- 1School of GeoSciences, University of Edinburgh, Edinburgh, United Kingdom
- 2Whale Wise, Swansea, United Kingdom
- 3School of Mathematics and Maxwell Institute for Mathematical Sciences, University of Edinburgh, Edinburgh, United Kingdom
- 4WhalesBermuda, Hamilton, Bermuda
We constructed annual abundance of a migratory baleen whale at an oceanic stopover site to elucidate temporal changes in Bermuda, an area with increasing anthropogenic activity. The annual abundance of North Atlantic humpback whales visiting Bermuda between 2011 and 2020 was estimated using photo-identification capture-recapture data for 1,204 whales, collected between December 2009 and May 2020. Owing to a sparse data set, we combined a Cormack-Jolly-Seber (CJS) model, fit through maximum likelihood estimation, with a Horvitz-Thompson estimator to calculate abundance and used stratified bootstrap resampling to derive 95% confidence intervals (CI). We accounted for temporal heterogeneity in detection and sighting rates via a catch-effort model and, guided by goodness-of-fit testing, considered models that accounted for transience. A model incorporating modified sighting effort and time-varying transience was selected using (corrected) Akaike’s Information Criterion (AICc). The survival probability of non-transient animals was 0.97 (95% CI 0.91-0.98), which is comparable with other studies. The rate of transience increased gradually from 2011 to 2018, before a large drop in 2019. Abundance varied from 786 individuals (95% CI 593-964) in 2016 to 1,434 (95% CI 924-1,908) in 2020, with a non-significant linear increase across the period and interannual fluctuations. These abundance estimates confirm the importance of Bermuda for migrating North Atlantic humpback whales and should encourage a review of cetacean conservation measures in Bermudian waters, including area-based management tools. Moreover, in line with the time series presented here, regional abundance estimates should be updated across the North Atlantic to facilitate population monitoring over the entire migratory range.
1 Introduction
Abundance time series are critical to wildlife management as they can inform conservation status and determine underlying drivers of observed trends (Lawton, 1993; Taylor and Gerrodette, 1993; Mace et al., 2008). As a population metric, abundance is relatively easy to understand and interpret, and values can be compared across different time periods (Moore and Barlow, 2011), populations (Barlow et al., 2011), and species (Víkingsson et al., 2015). Further, variation in abundance may relate to other population-scale changes (Madon et al., 2013). For example, temporal trends in baleen whale abundance have been related to variation in mortality, body condition, reproductive rates, and species range shifts (Moore, 2005; Patrician and Kenney, 2010; Ramp et al., 2014; Becker et al., 2019; Simard et al., 2019; Kügler et al., 2020). Combined, such indices allow an integrated assessment of population responses to environmental change and stressors (Hazen et al., 2019), and inform local-regional marine management (Gabriele et al., 2017).
The abundance of baleen whales is frequently estimated through capture-recapture (CR) methods (Hammond et al., 2021). Applied to humpback whales (Megaptera novaeangliae [Borowski 1781]), this typically involves photo-identification of naturally occurring markings on tail flukes to determine individual sighting histories over time (e.g., Katona and Whitehead, 1981; Franklin et al., 2020). Values for parameters including survival, detection and abundance are then estimated. To minimize bias of abundance estimates, analyses may account for heterogeneity in survival and detection, including features such as: transient animals, which are only available within the study system once across the study period (Pradel et al., 1997; Madon et al., 2013); temporal heterogeneity due to variable sighting effort (Marucco et al., 2009; Monnahan et al., 2019); and individual detection heterogeneity (IDH), where detection varies as a function of individual attributes and habitat features (Hammond, 1990; Cubaynes et al., 2010). CR methods have been used to construct humpback whale abundance time series at local, regional, and basin scales (e.g., Barlow et al., 2011; Madon et al., 2013; Monnahan et al., 2019). These have been used to inform conservation status for the global and regional populations (Bettridge et al., 2015; Cooke, 2018) and to inform species management (International Whaling Commission, 2009).
Abundance estimates for humpback whales rarely incorporate all parts of a population’s range. Like other mysticetes, humpback whales are capital breeders, undertaking long-distance annual migrations between low-latitude winter breeding grounds and high-latitude summer feeding grounds (Mackintosh, 1946; Carwardine, 2019). During this migration, animals may use stopover sites as transitory stops, from days to weeks, for activities such as feeding, resting, socializing or information gathering (Linscott and Senner, 2021). Stopover sites can be coastal, such as Hervey Bay in East Australia (Franklin et al., 2021), but are often oceanic, such as the Kermadec Islands in Oceania (Owen et al., 2019) and the Rio Grande Rise in the Southwest Atlantic (Horton et al., 2020). To date, whale abundance time series have been largely derived for the ‘end points’ of migration – feeding and breeding grounds (e.g., Chaloupka et al., 1999; Schleimer et al., 2019). These abundance estimates are crucial to monitor population size (Stevick et al., 2003; Punt et al., 2006). In contrast, there are few abundance estimates or time series for stopover sites, particularly mid-ocean areas, which may provide more information about migratory patterns in space and time (Findlay et al., 2011).
In the present study, we investigate humpback whale abundance around Bermuda, a North Atlantic mid-ocean stopover site (Figure 1; Payne and McVay, 1971; Stevenson, 2011). The North Atlantic is considered to be a discrete population of humpback whales (Cooke, 2018) with two distinct population segments (DPS), listed as ‘not at risk’ in the Caribbean breeding area and ‘endangered’ at Cape Verde (NOAA, 2016). The Caribbean DPS is subdivided into northern and southeastern Caribbean breeding grounds; and the principal feeding grounds are the Gulf of Maine, eastern Canada, West Greenland, Iceland, and north of Norway (Smith et al., 1999; Reeves et al., 2002; Stevick et al., 2003; Stevick et al., 2018; Wenzel et al., 2020), with each feeding ground considered as a separate management unit (International Whaling Commission, 2002; Hayes et al., 2019). Long-distance migration routes in the North Atlantic are not coastal (Stevick et al., 2006; Kennedy et al., 2014) and there are few mid-ocean migratory stopovers, including Bermuda and the Azores (Visser et al., 2011; Cucuzza et al., 2015). Following extensive commercial hunting in the 19th and 20th centuries (Smith and Reeves, 2010) and a commercial whaling ban in 1955 (Best, 1993), the population size of North Atlantic humpback whales steadily increased through the end of the 20th century (Punt et al., 2006). From CR analysis, modeled annual increase ranged from 1.2% to 3.1% in the principal breeding ground in the northern Caribbean, 1979-1992 (Stevick et al., 2003; Punt et al., 2006). Rates of annual increase varied widely across feeding grounds, from 3% in the Gulf of Maine, 2009-2016, determined by CR (Robbins and Pace, 2018); to ~15% in Icelandic waters, 1987-2001 (Pike et al., 2005), and a sharp decline in the West Greenland feeding ground, 2007-2015 (Heide-Jørgensen and Laidre, 2015; Hansen et al., 2018), determined by line transect sampling. Total abundance in the North Atlantic is still thought to be far below pre-exploitation population size (Punt et al., 2006; Ruegg et al., 2013), although the carrying capacity may have changed due to environmental shifts (Tulloch et al., 2019). To our knowledge, abundance estimates have not been provided for any part of this population more recently than 2016 (Robbins and Pace, 2018). Moreover, in 2018, the International Whaling Commission (IWC) recommended a range-wide, in-depth assessment for North Atlantic humpback whales, including abundance (International Whaling Commission, 2019).
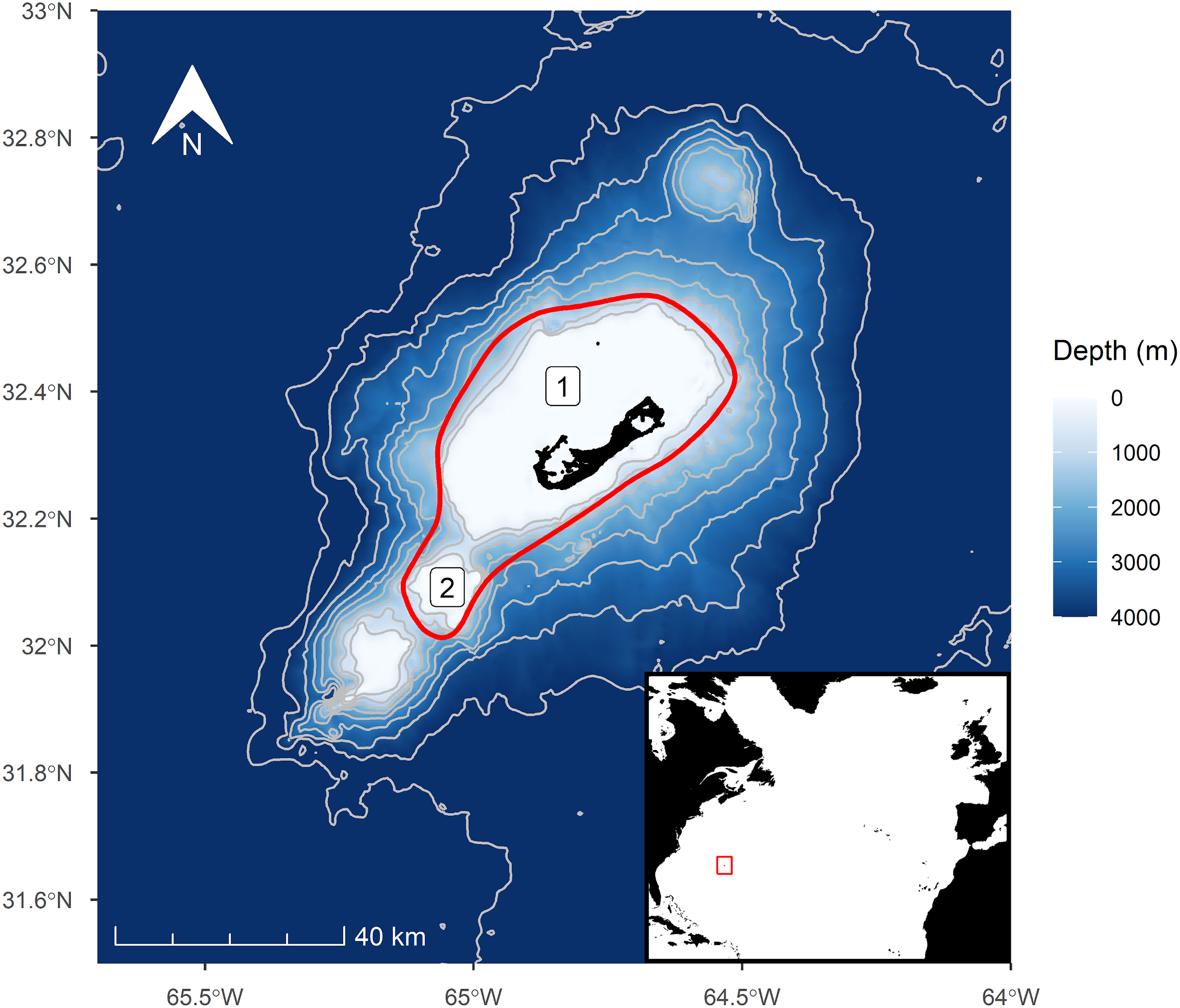
Figure 1 Map of the study area, the waters around Bermuda, and its position within the North Atlantic (inset). The approximate search area is denoted by the red line. The study area encompasses two major bathymetric features, 1) the Bermuda Platform and 2) Challenger Bank.
Bermuda has strong migratory connections to other parts of the North Atlantic, with evidence of stopover behavior. Using photo-identification, individuals sighted in Bermuda have been matched to all major feeding grounds, in addition to North Carolina (USA) and Franz Josef Land (Russia); and both Caribbean breeding grounds (Katona and Whitehead, 1981; Stone et al., 1987; A. S., unpublished data; Happywhale1). Re-sighting rates suggest that Bermuda is primarily a stopover between the northwest Caribbean and western feeding grounds (Beaudette et al., 2009). Meanwhile, breeding behavior in the form of extensive seasonal singing activity has been recorded at the Bermuda stopover site (Homfeldt et al., 2022), as well as preliminary evidence of foraging (Stone et al., 1987; Hamilton et al., 1997; Stevenson, 2011), aggregation and competitive (Stevenson, pers. comm.) behaviors, during south- and northbound migration.
Due to Bermuda’s position along the humpback whale migratory route, the exclusive economic zone was declared a Marine Mammal Sanctuary in 2012, as part of a ‘Sister Sanctuary Agreement’ with western North Atlantic feeding and breeding areas (NOAA and Government of Bermuda, 2012). However, this designation confers no additional legal protection to humpback whales, and the importance of these waters as a stopover site has not been confirmed through abundance estimates until the present study. With persistent and increasing levels of regional human activity, particularly large vessel traffic (Roberts, 2011; Halpern et al., 2015; Širović et al., 2016), and the announcement of a Blue Economy Strategy for Bermuda (Bermuda Ocean Prosperity Programme, 2021), such evidence could underpin future designations that do carry legally binding management measures, such as Particularly Sensitive Sea Areas (PSSAs; Kachel, 2008), to mitigate risks to humpback whales posed by these activities.
