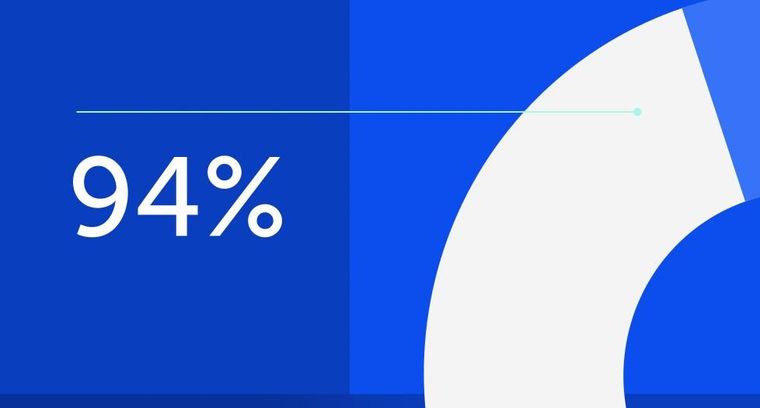
94% of researchers rate our articles as excellent or good
Learn more about the work of our research integrity team to safeguard the quality of each article we publish.
Find out more
ORIGINAL RESEARCH article
Front. Mar. Sci., 29 September 2022
Sec. Marine Ecosystem Ecology
Volume 9 - 2022 | https://doi.org/10.3389/fmars.2022.944851
This article is part of the Research TopicSharing Technical Knowledge to Understand the Distribution Patterns and Migration History of Marine OrganismsView all 11 articles
Seascape connectivity studies, informing the level of exchange of individuals between populations, can provide extremely valuable data for marine population biology and conservation strategy definition. Here we used a multidisciplinary approach to investigate the connectivity of the marbled crab (Pachygrapsus marmoratus), a high dispersal species, in the Adriatic and Ionian basins. A combination of genetic analyses (based on 15 microsatellites screened in 314 specimens), Lagrangian simulations (obtained with a biophysical model of larval dispersal) and individual-based forward-time simulations (incorporating species-specific fecundity and a wide range of population sizes) disclosed the realized and potential connectivity among eight different locations, including existing or planned Marine Protected Areas (MPAs). Overall, data indicated a general genetic homogeneity, after removing a single outlier locus potentially under directional selection. Lagrangian simulations showed that direct connections potentially exist between several sites, but most sites did not exchange larvae. Forward-time simulations indicated that a few generations of drift would produce detectable genetic differentiation in case of complete isolation as well as when considering the direct connections predicted by Lagrangian simulations.Overall, our results suggest that the observed genetic homogeneity reflects a high level of realized connectivity among sites, which might result from a regional metapopulation dynamics, rather than from direct exchange among populations of the existing or planned MPAs. Thus, in the Adriatic and Ionian basins, connectivity might be critically dependent on unsampled, unprotected, populations, even in species with very high dispersal potential like the marbled crab. Our study pointed out the pitfalls of using wide-dispersing species with broad habitat availability when assessing genetic connectivity among MPAs or areas deserving protection and prompts for the careful consideration of appropriate dispersing features, habitat suitability, reproductive timing and duration in the selection of informative species.
Seascape connectivity, i.e. connectivity among habitats in seascapes, informs about population interdependency and resilience, key to marine population biology and conservation strategy definition (Palumbi, 2003; Selkoe and Toonen, 2011; Olds et al., 2016). For most marine species, connectivity involves primarily dispersal of early developmental stages, planktonic larvae or propagules (Ward et al., 1994), which typically reach the open sea and are carried by currents to suitable habitats where they settle and develop into adults (Levin, 2006). As direct tracking of larvae is technically demanding (Cowen and Sponaugle, 2009), genetic data can be conveniently used to infer connectivity based on patterns of population differentiation (Palumbi, 2003). This approach, however, is particularly challenging when genetic differentiation is weak, as it occurs in many marine species, making it difficult to disentangle true genetic differentiation from background noise due to chance, experimental artefacts, and genetic patchiness (Hauser and Carvalho, 2008; Knutsen et al., 2011).
In this context, Lagrangian biophysical models have become increasingly popular, as they allow the modelling of larval dispersal, incorporating specific vital traits, and considering temporal variation in ocean currents (Gilg and Hilbish, 2003; Hohenlohe, 2004). The comparison of biophysical model outputs with genetic data can be effective to gain a better understanding of broad-scale genetic structure (Galindo et al., 2006; Galindo et al., 2010), to quantify the influence of dispersal on genetic differentiation, and to increase confidence in inferences when genetic differentiation is weak (Selkoe et al., 2010; Schiavina et al., 2014).
In the western Mediterranean Sea, Lagrangian simulations and genetic analyses have been first combined to infer directional gene flow in the comber Serranus cabrilla across the major oceanographic discontinuities of the Ibiza Channel and the Almeria-Oran front (Schunter et al., 2011). In the central Mediterranean Sea, a similar approach has been used for the white sea bream (Diplodus sargus) to demonstrate panmixia between a Marine Protected Area (MPA) of the southwestern Adriatic Sea and the neighbouring non-protected areas (Di Franco et al., 2012; Pujolar et al., 2013).
Coupled biophysical and genetic analyses concordantly showed high levels of connectivity between populations of the sea urchin Paracentrotus lividus (a keystone species of Mediterranean infralittoral rocky shores) in the Adriatic basin (Paterno et al., 2017) while, in the same region, both approaches indicated the presence of geographic subdivision and consequent population differentiation in the Mediterranean shore crab (Carcinus aestuarii), a global invader native to the Mediterranean Sea and inhabiting estuaries and lagoons (Schiavina et al., 2014). These different patterns of genetic structure in two species characterized by a long pelagic larval duration (about four weeks for the four crab pelagic zoeal stages: deRivera et al., 2007, Schiavina et al., 2014; about 30 days for the pelagic sea urchin larvae: Fenaux et al., 1985; Pedrotti, 1993; Paterno et al., 2017) suggest that other differences in biological and ecological traits or in evolutionary histories can play a key role in determining species-specific population genetic structures (Melià et al., 2016). Thus, further studies on species with long pelagic larval duration in the same area are needed.
The present work investigates the connectivity of the marbled crab Pachygrapsus marmoratus (Fabricius, 1787) (Brachyura: Grapsidae) in the Adriatic and Ionian seas, combining Lagrangian biophysical simulations and genetic microsatellite analyses. Considering the lack of habitat mapping information for this species, we assessed the direct connections among the locations sampled for genetics using Lagrangian simulations. In addition, we performed individual-based forward-time simulations to explicitly link the dispersal scenario estimated via Lagrangian simulations to the observed population genetics pattern.
The marbled crab is a species with a long larval duration and a high fecundity, thus similar to the Mediterranean shore crab C. aestuarii studied by Schiavina et al. (2014). However, it has a different habitat, being very common in the intertidal zone of rocky shores. P. marmoratus is a semiterrestrial crab occurring on upper and middle levels of rocky shores. It is widespread throughout the entire Mediterranean basin, in the Black Sea and along the eastern Atlantic coasts of Europe and Africa, including the Canary and Azores islands (Zariquiey Alvarez, 1968; Ingle, 1980). In the last decade, P. marmoratus expanded northward its Atlantic distribution range, as a consequence of the warming of sea surface waters (Dauvin, 2009). This species is also found in artificial habitats such as breakwaters, ports, and marinas, since it is tolerant to anthropogenic disturbance (Fratini et al., 2008; Baratti et al., 2022). Adults are relatively sedentary and faithful to a specific area (whose extent depends on the sex and size of the owner) that they actively defend from conspecific intrusion (Cannicci et al., 1999). Planktonic larval stages last approximately four weeks in the water column (Drake et al., 1998; Cuesta and Rodriguez, 2000) before settlement. P. marmoratus breeds from late spring to late summer depending on the geographic area (Vernet-Cornubert, 1958; Zariquiey Alvarez, 1968; Ingle, 1980), thus the settlement of megalopae has a peak between June and October (Cuesta and Rodriguez, 2000; Flores et al., 2002) and seems to follow a semilunar cycle, at least along the open Atlantic coast where tidal excursions are more marked than in the Mediterranean Sea (Flores et al., 2002). On a microgeographic scale, hydrological features operating in coastal areas (i.e., the magnitude of the across-shore wind component and of the onshore currents) are among the main factors affecting settlement, while oceanographic processes are involved on a meso- and macrogeographic scale (Flores et al., 2002).
Previous genetic studies based on microsatellite polymorphisms (Fratini et al., 2008; Silva et al., 2009; Fratini et al., 2011; Fratini et al., 2013; Deli et al., 2016; Iannucci et al., 2020; Çetin et al., 2022) and mtDNA sequencing (Fratini et al., 2011; Kalkan et al., 2013; Deli et al., 2016; Fratini et al., 2016; Çetin et al., 2022) revealed weak but significant genetic heterogeneity among marbled crab populations in different areas including the Atlantic Ocean, the Black Sea and several Mediterranean Sea regions. In addition, former microsatellite studies, based on population samples separated by a few hundred kilometres, indicated that deviation from panmixia might occur at a small geographic scale, albeit the lack of geographically coherent differentiation suggests the existence of genetic patchiness.
