al. (2003b). Geochemical characteristics and contamination of surface sediments in streams of Gwangju City. J. Korean Earth Sci. Soc. 24 (4), 346–360.
Kim S., Lee K., Kim J., Jung K., Ahn J., Kim H., et al. (2017). Change in geochemical characteristics of surface sediments in the nakdong river main stream. J. Environ. Sci. Int. 26 (3), 311–324. doi: 10.5322/JESI.2017.26.3.311
Kim S., Yang D. S., Kim Y. S. (2020). Distribution of metal contamination and grain size in the sediments of nakdong river, Korea. Environ. Monit. Assess. 192 (8), 1–15. doi: 10.1007/s10661-020-08475-z
Kunito T., Saeki K., Oyaizu H., Matsumoto S. (1999). Influences of copper forms on the toxicity to microorganisms in soils. Ecotoxicology Environ. Saf. 44 (2), 174–181. doi: 10.1006/eesa.1999.1820
Lai Y. J., Chuang P. C., Lee Y. T., Hung Y. H., Huang T. H., Chen S. D., et al. (2018). Establishment and discussion of soil heavy metal background concentrations in Taiwan. J. Soil Groundwater Remediation 5 (3), 143–162. doi: 10.6499/JSGR.201807_5(3).0003
Lee S. L. (2020). Bird survey on siangshan wetland in 2020. government authorized project final report ( in Chinese) Vol. 106 (Taiwan:Hsinchu City Government, Wild Bird Society of Hsinchu).
Lee K. H., Chen H. L., Leung C. M., Chen H. P., Hsu P. C. (2016). Indium acetate toxicity in male reproductive system in rats. Environ. Toxicol. 31 (1), 68–76. doi: 10.1002/tox.22022
Lee H., Heo Y. M., Kwon S. L., Yoo Y., Kim D., Lee J., et al. (2021). Environmental drivers affecting the bacterial community of intertidal sediments in the yellow Sea. Sci. Total Environ. 755, 142726. doi: 10.1016/j.scitotenv.2020.142726
Lee P.-K., Kang M.-J., Yu S., Ko K.-S., Ha K., Shin S.-C., et al. (2017). Enrichment and geochemical mobility of heavy metals in bottom sediment of the hoedong reservoir, Korea and their source apportionment. Chemosphere 184, 74–85. doi: 10.1016/j.chemosphere.2017.05.124
Lepš J., Šmilauer P. (2003). Multivariate analysis of ecological data using CANOCO (Cambridge, UK:Cambridge university press). doi: 10.1017/CBO9780511615146
Liao H., Yen J. Y., Guan Y., Ke D., Liu C. (2020). Differential responses of stream water and bed sediment microbial communities to watershed degradation. Environ. Int. 134, 105198. doi: 10.1016/j.envint.2019.105198
Lin Q., Liu E., Zhang E., Li K., Shen J. (2016). Spatial distribution, contamination and ecological risk assessment of heavy metals in surface sediments of erhai lake, a large eutrophic plateau lake in Southwest China. Catena 145, 193–203. doi: 10.1016/j.catena.2016.06.003
Li C., Quan Q., Gan Y., Dong J., Fang J., Wang L., et al. (2020a). Effects of heavy metals on microbial communities in sediments and establishment of bioindicators based on microbial taxa and function for environmental monitoring and management. Sci. Total Environ. 749, 141555. doi: 10.1016/j.scitotenv.2020.141555
Liu J., Liu W., Zhang Y., Chen C., Wu W., Zhang T. C. (2021b). Microbial communities in rare earth mining soil after in-situ leaching mining. Sci. Total Environ. 755, 142521. doi: 10.1016/j.scitotenv.2020.142521
Liu Z., Lozupone C., Hamady M., Bushman F. D., Knight R. (2007). Short pyrosequencing reads suffice for accurate microbial community analysis. Nucleic Acids Res. 35 (18), e120. doi: 10.1093/nar/gkm541
Liu T.-t., Yang H. (2020). Comparative analysis of the total and active bacterial communities in the surface sediment of lake taihu. FEMS Microbiol. Ecol. 96 (5), fiaa059. doi: 10.1093/femsec/fiaa059
Liu B., Yao J., Ma B., Chen Z., Zhao C., Zhu X., et al. (2021a). Microbial community profiles in soils adjacent to mining and smelting areas: Contrasting potentially toxic metals and co-occurrence patterns. Chemosphere 282, 130992. doi: 10.1016/j.chemosphere.2021.130992
Liu J., Zhu S., Liu X., Yao P., Ge T., Zhang X.-H. (2020). Spatiotemporal dynamics of the archaeal community in coastal sediments: Assembly process and co-occurrence relationship. ISME J. 14 (6), 1463–1478. doi: 10.1038/s41396-020-0621-7