Here, we use a CR framework to model the seasonal abundance of humpback whales around Bermuda from 2011 to 2020 using photo-identification sightings. The data set exhibits low inter-seasonal resighting rates and the resulting model framework does not provide an abundance estimate for the first season of data collection (2010). To reduce bias in our abundance estimates, we account for temporal heterogeneity in sighting effort and whale occurrence, and formally test for both transience and IDH in our data to guide subsequent model fitting and selection. We discuss the potential of this time series to inform marine management in Bermudian waters and establish new baselines to monitor changes through the migratory range of North Atlantic humpback whales.
2 Materials and methods
2.1 Data collection
Between December 2009 and May 2020, annual humpback whale photo-identification surveys were conducted from a small vessel (6.5-10 m length) in coastal and offshore waters around Bermuda (Figure 1). Surveys took place between December and May, the period during which humpback whales typically occur (A. S., unpublished data), and were daily whenever possible. In 2020, survey effort was limited by Covid-19 restrictions in Bermuda (Table 1). All surveys were conducted in calm weather (Beaufort Sea State<3, no precipitation, swell<2 m) with good visibility, and typically spanned daylight hours. Surveys followed a haphazard regime to maximize encounters with whales and focused spatially on the southwestern Bermuda Platform and the Challenger Bank seamount (Figure 1; Vogt and Jung, 2007), although survey routes and sighting locations were not consistently recorded. Surveys were conducted in closing mode; whales were approached on detection and a focal follow of variable duration was conducted at each encounter (Altmann, 1974), which generally lasted 20-30 minutes and up to 2 hours. When possible, the ventral side of the tail flukes of each encountered animal was photographed with a digital SLR camera (Nikon D200, 2010-2017; Nikon D700, 2018-2020; 70-300 mm lens).
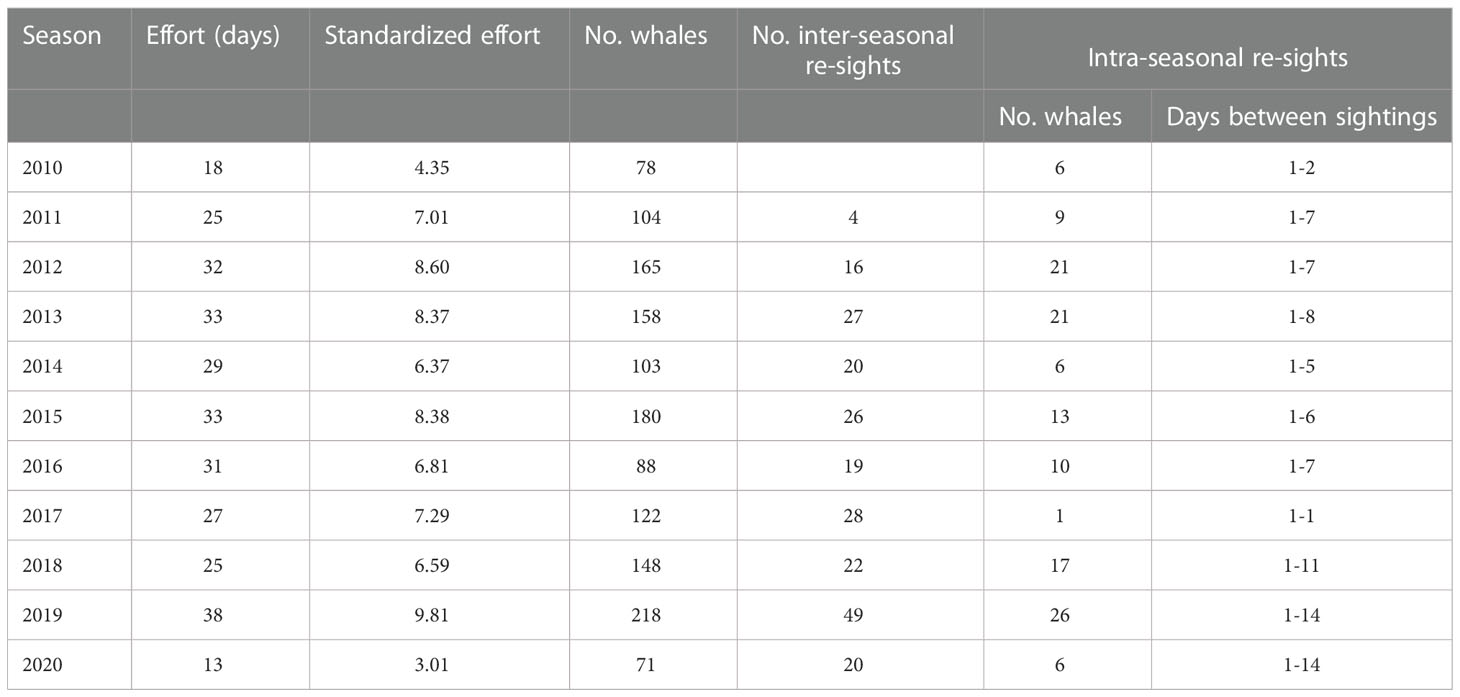
Table 1 Survey effort (absolute and standardized), sightings, inter-seasonal re-sights (from all previous years within the period) and intra-seasonal re-sights for each season (December-May, e.g., the 2010 season corresponds to December 2009-May 2010), determined through photo-identification.
Images were used for individual photo-identification via scarring patterns and coloration, following Calambokidis et al. (2001). Briefly, two trained researchers independently assigned each image a score of highest quality (1) to lowest quality (5) for five variables against photographic archetypes: proportion of fluke visible, fluke angle (relative to the water), lateral angle of the photographer, sharpness, and lighting. Scores from both researchers were then compared: where there was a discrepancy, the lowest quality (highest score) was retained. We adopted a conservative approach wherein photographs with a score of ≥4 in any category were rejected. Photographs were analyzed independently by two teams and matches detected by only one team were verified by the other.
Data were processed in two ways. For simplicity, each December-May season was named according to the January-May calendar year (e.g., the 2010 season refers to the period December 2009-May 2010). First, to explore residency and support existing evidence that Bermuda is used as a stopover site (a transitory stop, used by whales during long-distance migration for days to weeks), we determined the number of animals that were re-sighted within each season. We calculated the duration (in days) between first and last sightings for each animal within each season, which can be used to infer the minimum residence time in Bermuda. Because Bermuda is thought to encompass south- and north-bound migrations, we removed any durations greater than 20 days. Second, for capture-recapture modeling, each animal was recorded as present or absent for each season, resulting in a summary record of seasonal sighting histories. This was used to determine the number of inter-seasonal re-sights each season (from all previous seasons within the study period) and estimate abundance.
2.2 Model construction
Abundance trends were derived from annual sighting histories using capture-recapture (CR) methods (for example: King, 2014; McCrea and Morgan, 2015; Seber and Schofield, 2019; Hammond et al., 2021). Annual abundance was defined as the total number of animals passing through the approximate search area in a single December-May season. All model construction and analyses were performed in R (R Core Team, 2020), and outputs were visualized with the ggplot2 package (Wickham, 2016). CR models were fitted using MARK (White and Burnham, 1999), accessed through R using the RMark package (Laake and Rexstad, 2012).
With the majority of whales only sighted in one season across all survey years, the data set is information-poor, increasing the risk of over-parameterization and limiting the complexity of suitable CR frameworks (Lebreton et al., 1992; Forster, 2000). Therefore, using a two-step approach, we initially fit a modified Cormack-Jolly-Seber (CJS; Lebreton et al., 1992) model to the data, and then used the fitted model estimates to calculate annual abundance (Cubaynes et al., 2010 season). We also investigated the use of the more complex Jolly-Seber-Schwarz-Arnason (JSSA; Jolly, 1965; Seber, 1965; Schwarz and Arnason, 1996) and Pollock’s closed robust design (Pollock, 1982) models. However, for these data, both models led to identifiability issues in the estimation of model parameters (associated with boundary estimates) and thus were not investigated further.
The CJS framework provides estimates of apparent survival and detection, from which abundance can be subsequently derived. The model can be modified to account for variable survey effort, transience, and IDH. We defined each year as a single capture occasion. CJS conditions on the first sighting of each animal, so that we are not able to estimate the associated capture probabilities for the first season of the study, which consequently means that abundance estimates cannot be produced for the first capture occasion (i.e., 2010; Cubaynes et al., 2010). Capture histories were modeled as a function of two parameters: apparent survival rate, Φ, and per-animal detection probability, p. Parameters were linked to covariates with an inverse logit function and values were estimated from observed data using a maximum likelihood estimation (MLE) approach. To estimate total annual abundance, we followed Cubaynes et al. (2010) and used a Horvitz-Thompson estimator (Borchers et al, 2002; Horvitz and Thompson, 1952; McDonald and Amstrup, 2001) such that:
where N̂i denotes the estimated annual total abundance, p̂i the estimated detection probability and ni the number of sighted animals at occasion i. A stratified bootstrap approach (1000 replicates) was used to calculate 95% confidence intervals for p, Φ and N (Morgan, 2008; King and McCrea, 2019; Worthington et al., 2021). For each bootstrap replicate, the number of animals first sighted in a given year was set equal to the observed value.
Core CJS model assumptions include that each animal has an independent fate; that identifying marks are retained and recorded correctly; and that all emigration is permanent (Lebreton et al., 1992). Calves were excluded from the present study and humpback whales are thought to form only loose, temporary associations (Valsecchi et al., 2002), suggesting that individuals behave independently from one another. The long-term reliability of humpback whale fluke photo-identification has been exhaustively demonstrated (Katona et al., 1979; Katona and Whitehead, 1981; Carlson et al., 1990; Stevick et al., 2001; Franklin et al., 2020). Temporary emigration in this system was defined as animals that did not visit Bermuda for one or more seasons (years). Despite limited information, temporary emigration is thought to occur in humpback whale breeding grounds (Brown et al., 1995; Madon et al., 2013; Kowarski et al., 2018) and may occur at the Bermuda stopover site, which would violate a model assumption and could contribute to a transience signal.
Within the basic CJS framework, Φ and p can be constant or time dependent. Modifications to this framework were considered to account for heterogeneous detection and survival, based on system knowledge and goodness-of-fit testing, to minimize bias of abundance estimates.
2.2.1 Capture effort
Detection probability p is likely to vary between years as a function of seasonal survey effort (Marucco et al., 2009; Monnahan et al., 2019). However, in terms of explaining detection probabilities, the number of survey days is a coarse and perhaps naïve estimate of effort, given that a) the number of whales present changes during a season, with a consistent peak in sightings in March and April across all years; and b) the temporal distribution of effort varies between seasons (Figure 2). To account for this, we derived a standardized effort value for each season i (efforts,i). First, we calculated the mean number of whales seen per day (WPD) for a given calendar month m over all seasons (WPDm), defined as:
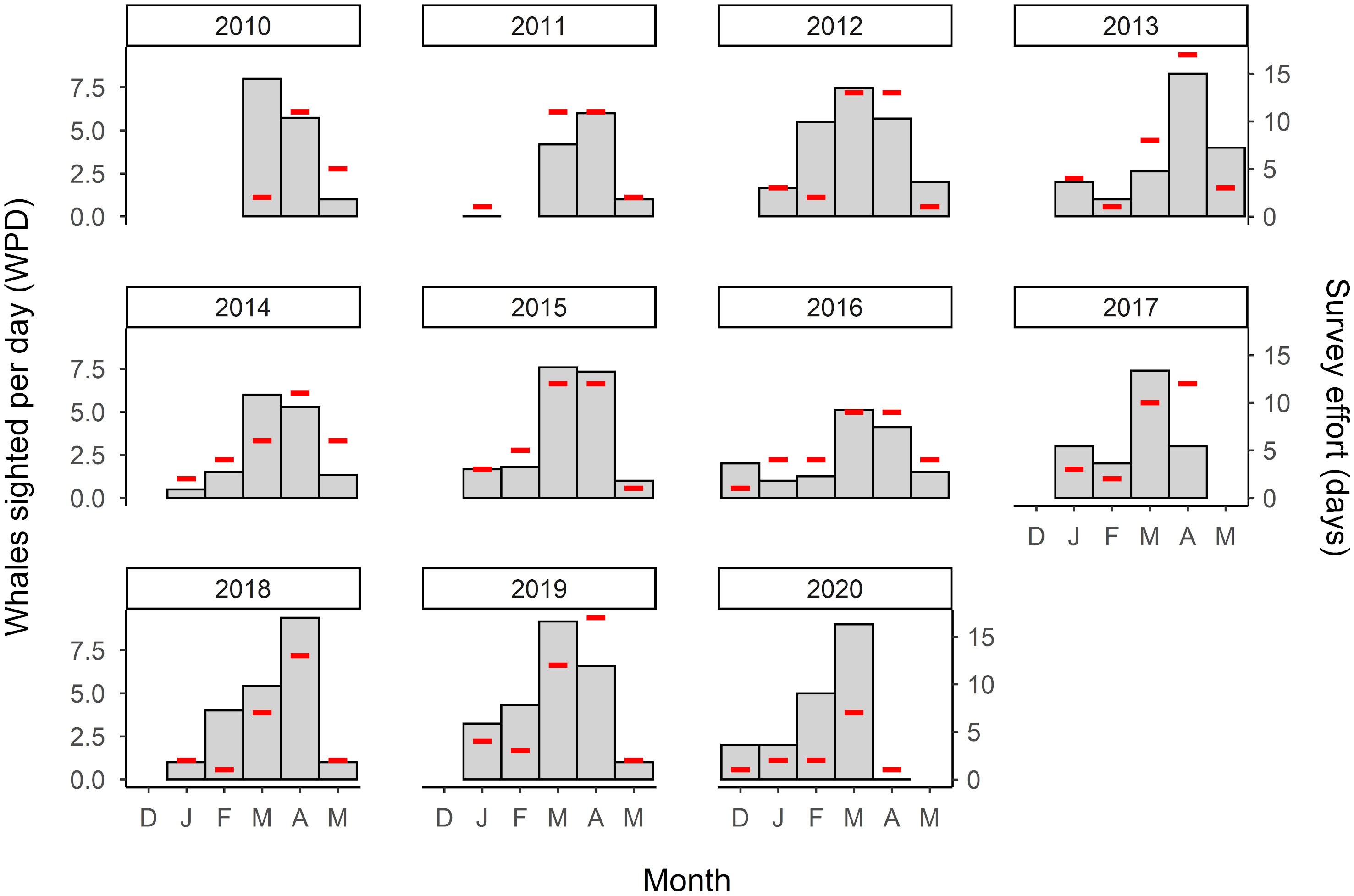
Figure 2 Whales sighted per day (WPD; gray bars, left-hand y-axis) and number of days of survey effort (red lines, right-hand y-axis) for each survey month and season.