This study aims at understanding the genetic structure of P. marmoratus among eight different locations spanning the central and the southern Adriatic Sea and the northern Ionian basins and including existing or planned MPAs. The study area has been selected for a Mediterranean Pilot Study in the framework of the CoCoNET FP7 project to identify groups of putatively interconnected MPAs, pointing out the importance of MPA networks instead of local single MPAs for conservation approaches (Boero et al., 2016). The inclusion of sampling sites along both the eastern and western coasts of the Adriatic and Ionian seas is of particular interest because both sides of the two basins include existing or planned MPAs. It is thus important to understand if they can act as an MPA network, using the marbled crab as a proxy for species with high dispersal potential. Using a multidisciplinary approach that combines microsatellite genetic analysis with Lagrangian simulations of dispersal and individual-based forward-time simulations, we: a) assessed nuclear genetic structure among P. marmoratus populations; b) estimated potential direct larval connectivity and retention at the sites used for genetics, with a biophysical model incorporating key vital traits affecting larval dispersal; c) investigated the genetic outcomes of different genetic drift-migration scenarios, with models incorporating species-specific fecundity and a wide range of population sizes; d) compared genetics and modelling results to elucidate and interpret the observed patterns of connectivity. In addition to these main goals, in this study we report 11 new microsatellite loci useful for population genetics studies of this widely investigated species.
Three hundred and fourteen samples of Pachygrapsus marmoratus were collected in 2013 and 2014 at eight different sites in the Adriatic and Ionian seas (Figure 1 and Table 1). All the locations, except Boka Kotorska (Montenegro) and Ammoudia (Greece), host a MPA or are reference areas for the establishment of a new MPA (Otranto, Italy). At each location, adult crabs were caught by hand from 30 cm above to 30 cm below the average sea level, and a cheliped or a pereiopod was removed from each individual and preserved in absolute ethanol. Genomic DNA was extracted using a standard salting out protocol (Patwary et al., 1994) and stored at –20°C before PCR amplifications.
Figure 1 Sampling locations of Pachygrapsus marmoratus. See Table 1 for location acronyms.
Fifteen polymorphic microsatellite loci specific for P. marmoratus were used for genetic analysis. Four of these loci (pm79, pm108, pm183, pm187) were previously isolated by Fratini et al. (2006), whereas eleven additional microsatellite loci (pmar01, pmar08, pmar10, pmar12, pmar13, pmar14, pmar15, pmar16, pmar17, pmar19, pmar20) were obtained ex novo, by an external service (http://www.genoscreen.fr), through a 454 GS-FLX Titanium pyrosequencing of enriched DNA libraries as described in Malausa et al. (2011). Forward primers of all 15 loci were labelled with fluorescent dyes for detection with ABI PRISM automatic sequencer and amplified together in two separate multiplex reactions. Table S1 (Supporting Information) reports details on all the microsatellite loci used in this study. PCRs were carried out in 10 μl total volume containing: 1X QIAGEN Multiple PCR Master mix (QIAGEN, HotStarTaq DNA Polymerase, Multiplex PCR Buffer, dNTP Mix), 0.2 μM primer mix and 100 ng of DNA template (diluted from DNA extractions). PCR conditions were as follows: an initial denaturation/activation step (95°C, 15 min) was followed by 35 cycles of denaturation (94°C, 30 s), annealing (61°C, 90 s) and extension (72°C, 60 s), and by a final elongation step (60°C, for 30 min). Fragment analysis was performed by an external service (http://www.bmr-genomics.it) on an ABI 3100 automated sequencer, and PCR products were sized with the internal standard LIZ500 (Applied Biosystems). To ensure reproducibility of results and minimize differences due to capillary electrophoresis, 10–15% of all specimens were re-genotyped and re-scored. Scoring was performed with PEAK SCANNER ver. 1.0 (Applied Biosystems). To minimize the negative consequences of poor allele calling, binning was automated with FLEXIBIN ver. 2 (Amos et al., 2007), which implements a method that does not assume collinearity between expected and measured fragment length. The scoring was manually checked and analysed with MICROCHECKER 2.2.3 (van Oosterhout et al., 2004) that allows detection of null alleles and estimation of their allelic frequency.
To detect outlier loci putatively under selection, whose inclusion in the analysis might distort genetic connectivity estimates, we applied two neutrality tests relying on the expectation that divergent selection should increase the observed level of genetic differentiation between populations. First, the FST‐outlier method implemented in LOSITAN (Antao et al., 2008), was run with the following settings: 1000000 simulations, stepwise mutation model (SMM), neutral FST and forced mean FST. Comparisons were performed first among all population samples and then between pairs of population. The second method, implemented in the R package DETSEL (Vitalis et al., 2003; Vitalis, 2012; R Team Core, 2015), relies on a model where a common ancestor population splits up into two populations, which afterward diverge only by random drift after a possible bottleneck event. In this case 500000 coalescent simulations were performed with a SMM mutation rate equal to 5×10-4 (Estoup and Angers, 1998) and, for nuisance parameters, ancestral population size varying from 500 to 10000 effective individuals, population size before the split from 0 to 500, number of generations since divergence from 50 to 100 generations, and time since the bottleneck varying from 0 to 200 generations. As suggested by the authors, because of the uncertainty in the nuisance parameter values, it is recommended to perform simulations using different combinations of values for the ancestral population size, divergence time and bottleneck parameters (Vitalis, 2012), that should correspond to the user knowledge of the biological model.
Using both tests, all loci falling outside the resulting 99% probability region under neutral expectations were considered as potentially under selection and excluded from further analysis.
Number of alleles (NA), observed (HO) and unbiased expected (HE) heterozygosity were estimated, using GENETIX ver. 4.05.2 (Belkir et al., 2005); allelic richness (AR) was computed with FSTAT 2.9.3.2 (Goudet, 2001) based on the smallest sample size across all population samples. We also tested for deviation from Hardy-Weinberg equilibrium (HWE) at each locus, and for linkage disequilibrium between pairs of loci using GENEPOP ver. online 4.2 (Raymond and Rousset, 1995; Rousset, 2008). Significance levels of HWE and linkage equilibrium were determined via the Markov Chain method (dememorizations = 10000, batches = 500, iterations = 10000); significance for tests involving multiple comparisons was obtained using the Benjamini-Hochberg correction (Benjamini and Hochberg, 1995).
Genetic differentiation was investigated using ARLEQUIN 3.5 (Excoffier and Lischer, 2010) by analysis of molecular variance (AMOVA; Excoffier et al., 1992) and pairwise FST estimation following Weir and Cockerham (1984). Significance of differentiation was determined using 10000 random permutations of individuals among populations. As in the case of HW and linkage equilibrium tests, the Benjamini-Hochberg correction (Benjamini and Hochberg, 1995) was applied in case of multiple tests.
Since FST distance has been criticized because it can underestimate genetic differentiation in the case of high mutation rates (Hedrick, 2005; Wang, 2015), we also calculated the unbiased estimator of Jost’s D, Dest (Jost, 2008), using the DIVERSITY package (Keenan et al., 2013). Dest considers the allele distribution among populations, and it is independent from the average within-population heterozygosity (Jost, 2008). In this case, the significance of pairwise comparisons was assessed by 1000 bootstrap replicates.
We also applied the Bayesian clustering approach implemented in STRUCTURE (Pritchard et al., 2000) and the discriminant analysis of principal components (DAPC) implemented in ADEGENET for R 3.2.3. (Jombart, 2008). Since both analyses identified a single population in the area, results are not further reported.
Potential connectivity of P. marmoratus among the eight sampling locations was investigated via Lagrangian simulations. Since basin-wide habitat mapping is not currently available for P. marmoratus, we conservatively assessed only the direct connections among the limited number of locations used for genetics. Considering that the species is very common in the study area, the inclusion of other release points would allow in the future a thorough and more realistic investigation of connectivity across the whole Adriatic–Ionian basin. At present, considering that sampling sites were representative of the existing MPA in the southern Adriatic and the northern Ionian seas, our results can provide conservative indications about the existence of a potential network of protected sites. The existence of additional unmodelled (and unprotected) P. marmoratus populations in suitable rocky shore habitats scattered across the surveyed area is expected to further increase the potential for dispersal among sampled locations through stepwise processes.
Daily average fields of current velocity produced by the Adriatic Forecasting System provided the physical engine of the model. The ocean circulation dataset (produced by the AREG model; Oddo et al., 2006; Guarnieri et al., 2010) covers the whole Adriatic basin and extends into the Ionian Sea down to the 39° N parallel, and it has a regular horizontal grid with a resolution of 1/45° (about 2.2 km) and 31 vertical sigma layers. Bathymetry has a horizontal resolution of 1/60°, and the coastline is set at the 10-m isobath.
