- 1Departament de Biologia Evolutiva, Ecologia i Ciències Ambientals, Facultat de Biologia, Universitat de Barcelona (UB), Barcelona, Spain
- 2Institut de Recerca de la Biodiversitat (IRBio), Universitat de Barcelona (UB), Barcelona, Spain
- 3Associação Projecto Vitó, São Filipe, Cape Verde
Phenological divergence between conspecific populations breeding sympatrically is increasingly recognized as an important evolutionary process that may lead to allochronic speciation. However, the extent to which adaptation to differences in the timing of breeding may contribute to this process remains unclear. In this study, we assessed breeding phenology, population connectivity, and niche differentiation of two allochronic populations we of the Cape Verde Storm-petrel (Hydrobates jabejabe). We monitored nesting activity, marked individuals, tracked individuals during both the breeding and nonbreeding periods, and determined the trophic niche during both the breeding and nonbreeding periods. Timing of breeding for the two allochronic populations segregated into a hot (March-August) and cool (September-February) season (hereafter, hot and cool populations). These periods matched the two annual pulses of oceanic productivity around Cabo Verde, suggesting allochrony was primarily driven by a biannual cyclicity in food availability. Despite their allochronic breeding, there was, however, low differentiation between the hot and cool populations in spatial use, daily activity patterns, and trophic niche during both the breeding and nonbreeding periods. Further, the exchange of breeders between seasons, as documented through the recapture of marked individuals, may hinder seasonal adaptation by each population and ultimately, allochronic speciation. Consequently, allochrony alone may not be sufficient to drive speciation unless reproductive isolation between populations is complete or populations become strongly adapted to the environmental conditions associated with their timing of breeding.
1 Introduction
Allochrony refers to the temporal divergence of breeding between populations that can occur at different timescales, i.e. in daily activity (e.g. butterflies, Devries et al., 2008), seasonally (e.g. oaks, Juri and Premoli, 2021; toads, Thomé et al., 2021) or in different years (e.g. cicadas Marshall et al., 2011; Sota et al., 2013). The most common form of allochrony is the phenological divergence in the timing of annual phenophases, which can limit population connectivity (i.e., the exchange of breeders among populations), promote assortative mating, and may ultimately result in reproductive isolation (Hendry and Day, 2005; Taylor and Friesen, 2017). Allochronic populations may experience different environmental conditions and subsequently, adapt the timing of breeding, thus enabling ecological differentiation to operate on multiple axes of the ecological niche, such as diet, habitat, space use, or daily activity (Taylor and Friesen, 2017). This differentiation may act concurrently with allochrony and ultimately lead to sympatric speciation by allochrony, a well-recognized process with increasing relevance in evolutionary biology (Hendry and Day, 2005; Taylor and Friesen, 2017).
Several genetic studies across multiple taxa have demonstrated that limited gene-flow between allochronic populations may result in a sympatric speciation process (e.g., fruit flies, Doellman et al., 2019; butterflies, Gradish et al., 2018; seaweeds, Homma et al., 2020; whitefish, Bitz-Thorsen et al., 2020). However, there is little understanding or empiric evidence of how population connectivity and ecological differentiation between allochronic populations may drive this evolutionary process, particularly in tetrapods. For example, phenological divergence may operate synergistically with seasonal differences in food resources, thus promoting spatial differentiation in foraging areas or non-breeding distributions (Friesen, 2015). To develop a more complete understanding of how allochrony may operate, we need to understand what traits are differentiated between the allochronic populations and subsequently how environmental drivers may promote allochrony. However, detailed comparative studies on phenological divergence, population connectivity, and ecological differentiation between allochronic populations are scarce. Furthermore, it is still unclear how phenological divergence may be associated with temporal pulses in food availability (Monteiro and Furness, 1998; Barros et al., 2019; Taylor et al., 2019), breeding habitat availability (Monteiro and Furness, 1998) or predation pressure (Monteiro and Furness, 1998). Phenology of predators is usually associated with pulses of food availability, which in marine systems may be indicated by oceanographic productivity (e.g. bony fish, Cushing, 1990; Schweigert et al., 2013; Malick et al., 2015, seabirds, Hipfner, 2008), and which in turn relates to oceanographic conditions such as changes in sea temperature, nutrient supply, salinity, or ocean mixing (Ji et al., 2013; Hernández-León et al., 2020). Seabirds have often been employed as study models to understand how marine organisms match their breeding phenology to pulses of ocean productivity, while also avoiding seasonally adverse weather conditions such as storms (e.g. temperate Ramírez et al., 2016 and polar systems, Moe et al., 2009; Ramírez et al., 2017). In tropical oceans, the intra-annual stability of conditions allows some seabirds to breed throughout the year (e.g., frigatebirds (Fregata spp.), tropicbirds (Phaeton spp.) and boobies (Sula spp. Furness and Monaghan, 1987)). Interestingly, several tropical and sub-tropical petrels show two annual phenological peaks, and these can occur either in nearby localities (e.g. Bulwer’s Petrel Bulweria bulwerii, Megysi & O’Daniel, 2020), or on the same breeding site (e.g. Trindade Petrel Pterodroma arminjoniana, Leal & Bugoni, 2021). Indeed, allochrony has been recognized as a major driver of speciation in small pelagic seabirds, i.e. storm-petrel species breeding in Guadalupe (Townsend’s storm-petrel, Hydrobates socorroensis and Ainley’s storm-petrel Hydrobates cheimnomnestes, Power and Ainley, 1986; Taylor et al., 2018), Iwo (Swinhoe’s storm-petrel Hydrobates monorhoi and Matsudaira’s storm-petrel Hydrobates matsudairae, Warham, 1992), and Azores Islands (Monteiro’s storm-petrel Hydrobates monteiroi and Band-rumped storm-petrel Hydrobates castro, Friesen et al., 2007; Taylor et al., 2019). Whether allochrony might be a driver of speciation in other systems such as the Galapagos or the Atacama Desert is still unclear (Band-rumped storm-petrel in Galapagos Hydrobates castro, Smith and Friesen, 2007; Taylor et al., 2019; Markham’s storm-petrel in Atacama, Hydrobates markhami, Barros et al., 2019).
The Cape Verde storm-petrel (Hydrobates jabejabe) is an endemic seabird of the Cabo Verde archipelago (Alexander, 1898; Murphy, 1924; Semedo et al., 2020). It is often considered a year-round breeder, but the occurrence of two breeding peaks, one in summer and one in winter, suggests the existence of two populations. These have been usually referred to as a hot (i.e., summer breeding) and a cool (i.e., winter breeding) population and preliminary evidence indicates genetic connectivity (Φst>0.35, Taylor et al., 2019). However, detailed studies on the phenology, population connectivity, spatial ecology, trophic ecology, and daily activity patterns of these hot and cool breeding populations have not been conducted to date. In this study, we sought to (i) determine the phenology of each population, (ii) assess population connectivity between the two populations, (iii) measure the degree of differentiation across multiple axes of the ecological niche in each population, (iv) assess divergence in ecological traits of each population, and (v) evaluate the role of the environment as a driver of allochrony in this species. Considering that a recent study did not identify a clear genetic structure between the hot and cool populations (Taylor et al., 2019), we predicted that some population connectivity would occur between the two populations, and therefore that differentiation along different axes of the ecological niche may occur but be relatively low.
2 Methods
2.1 Study species and area
Cape Verde storm-petrel was formerly considered a conspecific of the Band-rumped storm-petrel (Hydrobates castro) (Murphy, 1924), but has recently been recognized as a full species based on bioacoustics and genetic evidence (Bolton, 2007; Friesen et al., 2007; Sangster et al., 2012; Taylor et al., 2019). It is considered a year-round breeder, but with preliminary evidence of breeding peaks in summer (hot population) and winter (cool population) (Taylor et al., 2019). This study was conducted in Cima Islet (14.971°N, 24.637°W; see Appendix S1), an islet of the tropical volcanic archipelago of Cabo Verde located off tropical Western Africa. Fieldwork was conducted between 2018-2021. Sample sizes of all the analyses are summarized in Appendix S2.
2.2 Breeding phenology
To assess the phenology of Cape Verde storm-petrels, we actively searched for nests year-round and, once located, checked the contents of the known nests every 2-3 days, monitoring 27-56 nests each year from 2018-2021. We obtained hatching and fledging dates from monitoring data. Considering that in most of the nests we did not know the exact laying date, we inferred it from hatching dates assuming a 42-day incubation period (Harris, 1969). We used geolocators (see more details below in the spatial overlap section) to define departure and arrival dates in waters surrounding Cabo Verde, defined as waters within 400 km of the nesting island. We chose this buffer distance around the colony island to accommodate the potential error of the geolocators (Halpin et al., 2021). We also used geolocators to establish the first day individuals spent in the nest burrow (i.e., no light data recorded for a continuous 24-hour period indicating the bird spent the day inside a burrow). Sample size of phenological dates varied because geolocators were recovered at different breeding stages or because some nests were found after hatching. We used these phenological data to define the breeding period but also any period of time when birds of the hot and cool populations were present in Cima Islet. We plotted trip data using boxplots in R through the package “ggplot2” (Wickham, 2016), and calculated the median and quartiles using the quantil.circular function of the package “circular” (Lund et al., 2017).
2.3 Population connectivity
To evaluate the population connectivity between the hot and cool populations, we used capture-mark-recapture data from adults and chicks found in burrows as well as from 1,777 individuals mist-netted from 2009 to 2021. We assessed connectivity by analyzing: (1) birds ringed as chicks in the nest and recaptured in mist nets as prospectors in subsequent years; (2) breeders captured in their nest and recaptured breeding in the following years; and (3) birds captured in mist nets and recaptured in mist nets. We assessed the level of connectivity between both populations through chord diagrams, with the function chordDiagram of the “circlize” package in R (Gu et al., 2014).