Li S., Wu J., Huo Y., Zhao X., Xue L. (2021). Profiling multiple heavy metal contamination and bacterial communities surrounding an iron tailing pond in Northwest China. Sci. Total Environ. 752, 141827. doi: 10.1016/j.scitotenv.2020.141827
Li S., Zhao B., Jin M., Hu L., Zhong H., He Z. (2020b). A comprehensive survey on the horizontal and vertical distribution of heavy metals and microorganisms in soils of a Pb/Zn smelter. J. Hazardous Materials 400, 123255. doi: 10.1016/j.jhazmat.2020.123255
Long E. R., MacDonald D. D., Smith S. L., Calder F. D. (1995). Incidence of adverse biological effects within ranges of chemical concentrations in marine and estuarine sediments. Environ. Manage. 19 (1), 81–97. doi: 10.1007/BF02472006
Maanan M., Saddik M., Maanan M., Chaibi M., Assobhei O., Zourarah B. (2015). Environmental and ecological risk assessment of heavy metals in sediments of nador lagoon, Morocco. Ecol. Indic. 48, 616–626. doi: 10.1016/j.ecolind.2014.09.034
Ma Z., Chen K., Yuan Z., Bi J., Huang L. (2013). Ecological risk assessment of heavy metals in surface sediments of six major Chinese freshwater lakes. J. Environ. Qual. 42 (2), 341–350. doi: 10.2134/jeq2012.0178
Ma Q., Qu Y.-Y., Zhang X.-W., Shen W.-L., Liu Z.-Y., Wang J.-W., et al. (2015). Identification of the microbial community composition and structure of coal-mine wastewater treatment plants. Microbiological Res. 175, 1–5. doi: 10.1016/j.micres.2014.12.013
Ma C.-Y., Sheu Y.-T., Hsia K.-F., Dong C.-D., Chen C.-W., Huang Y.-C., et al. (2018). Development of water and sediment quality management strategies for an urban river basin: A case study in Taiwan. J. Water Supply: Res. Technology-Aqua 67 (8), 810–823. doi: 10.2166/aqua.2018.084
McMurdie P. J., Holmes S. (2013). Phyloseq: An r package for reproducible interactive analysis and graphics of microbiome census data. PloS One 8 (4), e61217. doi: 10.1371/journal.pone.0061217
Needham D. M., Sachdeva R., Fuhrman J. A. (2017). Ecological dynamics and co-occurrence among marine phytoplankton, bacteria and myoviruses shows microdiversity matters. ISME J. 11 (7), 1614–1629. doi: 10.1038/ismej.2017.29
Odum W. E., Heald E. J. (1975). The detritus-based food web of an. Estuar. Res. Chem. Biol. Estuar. Syst. 1, 265.
Oksanen J., Blanchet F. G., Friendly M., Kindt R., Legendre P., McGlinn D., et al. (2019) CRAN-package vegan. Available at: https://cran.r-project.org/web/packages/vegan/index.html (Accessed 26 November 2021).
Parks D. H., Chuvochina M., Rinke C., Mussig A. J., Chaumeil P.-A., Hugenholtz P. (2022). GTDB: An ongoing census of bacterial and archaeal diversity through a phylogenetically consistent, rank normalized and complete genome-based taxonomy. Nucleic Acids Res. 50 (D1), D785–D794. doi: 10.1093/nar/gkab776
Patil I. (2021). Visualizations with statistical details: The'ggstatsplot'approach. J. Open Source Software 6 (61), 3167. doi: 10.21105/joss.03167
Peech M. (1947). Methods of soil analysis for soil-fertility investigation. US Dept. Agr. Circ. 757, 7–11.
Prabhakaran P., Ashraf M. A., Aqma W. S. (2016). Microbial stress response to heavy metals in the environment. Rsc Adv. 6 (111), 109862–109877. doi: 10.1039/C6RA10966G
Rajeev M., Sushmitha T., Aravindraja C., Toleti S. R., Pandian S. K. (2021). Exploring the impacts of heavy metals on spatial variations of sediment-associated bacterial communities. Ecotoxicology Environ. Saf. 209, 111808. doi: 10.1016/j.ecoenv.2020.111808
Ram L., Masto R., Srivastava N., George J., Selvi V., Das T., et al. (2015). Potentially toxic elements in lignite and its combustion residues from a power plant. Environ. Monit. Assess. 187 (1), 1–14. doi: 10.1007/s10661-014-4148-0
Reddy M.S., Basha S., Kumar V.S., Joshi H., Ramachandraiah G (2004). Distribution, enrichment and accumulation of heavy metals in coastal sediments of Alang–Sosiya ship scrapping yard, India. Mar Pollut Bulletin 48 (11-12), 1055–1059. doi: 10.1016/j.marpolbul.2003.12.011.