Lagrangian particles, representing individual zoeae (larvae), were released from the eight sampling locations. Simulations were run over the period 2006–2013 to cover the temporal range in which sampled individuals were likely born (assuming an age at capture between 0 and 8 years). The release time was set to fit the available information regarding the duration of the zoea stage (1 month: Drake et al., 1998) and the subsequent settlement of P. marmoratus megalopae (which peaks between June and October; Cuesta and Rodriguez, 2000; Flores et al., 2002). Therefore, releases were randomly distributed in time with a Gaussian distribution centred in mid-July and with 95% confidence bounds extending from May to September to match the subsequent settlement in June-October. We described larval release as a continuous random process since different release settings can strongly affect the results of the simulations (Pires et al., 2020), and because larval release patterns recorded for P. marmoratus, differently from most brachyurans studied so far, did not show a strong synchronization with lunar phases and were rather continuous over the lunar month (Flores and Paula, 2002a).
At each spawning event, Lagrangian particles (625,000 per site per year) were released according to a 2-D Gaussian distribution within a 1-km radius from each site, with a uniform distribution between 0.5 and 1 m depth along the vertical direction (to mimic the neustonic habit of P. marmoratus larvae: Flores et al., 2002; dos Santos et al., 2008). Trajectories were stepped forward at a fixed depth via an explicit 4th-order Runge-Kutta integration method with a 6-minute time step. To account for the (unknown) duration of the larval competency period, as well as for the swimming and navigation ability of megalopae (which take advantage of tidal stream transport to reach settlement areas; Flores et al., 2002), larvae were considered to successfully colonize the destination location if their position at the end of the pelagic phase fell within a circular buffer with a 10-km radius from each of the eight sampling sites.
Connectivity intensity (sensu Melià et al., 2016) was calculated as the percentage of particles released from a location and successfully reaching another one, averaged over the whole simulation period. Connectivity persistence (measuring the continuity of the flux throughout the years, sensu Melià et al., 2016) was defined as the stabilization coefficient, which is the reciprocal of the coefficient of variation of connectivity intensity.
To establish the number of generations and the amount of gene flow needed to obtain an FST equal to or greater than that observed with the empirical dataset, we carried out individual-based forward-time simulations with SIMUPOP (Peng and Kimmel, 2005).
For each simulation, we modelled eight populations, one for each sampling site. All the populations were of equal size, reflecting the lack of significant differences at genetic variability statistics (see Results section), with no overlapping generations. This latter assumption was justified by the difficulties associated with aging crabs (Kilada and Driscoll, 2017) and the subsequent limited information about age structure in this species (Arab et al., 2015), though size-frequency distributions of Pachygrapsus marmoratus clearly indicates that several cohorts co-occur in the same population (Flores and Paula, 2002b). Due to this assumption, the correlation of allelic frequencies between generations in our simulated populations is smaller than the one expected in age structured populations (Jorde and Ryman, 1995), namely in the real ones. Individual genotypes were generated for 14 loci (after removing an outlier locus, see Results section) from the overall microsatellite allele frequencies of the Adriatic–Ionian samples and sex was assigned randomly according to a 1:1 sex ratio. A SMM with a rate of 5 × 10-4 mutations per generation was assumed (Estoup and Angers, 1998). Individuals mated randomly within each population with the number of matings arbitrarily fixed to half of the population size for computational reasons. Each mating produced a fixed number of 22402 larvae, according to the average fecundity reported for this species (Arab et al., 2015) and assuming no sperm limitation. After reproduction, the larvae of each population were re-allocated according to local recruitment estimates and to specific scenarios of isolation or migration. After allocation, local larval pools were resized to the original local population dimension, assuming a density dependence acting in a constant and homogeneous way at all the sites, to produce the next generation population. The process was repeated for 160 generations, which was estimated from pilot runs to produce well-defined patterns of differentiation, and population genotypes were saved in SIMUPOP format at generations 0, 10, 20, 30, 40, 80, 160. Then, we used a custom python script to randomly subsample individual genotypes from each simulated population, matching the original sample sizes of the empirical dataset, and to generate the appropriate file formats for subsequent analysis. We used ARLEQUIN and the R package DIVERSITY, as for the empirical dataset, to calculate from simulated data the global FST and the pairwise FST together with their significance, and the Jost’s D and its confidence intervals.
We tested two scenarios of population size with isolation and migration. To obtain the range of simulated populations sizes that is relevant for our dataset, an aspect that is often neglected in forward time individual based simulations (and obscure due to the non ideality of simulated populations), we proceeded as follows. We first estimated effective population sizes (Ne) from our empirical genetic data using different approaches including a SMM heterozygosity-based method (Ohta and Kimura, 1973; Papetti et al., 2009), Bayesian inference with MIGRATE (Beerli and Palczewski, 2010), and the LD method in NeEstimator (Do et al., 2014). Details on these methodologies are reported in Table S2. We then defined a reasonable range of Ne to be covered by the simulations, conservatively adding extreme values to take into account the uncertainty associated with Ne estimation by genetic methods, and we finally determined the simulated population sizes (N) needed to cover this range. To this end, we performed several pilot runs with SIMUPOP, using the “lineage” module of the package, which allows measuring the true Ne of simulated populations of size N, by tracing directly alleles across generations, and we adjusted the simulated population sizes until obtaining the desired true effective population sizes. At the end of this exercise, Ne ranging from 500 to 20000 were tested, corresponding to simulated local population sizes ranging from 125 and 5000 individuals.
For each population size, the simulations were first run under a pure drift model (0 migrants per generation) and the number of generations of divergence needed to reach and exceed the overall FST observed in the empirical dataset was determined. To this end, as mentioned before, the simulated data were exported at predefined numbers of generations, subsampled, converted to ARLEQUIN format, and used to calculate the overall FST observed in simulations at generations 0, 10, 20, 30, 40, 80, and 160. For the same number of generations, we calculated pairwise FST and Jost’s D to allow comparison of the simulated and empirical pattern of genetic differentiation. The same procedure was used to investigate the outcome of simulations performed under the scenario of migration outlined by Lagrangian simulations, with larvae allocated to sites according to the estimated connectivity intensity.
Both LOSITAN and DETSEL simulations identified locus pm108 as the only outlier (Figure S1). In LOSITAN, this locus showed a higher-than-expected FST both globally and in all pairwise comparisons involving Greece (AMM) and Croatia (KOR) populations. This locus fell outside the upper 99% CI of the FST versus HE expected distribution (P-value(Simul Fst < Sample Fst) = 1). In the analysis with DETSEL, locus pm108 consistently laid outside the 99% probability region of population-specific parameters F1 and F2, whereas loci pm79, pm183 and pm187 were detected as outliers only in few comparisons. Based on these results and considering that the aim of this study is to investigate connectivity among locations, the analyses were performed on the 14 “neutral” loci after removal of pm108.
The analysis of 14 microsatellite loci on 314 specimens of P. marmoratus revealed relatively high molecular variability in the Adriatic and Ionian seas. All microsatellites were polymorphic with a minimum of three alleles per locus (pm79) and a maximum of 28 (pm187). Observed and expected heterozygosity ranged from 0.3706 (locus pmar16) to 0.8646 (locus pmar12) and from 0.4077 (locus pmar16) to 0.8981 (locus pmar12), respectively. In general, an excess of homozygotes was found (Table 2). At the population level, observed and expected heterozygosity ranged from 0.5688 in Torre Guaceto (TOG) to 0.6598 in Croatia (KOR) and from 0.6508 in Tremiti Islands (TRE) to 0.6931 in Montenegro (BOK), respectively (Table 3). The average allelic richness, based on a minimum sample size of N = 16, was similar in all populations, ranging from 5.9298 in TRE to 6.5959 in BOK. Probability tests of HWE were conducted for each of the 14 loci and for each of the eight sites. Fourteen out of 112 tests showed a significant departure from HWE, with a nominal significance threshold of 0.05 corrected for multiple tests. Departures were distributed almost evenly across loci, although 11 significant tests involved loci pm183, pmar17 and pmar19. For these loci, MICROCHECKER suggested the presence of null alleles. After correction for null alleles with MICROCHECKER, all tests involving these three loci were still significant; for this reason, all the subsequent analyses were performed considering both uncorrected data and data corrected for the presence of null alleles and the results were cross-checked. However, since the correction did not affect the final results, only results for the original data are reported. Overall, tests for linkage disequilibrium among all loci showed no significant departure from expected values.
Table 3 Summary of genetic variability estimates across sampling locations averaged over all 14 microsatellite loci.