2.4 Ecological differentiation between the two allochronic populations (hot-cool populations)
2.4.1 Spatial differentiation during the breeding period
We measured the differentiation between the hot and cool populations in foraging areas during the breeding period by deploying Pathtrack® Nanofix-mini GPSs on breeders (∼0.95g, representing an average of 2.4% of the bird’s body weight, with a range of 1.80-3.13%) between 2018-2020. These devices were used successfully for studying the spatial ecology of lower-weighted species in the Northern Atlantic and the Mediterranean (Rotger et al., 2020; Bolton, 2021; Pascalis et al., 2021). The temporal resolution for location acquisition was one fix every 3 hours in 2018 and one fix every 2 hours in 2019 and 2020. GPS tags were attached to the base of the four central tail feathers with Tesa® tape and recovered on average after 10 days (range: 3-50 days). We did not deploy GPS on both parents of a nest simultaneously to avoid potentially impacting the success of the nest. We deployed 162 GPS tags and recovered 144 tags. We used data from recovered tags to describe space use and estimate 5%, 20%, 50%, 75%, and 95% kernel densities. We also calculated the overlap of the whole population (using complete and incomplete trips) between the first and the second year of data (i.e., between 2018/19 and 2019/20), between sexes, and between breeding stages (incubation/brooding/chick-rearing) in all sampled birds. We used the “adehabitatHR” package of R to calculate the kernels, and used the kernelUD and getverticeshr functions to create kernel contours and the kerneloverlap function to calculate their overlap (Calenge, 2006). For creating the kernel unit densities, we used the “href” smoothing factor and a grid of 1000.
We also calculated metrics from complete foraging trips, including trip duration, total distance covered, maximum distance from the colony. We compared these metrics and the 50% kernel size between hot and cool populations through Generalized Linear Mixed Models (GLMM). Our models included population, sex (birds were sexed molecularly, see methods in Appendix S3) and breeding stage (incubation/brooding/chick rearing) as fixed factors, and, year, and individual as random factors. We assigned Gaussian or Gamma distributions depending on the variable. We measured differences in the departure bearing of the foraging trip by calculating the bearing angle from the colony to the first position that was at least 72 km from the colony (maximum distance covered between the colony and the first GPS fix, 3h apart). To account for differences in environmental conditions between populations, we obtained wind direction and intensity before the departure of each trip. For extracting the wind data, we used the wind.dl function of the “rWind” package in R (Fernández-López and Schliep, 2019), which extract the data from the Global Forecast System of the USA’s National Weather Service. We used a spatial resolution of 0.5 degrees and temporal resolution of 3 hours. We compared the bearing and the wind data between allochronic populations using the Watson-Williamson test, with the watson.williams function of the “circular” package in R (Lund et al., 2017).
2.4.2 Spatial differentiation during the non-breeding period
We measured the differentiation between the hot and cool populations during the non-breeding period by deploying W65A9-Sea Migrate Technology geolocators (∼0.8g, representing an average of 2% of the bird’s body weight, with a range of 1.5%-2.6%) on breeders at the end of chick-rearing, between 2018-2020. We deployed 60 devices and recovered 19, 11 from the hot population and 8 from the cool population. We attached the geolocators to the tibia using a metallic ring with stainless steel cable. These devices have been used for studying individuals of similar-weighted species in the Northern Atlantic and Northern Pacific without generating significant impacts (e.g. Hydrobates leucorhous and Hydrobates furcatus Pollet et al., 2014; Halpin et al., 2018; Hedd et al., 2018). Geolocators were programmed to provide light levels every minute, and recorded maximum light levels every 5 minutes, providing two positions per day at a spatial error of up to 400 km (Halpin et al., 2021). To process the geolocator data, we used the IntiProc software of Migrate Technology to obtain the sun angle for calibrating the positions. This angle was obtained by calibrating the geolocators in an open location with known coordinates, and far from any artificial light for at least one week. Subsequently, we used the preprocessLight function of the “TwGeos” package in R (Lisovski et al., 2020) to process the light. With this function, we estimated the hour of sunrise and sunset, inspected the integrity of the light curve of each day, and manually corrected the transitions of sunrise or sunset with clear interferences. After that, we filtered and removed positions ± 20 days near the equinoxes, and those with quadratic speeds over the 0.95 quantile (43.14 km/h).
We assessed the degree of spatial overlap between the hot and cool populations for several parameters during the nonbreeding season, estimating the kernel densities at 5%, 20%, 50%, 75% and 95%. We also calculated the spatial overlap between the first and the second year of data (i.e., between 2018/19 and 2019/20), between sexes (male/female). We used the “adehabitatHR” package of R to calculate the kernels, and used the kernelUD and getverticeshr functions to create kernel contours and the kerneloverlap function to calculate their overlap (Calenge, 2006). To create the kernel unit densities, we used the “href” smoothing factor, and a grid of 1000. We also calculated some trip metrics, including the duration of the non-breeding period (since the departure until the arrival from the Cabo Verde waters); distance from the centroid of the core kernel density (50% density) during the nonbreeding period to the colony, and individual core kernel size. Comparisons between hot and cool populations were conducted through Generalized Linear Mixed Models (GLMM). Our models included population, and sex (birds were sexed molecularly, see methods in Appendix S3) as fixed factors, and year and individual as random factors. We assigned Gaussian or Gamma distributions depending on the variable.
2.4.3 Trophic differentiation
To assess the trophic differentiation between the hot and cool populations during both the breeding and non-breeding period, we analysed the carbon (δ13C) and nitrogen (δ15N) isotopic values for blood and feather samples collected during the breeding period and for feathers inferred to have grown during the non-breeding period (Ramos and González-Solís, 2012). All samples were collected from breeding birds. Specifically, we sampled the innermost primary (P1) and innermost secondary (S1) as indicators of the stable isotopes integrated at the end of the breeding season, and the eighth secondary (S8), outermost rectrix (R6), and body feathers as indicators of the non-breeding season (see details on the molting patterns in the Appendix S4, and Lab procedures for isotopes analyses in the Appendix S5).
We tested for differences in carbon (δ13C) and nitrogen (δ15N) isotopic values through a Generalized Linear Mixed Model, with season and sex as fixed factors and year and individual as random factors. We assigned a Gaussian or Gamma distribution, and a log or identity link function depending on the distribution of each variable. We calculated the isotopic niche amplitude using Stable Isotope Bayesian Ellipses (SIBER) and assessed resource differentiation and isotopic niche width by calculating the overlap and size of the ellipses, respectively. To calculate the isotopic niche amplitude and visualizing Stable Isotopic values of both populations we used the “SIBER” package in R (Jackson et al., 2011)
2.4.4 Daily activity differentiation
We assessed differences in the daily proportion of time spent on the water (resting periods) during the non-breeding period based on wet-dry data which was recorded by the geolocators every six seconds and subsequently packed in five minutes blocks. Because moon illumination can affect activity levels in some petrels (Harris, 1969; Watanuki, 2002), we obtained moon illumination from the function moonAngle in the “oce” package (Kelley, 2018). We also checked whether there were differences in the proportion of time spent on the water in the non-breeding period by dividing the activity into day-time and night-time, determined by the sunrise and sunset time of the geolocators, obtained with preprocessLight function of the “TwGeos” package in R (Lisovski et al., 2020). To test for differences in daily activity patterns between the hot and cool populations, we built Generalized Additive Mixed Models (GAMMs) with the proportion of time on the water as the response variable, and the hour, population, sex, day/night, and moon illumination as fixed factors. We included year and trip nested into the individual birds as random factors.
2.4.5 Habitat differentiation and potential environmental drivers of the allochronic process
To assess the potential influence of environmental variability on allochrony, we built an extended time series of three environmental variables thought to be potential drivers of foraging and nesting behavior for this species. The time series extended from 2001 – 2019 and each variable was collected at a temporal resolution of 10 days. To determine whether pulses in oceanic productivity might drive allochrony, and whether differentiation occurred in the oceanic features between both populations, we obtained primary productivity (mg m-3) values from the Global Ocean Biogeochemistry Hindcast of Copernicus (https://resources.marine.copernicus.eu/) at 0.25° resolution. We extracted daily primary productivity year-round values for each GPS position obtained during the chick-rearing period, as this is the breeding stage with the highest energetic requirement for parents. For the non-breeding period, we extracted the year-round primary productivity by using the daily average using a 50% density kernel. To assess if rainfall could be a driver of the phenology (since nests can be flooded), we also extracted rainfall data (mm) from Copernicus (https://climate.copernicus.eu/). Finally, we also compared the hours of light (i.e., night length) that birds experienced during nesting in the hot and cool seasons, since night length might influence on the amount of time available for foraging in this nocturnal species. For extracting the day length for both populations, we used the day length function of the “insol” package (Corripio, 2014). To determine if differences occurred between the hot and cool populations in primary productivity, rainfall, or day length, we performed GAMMs with the day of the year as a fixed factor, and the year and trip nested into the individual birds as a random factor.
2.5 Ecological traits
Breeding success: We assessed the proportion of successful and failed nests from nest monitoring, excluding suspected failures that happened after handling procedures (i.e., nests abandoned by one parent after deploying/recovering a tag, corresponding to 1-14 nests per season). After this exclusion, we analyzed data of 12-42 nests per season (75% of the nests monitored). We analyzed breeding success, defined as the proportion of nests were the chick fledged, using a logistic regression, with year and population as explanatory variables and breeding success as the response variable.
Foraging efficiency: We calculated the foraging efficiency for all the birds tagged with a GPS as the daily mass gain. We obtained mass gain by weighing parents with a Pesola® scale prior to colony departure (i.e., as part of the regular monitoring activities). In the case birds left the colony one or two days after the last weighing, we inferred their mass at departure by using the average daily loss of mass obtained from the population of incubating birds which were weighed in three-day intervals. Birds were always weighed within 3 days of colony departure (range: 1-3 days). Our models included population and sex (to account for potential dimorphism) as fixed factors, and the year, and individual as random factors. We used a Gamma distribution link function.
