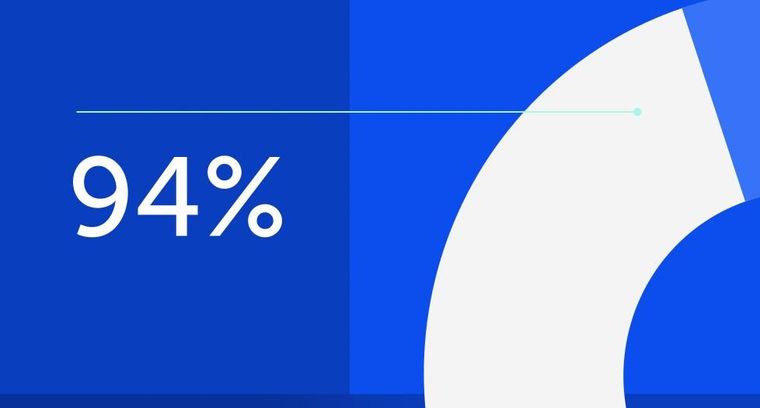
94% of researchers rate our articles as excellent or good
Learn more about the work of our research integrity team to safeguard the quality of each article we publish.
Find out more
ORIGINAL RESEARCH article
Front. Mar. Sci., 25 January 2023
Sec. Marine Fisheries, Aquaculture and Living Resources
Volume 9 - 2022 | https://doi.org/10.3389/fmars.2022.944630
Industrial structure adjustment is an important component of China’s supply-side structural reforms. This study examines the effect and action path of regional industrial structure transformation, through the lens of advancement and rationalization, on the economic fluctuation and quality of the marine fishery industry. The results show that regional industrial structure advancement (rationalization) promotes (reduces) economic fluctuation. Regarding the action paths, regional industrial structure advancement (rationalization) has a U-shaped (inverted U-shaped) relationship with economic quality. The regional industrial structure advancement level is greater than 5.108 in China’s coastal areas and on the right side of the U shape. Thus, the evolution of the industrial structure in these areas is conducive to improving the economic quality of marine fisheries. Meanwhile, The degree of regional industrial structure rationalization has not reached the optimum level, and most areas are far from optimum level. This suggests that the structural dividends from industrial structure rationalization not fully released and need further regulation. In terms of the overall effect, regional industrial structure transformation can cause economic fluctuations in marine fisheries, with the main force originating from industrial structure advancement. The path of regional industrial structure transformation affecting economic quality of marine fisheries is non-linear. At present, the industrial structure advancement transformation can improve economic quality, but the industrial structure rationalization needs to be further adjusted.
Supply side structural reforms are a major innovation and necessary measure since China’s economy transitioned into the new normal of high-quality growth. The country aims to transform its factor-dependent pattern of economic growth through structural reforms to one that focuses on innovation (Hu et al., 2016). Importantly, the effectiveness of reform is related to high-quality economic development. Regions can accelerate regional industrial transformation, optimize their industrial structure, and cultivate new drivers of economic development. Adjusting the industrial structure affects the stability of the national economy and the quality of economic development1 (hereafter, economic quality) by changing the relationship between industrial sectors and resource allocation. Marine fisheries have been one such important industry subject to industrial structure transformation.
As an important supplier of proteins, marine fisheries have played an important role in improving the national dietary structure and nutrition levels in China (Han and Li, 2015; Warren and Steenbergen, 2021). After decades of development and focused efforts, the industry’s production capacity has improved, accompanied by a diversity of products and improved integrity, modernization, and productivity of systems. For instance, there is diversity and abundance of fresh fish, shrimp, shellfish, mollusks, and other fresh fish products, as well as processed products such as minced fish, fish oil, and canned food. Meanwhile, there has been rapid development of nurseries; processing technology; fishery machinery manufacturing, feed, medicine, and construction, distribution, and transport; and recreational fishing around mariculture and marine fishing. For instance, the total amount of aquatic products in China reached 69.01 million tons in 2016, an increase of 15 times compared with 1979.2 The estimated amount of protein provided by marine fisheries was 2.6621 million tons, accounting for approximately 12.6% of the total amount provided by the food system (Han, 2018). This amount can replace approximately 22.97 million tons of meat (pork) or 91.88 million tons of food (raw grain), saving 16.9 million hectares of arable land and 75.8 billion tons of fresh water in 2016 (Li and Han, 2019).