Overall, 99.6% of the total genetic variation was within individuals; correspondingly, an extremely small, yet significant, FST value was detected (global FST = 0.0041, P < 0.01). Pairwise comparisons based on the analysis of neutral loci showed a general homogeneity among sites with fixation indices ranging from negative values to a maximum of 0.0111, none of them significant after Benjamini-Hochberg correction for multiple tests (Table 4). The few comparisons associated with P-values below the uncorrected 0.05 threshold involved the Greek (AMM) and Porto Cesareo (POC) samples but did not show a spatial genetic pattern (Table 4). Similar results were obtained with Jost’s D. The global Dest estimate was as small as 0.0017 (95% CI: -0.0092 – 0.0152). Dest between sampling locations ranged from –0.0022 to 0.0062 and all pairwise comparisons had a negative lower 95% CI boundary, which indicates lack of significant differentiation among samples (Table 5).
Table 5 Pachygrapsus marmoratus pairwise genetic differentiation measured as Jost’s D based on 14 neutral loci.
Figures 2, 3 summarize the results of the biophysical simulations and show the trajectories followed by Lagrangian particles across the study area and the links between the study sites over the simulation period (2006–2013). Estimates of connectivity intensity among the eight locations and the corresponding connectivity persistence are shown in Tables 6 and 7, respectively. Estimated local retention was maximum in TOG (94%), KAP (40%) and BOK (30%), while it was lower in AMM, KOR and POC (between 10 and 20%) and negligible in TRE and OTR (Table 6). The intensity of estimated connections between sampling sites was, on average, two orders of magnitude lower than that of local retention (Table 6). “Alongshore” potential connections were highest in the western side of the basin (Figure 3A), with connectivity intensity between TRE, TOG and OTR ranging from 0.202 to 1.458% (Table 6), and a directional exchange orientated from north to south in the Adriatic and extending further along the Italian coastline in the Ionian (connectivity intensity range with POC: 0.008 – 0.293%, Table 6). In contrast, potential connections along the eastern shore of the basin were much weaker (connectivity intensity range: <0.001 – 0.022%) and restricted to neighbouring populations (Table 6). On the other hand, East to West connections were found, potentially linking the two sides of the Adriatic Sea (Figure 3A). The most intense connected Montenegro (BOK) with Apulia (especially TOG and OTR; connectivity intensity range: 1.265 – 1.573), as reported in Table 6. The Tremiti Islands (TRE) was one of the most connected sites, exchanging propagules with KOR (along a bidirectional link) and potentially providing a recruitment subsidy to the remaining Adriatic Apulian sites (especially TOG and OTR), with minor connections towards POC and BOK. The Greek site (AMM) was almost isolated from the others, with no incoming connections and only minor outgoing connections with Albania (KAP) and southern Apulia (POC, OTR). In terms of persistence, the most stable connections were those linking KOR and TRE, as well as those driven by the coastal current linking Apulian sites from north to south (Table 7 and Figure 3B).
Figure 2 Larval dispersal resulting from Lagrangian simulation. Coloured circles indicate the locations of larval release (black: AMM; violet: KAP; blue: BOK; red: KOR; orange: TRE; green: TOG; yellow: OTR; cyan: POC). Each dot represents the arrival location of a particle released from each site over the time horizon of the simulation (2006–2013).
Figure 3 (A) Connectivity intensity graph for Pachygrapsus marmoratus. Connectivity intensity (estimated via Lagrangian simulation) is measured as the percentage (averaged across the simulation period) of larvae successfully moving from a site of origin to the destination sites. Loops (i.e. edges connecting a location with itself) indicate local retention. (B) Connectivity persistence graph for Pachygrapsus marmoratus. Connectivity persistence is defined as the stabilization coefficient (i.e. the reciprocal of the coefficient of variation) of the average annual flux of larvae successfully moving from a site of origin to the destination sites, calculated over the simulation period.
Individual-based forward-time simulations were performed to investigate if the observed patterns of genetic differentiation are compatible with different scenarios of isolation and migration. In order to set up simulations, global effective population size (Ne) was first estimated from our marbled crab empirical dataset using different methods, resulting in values ranging from about 600 to 2700 individuals (Table S2). When performing SIMUPOP simulations and calculating global effective population size by directly tracing alleles across generations in SIMUPOP (see Materials and methods section), these Ne values are obtained when the simulated local population sizes (N) are set to 150 – 675 individuals, considering 8 populations and panmixia (see Nunney, 1999 for the influence of inbreeding and genetic structure). Taking into account the many assumptions behind Ne estimations from genetic data, we included a wider range of local population sizes in SIMUPOP, ranging from 125 to 5000 individuals (Tables S3, S4).
SIMUPOP forward-time simulations revealed that in the case of complete isolation under pure drift, the FST values observed among Adriatic–Ionian population samples are reached and exceeded in few generations. Even when considering the biggest local population size tested (N = 5000), 30 generations of drift were sufficient to produce FST values higher than the 0.0041 of the empirical dataset (Table S3). Importantly, under this scenario, a large fraction of the pairwise comparisons of genetic differentiation (FST) becomes quickly significant after correction for multiple tests, thus contrasting the non-significant values observed for the empirical dataset (Table 4). Jost’s D exhibited a somewhat lower power to detect differences, but still showed some confidence intervals excluding zero, differently from the original dataset (Table 5), starting from 40 generations of drift. This difference between the dataset simulated in case of isolation and the empirical dataset is even clearer when considering the smallest population sizes (N = 125 – 250), for which 10 generations of drift allow to reach complete significance with all the statistics.
The pattern remains similar when considering simulations incorporating Lagrangian estimates of dispersal (Table S4). A reduction of FST values in simulated samples is observed with respect to the case of isolation. However, also in this case all the genetic differentiation statistics increased monotonically, quickly exceeding the values observed in the empirical dataset. For instance, with all the local population sizes tested, 30 generations already produced significant pairwise FST (minimum 6 comparisons when N = 5000), not observed with the original dataset. As before, Jost’s D were slower to reach significance, but still showed confidence intervals excluding 0 starting from 10 – 80 generations, depending on local population size. In summary, the level of direct migration estimated by Lagrangian simulations was too low to explain the lack of genetic differentiation observed in the original dataset: the observed differentiation statistics were exceeded in few generations in the individual-based simulated dataset.
This study, examining eight different locations spanning the central and the southern Adriatic Sea and the northern Ionian basins including existing or planned MPAs, unearthed the seascape connectivity of P. marmoratus, furthering our understanding of the impact of ocean currents on larval dispersal and genetic structure of this species and thus providing crucial information for conservation strategy definition.
The study focused on marbled crab, that has been selected as a model species with long larval duration. Nevertheless, the study significance goes beyond this target species, and its outcomes contribute to the general framework of larval dispersion and connectivity modelling key to marine area management. Our results suggest the existence of a genetically homogeneous population of marbled crab in the study area, pointing out a high level of exchange, but the direct connections between several sites highlighted by Lagrangian simulations did not completely explain how genetic homogeneity is maintained, according to the results of individual-based forward-time simulations. Noticeably, we identified a single outlier locus potentially under selection, whose inclusion in the analysis would produce a different picture, largely inflating genetic differentiation estimates. Collectively, our results indicate a high realized connectivity in the study area, which however, even for this species characterized by a relatively long larval duration, is not sustained by direct exchange between the existing and planned MPAs considered in this study.
When excluding the outlier locus pm108, no significant pairwise FST nor Jost’s D comparisons were found. However, an extremely small, though still significant, global FST was detected, due to five FST pairwise comparisons with no clear spatial clustering.
Individual-based forward-time simulations clearly showed that this pattern is not expected in the case of isolation, where all the genetic differentiation statistics are expected to increase sharply and to quickly reach significance in few generations under a wide range of simulated population sizes. Importantly, strong genetic differentiation should arise under isolation after less than 10 generations when considering the smallest local population sizes, likely the most appropriate for the marbled crab, whereas a pattern similar to that observed in the empirical dataset is obtained for small population sizes at generation 0, i.e. by instantaneous drift due to sampling from a homogeneous gene pool.
Lagrangian simulations highlighted the existence of potential connections among sites, strongest for a group of sites in the southern Adriatic Sea and in the Italian portion of the Ionian Sea (KAP, BOK, TOG, OTR, POC), which were only partially connected to the Greek Ionian site (AMM) and to the two northernmost Adriatic populations (KOR and TRE). However, individual-based forward-time simulations clearly indicated that direct connections are not strong enough to maintain genetic homogeneity, and that, considering them alone, would produce a very mild effect compared with pure isolation. Importantly, a close inspection of simulation results showed that genetic homogeneity is not achieved even for the strongest potential connections (i.e. TRE to TOG and BOK to OTR, range intensity 1.458 – 1.573%). In fact, the few comparisons not achieving significance at the end of our simulations (1/28 to 4/28 depending on the simulated population size, Table S4) involved consistently only the TRE-KOR pair of sites, which was apparently characterized by a low potential recruitment at TRE (0.104%) rather than a higher exchange of larvae (unidirectional 0.775% from KOR to TRE).
This result has potential conservation implications, suggesting that direct connections among existing and planned MPA are not sufficient to maintain genetic homogeneity, and deserve further consideration.