where sightingsm is the total number of sightings (one sighting is equal to one identifiable whale seen at least once on one day) and daysm is the total number of effort days, for calendar month m across all seasons. We then derived an associated correction factor for each month (corm) as:
This correction factor is an empirical distribution of the average WPD across months, and thus takes into account differences over time relating to whale presence in the area. Finally, we defined the standardized effort (efforts) for season i as:
where efforti,m denotes the number of days of effort for month m in season i. In this way, our standardized effort accounts for intra-seasonal patterns in whale occurrence and the heterogeneous temporal distribution of effort between seasons (years). We considered models in which standardized effort was included as a covariate with p via logistic regression.
2.2.2 Transience
We considered the presence of transients, defined as animals that visited Bermuda in only one season during the study period. By definition, transients cannot be observed again and are unavailable for recapture within the study (i.e., have zero “survival” probability; Pradel et al., 1997). Transience has been detected in humpback whale breeding grounds (Constantine et al., 2012; Madon et al., 2013; Chero et al., 2020), although the source of the signal is uncertain. Failure to account for this feature will typically lead to an overestimation of abundance (Madon et al., 2013; Genovart and Pradel, 2019), due to an associated underestimation of capture probability.
We used goodness-of-fit (GoF) testing, implemented through the R2ucare package (Choquet et al., 2009; Gimenez et al., 2018), to investigate the presence of transient animals. A significant chi-squared test result (χ2 = 121, df = 44, p< 0.0001) suggested a poor fit of the basic CJS model to sighting histories. This test was decomposed to four interpretable components using contingency tables; the TEST 3.SR (Pradel et al., 2005) component yielded a significant result (Z = 89, p< 0.0001), indicating that newly encountered and previously encountered animals have different probabilities of subsequent re-sighting. This is typically interpreted as a strong signal for transience and removing this component improved goodness of fit considerably (χ2 = 32, df = 35, p = 0.60). To account for transience, we followed Pradel et al. (1997) and structured Φ into two age classes (Φ ~ transient), 0 and 1+ years, where ‘age’ is the time elapsed since first capture and not the actual age of the animal. Φ0 (model age 0) can be interpreted as the combined apparent survival of ‘transient’ animals (zero survival probability) and ‘resident’ animals, and Φ1+ (model age 1+) as the survival of residents alone. The proportion of transients among newly sighted animals, τ, was estimated using:
The proportion of transients in the population, T, was then estimated via,
where E(ui) is the expected number of newly sighted animals and E(mi) the expected number of re-sighted animals. Following Perret et al. (2003), we used observed ui and mi as estimates for expected values.
We considered models in which Φ0 and Φ1+ were constant (single value) or time-varying in an additive way (Φ ~ time + transient). Additionally, to account for the possibility that temporal variation in Φ was driven by variable transience and not survival, we considered models in which Φ0 varied over time but Φ1+ was constant by using age class as a dummy variable (Φ ~ time:transient; Laake and Rexstad, 2012).
2.2.3 Individual detection heterogeneity
Detectability may also vary between animals as a function of individual attributes, including fluke-up behavior for humpback whales (Barendse et al., 2011) and habitat features, such that each animal within the study system has a unique sighting probability (Cubaynes et al., 2010; Gimenez and Choquet, 2010). Failing to account for individual detection heterogeneity (IDH) can lead to an underestimation of abundance (Hammond, 1990; Hwang and Huggins, 2005; Cubaynes et al., 2010). To test for this feature, following Jeyam et al. (2018), we applied tests of positive association between previous and future encounters using Goodman-Kruskal’s gamma. A global version of the test (all capture occasions pooled) was only marginally significant (γ = 0.47, p = 0.03). From occasion-specific tests, only 2013, 2015 and 2016 were (marginally) significant. Therefore, modifications of the detection process p to account for IDH were not included. Of note, in a preliminary analysis, including IDH had little effect on parameter estimates but did lead to some additional identifiability issues.
2.2.4 Model selection
In total, we fitted and compared 16 CJS models, constructed from combinations of the different parameter specifications: three for p (constant, time, standardized effort) and five for Φ (constant, time, transient, time + transient, time:transient). Selection of the final, best-fitting model was achieved with Akaike’s Information Criterion corrected for small sample size (AICc; Burnham and Anderson, 2004), where a low score indicates improved model fit offset against model complexity, supplemented with visual inspection of parameter values and associated confidence intervals. The final annual abundance estimates were linearly regressed against time to detect a significant temporal trend. The regression was weighted by the inverse of the variance of each abundance estimate to account for varying precision (Stevick et al., 2003; Kutner et al., 2005; Somerford et al., 2022).
3 Results
In total, 304 days of surveys were conducted between December 2009 and May 2020. Seasonal survey effort ranged from 13 days (2020 season) to 38 days (2019 season; Table 1), with a mean of 27 days (SD = 7.2 days). Seasonal standardized effort, which accounted for intra-seasonal variation in whale sighting rates, varied from 3.01 (2020) to 9.81 (2019), with a mean of 6.96 (SD = 1.95). Out of 2,038 sightings with fluke images, 1,594 sightings on 276 days had images of sufficient quality (a score of ≤3 in all five variables). From these filtered sightings, 1,204 individual whales were identified (Figure 3). The number of identifiable whales per season ranged from 71 (2020) to 218 (2019; Table 1, Figure 3), with a mean of 130 whales (SD = 47 whales). The majority of whales (1,042, 87%) were sighted in only one year, with whales sighted in up to seven different seasons (one whale). The number of animals that were re-sighted between years ranged from 4 (2011) to 49 (2019; Table 1) for each year of re-sighting. Between 1 (2017) and 26 (2019) animals were re-sighted within each season, with a maximum duration of 14 days between first and last sightings (after removing durations >20 days; Table 1).
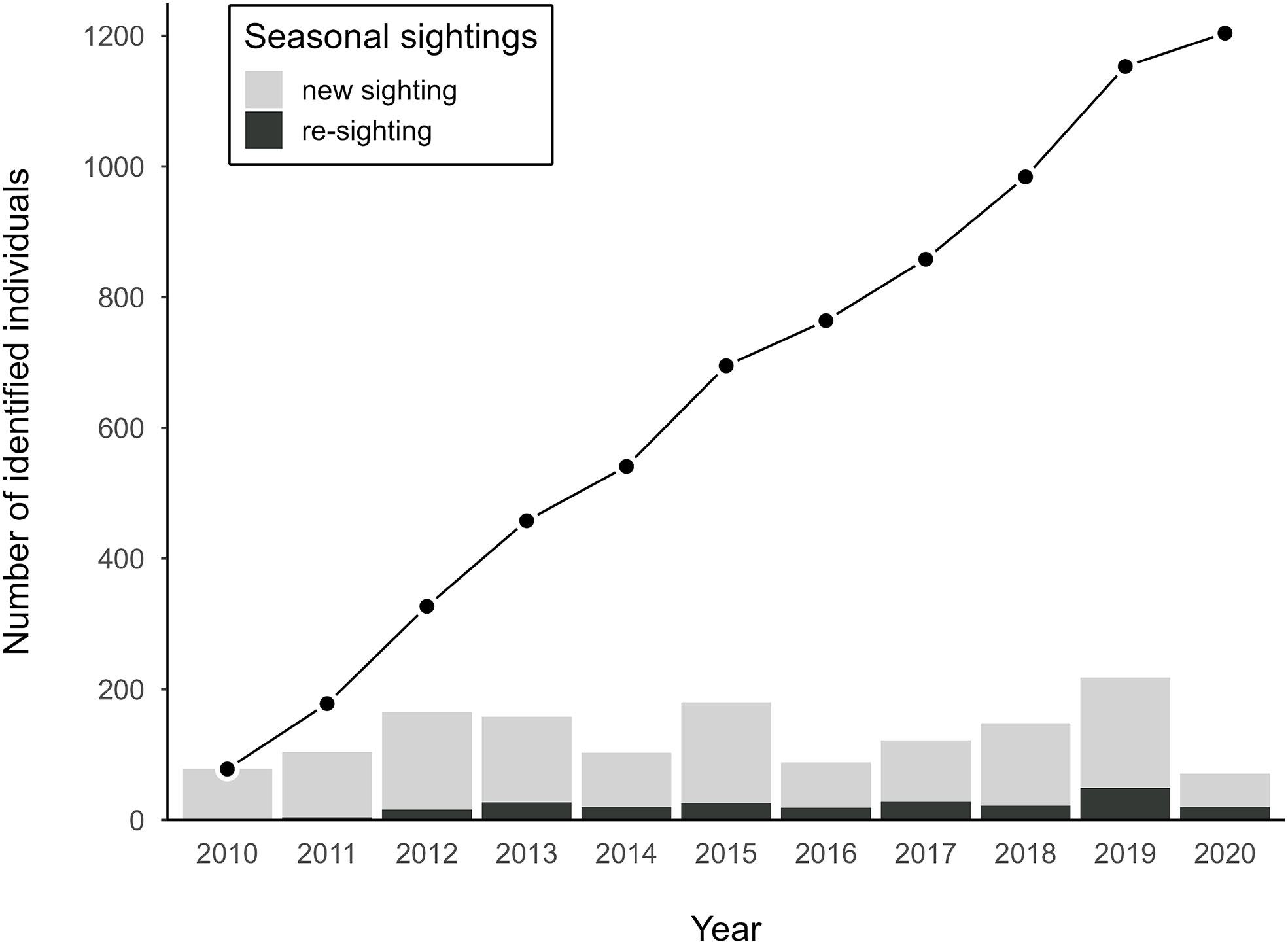
Figure 3 Discovery curve of the cumulative number of photo-identified individuals from 2010 to 2020 (points), with bar plots showing the number of new and previously identified (re-sighted) individuals seen each year.
Candidate CJS models were compared with AICc values (Table 2). The top two models represented 95% of the weight of evidence, had similar AICc values (ΔAICc = 0.6 for second model) and both contained detection p linked to standardized effort and transient Φ. The only difference in model structure was the time dependence of Φ (apparent survival): in the best-fitting model, Φ0 was time-varying and Φ1+ was constant (the apparent survival of non-transient animals did not vary with time), whereas both were time-varying in an additive way in the second best-fitting model. In this way, the best-fitting model, with constant survival following re-sighting, can be regarded as a simpler version of the second best-fitting model. In both these models, the CJS model parameters and associated estimates of abundance are all very similar (Table S1, Figure S1), including substantially overlapping 95% CIs. Thus, in this case we did not compute weighted averages (which would be similar).