Reis M. P., Barbosa F. A., Chartone-Souza E., Nascimento A. (2013). The prokaryotic community of a historically mining-impacted tropical stream sediment is as diverse as that from a pristine stream sediment. Extremophiles 17 (2), 301–309. doi: 10.1007/s00792-013-0517-9
Rocchetti L., Amato A., Fonti V., Ubaldini S., De Michelis I., Kopacek B., et al. (2015). Cross-current leaching of indium from end-of-life LCD panels. Waste Manage. 42, 180–187. doi: 10.1016/j.wasman.2015.04.035
Sakan S. M., Đorđević D. S., Manojlović D. D., Predrag P. S. (2009). Assessment of heavy metal pollutants accumulation in the tisza river sediments. J. Environ. Manage. 90 (11), 3382–3390. doi: 10.1016/j.jenvman.2009.05.013
Salam M. A., Paul S. C., Shaari F. I., Rak A. E., Ahmad R. B., Kadir W. R. (2019). Geostatistical distribution and contamination status of heavy metals in the sediment of perak river, Malaysia. Hydrology 6 (2), 30. doi: 10.3390/hydrology6020030
Sarkar S. K. (2018). “Geochemical speciation and risk assessment of trace metals in sediments of sundarban wetland,” in Trace metals in a tropical mangrove wetland (Singapore:Springer), 145–172. doi: 10.1007/978-981-10-2793-2_6
Serrana J. M., Watanabe K. (2022). Sediment-associated microbial community profiling: sample pre-processing through sequential membrane filtration for 16S rRNA amplicon sequencing. BMC Microbiol. 22 (1), 1–14. doi: 10.1186/s12866-022-02441-0
Steyaert M., Priestley V., Osborne O., Herraiz A., Arnold R., Savolainen V. (2020). Advances in metabarcoding techniques bring us closer to reliable monitoring of the marine benthos. J. Appl. Ecol. 57 (11), 2234–2245. doi: 10.1111/1365-2664.13729
Sutherland R. (2000). Bed sediment-associated trace metals in an urban stream, Oahu, Hawaii. Environ. Geology 39 (6), 611–627. doi: 10.1007/s002540050473
Taiwan E. (2010). Soil and groundwater pollution remediation act (Taipei, Taiwan: Taiwan Environmental Protection Administration).
Tao R., Wakelin S. A., Liang Y., Chu G. (2017). Response of ammonia-oxidizing archaea and bacteria in calcareous soil to mineral and organic fertilizer application and their relative contribution to nitrification. Soil Biol. Biochem. 114, 20–30. doi: 10.1016/j.soilbio.2017.06.027
Tavakoly Sany S. B., Hashim R., Rezayi M., Salleh A., Safari O. (2014). A review of strategies to monitor water and sediment quality for a sustainability assessment of marine environment. Environ. Sci. Pollut. Res. 21 (2), 813–833. doi: 10.1007/s11356-013-2217-5
Thies J. E. (2015). “Molecular approaches to studying the soil biota,” in Soil microbiology, ecology, and biochemistry. 151–185. doi: 10.1016/B978-0-12-415955-6.00006-2
Tian K., Huang B., Xing Z., Hu W. (2017). Geochemical baseline establishment and ecological risk evaluation of heavy metals in greenhouse soils from dongtai, China. Ecol. Indic. 72, 510–520. doi: 10.1016/j.ecolind.2016.08.037
Tian K., Wu Q., Liu P., Hu W., Huang B., Shi B., et al. (2020). Ecological risk assessment of heavy metals in sediments and water from the coastal areas of the bohai Sea and the yellow Sea. Environ. Int. 136, 105512. doi: 10.1016/j.envint.2020.105512
Tomlinson D., Wilson J., Harris C., Jeffrey D. (1980). Problems in the assessment of heavy-metal levels in estuaries and the formation of a pollution index. Helgoländer meeresuntersuchungen 33 (1), 566–575. doi: 10.1007/BF02414780
Tseng S.-c., Liang C.-m., Chia T., Ton S.-s. (2021). Changes in the composition of the soil bacterial community in heavy metal-contaminated farmland. Int. J. Environ. Res. Public Health 18 (16), 8661. doi: 10.3390/ijerph18168661
Turekian K. K., Wedepohl K. H. (1961). Distribution of the elements in some major units of the earth's crust. Geological Soc. America Bull. 72 (2), 175–192. doi: 10.1130/0016-7606(1961)72[175:DOTEIS]2.0.CO;2
Ul-Hasan S., Bowers R. M., Figueroa-Montiel A., Licea-Navarro A. F., Beman J. M., Woyke T., et al. (2019). Community ecology across bacteria, archaea and microbial eukaryotes in the sediment and seawater of coastal Puerto Nuevo, Baja California. PloS One 14 (2), e0212355. doi: 10.1371/journal.pone.0212355
Vareda J. P., Valente A. J., Durães L. (2019). Assessment of heavy metal pollution from anthropogenic activities and remediation strategies: A review. J. Environ. Manage. 246, 101–118. doi: 10.1016/j.jenvman.2019.05.126
Walker C., Goodyear C., Anderson D., Titball R. (2000). Identification of arsenic-resistant bacteria in the soil of a former munitions factory at löcknitz, Germany. Land Contamination Reclamation 8 (1), 13–18.