2.6 Model building, selection, and assumptions
We did all the comparisons through Generalized Linear Mixed Models (GLMM) using the functions lmer and glmer of the “lme4” package in R (Bates et al., 2015). In the case of Generalized Additive Mixed Models (GAMM), we conducted the analysis through the gamm function, in the “mgcv” package of R (Wood, 2001). We did a backward selection, dropping variables and selected the best models based on corrected AIC values. Sample sizes of all the analyses are included in Appendix S2. We checked the selected model’ normality of the residuals through a Q-Q plot and the homogeneity of the residuals through Cleveland dotplots (Zuur et al., 2010).
3 Results
Phenology of hot and cool populations: We found two clearly segregated breeding phenologies for successful breeders, with no temporal overlap within any given phase of breeding, although some overlap occurred between different phases (i.e., range of laying, hatching, and fledging dates based on nest monitoring; see Figure 1). Also, there were two failed breeding attempts that did not fit within the hot or cool population phenologies, because they bred between summer and winter (August). One of the nests failed flooded by the rain. Although two breeding phenologies were defined, geolocators data indicated that the arrival and departure dates from Cabo Verde waters of birds from each population overlapped extensively, thus defining intermediate periods in which birds from the two populations occurred within the waters near the breeding area (Figure 1).
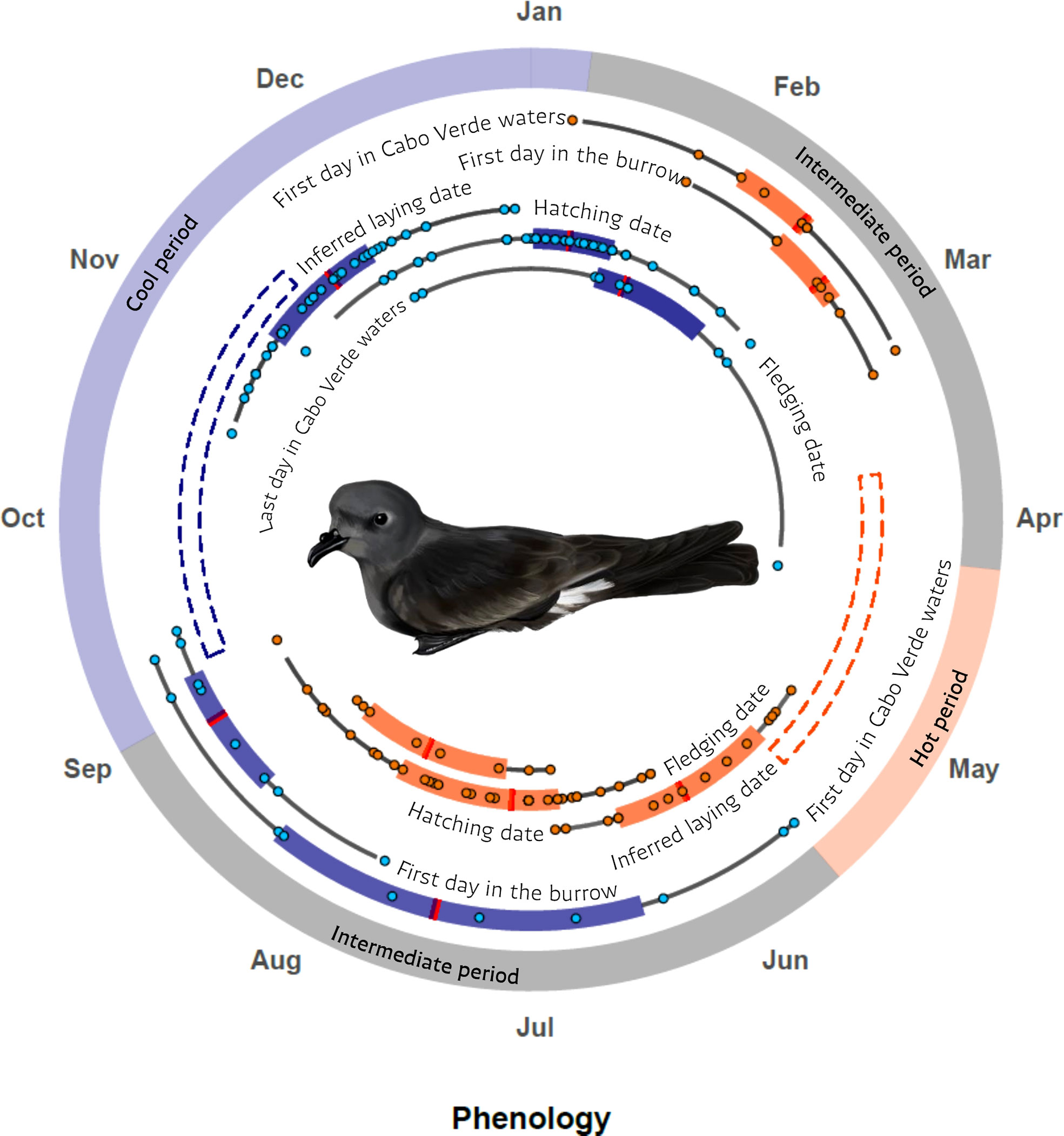
Figure 1 Phenology of the hot and cool populations of the Cape Verde storm-petrel in Cima Islet (Cabo Verde) based on nest monitoring and light-level geolocators. Blue shading indicates the phenology for the cool population while red/orange shading indicates the phenology of the hot population. Laying dates were inferred from hatching dates based on an incubation period of 42 days. Hot and cool seasons correspond to periods when only hot or cool breeders can be found in the colony whereas intermediate periods correspond to periods when birds from both populations can be found in the colony. Each boxplot shows the median, the first and third quartiles at the end of the box, and the first and 99th quantiles on the tails. Artwork by María Jesús Mallea S.
Based on nest monitoring and geolocation dates, we define the hot population as those birds breeding from the end of March to the end of August and the cool populations as those breeding from the beginning of September to the end of March. The data gathered from mist-netting generally support the seasons we propose, but the exact details differ slightly. For example, our mist-netting data indicated that the birds from one population can arrive at the colony and initiate breeding before the other population finishes breeding and departs the colony. This results in a reproductive season that is shorter than that defined solely from tracking data. Thus, the period in which mist-netting birds can be assigned to one season spans from early April to mid-May for the hot season, and from early September to early January for the cool season. There are two intermediate periods when the birds captured through mist-netting could correspond to any of the populations, spanning from early January to early April and from mid-May and late August. Details on the phenology are provided in Appendix S6.
3.1 Population connectivity
Population connectivity between hot and cool populations: From 2018 to 2021, we ringed 145 chicks (n = 58 cool season, n = 87 hot season). We recaptured eight of these chicks after an average of 1.9 years (range: 1.7—2.6 years). Five chicks ringed in the cool season were recaptured in mist-nets also during the cool season. Of the three chicks ringed in the hot season, one was recaptured in the cool period, and two were recaptured in the intermediate periods (Figure 2A). We ringed 276 breeders from 2018 to 2021 (n = 156 cool season, n = 120 hot season), and 67 were subsequently recaptured as breeders (n = 50 cool season, n = 17 hot season). Of the 156 individuals ringed in the cool season, 49 were recaptured as breeders in the cool season, while one individual was recaptured in the hot season. Of the 120 individuals ringed in the hot season, 15 were recaptured as breeders in the hot season, while two individuals were recaptured as breeders in the cool season (Figure 2B). All the three breeders that switched seasons were unsuccessful breeders in the first breeding attempt, but one breeder that failed in the hot season was successful in the subsequent cool season. Also, we ringed a total of 1,222 birds in mist-nets, of which we recaptured 79. Of the birds initially captured in the cool season, 43 were subsequently recaptured in the cool season, 1 bird in the hot season, and 26 birds in the intermediate period (Figure 2C). Of the birds initially captured in the hot season, one was subsequently recaptured in the hot season and 8 in the intermediate period. Ringing and recapture data are summarized in Table 1.
