- 1 Management Department, Universitat Politècnica de València, València, Spain
- 2 Research Institute for Integrated Management of Coastal Areas (IGIC), Universitat Politècnica de València, Gandia, Spain,
- 3 Department of Agricultural, Food and Forest Science, University of Palermo, Palermo, Italy
Customers’ attention to sustainability labels in fishery and aquaculture products (FAPs) has been increasing in the last decades, and the industry has adapted to this growing interest by adopting fish ecolabels. However, there is a growing interest to widen the sustainability concept to include the social and ethical information of the fishery and aquaculture industry and to go further from the voluntary approach on the labeling of these aspects in FAPs. For this reason, using data from 2021 Eurobarometer and using machine learning techniques, we disentangle the characteristics of the FAP buyers that consider the importance of environmental impact, ethical, and social information appearing on FAP labeling. The results confirmed that most of the consumers who consider environmental, social, and ethical aspects when buying FAPs also think that this information should be labeled. In line with other works, young, educated, and environmentally aware consumers in high-income countries are more likely to request this information in the FAP label. One interesting finding of the study relates with the asymmetric impact of the variables and the important group of respondents who do not consider these aspects but also advocate to include them in the FAP label. The study outcomes can be beneficial for policymakers to design future public policies regarding FAP labeling, as well as to be taken into consideration in the marketing policies of fishery and aquaculture producers and retailers.
1 Introduction
The production and consumption of fishery and aquaculture products (FAPs) have a range of social, ethical, and environmental effects that condition the livelihood and well-being of many coastal communities and threaten the sustainability of seas and oceans (Nelson et al., 2022). Increasing the sustainability of these markets is a challenge for public authorities and even for the sectors and companies involved. However, the consumer does not play a minor or passive role in this process (Penca, 2020). In fact, in recent years, there has been a growing concern on the part of many consumers regarding the ethical, social, and environmental consequences of their purchases (Del Giudice et al., 2018; Panda et al., 2020), and this perception even seems to have increased after the period of the COVID-19 pandemic (Birtus and Lăzăroiu, 2021; Rydell and Suler, 2021; Glogovețan et al., 2022). This awareness manifests itself not only in an increase in supply and demand for products that certify their sustainability, but also in a demand for information that goes beyond the mandatory label.
Traceability and labeling of seafood are key elements to protect the interests and health of consumers and to move towards sustainable exploitation of maritime resources (Lewis and Boyle, 2017; Tinacci et al., 2019). Labels, in addition to providing the consumer with information on aspects such as safety, quality, health, and origin of products, also allow the communication of statements on ethical issues (Penca, 2020), which, in the fisheries sector, are related to the overexploitation of fish resources, the impoverishment of marine ecosystems, and the expression of the link between human and ecosystem wellbeing (Galati and Crescimanno, 2012; Autzen and Ounanian, 2021). In the present study, the regulations on seafood labeling have focused on requiring useful, understandable, and credible labeling that provides a minimum guarantee for the purchase and consumption process (Paolacci et al., 2021). For instance, in the European scenario, the regulations (EU) No. 1169/2011 and (EU) No. 1379/2013 specify the mandatory information that must be present on seafood labels. These standards also outline some of the voluntary information that may appear on the label, but always bearing in mind that voluntary information must not be displayed to the detriment of the space available for mandatory information. Nevertheless, the seafood market is considered to be one of the sectors where most food fraud takes place (Mariani et al., 2015; Silva et al., 2021), which can increase consumer distrust and fear. This is why the FAO itself recommends reinforcing traceability and labeling systems (FAO, 2018).
In an environment where consumer demands are continuously increasing and environmental awareness is considerable (Jacquet and Pauly, 2007), it seems that a significant group of consumers are beginning to demand labeling that goes beyond the legal requirements. This consumer interest has not gone unnoticed by companies, in both production and distribution. In fact, corporate social responsibility has become a key aspect of the current business strategy, which is partly due to the existence of a more responsible consumer concerned about the consequences of his or her purchase. This is especially significant in food products and seafoods, where this altruistic concern (self-transcendent) is mixed with one’s own concern (self-enhancing) for food safety and quality (De Dominicis et al., 2017) and quickly identifies with marine environmental problems such as overfishing, habitat degradation, the presence of micro-plastics or mercury, and the disappearance of species (Galati et al., 2015; Giacomarra et al., 2021).
To date, few studies have been carried out in order to analyze the consumers’ knowledge and perception toward seafood ecolabels and their willingness to pay a premium price for ecolabeled products (Brécard et al., 2009; Uchida et al., 2014; Bronnmann and Hoffmann, 2018). Findings reveal a positive perception and WTP of consumers for fish ecolabeling, such as MSC, FSC, or organic aquaculture, as a consequence of their awareness of the social, environmental, and economic impacts linked to their decision-making process (Onozaka et al., 2010; Salladarré et al., 2010; Uchida et al., 2014; Menozzi et al., 2020; Galati et al., 2021). In particular, the greater the environmental awareness, the more attention consumers will pay to ecolabels (Galati et al., 2021). This environmental awareness can be related to concepts such as ecological citizenship (Dobson, 2007) that encompasses a theory of change towards sustainable lifestyles and living standards (Wolf et al., 2009). This ecological citizenship can manifest itself in different ways, such as concern about climate change, the deterioration of nature, or the ecological footprint. In a similar way, the analysis of consumer altruism is the object of this study. A recent cross-country study (Galati et al., 2021) reveals that Italian and Spanish consumers with an altruistic attitude, who identify seafood products produced in a sustainable way with a greater protection of the environment and workers, pay more attention to ecolabeling during the decision-making process. However, as several authors emphasize, if it is true that consumers attach importance to the sustainability of fish products, this attention is not reflected on their choices highlighting that ethical and social considerations do not shape the fish consumers’ behavior (Verbeke et al., 2007). In line with this, McClenachan et al. (2016) find that consumers of seafood attach less importance to the benefits that their choice may have on coastal communities, highlighting the need for more information on ethical and social aspects of certifications. Moreover, as some authors emphasize, there is a lack of initial awareness of seafood ecolabels, deriving from a poor attitude or lack of interest in reading labels, and often a lack of reliable information on the environmental impacts of fishing activity (Travaille et al., 2019). This is linked to what is known as “food illiteracy” (i.e., the general lack of knowledge about production processes and their environmental impacts) and the influence that this “food illiteracy” may have on the consumers’ choices. Therefore, as some authors emphasize, knowledge of the ecolabel meaning, also through the information contained therein, is a key aspect that can make consumers’ choices increasingly responsible (Jonell et al., 2016; Winson et al., 2022). Thereby, the influence of socio-demographic characteristics on purchasing decisions is relevant. Aspects such as age, gender, education levels, and income levels have been extensively studied and tend to play a significant role in determining preferences for certified or labeled products (Pérez-Ramírez et al., 2015; Kim and Lee, 2018; Galati et al., 2021). Lastly, most of the studies cited have incorporated aspects related to the purchasing and consumption process.
In any case, the cited literature is based on determining the profile of the consumer who demands ecolabeled seafood products based on psychological and socio-demographic indicators as explanatory variables and on small samples. In light of this, the aim of this paper is to study the profile of consumers who demand additional information on the ethical, social, and environmental (ESE) effects of FAPs consumed. In detail, this study is based on the application of the machine learning ensemble algorithm of the 2021 Eurobarometer 95.1, which provides data on more than 20,000 respondents from the 27 EU countries. Machine learning techniques have recently been used to accurately predict consumer preferences, as well as to capture consumer attitudes and feelings (Hopkins, 2022; Kliestik et al., 2022; Nica et al., 2022).
