- 1Earth Surface Process Modelling, German Research Centre for Geosciences (GFZ), Potsdam, Germany
- 2Institut Français de Recherche pour l'Exploitation de la Mer (IFREMER), Dyneco Pelagos, Plouzané, France
- 3Systems Ecology Group, Department of Integrated Modelling, Leibniz Centre for Tropical Marine Research (ZMT), Bremen, Germany
- 4Department of Mathematics and Statistics, University of Strathclyde, Glasgow, United Kingdom
- 5Southern Marine Science and Engineering Guangdong Laboratory, Guangzhou, China
- 6Ocean Sciences Department, University of California Santa Cruz, Santa Cruz, CA, United States
- 7Gulf of Maine Research Institute, Portland, ME, United States
- 8Mercator Ocean International, Toulouse, France
- 9Institute of Biochemistry and Biology, University of Potsdam, Potsdam, Germany
- 10Department of Biological Oceanography, Leibniz Institute for Baltic Sea Research, Rostock, Germany
- 11Institute for Chemistry and Biology of the Marine Environment, University of Oldenburg, Oldenburg, Germany
- 12Department of Biological Sciences, University of Bergen, Bergen, Norway
- 13International Ocean Carbon Coordination Project, Institute of Oceanology Polish Academy of Sciences, Sopot, Poland
- 14Helmholtz Centre for Ocean Research Kiel (GEOMAR), Kiel, Germany
- 15Institute of Biosciences and Bioresources, National Research Council of Italy, Sesto Fiorentino, Firenze, Italy
- 16Earth SURFACE Research Centre, Research Institute for Global Change, Japan Agency for Marine-Earth Science and Technology (JAMSTEC), Yokosuka, Japan
Ecosystem models need to capture biodiversity, because it is a fundamental determinant of food web dynamics and consequently of the cycling of energy and matter in ecosystems. In oceanic food webs, the plankton compartment encompasses by far most of the biomass and diversity. Therefore, capturing plankton diversity is paramount for marine ecosystem modelling. In recent years, many models have been developed, each representing different aspects of plankton diversity, but a systematic comparison remains lacking. Here we present established modelling approaches to study plankton ecology and diversity, discussing the limitations and strengths of each approach. We emphasize their different spatial and temporal resolutions and consider the potential of these approaches as tools to address societal challenges. Finally, we make suggestions as to how better integration of field and experimental data with modelling could advance understanding of both plankton biodiversity specifically and more broadly the response of marine ecosystems to environmental change, including climate change.
Introduction
Plankton diversity, in terms of traits and life history strategies, mediates some of the most important ecological processes, from local to planetary scales, including the biological carbon pump (Basu and Mackey, 2018), element cycling and food web dynamics (Sailley et al., 2015), energy transfer to higher trophic levels (Havens, 1998; Sommer et al., 2018) and system productivity (Hammer and Pitchford, 2005). However, we still do not fully understand how different dimensions of plankton biodiversity impact ecological functions and services at various spatial and temporal scales. These shortcomings limit our ability to project the magnitude or even direction of their change under future scenarios.
Various mathematical and statistical tools, generically termed “models”, are used to capture different dimensions of plankton diversity at various scales (e.g., Banas, 2011; D’Alelio et al., 2016; Righetti et al., 2019; Henson et al., 2021) and include particular effects of biodiversity in their projections. The specific underlying assumptions, strengths, and weaknesses of each approach may affect our interpretation of how plankton biodiversity impacts ecosystem functions. Furthermore, the necessary quantity, quality, and type of data required to validate each model differ, and in many cases observations are insufficient or not accessible for model validation (Grigoratou et al., 2022). Importantly, the diversity of modelling approaches also hinders the establishment of a dialogue and the transfer of information between data providers and users.
To address these issues, we provide a concise comparative assessment of common modelling approaches capturing plankton biodiversity to inform future choices of modelling methods and interpretation of results. We present our perspectives on the main strengths and limitations of these approaches, as well as their societal applications and data needs (Everett et al., 2017; Bardon et al., 2021).
Common modelling approaches to capture plankton biodiversity
Scientists from different fields of study have developed a wide variety of modelling approaches employed to study plankton biodiversity patterns, with different purposes, in some cases not even focused specifically on biodiversity. We aim to point out the general characteristics and examples of six commonly used approaches, which we categorize as: Statistical (STM), Ecological Network Analysis (ENA), Individual-Based (IBM), Plankton Functional Type (PFT), Acclimation (ACC) and Adaptive Trait-based (ATM) models. These approaches cover the common dimensions of biodiversity, including variability of genotypes, phenotypes, and the composition of communities and ecosystems (vertical axis on Figure 1). We further categorise these six modelling approaches along a “statistical vs. mechanistic” axis (horizontal axis on Figure 1), to better distinguish those models that require explicit descriptions of ecological or biological processes (mechanistic) from those methods that describe mainly empirical relationships based on field, satellite and laboratory observations (statistical).
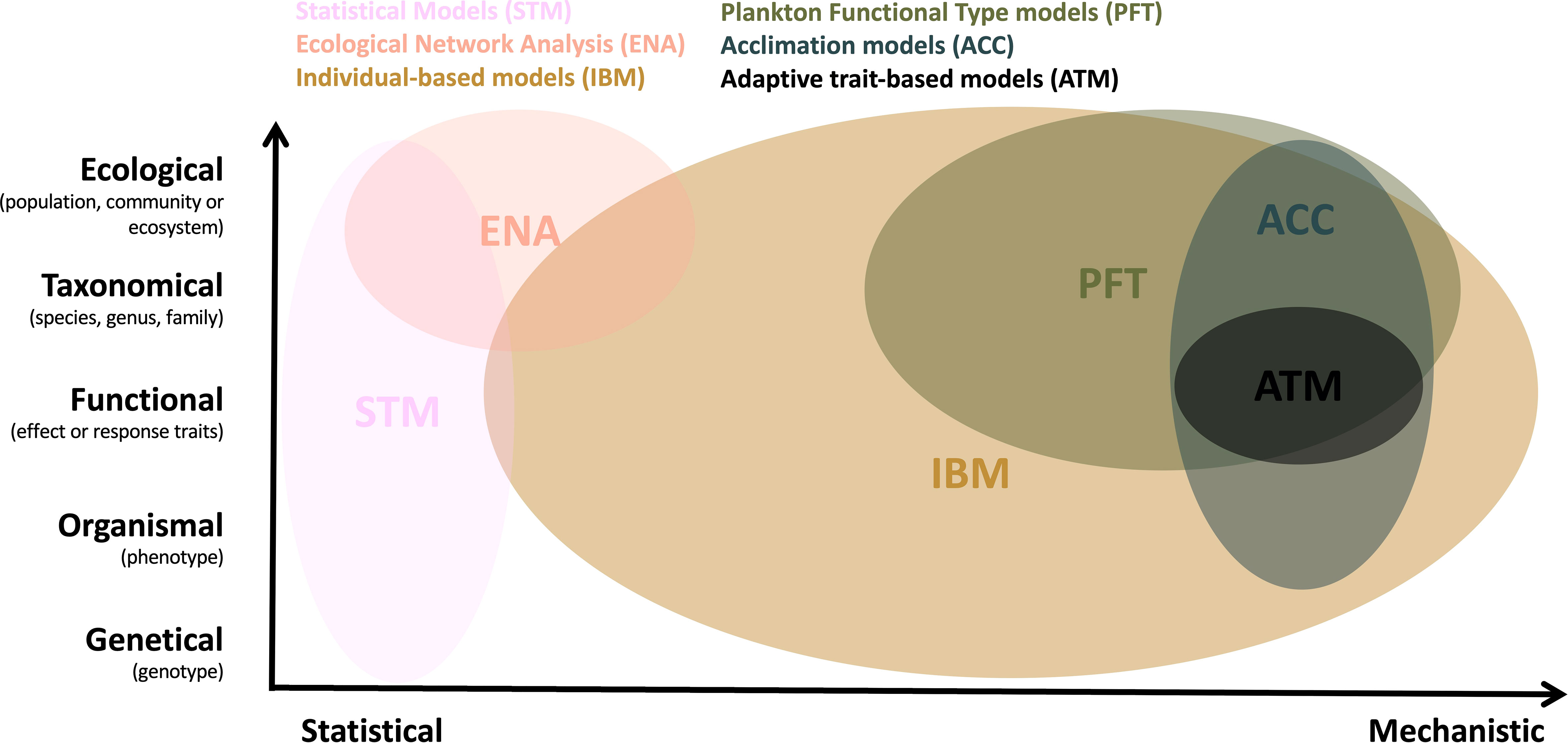
Figure 1 Six modelling approaches commonly used to capture various dimensions of plankton biodiversity. We further organize the modelling approaches along an axis spanning from those models that require explicit descriptions of ecological or biological processes (mechanistic) to those methods that do not (statistical).