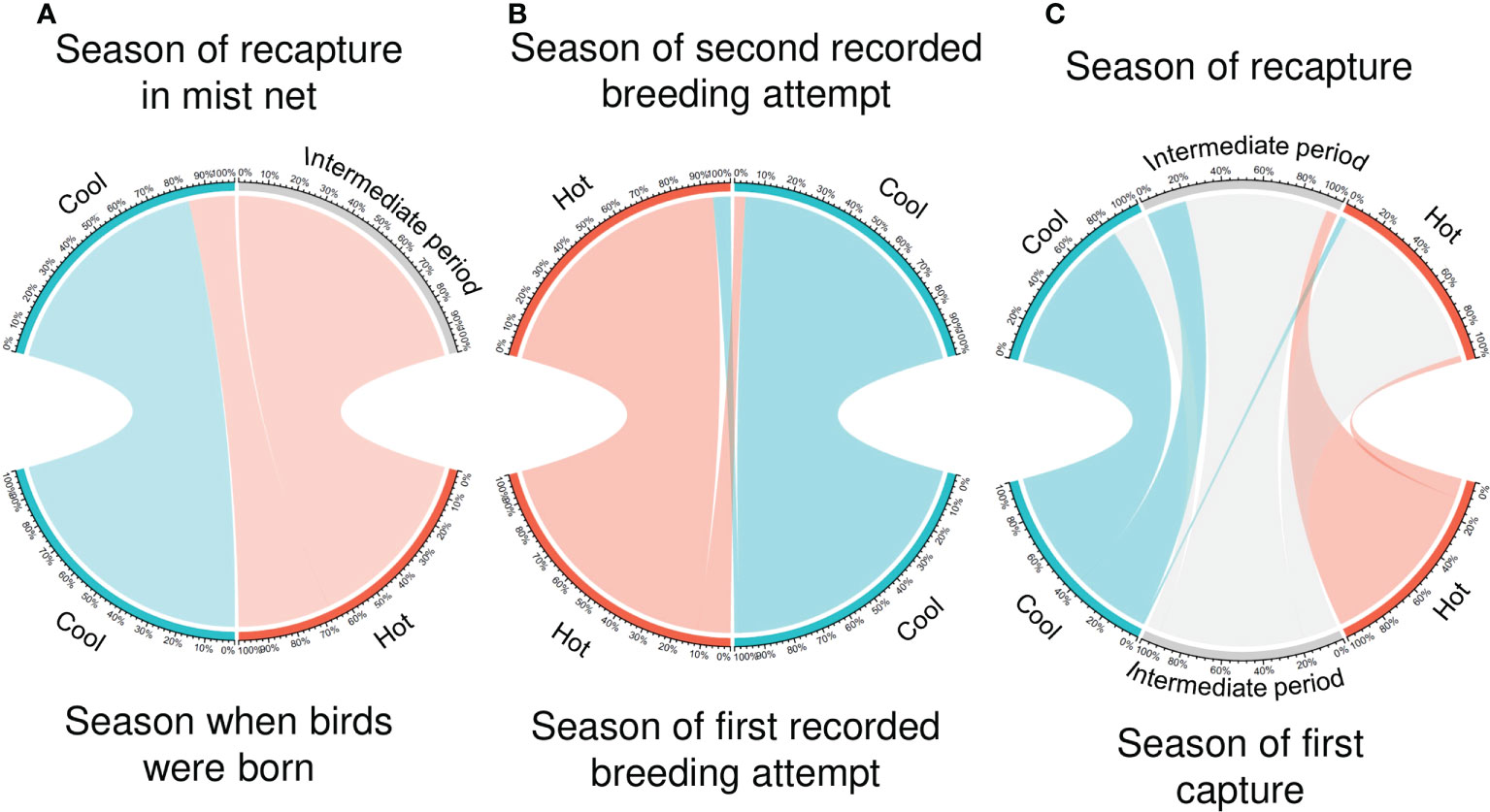
Figure 2 Chord diagrams assessing connectivity between hot and cool populations of the Cape Verde storm-petrel in Cima Islet (Cabo Verde). Hot season is depicted in orange, cool season in blue, and intermediate periods in grey. The bottom of the diagram shows the percentage of individuals that were initially captured in each season while the top shows the percentage of individuals during the period when they were recaptured for (A) birds ringed as chicks; (B) birds ringed as breeding adults and; (C) birds ringed in mist-nets. Sample sizes are summarized in Table 1.
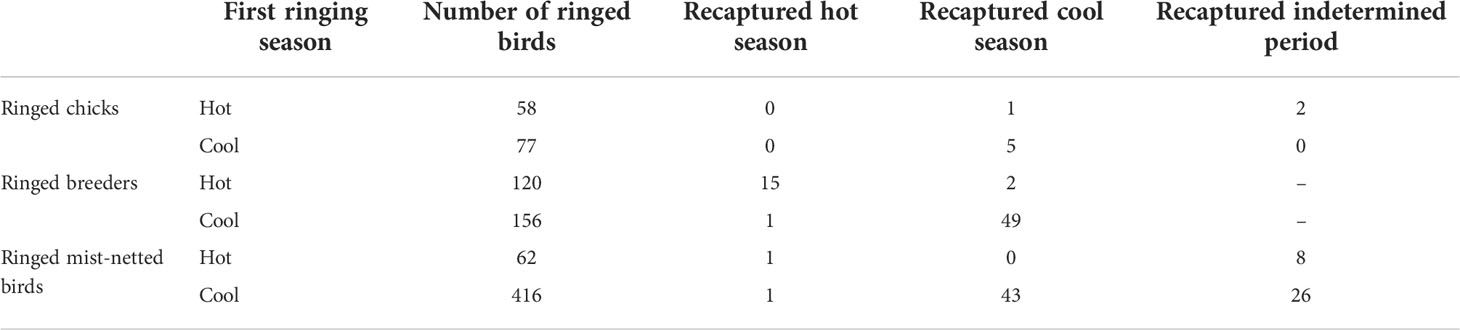
Table 1 Summary of the captures and recaptures made for (a) chicks, captured as prospectors in subsequent years; (b) breeders and (c) birds captured through mist-netting.
3.2 Ecological differentiation
3.2.1 Spatial differentiation during the breeding period
We compared foraging areas of breeding birds within years between populations and within populations between years. The mean (± SD) of spatial overlap of foraging areas within years between the hot and cool populations for each kernel density was 55.4 ± 21.9 (Figure 3A). The mean (± SD) of spatial overlap of foraging areas between years within populations was 65.5 ± 19.5 (Appendix S7), and therefore appeared to be slightly higher than that within years between populations. The maximum distance (x̄:225 km; range: 40—738 km; p-value = 0.48), duration (x̄: 2.7 days; range: 0.7—10.6 days; p-value = 0.59), distance covered (x̄: 728 km; range: 107—2,167 km; p-value = 0.37) and the individual core kernel size (x̄: 18,585 km2; range: 1,365—151,781 km2; p-value = 0.55) do not differ significantly between the two populations (boxplots available in Appendix S8, statistics available in Appendix S9). The bearing angle of departure from the colony during the breeding season differs significantly between the two seasons by 77.9° (F=58.18, df=184, p-value<0.01), with birds of the hot population heading North-West (x̄: 261.9°; range: 46.5° — 346.8°), while birds of the cool population head mainly to the South-East (x̄: 184°; range: 107.7°—316.2°) (see Appendix S10). Wind direction was to the South-West in both seasons, but differed significantly by 4.7° (F=6.44, df=184, p-value<0.01), with an average wind direction of 226.7° for the hot population and 231.06° for the cool population. Wind intensity did not differ significantly between the two seasons, with a mean of 7.3 km/h (p-value>0.05).
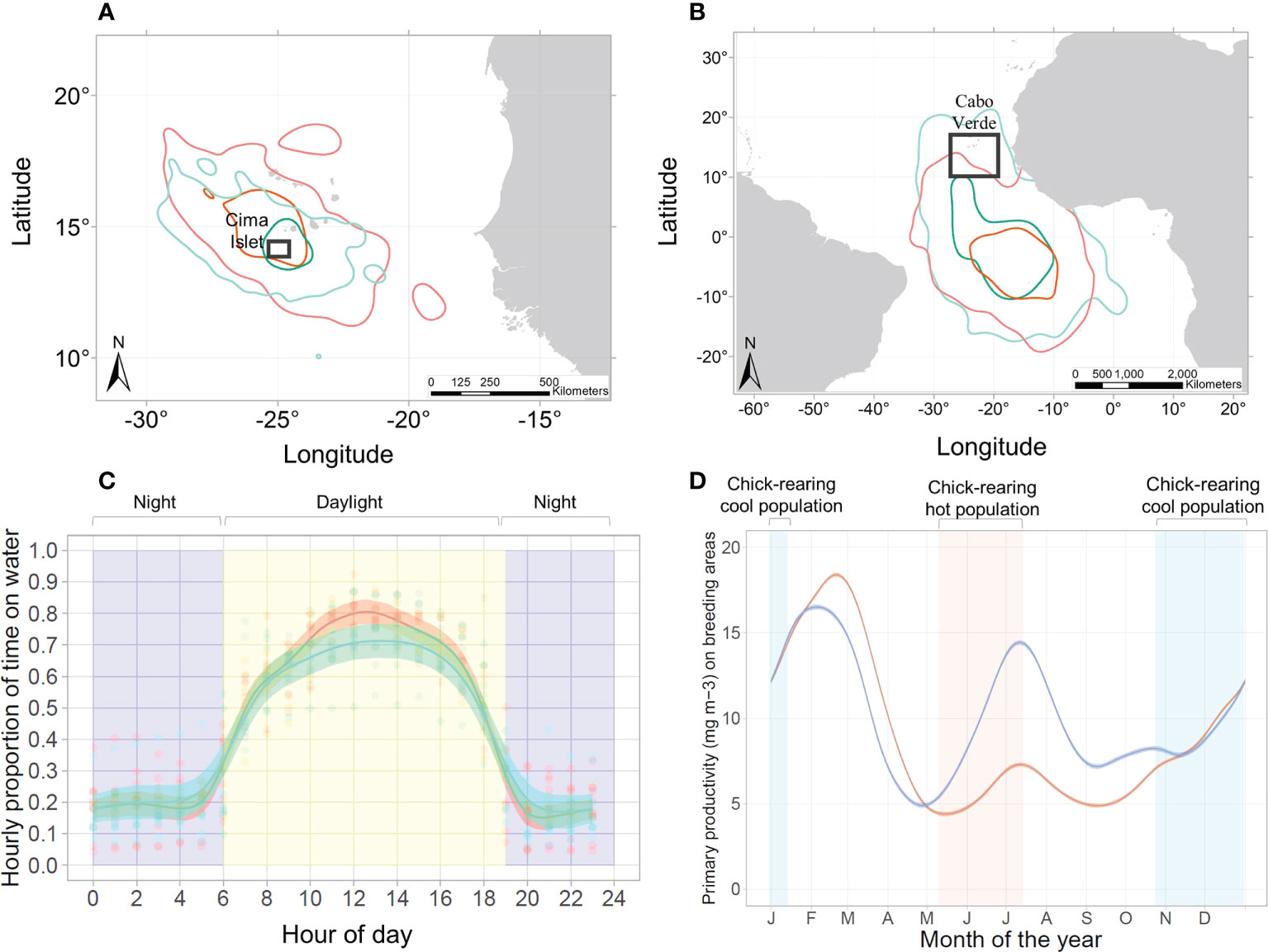
Figure 3 Niche overlap of both populations (cool population indicated by blue/green shading and hot population by orange/red shading) across different niche axes: (A) home-range and core area (95% and 50% kernel densities, respectively) during the breeding season for birds from the hot (N=95) and cool (N=122) populations; (B) home-range and core area during the non-breeding season for birds from the hot (N=11) and cool (N=8) populations; (C) daily activity patterns of birds from hot and cool populations, as determined from wet/dry data from geolocators and indicated by the proportion of time spent on the water during the non-breeding season (yellow background indicates day time and purple background indicated night time); GAMM shows the mean and the standard deviation interval, and (D) primary productivity in the marine areas used during the breeding season by the hot and cool populations; red background indicates the chick-rearing period of the hot season population, and blue background indicates the chick-rearing period of the cool season population. Sample sizes are summarized in Appendix S2.