Obviously, this demand for information is not widespread or homogeneous across the population. In a very simplistic way, we could identify this consumer as a citizen aware of these ethical and environmental issues, and even go further and identify it with the consumer of ecolabeled products. The study of green consumer profile has shown that these characterizations are often more complex.
The European Union (EU) market, chosen as a study area, is a mature and huge market. In 2020, the EU trade of FAPs, which is the combined amounts of imports and exports with third countries, was the highest in the world, totaling EUR 31.17 billion and 8.72 million tons (EUMOFA, 2021). EU is the fifth largest producer of FAPs in the world but reports the highest overall expenditure in FAPs. The EU supply of FAPs for human consumption totaled 14.53 million tons of live weight, with an apparent consumption of 23.97 kg per capita (EUMOFA, 2021), ranking in the eighth position in terms of expenditure per capita.
In this study, we disentangle the characteristics of the FAP buyers that consider environmental impact, ethical, and social information that should appear on FAP labeling. For this objective, we started from the previous analyses based on the characterization of an ecolabeled seafood product consumer. As expected, some of the results agree with these analyses; for example, most of the consumers who consider environmental, social, and ethical aspects when buying FAPs also think that this information should be labeled. However, an important group of those that do not consider these aspects also advocate to include them in the FAP label. Moreover, environmental awareness (reflected by their concern for issues such as climate change or the deterioration of nature) is also a distinctive aspect that dramatically increases the awareness for sustainable labeling in FAPs. Finally, and in line with other works, a whole series of socio-demographic aspects are considered and provide very interesting information. In our case, we uncovered that young, educated consumers in high-income countries are more likely to request this information in the FAP label.
The study outcomes can be beneficial for policymakers to design future public policies regarding seafood labeling, as well as to be taken into consideration in the marketing policies of fishery and aquaculture producers and retailers.
2 Methodology and Materials
2.1 Sample and Variables of the Study
For this study, we used data from the Eurobarometer 95.1 (European Commission and European Parliament, Brussels, 2021) wave that was collected by Kantar at the request of the European Commission, Directorate-General for Maritime Affairs and Fisheries, between March and April 2021. We thought that this survey is relevant as it is the third on this topic (previous ones were conducted on 2016 and 2018), and it is the main tool the European Commission uses to seek out the views of its citizens related to the state of fisheries and the maritime industry. It is used for the understanding of the EU internal market for FAPs aiming for the sustainability and prosperity of the oceans, the coastal communities, and the fishing sector, thus providing important information for companies and policymakers to adapt their strategies to the changing needs of consumers. Although the survey covered different areas of interest for the European Commission, we particularly focused on the area related to EU consumer habits regarding FAPs. This includes edible fish, shellfish (prawns or crayfish), mollusks (oysters or octopus), and seaweeds. It covers FAPs that are farmed or wild and come from the sea or freshwater sources, including raw and prepared products (fresh, frozen, or tinned). From the original 26,669 respondents, we disregarded (using qd1_3) those respondents who do not buy FAPs as these subjects were not interviewed about the habits of consumption and, therefore, about our variable of interest. The final sample is composed of 21,627 respondents, of whom 10,117 (46.78%) reported that sustainability information should not be contained in the label of fishery and aquaculture.
In the database, our objective was to characterize consumers attending their desire for labeling the environmental, social, or ethical information in FAPs. ESE information is reported in 3 separate variables with a value of 1 if it was mentioned by the subject as necessary to be included in the label or 0 if it was not mentioned (q11.4, q11.5, and q11.6 in the survey). We constructed a new variable capturing the desire for sustainable labeling as a composite of the 3 variables; thus, if a respondent mentioned any of the three, we coded it as 1, and as a 0 if it did not (q11 in Table 1). We considered this option appropriate because in the same survey, the “environmental, social, or ethical impact” as a whole is one of the possible aspects to be evaluated as the most important for the respondent when buying FAPs (qd8.6).
Regarding the predictor variables, we can catalogue them into three groups: the habits regarding FAPs, the socio-economic characteristics, and the environmental awareness of the respondents. The habits regarding FAPs include questions regarding the frequency on the consumption and purchase of these products, the aspects that are considered when buying these products, or the information that should be included in the product labeling. The greater awareness about ESE issues in society is considered in the buying and decision-making process (Del Giudice et al., 2018; Panda et al., 2020; Galati et al., 2021) and, therefore, has affected the demand for sustainable certifications and labels in FAP products (Onozaka et al., 2010; Salladarré et al., 2010; Uchida et al., 2014; Lewis and Boyle, 2017; Tinacci et al., 2019). Thus, we can expect that consumer consideration of ESE aspects when purchasing FAPs is positively impacting the willingness to have ESE information included on FAP labels.
Additionally, the survey includes a complete set of descriptive variables such as age, sex, country, or years of education of the respondents. Besides the socio-demographic interest related to characterize the respondents of any survey, in the preferences for certification and labeling of products, some of these respondent characteristics have been highlighted as significant (Pérez-Ramírez et al., 2015; Kral et al., 2020; Pocol et al., 2021; Galati et al., 2021). Thus, we can expect that consumer socio-demographic characteristics impact the willingness to have ESE information included on FAP labels.
Besides the consumer habits, we included the respondent characterization variables, whether the subject perceived climate change or the deterioration of nature as the single most serious problem facing the world, and to what extent climate change is a problem at this moment. Although these variables were from another area in the Eurobarometer survey on the same respondents, previous studies (see Galati et al., 2021) suggest that environmental awareness is positively related to the attention consumers pay to ecolabels, which is expected to be highly relevant to the aim of the study. Thus, we can expect that the consumer awareness is positively impacting the willingness to have environmental, social, and ethical information included on FAP labels. Table 1 includes the different groups of variables that we decided to include as predictors in previous studies, their description, and the possible values and total number of cases in the sample.
2.2 Methodtology
In this study, we aimed to identify the main characteristics that define the customers that look for sustainability information in FAP labels. In other words, what characteristics can predict if a FAP customer considers that sustainability information should be on the FAP label. This is a classification problem in which we are looking to classify an individual into two groups, those that consider that sustainability information should be in the FAP label and those that do not. In this particular case, we have a large dataset with a large number of variables and traditional multivariate techniques have difficulties in capturing the complex interrelations between the multiple variables that can affect customers’ behavior and decisions. The complexity of the problem requires exploring machine learning techniques that have been demonstrated to outperform traditional multivariate techniques in these particular environments. Machine learning algorithms used for research applications have spread broadly in a few years (Web of Science hits for machine learning have grown from around 15,000 to near 100,000 from 2011 to 2021). However, there are still areas, such as those related to the environmental management, where few studies have used the potential of these evaluation techniques. For example, Malefors et al. (2021) or Grainger et al. (2018) applied these techniques for food waste prediction, or Peiró-Signes et al. (2022) used them to classify a large amount of companies into 4 different levels of environmental orientation, to determine its drivers and to expose the combinations of endogenous–exogenous drivers for companies’ ecoinnovative orientation.