Statistical models (STM) – interpreting natural diversity
These models describe observed patterns of plankton diversity using a myriad of statistical and machine learning methods. For the most common proxy of taxonomic biodiversity, species richness, various statistical and machine learning methods (Melo-Merino et al., 2020) can be used to develop species distribution models (SDMs) based on occurrence observations e.g. from open databases (GBIF1, OBIS2). Similarly, DNA/RNA sequencing data can be used to characterize diversity patterns of particular groups (e.g. viruses, bacteria, and diazotrophs). The recent availability and lower cost of these data have provided a global scale perspective on the diversity patterns of marine phytoplankton (Righetti et al., 2019), zooplankton (Brun et al., 2016), and of various planktonic groups of organisms (Ibarbalz et al., 2019). However, statistical approaches such as SDMs still face challenges associated with their static representation of dynamic marine ecosystems, where organisms disperse widely (Melo-Merino et al., 2020). Furthermore, the limited and biased distribution of observations limits our ability to untangle the temporal and spatial scale-dependance of species diversity (McGill et al., 2015). These methods are potentially useful to describe large-scale patterns with an ever-increasing set of observations, however, it remains difficult to unravel the mechanisms underlying diversity patterns and their links to ecosystem functions and services.
Ecological network analysis (ENA) – tools for ecosystem management
Models of static ecological networks provide a discrete representation of ecosystems by depicting energy flows from prey/resources to predators/consumers, assuming that all nodes are at steady state, i.e. equal net energy flows into and out of each trophic group. These networks are often built starting from biomass and energy budgets for each trophic group, based on metabolic parameters such as consumption/biomass and production/biomass ratios. Various studies apply ENA to model planktonic food web functioning. They employ information theory indices to assess ecosystem stability (Scotti et al., 2022), quantify carbon reuse through cycling analysis (Tecchio et al., 2016), and rely on input-output analysis to estimate transfer efficiency along a chain of discrete trophic levels (Saint-Béat et al., 2020). For example, D’Alelio et al. (2016) studied the structure of energy circulation and found little difference in trophic efficiency between phytoplankton bloom and non-bloom periods. ENA indices can also detect the impacts of anthropogenic stressors on planktonic food webs, and were used to show that eutrophication disrupts the prevalence of pathways for energy transfer to fish (Meddeb et al., 2018). Limitations of this approach include the strong assumption that each node remains at a steady state, and the amount of data required to validate models including many different trophic levels.
Individual-based models (IBM) – close to real life interactions and evolution
Individual-based models (IBMs, also called agent-based models) are iterative algorithms that apply a set of rules to, e.g., individuals of a population, thereby simulating life cycles, from birth to death (DeAngelis and Mooij, 2005; Grimm et al., 2005; Hellweger and Bucci, 2009). IBMs allow ecosystem properties to emerge from traits and interactions of individuals, including randomness through the process of replication, where offspring inherit traits from their parent(s), with mutation between each generation (Melián et al., 2011). Furthermore, events like random death or encounter may be modeled, which allows studying the importance of such discrete events in population dynamics (Picq et al., 2019). IBMs are used to investigate the effects of molecular and physiological processes on global plankton biogeography (Hellweger et al., 2014) and global N:P ratios (Toseland et al., 2013). In combination with individual-level observations, these models hold great potential to advance understanding of how individual-level processes impact multiple levels of ecological organization across spatial scales (Kreft et al., 2013; Hellweger et al., 2016). The general lack of any analytical treatment limits the derivation of insights for correlative analysis, but see Black and McKane (2012). The most important limitation for IBMs is likely their massive computational requirements for implementing simulations and analyzing the output.
Plankton functional type models (PFT)– modularity and function
Plankton functional type (PFT) models group organisms based on the similarity of their ecological or biogeochemical functionality. PFT models originated from the first, simple plankton community models resolving nutrients, phytoplankton, zooplankton and detritus (NPZD), and later the microbial loop (e.g. Fasham et al., 1990). As traditionally PFT models have been used to study the biogeochemical function of plankton, they have been criticized for representing poorly the eco-physiological differences between functional types (Anderson, 2005), and have recently been extended to include such detail by describing key traits and trade-offs (Follows et al., 2007; Follows and Dutkiewicz, 2011). PFT models are applied for time scales from days to millenia, and commonly used in regional, basin-scale and global applications for understanding and assessing the ecosystem response to environmental conditions, such as nutrient loading rates (Lancelot et al., 2007), acidification (Artioli et al., 2012), intensity of fishing efforts (Petrik et al., 2019), and climate change (Kwiatkowski et al., 2019). However, diversity is typically limited to the number of functional groups modelled, and is typically resolved in detail only for selected trophic levels, at substantial computational cost. This makes it challenging to analyse and disentangle diversity effects from other spatio-temporal dynamics. Furthermore, PFT models that describe trait-spaces typically assume fixed (non-adaptable) trait values along multiple trait dimensions, while resolving many fewer ecotypes than are observed in nature. This limits their ability to capture the adaptive capacity of ecosystems in response to environmental changes (Ward et al., 2019).