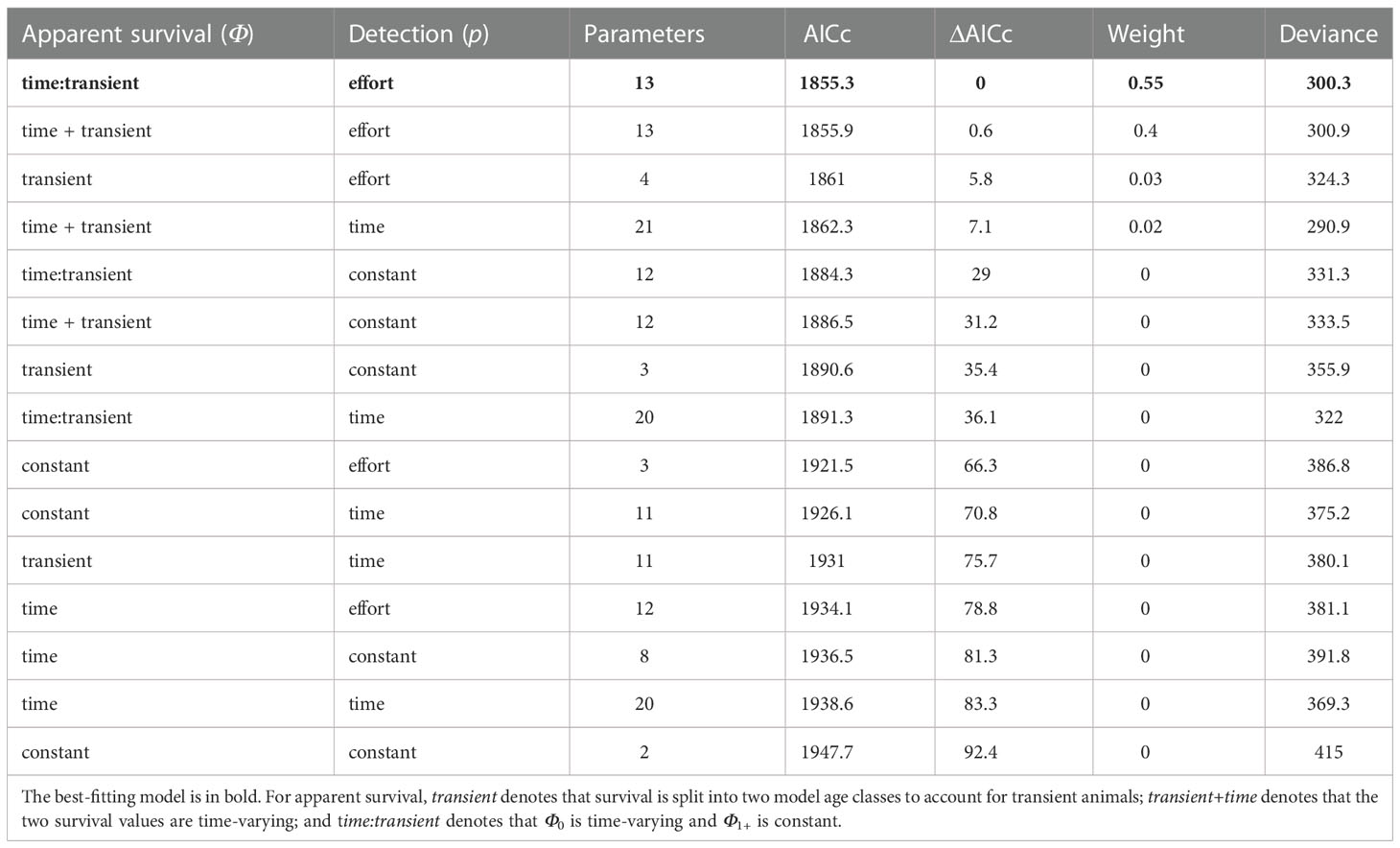
Table 2 Parameter specification and summary of each candidate CJS model, including AICc score used for model selection.
From the best-fitting CJS model (constant Φ1+; Figure 4), detection probabilities, p, ranged from 0.05 (95% CI 0.03-0.08) in 2020 to 0.20 (95% CI 0.15-0.28) in 2019. The survival of non-transients, Φ1+,was 0.97 (95% CI 0.91-0.98) and the proportion of transients, T, ranged from 0.2 (95% CI 0.04-0.64) in 2019 to 0.8 (95% CI 0.69-0.86) in 2018. The number of transient (NT) and non-transient (NR) animals showed differing trends, particularly towards the end of the period, with non-transients increasing and transients decreasing considerably from 2018 to 2019 (Figure 4). Total abundance, Ntot, ranged from 786 (95% CI 593-964) in 2016 to 1,434 (95% CI 924-1,908) in 2020, with a non-significant increasing linear trend across the period determined by weighted linear regression (R2 = 0.28, F = 4.43, p = 0.07). Full model results are available at Grove et al. (2022). Abundance estimates from CJS models with alternative specifications of p (time-varying or linked to unmodified effort) showed similar trends and overlapping confidence intervals (Figure S2).
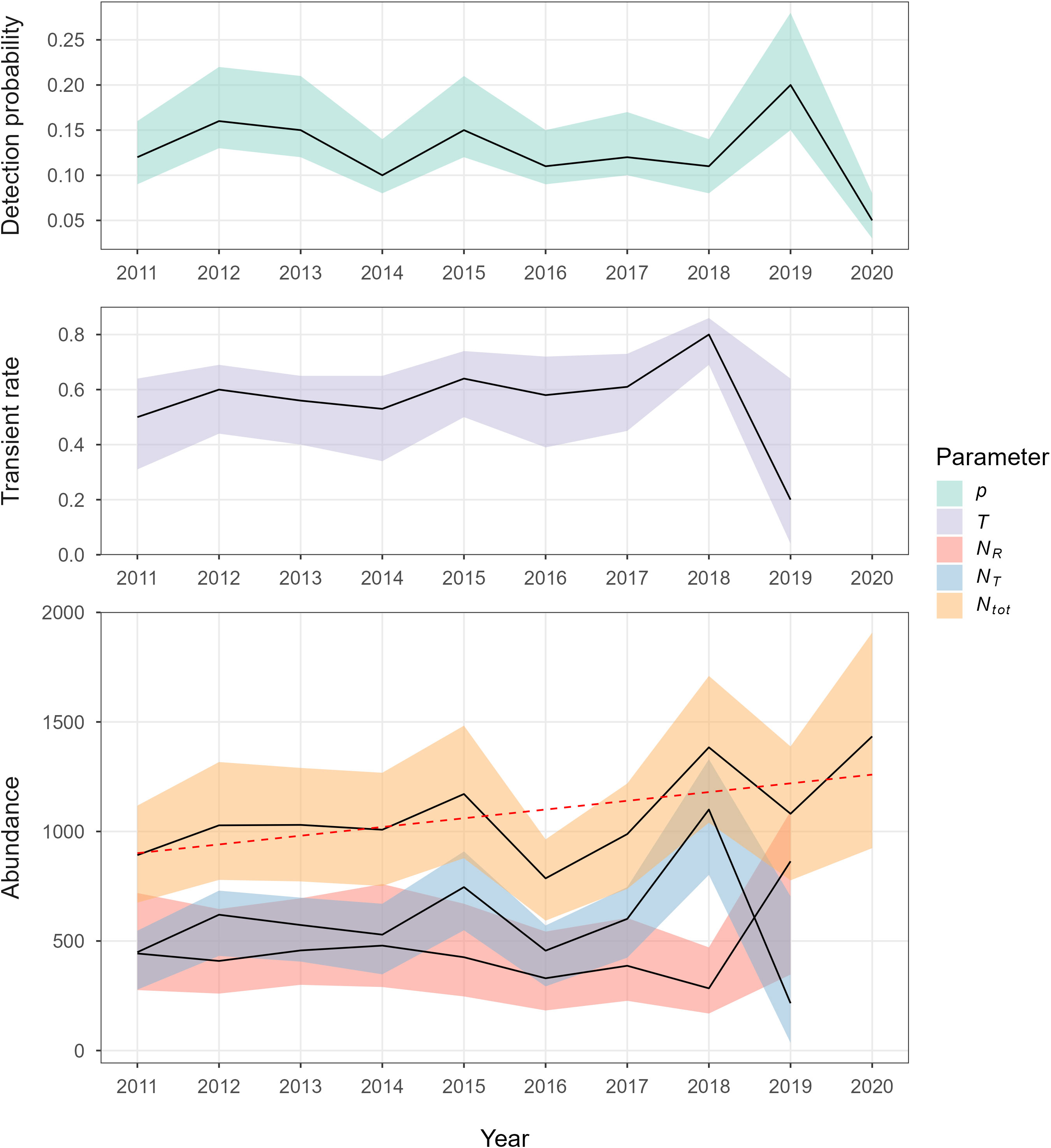
Figure 4 Annual estimates for detection probability (p), transient rate (T), abundance of non-transients (NR), abundance of transients (NT), and total abundance (Ntot), with 95% confidence intervals (CI, shaded regions), for the best-fitting model (effort-dependent p, transient Φ, time-dependent Φ0, constant Φ1+). The weighted linear trend (non-significant) for total abundance is denoted by the dashed red line.
4 Discussion
Abundance estimates for mysticetes are usually derived for feeding and breeding grounds, the endpoints of migration. Here, we provide a ten-year abundance time series at an oceanic, migratory stopover site. According to our best-fitting CR model, up to 1,434 North Atlantic humpback whales (95% CI 924-1,908) visited Bermuda annually between 2011 and 2020, with fluctuations between consecutive years and a slight increase overall. These abundances are comparable with some destination feeding grounds and confirm the contemporary importance of this Marine Mammal Sanctuary as a stopover site. Given the limited protection afforded to humpback whales in Bermudian waters, these estimates should encourage the implementation of area-based management tools to mitigate risks from increasing human activities such as shipping, commercial fishing, and marine wildlife tourism in the waters around Bermuda. Moreover, with Bermuda’s migratory connections to both feeding and breeding grounds across the entire North Atlantic, this time series may facilitate population monitoring at a basin scale.
The capture-recapture data set used here is relatively information-poor, with only 13% of whales re-sighted between seasons across the decade. This is unsurprising, given the challenges of monitoring migratory humpback whales around the Bermuda stopover site: inclement weather frequently prevented survey effort, with the number of survey days per December-May season ranging from 13 in 2020 to 38 in 2019 (out of 182 days); Challenger Bank, a major geographical focus of this study, is 15-20 km offshore; and it was often not possible to survey the entire area of interest within a single day. Furthermore, satellite tagging (Kennedy et al., 2014) and historical sightings from whaling ships (Reeves et al., 2004) suggest that North Atlantic humpback whales follow diffuse, poorly defined migration routes from the Caribbean to northern feeding grounds, over several months. The general migration route passing Bermuda may, therefore, be a very wide corridor (hundreds to thousands of kilometers), and the full extent of the stopover site may be greater than the surveyed area, further limiting detectability.
The resulting sparseness of individual sighting histories limits the complexity (number of parameters) of suitable CR models (Lebreton et al., 1992). We derived abundance estimates with a modified CJS model of detection and apparent survival, and a modified Horvitz-Thompson estimator. Using a combination of system knowledge, goodness-of-fit testing, and information criterion, we identified time dependence on detection probabilities, modeled as a function of modified survey effort; as well a considerable time-varying proportion of transients in the population (Figure 4). Strictly, in this study, a transient animal is only present in the study area (waters around Bermuda) for one occasion (season). We acknowledge that sightings data were used twice for model fitting, in terms of capture-recapture data and applying an availability weighting to the catch-effort covariate. However, the latter may be considered a ‘long-term average’ of monthly sighting rates over the duration of the study period, that is then applied to all years individually. Sensitivity analyses demonstrated that, in practice, the impact of this effort modification on abundance estimates was negligible (Figure S2). Furthermore, a comparable (simple) JSSA model yielded parameter estimates that are broadly similar to those derived from the best-fitting CJS model (Table S2, Figure S3), lending further confidence to our abundance estimates, but the use of JSSA models for these data was strictly limited as a result of associated identifiability issues due to the additional model complexity and parameters to be estimated. Future research could explore the suitability of other CR modeling approaches, such as a recently developed integrated stopover model (Worthington et al., 2019), for this data set.
From the best-fitting CJS model, apparent survival was high (Φ1+ = 0.97, 95% CI 0.92-0.98), yet comparable with estimates obtained from other studies, both within and outside the North Atlantic, with such values ranging from 0.9 to 0.99 (Barlow and Clapham, 1997; Mizroch et al., 2004; Ramp et al., 2010; Félix et al., 2011; Hendrix et al., 2012). The modeled proportion of transients, T, was also high between 2011 and 2018 (Figure 4); this was unsurprising, given the high percentage of animals sighted in only one season (87%). However, T declined steeply to 0.2 (95% CI 0.04-0.64) in 2019, which matches a higher inter-seasonal re-sighting rate (Table 1, Figure 3). A transience signal, as defined in this study, has previously been detected at humpback whale breeding grounds (Chero et al., 2020), but, to our knowledge, not feeding grounds or migratory stopovers (Bertulli et al., 2018). Madon et al. (2013) quantified transience using a similar method at a South Pacific breeding ground (New Caledonia), using both photographic and genetic CR. Whilst the values of T were lower from photographic CR (0.19-0.3), values from genetic CR were similar to our results (0.39-0.66; Madon et al., 2013).