3.2.2 Spatial differentiation during the non-breeding period
We compared areas of non-breeding birds within years between populations and within populations between years. The mean (± SD) of spatial overlap of non-breeding areas within years between the hot and cool populations for each kernel density was 60.7 ± 20.1 (Figure 3B). The mean (± SD) of spatial overlap of non-breeding areas between years within populations was 53.6 ± 25.3 (Appendix S11), and therefore appeared to be higher than that within years between populations. Duration of the non-breeding period (x̄: 168.8 days; range: 7.0—266.5 days; p-value = 0.69), distance of the centroid of the non-breeding area to the colony (x̄: 2,155.6 km, range: 650.8—3,033.2 km; p-value = 0.34) and 50% individual core kernel density size (x̄: 1,296,086 km2, range: 391,232.5 — 3,105,773 km2; p-value = 0.29) per non-breeding trip did not differ between the two populations (Appendix S12, statistics in Appendix S13).
Trophic differentiation between hot and cool populations: We found a high overlap of the Stable Isotopes Bayesian Ellipses between the hot and cool populations for both the first primary feather and for blood, but a lower overlap for the first and eighth secondary feathers, and no overlap for the sixth rectrix, and body feathers (Figure 4). Nitrogen values were higher for the hot than for the cool population for the sixth rectrix (GLM estimate =-0.0006, p-value<0.01), eighth secondary (GLM estimate=-0.003, p-value<0.05), and body feathers (GLM estimate=-0.0001, p-value<0.01), but no difference was found for blood (p-value=0.220), first primary (p-value=0.375) and first secondary (p-value=0.111). Carbon values did not differ between populations in any of the tissues. Furthermore, the standard Bayesian ellipses areas were larger for the cool population, particularly for blood samples (Appendix S14).
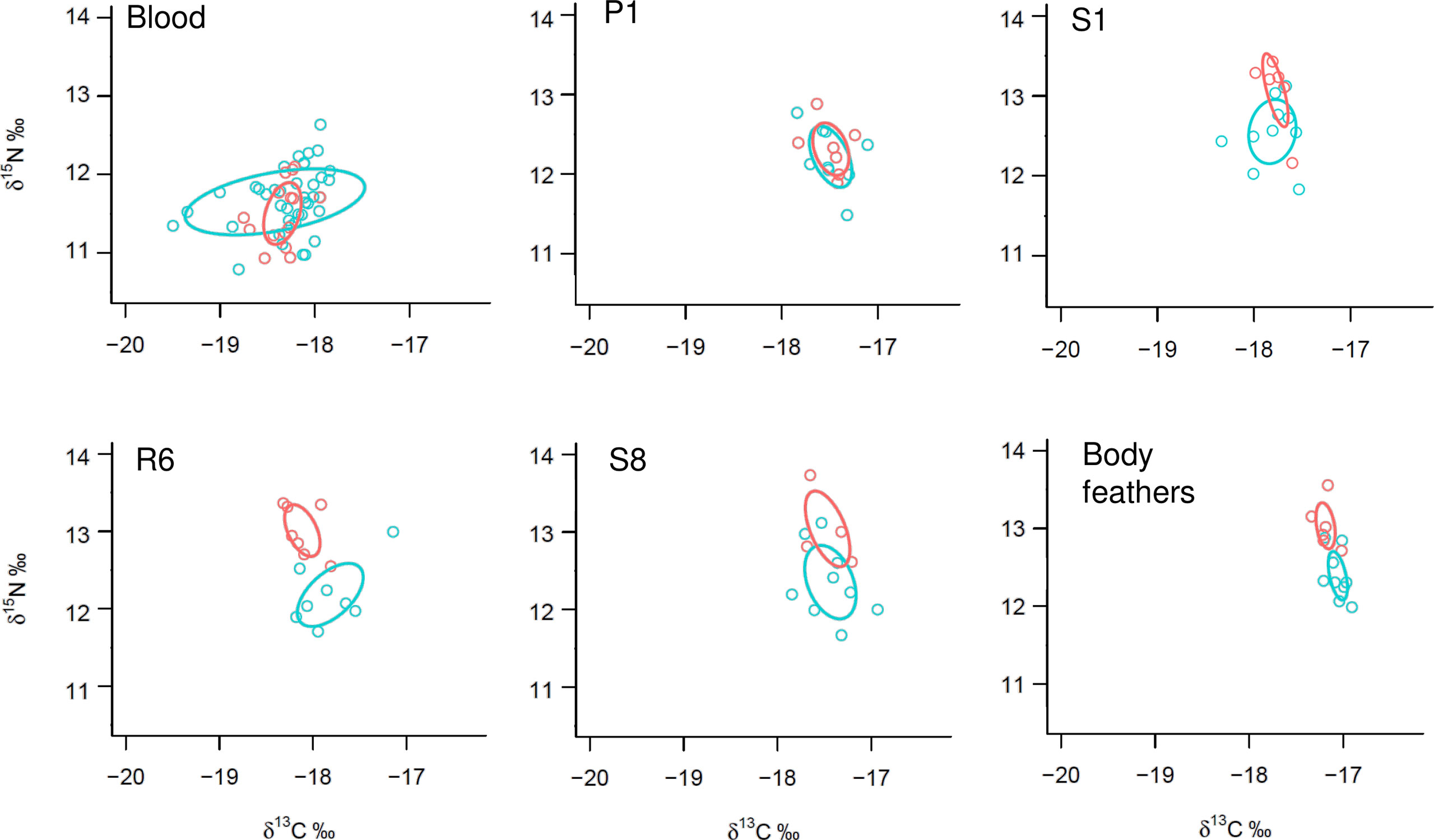
Figure 4 Comparison of the Bayesian Ellipses for stable isotopes of carbon and nitrogen for the hot (orange) and cool (blue) populations of Cape Verde storm-petrels. Stable isotopes were sampled for the breeding areas from blood and the first primary feather (P1), and for the non-breeding areas from the first secondary feather (S1), sixth rectrix (R6), eighth secondary feather (S8) and body feathers. Sample sizes are summarized in Appendix S2.
Daily activity differentiation between hot and cool populations: Overall, we found that birds from the hot population spent more time on the water than birds of the cool population (Figure 3C) during daylight (GAMM estimate=-0.05, p-value<0.05) and night period (GAMM estimate=-0.19, p-value<0.01). Birds of the hot population spent on average 42.3% of the time on the water (range: 0—95.7% of the time per hour), while birds of the cool population spent on average 40.4% of the time on the water (range: 0—92.9% of the time per hour). Furthermore, we found no association between the night activity and the moon illumination (p-value=0.7).
3.2.3 Habitat niche and potential environmental drivers of the allochronic process
We identified two annual pulses of productivity in the foraging areas used by the Cape Verde storm-petrel during chick-rearing (Figure 3D; Appendix S15). The first pulse of the year occurred during the months of February-March, while the second occurred during the months of July-August. Productivity pulses in the areas visited by the hot population were overall significantly higher than for the areas visited by the cool population (GAMM estimate: 1.42, p-value<0.01; Figure 3D; Appendix S15). In addition, the areas visited during the non-breeding season only showed one productivity pulse that matched the period when the hot population were in that areas (Appendix S16). Rainfall in Cabo Verde was concentrated in September (Appendix S17). The number of hours available for foraging at night in the waters surrounding the colony was greater for the cool population, with up to 2 more hours per night, than for the hot population (Appendix S17).
3.3 Ecological traits
Breeding success: We found no differences in the breeding success between the two populations (GLM p-value=0.7, Appendix S18), but there was an interannual difference within populations.
Foraging efficiency: The daily weight gain per trip (x̄: 0.6 g; range:-0.9—2 g) did not differ between the two populations (Linear Model p-value =0.8).
4 Discussion
In this study, we assess the phenological divergence, population connectivity, and ecological differentiation of two allochronic populations of seabird breeding at the same site. Our data indicated that two reproductive seasons occurred during the year, and that the peaks of reproductive activity in each of these seasons did not overlap temporally. We posit that these two distinct reproductive seasons are driven by two annual pulses of productivity in the waters around the breeding area. We identified facets of population connectivity between the two allochronic populations, including individuals that switched from one breeding season to the other. Further, we also found that, for the most part, the extent of differentiation between the two allochronic populations in space use, daily-use patterns, habitat use, and trophic ecology was relatively low during the breeding and non-breeding periods. These data indicate that the two allochronic populations (i.e., hot and cool populations) of Cape Verde storm-petrels appear to have not developed specific adaptations despite breeding during different periods of the year, and as such the exchange of individuals between populations may continue to be facilitated.
Cape Verde storm-petrels have long been assumed to breed year-round (Friesen et al., 2007; Taylor et al., 2019). Based on three years of continuous nest monitoring, we consistently found that there are two seasonal peaks of reproduction within the year, with no temporal overlap between them for any given breeding phase, similar to those described for the closely related storm-petrels breeding in the Azores (Hydrobates castro/monteiroi Bolton et al., 2008; Taylor et al., 2018). Seasonal peaks of reproduction suggest a disruptive selection towards two optimum environmental conditions in two different periods, driving birds to center their breeding activity on one of these two periods of the year (Monteiro and Furness, 1998; Barros et al., 2019; Taylor et al., 2019). Indeed, environmental conditions in Cabo Verde waters also show two annual pulses of productivity, and the hot and cool populations we defined appear to match their chick-rearing stage with these two periods. Although a time lag of about three months does occur between each pulse in the primary productivity and the respective peak in the breeding phenology of each population after the pulse, we posit this is related to the time needed for the biomass and energy to be transferred from phytoplankton productivity to zooplankton productivity, the latter being a primary food source for the storm-petrels (Ramírez et al., 2016). This disruptive selection process could be reinforced by rainy conditions during one of the intermediate periods. That is, birds may avoid rainfall in August and September to reduce the risk of flooding at the nest site. Indeed, one out of the two breeding attempts occurring in the intermediate period failed due to heavy rains.