Acclimation models (ACC) – from physiology to community dynamics
Acclimation is the ability of organisms to adjust their physiology and behavior (phenotypes or traits that are not inherited) to enhance their fitness in a changing environment (Laws and Bannister, 1980; Smith et al., 2011). Although many models ignore this important ability, plankton acclimation models exist (Pahlow et al., 2008; Bonachela et al., 2013; Pahlow and Oschlies, 2013; Wirtz and Kerimoglu, 2016). It is challenging to disentangle the relative contributions of acclimation and evolutionary adaptation to overall ecosystem response, because in the short term they may have either similar or very different tendencies (Moreno and Martiny, 2018), which in the long term are inter-dependent (Edelaar and Bolnick, 2019). Representing physiological flexibilities as an acclimative response is an effective and efficient way of modelling certain effects of diversity, which avoids the heavy computational burden of representing biodiversity explicitly, e.g., by using multiple PFTs (see above). This approach has been used to study how physiological flexibilities impact the global and regional biogeography of elemental ratios and primary production (Pahlow et al., 2020), energy transfer efficiency to higher trophic levels (Chakraborty et al., 2020), response to eutrophication (Kerimoglu et al., 2018) and climate change (Kwiatkowski et al., 2018), and formation of deep chlorophyll maxima (Masuda et al., 2021). Acclimation models are often not standalone, and one of their strengths is that they are readily incorporated into a variety of models, such as PFTs, IBMs, and ATMs (see below), to represent plankton diversity (Ross et al., 2011; Smith et al., 2016; Chen et al., 2019; Cadier et al., 2020). Arguably the greatest limitation of ACCs is that optimality solutions can become quite intricate and, because of inter-dependencies between various cellular functions, any change in a model formulation may require the re-derivation of existing solutions.
Adaptive trait-based models (ATM) – describing trait dynamics of communities across ecological scales
Adaptive trait-based approaches focus on the dynamics of functional traits as key outputs, rather than inputs to models (Klausmeier et al., 2020), and they often overlap with more widely used modelling approaches such as PFTs and ACCs. ATMs commonly represent traits in two contrasting ways: as either a full distribution or an aggregate approximation via the moment-closure method (Wirtz and Eckhardt, 1996; Norberg et al., 2001; Merico et al., 2009). In the full distribution approach traits are “free” to evolve in response to the current selection pressure (Bruggeman and Kooijman, 2007; Banas, 2011; Gaedke and Klauschies, 2017). This approach can be computationally demanding for large-scale applications, which can hinder mechanistic understanding of biomass-trait feedbacks. In contrast, the aggregate approach, typically applied to communities, must assume a specific shape for the trait distribution, which makes it computationally efficient and allows direct insights into the mechanisms underlying changes in aggregate properties, namely total biomass, mean trait, and trait variance (Chen and Smith, 2018; Klauschies et al., 2018). The concise nature of the aggregate approach makes it useful for both applied (e.g. Terseleer et al., 2014; Acevedo-Trejos et al., 2015) and fundamental (e.g. Coutinho et al., 2016; Guill et al., 2021) research questions related to plankton diversity, but this comes at the cost of limited ability to accurately resolve the fitness landscape and inability to capture certain observed diversity distributions.
Discussion
The modelling approaches described above have both shared and contrasting characteristics, which allow them to address specific societal applications (see section 3.2 below). However, they all share a common need for improved data inputs (see section 3.3 below). We illustrate these differences and similarities with examples from the literature (Figure 2) and elaborate in the sections below.
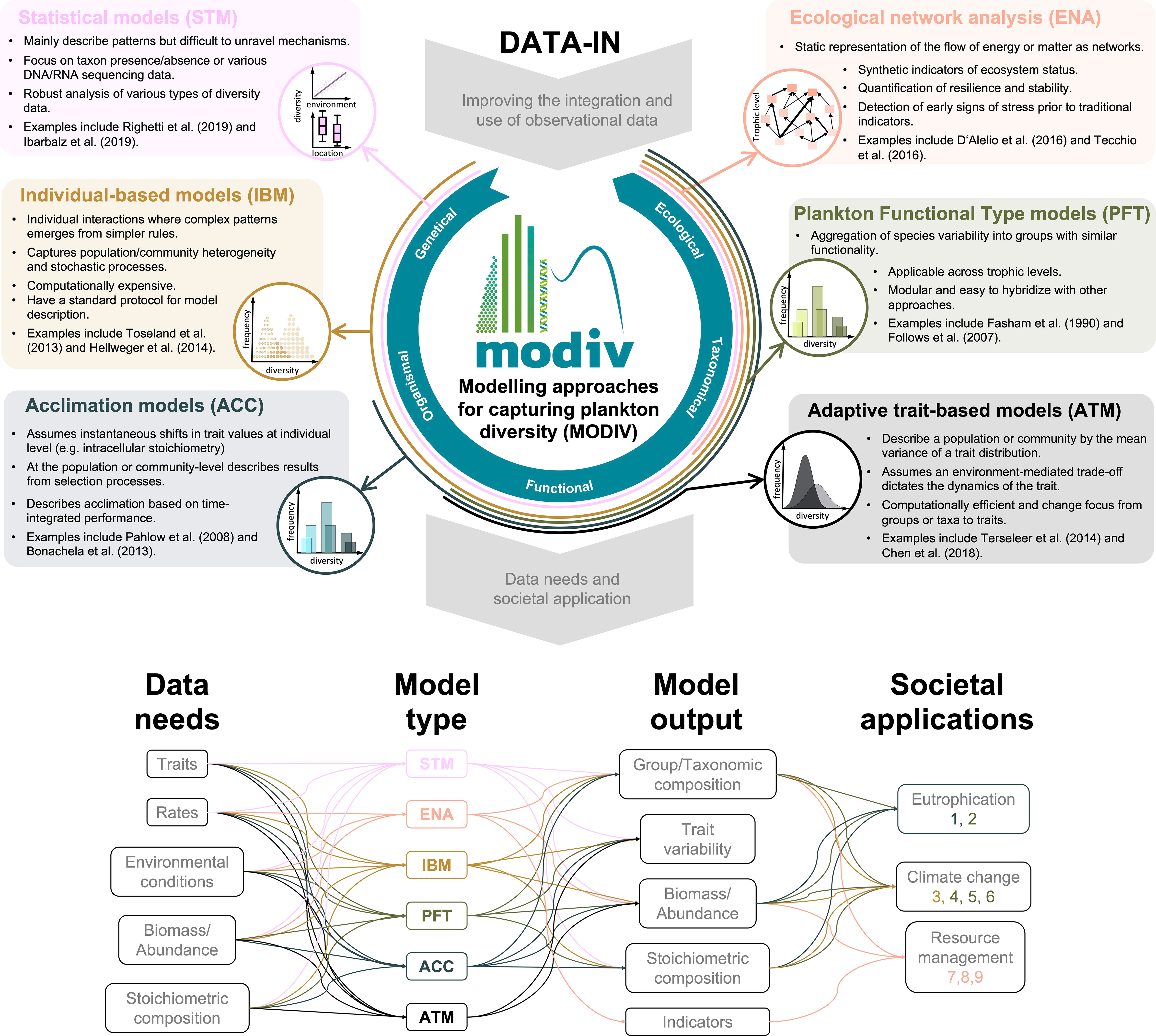
Figure 2 Key characteristics of six modelling approaches commonly used to capture various dimensions of plankton diversity. The inset of each model description depicts an idealized model output and where the term "diversity" represents a specific measure of diversity for each dimension, i.e. Genetical, Organismal, Functional, Taxonomical, or Ecological. A better overview of model outputs is available through the examples mentioned for each method. In addition, we summarized main data requirements, outputs and common societal applications for each approach. Notice that some entries can both be data needs and model outputs. The latter is because variables in a modelling approach can be used to parametrize and/or validate a model but can also be a variable computed or predicted by the model. Code numbers: 1) Kerimoglu et al. (2018) (ACC), 2) Lancelot et al. (2007) (PFT), 3) Toseland et al. (2013) (IBM), 4) Kwiatkowski et al. (2018) (PFT), 5) Kwiatkowski et al. (2019) (PFT), 6) Henson et al. (2021) (PFT), 7) de la Vega et al. (2018) (ENA), 8) Meddeb et al. (2018) (ENA), 9) Saint-Béat et al. (2020) (ENA).