For our study, we tested a set machine learning algorithms to look for the optimal one for our classification problem. Table 2 summarizes the accuracy results (average and standard deviation) of the different machine learning algorithms. To ensure that we properly capture the algorithm performance and that the subsamples effectively represent the original sample, we used all the initial variables, algorithms default parameters, and a stratified k-fold cross-validation with five splits and three repeats. Results on Table 2 recommended XGBoost for its higher average and lower standard deviation.
Therefore, we used Extreme Gradient Boosting algorithm (XGBoost) to answer our research questions. Figure 1 summarizes the methodological process of the study.
XGBoost (Chen & Guestrin, 2016) is a machine learning ensemble algorithm that has been proven to capture complex interrelations between the predictor variables and to outperform other linear and non-linear algorithms in exchange for interpretability. XGBoost adds trees (weak learners) to the model of a stage-wise procedure. Classification trees are added following a gradient descent procedure to reduce the log loss. Then, the model containing multiple trees is used to predict the class of new individuals. To evaluate the performance of the model, we used accuracy that measures the percentage of cases that have been correctly predicted by a model.
2.2.1 Data Preparation
Before applying a machine learning algorithm, there is a need of data preparation to adjust for the algorithm requirements and to facilitate interpretation of the model results afterwards. XGBoost has little requirements because it can handle categorical numerical data directly. However, for interpretation purposes, we decided to convert all the categorical nominal variables to dummies. The survey also contained multiclass variables, with multiple groups to classify the respondents. Some of these variables are an aggregation of a continuous variable (i.e., age) and others are the representation of different groups (i.e., country or living area). In these cases, we decided to use predefined groups that are included in the database (for age, we use 4 class classification: 55–98, 40–54, 25–39, and 15–24) or to create groups (for the variable “country”, we classified each respondent in two groups depending on whether the GDP per capita of his or her home country is above or below the EU average). Thus, we transformed the 47 variables included in the study from the original database into 132 recoded variables.
However, not all of these variables will be relevant to determine our outcome. These “extra” variables add no valuable information and complicate the model unnecessarily. Moreover, they increase computing requirements and, in many cases, reduce the performance (Kohavi & John, 1997; Kuhn & Johnson, 2013). Using a smaller number of variables that optimize the performance is recommended (Nilsson et al., 2007). This process is called feature selection.
2.2.2 Feature Selection
To reduce the number of features for machine learning, they typically have built in algorithms based on the search for the minimal optimal (non-redundant) set of features that are useful for the prediction. These feature selection algorithms depend on the classifier and rely on accuracy to select the features, which is a sufficient condition to declare a feature as important but not sufficient to declare it as unimportant (Kursa & Rudnicki, 2010). Additionally, it requires the researcher to determine a cutoff level to include or exclude features with no objective criteria. Thus, we decided to use the BorutaShap algorithm (Keany, 2020) to determine the relevant features. Boruta uses an iterative process with a statistical test to remove the features that are demonstrated to be less relevant than their random probes (Stoppiglia et al., 2003), leading to an unbiased and consistent classification of important and non-important features.
The first step in this process is to determine the baseline performance of the model. We calculated the initial accuracy of the XGBoost model with all the features and the default options. The evaluation of the model requires dividing the dataset into two sets. The first set is used to build the model, the training dataset, and the second to validate it, the test dataset. We used a stratified 80%–20% split for training and testing. The baseline model classified correctly 67.15% (accuracy) of the cases in the testing dataset.
Secondly, we applied the BorutaShap algorithm to uncover 21 important features among all the variables (see Figure 2). Then, for the next steps in the model development, we kept only the selected features. The new accuracy for this more parsimonious model with the 21 relevant variables and the default learning parameters was 66.32%.
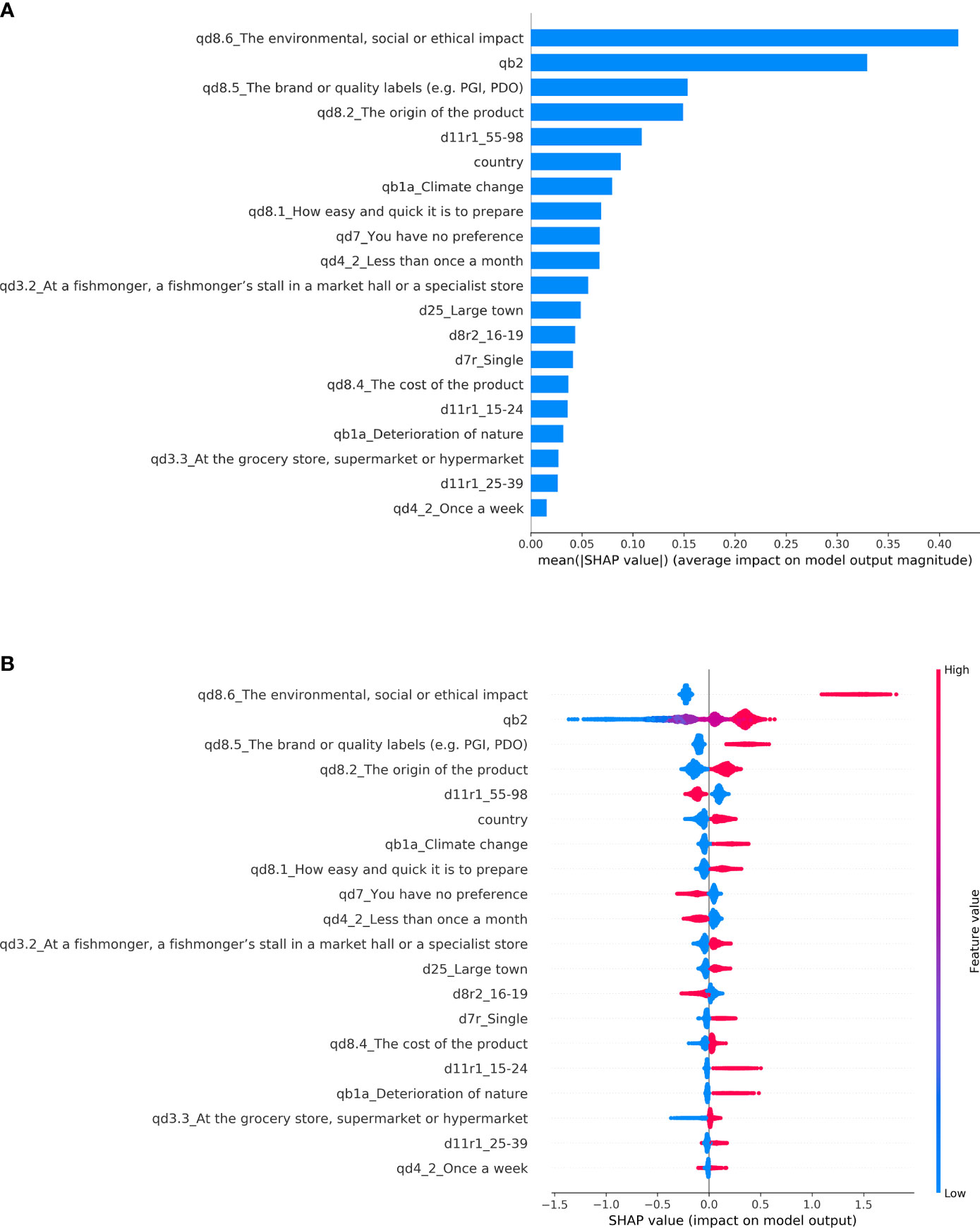
Figure 2 Feature importance and summary plot. (A) SHAP feature importance, (B) Distribution of the SHAP values per feature.