Walkley A. (1947). A critical examination of a rapid method for determining organic carbon in soils–effect of variations in digestion conditions and of inorganic soil constituents. Soil Sci. 63 (4), 251–264. doi: 10.1097/00010694-194704000-00001
Wang H., Gu Y., Wu Y., Zhang Y.-N., Wang W. (2015a). An evaluation of the potential yield of indium recycled from end-of-life LCDs: A case study in China. Waste Manage. 46, 480–487. doi: 10.1016/j.wasman.2015.07.047
Wang Y., Hu J., Xiong K., Huang X., Duan S. (2012). Distribution of heavy metals in core sediments from baihua lake. Proc. Environ. Sci. 16, 51–58. doi: 10.1016/j.proenv.2012.10.008
Wang Y.-M., Kinraide T. B., Wang P., Zhou D.-M., Hao X.-Z. (2013). Modeling rhizotoxicity and uptake of zn and Co singly and in binary mixture in wheat in terms of the cell membrane surface electrical potential. Environ. Sci. Technol. 47 (6), 2831–2838. doi: 10.1021/es3022107
Wang Y., Yang L., Kong L., Liu E., Wang L., Zhu J. (2015b). Spatial distribution, ecological risk assessment and source identification for heavy metals in surface sediments from dongping lake, Shandong, East China. Catena 125, 200–205. doi: 10.1016/j.catena.2014.10.023
Wang F., Yao J., Si Y., Chen H., Russel M., Chen K., et al. (2010). Short-time effect of heavy metals upon microbial community activity. J. Hazardous Materials 173 (1-3), 510–516. doi: 10.1016/j.jhazmat.2009.08.114
Welch B. L. (1947). The generalization of ‘STUDENT'S’problem when several different population varlances are involved. Biometrika 34 (1-2), 28–35.
White S. J. O., Hussain F. A., Hemond H. F., Sacco S. A., Shine J. P., Runkel R. L., et al. (2017). The precipitation of indium at elevated pH in a stream influenced by acid mine drainage. Sci. Total Environ. 574, 1484–1491. doi: 10.1016/j.scitotenv.2016.08.136
Wu W., Dong C., Wu J., Liu X., Wu Y., Chen X., et al. (2017). Ecological effects of soil properties and metal concentrations on the composition and diversity of microbial communities associated with land use patterns in an electronic waste recycling region. Sci. Total Environ. 601, 57–65. doi: 10.1016/j.scitotenv.2017.05.165
Xu F., Liu Z., Cao Y., Qiu L., Feng J., Xu F., et al. (2017). Assessment of heavy metal contamination in urban river sediments in the jiaozhou bay catchment, qingdao, China. Catena 150, 9–16. doi: 10.1016/j.catena.2016.11.004
Xu S., Tang F. (2018). Study on soil environmental pollution and its remediation in mining area. Tech Innov. Appl. 21, 81–82. doi: 10.1016/j.envpol.2018.11.062
Yang J.-L., Chen L.-H. (2018). Toxicity of antimony, gallium, and indium toward a teleost model and a native fish species of semiconductor manufacturing districts of Taiwan. J. Elementology 23 (1), 191–199. doi: 10.5601/jelem.2017.22.3.1470
Yan X., Luo X., Zhao M. (2016). Metagenomic analysis of microbial community in uranium-contaminated soil. Appl. Microbiol. Biotechnol. 100 (1), 299–310. doi: 10.1007/s00253-015-7003-5
Yan C., Wang F., Geng H., Liu H., Pu S., Tian Z., et al. (2020). Integrating high-throughput sequencing and metagenome analysis to reveal the characteristic and resistance mechanism of microbial community in metal contaminated sediments. Sci. Total Environ. 707, 136116. doi: 10.1016/j.scitotenv.2019.136116
Yazidi A., Saidi S., Mbarek N. B., Darragi F. (2017). Contribution of GIS to evaluate surface water pollution by heavy metals: Case of ichkeul lake (Northern Tunisia). J. Afr. Earth Sci. 134, 166–173. doi: 10.1016/j.jafrearsci.2017.06.009
Ye Y. T., Young S. S. (2008). An investigation of the heavy metals distribution of the main rivers and wetlands in hsinchu. [dissertation/master thesis in Chinese]. [Taiwan]:National Tsing Hua University.