Strengths and limitations
Strengths of these modelling approaches are that they (1) facilitate direct links and comparisons to observed diversity patterns (STMs, IBMs, ATMs), (2) have applications to societal benefits (STMs, ENAs, PFTs), (3) can capture multilevel ecological complexity (IBMs and ACCs), and (4) have computational efficiency and analytical accessibility (ACCs and ATMs). Some of their respective disadvantages are: (1) heavy computational requirements that limit applications (mainly IBMs, but also PFTs), (2) static representation of plankton dynamics (STMs, ENAs), and (3) lack of ecological or biological complexity (ENAs, PFTs, ATMs, ACCs). These strengths and limitations demonstrate that each of the above approaches are particularly suited to specific applications, with no single approach capturing all aspects of plankton diversity. Therefore, different approaches may be needed to capture biodiversity within different trophic levels or functional groups.
Societal applications
Some modelling approaches, such as ENA and PFTs, can inform managers and policy-makers about the status of ecosystem integrity and its deterioration, for example, due to anthropogenic stressors (de la Vega et al., 2018; Fath et al., 2019; Nogues et al., 2021). Trophic networks studied with ENA often include all ecosystem components, making them suitable to assess the balance between trophic guilds as requested by EU legislation (i.e. see MSFD descriptor D4C23). Moreover, ENA indices are among the food web indicators proposed in the framework of OSPAR4 to capture the whole-system properties of marine food webs (Niquil et al., 2014). Nevertheless, defining the reference levels or thresholds to quantify deviations from the Good Environmental Status (GES) remains challenging, as ecosystems attain different stable configurations over time (Tomczak et al., 2021). PFTs are used as the biogeochemical cores of Earth System Models (ESMs), thus they play a key role in future climate simulations. PFTs are also used to model Harmful Algal Blooms (HABs) and as part of larger models for predicting the effects of climate and fishing yield on key populations, which in turn inform stock assessments and policy decisions. Both ESM and fisheries models typically resolve only simplistic abstractions of plankton diversity (Kwiatkowski et al., 2019; Petrik et al., 2020), which is insufficient to capture functional diversity. This has raised concerns about the ability of ESMs and fisheries models to project future responses, especially in terms of community resilience and energy fluxes to higher trophic levels (Heneghan et al., 2016; Tittensor et al., 2021).
Unmet needs for data and collaboration
There is a growing need and recurrent call to contrast model estimates and predictions with empirical observations (Kremer et al., 2017). However, relevant traits across trophic levels remain scarcely measured, rarely consistently reported (different units, lack of adequate metadata including details on data quality and methods) and rarely shared in public data repositories (if so, typically with poor data latency). With only 7% of the ocean so far actively covered by long-term biological observations (Satterthwaite et al., 2021), current access to even the most basic biogeographic data on plankton biomass and abundance remains very limited (and virtually non-existent for microbes/protists) and often hampered by its spread over various platforms and institutions. This is despite the recognition of phytoplankton, zooplankton and microbial biomass and abundance as Essential Ocean Variables (EOV), Essential Biodiversity Variables (EBV) (Miloslavich et al., 2018) and even Essential Climate Variables (GCOS, 2021). Development and validation of all presented modelling approaches would benefit in particular from data on stoichiometric composition (C, N, P, Chl-a), traits (e.g. cell/body size and shape) and biomass measured across a full spectrum of environmental conditions (temperature, light, nutrients), with photosynthetic rates, nutrient uptake and respiration rates also critically needed. In addition, comparisons of modelling approaches with molecular data remain scarce, despite the recent availability of a considerable amount of data, which hold great potential as shown with individual-based comparisons (Hellweger, 2020). Obtaining quantitative individual-level data is crucial, yet remains a particular challenge because widely available genetic data cannot be converted accurately into biomass or cell abundance (Kelly et al., 2019; Piwosz et al., 2020; Milivojević et al., 2021).
We call for free, open access to plankton EOV and EBV data, and their enhanced and sustained observations, which would enable further integration of field observations, remote sensing products, and experimental studies with modelling approaches. Therefore, we advocate to follow the FAIR principles, i.e. Findability, Accessibility, Interoperability and Reusability (Wilkinson et al., 2016) for the sustainable management of plankton data. Adherence to these principles would ensure exploitation of the potential that the various approaches hold and foster building a new generation of models and decision-support tools for effective management of ecosystem services linked to plankton diversity.
More meaningful collaborations are also needed between the scientists who plan and conduct laboratory experiments, oceanic observations, and modelling studies. Proposals are rarely planned with a holistic view for combining experiments, observations and modelling. Hence, the scientists who develop and apply models are often not aware of the quantity and quality of available data relevant to their applications, while the scientists who plan and conduct laboratory experiments and oceanic observations are often not aware of the limitations of relevant modelling approaches and their results (e.g., Everett et al., 2017). The comparative assessment provided here aims to raise awareness and stimulate discussions in the planning phase of collaborative studies seeking to combine observations and modelling, which is the best and perhaps only hope for understanding any complex system (Bar-Yam, 2016).
Challenges and future directions
Approaches differ in their diversity-sustaining mechanisms, which relate to the positive effect of species diversity on productivity (Loreau, 2004). However, a higher diversity of coexisting species does not necessarily imply higher productivity. For example, although species can coexist via the competition-colonisation trade-off (i.e., species that are better at exploring unutilized spaces have poorer competitive capabilities), this coexistence does not lead to transgressive overyielding (Loreau, 2004). This may be particularly relevant in spatially explicit ocean models, where mixing can sustain plankton diversity (Chen et al., 2019; Dutkiewicz et al., 2020; Masuda et al., 2021). Another potentially relevant determinant of individual-level fitness (Edelaar and Bolnick, 2019), and therefore coexistence and diversity maintenance, is acclimative flexibility, which is being actively studied within the framework of Modern Coexistence Theory (Chesson, 2000; Barabás et al., 2018). One promising target for further research would be to examine how acclimation affects equalizing and stabilizing effects (Adler et al., 2007; Lankau, 2011). Since rapid evolution may promote species coexistence in diverse predator and prey communities by altering interspecific niche and fitness differences (Klauschies et al., 2016), we may also expect a positive impact of acclimation on species coexistence.