The cause of this ‘transience’ signal and its temporal variability have not been determined for humpback whales (including this study), may not meet the definition of biological transients, and are likely to differ between study systems. The CJS framework is relatively simple and specifies two parameters, p and Φ; therefore, it is unable to distinguish transience from other processes such as temporary emigration. In addition, transience may be confounded with low re-capture probabilities (Genovart and Pradel, 2019), but this is considered unlikely in our model due to the estimation of both low values for p (0.05-0.2) and high transient rates. Alternatively, the ‘transience’ signal may be driven by several factors. First, animals may not use the same migration route every year. For example, animals may switch breeding or feeding ground and shift migration route in the process (Katona, 1986; Kennedy et al., 2014). However, this is unlikely to be prevalent because exchange rates are very low between breeding grounds (Mattila et al., 1989; Stevick et al., 1999; Stevick et al., 2016) and feeding grounds (Palsbøll et al., 1995; Stevick et al., 2006), particularly between the western and eastern Atlantic. Alternatively, animals may use the same general migration route but be transient in their localized use of Bermuda. Whilst multidecadal route fidelity has been demonstrated for Southwest Atlantic humpback whales (Horton et al., 2020), migratory corridors in the North Atlantic appear to be wide and diffuse between the Caribbean and northern feeding grounds (Reeves et al., 2004; Kennedy et al., 2014). Therefore, animals may follow this corridor annually but be transient in their use of Bermuda as a stopover.
Second, animals may faithfully use the migration route, but not annually. For example, females may take ‘rest years’ after giving birth, during which they remain in high latitudes and do not visit breeding grounds (Craig et al., 2003). This is supported by a large discrepancy between sex ratios in humpback whale breeding grounds (1.5-2.5 males for every female) and feeding grounds (near to 1:1) around the world (Brown et al., 1995; Craig and Herman, 1997; Chero et al., 2020), including the North Atlantic (Palsbøll et al., 1997; Smith et al., 1999). Furthermore, persistent humpback whale occurrence in North Atlantic feeding grounds in the winter (Kowarski et al., 2018; Martin et al., 2021) suggests that possibly not all animals migrate south every year. This non-annual visitation would constitute temporary ‘emigration’ from the migration route and not true transience. Temporary emigration can be investigated and characterized using a robust design framework (Pollock, 1982), which structures capture occasions into primary and secondary periods (e.g., Boys et al., 2019). However, this model is more complex, in terms of additional parameters compared to CJS and JSSA, and was unsuitable for our data set, given the low intra- and inter-seasonal re-sighting rates.
To our knowledge, the results presented here provide the most recent abundance time series (2011-2020) for humpback whales within the North Atlantic (Robbins and Pace (2018) provided estimates for the Gulf of Maine up to 2016). From the best-fitting model, annual abundance showed a weak, non-significant, positive linear trend across the decade (Figure 4). Fluctuations in total abundance were sometimes large between years, with a 34% decrease from 2015 to 2016, and a 40% increase from 2018 to 2019 (Figure 4, Table S1). However, our abundance estimates are imprecise, with largely overlapping confidence intervals (Figure 4), so true inter-annual changes may be far smaller. Nevertheless, these fluctuations may have obscured an underlying temporal trend in abundance (Legendre & Legendre, 2012), and are far greater than the maximum plausible rate of increase in population size for humpback whales (11.8% annually; Zerbini et al., 2010). As such, abundance trends at Bermuda alone should not be used as a proxy for variation in the size of the entire North Atlantic population.
Beyond population size, fluctuations may additionally be explained by (1) annual changes in the number of animals undertaking migration (Gabriele et al., 2017; Cartwright et al., 2019); or (2) annual changes in the migration route itself (Zerbini et al., 2016). The potential drivers of migratory changes are unknown for this stopover site but could include variability in oceanographic conditions and, therefore, habitat suitability, either at distant feeding grounds or Bermuda itself. Prey availability at feeding grounds may influence the likelihood of migration to breeding grounds (Frankel et al., 2022), and there is evidence of both foraging and breeding behavior at the Bermuda stopover (Payne and McVay, 1971; Hamilton et al., 1997; Stevenson, 2011; Homfeldt et al., 2022). Studies in Hawai’i, the principal North Pacific breeding ground, found interannual changes of similar magnitude in humpback whale abundance (Frankel et al., 2022), reproductive rates (Cartwright et al., 2019), and male singing activity (Kügler et al., 2020) in response to the North Pacific Marine Heatwave of 2014-2016. Meanwhile, in North Atlantic feeding grounds, a sharp decline in baleen whale abundance in West Greenland was attributed to species range shifts driven by climate-induced changes in pelagic productivity (Heide-Jørgensen and Laidre, 2015; Hansen et al., 2018); and phenological shifts related to increasing water temperature in the Gulf of Maine (Pendleton et al., 2022). In the Azores, a foraging stopover site in the eastern Atlantic, the seasonal timing of baleen whale sightings (including humpback whales) during northbound migration was related to the timing of the regional spring phytoplankton bloom (Visser et al., 2011). Future work should relate local and regional variation in dynamic oceanography to a Bermuda abundance time series, preferably extended to improve statistical power.
Despite these potential fluctuations, our abundance time series suggests that up to 1,434 whales (95% CI 924-1,908) visited the study area each year, with intra-seasonal sightings demonstrating stopover residency (Table 1). Together, these results confirm the use and importance of Bermuda as a migratory stopover for humpback whales. Due to the lack of recent abundance estimates for North Atlantic feeding and breeding grounds (Kennedy and Clapham, 2017), it is challenging to place these results in the context of the wider North Atlantic and comparisons with outdated abundance estimates should be made cautiously. Total abundance in the primary Caribbean breeding ground was estimated to be 10,752 in 1992-93 (CV = 0.068, Stevick et al., 2003), and it is plausible that the growth rate of 3% has continued (Punt et al., 2006). Abundances for Bermuda are smaller than estimates from some feeding areas, including Iceland (>10,000 during 1995-2007; Víkingsson et al., 2015), and Norway (4,695 during 1996-2001; Øien, 2009), but are comparable with other feeding grounds such as the Gulf of Maine (1,317 in 2016; Robbins and Pace, 2018) and eastern Canada (1,903 in 1982; Katona and Beard, 1990). Therefore, whilst Bermuda is apparently visited by a small proportion of the total North Atlantic population, the island may serve as an important migratory stopover site for individual feeding grounds, particularly in western areas (Beaudette et al., 2009). These feeding grounds are treated as distinct management units at a basin scale (Hayes et al., 2019). Further analysis of the migratory connectivity between Bermuda and other parts of North Atlantic will improve our understanding of Bermuda’s role within the wider population.
These results are timely, given the recent announcement of a draft Blue Economy Strategy for Bermuda (Bermuda Ocean Prosperity Programme, 2021) and the lack of specific protection afforded to humpback whales in these waters. The declaration of Bermuda’s exclusive economic zone as a Marine Mammal Sanctuary in 2012 formed part of a transboundary network of ‘protected areas’ (Wenzel et al., 2019) but provided no management measures (NOAA and Government of Bermuda, 2012); the inclusion of humpback whales in Bermuda’s Protected Species Act of 2003 broadly prohibits disturbance, harassment and injury (Government of Bermuda, 2003; Government of Bermuda, 2016); and existing whale-watching guidelines are voluntary (Department of Environment and Natural Resources, 2017). Moreover, around Bermuda and the wider Sargasso Sea, anthropogenic activity, particularly large vessel traffic, has increased in recent years and this growth is forecast to continue (Roberts, 2011; Halpern et al., 2015; Širović et al., 2016). As a result, ocean ambient sound is now dominated by shipping in Bermudian waters (Širović et al., 2016). Combined with possible increases in commercial fishing activity and whale-watching tourism (Bermuda Tourism Authority, 2019), any resulting mortality or disturbance may impact whales across the North Atlantic, particularly western feeding grounds and northern Caribbean breeding grounds. Our time series should encourage consideration of area-based management tools to mitigate risks from human activities around Bermuda, such as PSSAs and marine protected areas, as well as a review of existing whale-watching guidelines (Department of Environment and Natural Resources, 2017). Abundance estimates can be combined with current acoustic monitoring (Homfeldt et al., 2022) and behavioral observation to provide evidence of spatiotemporal patterns in habitat use to guide specific policies (Minton et al., 2011; Rossi-Santos, 2015; Stepanuk et al., 2021). Moreover, such evidence may determine whether Bermuda constitutes critical habitat, defined as areas that are regularly used by a population to perform essential tasks for survival and reproduction (Hoyt, 2011), and may require special consideration for species conservation (Endangered Species Act, 1973).
Beyond Bermuda, the large sightings database (1,204 whales) and ten-year abundance time series may facilitate wider population monitoring on a basin scale (Moore, 2008; Hazen et al., 2019), in conjunction with comparable time series in breeding and feeding grounds (e.g., Robbins and Pace, 2018). Due to Bermuda’s unique migratory connections (Katona, 1986) and its role as a stopover site (Payne and McVay, 1971; Stevenson, 2011), changes in abundance around Bermuda may reflect shifts in migratory patterns and population dynamics in distant feeding or breeding grounds across the North Atlantic, and provide extra information on population-level processes. Therefore, we encourage the construction of contemporary, separate abundance time series in other parts of the North Atlantic (especially feeding and breeding grounds), for example, by using existing photo-identification databases, e.g., Happywhale (https://happywhale.com) and the North Atlantic Humpback Whale Catalogue (Katona and Beard, 1990). This aligns with the IWC’s recommendation for a range-wide assessment of abundance to replace outdated estimates (International Whaling Commission, 2019). Furthermore, humpback whales are a cosmopolitan marine predator and sensitive to ecosystem change (Cartwright et al., 2019). Better understanding basin-scale population processes, including migratory patterns over space and time, will facilitate assessing the response to environmental change, which may be used as a sentinel for ecosystem monitoring (Simmons et al., 2017; Miloslavich et al., 2018).
Using a capture-recapture framework, guided by goodness-of-fit-testing, we provide an abundance time series for a baleen whale migratory stopover site. Accounting for heterogeneity in survival and detection, we generate robust abundance estimates across a decade and demonstrate the importance of Bermuda for migrating North Atlantic humpback whales. Future marine spatial planning around Bermuda should consider the potential impact that growing anthropogenic pressures, such as vessel traffic and marine wildlife tourism, may have on thousands of humpback whales within potential critical habitat. Furthermore, with Bermuda’s migratory connections to feeding and breeding grounds, this time series may facilitate monitoring of population-level processes across the North Atlantic. More generally, we encourage the investigation of whale abundance across a species’ range as a potential sentinel for basin-scale ecosystem change. As marine environmental change accelerates across the North Atlantic, sustained photo-identification survey effort in Bermudian waters should be supported to extend this time series.
Data availability statement
The datasets presented in this study can be found in online repositories. The names of the repository/repositories and accession number(s) can be found below: The model outputs generated from this study are available at: https://doi.pangaea.de/10.1594/PANGAEA.945442.
Ethics statement
Ethical review and approval was not required for the animal study because Humpback whales were photographed from a vessel, which did not require any contact or close approach. Nevertheless, WhalesBermuda (Andrew Stevenson) obtained an annual research from the Department of Environmental and Natural Resources, Government of Bermuda, for each year of the study.
Author contributions
L-AH conceptualized the study and acquired funding for analysis and submission. AS conducted all fieldwork and provided resources for data collection. TG and RK designed the study methodology. AS and TG conducted image quality filtering and photo-identification. TG subsequently processed the data, performed analyses, created graphics and led manuscript writing. L-AH and RK supervised the project. RK, AS, and L-AH provided regular feedback; and contributed to and reviewed the manuscript. All authors contributed to the article and approved the submitted version.
Funding
This study received funding from the European Union’s Horizon 2020 research and innovation program under grant agreement no. 818123 (iAtlantic). This output reflects only the authors’ views and the European Union cannot be held responsible for any use that may be made of the information contained therein. RK was supported by the Leverhulme research fellowship RF-2019-299.
Acknowledgments
On behalf of WhalesBermuda, AS would like to acknowledge support of the Atlantic Conservation Partnership. The authors are grateful to Olivier Gimenez for providing open-access R code to construct CJS models in RMark and derive abundances. Thank you to Alyssa Stoller for contributing to image scoring for photo-identification, and to Jeff Laake for assistance with JSSA model formulation. All data were collected under an annual Protected Species License issued by the Department of Conservation Services, Government of Bermuda.
Conflict of interest
The authors declare that the research was conducted in the absence of any commercial or financial relationships that could be construed as a potential conflict of interest.