The results of our Lagrangian simulations might have underestimated potential connectivity due to limits in our model. For instance, the two Ionian sites (AMM and POC) are very close to the border of the model domain, so edge effects may have negatively affected estimates of their connectivity strength. In fact, larvae moving south of 39°N and falling outside the oceanographic domain considered in our model are indeed lost. In addition, the oceanographic engine is likely unable to describe hydrodynamic processes acting at a very fine scale along the coast. Moreover, disregarding the information on key behavioural features of P. marmoratus larvae (e.g., vertical migration and distribution and degree of active swimming) might have contributed to the underestimation of connectivity among sites.
Besides, the modelled potential connectivity could itself not translate into realized dispersal for a variety of reasons. For instance, we modelled arrival to a wide area (circular buffer with 10-km radius from destination) in a precise day corresponding to the end of the specified larval duration. Especially for long distances, such as those separating many of our sites, this assumption excludes the possibility of settlement before the end of the pelagic larval duration or adaptations to limit dispersal when favourable settlement habitats are found. Finally, we did not include mortality and predation, which can still reduce success of individual larvae in reaching distant places.
For all these reasons, we believe that the result of no direct connection should be considered conservative and that a high level of gene flow, much higher than that predicted by our simulations, is needed to maintain genetic homogeneity. This aspect deserves further attention, and, in particular, the inclusion of other aspects in the individual-based forward-time simulations, such as variability in fecundity and recruitment, a more skewed reproductive success, age structure, directionality in migration, is expected to add details and increase the level of connectivity needed to maintain genetic homogeneity.
The marbled crab is very common, typically occurring in habitats including rocks, crevices and cleavages, as well as artificial substrates such as breakwaters, pier piles and other (Edwards, 2005). Thus, considering the prevalence of rocky habitats along the coasts of our study area, many additional intermediate populations exist and could act as steppingstones between the sites at which genetic sampling and modelling was performed, strongly increasing the level of realized connectivity. Thus, the addition of other intermediate sites in the analysis should be considered for further detailed analysis.
Integrating results of population genetics and Lagrangian connectivity, a general mixing in the area of study is evident for the marbled crab. Compared with previous studies on rocky shore species, our findings are in line with those obtained for Diplodus sargus in the southwestern Adriatic Sea (Di Franco et al., 2012; Pujolar et al., 2013). For this species, genetic homogeneity was observed at the local scale of Torre Guaceto and surrounding non-protected areas extending 100 km north and south of the MPA (Di Franco et al., 2012), and biophysical models suggested a potential dispersal extending up to the Ionian Sea (Pujolar et al., 2013). Our data confirm the connection of the western portion of South Adriatic and Ionian seas but extend the scale at which mixing occurs to the eastern side of the two basins. In the case of the black scorpionfish (Scorpaena porcus), a benthic coastal species inhabiting the same region, and the Mediterranean mussel (Mytilus galloprovincialis), a sessile filter-feeder with pelagic larvae very common in the Mediterranean and Black seas, a pattern of genetic differentiation slightly different from that of the marbled crab was observed (Boissin et al., 2016; Paterno, et al., 2019), with a clear break between the eastern and western part of the Adriatic basin indicated by genetic data. This is not in line with the results of Lagrangian simulations produced by Melià et al. (2016) for the black scorpionfish, which suggested that the eastern side of the Adriatic Sea can act as a potential source of propagules directed toward Apulia. On the other hand, genetic homogeneity among black scorpionfish samples from the western Adriatic and Ionian seas (Boissin et al., 2016) is consistent with the view of a strong potential connectivity within this part of the basin (Melià et al., 2016), in agreement with the results obtained for the marbled crab.
A high genetic connectivity along the western Adriatic and Ionian coasts was also observed in the estuarine and lagoon species Atherina boyeri (Congiu et al., 2002; Astolfi et al., 2005) and Flexopecten glaber (Pujolar et al., 2010). Genetic homogeneity and high potential connectivity between southwestern Adriatic and northern Ionian seas has also been found in the Mediterranean mussel (Paterno et al., 2019) and in the Mediterranean shore crab Carcinus aestuarii, another species inhabiting estuaries and lagoons (Schiavina et al., 2014). In the shore crab, however, a clear subdivision between northern, central, and southern Adriatic Sea was found both with genetic markers and biophysical models. In contrast, genetic homogeneity and potential dispersal in P. marmoratus were found to extend also to the two northernmost samples, in the central Adriatic (TRE and KOR). While Schiavina et al. (2014) did not include any sample geographically close to KOR, their sample from the Lesina lagoon is approximately at the same latitude of our western Adriatic sample (TRE). The power to detect differences is also similar in the two studies (statistical power to detect an FST as low as 0.0025 was 100% and > 95% in C. aestuarii and P. marmoratus, respectively). However, in C. aestuarii the sample from Lesina was genetically differentiated from the closest South Adriatic sample (FST = 0.0030, P = 0.035; Lagrangian larval connectivity intensity = 0.001; Schiavina et al., 2014), in contrast with the TRE sample of P. marmoratus, which was homogeneous with the closest South Adriatic sample (TRE-TOG: FST = –0.0026, P = 0.937; Lagrangian larval connectivity intensity = 1.458; present study). This difference warns from generalizing results to different species/habitats in the absence of specific population genetic data and Lagrangian simulations suitably tailored to the specific dispersing traits of each species (as pointed out also by Melià et al., 2016 based on their analysis, conducted at the community level, in the same study area). The distinct genetic structures found for C. aestuarii and P. marmoratus could be related to a slightly different current pattern along and off the shore and can be further explained by the different habitat availability for the two species. In fact, the southwestern Adriatic coast is mostly rocky, with very few lagoons, likely determining a different geographical arrangement of sites acting as possible steppingstones with distinct effects on the connectivity of the two species. Thus, while a steppingstone effect would strongly increase connectivity for a rocky shore species such as the marbled crab, the same effect would be weaker (at least in this area) for a species inhabiting estuaries and lagoons such as the Mediterranean shore crab.
Interestingly, our genetic results identified one locus (pm108) as an outlier potentially subject to directional selection. Indeed, this locus revealed a much higher genetic divergence than the remaining 14 loci analysed, despite being a Type II marker, randomly isolated from genomic DNA, and not showing (at the time of the present study) significant BLAST matches with the NCBI nucleotide database.
This pattern contrasts with the results emerging from biophysical simulations and provides an indication about the utility of cross comparing the outcomes of genetic analyses and Lagrangian simulations. This is especially true when genetic differences are very small, and it is difficult to recognise a biologically meaningful signal of differentiation from noise and artefacts due to sampling and laboratory procedures. The advantage of cross comparisons is twofold: while genetic data can potentially validate and improve biophysical simulations (Selkoe et al., 2010), the latter can point out unrealistic genetic results. At present, any interpretation of the significance of the results obtained with the outlier locus would be highly speculative but, considering that the locus pm108 was used in all the microsatellite studies of P. marmoratus published so far, it would be useful to understand to what extent its inclusion in the analyses has contributed to determining the weak genetic differentiation reported in the literature. Therefore, this locus clearly deserves further consideration in future studies.
From the methodological point of view, we believe that neutrality tests, or, at least, an examination of how many loci support a given differentiation pattern, should be routinely performed, and reported in studies dealing with weak differentiation results. While this was a very well-established procedure in early times when permutation tests were not available to check for significance, and it has always been considered a good practice in case of weak differentiation (e.g. Wirth and Bernatchez, 2001), this approach has been neglected in many recent microsatellite studies. Now, with an increasing number of microsatellite loci used to perform genetic analyses, the probability to pick up loci putatively under selection is increasing, and we think that neutrality tests should be systematically applied to avoid reporting biased estimates. As a side note, no outliers were indeed detected in the previously cited C. aestuarii study (Schiavina et al., 2014; original data at http://dx.doi.org/10.5061/dryad.r0d1q).
In conclusion, our study pointed out the pitfalls of using P. marmoratus as a model species to assess the genetic connectivity among MPAs or areas deserving protection at the considered spatial scale. Indeed, the broad availability of rocky shore habitats within the study area likely results in the presence of several unsampled connected populations that operate as steppingstones interposed among the sampled locations. Nevertheless, while this could be true for a wide-dispersing species like the marbled crab, a completely different picture might emerge considering habitat former species with low dispersal such as those found in bioconstructions (Ingrosso et al., 2018) such as the sunset cup coral Leptopsammia pruvoti (Boscari et al., 2019). Indeed, connectivity patterns may vary widely among species, due to differences in reproductive phenology, duration of the larval phase and/or habitat range (Boissin et al., 2016; Melià et al., 2016; Carreras et al., 2017). Thus, when using genetic data to assist design and management of MPA networks, it is crucial to carefully choose informative species, with appropriate dispersing features, habitat suitability, and reproductive timing and duration. The explicit incorporation of these aspects, together with a representative sampling and the use of genome-wide approaches, will allow a better understanding of the factors that shape oceanographic, demographic, and genetic connectivity.
The datasets presented in this study can be found in online repositories. The names of the repository/repositories and accession number(s) can be found below: https://doi.org/10.5061/dryad.brv15dvc4. Sequences of new microsatellite loci have been deposited in Genbank under accession numbers KX185954-KX185964.