For understanding the response of plankton biodiversity in particular, and aquatic ecosystems more broadly, to global and other environmental changes, it is essential to develop better representations of the adaptive capacity of life in large scale simulations, e.g., in ESMs used to model climate change. Hence, there is a pressing need to validate ACC and ATM approaches in such spatially explicit applications. These approaches could potentially capture important feedback responses between ecology, evolution, and environmental conditions in ESMs (Bonan and Doney, 2018; Ward et al., 2019).
Author contributions
FP and EA-T contributed with study and manuscript design, model descriptions and revisions. SC, BC, SYC, MG, CG, CH, OK, TK, CL, AP, AR, MS, SS, and SV contributed with model descriptions and revisions. All authors participated and actively contributed to the MODIV workshop, which delineated the ideas summarized in this manuscript. All authors contributed to the article and approved the submitted version.
Funding
The German Research Foundation (DFG) supported EA-T (AC 331/1-1) and OK (KE 1970/2-1). BC was supported by Southern Marine Science and Engineering Guangdong Laboratory (Guangzhou) (SMSEGL20SC02) and a Leverhulme Trust Research Project Grant (RPG-2020-389). MG was supported by National Science Foundation (OCE-1851866). CL received funding from the EU Horizons 2020 project SUMMER (817806). AP received funding from the European Union’s Horizon 2020 Research and Innovation Programme under Grant Agreement No. 869383 (ECOTIP), and acknowledges support from the United States National Science Foundation grant OCE-2140395 to the Scientific Committee on Oceanic Research (SCOR, United States) for International Ocean Carbon Coordination Project. AR was partly supported by the Federal Ministry of Education and Research BMBF Germany Project PEKRIS II (03F0828). The German Federal Agency for Nature Conservation (Bundesamt für Naturschutz, BfN) provided financial support to MS with funds from the Federal Ministry of the Environment, Nature Conservation and Nuclear Safety (BMU), under grant agreement FKZ: 3521532201. SS was supported by a JRPs-LEAD grant from the Japan Society for the Promotion of Science. SV was supported by Trond Mohn Research Foundation starting grant TMS2018REK02. FP was supported by the GEOMAR Helmholtz Centre for Ocean Research Kiel. Addional financial support to MODIV was provided by the FILAMO project, funded by The Research Council of Norway.
Acknowledgments
This paper and the related Foresight Workshop MODIV have been supported by EuroMarine. We thank the Marine Biodiversity Observing Network (MBON), the DFG-Priority Programme DynaTrait (SPP 1704), the Centre for Ocean Life, the organisers and participants of the International Workshops on Trait-based Approaches to Ocean Life, and the International Ocean Carbon Coordination Project for their support and for fostering a decade of collaborations and exchange around the topic of plankton diversity.
Conflict of interest
The authors declare that the research was conducted in the absence of any commercial or financial relationships that could be construed as a potential conflict of interest.
Publisher’s note
All claims expressed in this article are solely those of the authors and do not necessarily represent those of their affiliated organizations, or those of the publisher, the editors and the reviewers. Any product that may be evaluated in this article, or claim that may be made by its manufacturer, is not guaranteed or endorsed by the publisher.
Footnotes
- ^ Global Biodiversity Information Facility https://www.gbif.org/
- ^ Ocean Biodiversity Information System https://obis.org/
- ^ https://mcc.jrc.ec.europa.eu/main/index.py
- ^ The Convention for the Protection of the Marine Environment of the North-East Atlantic - www.ospar.org
References
Acevedo-Trejos E., Brandt G., Bruggeman J., Merico A. (2015). Mechanisms shaping phytoplankton community structure and diversity in the ocean. Sci. Rep. 5, 8918. doi: 10.1038/srep08918
Adler P. B., HilleRisLambers J., Levine J. M. (2007). A niche for neutrality. Ecol. Lett. 10 (2), 95–104. doi: 10.1111/j.1461-0248.2006.00996.x
Anderson T. R. (2005). Plankton functional type modelling: Running before we can walk? J. Plankton Res. 27, 1073–1081. doi: 10.1093/plankt/fbi076
Artioli Y., Blackford J. C., Butenschön M., Holt J. T., Wakelin S. L., Thomas H., et al (2012). The carbonate system in the north Sea: Sensitivity and model validation. J. Mar. Syst. 102, 1–13. doi: 10.1016/j.jmarsys.2012.04.006
Banas N. S. (2011). Adding complex trophic interactions to a size-spectral plankton model: Emergent diversity patterns and limits on predictability. Ecol. Modell. 222, 2663–2675. doi: 10.1016/j.ecolmodel.2011.05.018
Barabás G., D'Andrea R., Stump S. M. (2018). Chesson's coexistence theory. Ecol. Monogr. 88 (3), 277–303. doi: 10.1002/ecm.1302
Bardon L. R., Ward B. A., Dutkiewicz S., Cael B. B. (2021). Testing the skill of a species distribution model using a 21st century virtual ecosystem. Geophys. Res. Lett. 48 (22), e2021GL093455. doi: 10.1029/2021GL093455
Bar-Yam Y. (2016). The limits of phenomenology: From behaviorism to drug testing and engineering design. Complexity 21 (S1), 181–189. doi: 10.1002/cplx.21730
Basu S., Mackey K. R. M. (2018). Phytoplankton as key mediators of the biological carbon pump: Their responses to a changing climate. Sustainability 10 (3), 869. doi: 10.3390/su10030869
Black A. J., McKane A. J. (2012). Stochastic formulation of ecological models and their applications. Trends Ecol. Evol. 27, 337–345. doi: 10.1016/j.tree.2012.01.014
Bonachela J. A., Allison S. D., Martiny A. C., Levin S. A. (2013). A model for variable phytoplankton stoichiometry based on cell protein regulation. Biogeosciences 10, 4341–4356. doi: 10.5194/bg-10-4341-2013
Bonan G. B., Doney S. C. (2018). Climate, ecosystems, and planetary futures: The challenge to predict life in earth system models. Science 359, eaam8328. doi: 10.1126/science.aam8328
Bruggeman J., Kooijman S. A. L. M. (2007). A biodiversity-inspired approach to aquatic ecosystem modeling. Limnol. Oceanogr. 52, 1533–1544. doi: 10.4319/lo.2007.52.4.1533
Brun P., Payne M. R., Kiørboe T. (2016). Trait biogeography of marine copepods – An analysis across scales. Ecol. Lett. 19, 1403–1413. doi: 10.1111/ele.12688
Cadier M., Hansen A. N., Andersen K. H., Visser A. W. (2020). Competition between vacuolated and mixotrophic unicellular plankton. J. Plankton Res. 42, 425–439. doi: 10.1093/plankt/fbaa025
Chakraborty S., Cadier M., Visser A. W., Bruggeman J., Andersen K. H. (2020). Latitudinal variation in plankton traits and ecosystem function. Glob. Biogeochem. Cycles 32, e2020GB006564. doi: 10.1029/2020GB006564