Publisher’s note
All claims expressed in this article are solely those of the authors and do not necessarily represent those of their affiliated organizations, or those of the publisher, the editors and the reviewers. Any product that may be evaluated in this article, or claim that may be made by its manufacturer, is not guaranteed or endorsed by the publisher.
Supplementary material
The Supplementary Material for this article can be found online at: https://www.frontiersin.org/articles/10.3389/fmars.2022.971801/full#supplementary-material
Footnotes
- ^ Happywhale, https://www.happewhale.com [last accessed 12 September, 2022]
References
Øien N. (2009). Distribution and abundance of large whales in Norwegian and adjacent waters based on ship surveys 1995-2001. NAMMCO Sci. Publ. 7, 31–47. doi: 10.7557/3.2704
Altmann J. (1974). Observational study of behavior: sampling methods. Behaviour 49, 227–266. doi: 10.1163/156853974X00534
Barendse J., Best P. B., Thornton M., Elwen S. H., Rosenbaum H. C., Carvalho I., et al. (2011). Transit station or destination? attendance patterns, movements and abundance estimate of humpback whales off west south Africa from photographic and genotypic matching. Afr. J. Mar. Sci. 33, 353–373. doi: 10.2989/1814232X.2011.637343
Barlow J., Calambokidis J., Falcone E. A., Baker C. S., Burdin A. M., Clapham P. J., et al. (2011). Humpback whale abundance in the north pacific estimated by photographic capture-recapture with bias correction from simulation studies. Mar. Mammal Sci. 27, 793–818. doi: 10.1111/j.1748-7692.2010.00444.x
Barlow J., Clapham P. J. (1997). A new birth-interval approach to estimating demographic parameters of humpback whales. Ecology 78, 535–546. doi: 10.1890/0012-9658(1997)078[0535:ANBIAT]2.0.CO;2
Beaudette A., Allen J., Bort J., Stevenson A., Stevick P., Stone G. (2009). “Movement patterns of North Atlantic humpback whales identified at Bermuda,” in Quebec City: Presentation at the Biennial Conference of the Society for Marine Mammology Biennial Meeting.
Becker E. A., Forney K. A., Redfern J. V., Barlow J., Jacox M. G., Roberts J. J., et al. (2019). Predicting cetacean abundance and distribution in a changing climate. Divers. Distrib. 25, 626–643. doi: 10.1111/ddi.12867
Bermuda Ocean Prosperity Programme (2021) Blue economy. Available at: https://www.bermudaoceanprosperity.org/blue-economy (Accessed May 10, 2021).
Bermuda Tourism Authority (2019). Bermuda National tourism plan 2019-2025 (Hamilton, Bermuda). Available at: https://www.gotobermuda.com/bta/document/national-tourism-plan
Bertulli C. G., Guéry L., McGinty N., Suzuki A., Brannan N., Marques T., et al. (2018). Capture-recapture abundance and survival estimates of three cetacean species in icelandic coastal waters using trained scientist-volunteers. J. Sea Res. 131, 22–31. doi: 10.1016/J.SEARES.2017.10.001
Best P. B. (1993). Increase rates in severely depleted stocks of baleen whales. ICES J. Mar. Sci. 50, 169–186. doi: 10.1006/JMSC.1993.1018
Bettridge S., Baker C. S., Barlow J., Clapham P. J., Ford M., Gouveia D., et al. (2015). Status review of the humpback whale (Megaptera novaeangliae) under the Endangered Species Act. NOAA Technical Memorandum. NOAA-TM-NMFSSWFSC-540. U.S. Department of Commerce
Borchers D. L., Buckland S. T., Zucchini W. (2002). Estimating animal abundance: Closed populations (New York, NY: Springer).
Boys R. M., Oliveira C., Pérez-Jorge S., Prieto R., Steiner L., et al. (2019). Multi-state open robust design applied to opportunistic data reveals dynamics of wide-ranging taxa: the sperm whale case. Ecosphere 10, e02610. doi: 10.1002/ecs2.2610
Brown M. R., Corkeron P. J., Hale P. T., Schultz K. W., Bryden M. M. (1995). Evidence for a sex-segregated migration in the humpback whale (Megaptera novaeangliae). Proc. R. Soc B Biol. Sci. 259, 229–234. doi: 10.1098/rspb.1995.0034
Burnham K. P., Anderson D. R. (2004). Multimodel inference: understanding AIC and BIC in model selection. Sociol. Methods Res. 33, 261–304. doi: 10.1177/0049124104268644
Calambokidis J., Steiger G. H., Straley J. M., Herman L. M., Cerchio S., Salden D. R., et al. (2001). Movements and population structure of humpback whales in the north pacific. Mar. Mammal Sci. 17, 769–794. doi: 10.1111/j.1748-7692.2001.tb01298.x
Carlson C. A., Mayo C. A., Whitehead H. (1990). Changes in the ventral fluke pattern of the humpback whale (Megaptera novaeangliae), and its effects on matching; evaluation of its significance to photo-identification research. Rep. Int. Whal. Commun. Special Isue 12, 105–111.
Cartwright R., Venema A., Hernandez V., Wyels C., Cesere J., Cesere D. (2019). Fluctuating reproductive rates in hawaii’s humpback whales, Megaptera novaeangliae, reflect recent climate anomalies in the north pacific. R. Soc Open Sci. 6, 181463. doi: 10.1098/rsos.181463
Carwardine M. (2019). Handbook of whales, dolphins and porpoises (London, UK: Bloomsbury Publishing).
Chaloupka M., Osmond M., Kaufman G. (1999). Estimating seasonal abundance trends and survival probabilities of humpback whales in Hervey Bay (east coast Australia). Mar. Ecol. Prog. Ser. 184, 291–301. doi: 10.3354/meps184291
Chero G., Pradel R., Derville S., Bonneville C., Gimenez O., Garrigue C. (2020). Reproductive capacity of an endangered and recovering population of humpback whales in the Southern Hemisphere. Mar. Ecol. Prog. Ser. 643, 219–227. doi: 10.3354/meps13329
Choquet R., Lebreton J.-D., Gimenez O., Reboulet A.-M., Pradel R. (2009). U-CARE: Utilities for performing goodness of fit tests and manipulating CApture-REcapture data. Ecography. 32, 1071–1074. doi: 10.1111/j.1600-0587.2009.05968.x
Constantine R., Jackson J., Steel D., Baker C., Brooks L., Burns D., et al. (2012). Abundance of humpback whales in Oceania using photo-identification and microsatellite genotyping. Mar. Ecol. Prog. Ser. 453, 249–261. doi: 10.3354/meps09613
Cooke J. G. (2018). Megaptera novaeangliae. IUCN Red List Threat. Species 2018, e.T13006A50362794. doi: 10.2305/IUCN.UK.2018-2.RLTS.T13006A50362794.en
Craig A. S., Herman L. M. (1997). Sex differences in site fidelity and migration of humpback whales (Megaptera novaeangliae) to the Hawaiian islands. Can. J. Zool. 75, 1923–1933. doi: 10.1139/z97-822
Craig A. S., Herman L. M., Gabriele C. M., Pack A. A. (2003). Migratory timing of humpback whales (Megaptera novaeangliae) in the Central North Pacific varies with age, sex and reproductive status. Behaviour 140, 981–1001. doi: 10.1163/156853903322589605
Cubaynes S., Pradel R., Choquet R., Duchamp C., Gaillard J.-M., Lebreton J.-D., et al. (2010). Importance of accounting for detection heterogeneity when estimating abundance: the case of French wolves. Conserv. Biol. 24, 621–626. doi: 10.1111/j.1523-1739.2009.01431.x
Cucuzza M., Hartman K., Olio M., Santos R. P., Steiner L., Stevick P. T. (2015). “The Azores constitute a migratory stopover for humpback whales in the North Atlantic Ocean,” in Malta: Poster presented at 29th conference of the European Cetacean Society.
Department of Environment and Natural Resources (2017) Guidelines for whale watching in Bermuda. Available at: https://environment.bm/whale-watching-guidelines (Accessed May 20, 2022).
Endangered Species Act (1973). Endangered species act of 1973 (Washington DC: Department of the Interior, U.S. Fish and Wildlife Service).
Félix F., Castro C., Laake J. L., Haase B., Scheidat M. (2011). Abundance and survival estimates of the southeastern Pacific humpback whale stock from 1991-2006 photo-identification surveys in Ecuador. J. Cetacean Res. Manage. (Special Issue) 3, 301–307.
Findlay K. P., Best P. B., Meÿer M. A. (2011). Migrations of humpback whales past Cape Vidal, South Africa, and an estimate of the population increase rate, (1988–2002). Afr. J. Mar. Sci. 33, 375–392. doi: 10.2989/1814232X.2011.637345
Forster M. R. (2000). Key concepts in model selection: performance and generalizability. J. Math. Psychol. 44, 205–231. doi: 10.1006/JMPS.1999.1284
Frankel A. S., Gabriele C. M., Yin S., Rickards S. H. (2022). Humpback whale abundance in Hawai’i: Temporal trends and response to climatic drivers. Mar. Mammal Sci. 38, 118–138. doi: 10.1111/MMS.12856
Franklin T., Franklin W., Brooks L., Harrison P., Burns D., Holmberg J., et al. (2020). Photo-identification of individual humpback whales (Megaptera novaeangliae) using all available natural marks: Implications for misidentification and automated algorithm matching technology. J. Cetacean Res. Manage. 21, 71–83. doi: 10.47536/jcrm.v21i1.186
Franklin T., Franklin W., Brooks L., Harrison P., Pack A. A., Clapham P. J (2021). Social behaviour of humpback whales (Megaptera novaeangliae) in hervey bay, Eastern Australia, a preferential female stopover during the southern migration. Front. Mar. Sci. 8. doi: 10.3389/FMARS.2021.652147
Gabriele C. M., Neilson J. L., Straley J. M., Scott Baker C., Cedarleaf J. A., Saracco J. F., et al. (2017). Natural history, population dynamics, and habitat use of humpback whales over 30 years on an Alaska feeding ground. Ecosphere 8, e01641. doi: 10.1002/ECS2.1641
Genovart M., Pradel R. (2019). Transience effect in capture-recapture studies: The importance of its biological meaning. PloS One 14, e0222241. doi: 10.1371/journal.pone.0222241
Gimenez O., Choquet R. (2010). Individual heterogeneity in studies on marked animals using numerical integration: capture–recapture mixed models. Ecology 91, 951–957. doi: 10.1890/09-1903.1
Gimenez O., Lebreton J., Choquet R., Pradel R. (2018). R2ucare: An r package to perform goodness-of-fit tests for capture–recapture models. Methods Ecol. Evol. 9, 1749–1754. doi: 10.1111/2041-210X.13014
Government of Bermuda (2003) Bermuda Protected species act 2003. Available at: http://www.bermudalaws.bm/laws/ConsolidatedLaws/ProtectedSpeciesAct2003.pdf (Accessed May 20, 2022).
Government of Bermuda (2016) Bermuda Protected species amendment order 782 2016. BR4/2016. Available at: http://www.bermudalaws.bm/laws/Annual%0A783Laws/2016/StatutoryInstruments/ProtectedSpeciesAmendmentOrder2016.pdf (Accessed May 20, 2022).
Grove T., King R., Henry L.-A., Stevenson A. (2022). Modelled annual abundance of humpback whales Megaptera novaeangliae around Bermuda 2011-2020. PANGAEA. doi: 10.1594/PANGAEA.945442
Halpern B. S., Frazier M., Potapenko J., Casey K. S., Koenig K., Longo C., et al. (2015). Spatial and temporal changes in cumulative human impacts on the world’s ocean. Nat. Commun. 6, 7615. doi: 10.1038/ncomms8615
Hamilton P. K., Stone G. S., Martin S. M. (1997). Note on a deep humpback whale Megaptera novaeangliae dive near Bermuda. Bull. Mar. Sci. 61, 491–494.
Hammond P. S. (1990). Heterogeneity in the Gulf of Maine? estimating humpback whale population size when capture probabilities are not equal. Rep. Int. Whal. Commun. 12, 135–139.
Hammond P. S., Francis T. B., Heinemann D., Long K. J., Moore J. E., Punt A. E., et al. (2021). Estimating the abundance of marine mammal populations. Front. Mar. Sci. 8. doi: 10.3389/FMARS.2021.735770
Hansen R. G., Boye T. K., Larsen R. S., Nielsen N. H., Tervo O., Nielsen R. D., et al. (2018). Abundance of whales in West and East Greenland in summer 2015. NAMMCO Sci. Publ. 11. doi: 10.7557/3.4689
Hayes S. A., Josephson E., Maze-Foley K., Rosel P. E. (2019). US Atlantic and Gulf of Mexico marine mammal stock assessments. NOAA Technical Memorandum. Woods Hole, MA: U.S. Department of Commerce. doi: 10.25923/ngsq-qc69
Hazen E. L., Abrahms B., Brodie S., Carroll G., Jacox M. G., Savoca M. S., et al. (2019). Marine top predators as climate and ecosystem sentinels. Front. Ecol. Environ. 17, 565–574. doi: 10.1002/fee.2125
Heide-Jørgensen M. P., Laidre K. L. (2015). Surfacing time, availability bias and abundance of humpback whales in West Greenland. J. Cetacean Res. Manage. 15, 1–8.