However, the resource-based nature of the marine fishery industry means that it may suffer from the regional industrial structure adjustment (RISA) efforts, which may be mitigated by reinjection of resources for rural revitalization and innovation via regional industrial structure rationalization (RISR). RISA promotes the flow of resource and production factors from low productivity or growth sectors to high productivity or growth sectors; this is characterized as the “siphon effect.” This reduced availability of resources and factors can affect the economic development quality and economic fluctuations3 in marine fisheries with relatively low productivity or growth (Gan et al., 2011).
Meanwhile, under the promotion of rural revitalization and innovation, RISR will drive some high-quality resource (technology, talents, etc.) to flow back to the marine fishery industry, such as returning migrant workers starting their own business. This may promote the industry’s economic development. We define this phenomenon as the “backflow effect.” Then, we need to understand how the siphon and backflow effects influence the economic stability and quality of the marine fisheries.
Most studies show that industrial structure transformation is a key factor that influences production efficiency and economic fluctuation (Burns, 1960; Samuels, 2017). However, these influences manifest in two ways. First, RISA can improve the overall economic efficiency and provide a “structural dividend” (Wang et al., 2004; Liu and Zhang, 2008; Bah and Brada, 2009; Ahmad et al., 2015; Tu et al., 2016; Xu and Tao, 2017; Zhang and Ping, 2017). Simultaneously, the redistribution of factors can promote the growth and decline of different industries and thus cause economic fluctuations (Baumol, 1967; Peneder, 2003). Thus, RISA becomes an important source of economic fluctuations in industries. Second, RISR is conducive to stable economic development by revitalizing regions by redistributing resources (Eggers and Loannides, 2006; Fang and Zhan, 2011; Peng et al., 2013). However, it may not affect productivity and, hence, may not yield a “structural dividend” (Fagerberg, 2000; Zhu and Wang, 2016; Shen et al., 2020).
Studies have used stochastic frontier, mixed data, shift share analysis, and panel threshold models to empirically analyze the impact of industrial structure adjustment on fishery economic growth. These studies show that this adjustment is important for marine fishery’s economic growth (Meng, 2015) and an important way to promote the fishery economy’s transformative development (Wang and Ning, 2017). Many scholars hold that the current fishery industry’s economic growth mainly depends on the primary industry, whereas the contribution of secondary and tertiary industries is not high. This industrial structure may not be conducive to the fishery economy’s growth and the improvement of the industry’s technical efficiency and international competitiveness (Ping and Zhao, 2018; Le et al., 2019). Improving this structure is important for promoting the fishery industry’s economic growth and, thus, fishermen’s income (Yang and Yan, 2018; Jin et al., 2020).
Other studies have analyzed this lopsided industrial structure from the perspective of advancement and rationalization. They also agree that the advancement of fisheries’ industrial structure can be conducive to promoting the industry’s economic growth (Liu, 2018; Wang et al., 2019a). Liu (2018) believed that the rationalization of the current fisheries’ industrial structure negatively affected fishery economic growth. Meanwhile, Wang et al. (2019) believed that this relationship is non-linear with periodic characteristics. Based on this, research has also examined countermeasures for the optimization and upgrading of the marine fishery industry structure (Yang and Su, 2010).
In summary, the literature analyzes the internal mechanism of industrial structure adjustment affecting the marine fishery industry’s economic growth. However, the focus has been mainly on only analyzing this specific industry’s transformation. Few have examined these impacts at a larger scale, such as the impact of region industrial structure transformations. Here, we analyzed this problem from the perspective of regional industrial structure adjustment to gain insights on effectively promoting the upgrading and high-quality development of the marine fishery economy in China.