Chen B., Smith S. L. (2018). CITRATE 1.0: Phytoplankton continuous trait-distribution model with one-dimensional physical transport applied to the north pacific. Geosci. Model. Dev. 11, 467–495. doi: 10.5194/gmd-11-467-2018
Chen B., Smith S. L., Wirtz K. W. (2019). Effect of phytoplankton size diversity on primary productivity in the north pacific: Trait distributions under environmental variability. Ecol. Lett. 22, 56–66. doi: 10.1111/ele.13167
Chesson P. (2000). Mechanisms of maintenance of species diversity. Ann. Rev. Ecol. Syst. 31, 343–366. doi: 10.1146/annurev.ecolsys.31.1.343
Coutinho R., Klauschies T., Gaedke U. (2016). Bimodal trait distributions with large variances question the reliability of trait-based aggregate models. Theor. Ecol. 9, 389–408. doi: 10.1007/s12080-016-0297-9
D’Alelio D., Libralato S., Wyatt T., Ribera D’Alcalà M. (2016). Ecological-network models link diversity, structure and function in the plankton food-web. Sci. Rep. 6, 1–13. doi: 10.1038/srep21806
DeAngelis D. L., Mooij W. M. (2005). Individual-based modeling of ecological and evolutionary processes. Annu. Rev. Ecol. Evol. Syst. 36, 147–168. doi: 10.1146/annurev.ecolsys.36.102003.152644
de la Vega C., Schückel U., Horn S., Kröncke I., Asmus R., Asmus H. (2018). How to include ecological network analysis results in management? A case study of three tidal basins of the wadden Sea, south-eastern north Sea. Ocean Coast. Manage. 163, 401–416. doi: 10.1016/j.ocecoaman.2018.07.019
Dutkiewicz S., Cermeno P., Jahn O., Follows M. J., Hickman A. E., Taniguchi D. A. A., et al (2020). Dimensions of marine phytoplankton diversity. Biogeosciences 17, 609–634. doi: 10.5194/bg-17-609-2020
Edelaar P., Bolnick D. I. (2019). Appreciating the mulitple processes increasing individual or population fitness. Trends Ecol. Evol. 34, 435–446. doi: 10.1016/j.tree.2019.02.001
Everett J. D., Baird M. E., Buchanan P., Bulman C., Davies C., Downie R., et al (2017). Modeling what we sample and sampling what we model: Challenges for zooplankton model assessment. Front. Mar. Sci. 4. doi: 10.3389/fmars.2017.00077
Fasham M. J. R., Ducklow H. W., Mckelvie S. M. (1990). A nitrogen-based model of plankton dynamics in the oceanic mixed layer. J. Mar. Res. 48, 591–639. doi: 10.1357/002224090784984678
Fath B. D., Asmus H., Asmus R., Baird D., Borrett S. R., de Jonge V. N., et al (2019). Ecological network analysis metrics: The need for an entire ecosystem approach in management and policy. Ocean Coast. Manage. 174, 1–14. doi: 10.1016/j.ocecoaman.2019.03.007
Follows M. J., Dutkiewicz S. (2011). Modeling diverse communities of marine microbes. Ann. Rev. Mar. Sci. 3, 427–451. doi: 10.1146/annurev-marine-120709-142848
Follows M. J., Dutkiewicz S., Grant S., Chisholm S. W. (2007). Emergent biogeography of microbial communities in a model ocean. Science 315, 1843–1846. doi: 10.1126/science.1138544
Gaedke U., Klauschies T. (2017). Analyzing the shape of observed trait distributions enables a data-based moment closure of aggregate models. Limnol. Oceanogr. Methods 15 (12), 979–994. doi: 10.1002/lom3.10218
GCOS (2021). The status of the global climate observing system 2021: The GCOS status report (GCOS-240) (Geneva: WMO). Available at: https://gcos.wmo.int/en/gcos-status-report-2021.
Grigoratou M., Montes E., Richardson A. J., Everett J. D., Acevedo-Trejos E., Anderson C., et al (2022). The marine biodiversity observation network plankton workshops: Plankton ecosystem function, biodiversity, and forecasting–research requirements and applications. Limnol. Oceanogr. Bull. 31 (1), 1–4. doi: 10.1002/lob.10479
Grimm V., Revilla E., Berger U., Jeltsch F., Mooij W. M., Railsback S. F., et al (2005). Pattern-oriented modeling of agent-based complex systems: Lessons from ecology. Science 310, 987–991. doi: 10.1126/science.1116681
Guill C., Huelsemann J., Klauschies T. (2021). Self-organized pattern formation increases local diversity in metacommunities. Ecol. Lett. 24, 2624–2634. doi: 10.1111/ele.13880
Hammer A. C., Pitchford J. W. (2005). The role of mixotrophy in plankton bloom dynamics, and the consequences for productivity. ICES J. Mar. Sci. 62 (5), 833–840. doi: 10.1016/j.icesjms.2005.03.001
Havens K. E. (1998). Size structure and energetics in a plankton food web. Oikos 81 (2), 346–358. doi: 10.2307/3547055
Hellweger F. L. (2020). Combining molecular observations and microbial ecosystem modeling: A practical guide. Ann. Rev. Mar. Sci. 12, 1–23. doi: 10.1146/annurev-marine-010419-010829
Hellweger F. L., Bucci V. (2009). A bunch of tiny individuals-individual-based modeling for microbes. Ecol. Modell. 220, 8–22. doi: 10.1016/j.ecolmodel.2008.09.004
Hellweger F. L., Clegg R. J., Clark J. R., Plugge C. M., Kreft J. U. (2016). Advancing microbial sciences by individual-based modelling. Nat. Rev. Microbiol. 14, 461–471. doi: 10.1038/nrmicro.2016.62
Hellweger F. L., Van Sebille E., Fredrick N. D. (2014). Biogeographic patterns in ocean microbes emerge in a neutral agent-based model. Science 345, 1346–1349. doi: 10.1126/science.1254421
Heneghan R. F., Everett J. D., Blanchard J. L., Richardson A. J. (2016). Zooplankton are not fish: Improving zooplankton realism in size-spectrum models mediates energy transfer in food webs. Front. Mar. Sci. 3. doi: 10.3389/fmars.2016.00201
Henson S. A., Cael B. B., Allen S. R., Dutkiewicz S. (2021). Future phytoplankton diversity in a changing climate. Nat. Commun. 12, 1–8. doi: 10.1038/s41467-021-25699-w
Ibarbalz F. M., Henry N., Brandão M. C., Martini S., Busseni G., Byrne H., et al (2019). Global trends in marine plankton diversity across kingdoms of life. Cell 179, 1084–1097.e21. doi: 10.1016/j.cell.2019.10.008
Kelly R. P., Shelton A. O., Gallego R. (2019). Understanding PCR processes to draw meaningful conclusions from environmental DNA studies. Sci. Rep. 9, 1–14. doi: 10.1038/s41598-019-48546-x
Kerimoglu O., Große F., Kreus M., Lenhart H.-J., van Beusekom J. E. (2018). A model-based projection of historical state of a coastal ecosystem: Relevance of phytoplankton stoichiometry. Sci. Total Environ. 639, 1311–1323. doi: 10.1016/j.scitotenv.2018.05.215
Klauschies T., Coutinho R. M., Gaedke U. (2018). A beta distribution-based moment closure enhances the reliability of trait-based aggregate models for natural populations and communities. Ecol. Modell. 381, 46–77. doi: 10.1016/j.ecolmodel.2018.02.001
Klauschies T., Vasseur D. A., Gaedke U. (2016). Trait adaptation promotes species coexistence in diverse predator and prey communities. Ecol. Evol. 6, 4141–4159. doi: 10.1002/ece3.2172
Klausmeier C. A., Kremer C. T., Koffel T. (2020). “Trait-based ecological eco-evolutionary theory,” in Thoeretical ecology: Concepts and applications. Eds. McCann K. S., Gellner G. (Oxford, UK: Oxford University Press).