When assessing allochrony among populations, it is critical to determine the extent of connectivity, i.e. whether there is any exchange of breeders between populations. Based on three years of continuous and intensive monitoring, we found that two out of 15 recaptured breeders (~13.3%) switched from breeding during the hot to the cool season, while one out of 50 breeders (2%) switched from breeding during the cool to the hot season. Thus, birds occasionally changed their breeding season, particularly after a breeding failure. Despite breeding phenology in birds typically being considered to be under genetic control (Liedvogel et al., 2009; Wilczek et al., 2009; Tang et al., 2016), our results show some phenotypic plasticity in breeding phenology, which might be driven by individual breeding performance. A similar pattern has been described among albatrosses, where the timing of breeding is driven by the past breeding success (Lewis et al., 2012). In cases where individuals switched breeding seasons, it is still unclear the extent to which switching the timing of breeding could affect other aspects of the annual cycle, such as molting and subsequent breeding attempts. For example, failed breeders of a past breeding season might advance their timing of molting (Ramos et al., 2018). Further, in our study the populations show a higher level of connectivity than one exchange per generation, which may explain the gene-flux between both populations (Taylor et al., 2019), and consequently the conditions under which speciation is unlikely to happen (Frankham et al., 2002).
Given the divergence in phenology between the hot and cool populations, we expected to find some seasonal adaptations and ecological divergence between populations, a pattern observed in other seabirds (e.g., penguins Thiebot et al., 2012; Green et al., 2022; Giant-petrels Granroth-Wilding & Phillips, 2019). However, we found low spatial differentiation between the hot and cool populations during both the breeding and non-breeding seasons. During the breeding season, the high spatial overlap may be mediated by the wind direction, which is similar between the two seasons. Consistent wind patterns during each breeding season may result in a similar foraging direction of each population as individuals seek to avoid headwinds and take advantage of crosswinds (Spear and Ainley, 2008; Paiva et al., 2010; Ventura et al., 2022). We also observed similar space use during the non-breeding seasons and posit this may be due to food availability in that area, although the current data are insufficient to assess this hypothesis.
We also found that isotope values in tissues generated during the breeding season (blood and P1) did not differ between the two populations, suggesting both populations were exploiting similar resources. This result contrasts with what was found in two allochronic species of storm-petrels in a temperate archipelago (Azores), where DNA metabarcoding data revealed that the two populations had different diets (Carreiro et al., 2022). In contrast, the isotopic values of the tissues regenerated during the non-breeding period (R6, S8, and body feathers) showed significant differences between the two populations. One mechanism underlying this difference may be that diet differs between the two populations during this phase of the annual cycle. Alternatively, isotopic differences during the non-breeding period may arise from seasonal changes in the isotopic baseline related to seasonal differences in the productivity and the nutrient dynamics of the Canary, North Equatorial and South Equatorial currents throughout the year (Stramma and Schott, 1999). Our understanding of the meaning of the isotopic overlap could be enhanced by measuring the trophic niche through other approaches, either analyzing the diet with DNA metabarcoding (e.g. Carreiro et al., 2022), or collecting baseline samples from the oceanic system to better understand annual fluctuations (e.g. Price et al., 2014).
It also appears that there are few environmental differences in the ocean (i.e., the foraging habitat) during the time periods it is occupied by each population. Consequently, differences in ecological traits between populations may be limited. For example, the productivity pulse occurring before the breeding season of the hot population is generally higher than that occurring before the breeding season of the cool population, suggesting the hot population may benefit from greater food availability. However, birds of both populations show a similar mass gain after a foraging trip, which indicates that food acquisition in terms of mass is similar between the two seasons. We also found that productivity was higher in waters visited during the non-breeding period by birds from the hot population, which may explain why birds from the hot population spent slightly more time on the water (i.e., presumably resting) compared to flying during the non-breeding season than those from the cool population. Our data also demonstrate that the breeding phenology of the hot population matches that of other small seabirds that nest in our study area (i.e., in the Cima Islet; white-faced storm-petrels, Bulwer’s petrels and Boyd’s shearwaters). Having multiple species breeding simultaneously may impose interspecific competition for breeding cavities, as it has been documented in an analogous seabird community in the Azores (Ramos et al., 1997). Indeed, the avoidance of competition for nesting cavities was one of the mechanisms suggested to cause the onset of the allochronic process between Monteiro’s and Band-rumped storm-petrels (Monteiro and Furness, 1998). Finally, given that Cape Verde storm-petrels are mainly nocturnal, birds of the cool population may benefit from the longer period of darkness in Cabo Verde during winter (with 2 more hours than the hot population; Appendix S17), providing them more opportunities for foraging than birds from the hot season (Monteiro and Furness, 1998). The influence of each factor on different life-history traits requires further research but we did not find differences in the breeding success between the two populations.
In summary, despite a clear divergence in the timing of breeding, we did not find high levels of ecological differentiation, raising the question of whether allochrony can drive phenotypic divergence alone (genetic drift), or if allochrony needs to be coupled with adaptation to seasonal conditions (natural selection) to culminate in allochronic speciation (Dieckmann et al., 2004). Ultimately, systems with relatively little seasonal differentiation, such as those occurring in tropical oceanic environments, may not differ enough throughout the year to promote specific seasonal adaptations to the environment. In contrast, seasonal environments were conducive to allochronic speciation of the Band-rumped storm-petrel and the Monteiro’s storm-petrel in the Azores (Monteiro and Furness, 1998; Friesen et al., 2007) and Townsend storm-petrel and Ainley’s storm-petrel in the Guadalupe Island (Taylor et al., 2019), and presumably promoted ecological differentiation and ultimately speciation. Alternatively, considering that in our study population connectivity occurs, gene flow might be high enough to maintain the homogeneity of the ecological traits in both populations, preventing differentiation to take place until reproductive isolation increases. For better insights in both topics, new studies of ecological differentiation in other allochronic species are key to get a deeper understanding on how ecological differentiation could reinforce speciation, and which types of systems are more likely to generate differentiation, and consequently speciation.
Data availability statement
Data presented in the study are deposited in different repositories. Tracking data (GPS and geolocators) is available in the Seabird Tracking Database repository, identifiers: 1568, 1569, 1778, 1779. Isotopic data is deposited in the Figshare repository, accession number 10.6084/m9.figshare.21388869.
Ethics statement
All procedures involving animal manipulations were in accordance with required European legislation. Deployment and recovery of GPSs and geolocators, and associated sampling procedures, were accomplished in < 10 minutes per bird and did not have any visible detrimental effects on individuals (e.g., external signs of panting or weakness). Additionally, we did not detect any differences in the breeding success between nests where a parent carried a GPS (N=60) and control nests (N=87) in a chi-squared test (c2 = 2.37, p=0.12, df=1) nor in return rates between birds that carried a geolocator (N=60) and breeders with no geolocators (N=235) in a chisquared test (c2 = 0.01, p=0.89, df=1). All research and monitoring was conducted under permission from the Direção Nacional do Ambiente from Cabo Verde “Autorização N.°91/ 2018; Autorização N.°107/2019; Autorização N.°016/ DNA/2020”.
Author contributions
FM conceived the study, carried out fieldwork, data curation and analysis, lab analyses, and wrote the first draft. TM coordinated and carried out fieldwork, supervised the analyses, and reviewed the draft. IG led fieldwork. MS-S coordinated fieldwork and conducted lab analyses. MF carried out fieldwork and lab analyses. HD coordinated logistics and resources. JG-S led fieldwork, designed the study, supervised analyses, and helped drafting the manuscript. All authors contributed to the article and approved the submitted version.
Funding
The PhD of FM was granted by Agencia Nacional de Investigación y Desarrollo de Chile (ANID)/DoctoradoBecasChile/2019-72200117. This work was supported by MAVA Foundation through the projects “Alcyon Programme – Promoting the conservation of seabirds in Cape Verde” [MAVA17022] and “Conserving the seabirds of Cabo Verde” [MAVA4880], by the Spanish Ministerio de Economia, Industria y Competitividad and Ministerio de Ciencia, Innovación y Universidades [CGL2016-78530-R] and [PID2020-117155GB-I00/AEI/10.13039/501100011033] and by the award ICREA Acadèmia.
Acknowledgments
We are grateful to the handling editor, Patrick Jodice, and the reviewers: Daniel Paul Costa, Ingrid L Pollet, and Cecilia Soldatini who improved substantially the quality and clarity of the ideas presented in this manuscript. We are grateful to Admilton Pina, Cátio Pina, Ariete Pina, Gilson Montrond, Nadito Barbosa, Andreia Leal, and all the volunteers who had done fieldwork in Cima Islet. We also thank Zuzana Zajková and Virginia Moreira for sharing their spatial and activity analysis knowledge, and Sarah Saldanha for general insights on this research. We are also thankful to María Jesús Mallea S and Sergi Torné, who did the artwork for this article.
Conflict of interest
The authors declare that the research was conducted in the absence of any commercial or financial relationships that could be construed as a potential conflict of interest.
Publisher’s note
All claims expressed in this article are solely those of the authors and do not necessarily represent those of their affiliated organizations, or those of the publisher, the editors and the reviewers. Any product that may be evaluated in this article, or claim that may be made by its manufacturer, is not guaranteed or endorsed by the publisher.