The State Council of the People’s Republic of China issued Central Document No. 1 in 2016,4 which had “several opinions on promoting the structural reform of agricultural supply side deeply and accelerating the cultivation of new driving forces for agricultural and rural development.” It noted that “it is necessary to promote the transformation of agricultural and rural development from over-reliance on resource consumption and emphasis on quantitative needs to the pursuit of green ecological sustainability and focusing on satisfying qualitative needs.” Marine fishery is also faced with the structural problem of an effective supply shortage, whose solution, intuitively, is on the supply side. Indeed, the “key points of fishery administration work” issued by China’s Ministry of Agriculture and Rural Affairs in 2018, 2019, and 2020 clearly pointed out that “the supply side structural reform should be taken as the main line in future fishery development.” Therefore, based on the perspective of advancement and rationalization, this study analyzes the relationship between regional industrial structure transformation and marine fishery’s economic development (Figure 1).
RISA’s characteristic is that the leading industries are successively replaced. Even without considering the influence of government regulatory policies, it promotes the agglomeration of labor, capital, technology, and other resources in emerging industries with high efficiency or growth, whereas traditional industries with low efficiency or growth face the loss of resource. The characteristics of high risk and investments and the long capital payback period of marine fisheries mean that high growth and productivity may be elusive in this industry; consequently, under RISA, many economic factors will flow to non-fishing industries. The loss of production factors will inevitably affect the industry’s development, marine aquaculture, marine fishing, seafood processing, seafood storage and logistics, and other industries, such as industrial-scale compression (Wang, 2022). This will cause economic fluctuations in marine fisheries. This shows that RISA can promote the economic fluctuations of the marine fishery industry, with an obvious leverage effect.
Furthermore, RISA may also pose challenges for attracting talent. Over the years, marine fisheries have become highly dependent on capital-intensive equipment, such as fishing boats, fishing gear, and deep-water cages (Han et al., 2007), as they help improve economic efficiency. Meanwhile, with the decline in offshore fishery resources and greater industrial sea usage, deep-sea mariculture and oceanic or trans-oceanic fishery will become the main focus of marine fisheries in the future (Han et al., 2016; Fu et al., 2019). Indeed, in 2020, Central Document No. 1 noted “focusing on supporting the development of pond engineering and industrial recirculating aquaculture,” and “support[ing] deep-sea aquaculture.” Together, this means the further development of marine fishery using modern high-end equipment, such as large fishing vessels, deep-sea cages, testing equipment, cold chain transportation, etc. This will inevitably lead to increased demand for highly skilled talent. However, RISA attracts highly skilled talent in non-fishing industries with high productivity or growth; this will lead to the insufficient supply of highly technical talent in marine fisheries.
Thus, in the early stages of RISA, production factors will flow from the fishery to non-fishery industries. The loss of capital and lack of talent will restrict the improvements in the efficiency of marine fishery development. However, in the later stages of RISA, regional scientific and technological innovations, and advanced technologies (such as big data, Internet, artificial intelligence, and BeiDou/GPS), and equipment (such as intelligent sensors, underwater robots, and deep-sea potential devices) will gradually flow to the marine fishery industry (Valdemarsen, 2001). This will drive the industry’s transformation and upgrading. Overall in the early (later) stage, RISA’s evolution has a negative (positive) impact on the economic quality of marine fishery.
Based on this discussion, we propose the following hypotheses.
H1: RISA’s evolution can cause economic fluctuations in the marine fishery industry.
H2: RISA’s impact on the economic quality of the marine fishery industry varies across stages of RISA.
RISR aims to pursue the balanced development among industries, which is different from the emphasis of RISA. This evolution can optimize the allocation of resource elements, coordinate the relationship between industrial development, promote industrial relations from unbalanced to balanced, and finally, achieve balanced economic development. This dampens the volatility induced by RISA’s evolution in the marine fishery economy.
Specifically, RISR’s evolution will help reinject part of the resources from non-fishery industries to the marine fishery industry, thereby mitigating some of RISA-induced economic fluctuations. This can help the steady development of the marine fishery economy
The evolution of RISR also affects the marine fishery efficiency. Specifically, RISR’s evolution can mitigate the impact of resource loss experienced by the industry by optimizing the resource allocation structure between different industries through two ways. First, RISR can restrict the flow of resources in high growth sectors and redirecting these resources to sectors with low growth. Ultimately, the economic efficiency of marine fisheries is reduced due to the misallocation of resource elements; however, this effect is likely small given the criticality of marine fisheries to fulfilling food requirements. Second, RISR’s evolution will help coordinate the flow of high-innovation and advanced production factors, such as advanced technology, high-end talents, and intelligent equipment, from non-fishery industries to the marine fishery industry. In addition to helping alleviate the misallocation of resources (Deng etal., 2018), it will improve the efficiency of marine fisheries.