2.2.3 Tuning the Model
Tuning consists of the determination of the learning task parameters that are optimal for the classification problem. The XGBoost algorithm tends to overfit to the training dataset, which eventually reduces the performance (accuracy) in the classification when we apply the model to the testing database. Controlling for the learning rate and the maximum depth of the trees, or using random subsamples of the training database, among others, creates slightly different trees, adding variance and improving the model performance.
We used a grid search procedure on the most relevant task parameters to find an optimal solution to our classification problem. Taking the default parameters accuracy as a baseline, we introduced in a step-by-step procedure that performed different task parameters. In each step, we tested one or two task parameters with multiple values. Then, we moved to the next step (the next task parameters) keeping the best parameter value from that point forward. Table 3 shows the tuning process and the corresponding accuracy for each step.
2.2.4 Interpreting the Model
There are two steps in the model interpretation. The first evaluates the goodness of the model in terms of accuracy. In other words, is the model able to perform well? Second, we want to evaluate the importance and direction of the predictors in the model.
Based on multivariate thresholds, a good classification model should have a higher accuracy than the maximum chance criterion and an accuracy of 25% higher than the proportional chance criterion (Hair et al., 2014). On the other side, to evaluate the feature impact in the model, we can use Shapley or Shap values. SHapley Additive exPlanations (Lundberg & Lee, 2017; Lundberg et al., 2020) or Shap values represent a feature contribution to the machine learning model. The feature importance based on Shap values represents the average of the marginal contributions of that feature to the model. Then, the predictions are explained as the sum of the values attributed to each feature.
3 Results
3.1 Model Results
At the end of the tuning process, the accuracy of the model was 67.13%. In Table 4, we compared actual vs. predicted classes for the test sample. This accuracy is higher than the maximum chance criterion (53.2%) and an accuracy higher than 25% of the proportional chance criterion (62.7%), indicating that the model is good enough. Table 4 shows the diagonal cases that have been classified correctly, and the misclassified cases are outside the diagonal. The model performed slightly better on classifying customers who wanted sustainable information to be on the FAP labels than those who do not (69% vs. 64%).
Regarding the feature importance, Figure 2A collects the feature Shap values sorted by its importance (average contribution) in the model. Figure 2B shows the direction of each feature contribution and how case Shap values are distributed. Red and blue points indicate high and low values of the feature, respectively.
For example, qd8.6 represents whether the Environmental, Social, and Ethical (ESE) impact is considered among the most important aspects when buying FAPs. As this variable is either present or absent, a value of 1 (red color), that is, that sustainability aspects are considered when buying FAPs, gets high positive Shap values (between 1.2 and 2), increasing the likelihood to view sustainable impact as important to be included in FAP labels (see Figures 2B, 3A). However, we can see a high concentration of blue points close to 0, which indicates a value of 0 in the qd8.6 variable, which represents that the respondent does not consider ESE impact when buying and contributes little to the chances of the person to consider ESE impact information as important to be included in FAP labeling.
Similarly, we can evaluate the impact of the different levels of respondents’ climate change concern (qb2) in the chances to consider ESE impact information as important in FAP labeling. Figure 3B shows the increase of the Shap values as respondents’ concerns about climate change growth. Then, the higher the concern, which is a higher Shap value assigned by the algorithm, the higher the likelihood to be classified as an ESE labeling supporter.
Overall, only few features emerge as very important. In addition to the aforementioned consideration of ESE impact, respondents perceiving climate change or the deterioration of nature as the single most serious problem facing the world and those that evaluate more seriously climate change as a problem at this moment are more likely to request for sustainable labeling in FAPs. Regarding the socio-demographic aspects, we can see that being single (d7r), younger than 55 years old (d11r1), living in a large town (d25), in a high GDP per capita country (country), and not in the group with 16 to 19 years of education (d8r2) increase the chances to be classified as demanding sustainable labeling.
Regarding the consumer habits on FAPs, those that consider several aspects when buying, such as brand or quality labels (qd8_5) or the origin of the product (qd8_2), are more likely to demand sustainable labeling. This also happens with EU consumers who have preference for wild products (qd7) or who buy FAPs in a specialty store (qd3.2). On the contrary, consumers who do not have any preference for wild of farmed products (qd7) or who consume fresh fish less than once a week (qd4.2) are less likely to demand ESE information to be included in the labeling.
The Shap also allows for local interpretation. That is, we can evaluate in each particular case (respondent) how each of the characteristics and FAPs’ consumption habits are working for the classification in either one of the two groups of interest. Figures 4A, B show two particular individuals with high and low Shap values, respectively.
For example, respondents in Figure 4A show the consideration of the ESE impact when buying FAPs (qd8.6 = 1) and is the feature that pushes 1.62 units of the Shap value to the right and, therefore, increases the likelihood to be classified as a consumer who would request ESE labeling in FAPs. Similarly, in Figure 4B, the respondent correctly answering 3 out of 10 questions on how serious a problem climate change is at the moment (qb2) pushes the Shap value 0.99 units to the left (negative value), reducing the chances to be classified as a consumer who would request ESE labeling in FAPs.
Additionally, we performed a Pearson χ2 test and Cochran–Armitage trend test for the dummies and ordinal selected variables. The test will allow us to see whether the distribution of respondents who consider or do not consider that sustainability information should be in FAP labels varies across the levels of the variables that were relevant in our model. Table 5 summarizes the corresponding results.
Results indicate that respondents who considered environmental, social, and ethical impact when buying FAPs are more likely to request this information to be included in the FAP label. For example, 85.38% (2,891/3,386) of the respondents who consider ESE impact when buying advocate for ESE information to be included in the FAP label, while this percentage is only 47.25% (8,619/18,241) for the group that do not consider this information should be included in the FAP label. Similarly, 82.1% (2,780/3,386) of the respondents who feel climate change as the single most serious problem today advocate for sustainable FAP labeling while this percentage is only 47.8% (8,730/18,241) for those who do not consider sustainable information necessary in FAP labels.
This raises a discussion about why people who take into consideration sustainability when buying do not feel the need to give this information through labeling (14.62%). More importantly, why do more than half of the consumers who do not consider sustainable aspects when buying actually request this information to be included in the label?
4 Discussion and Conclusion
Many studies have been focused on the consumer interest in fish ecolabels, especially on the recognition of this label (Taufique et al., 2016) and the willingness to pay for ecolabeled products (Thøgersen et al., 2010; Mulazzani et al., 2021). Ecolabels have been promoted by very diverse organizations and have been related to different specific or general aspects linked to sustainability. In fact, the first ecological labels were linked to the protection of certain species such as the Dolphin Safe, but in recent years, there has been a boom in more general ecolabels related to FAPs (i.e., MSC, ASC, FOS, BAP, and CGN). This has caused a growing interest not only from the industry but also from the academic community for studying this phenomenon from different perspectives, among which the characterization of the consumer who demands these products stands out. In any case, this type of labeling is based on voluntary approaches to sustainability; however, here we face consumer demand for sustainability information in mandatory labeling.