IAMM, PM, MS, and LZ conceived and designed the experiments. SF, IAMM, and MS performed the experiments. SF, IAMM, PM, MS, and LZ analysed the data. PM, TP, and LZ contributed reagents/materials/analysis tools. GA, SB, EB, LC, SF, CK, IAMM, PM, CP, TP, MP, MS, EV, and LZ critically discussed results. IAMM, PM, MS and LZ wrote the paper, which was approved by all the co-authors. All authors contributed to the article and approved the submitted version.
This project has received funding from the European Union’s Seventh Framework Programme for Research, Technological Development and Demonstration under Grant Agreement No. 287844 (CoCoNET), which also supported IAMM post doc fellowship, and by the Italian Ministry of Research (PRIN 2020, Prot. 2020J3W3WC). We are thankful to the following people for their precious help for logistics and for collecting samples on the field: “Antheus srl (Lecce, Italy)”; G Guarnieri, F Boero, S Fraschetti and A Terlizzi (University of Salento, Italy); V Mačić (Institute of Marine Biology Kotor, Montenegro); D Micu (National Institute of Marine Research and Development, Romania); S Reizopoulou and MA Pancucci-Papadopoulou (Hellenic Centre for Marine Research, Greece); S Beqiraj (University of Tirana, Albania); E Hajdëri (Catholic University “Our Lady of Good Counsel”, Albania).
The authors declare that the research was conducted in the absence of any commercial or financial relationships that could be construed as a potential conflict of interest.
All claims expressed in this article are solely those of the authors and do not necessarily represent those of their affiliated organizations, or those of the publisher, the editors and the reviewers. Any product that may be evaluated in this article, or claim that may be made by its manufacturer, is not guaranteed or endorsed by the publisher.
The Supplementary Material for this article can be found online at: https://www.frontiersin.org/articles/10.3389/fmars.2022.944851/full#supplementary-material
Amos W., Hoffman J. I., Frodsham A., Zhang L., Best S., Hill V. S. (2007). Automated binning of microsatellite alleles: Problems and solutions. Mol. Ecol. Notes 7, 10–14. doi: 10.1111/j.1471-8286.2006.01560.x
Antao T., Lopes A., Lopes R. J., Beja-Pereira A., Luikart G. (2008). LOSITAN: A workbench to detect molecular adaptation based on a fst-outlier method. BMC Bioinf. 9, 323. doi: 10.1186/1471-2105-9-323
Arab A., Kazanjian G., Bariche M. (2015). Biological traits suggest a niche overlap between two grapsid crabs sharing the rocky intertidal of the eastern Mediterranean. J. Mar. Biol. Assoc. UK 95, 1685–1692. doi: 10.1017/S0025315415001010
Astolfi L., Dupanloup I., Rossi R., Bisol P. M., Faure E., Congiu L. (2005). Mitochondrial variability of sand smelt Atherina boyeri populations from north Mediterranean coastal lagoons. Mar. Ecol. Prog. Ser. 297, 233–243. doi: 10.3354/meps297233
Baratti M., Pinosio S., Gori M., Biricolti S., Chini G., Fratini S., et al. (2022). Differential gene expression and chemical patterns of an intertidal crab inhabiting a polluted port and an adjacent marine protected area. Sci. Tot. Env. 822, 153463. doi: 10.1016/j.scitotenv.2022.153463
Beerli P., Palczewski M. (2010). Unified framework to evaluate panmixia and migration direction among multiple sampling locations. Genetics 185, 313–326. doi: 10.1534/genetics.109.112532
Belkir K., Borsa P., Goudet J., Bonhomme F. (2005). Genetix v. 4.05, logiciel sous windows pour la génétique des populations (Université Montpellier II: Laboratoire Génome et Populations. CNRS UPR 9060).
Benjamini Y., Hochberg Y. (1995). Controlling the false discovery rate: A practical and powerful approach to multiple testing. J. R. Stat. Soc B Met. 57, 289–300. doi: 10.1111/j.2517-6161.1995.tb02031.x
Boero F., Foglini F., Fraschetti S., Goriup P., Planes S., Soukissian T., et al. (2016). CoCoNet: towards coast-to-coast networks of marine protected areas (From the shore to the high and deep sea), coupled with sea-based wind energy potential. SCIRES IT 6, 1–95. doi: 10.2423/i22394303v6Sp1
Boissin E., Micu D., Janczyszyn-Le Goff M., Neglia V., Bat L., Todorova V., et al. (2016). Contemporary genetic structure and post-glacial demographic history of the Black scorpionfish, Scorpaena porcus, in the Mediterranean and the Black seas. Mol. Ecol. 25, 2195–2209. doi: 10.1111/mec.13616
Boscari E., Abbiati M., Badalamenti F., Bavestrello G., Benedetti-Cecchi L., Cannas R., et al. (2019). A population genomics insight by 2b-RAD reveals populations’ uniqueness along the Italian coastline in Leptopsammia pruvoti (Scleractinia, dendrophylliidae). Divers. Distrib. 25, 1101–1117. doi: 10.1111/ddi.12918
Cannicci S., Paula J., Vannini M. (1999). Activity pattern and spatial strategy in Pachygrapsus marmoratus (Decapoda: grapsidae) from Mediterranean and Atlantic shores. Mar. Biol. 133, 429–435. doi: 10.1007/s002270050481
Carreras C., Ordóñez V., Zane L., Krushel C., Nasto I., Macpherson E., et al. (2017). Population genomics of an endemic Mediterranean fish: Differentiation by fine scale dispersal and adaptation. Sci. Rep. 7, 43417. doi: 10.1038/srep43417
Çetin C., Furman A., Kalkan E., Bilgin R. (2022). Mitonuclear genetic patterns of divergence in the marbled crab, Pachygrapsus marmoratus (Fabriciu) along the Turkish seas. PloS One 7, e0266506. doi: 10.1371/journal.pone.0266506
Congiu L., Rossi R., Colombo G. (2002). Population analysis of the sand smelt Atherina boyeri (Teleostei atherinidae), from Italian coastal lagoons by random amplified polymorphic DNA. Mar. Ecol. Prog. Ser. 229, 279–289. doi: 10.3354/meps229279
Cowen R. K., Sponaugle S. (2009). Larval dispersal and marine population connectivity. Annu. Rev. Mar. Sci. 1, 443–466. doi: 10.1146/annurev.marine.010908.163757
Cuesta J. A., Rodriguez A. (2000). Zoeal stages of the intertidal crab Pachygrapsus marmoratus (Fabricius) (Brachyura, grapsidae) reared in the laboratory. Hydrobiologia 436, 119–130. doi: 10.1023/A:1026576614590
Dauvin J. C. (2009). Record of the marbled crab Pachygrapsus marmoratus (Crustacea: Brachyura: Grapsidea) on the coast of northern cotentin, Normandy, western English channel. Mar. Biodivers. Rec. 2, e92. doi: 10.1017/S1755267209001109
Deli T., Fratini S., Ragionieri L., Said K., Chatti N., Schubart C. D. (2016). Phylogeography of the marbled crab Pachygrapsus marmoratus (Decapoda, grapsidae) along part of the African Mediterranean coast reveals genetic homogeneity across the siculo-Tunisian strait versus heterogeneity across the Gibraltar strait. Mar. Biol. Res. 12, 471–487. doi: 10.1080/17451000.2016.1154972
deRivera C. E., Hitchcock N. G., Teck S. J., Steves B. P., Hines A. H., Ruiz. G. M. (2007). Larval development rate predicts range expansion of an introduced crab. Mar. Biol. 150, 1275–1288. doi: 10.1007/s00227-006-0451-9
Di Franco A., Coppini G., Pujolar J. M., De Leo G. A., Gatto M., Lyubartsev V., et al. (2012). Assessing dispersal patterns of fish propagules from an effective Mediterranean marine protected area. PloS One 7, e52108. doi: 10.1371/journal.pone.0052108
dos Santos A., Santos A. M. P., Conway D. V. P., Bartilotti C., Lourenço P., Queiroga H. (2008). Diel vertical migration of decapod larvae in the Portuguese coastal upwelling ecosystem: Implications for offshore transport. Mar. Ecol. Prog. Ser. 359, 171–183. doi: 10.3354/meps07341
Do C., Waples R. S., Peel D., Macbeth G. M., Tillett B. J., Ovenden J. R. (2014). NeEstimator V2: Re-implementation of software for the estimation of contemporary effective population size (Ne) from genetic data. Mol. Ecol. Resour. 14, 209–214. doi: 10.1111/1755-0998.12157
Drake P., Arias A. M., Rodriguez A. (1998). Seasonal and tidal abundance patterns of decapod crustacean larvae in a shallow inlet (SW Spain). J. Plankton Res. 20, 585–601. doi: 10.1093/plankt/20.3.585
Edwards R. V. (2005). “Pachygrapsus marmoratus a marbled rock crab,” in Marine life information network: Biology and sensitivity key information reviews. Eds. Tyler-Walters H., Hiscock K. (Plymouth: Marine Biological Association of the United Kingdom). Available at: http://192.171.193.68/species/detail/1978.