Kreft J.-U., Plugge C. M., Grimm V., Prats C., Leveau J. H. J., Banitz T., et al (2013). Mighty small: Observing and modeling individual microbes becomes big science. Proc. Natl. Acad. Sci. 110, 18027–18028. doi: 10.1073/pnas.1317472110
Kremer C. T., Williams A. K., Finiguerra M., Fong A. A., Kellerman A., Paver S. F., et al (2017). Realizing the potential of trait-based aquatic ecology: New tools and collaborative approaches trait-based. Limnol. Oceanogr. 62, 253–271. doi: 10.1002/lno.10392
Kwiatkowski L., Aumont O., Bopp L. (2019). Consistent trophic amplification of marine biomass declines under climate change. Glob. Change Biol. 25 (1), 218–229. doi: 10.1111/gcb.14468
Kwiatkowski L., Aumont O., Bopp L., Ciais P. (2018). The impact of variable phytoplankton stoichiometry on projections of primary production, food quality, and carbon uptake in the global ocean. Glob. Biogeochem. Cycles 32, 516–528. doi: 10.1002/2017GB005799
Lancelot C., Gypens N., Billen G., Garnier J., Roubeix V. (2007). Testing an integrated river–ocean mathematical tool for linking marine eutrophication to land use: The phaeocystis-dominated Belgian coastal zone (Southern north Sea) over the past 50 years. J. Mar. Syst. 64 (1-4), 216–228. doi: 10.1016/j.jmarsys.2006.03.010
Lankau R. A. (2011). Rapid evolutionary change and the coexistence of species. Ann. Rev. Ecol. Evo. Syst. 42, 335–354. doi: 10.1146/annurev-ecolsys-102710-145100
Laws E. A., Bannister T. T. (1980). Nutrient-and light-limited growth of thalassiosira fluviatilis in continuous culture, with implications for phytoplankton growth in the ocean. Limnol. Oceanogr. 25 (3), 457–473. doi: 10.4319/lo.1980.25.3.0457
Loreau M. (2004). Does functional redundancy exist? Oikos 104 (3), 606–611. doi: 10.1111/j.0030-1299.2004.12685.x
Masuda Y., Yamanaka Y., Smith S. L., Hirata T., Nakano H., Oka A., et al (2021). Photoacclimation by phytoplankton determines the distribution of global subsurface chlorophyll maxima in the ocean. Commun. Earth Environ. 2, 1–8. doi: 10.1038/s43247-021-00201-y
McGill B. J., Dornelas M., Gotelli N. J., Magurran A. E. (2015). Fifteen forms of biodiversity trend in the anthropocene. Trends Ecol. Evol. 30, 104. doi: 10.1016/j.tree.2014.11.006
Meddeb M., Grami B., Chaalali A., Haraldsson M., Niquil N., Pringault O., et al (2018). Plankton food-web functioning in anthropogenically impacted coastal waters (SW Mediterranean sea): An ecological network analysis. Prog. Oceanogr. 162, 66–82. doi: 10.1016/j.pocean.2018.02.013
Melián C. J., Vilas C., Baldó F., González-Ortegón E., Drake P., Williams R. J. (2011). Eco-evolutionary dynamics of individual-based food webs. Adv. Ecol. Res. 45, 225–268. doi: 10.1016/B978-0-12-386475-8.00006-X
Melo-Merino S. M., Reyes-Bonilla H., Lira-Noriega A. (2020). Ecological niche models and species distribution models in marine environments: A literature review and spatial analysis of evidence. Ecol. Modell. 415, 108837. doi: 10.1016/j.ecolmodel.2019.108837
Merico A., Bruggeman J., Wirtz K. (2009). A trait-based approach for downscaling complexity in plankton ecosystem models. Ecol. Modell. 220, 3001–3010. doi: 10.1016/j.ecolmodel.2009.05.005
Milivojević T., Rahman S. N., Raposo D., Siccha M., Kucera M., Morard R. (2021). High variability in SSU rDNA gene copy number among planktonic foraminifera revealed by single-cell qPCR. ISME Commun. 1, 1–8. doi: 10.1038/s43705-021-00067-3
Miloslavich P., Bax N. J., Simmons S. E., Klein E., Appeltans W., Aburto-Oropeza O., et al (2018). Essential ocean variables for global sustained observations of biodiversity and ecosystem changes. Glob. Change Biol. 24, 2416–2433. doi: 10.1111/gcb.14108
Moreno A. R., Martiny A. C. (2018). Ecological stoichiometry of ocean plankton. Ann. Rev. Mar. Sci. 10, 43–69. doi: 10.1146/annurev-marine-121916-063126
Niquil N., Le Loc’h F., Tecchio S., Chaalali A., Vouriot P., Mialet B., et al (2014). “Ongoing research on ecosystem health indicators for food webs in the MSFD context,” in Trans-Channel Forum Proceedings “Science and Governance of the Channel Marine Ecosystem”. (Caen, France: INTERREG IV A Programme, Université de Caen Basse-Normandie) 14–15.