The growing concern about the environmental or ethical aspects involved in purchasing is evident. The adoption of labels and certificates that guarantee or highlight the sustainability of products has been the response of the industry; however, the consumer seems to demand that this information appear on the mandatory label. This impression is confirmed by the Eurobarometer 95.1 that serves as the basis for this analysis. One of the questions shows us that more than more than half of European fish consumers are in favor of additional sustainability information appearing on the label.
This key result does not require any statistical analysis, although it should lead to the reflection by public authorities and sectors. In any case, it opens an important discussion, which leads us to ask who demands this mandatory information on sustainability (and who does not). This novel approach changes the focus from previous studies that were centered on voluntary ecolabeling and the consumer, to mandatory labeling for an extended sustainability concept, including not only environmental information, but also social and ethical information.
To pose this question, we based our research on previous analyses of the profile of consumers who chose products that guaranteed sustainability through ecolabeling. In the line of these previous analyses, a first battery of indicators related to socio-demographic issues or consumptions habits were tested.
From a socio-demographic perspective, we can differentiate characteristics that are increasing the chances of being classified (or not) as an ESE-label petitioner. In particular, respondents who are between 14 and 39, are single, or live in large towns are more likely to think that ESE should be mentioned on the label for all FAPs. On the contrary, respondents with 16 to 19 years of education and aged 55 and over are less likely to think that ESE should be mentioned on the label for all FAPs. These results align with previous studies related to the purchase intention of ecolabeled products. Indeed, the knowledge of a food ecolabel is linked to the consumers’ educational level (Thøgersen, 2017) and fish ecolabels impact positively on consumers with higher levels of education (Haghiri, 2014; Rihn et al., 2019; Wang and Somogyi, 2019). Age has been identified as an element that influences the attention paid to ecolabels. Most of the studies found that young people are more concerned about products with ecolabels (Salladarré et al., 2010; Asche and Bronnmann, 2017; Mulazzani et al., 2021), but others like Galati et al. (2021) show the opposite. These studies generally had a limited scope in terms of the geographic location (the country) and the type of products included in the study, which explains the heterogeneity of the results. Indeed, we also found the economic level of the country as a significant factor in differentiating ESE-label petitioners from non-petitioners. Finally, regarding the socio-demographic characteristics of the respondents, being single increases the chances to be classified as an ESE-label petitioner.
Respondent consumption habits reveal to be marginal in the chances of being classified as an ESE-label petitioner. However, it has a greater impact on the chances of being classified as a non-ESE-label petitioner. In particular, respondents who have a very low FAP consumption (never or less than once a year) or do not prefer wild or farmed products do not think ESE information should be mentioned on the label for FAPs. Consistent with this, Mulazzani et al. (2021) found that a higher frequency of consumption is associated with a greater willingness of consumers to pay a premium price for certified products probably because they pay attention to the health and nutritional benefits (Murray et al., 2017). Other aspects that the consumer evaluates when buying FAPs, like the brand or quality labels or the origin of the product, were revealed to also have a positive impact.
A determining issue that appears in previous studies on ecolabeling is the evident relationship between environmental awareness and the consumption of sustainable products. This relationship is also evident here when we ask about the degree of concern about climate change or the destruction of nature. As expected, the most relevant aspect for consumers to demand ESE information is related to the consideration of ESE impact when purchasing FAPs. However, results indicate that an important number of consumers who do not consider ESE impact do demand ESE information to be included in the labels. In fact, considering ESE impact is an important aspect to request of ESE labeling but not considering it is not as important to the opposite. These asymmetries in the impact of the different variables are an important finding of our study. Typically, regression-based models assume symmetric relationships between the variable of interest and the predictors. That is, when one independent variable increases, that would increase the value of the dependent variable, and when it decreases, the independent variable will decrease in the same proportion. In our study, the methodology allowed us to reveal that the majority of the variables are asymmetrically related to our variable of interest (the willingness to have ESE information in FAP labels). Then, when they increase (or are present), they work to increase the likelihood of being classified in one group, but when they decrease (or are absent), they have a very small impact on the chances of being classified in the other group. Previous studies have already detected consumer behavior asymmetries (Putler, 1992; Rajagopal, 2006; Strong et al., 2019). The asymmetric relationships between the variable of interest and the predictors might indicate that the patterns or combinations of attributes that characterize respondents who think that ESE information should be mentioned on the label for all FAPs are different from those respondents who think the opposite.
Finally, the study also revealed that consumers who are sensible to climate change and to the deterioration of nature are also more likely to think that ESE information should be mentioned on the label for all FAPs. Unlike what occurred with the consideration of the ESE impact when purchasing FAPs, low values in the importance that customers give to climate change as a problem today increases dramatically the chances to be classified as a non-ESE-label seeker, while high values given to climate change have a positive but moderate impact, highlighting again the asymmetry of the drivers to the willingness to have ESE information on FAP labels.
Overall, these findings confirm that concerns for environmental issues such as climate change, which affect the marine ecosystem, are important drivers affecting the consumer buying process. The growing attention to environmental issues and the impacts of one’s choices, as some authors point out, are linked to altruism, which is linked to the desire to help others but that at the same time produce moral satisfaction and psychological benefits (Batson and Powell, 2003; Yadav and Pathak, 2016). Galati et al. (2021) found that the interest of Italian and Spanish consumers in the information contained in the labels of fish products is closely linked to the altruistic values of consumers.
The results of this research shed light on the profile of the FAP consumer and its relationship with labeling. Until now, industry and public sector efforts seem to have been directed at promoting voluntary ecolabeling aimed at attracting a highly conscious public. However, the first thing that strikes the Eurobarometer is that a major proportion would like the mandatory labeling to contain information on ethical, social, and ecological aspects. Indeed, the inclusion of the ESE information has increased since the previous Eurobarometer in 2018 (i.e., for the environmental information + 5%). This may mark a trend in the future, and it would be a key change to stop considering the ESE information as voluntary, and to start considering it as mandatory. Studying the profile of the consumer who demands information has been the objective of this research. In fact, a good part of the results are in line with previous works on the profile of the consumer of ecolabeled seafood products. However, the innovative methodology used here has allowed us to work with a large database that covers different countries and encompasses different issues. This study provides both managerial and political implications. From a practical point of view, this study could be useful for fishermen and fish farmers to define more effective marketing strategies capable of providing information of interest to consumers in order to make them more aware of their choices. In terms of political perspectives, results underline the need to launch communication campaigns aimed at informing and educating consumers about environmental values and the importance of protecting maritime resources. Improvement of the information literacy through education is an important way of reducing information asymmetry and guaranteeing informed choices among consumers. The latter should clearly understand not only environmental but also ethical and social implications of adopting responsible behavior in the fishery and aquaculture sector. In light of this, future amendments to the EU regulation on mandatory information in seafood labels (EU 1379/2013) should provide for the inclusion of environmental, ethical, and social information in order to create greater consumer awareness on the consequences of their choices on the marine ecosystem and on marine communities.
5 Limitations and Future Directions
The study is not without limitations. The data were collected during the coronavirus pandemic; therefore, it can deviate from previous regular citizen behavior. In particular, the majority of the respondents (80%–84%) reported that their consumption of FAPs remained about the same as a result of COVID-19. Respondents who changed their consumption as a result of the COVID-19 pandemic reported that the main reasons to change is related to health and financial reasons. COVID-19 has accelerated some changes in society. We can probably expect a faster evolution on the environmental, social, and ethical awareness of FAP consumers’ tendency in the different waves of the survey that will have to be monitored in further research.