Estoup A., Angers B. (1998). “Microsatellites and minisatellites for molecular ecology: theoretical and empirical considerations,” in Advances in molecular ecology. Ed. Carvalho G. (Amsterdam: IOS Press), 55–86.
Excoffier L., Lischer H. E. L. (2010). Arlequin suite ver 3.5: a new series of programs to perform population genetics analyses under Linux and windows. Mol. Ecol. Resour. 10, 564–567. doi: 10.1111/j.1755-0998.2010.02847.x
Excoffier L., Smouse P. E., Quattro J. M. (1992). Analysis of molecular variance inferred from metric distances among DNA haplotypes: Application to human mitochondrial DNA restriction data. Genetics 131, 479–491. doi: 10.1093/genetics/131.2.479
Fenaux L., Cellario C., Etienne M. (1985). Croissance de la larve de l’Oursin Paracentrotus lividus. Mar. Biol. 86, 151–157. doi: 10.1007/BF00399021
Flores A. A. V., Cruz J., Paula J. (2002). Temporal and spatial patterns of settlement of brachyuran crab megalopae at a rocky coast in central Portugal. Mar. Ecol. Prog. Ser. 229, 207–220. doi: 10.3354/meps229207
Flores A. A. V., Paula J. (2002a). Sexual maturity, larval release and reproductive output of two brachyuran crabs from a rocky intertidal area in central Portugal. Invert. Reprod. Dev. 42, 21–34. doi: 10.1080/07924259.2002.9652506
Flores A., Paula J. (2002b). Population dynamics of the shore crab Pachygrapsus marmoratus (Brachyura: Grapsidae) in the central Portuguese coast. J. Mar. Biol. Ass. UK 82, 229–241. doi: 10.1017/S0025315402005404
Fratini S., Ragionieri L., Cutuli G., Vannini M., Cannicci S. (2013). Pattern of genetic isolation in the crab Pachygrapsus marmoratus within the Tuscan archipelago (Mediterranean Sea). Mar. Ecol. Prog. Ser. 478, 173–183. doi: 10.3354/meps10247
Fratini S., Ragionieri L., Deli T., Harrer A., Marino I. A. M., Cannicci S., et al. (2016). Unravelling population genetic structure with mitochondrial DNA in a notional panmictic coastal crab species: Sample size makes the difference. BMC Evol. Biol. 16, 150. doi: 10.1186/s12862-016-0720-2
Fratini S., Ragionieri L., Papetti C., Pitruzzella G., Rorandelli R., Barbaresi S., et al. (2006). Isolation and characterization of microsatellites in Pachygrapsus marmoratus (Grapsidae; decapoda; brachyura). Mol. Ecol. Notes 6, 179–181. doi: 10.1111/j.1471-8286.2005.01184.x
Fratini S., Schubart C. D., Ragionieri L. (2011). “Population genetics in the rocky shore crab Pachygrapsus marmoratus from the western Mediterranean and eastern Atlantic: Complementary results from mtDNA and microsatellites at different geographic scales,” in Phylogeography and population genetic in Crustacea. Eds. Held C., Koenemann S., Schubart C. D. (Boca Raton: Taylor & Francis/CRC Press), 191–213.
Fratini S., Zane L., Ragionieri L., Vannini M., Cannicci S. (2008). Relationship between heavy metal accumulation and genetic variability decrease in the intertidal crab Pachygrapsus marmoratus (Decapoda; grapsidae). Estuar. Coast. Shelf Sci. 79, 679–686. doi: 10.1016/j.ecss.2008.06.009
Galindo H. M., Olson D. B., Palumbi S. R. (2006). Seascape genetics: A coupled oceanographic-genetic model predicts population structure of Caribbean corals. Curr. Biol. 16, 1622–1626. doi: 10.1016/j.cub.2006.06.052
Galindo H. M., Pfeiffer-Herbert A. S., McManus M. A., Chao Y. I., Chai F., Palumbi S. R. (2010). Seascape genetics along a steep cline: Using genetic patterns to test predictions of marine larval dispersal. Mol. Ecol. 19, 3692–3707. doi: 10.1111/j.1365-294X.2010.04694.x
Gilg M. R., Hilbish T. J. (2003). The geography of marine larval dispersal: coupling genetics with fine-scale physical oceanography. Ecology 84, 2989–2998. doi: 10.1890/02-0498
Goudet J. (2001) FSTAT, a program to estimate and test gene diversities and fixation indices (version 2.9.3). Available at: www.unil.ch/popgen/softwares/fstat.html.
Guarnieri A., Oddo P., Pastore M., Pinardi N., Ravaioli M. (2010). “The Adriatic basin forecasting system: New model and system development,” in Proceeding of the Fifth International Conference on EuroGOOS, 184–190 (Exeter, UK: EuroGOOS Publication).
Hauser L., Carvalho G. R. (2008). Paradigm shifts in marine fisheries genetics: Ugly hypotheses slain by beautiful facts. Fish. 9, 333–362. doi: 10.1111/j.1467-2979.2008.00299.x
Hedrick P. W. (2005). A standardized genetic differentiation measure. Evolution 59, 1633–1638. doi: 10.1111/j.0014-3820.2005.tb01814.x
Hohenlohe P. A. (2004). Limits to gene flow in marine animals with planktonic larvae: models of Littorina species around point conception, California. Biol. J. Linn. Soc 82, 169–187. doi: 10.1111/j.1095-8312.2004.00318.x
Iannucci A., Cannicci S., Caliani I., Baratti M., Pretti C., Fratini S. (2020). Investigation of mechanisms underlying chaotic genetic patchiness in the intertidal marbled crab Pachygrapsus marmoratus (Brachyura: Grapsidae) across the Ligurian Sea. BMC Evol. Biol. 20, 108. doi: 10.1186/s12862-020-01672-x
Ingrosso G., Abbiati M., Badalamenti F., Bavestrello G., Belmonte G., Cannas R., et al. (2018). Mediterranean Bioconstructions along the Italian coast. Adv. Mar. Biol. 79, 61–136. doi: 10.1016/bs.amb.2018.05.001
Jombart T. (2008). Adegenet: A r package for the multivariate analysis of genetic markers. Bioinformatics 24, 1403–1405. doi: 10.1093/bioinformatics/btn129
Jorde P. E., Ryman N. (1995). Temporal allele frequency change and estimation of effective size in populations with overlapping generations. Genetics 139, 1077–1090. doi: 10.1093/genetics/139.2.1077
Jost L. (2008). GST and its relatives do not measure differentiation. Mol. Ecol. 17, 4015–4026. doi: 10.1111/j.1365-294X.2008.03887.x
Kalkan E., Karhan S.Ü., Bilgin R. (2013). Population genetic structure of the marbled crab, Pachygrapsus marmoratus from Turkish coasts of the Black Sea and the eastern Mediterranean. Rapp. Commun. Int. Mer Médit. 40, 713.