Supplementary material
The Supplementary Material for this article can be found online at: https://www.frontiersin.org/articles/10.3389/fmars.2022.975716/full#supplementary-material
References
Alexander B. (1898). VII.–an ornithological expedition to the cape Verde islands. Ibis 40, 74–118. doi: 10.1111/j.1474-919X.1898.tb05509.x
Barros R., Medrano F., Norambuena H. V., Peredo R., Silva R., de Groote F., et al. (2019). Breeding phenology, distribution and conservation status of markham’s storm-petrel Oceanodroma markhami in the atacama desert. Ardea 107, 75–84. doi: 10.5253/arde.v107i1.a1
Bates D., Mächler M., Bolker B. M., Walker S. C. (2015). Fitting linear mixed-effects models using lme4. J. Stat. Software 67, 1–48. doi: 10.18637/jss.v067.i01
Bitz-Thorsen J., Häkli K., Bhat S., Præbel K. (2020). Allochrony as a potential driver for reproductive isolation in adaptive radiations of European whitefish ecomorphs. Ecol. Freshw. Fish 29, 40–49. doi: 10.1111/EFF.12486
Bolton M. (2007). Playback experiments indicate absence of vocal recognition among temporally and geographically separated populations of madeiran storm-petrels Oceanodroma castro. Ibis 149, 255–263. doi: 10.1111/j.1474-919X.2006.00624.x
Bolton M. (2021). GPS Tracking reveals highly consistent use of restricted foraging areas by European storm-petrels Hydrobates pelagicus breeding at the largest UK colony: implications for conservation management. Bird Conserv. Int. 31, 35–52. doi: 10.1017/S0959270920000374
Bolton M., Smith A. L., Gómez-Díaz E., Friesen V. L., Medeiros R., Bried J., et al. (2008). Monteiro’s storm-petrel Oceanodroma monteiroi: A new species from the Azores. Ibis 150, 717–727. doi: 10.1111/j.1474-919X.2008.00854.x
Calenge C. (2006). The package “adehabitat” for the r software: A tool for the analysis of space and habitat use by animals. Ecol. Modell. 197 (3-4), 516–519. doi: 10.1016/j.ecolmodel.2006.03.017
Carreiro A. R., Bried J., Deakin Z., Jones K. B., Thomas R. J., Symondson W. O. C., et al. (2022). First insights into the diet composition of madeiran and monteiro’s storm petrels (Hydrobates castro and H. monteiroi) breeding in the Azores. Waterbirds 44, 300–307. doi: 10.1675/063.044.0304
Cushing D. H. (1990). Plankton production and year-class strength in fish populations: An update of the match/mismatch hypothesis. Adv. Mar. Biol. 26, 249–293. doi: 10.1016/S0065-2881(08)60202-3
Devries P. J., Austin G. T., Martin N. H. (2008). Diel activity and reproductive isolation in a diverse assemblage of Neotropical skippers (Lepidoptera: Hesperiidae). Biol. Journ. Linn. Soc 94, 723–736. doi: 10.1111/j.1095-8312.2008.01037.x
Dieckmann U., Doebeli M., Metz J., Tautz D. (2004). Adaptative speciation (Cambridge: Cambridge University Press).
Doellman M. M., Egan S. P., Ragland G. J., Meyers P. J., Hood G. R., Powell T. H. Q., et al. (2019). Standing geographic variation in eclosion time and the genomics of host race formation in Rhagoletis pomonella fruit flies. Ecol. Evol. 9, 393–409. doi: 10.1002/ECE3.4758
Fernández-López J., Schliep K. (2019). rWind: download, edit and include wind data in ecological and evolutionary analysis. Ecography 42, 804–810. doi: 10.1111/ecog.03730
Frankham R., Ballou J. D., Briscoe D. A. (2002). Introduction to conservation genetics (Cambridge: Cambridge University Press).
Friesen V. L. (2015). Speciation in seabirds: why are there so many species … and why aren’t there more? J. Ornithol. 156, 27–39. doi: 10.1007/s10336-015-1235-0
Friesen V. L., Smith A. L., Gómez-Díaz E., Bolton M., Furness R. W., González-Solís J., et al. (2007). Sympatric speciation by allochrony in a seabird. Proc. Natl. Acad. Sci. U.S.A. 104, 18589–18594. doi: 10.1073/pnas.0700446104
Gradish A. E., Keyghobadi N., Sperling F. A. H., Otis G. W. (2018). Population genetic structure and assessment of allochronic divergence in the macoun’s Arctic (Oeneis macounii) butterfly. Can. J. Zool. 97, 121–130. doi: 10.1139/CJZ-2018-0117
Granroth-Wilding H. M. V., Phillips R. A. (2019). Segregation in space and time explains the coexistence of two sympatric sub-Antarctic petrels. Ibis 161, 101–116. doi: 10.1111/IBI.12584
Green C. P., Ratcliffe N., Mattern T., Thompson D., Lea M. A., Wotherspoon S., et al. (2022). The role of allochrony in influencing interspecific differences in foraging distribution during the non-breeding season between two congeneric crested penguin species. PloS One 17, e0262901. doi: 10.1371/JOURNAL.PONE.0262901
Gu Z., Gu L., Eils R., Schlesner M., Brors B. (2014). Circlize implements and enhances circular visualization in r. Bioinformatics 30, 2811–2812. doi: 10.1093/bioinformatics/btu393
Halpin L. R., Pollet I. L., Lee C., Morgan K. H., Carter H. R. (2018). Year-round movements of sympatric fork-tailed (Oceanodroma furcata) and leach’s (O. leucorhoa) storm-petrels. J. Field Ornithol. 89, 207–220. doi: 10.1111/jofo.12255
Halpin L. R., Ross J. D., Ramos R., Mott R., Carlile N., Golding N., et al. (2021). Double-tagging scores of seabirds reveals that light-level geolocator accuracy is limited by species idiosyncrasies and equatorial solar profiles. Methods Ecol. Evol. 12, 2243–2255. doi: 10.1111/2041-210X.13698
Harris M. P. (1969). The biology of storm petrels in the Galapagos islands. Proc. Calif. Acad. Sci. 4, 95–166.
Hedd A., Pollet I. L., Mauck R. A., Burke C. M., Mallory M. L., McFarlane Tranquilla L. A., et al. (2018). Foraging areas, offshore habitat use, and colony overlap by incubating leach’s storm-petrels Oceanodroma leucorhoa in the Northwest Atlantic. PloS One 13, 1–18. doi: 10.1371/JOURNAL.PONE.0194389
Hendry A. P., Day T. (2005). Population structure attributable to reproductive time: Isolation by time and adaptation by time. Mol. Ecol. 14, 901–916. doi: 10.1111/j.1365-294X.2005.02480.x
Hernández-León S., Koppelmann R., Fraile-Nuez E., Bode A., Mompeán C., Irigoien X., et al. (2020). Large Deep-sea zooplankton biomass mirrors primary production in the global ocean. Nat. Commun 6048, 1–8. doi: 10.1038/s41467-020-19875-7
Hipfner J. M. (2008). Matches and mismatches: Ocean climate, prey phenology and breeding success in a zooplanktivorous seabird. Mar. Ecol. Prog. Ser. 368, 295–304. doi: 10.3354/meps07603
Homma Y., Okuda S., Kasahara M., Takahashi F., Yoshikawa S., Uwai S. (2020). Phenological shifts and genetic differentiation between sympatric populations of Sargassum horneri (Fucales, phaeophyceae) in Japan. Mar. Ecol. Prog. Ser. 642, 103–116. doi: 10.3354/MEPS13332
Jackson A. L., Inger R., Parnell A. C., Bearhop S. (2011). Comparing isotopic niche widths among and within communities: SIBER - stable isotope Bayesian ellipses in r. J. Anim. Ecol. 80, 595–602. doi: 10.1111/j.1365-2656.2011.01806.x
Ji R., Jin M., Varpe Ø. (2013). Sea Ice phenology and timing of primary production pulses in the Arctic ocean. Glob. Chang. Biol. 19, 734–741. doi: 10.1111/gcb.12074
Juri G., Premoli A. C. (2021). Allochrony of neighbour ecological species: Can isolation by time maintain divergence? the natural experiment of sympatric Nothofagus. For Ecol. Manage. 497, 119466. doi: 10.1016/J.FORECO.2021.119466
Kelley D. E., Richards C., Layton C. (2022). Oce: an R package for Oceanographic Analysis. Journal of Open Source Software 7, 1–5. doi: 10.21105/joss.03594
Leal G. R., Bugoni L. (2021). Individual variability in habitat, migration routes and niche used by trindade petrels, Pterodroma arminjoniana. Mar. Biol. 68, 1–14. doi: 10.1007/S00227-021-03938-4
Lewis S., Nussey D. H., Wood A. G., Croxall J. P., Phillips R. A. (2012). Intrinsic determinants of a population trend in timing of breeding in the wandering albatross. Oikos 121, 2061–2071. doi: 10.1111/J.1600-0706.2012.20293.X
Liedvogel M., Szulkin M., Knowles S. C. L., Wood M. J., Sheldon B. C. (2009). Phenotypic correlates of clock gene variation in a wild blue tit population: evidence for a role in seasonal timing of reproduction. Mol. Ecol. 18, 2444–2456. doi: 10.1111/J.1365-294X.2009.04204.X
Lisovski S., Bauer S., Briedis M., Davidson S. C., Dhanjal-Adams K. L., Hallworth M. T., et al. (2020). Light-level geolocator analyses: A user’s guide. J. Anim. Ecol. 89, 221–236. doi: 10.1111/1365-2656.13036
Lund U., Agostinelli C., Arai H., Gagliardi A., Garcia Portuges E., Giunchi D., et al (2017). R package “circular”: Circular statistics R package.