In addition, due to COVID-19, some countries only conducted online interviews in the form of probabilistic or access panels while other countries did it face-to-face. The different interview methods can cause interviewer effect and social desirability, attitudinal and demographic differences, or mode differences that can bias the results (Duffy et al., 2005).
The study was restricted to the socio-demographic and consumer habits regarding FAP data collected in the survey. Other socio-demographic information or consumer habits regarding other products, particularly food products, can also have an influence on consumer behavior and particularly to their position regarding the inclusion of ESE information in food products and, particularly, in FAPs. Further research on how general behavior and food consumer behavior affect the request for ESE information in FAPs is necessary.
Machine learning algorithms have a typically better performance in classification problems than traditional multivariate techniques, and they are very useful in environments with large datasets and a considerable number of variables. However, to the researcher, the model is like a black box, making it difficult to disentangle the logic and the connections between the variables involved in the prediction. For example, the consumer combinations of conditions that lead to a certain consumer behavior cannot be deduced from the model. This limitation opens the possibility for future research to combine the use of machine learning algorithms with other methodologies, like in Peiró-Signes et al. (2022), to complement the analysis, the results, and the conclusions of the study.
Finally, some study results (e.g., more than half of the consumers who do not consider sustainable aspects when buying actually request for ESE information to be included in the label) require further research to reveal the underlying reasons for these consumers’ point of view.
Thus, the results obtained and the methodology used have opened the door to future research that delve into this key issue for the future of the fishery and aquaculture sector, as well as for the sustainability of the entire maritime system.
Data Availability Statement
Publicly available datasets were analyzed in this study. This data can be found here: Eurobarometer 95.1 (2021). GESIS Data Archive, Cologne. ZA7781 Data file Version 1.0.0 https://doi.org/10.4232/1.13791.
Author Contributions
AP-S: Data curation, methodology, calculation, and interpretation of statistical results. LM-P, AG, and MS-O: Conceptualization, literature review, and writing. All authors contributed to the article and approved the submitted version.
Funding
This work was supported by the National Plan for Scientific and Technological Research and Innovation (Spanish Economy and Competitiveness Ministry), the Research Project PID2019-105497 GB-I00, the European Fisheries Funds, opportunities for the fisheries sector through diversification, and the FLAGs management (DivPesc).
Conflict of Interest
The authors declare that the research was conducted in the absence of any commercial or financial relationships that could be construed as a potential conflict of interest.
Publisher’s Note
All claims expressed in this article are solely those of the authors and do not necessarily represent those of their affiliated organizations, or those of the publisher, the editors and the reviewers. Any product that may be evaluated in this article, or claim that may be made by its manufacturer, is not guaranteed or endorsed by the publisher.
References
Asche F., Bronnmann J. (2017). Price Premiums for Ecolabelled Seafood: MSC Certification in Germany. Aust. J. Agric. Resource Economics 61 (4), 576–589. doi: 10.1111/1467-8489.12217
Autzen M., Ounanian K. (2021). ‘It’s How You Catch the Fish’: Debates on Ecolabelling, Yield Thinking, and Care in Denmark. Gender Place Culture, 1–24. doi: 10.1080/0966369X.
Batson C. D., Powell A. A 2003 "Altruism and Prosocial Behavior". In Millon T., Lerner M. J. (Eds.), Handbook of psychology: Personality and Social psychology John Wiley & Sons, Inc. Vol. 5, pp. 463–484) doi: 10.1002/0471264385.wei0519
Birtus M., Lăzăroiu G. (2021). The Neurobehavioral Economics of the COVID-19 Pandemic: Consumer Cognition, Perception, Sentiment, Choice, and Decision-Making. Anal. Metaphysics 20, 89–101. doi: 10.22381/AM2020216
Brécard D., Hlaimi B., Lucas S., Perraudeau Y., Salladarré F. (2009). Determinants of Demand for Green Products: An Application to Eco-Label Demand for Fish in Europe. Ecol. economics 69 (1), 115–125. doi: 10.1016/j.ecolecon.2009.07.017
Bronnmann J., Hoffmann J. (2018). Consumer Preferences for Farmed and Ecolabeled Turbot: A North German Perspective. Aquacult. Economics Manage. 22 (3), 342–361. doi: 10.1080/13657305.2018.1398788
Chen T., Guestrin C. (2016). “Xgboost: A Scalable Tree Boosting System,” in In Proceedings of the 22nd Acm Sigkdd International Conference on Knowledge Discovery and Data Mining, 785–794). Assotiation for Computing Machinery, New York. doi: 10.1145/2939672.2939785
De Dominicis S., Schultz P. W., Bonaiuto M. (2017). Protecting the Environment for Self-Interested Reasons: Altruism is Not the Only Pathway to Sustainability. Front. Psychol. 8, 1065. doi: 10.3389/fpsyg.2017.01065
Del Giudice T., Stranieri S., Caracciolo F., Ricci E. C., Cembalo L., Banterle A., et al. (2018). Corporate Social Responsibility Certifications Influence Consumer Preferences and Seafood Market Price. J. Cleaner Production 178, 526–533. doi: 10.1016/j.jclepro.2017.12.276
Dobson A. (2007). Environmental Citizenship: Towards Sustainable Development. Sustain. Dev. 15 (5), 276–285. doi: 10.1002/sd.344
Duffy B., Smith K., Terhanian G., Bremer J. (2005). Comparing Data From Online and Face-to-Face Surveys. Int. J. market Res. 47 (6), 615–639. doi: 10.1177/147078530504700602
EU (2013) Common Fisheries Policy. Available at: https://eur-lex.europa.eu/legal-content/EN/TXT/?uri=celex%3A32013R1380.
EU (2022) Common Market Organisation in Fishery and Aquaculture Products. Available at: https://www.europarl.europa.eu/factsheets/en/sheet/118/common-market-organisation-in-fishery-and-aquaculture-products.
EUMOFA (2021) The EU Fish Market. 2021 Edition. Available at: https://www.eumofa.eu/documents/20178/477018/EN_The+EU+fish+market_2021.pdf/27a6d912-a758-6065-c973-c1146ac93d30?t=1636964632989.
European Commission and European Parliament, Brussels (2021) Eurobarometer 95.1 (2021). GESIS Data Archive, Cologne. ZA7781 Data File Version 1.0.0. Available at: 10.4232/1.13791.
FAO (2018) Overview of Food Fraud in the Fisheries Sector. Available at: https://www.fao.org/documents/card/en/c/I8791EN/.
Galati A., Crescimanno M. (2012). The Atlantic Bluefin Tuna: Structure and Competitiveness of Italian Fishing in the International Trade. New Medit: Mediterr. J. Economics Agric. Environment= Rev. Meíditerraneíenne d′Economie Agric. Environ. 11 (1), 58.