Based on this discussion, we propose the following hypotheses.
H3: RISR’s evolution dampens the economic fluctuations in marine fisheries and promotes its stable development.
H4: RISR’s evolution affects the economic quality of marine fisheries and has periodic characteristics.
We introduce regional industrial structure as an addition factor in the Cobb–Douglas production function to explore the impact of the evolution of RISA and RISR on economic fluctuations in the marine fishery industry. The function is as follows:
where y is the gross economic product of marine fishery; A represents marine fishery technology; K is the capital input of marine fishery; L is the labor input of marine fishery; S is the regional industrial structure, including RISA and RISR; and α, β, and γ represent the elasticity coefficients of marine fishery capital, labor force, and regional industrial structure evolution on the marine fishery industry, respectively, and meet the condition α + β + γ = 1. To improve the accuracy of measurement, we take the logarithm of both sides of Equation (1) to obtain the following:
We assume that the actual economic input and output are composed of two parts: the potential part and the difference between the actual and potential parts. The difference reflects the economic fluctuations. Then, the indicators of economic output, labor force, capital input, and industrial structure can be transformed into two parts as follows:
where lny', lnL', lnK', and lnS' represent the actual output value, labor and capital input values, and the trend component of industrial structure, respectively; the potential parts Λy, ΛL, ΛK , and ΛS represent the difference between the actual and potential parts of the actual output value, labor and capital input values, and industrial structure, respectively.
Following Ding and Zhang (2013), we assume that the industrial structure adjustment occurs in one period. Thus, the stable value of industrial structure adjustment in period t is the actual value of industrial structure adjustment in period t-1: ; then, ΛSt can be expressed as follows:
Because this is an index to measure the speed of industrial structure change, it can be expressed as
gSt, where g is the rate of regional industrial structure change. By removing the trend component from Equation (2), we obtain:
where i indicates the region. We refer to Fang and Zhan"s (2011) treatment method to explore the impact of regional industrial structure evolution on the economic fluctuation in the marine fishery industry. Specifically, we take the absolute value of labor, capital, and output fluctuations: wyi,t=|Λyi,t|, wLi,t=|ΛLi,t|, and wKi,t=|ΛKi,t|. Meanwhile, let lnA=β0, α=β1, β=β2, and γ=β3. Finally, adding the intercept term ui and random disturbance term ϵi,t. of individual heterogeneity to the right-hand side of the equation, we obtain the following econometric model:
β3 is our main coefficient of interest. If β3<0, the evolution of regional industrial structure will weaken the economic fluctuations in the marine fishery industry. This phenomenon is defined as the “mitigating effect.” Meanwhile, If β3>0, evolution in the regional industrial structure will increase the economic fluctuations in marine fishery industry. This phenomenon is defined as the “leverage effect.”
To improve the model"s accuracy, we introduce some control variables. Referring to Frank (2005), we use the interaction between economic fluctuation and industrial structure as a control variable. Further, considering the dynamic nature of economic development, this study selects a dynamic model for estimation [Equation (7)]. Finally, to eliminate the influence of individual effect on the model, we also use the dynamic difference panel model [Equation (8)]. These models are outlined as follows:
To avoid the correlation of the random disturbance terms after the difference, this study introduces the panel robustness standard deviation in the model estimation. Furthermore, the instrumental variable method is used to address the endogeneity problem. We use Baum and Schaffer’s method (Baum et al., 2007) to select the lag or difference items of independent variables as instrumental variables. To avoid overidentification, the Sargan tests5 were used to judge the effectiveness of instrumental variables.