Malick M. J., Cox S. P., Mueter F. J., Peterman R. M. (2015). Linking phytoplankton phenology to salmon productivity along a north-south gradient in the northeast pacific ocean. Can. J. Fish. Aquat. Sci. 72, 697–708. doi: 10.1139/cjfas-2014-0298
Marshall D. C., Cooley J. R., Hill K. B. R. (2011). Developmental plasticity of life-cycle length in thirteen-year periodical cicadas (Hemiptera: Cicadidae). Ann. Entomol. Soc. Am. 104, 443–450. doi: 10.1603/AN10087
Megysi J. L., O’Daniel D. L. (2020). “Bulwer’s petrel (Bulweria bulwerii),” in Birds of the world (Ithaca, NY, USA: Cornell Lab of Ornithology). doi: 10.2173/bow.bulpet.01
Moe B., Stempniewicz L., Jakubas D., Angelier F., Chastel O., Dinessen F., et al. (2009). Climate change and phenological responses of two seabird species breeding in the high-Arctic. Mar. Ecol. Prog. Ser. 393, 235–246. doi: 10.3354/meps08222
Monteiro L. R., Furness R. W. (1998). Speciation through temporal segregation of madeiran storm petrel (Oceanodroma castro) populations in the Azores? Phi. Trans. Roy. Soc. B.: Biol. Sci. 353, 945–953. doi: 10.1098/rstb.1998.0259
Murphy R. C. (1924). The marine ornithology of the cape Verde islands, with a list of all the birds of the archipelago. Bull. Am. Mus. Nat. Hist. 50, 211–278.
Paiva V. H., Guilford T., Meade J., Geraldes P., Ramos J. A., Garthe S. (2010). Flight dynamics of cory’s shearwater foraging in a coastal environment. Zoology 113, 47–56. doi: 10.1016/j.zool.2009.05.003
Pascalis F., Pala D., Pisu D., Morinay J., Benvenuti A., Spano C., et al. (2021). Searching on the edge: dynamic oceanographic features increase foraging opportunities in a small pelagic seabird. Mar. Ecol. Prog. Ser. 668, 121–132. doi: 10.3354/MEPS13726
Pollet I. L., Ronconi R. A., Jonsen I. D., Leonard M. L., Taylor P. D., Shutler D. (2014). Foraging movements of leach’s storm-petrels Oceanodroma leucorhoa during incubation. J. Av. Biol. 45, 305–314. doi: 10.1111/jav.00361
Power D. M., Ainley D. G. (1986). Seabird geographic variation: Similarity among populations of leach’s storm-petrel. Auk 103, 575–585. doi: 10.1093/auk/103.3.575
Price T. D., Frei K. M., Naumann E. (2014). Isotopic baselines in the north Atlantic region. J. North Atlantic. 2014, 103–136. doi: 10.3721/037.002.sp707
Ramírez F., Afán I., Tavecchia G., Catalán I. A., Oro D., Sanz-Aguilar A. (2016). Oceanographic drivers and mistiming processes shape breeding success in a seabird. Proc. Roy. Soc. B.: Bio Sci. 283 (1826), 1–9. doi: 10.1098/rspb.2015.2287
Ramírez F., Tarroux A., Hovinen J., Navarro J., Afán I., Forero M. G., et al. (2017). Sea Ice phenology and primary productivity pulses shape breeding success in Arctic seabirds. Sci. Rep. 7, 1–9. doi: 10.1038/s41598-017-04775-6
Ramos R., González-Solís J. (2012). Trace me if you can: The use of intrinsic biogeochemical markers in marine top predators. Front. Ecol. Environ. 10, 258–266. doi: 10.1890/110140
Ramos R., Llabrés V., Monclús L., López-Béjar M., González-Solís J. (2018). Costs of breeding are rapidly buffered and do not affect migratory behavior in a long-lived bird species. Ecology 99, 2010–2024. doi: 10.1002/ECY.2435
Ramos J. A., Monteiro L. R., Sola E., Moniz Z. (1997). Characteristics and competition for nest cavities in burrowing procellariiformes. Condor 99, 634–641. doi: 10.2307/1370475
Rotger A., Sola A., Tavecchia G., Sanz-Aguilar A. (2020). Foraging far from home: Gps-tracking of Mediterranean storm-petrels Hydrobates pelagicus melitensis reveals long-distance foraging movements. Ardeola 68, 3–16. doi: 10.13157/arla.68.1.2021.ra1
Sangster G., Collinson J. M., Crochet P. A., Knox A. G., Parkin D. T., Votier S. C. (2012). Taxonomic recommendations for British birds: Eighth report. Ibis 154, 874–883. doi: 10.1111/j.1474-919X.2012.01273.x
Schweigert J. F., Thompson M., Fort C., Hay D. E., Therriault T. W., Brown L. N. (2013). Factors linking pacific herring (Clupea pallasi) productivity and the spring plankton bloom in the strait of Georgia, British Columbia, Canada. Prog. Oceanogr. 115, 103–110. doi: 10.1016/j.pocean.2013.05.017
Semedo G., Paiva V. H., Militaõ T., Rodrigues I., Dinis H. A., Pereira J., et al. (2020). Distribution, abundance, and on-land threats to cabo Verde seabirds. Bird Conserv. Int. 31, 53–76. doi: 10.1017/S0959270920000428
Smith A. L., Friesen V. L. (2007). Differentiation of sympatric populations of the band-rumped storm-petrel in the Galapagos islands: An examination of genetics, morphology, and vocalizations. Mol. Ecol. 16, 1593–1603. doi: 10.1111/j.1365-294X.2006.03154.x
Sota T., Yamamoto S., Cooley J. R., Hill K. B. R., Simon C., Yoshimura J. (2013) Independent divergence of 13-and 17-y life cycles among three periodical cicada lineages Proc. Natl. Acad. Sci. U S A. 110, 6919–6924. doi: 10.1073/pnas.1220060110
Spear L. B., Ainley D. G. (2008). Flight speed of seabirds in relation to wind speed and direction. Ibis 139, 234–251. doi: 10.1111/j.1474-919X.1997.tb04621.x
Stramma L., Schott F. (1999). The mean flow field of the tropical Atlantic ocean. Deep Sea Res. Part II: Topic. Stud. Oceanogr 46, 279–303. doi: 10.1016/S0967-0645(98)00109-X
Tang J., Körner C., Muraoka H., Piao S., Shen M., Thackeray S. J., et al. (2016). Emerging opportunities and challenges in phenology: a review. Ecosphere 7, e01436. doi: 10.1002/ECS2.1436
Taylor R. S., Bailie A., Gulavita P., Birt T., Aarvak T., Anker-Nilssen T., et al. (2018). Sympatric population divergence within a highly pelagic seabird species complex (Hydrobates spp.). J. Avian Biol 49, 1–14. doi: 10.1111/jav.01515
Taylor R. S., Bolton M., Beard A., Birt T., Deane-Coe P., Raine A. F., et al. (2019). Cryptic species and independent origins of allochronic populations within a seabird species complex (Hydrobates spp.). Mol. Phylogenet. Evol 139, 1–15. doi: 10.1016/j.ympev.2019.106552
Taylor R. S., Friesen V. L. (2017). The role of allochrony in speciation. Mol. Ecol. 26, 3330–3342. doi: 10.1111/mec.14126
Thiebot J. B., Cherel Y., Trathan P. N., Bost C. A. (2012). Coexistence of oceanic predators on wintering areas explained by population-scale foraging segregation in space or time. Ecology 93, 122–130. doi: 10.1890/11-0385.1
Thomé M. T. C., Carstens B. C., Rodrigues M. T., Galetti P. M. Jr., Alexandrino J., Haddad C. F. B. (2021). A role of asynchrony of seasons in explaining genetic differentiation in a Neotropical toad. Heredity 2021, 1–10. doi: 10.1038/s41437-021-00460-7
Ventura F., Catry P., Dias M. P., Breed G. A., Folch A., Granadeiro J. P. (2022). A central place foraging seabird flies at right angles to the wind to jointly optimize locomotor and olfactory search efficiency. Proc. Roy. Soc. B. 289, 1–10. doi: 10.1098/RSPB.2022.0895
Watanuki Y. (2002). Moonlight and activity of breeders and non-breeders of leach’s storm-petrels. J. Yam. Inst. Orn. 34, 245–249. doi: 10.3312/JYIO1952.34.245
Wilczek A. M., Roe J. L., Knapp M. C., Cooper M. D., Lopez-Gallego C., Martin L. J., et al. (2009). Effects of genetic perturbation on seasonal life history plasticity. Science 1979) 323, 930–934. doi: 10.1126/SCIENCE.1165826
Keywords: allochrony, sympatric speciation, petrels, storm-petrel, adaptation-by-time, sympatry, hydrobatidae
Citation: Medrano F, Militão T, Gomes I, Sardà-Serra M, de la Fuente M, Dinis HA and González-Solís J (2022) Phenological divergence, population connectivity and ecological differentiation in two allochronic seabird populations. Front. Mar. Sci. 9:975716. doi: 10.3389/fmars.2022.975716
Received: 22 June 2022; Accepted: 17 October 2022;
Published: 04 November 2022.
Edited by:
Patrick Jodice, Clemson University, United StatesReviewed by:
Daniel Paul Costa, University of California, Santa Cruz, United StatesIngrid L. Pollet, Acadia University, Canada
Cecilia Soldatini, Center for Scientific Research and Higher Education in Ensenada (CICESE), Mexico
Copyright © 2022 Medrano, Militão, Gomes, Sardà-Serra, de la Fuente, Dinis and González-Solís. This is an open-access article distributed under the terms of the Creative Commons Attribution License (CC BY). The use, distribution or reproduction in other forums is permitted, provided the original author(s) and the copyright owner(s) are credited and that the original publication in this journal is cited, in accordance with accepted academic practice. No use, distribution or reproduction is permitted which does not comply with these terms.
*Correspondence: Fernando Medrano, fernandomedranomartinez@gmail.com