Keenan K., McGinnity P., Cross T. F., Crozier W. W., Prodöhl P. A. (2013). diveRsity: An r package for the estimation of population genetics parameters and their associated errors. Methods Ecol. Evol. 4, 782–788. doi: 10.1111/2041-210X.12067
Kilada R., Driscoll J. G. (2017). Age determination in crustaceans: A review. Hydrobiologia 799, 21–36. doi: 10.1007/s10750-017-3233-0
Knutsen H., Olsen E. M., Jorde P. E., Espeland S. H., André C., Stenseth. N. C. (2011). Are low but statistically significant levels of genetic differentiation in marine fishes ‘biologically meaningful’? A case study of coastal Atlantic cod. Mol. Ecol. 20, 768–783. doi: 10.1111/j.1365-294X.2010.04979.x
Levin L. A. (2006). Recent progress in understanding larval dispersal: New directions and digressions. Integr. Comp. Biol. 46, 282–297. doi: 10.1093/icb/icj024
Malausa T., Gilles A., Meglécz E., Blanquart H., Duthoy S., Costedoat C., et al. (2011). High-throughput microsatellite isolation through 454 GS-FLX titanium pyrosequencing of enriched DNA libraries. Mol. Ecol. Resour. 11, 638–644. doi: 10.1111/j.1755-0998.2011.02992.x
Melià P., Schiavina M., Rossetto M., Gatto M., Fraschetti S., Casagrandi R. (2016). Looking for hotspots of marine metacommunity connectivity: A methodological framework. Sci. Rep. 6, 23705. doi: 10.1038/srep23705
Nunney L. (1999). The effective size of a hierarchically structured population. Evolution 53, 1–10. doi: 10.1111/j.1558-5646.1999.tb05328.x
Oddo P., Pinardi N., Zavatarelli M., Coluccelli A. (2006). The Adriatic basin forecasting system. Acta Adriat. S47, 169–184. Available at: https://hrcak.srce.hr/8550
Ohta T., Kimura M. (1973). A model of mutation appropriate to estimate the number of electrophoretically detectable alleles in a finite population. Genet. Res. 22, 201–204. doi: 10.1017/S0016672300012994
Olds A. D., Connolly R. M., Pitt K. A., Pittman S. J., Maxwell P. S., Huijbers C. M., et al. (2016). The conservation value of seascape connectivity. Glob. Ecol. Biogeogr. 25, 3–15. doi: 10.1111/geb.12388
Palumbi S. R. (2003). Population genetics, demographic connectivity, and the design of marine reserves. Ecol. Appl. 13, S146–S158. doi: 10.1890/1051-0761(2003)013[0146:PGDCAT]2.0.CO;2
Papetti C., Susana E., Patarnello T., Zane L. (2009). Spatial and temporal boundaries to gene flow between Chaenocephalus aceratus populations at South Orkney and South Shetlands. Mar. Ecol. Prog. Ser. 376, 269–281. doi: 10.3354/meps07831
Paterno M., Bat L., Souissi J. B., Boscari E., Chassanite A., Congiu L., et al. (2019). A genome-wide approach to the phylogeography of the mussel Mytilus galloprovincialis in the Adriatic and the Black seas. Front. Mar. Sci. 6, 566. doi: 10.3389/fmars.2019.00566
Paterno M., Schiavina M., Aglieri G., Ben Souissi J., Boscari E., Casagrandi R., et al. (2017). Population genomics meet Lagrangian simulations: Oceanographic patterns and long larval duration ensure connectivity among Paracentrotus lividus populations in the Adriatic and Ionian seas. Ecol. Evol. 7, 2463–2479. doi: 10.1002/ece3.2844
Patwary M. U., Kenchington E. L., Bird C. J., Zouros E. (1994). The use of random amplified polymorphic DNA markers in genetic studies of the sea scallop Placopecten magellanicus (Gmelin 1791). J. Shellfish Res. 13, 547–553.
Pedrotti M. L. (1993). Spatial and temporal distribution and recruitment of echinoderm larvae in the Ligurian Sea. J. Mar. Biol. Assoc. UK 73, 513–530. doi: 10.1017/S0025315400033075
Peng B., Kimmel M. (2005). simuPOP: A forward-time population genetics simulation environment. Bioinformatics 21, 3686–3687. doi: 10.1093/bioinformatics/bti584
Pires R. F.T., Peliz Á., Pan M., dos Santos A. (2020). “There and Back Again” – How Decapod Megalopae Find the Way Home: A Modelling Exercise for Pachygrapsus marmoratus. Prog. Oceanogr. 184, 102231. doi: 10.1016/j.pocean.2020.102331
Pritchard J. K., Stephens M., Donnelly P. (2000). Inference of population structure using multilocus genotype data. Genetics 155, 945–959. doi: 10.1093/genetics/155.2.945
Pujolar J. M., Marčeta T., Saavedra C., Bressan M., Zane L. (2010). Inferring the demographic history of the Adriatic Flexopecten complex. Mol. Phylogenet. Evol. 57, 942–947. doi: 10.1016/j.ympev.2010.08.002
Pujolar J. M., Schiavina M., Di Franco A., Melià P., Guidetti P., Gatto. M., et al. (2013). Understanding the effectiveness of marine protected areas using genetic connectivity patterns and Lagrangian simulations. Divers. Distrib. 19, 1531–1542. doi: 10.1111/ddi.12114
Raymond M., Rousset F. (1995). GENEPOP (Version 1.2): Population genetics software for exact tests and ecumenicism. J. Hered. 86, 248–249. doi: 10.1093/oxfordjournals.jhered.a111573
Rousset F. (2008). GENEPOP’007: A complete re-implementation of the GENEPOP software for windows and Linux. Mol. Ecol. Resour. 8, 103–106. doi: 10.1111/j.1471-8286.2007.01931.x
R Team Core (2015). R: a language and environment for statistical computing (Vienna, Austria: R Foundation for Statistical Computing). Available at: http://www.R–project.org.
Schiavina M., Marino I. A. M., Zane L., Melià P. (2014). Matching oceanography and genetics at the basin scale. Seascape connectivity of the Mediterranean shore crab in the Adriatic Sea. Mol. Ecol. 23, 5496–5507. doi: 10.1111/mec.12956
Schunter C., Carreras-Carbonell J., Macpherson E., Tintoré J., Vidal-Vijande E., Pascual A., et al. (2011). Matching genetics with oceanography: Directional gene flow in a Mediterranean fish species. Mol. Ecol. 20, 5167–5181. doi: 10.1111/j.1365-294X.2011.05355.x
Selkoe K. A., Toonen R. J. (2011). Marine connectivity: A new look at pelagic larval duration and genetic metrics of dispersal. Mar. Ecol. Prog. Ser. 436, 291–305. doi: 10.3354/meps09238
Selkoe K. A., Watson J. R., White C., Horin T. B., Iacchei M., Mitarai S., et al. (2010). Taking the chaos out of genetic patchiness: Seascape genetics reveals ecological and oceanographic drivers of genetic patterns in three temperate reef species. Mol. Ecol. 19, 3708–3726. doi: 10.1111/j.1365-294X.2010.04658.x
Silva I. C., Mesquita N., Schubart C. D., Judite Alves M., Paula J. (2009). Genetic patchiness of the shore crab Pachygrapsus marmoratus along the Portuguese coast. J. Exp. Mar. Biol. Ecol. 378, 50–57. doi: 10.1016/j.jembe.2009.07.032
van Oosterhout C., Hutchinson W. F., Wills D. P. M., Shipley P. (2004). MICRO-CHECKER: software for identifying and correcting genotyping errors in microsatellite data. Mol. Ecol. Notes 4, 535–538. doi: 10.1111/j.1471-8286.2004.00684.x
Vernet-Cornubert G. (1958). Recherches sur la sexualité du crabe Pachygrapsus marmoratus (Fabricius). Arch. Zool. Exp. Gen. 96, 101–276.
Vitalis R. (2012). DetSel: an r-package to detect marker loci responding to selection. Methods Mol. Biol. 888, 277–293. doi: 10.1007/978-1-61779-870-2_16
Vitalis R., Dawson K., Boursot P., Belkhir K. (2003). DetSel 1.0: A computer program to detect markers responding to selection. J. Hered. 94, 429–431. doi: 10.1093/jhered/esg083
Wang J. (2015). Does GST underestimate genetic differentiation from marker data? Mol. Ecol. 24, 3546–3558. doi: 10.1111/mec.13204
Ward R. D., Woodwark M., Skibinski D. O. F. (1994). A comparison of genetic diversity levels in marine, freshwater, and anadromous fishes. J. Fish. Biol. 44, 213–232. doi: 10.1111/j.1095-8649.1994.tb01200.x
Weir B. S., Cockerham C. C. (1984). Estimating f-statistics for the analysis of population structure. Evolution 38, 1358–1370. doi: 10.2307/2408641
Wirth T., Bernatchez L. (2001). Genetic evidence against panmixia in the European eel. Nature 409, 1037–1040. doi: 10.1038/35059079
Keywords: Lagrangian simulations, marbled crab, microsatellites, seascape genetics, individual-based simulations
Citation: Marino IAM, Schiavina M, Aglieri G, Bevilacqua S, Boscari E, Congiu L, Faggion S, Kruschel C, Papetti C, Patarnello T, Paterno M, Voutsinas E, Zane L and Melià P (2022) Assessment of connectivity patterns of the marbled crab Pachygrapsus marmoratus in the Adriatic and Ionian seas through combination of genetic data and Lagrangian simulations. Front. Mar. Sci. 9:944851. doi: 10.3389/fmars.2022.944851
Received: 15 May 2022; Accepted: 01 September 2022;
Published: 29 September 2022.
Edited by:
Alberto Basset, University of Salento, ItalyReviewed by:
Temim Deli, University of Regensburg, GermanyCopyright © 2022 Marino, Schiavina, Aglieri, Bevilacqua, Boscari, Congiu, Faggion, Kruschel, Papetti, Patarnello, Paterno, Voutsinas, Zane and Melià. This is an open-access article distributed under the terms of the Creative Commons Attribution License (CC BY). The use, distribution or reproduction in other forums is permitted, provided the original author(s) and the copyright owner(s) are credited and that the original publication in this journal is cited, in accordance with accepted academic practice. No use, distribution or reproduction is permitted which does not comply with these terms.
*Correspondence: Lorenzo Zane, bG9yZW56by56YW5lQHVuaXBkLml0
†These authors have contributed equally to this work
Disclaimer: All claims expressed in this article are solely those of the authors and do not necessarily represent those of their affiliated organizations, or those of the publisher, the editors and the reviewers. Any product that may be evaluated in this article or claim that may be made by its manufacturer is not guaranteed or endorsed by the publisher.
Research integrity at Frontiers
Learn more about the work of our research integrity team to safeguard the quality of each article we publish.