Galati A., Miret-Pastor L., Crescimanno M., Giaimo R., Giacomarra M. (2015). Sustainable European Fishery and the Friend of the Sea Scheme: Tools to Achieve Sustainable Development in the Fishery Sector. Int. J. Globalisation Small Business 7 (3-4), 247–265. doi: 10.1504/IJGSB.2015.072695
Galati A., Miret-Pastor L., Siggia D., Crescimanno M., Fiore M. (2021). Determinants Affecting Consumers’ Attention to Fish Eco-Labels in Purchase Decisions: A Cross-Country Study. Br. Food J. doi: 10.1108/BFJ-05-2021-0498
Giacomarra M., Crescimanno M., Vrontis D., Miret-Pastor L., Galati A. (2021). “The Ability of Fish Ecolabels to Promote a Change in the Sustainability Awareness”. Mar. Policy 123, 104292. doi: 10.1016/j.marpol.2020.104292
Glogovețan A. I., Dabija D. C., Fiore M., Pocol C. B. (2022). “Consumer Perception and Understanding of European Union Quality Schemes: A Systematic Literature Review,”. Sustainability 2022 14, (1667), pp. 1–16 doi: 10.3390/su14031667
Grainger M. J., Aramyan L., Piras S., Quested T. E., Righi S., Setti M., et al. (2018). Model Selection and Averaging in the Assessment of the Drivers of Household Food Waste to Reduce the Probability of False Positives. PloS One 13 (2), e0192075. doi: 10.1371/journal.pone.0192075
Haghiri M. (2014). An Evaluation of Consumers’ Preferences for Certified Farmed Atlantic Salmon. Br. Food J. 16, (7), 092–1105. doi: 10.1108/BFJ-11-2012-0289
Hair J. F., Black W. C., Babin B. J., Anderson R. E., Tatham R. L. (2014). “Pearson New International Edition,” in Multivariate Data Analysis, Seventh Edition. Pearson Education Limited Harlow, Essex.
Hopkins E. (2022). Machine Learning Tools, Algorithms, and Techniques in Retail Business Operations: Consumer Perceptions, Expectations, and Habits. J. Self-Governance Manage. Economics 10 (1), 43–55. doi: 10.22381/jsme10120223
Jacquet J. L., Pauly D. (2007). The Rise of Seafood Awareness Campaigns in an Era of Collapsing Fisheries. Mar. Policy 31 (3), 308–313. doi: 10.1016/j.marpol.2006.09.003
Jonell M., Crona B., Brown K., R€onnb€ack P., Troell M. (2016). “Eco-Labeled Seafood: Determinants for (Blue) Green Consumption”. Sustainability 8 (9), 884. doi: 10.3390/su8090884
Kim B. T., Lee M. K. (2018). Consumer Preference for Eco-Labeled Seafood in Korea. Sustainability 10 (9), 3276. doi: 10.3390/su10093276
Kliestik T., Kovalova E., Lăzăroiu G. (2022). “Cognitive Decision-Making Algorithms in Data-Driven Retail Intelligence: Consumer Sentiments, Choices, and Shopping Behaviors,”. J. Self-Governance Manage. Economics 10 (1), 30–42. doi: 10.22381/jsme10120222
Kohavi R., John G. H. (1997). Wrappers for Feature Subset Selection. Artif. Intell. 97 (1-2), 273–324. doi: 10.1016/S0004-3702(97)00043-X
Kral P., Janoskova K., Lazaroiu G., Suler P. (2020). Impact of Selected Socio-Demographic Characteristics on Branded Product Preference in Consumer Markets. Manage. Marketing 15 (4), 570–586 doi: 10.2478/mmcks-2020-0033
Kursa M. B., Rudnicki W. R. (2010). Feature Selection With the Boruta Package. J. Stat. Software 36, 1–13. doi: 10.18637/jss.v036.i11
Lewis S. G., Boyle M. (2017). The Expanding Role of Traceability in Seafood: Tools and Key Initiatives. J. Food Sci. 82 (S1), A13–A21. doi: 10.1111/1750-3841.13743
Lundberg S. M., Erion G., Chen H., DeGrave A., Prutkin J. M., Nair B., et al. (2020). From Local Explanations to Global Understanding With Explainable AI for Trees. Nat. Mach. Intell. 2 (1), 56–67. doi: 10.1038/s42256-019-0138-9
Lundberg S. M., Lee S. I. (2017). A Unified Approach to Interpreting Model Predictions. Adv. Neural Inf. Process. Syst. 30. pp. 4768–4777
Malefors C., Secondi L., Marchetti S., Eriksson M. (2021). Food Waste Reduction and Economic Savings in Times of Crisis: The Potential of Machine Learning Methods to Plan Guest Attendance in Swedish Public Catering During the Covid-19 Pandemic. Socio-Economic Plann. Sci. 101041. doi: 10.1016/j.seps.2021.101041
Mariani S., Griffiths A. M., Velasco A., Kappel K., Jérôme M., Perez-Martin R. I., et al. (2015). Low Mislabeling Rates Indicate Marked Improvements in European Seafood Market Operations. Front. Ecol. Environ. 13 (10), 536–540. doi: 10.1890/150119
McClenachan L., Dissanayake S. T., Chen X. (2016). Fair Trade Fish: Consumer Support for Broader Seafood Sustainability. Fish Fish. 17 (3), 825–838. doi: 10.1111/faf.12148
Menozzi D., Nguyen T. T., Sogari G., Taskov D., Lucas S., Castro-Rial J. L. S., et al. (2020). Consumers’ Preferences and Willingness to Pay for Fish Products With Health and Environmental Labels: Evidence From Five European Countries. Nutrients 12 (9), 2650. doi: 10.3390/nu12092650
Mulazzani L., Piredda L., Cerjak M., Camanzi L. (2021). Consumer Appreciation of a Shark-Free Eco-Label for Small Pelagics. Br. Food J. 123 (13), 88–104. doi: 10.1108/BFJ-10-2020-0899
Murray G., Wolff K., Patterson M. (2017). Why Eat Fish? Factors Influencing Seafood Consumer Choices in British Columbia, Canada. Ocean Coast. Manage. 144, 16–22. doi: 10.1016/j.ocecoaman.2017.04.007
Nelson L. K., Bogeberg M., Cullen A., Koehn L. E., Strawn A., Levin P. S. (2022). Perspectives on Managing Fisheries for Community Wellbeing in the Face of Climate Change. Maritime Stud.1–20. doi: 10.1007/s40152-021-00252-z
Nica E., Sabie O.-M., Mascu S., Luțan (Petre) A. G. (2022). “Artificial Intelligence Decision-Making in Shopping Patterns: Consumer Values, Cognition, and Attitudes,”. Economics Management Financial Markets 17 (1), 31–43. doi: 10.22381/emfm17120222
Nilsson R., Pena J. M., Björkegren J., Tegnér J. (2007). Consistent Feature Selection for Pattern Recognition in Polynomial Time. J. Mach. Learn. Res. 8, 589–612.
Onozaka Y., Uchida H., Morita T., Managi S. (2010). “Uninformed or Uninterested? Surveys Examine Japanese Consumers’ Interest in Sustainable Seafood”. Global Aquacult. Advocate 1 (1), 58–60.