Hendrix A. N., Straley J., Gabriele C. M., Gende S. M. (2012). Bayesian Estimation of humpback whale (Megaptera novaeangliae) population abundance and movement patterns in southeastern Alaska. Can. J. Fish. Aquat. Sci. 69, 1783–1797. doi: 10.1139/F2012-101
Homfeldt T. N., Risch D., Stevenson A., Henry L.-A. (2022). Seasonal and diel patterns in singing activity of humpback whales migrating through Bermuda. Front. Mar. Sci. 9. doi: 10.3389/fmars.2022.941793
Horton T. W., Zerbini A. N., Andriolo A., Danilewicz D., Sucunza F. (2020). Multi-decadal humpback whale migratory route fidelity despite oceanographic and geomagnetic change. Front. Mar. Sci. 7. doi: 10.3389/fmars.2020.00414
Horvitz D. G., Thompson D. J. (1952). A generalization of sampling without replacement from a finite universe. J. Am. Stat. Assoc. 47, 663–685. doi: 10.1080/01621459.1952.10483446
Hoyt E. (2011). Marine protected areas for whales, dolphins and porpoises: A world handbook for cetacean habitat conservation and planning (London and New York: Earthscan/Taylor & Francis).
Hwang W.-H., Huggins R. (2005). An examination of the effect of heterogeneity on the estimation of population size using capture-recapture data. Biometrika 92, 229–233. doi: 10.1093/biomet/92.1.229
International Whaling Commission (2002). Report of the sub committee on the comprehensive assessment of North Atlantic humpback whales. Annex H to the report of the scientific committee. J. Cetacean Res. Manage. 4, 230–260.
International Whaling Commission (2009). Report of the scientific committee. Annex E. Report of the standing working group on the aboriginal whaling management procedures. J. Cetacean Res. Manage. (Suppl.), 11, 145–168.
International Whaling Commission (2019). Report of the scientific committee. J. Cetacean Res. Manage. 20 (Suppl.), 1–78.
Jeyam A., McCrea R. S., Bregnballe T., Frederiksen M., Pradel R. (2018). A test of positive association for detecting heterogeneity in capture for capture–recapture data. J. Agric. Biol. Environ. Stat. 23, 1–19. doi: 10.1007/s13253-017-0315-4
Jolly G. M. (1965). Explicit estimates from capture-recapture data with both death and immigration-stochastic model. Biometrika 52, 225–247. doi: 10.2307/2333826
Kachel M. J. (2008). “Particularly sensitive sea areas,” in Hamburg Studies on Maritime Affairs, vol. 13, 376. Berlin: Springer. doi: 10.1007/978-3-540-78779-2
Katona S. K. (1986). “Biogeography of the humpback whale megaptera novaeangliae, in the North Atlantic,” in Pelagic biogeography, vol. 49 . Eds. Pierrot-Baits A. C., van der Spoel S., Zahuranec B. J., Johnson R. K. (Paris, France: UNESCO Technical Paper in Marine Science), 166–171.
Katona S., Baxter B., Brazier O., Kraus S., Perkins J., Whitehead H. (1979). “Identification of humpback whales by fluke photographs,” in Behavior of marine animals: Current perspectives in research (New York, NY: Plenum Press), 33–44.
Katona S. K., Beard J. A. (1990). Population size, migrations and feeding aggregations of the humpback whale (Megaptera novaeangliae) in the western North Atlantic Ocean. Rep. Int. Whal. Commun. Spec. Issue 12, 295–305.
Katona S. K., Whitehead H. P. (1981). Identifying humpback whales using their natural markings. Polar Rec. 20, 439–444. doi: 10.1017/S003224740000365X
Kennedy A. S., Clapham P. J. (2017). From whaling to tagging: The evolution of North Atlantic humpback whale research in the West indies. Mar. Fish. Rev. 79, 23–37. doi: 10.7755/MFR.79.2.2
Kennedy A. S., Zerbini A. N., Vásquez O. V., Gandilhon N., Clapham P. J., Adam O. (2014). Local and migratory movements of humpback whales (Megaptera novaeangliae) satellite-tracked in the North Atlantic Ocean. Can. J. Zool. 92, 9–18. doi: 10.1139/cjz-2013-0161
King R. (2014). Statistical ecology. Annu. Rev. Stat. Its Appl. 1, 401–426. doi: 10.1146/ANNUREV-STATISTICS-022513-115633
King R., Brooks S. P. (2004). A classical study of catch-effort models for hector’s dolphins. J. Am. Stat. Assoc. 99, 325–333. doi: 10.1198/016214504000000304
King R., McCrea R. S. (2019). “Capture-recapture methods and models: Estimating population size,” in Handbook of statistics volume 40 (Elsevier), 33–83. doi: 10.1016/bs.host.2018.09.006
Kowarski K., Evers C., Moors-Murphy H., Martin B., Denes S. L. (2018). Singing through winter nights: Seasonal and diel occurrence of humpback whale (Megaptera novaeangliae) calls in and around The Gully MPA, offshore eastern Canada. Mar. Mammal Sci. 34, 169–189. doi: 10.1111/mms.12447
Kügler A., Lammers M. O., Zang E. J., Kaplan M. B., Aran Mooney T. (2020). Fluctuations in Hawaii’s humpback whale Megaptera novaeangliae population inferred from male song chorusing off Maui. Endanger. Species Res. 43, 421–434. doi: 10.3354/ESR01080
Kutner M. H., Nachtsheim C. J., Neter J., Li W. (2005). “Building the regression model III: Remedial measures,” in Applied linear statistical models. Ed. Neter J. (New York, NY: McGraw-Hill/Irwin), 421–480.
Laake J., Rexstad E. (2012). “Appendix c. RMark–an alternative approach to building linear models in MARK,” in Program MARK: A gentle introduction. Eds. Cooch E., White G. (Fort Collins, CO: Colorado State University).
Lawton J. H. (1993). Range, population abundance and conservation. Trends Ecol. Evol. 8, 409–413. doi: 10.1016/0169-5347(93)90043-O
Lebreton J.-D., Burnham K. P., Clobert J., Anderson D. R. (1992). Modeling survival and testing biological hypotheses using marked animals: A unified approach with case studies. Ecol. Monogr. 62, 67–118. doi: 10.2307/2937171
Legendre P., Legendre L. (2012). “Ecological data series,” in Developments in Environmental Modelling, 711–783. (Amsterdam: Elsevier) doi: 10.1016/B978-0-444-53868-0.50012-5
Linscott J. A., Senner N. R. (2021). Beyond refueling: Investigating the diversity of functions of migratory stopover events. Ornithol. Appl. 123, 1–14. doi: 10.1093/ORNITHAPP/DUAA074
Mace G. M., Collar N. J., Gaston K. J., Hilton-Taylor C., Akçakaya H. R., Leader-Williams N., et al. (2008). Quantification of extinction risk: IUCN’s system for classifying threatened species. Conserv. Biol. 22, 1424–1442. doi: 10.1111/j.1523-1739.2008.01044.x
Mackintosh N. A. (1946). The natural history of whalebone whales. Biol. Rev. 21, 60–74. doi: 10.1111/j.1469-185X.1946.tb00453.x
Madon B., Garrigue C., Pradel R., Gimenez O. (2013). Transience in the humpback whale population of New Caledonia and implications for abundance estimation. Mar. Mammal Sci. 29, 669–678. doi: 10.1111/j.1748-7692.2012.00610.x
Martin S. C., Aniceto A. S., Ahonen H., Pedersen G., Lindstrøm U. (2021). Humpback whale (Megaptera novaeangliae) song on a subarctic feeding ground. Front. Mar. Sci. 8. doi: 10.3389/FMARS.2021.669748
Marucco F., Pletscher D. H., Boitani L., Schwartz M. K., Pilgrim K. L., Lebreton J. D. (2009). Wolf survival and population trend using non-invasive capture-recapture techniques in the Western Alps. J. Appl. Ecol. 46, 1003–1010. doi: 10.1111/j.1365-2664.2009.01696.x
Mattila D. K., Clapham P. J., Katona S. K., Stone G. S. (1989). Population composition of humpback whales, Megaptera novaeangliae, on Silver Bank 1984. Can. J. Zool. 67, 281–285. doi: 10.1139/z89-041
McCrea R. S., Morgan B. J. T. (2015). Analysis of capture-recapture data (Boca Raton, FL: CRC Research Press).
McDonald T. L., Amstrup S. C. (2001). Estimation of population size using open capture-recapture models. J. Agric. Biol. Environ. Stat. 6, 206–220. doi: 10.1198/108571101750524553
Miloslavich P., Bax N. J., Simmons S. E., Klein E., Appeltans W., Aburto-Oropeza O., et al. (2018). Essential ocean variables for global sustained observations of biodiversity and ecosystem changes. Glob. Change Biol. 24, 2416–2433. doi: 10.1111/gcb.14108
Minton G. T. J. Q., Collins T., Findlay K. P., Ersts P. J., Rosenbaum H. C., Berggren P., et al. (2011). Seasonal distribution, abundance, habitat use and population identity of humpback whales in Oman. J. Cetacean Res. Manage. (Special Issue South. Hemisph. Humpback Whales) 3, 185–198.
Mizroch S. A., Herman L. M., Straley J. M., Glockner-Ferrari D. A., Jurasz C., Darling J., et al. (2004). Estimating the adult survival rate of Central North Pacific humpback whales (Megaptera novaeangliae). J. Mammal. 85, 963–972. doi: 10.1644/bos-123
Monnahan C. C., Acevedo J., Noble Hendrix A., Gende S., Aguayo-Lobo A., Martinez F. (2019). Population trends for humpback whales (Megaptera novaeangliae) foraging in the Francisco coloane coastal-marine protected area, Magellan Strait, Chile. Mar. Mammal Sci. 35, 1212–1231. doi: 10.1111/mms.12582
Moore S. E. (2005). “Long-term environmental change and marine mammals,” in Marine mammal research: conservation beyond crisis. Eds. Reynolds J. E. III, Perrin W. F., Reeves R. R., Montgomery S., Ragen T. J. (Baltimore, MD: John Hopkins University Press), 137–147.
Moore S. E. (2008). Marine mammals as ecosystem sentinels. J. Mammal. 89, 534–540. doi: 10.1644/07-mamm-s-312r1.1
Moore J. E., Barlow J. (2011). Bayesian State-space model of fin whale abundance trends from a 1991-2008 time series of line-transect surveys in the California Current. J. Appl. Ecol. 48, 1195–1205. doi: 10.1111/j.1365-2664.2011.02018.x
NOAA, Government of Bermuda (2012). Memorandum of understanding between the united states of America, US department of commerce national oceanic and atmospheric administration, national ocean service, office of marine sanctuaries - and the government of Bermuda, ministry of the environment.
NOAA (2016). Endangered and Threatened Species; Identification of 14 Distinct Population Segments of the humpback whale (Megaptera novaeangliae) and revision of species-wide listing. Fed. Regist. 81, 62259–62320. Available at: https://www.gpo.gov/fdsys/pkg/FR-2016-09-08/pdf/2016-21276.pdf.
Owen C., Rendell L., Constantine R., Noad M. J., Allen J., Andrews O., et al. (2019). Migratory convergence facilitates cultural transmission of humpback whale song. R. Soc Open Sci. 6, 190337. doi: 10.1098/RSOS.190337
Palsbøll P. J., Allen J., Bérubé M., Clapham P. J., Feddersen T. P., Hammond P. S., et al. (1997). Genetic tagging of humpback whales. Nature 388, 767–769. doi: 10.1038/42005
Palsbøll P. J., Clapham P. J., Mattila D. K., Larsen F., Sears R., Siegesmund H. R., et al. (1995). Distribution of mtDNA haplotypes in North Atlantic humpback whales: the influence of behaviour on population structure. Mar. Ecol. Prog. Ser. 116, 1–10. doi: 10.3354/meps116001
Patrician M. R., Kenney R. D. (2010). Using the continuous plankton recorder to investigate the absence of North Atlantic right whales (Eubalaena glacialis) from the Roseway Basin foraging ground. J. Plankton Res. 32, 1685–1695. doi: 10.1093/plankt/fbq073
Payne R. S., McVay S. (1971). Songs of humpback whales. Science 173, 585–597. doi: 10.1126/science.173.3997.585
Pendleton D. E., Tingley M. W., Ganley L. C., Friedland K. D., Mayo C., Brown M. W., et al. (2022). Decadal-scale phenology and seasonal climate drivers of migratory baleen whales in a rapidly warming marine ecosystem. Glob. Change Biol. 28, 4989–5005. doi: 10.1111/gcb.16225
Perret N., Pradel R., Miaud C., Grolet O., Joly P. (2003). Transience, dispersal and survival rates in newt patchy populations. J. Anim. Ecol. 72, 567–575. doi: 10.1046/j.1365-2656.2003.00726.x
Pike D. G., Gunnlaugsson T. H., Øien N., Desportes G., Víkingsson G. A., Paxton C. G. M., et al. (2005). Distribution, abundance and trends in abundance of fin and humpback whales in the North Atlantic (Copenhagen: ICESCM2005/R:12).