Nogues Q., Raoux A., Araignous E., Chaalali A., Hattab T., Leroy B., et al (2021). Cumulative effects of marine renewable energy and climate change on ecosystem properties: Sensitivity of ecological network analysis. Ecol. Indic. 121, 107128. doi: 10.1016/j.ecolind.2020.107128
Norberg J., Swaney D. P., Dushoff J., Lin J., Casagrandi R., Levin S. A. (2001). Phenotypic diversity and ecosystem functioning in changing environments: A theoretical framework. Proc. Natl. Acad. Sci. 98, 11376–11381. doi: 10.1073/pnas.171315998
Pahlow M., Chien C.-T., Arteaga L. A., Oschlies A. (2020). Optimality-based non-redfield plankton–ecosystem model (OPEM v1.1) in UVic-ESCM 2.9 – part 1: Implementation and model behaviour. Geosci. Model. Dev. 13, 4663–4690. doi: 10.5194/gmd-13-4663-2020
Pahlow M., Oschlies A. (2013). Optimal allocation backs droop’s cell-quota model. Mar. Ecol. Prog. Ser. 473, 1–5. doi: 10.3354/meps10181
Pahlow M., Vézina A. F., Casault B., Maass H., Malloch L., Wright D. G., et al (2008). Adaptive model of plankton dynamics for the North Atlantic. Prog. Oceanogr. 76, 151–191. doi: 10.1016/j.pocean.2007.11.001
Petrik C. M., Stock C. A., Andersen K. H., van Denderen P. D., Watson J. R. (2019). Bottom-up drivers of global patterns of demersal, forage, and pelagic fishes. Prog. Oceanogr. 176, 102124. doi: 10.1016/j.pocean.2019.102124
Petrik C. M., Stock C. A., Andersen K. H., van Denderen P. D., Watson J. R. (2020). Large Pelagic fish are most sensitive to climate change despite pelagification of ocean food webs. Front. Mar. Sci. 7. doi: 10.3389/fmars.2020.588482
Picq S., Scotti M., Puebla O. (2019). Behavioural syndromes as a link between ecology and mate choice: A field study in a reef fish population. Anim. Behav. 150, 219–237. doi: 10.1016/j.anbehav.2019.02.016
Piwosz K., Shabarova T., Pernthaler J., Posch T., Šimek K., Porcal P., et al (2020). Bacterial and eukaryotic small-subunit amplicon data do not provide a quantitative picture of microbial communities, but they are reliable in the context of ecological interpretations. mSphere 5, e00052-20. doi: 10.1128/msphere.00052-20
Righetti D., Vogt M., Gruber N., Psomas A., Zimmermann N. E. (2019). Global pattern of phytoplankton diversity driven by temperature and environmental variability. Sci. Adv. 5, 1–11. doi: 10.1126/sciadv.aau6253
Ross O. N., Geider R. J., Berdalet E., Artigas M. L., Piera J. (2011). Modelling the effect of vertical mixing on bottle incubations for determining in situ phytoplankton dynamics. i. growth rates. Mar. Ecol. Prog. Ser. 435, 13–31. doi: 10.3354/meps09194
Sailley S. F., Polimene L., Mitra A., Atkinson A., Allen J. I. (2015). Impact of zooplankton food selectivity on plankton dynamics and nutrient cycling. J. Plankt. Res. 37 (3), 519–529. doi: 10.1093/plankt/fbv020
Saint-Béat B., Fath B. D., Aubry C., Colombet J., Dinasquet J., Fortier L., et al (2020). Contrasting pelagic ecosystem functioning in eastern and western Baffin bay revealed by trophic network modeling. Elementa: Sci. Anthropocene 8, 1. doi: 10.1525/elementa.397
Satterthwaite E. V., Bax N. J., Miloslavich P., Ratnarajah L., Canonico G., Dunn D., et al (2021). Establishing the foundation for the global observing system for marine life. Front. Mar. Sci. 8. doi: 10.3389/fmars.2021.737416
Scotti M., Bondavalli C., Rossetti G., Bodini A. (2022). Flow network indices signal a directional change in ecosystems: Evidence from a small mountain lake (Lake Santo, northern Italy). Indic 139, 108896. doi: 10.1016/j.ecolind.2022.108896
Smith S. L., Pahlow M., Merico A., Wirtz K. W. (2011). Optimality-based modeling of planktonic organisms. Limnol. Oceanogr. 56, 2080–2094. doi: 10.4319/lo.2011.56.6.2080
Smith S. L., Vallina S. M., Merico A. (2016). Phytoplankton size-diversity mediates an emergent trade-off in ecosystem functioning for rare versus frequent disturbances. Sci. Rep. 6, 34170. doi: 10.1038/srep34170
Sommer U., Charalampous E., Scotti M., Moustaka-Gouni M. (2018). Big fish eat small fish: Implications for food chain length? Community Ecol. 19, 107–115. doi: 10.1556/168.2018.19.2.2
Tecchio S., Chaalali A., Raoux A., Rius A. T., Lequesne J., Girardin V., et al (2016). Evaluating ecosystem-level anthropogenic impacts in a stressed transitional environment: The case of the seine estuary. Ecol. Indic. 61, 833–845. doi: 10.1016/j.ecolind.2015.10.036
Terseleer N., Bruggeman J., Lancelot C., Gypens N. (2014). Trait-based representation of diatom functional diversity in a plankton functional type model of the eutrophied southern north Sea. Limnol. Oceanogr. 59, 1–16. doi: 10.4319/lo.2014.59.6.0000
Tittensor D. P., Novaglio C., Harrison C. S., Heneghan R. F., Barrier N., Bianchi D., et al (2021). Next-generation ensemble projections reveal higher climate risks for marine ecosystems. Nat. Clim. Change 11, 973–981. doi: 10.1038/s41558-021-01173-9
Tomczak M. T., Müller-Karulis B., Blenckner T., Ehrnsten E., Eero M., Gustafsson B., et al (2021). Reference state, structure, regime shifts, and regulatory drivers in a coastal sea over the last century: The central Baltic Sea case. Limnol. Oceanogr. 67, S266–S284. doi: 10.1002/lno.11975
Toseland A., Daines S. J., Clark J. R., Kirkham A., Strauss J., Uhlig C., et al (2013). The impact of temperature on marine phytoplankton resource allocation and metabolism. Nat. Clim. Change 3, 1–6. doi: 10.1038/nclimate1989
Ward B. A., Collins S., Dutkiewicz S., Gibbs S., Bown P., Ridgwell A., et al (2019). Considering the role of adaptive evolution in models of the ocean and climate system. J. Adv. Model. Earth Syst. 11, 3343–3361. doi: 10.1029/2018MS001452
Wilkinson M. D., Dumontier M., Aalbersberg I. J., Appleton G., Axton M., Baak A., et al (2016). The FAIR guiding principles for scientific data management and stewardship. Sci. Data 3, 160018. doi: 10.1038/sdata.2016.18
Wirtz K.-W., Eckhardt B. (1996). Effective variables in ecosystem models with an application to phytoplankton succession. Ecol. Modell. 92, 33–53. doi: 10.1016/0304-3800(95)00196-4
Keywords: species distribution models, ecological network analysis, individual-based models, plankton functional type, acclimation, adaptation, trait-based
Citation: Acevedo-Trejos E, Cadier M, Chakraborty S, Chen B, Cheung SY, Grigoratou M, Guill C, Hassenrück C, Kerimoglu O, Klauschies T, Lindemann C, Palacz A, Ryabov A, Scotti M, Smith SL, Våge S and Prowe F (2022) Modelling approaches for capturing plankton diversity (MODIV), their societal applications and data needs. Front. Mar. Sci. 9:975414. doi: 10.3389/fmars.2022.975414
Received: 22 June 2022; Accepted: 29 July 2022;
Published: 16 August 2022.
Edited by:
Rachel Ann Foster, Stockholm University, SwedenReviewed by:
Jeffrey Morris, University of Alabama at Birmingham, United StatesCopyright © 2022 Acevedo-Trejos, Cadier, Chakraborty, Chen, Cheung, Grigoratou, Guill, Hassenrück, Kerimoglu, Klauschies, Lindemann, Palacz, Ryabov, Scotti, Smith, Våge and Prowe. This is an open-access article distributed under the terms of the Creative Commons Attribution License (CC BY). The use, distribution or reproduction in other forums is permitted, provided the original author(s) and the copyright owner(s) are credited and that the original publication in this journal is cited, in accordance with accepted academic practice. No use, distribution or reproduction is permitted which does not comply with these terms.
*Correspondence: Esteban Acevedo-Trejos, esteban.acevedo-trejos@gfz-potsdam.de; Friederike Prowe, fprowe@geomar.de