Panda T. K., Kumar A., Jakhar S., Luthra S., Garza-Reyes J. A., Kazancoglu I., et al. (2020). Social and Environmental Sustainability Model on Consumers’ Altruism, Green Purchase Intention, Green Brand Loyalty and Evangelism. J. Cleaner production 243, 118575. doi: 10.1016/j.jclepro.2019.118575
Paolacci S., Mendes R., Klapper R., Velasco A., Ramilo-Fernandez G., Muñoz-Colmenero M., et al. (2021). Labels on Seafood Products in Different European Countries and Their Compliance to EU Legislation. Mar. Policy 134, 104810. doi: 10.1016/j.marpol.2021.104810
Peiró-Signes Á., Segarra-Oña M., Trull-Domínguez Ó., Sánchez-Planelles J. (2022). Exposing the Ideal Combination of Endogenous–Exogenous Drivers for Companies’ Ecoinnovative Orientation: Results From Machine-Learning Methods. Socio-Economic Plann. Sci. 79, 101145. doi: 10.1016/j.seps.2021.101145
Penca J. (2020). Mainstreaming Sustainable Consumption of Seafood Through Enhanced Mandatory Food Labeling. Front. Mar. Sci. 7, 1084. doi: 10.3389/fmars.2020.598682
Pérez-Ramírez M., Almendarez-Hernández M. A., Avilés-Polanco G., Beltrán-Morales L. F. (2015). Consumer Acceptance of Eco-Labeled Fish: A Mexican Case Study. Sustainability 7 (4), 4625–4642. doi: 10.3390/su7044625
Pocol C. B., Marinescu V., Dabija D. C., Amuza A. (2021). Clustering Generation Z University Students Based on Daily Fruit and Vegetable Consumption: Empirical Research in an Emerging Market. Br. Food J. 123 (8), 2705–2727. doi: 10.1108/BFJ-10-2020-0900
Putler D. S. (1992). Incorporating Reference Price Effects Into a Theory of Consumer Choice. Marketing Sci. 11 (3), 287–309. doi: 10.1287/mksc.11.3.287
Rajagopal D. (2006). Measuring Asymmetric Consumer Behavior Towards Retail Buying: Application of Demand Equation Models. Monterrey Instititue of Technology. Marketing Business Working Paper 11/2006. doi: 10.2139/ssrn.942651
Rihn A., Wei X., Khachatryan H. (2019). Text vs. Logo: Does Eco-Label Format Influence Consumers’ Visual Attention and Willingness-to-Pay for Fruit Plants? An Experimental Auction Approach. J. Behav. Exp. Economics 82, 101452. doi: 10.1016/j.socec.2019.101452
Rydell L., Suler P. (2021). Underlying Values That Motivate Behavioral Intentions and Purchase Decisions: Lessons From the COVID-19 Pandemic. Anal. Metaphysics 20, 116–129. doi: 10.22381/AM2020218
Salladarré F., Guillotreau P., Perraudeau Y., Monfort M. C. (2010). The Demand for Seafood Eco-Labels in France. J. Agric. Food Ind. Organ. 8 (1), 1–24 doi: 10.2202/1542-0485.1308
Silva A. J., Hellberg R. S., Hanner R. H. (2021). “Seafood Fraud,” in Food Fraud ( Academic Press), 109–137. doi: 10.1016/B978-0-12-817242-1.00008-7
Stoppiglia H., Dreyfus G., Dubois R., Oussar Y. (2003). Ranking a Random Feature for Variable and Feature Selection. J. Mach. Learn. Res. 3, 1399–1414.
Strong J. T., Soydemir G., Petratos P. (2019). Asymmetric Impact of Advertising Revenues on Consumer Behavior: A Bivariate Approach. Cent. Eur. Business Rev. 8 (2). doi: 10.18267/j.cebr.212
Taufique K. M. R., Siwar C., Chamhuri N., Sarah F. H. (2016). Integrating General Environmental Knowledge and Eco-Label Knowledge in Understanding Ecologically Conscious Consumer Behavior. Proc. Economics Finance 37, 39–45. doi: 10.1016/S2212-5671(16)30090-9
Thøgersen J., Haugaard P., Olesen A. (2010). Consumer Responses to Ecolabels. Eur. J. marketing. doi: 10.1108/03090561011079882
Thøgersen J. 2017), Sustainable Food Consumption in the Nexus Between National Context and Private Lifestyle: A Multi-Level Study, Food Quality and Preference, 55, 16–25
Tinacci L., Guardone L., Rubio J. C. P., Riina M. V., Stratev D., Guidi A., et al (2019). Labelling Compliance and Species Identification of Herring Products Sold at Large Scale Retail Level Within the Italian Market. Food Control 106, 106707. doi: 10.1016/j.foodcont.2019.106707
Travaille K. L. T., Lindley J., Kendrick G. A., Crowder L. B., Clifton J. (2019). The Market for Sustainable Seafood Drives Transformative Change in Fishery Social-Ecological Systems. Global Environ. Change 57, 101919. doi: 10.1016/j.gloenvcha.2019.05.003
Uchida H., Onozaka Y., Morita T., Managi S. (2014). “Demand for Ecolabeled Seafood in the Japanese Market: A Conjoint Analysis of the Impact of Information and Interaction With Other Labels”. Food Policy 44, 68–76. doi: 10.1016/j.foodpol.2013.10.002
Verbeke W., Vanhonacker F., Sioen I., Van Camp J., De Henauw S. (2007). Perceived Importance of Sustainability and Ethics Related to Fish: A Consumer Behavior Perspective. Ambio 36, 580–585. doi: 10.1579/0044-7447(2007)36[580:PIOSAE]2.0.CO;2
Wang O., Somogyi S. (2019). Consumer Adoption of Sustainable Shellfish in China: Effects of Psychological Factors and Segmentation. J. Cleaner Production 206, 966–975. doi: 10.1016/j.jclepro.2018.09.189
Winson A., Choi J. Y., Hunter D., Ramsundar C. (2022). Ecolabeled Seafood and Sustainable Consumption in the Canadian Context: Issues and Insights From a Survey of Seafood Consumers. Maritime Stud. 21 (1), 99–113. doi: 10.1007/s40152-021-00245-y
Wolf J., Brown K., Conway D. (2009). Ecological Citizenship and Climate Change: Perceptions and Practice. Environ. Politics 18 (4), 503–521. doi: 10.1080/09644010903007377
Keywords: ecolabels, European market, fishery and aquaculture products, labels information, mandatory labels, seafood customers
Citation: Peiró-Signes A, Miret-Pastor L, Galati A and Segarra-Oña MV (2022) Consumer Demand for Environmental, Social, and Ethical Information in Fishery and Aquaculture Product Labels. Front. Mar. Sci. 9:948437. doi: 10.3389/fmars.2022.948437
Received: 19 May 2022; Accepted: 15 June 2022;
Published: 22 July 2022.
Edited by:
Dan-Cristian Dabija, Babeș-Bolyai University, RomaniaReviewed by:
Loveleen Gaur, Amity University, IndiaAurel Pera, University of Craiova, Romania
Luminita Ionescu, Spiru Haret University, Romania
Dyah Ismoyowati, Gadjah Mada University, Indonesia
Copyright © 2022 Peiró-Signes, Miret-Pastor, Galati and Segarra-Oña. This is an open-access article distributed under the terms of the Creative Commons Attribution License (CC BY). The use, distribution or reproduction in other forums is permitted, provided the original author(s) and the copyright owner(s) are credited and that the original publication in this journal is cited, in accordance with accepted academic practice. No use, distribution or reproduction is permitted which does not comply with these terms.
*Correspondence: Lluís Miret-Pastor, bHVpbWlwYXNAZXNwLnVwdi5lcw==