Pollock K. H. (1982). A capture-recapture design robust to unequal probability of capture. J. Wildl. Manage. 46, 752–757. doi: 10.2307/3808568
Pradel R., Gimenez O., Lebreton J.-D. (2005). Principles and interest of GOF tests for multistate capture-recapture models. Anim. Biodivers. Conserv. 28, 189–204.
Pradel R., Hines J. E., Lebreton J.-D., Nichols J. D. (1997). Capture-recapture survival models taking account of transients. Biometrics 53, 60–72. doi: 10.2307/2533097
Punt A., Friday N., Smith T. (2006). Reconciling data on the trends and abundance of North Atlantic humpback whales within a population modelling framework. J. Cetacean Res. Manage. 8, 145–160.
Ramp C., Bérubé M., Palsbøll P., Hagen W., Sears R. (2010). Sex-specific survival in the humpback whale Megaptera novaeangliae in the Gulf of St. Lawrence, Canada. Mar. Ecol. Prog. Ser. 400, 267–276. doi: 10.3354/meps08426
Ramp C., Delarue J., Bérubé M., Hammond P., Sears R. (2014). Fin whale survival and abundance in the Gulf of St. Lawrence, Canada. Endanger. Species Res. 23, 125–132. doi: 10.3354/esr00571
R Core Team (2020) R: A language and environment for statistical computing. Available at: https://www.r-project.org/.
Reeves R. R., Clapham P. J., Wetmore S. E. (2002). Humpback whale (Megaptera novaeangliae) occurrence near the Cape Verde Islands, based on American 19th century whaling records. J. Cetacean Res. Manage. 4, 235–253.
Reeves R. R., Smith T. D., Josephson E. A., Clapham P. J., Woolmer G. (2004). Historical observations of humpback and blue whales in the North Atlantic Ocean: clues to migratory routes and possibly additional feeding grounds. Mar. Mammal Sci. 20, 774–786. doi: 10.1111/j.1748-7692.2004.tb01192.x
Robbins J., Pace R. M. (2018) Trends in abundance of North Atlantic humpback whales in the gulf of Maine (Woods Hole, MA: EE133F-17-SE-1320 Task I Contract Report). Available at: https://www.gpo.gov/fdsys/pkg/FR-2016-09-08/pdf/2016-21276.pdf (Accessed May 10, 2021).
Roberts J. (2011). Maritime traffic in the Sargasso Sea: An analysis of international shipping activities and their potential environmental impacts (Hampshire, UK: Report to IUCN Sargasso Sea Alliance Legal Working Group by Coastal & Ocean Management).
Rossi-Santos M. R. (2015). Oil industry and noise pollution in the humpback whale (Megaptera novaeangliae) soundscape ecology of the southwestern Atlantic breeding ground. J. Coast. Res. 31, 184–195. doi: 10.2112/JCOASTRES-D-13-00195.1
Ruegg K., Rosenbaum H. C., Anderson E. C., Engel M., Rothschild A., Baker C. S., et al. (2013). Long-term population size of the North Atlantic humpback whale within the context of worldwide population structure. Conserv. Genet. 14, 103–114. doi: 10.1007/s10592-012-0432-0
Schleimer A., Ramp C., Delarue J., Carpentier A., Bérubé M., Palsbøll P. J., et al. (2019). Decline in abundance and apparent survival rates of fin whales (Balaenoptera physalus) in the northern Gulf of St. Lawrence. Ecol. Evol. 9, 4231–4244. doi: 10.1002/ece3.5055
Schwarz C. J., Arnason A. N. (1996). A general methodology for the analysis of capture-recapture experiments in open populations. Biometrics 52, 860–873. doi: 10.2307/2533048
Seber G. A. F. (1965). A note on the multiple-recapture census. Biometrika 52, 249–259. doi: 10.2307/2333827
Seber G. A., Schofield M. R. (2019). Capture-recapture: Parameter estimation for open animal populations (Cham, Switzerland: Springer).
Simard Y., Roy N., Giard S., Aulanier F. (2019). North Atlantic right whale shift to the Gulf of St. Lawrence in 2015, revealed by long-term passive acoustics. Endanger. Species Res. 40, 271–284. doi: 10.3354/esr01005
Simmons S., Bax N., Costa D., Wallace B. (2017) Essential ocean variables (EOV) for biology and ecosystems: Marine turtle, bird and mammal abundance and distribution. Available at: https://www.goosocean.org/index.php?option=com_oe&task=viewDocumentRecord&docID=17511 (Accessed May 10, 2021).
Širović A., Hildebrand J. A., McDonald M. A. (2016). Ocean ambient sound south of Bermuda and Panama Canal traffic. J. Acoust. Soc Am. 139, 2417–2423. doi: 10.1121/1.4947517
Smith T. D., Allen J., Clapham P. J., Hammond P. S., Katona S., Larsen F., et al. (1999). An ocean-basin-wide mark-recapture study of the North Atlantic humpback whale (Megaptera novaeangliae). Mar. Mammal Sci. 15, 1–32. doi: 10.1111/j.1748-7692.1999.tb00779.x
Smith T. D., Reeves R. R. (2010). Historical catches of humpback whales, Megaptera novaeangliae, in the North Atlantic Ocean: Estimates of landings and removals. Mar. Fish. Rev. 72, 1–42.
Somerford T. R., Dawson S. M., Slooten E., Guerra M., Childerhouse S. J., Richter C. F., et al. (2022). Long-term decline in abundance of male sperm whales visiting Kaikōura, New Zealand. Mar. Mammal Sci. 38, 606–625. doi: 10.1111/MMS.12886
Stepanuk J. E. F., Heywood E. I., Lopez J. F. Jr., DiGiovanni R. A., Thorne L. H. (2021). Age-specific behavior and habitat use in humpback whales: implications for vessel strike. Mar. Ecol. Prog. Ser. 663, 209–222. doi: 10.3354/MEPS13638
Stevenson A. (2011) Humpback whale research project, bermuda. Sargasso Sea alliance sci. rep. ser. Available at: http://www.sargassoseacommission.org/storage/documents/No.11_WhaleResearch_HI.pdf (Accessed May 20, 2022).
Stevick P., Allen J., Clapham P., Friday N., Katona S., Larsen F., et al. (2003). North Atlantic humpback whale abundance and rate of increase four decades after protection from whaling. Mar. Ecol. Prog. Ser. 258, 263–273. doi: 10.3354/meps258263
Stevick P. T., Allen J., Clapham P. J., Katona S. K., Larsen F., Lien J., et al. (2006). Population spatial structuring on the feeding grounds in North Atlantic humpback whales (Megaptera novaeangliae). J. Zool. 270, 244–255. doi: 10.1111/j.1469-7998.2006.00128.x
Stevick P. T., Berrow S. D., Bérubé M., Bouveret L., Broms F., Jann B., et al. (2016). There and back again: Multiple and return exchange of humpback whales between breeding habitats separated by an ocean basin. J. Mar. Biol. Assoc. United Kingdom 96, 885–890. doi: 10.1017/S0025315416000321
Stevick P. T., Bouveret L., Gandilhon N., Rinaldi C., Rinaldi R., Broms F., et al. (2018). Migratory destinations and timing of humpback whales in the southeastern Caribbean differ from those off the Dominican Republic. J. Cetacean Res. Manage. 18, 127–133.
Stevick P. T., Carlson C. A., Balcomb K. C. (1999). A note on migratory destinations of humpback whales from the eastern Caribbean. J. Cetacean Res. Manage. 1, 251–254.
Stevick P. T., Palsbøll P. J., Smith T. D., Bravington M. V., Hammond P. S. (2001). Errors in identification using natural markings: rates, sources, and effects on capture-recapture estimates of abundance. Can. J. Fish. Aquat. Sci. 58, 1861–1870. doi: 10.1139/f01-131
Stone G. S., Katona S. K., Tucker E. B. (1987). History, migration and present status of humpback whales Megaptera novaeangliae at Bermuda. Biol. Conserv. 42, 133–145. doi: 10.1016/0006-3207(87)90019-X
Taylor B. L., Gerrodette T. (1993). The uses of statistical power in conservation biology: The vaquita and northern spotted owl. Conserv. Biol. 7, 489–500. doi: 10.1046/j.1523-1739.1993.07030489.x
Tulloch V. J. D., Plagányi É. E., Brown C., Richardson A. J., Matear R. (2019). Future recovery of baleen whales is imperiled by climate change. Glob. Change Biol. 25, 1263–1281. doi: 10.1111/gcb.14573
Valsecchi E., Hale P., Corkeron P., Amos W. (2002). Social structure in migrating humpback whales (Megaptera novaeangliae). Mol. Ecol. 11, 507–518. doi: 10.1046/j.0962-1083.2001.01459.x
Víkingsson G. A., Pike D. G., Valdimarsson H., Schleimer A., Gunnlaugsson T., Silva T., et al. (2015). Distribution, abundance, and feeding ecology of baleen whales in Icelandic waters: have recent environmental changes had an effect? Front. Ecol. Evol. 3. doi: 10.3389/fevo.2015.00006
Visser F., Hartman K., Pierce G., Valavanis V., Huisman J. (2011). Timing of migratory baleen whales at the Azores in relation to the North Atlantic spring bloom. Mar. Ecol. Prog. Ser. 440, 267–279. doi: 10.3354/meps09349
Vogt P. R., Jung W.-Y. (2007). “Origin of the Bermuda volcanoes and the Bermuda rise: History, observations, models, and puzzles,” in Plates, plumes and planetary processes (Boulder, CO: Geological Society of America), 553–591. doi: 10.1130/2007.2430(27
Wenzel F. W., Broms F., López-Suárez P., Lopes K., Veiga N., Yeoman K., et al. (2020). Humpback whales (Megaptera novaeangliae) in the Cape Verde Islands: Migratory patterns, resightings, and abundance. Aquat. Mamm. 46, 21–31. doi: 10.1578/AM.46.1.2020.21
Wenzel L., Cid G., Haskell B., Clark A., Quiocho K., Kiene W., et al. (2019). You can choose your relatives: Building marine protected area networks from sister sites. Aquat. Conserv. Mar. Freshw. Ecosyst. 29, 152–161. doi: 10.1002/aqc.3041
White G. C., Burnham K. P. (1999). Program MARK: Survival estimation from populations of marked animals. Bird Study 46, S120–S139. doi: 10.1080/00063659909477239
Wickham H. (2016) ggplot2: Elegant graphics for data analysis (New York: Springer-Verlag). Available at: https://ggplot2.tidyverse.org (Accessed April 1, 2020).
Worthington H., King R., McCrea R., Smout S., Pomeroy P. (2021). Modeling recruitment of birth cohorts to the breeding population: A hidden Markov model approach. Front. Ecol. Evol. 9. doi: 10.3389/fevo.2021.600967
Worthington H., McCrea R., King R., Griffiths R. (2019). Estimating abundance from multiple sampling capture-recapture data via a multi-state multi-period stopover model. Ann. Appl. Stat. 13, 2043–2064. doi: 10.1214/19-AOAS1264
Zerbini A. N., Clapham P. J., Wade P. R. (2010). Assessing plausible rates of population growth in humpback whales from life-history data. Mar. Biol. 157, 1225–1236. doi: 10.1007/s00227-010-1403-y
Keywords: baleen whale, humpback whale, Megaptera novaeangliae, abundance, capture-recapture, migratory stopover
Citation: Grove T, King R, Stevenson A and Henry L-A (2023) A decade of humpback whale abundance estimates at Bermuda, an oceanic migratory stopover site. Front. Mar. Sci. 9:971801. doi: 10.3389/fmars.2022.971801
Received: 17 June 2022; Accepted: 09 December 2022;
Published: 06 January 2023.
Edited by:
Albertus J. Smit, University of the Western Cape, South AfricaReviewed by:
Simon Elwen, Stellenbosch University, South AfricaLeah M. Crowe, Integrated Statistics, United States
Copyright © 2023 Grove, King, Stevenson and Henry. This is an open-access article distributed under the terms of the Creative Commons Attribution License (CC BY). The use, distribution or reproduction in other forums is permitted, provided the original author(s) and the copyright owner(s) are credited and that the original publication in this journal is cited, in accordance with accepted academic practice. No use, distribution or reproduction is permitted which does not comply with these terms.
*Correspondence: Thomas Grove, dG9tZ3JvdmUyMEB5YWhvby5jby51aw==