Yi J., Lo L. S. H., Liu H., Qian P.-Y., Cheng J. (2021). Study of heavy metals and microbial communities in contaminated sediments along an urban estuary. Front. Mar. Sci 8, 741912. doi: 10.3389/fmars.2021.741912
Yi Y., Yang Z., Zhang S. (2011). Ecological risk assessment of heavy metals in sediment and human health risk assessment of heavy metals in fishes in the middle and lower reaches of the Yangtze river basin. Environ. Pollut. 159 (10), 2575–2585. doi: 10.1016/j.envpol.2011.06.011
Young S. S. (2009). The study of polychaeta and sipuncula from hisinchu city costal wildlife sanctuary, habitats and species distribution on temporal and spatial scale. government authorized project final report (in Chinese) Vol. 169 (Taiwan:Hsinchu City Government, National Hsinchu University of Education).
Young S. S. (2019). The ecological and water quality monitoring on siangshan wetland (national scale) 2018-2019. government-authorized project final report (in Chinese) Vol. 204 (Taiwan:Hsinchu City Government, National Tsing Hua University).
Young S. S., Jiang H. J., Syu R. L., Huang S. J. (2005). The biodiversity of siangshan wetland. government-authorized project final report (in Chinese) Vol. 210 (Taiwan:Hsinchu City Government, National Hsinchu teacher’s College).
Yu T., Zhang Y., Zhang Y (2012). Distribution and bioavailability of heavy metals in different particle-size fractions of sediments in Taihu Lake, China. Chemical Speciation & Bioavailability 24 (4), 205–215. doi: 10.3184/095422912X13488240379124.
Zeng X.-Y., Li S.-W., Leng Y., Kang X.-H. (2020). Structural and functional responses of bacterial and fungal communities to multiple heavy metal exposure in arid loess. Sci. Total Environ. 723, 138081. doi: 10.1016/j.scitotenv.2020.138081
Zhang W., Feng H., Chang J., Qu J., Xie H., Yu L. (2009). Heavy metal contamination in surface sediments of Yangtze river intertidal zone: An assessment from different indexes. Environ. Pollut. 157 (5), 1533–1543. doi: 10.1016/j.envpol.2009.01.007
Keywords: environmental DNA, 16S rRNA, metagenomic sequencing, microbiome diversity, Siangshan wetland, heavy metals, pollution index, RDA analysis
Citation: Salah-Tantawy A, Chang C-SG, Liu M-Y and Young S-S (2022) Exploring the diversity and structural response of sediment-associated microbiota communities to environmental pollution at the siangshan wetland in Taiwan using environmental DNA metagenomic approach. Front. Mar. Sci. 9:990428. doi: 10.3389/fmars.2022.990428
Received: 10 July 2022; Accepted: 04 August 2022;
Published: 29 August 2022.
Edited by:
Khaled Mohammed-Geba, University of Maryland, United StatesReviewed by:
Chi-Ying Hsieh, National Pingtung University of Science and Technology, TaiwanDaniel Liu, Da-Yeh University, Taiwan
Alaa El-Din Hamid Sayed, Assiut University, Egypt
Copyright © 2022 Salah-Tantawy, Chang, Liu and Young. This is an open-access article distributed under the terms of the Creative Commons Attribution License (CC BY). The use, distribution or reproduction in other forums is permitted, provided the original author(s) and the copyright owner(s) are credited and that the original publication in this journal is cited, in accordance with accepted academic practice. No use, distribution or reproduction is permitted which does not comply with these terms.
*Correspondence: Shuh-Sen Young, shusen@mx.nthu.edu.tw