The evolution of regional industrial structure also affects the economic quality of the marine fishery industry. Extant research has inconsistent evidence on the impact of industrial structure evolution on economic quality, with some scholars showing that that this relationship is non-linear (Deng et al., 2018). To clarify the nature of this relationship, this study assumes that the relationship between the evolution of regional industrial structure and the quality of marine fishery development is non-linear. We then establish a dynamic panel regression model with quadratic terms as follows:
where mftfpi,t refers to the level of economic quality of the marine fishery industry in t year of region i, and Si,t is the level of regional industrial structure in t year of region i, which mainly includes two indicators: regional industrial structure advancement (RISAi,t) and regional industrial structure rationalization (RISRi,t), that is, Si,t={RISAi,t, RISRi,t} . Given the non-linearity and endogeneity concerns, we use the generalized method of moments (GMM)6 for estimation of Equation (9).
The main dependent variables are economic fluctuation and economic quality, whereas the main independent variables are regional industrial structure advancement, regional industrial structure rationalization, and marine fishery capital and labor force. The relevant variables are described below.
The fluctuation in the marine fishery economy ( Λy ). The Hodrick and Prescott (1997) proposed filtering method is used to separate the logarithm-processed gross marine fishery production series (lny) to obtain the long-term trend and periodic component. The periodic component represents the value of marine fishery fluctuation. As we use annual data, the conversion factor for the trend component fluctuation is 100.
Marine fishery economic quality (mftfp). It is measured by marine fishery total factor productivity. Following Gómez and Maynou (2020) and based on the constant returns to scale (CRS) model, we use the data envelopment analysis-Malmquist (DEA-Malmquist) to calculate the total factor productivity. Before calculating the Malmquist index, we need to objectively select economic input and output indexes. The input index for marine fishery mainly includes labor force, capital, technology, fishing boat, and mariculture area. Marine fishery labor force is measured by the number of marine fishery employees at the end of the year. Capital investment is equal to the input of fixed assets in agriculture, forestry, animal husbandry, and fishery multiplied by the proportion of marine fishery in the total output value of agriculture, forestry, animal husbandry, and fishery. The capital stock is calculated as an input index following Wang and Han (2017). We measure marine fishery technology investment by marine fishery technology promotion funds. Fishing boat input is measured by fishing boat power, and mariculture area is directly measured by the statistical data in China Fisheries Statistical Yearbook.
We refer to Wang et al. (2021) to measure RISA and RISR. We adopt the adjusted Mre’s structural change coefficient for RISA and the Taier index for RISR. The Taier index and industrial structure rationalization have an inverse relationship. The larger RISRi,t , the more unreasonable the industrial structure. S are measured using interaction terms of RISA and RISR. The variable classifications, names and codes are shown in Table 1.
We focused on the 10 coastal provinces, cities, and autonomous regions of China, including Liaoning, Tianjin, Hebei, Jiangsu, Zhejiang, Fujian, Shandong, Guangdong, Guangxi, and Hainan. The sample data are marine fishery economic data from 2003 to 2020 collected mainly from the China Fishery Statistical Yearbook, China Agricultural Statistical Yearbook, and China Statistical Yearbook. Data on seawater breeding, seafood processing, marine fishing vessel and fishing machine repair, marine fishing rope and net manufacturing, marine fishery feed and medicine, marine fishery circulation, marine fishery (storage) transportation, and marine leisure fishery are not listed separately in the relevant statistical yearbook. Hence, we borrow the adjustment method of Wang et al. (2019b) to calculate these data. Finally, to improve the accuracy of empirical test by considering the impact of the inflation rate, we take 2002 as the base period and use the price index of gross product of fishery to adjust the output value data.
The Fisher-augmented Dicker Fuller and Levin–Lin–Chu tests are used to test the stability of the data series. The test results (Table 2) show that wyi,t, wLi,t, wKi,t, RISAi,t, RISRi,t, g·RISAi,t, g·RISRi,t, g·RISAi,t*wyi,t, g·RISRi,t*wyi,t, lnRISAi,t, (lnRISAi,t)2, Mftfpi,t* lnRISRi,t, and Mftfpi,t*lnRISAi,t pass the 5% significance level, which indicates that there is no unit root in the variable sequence and they are stable and meet the 0-order integer. However, Si,t, mftfpi,t*Si,t, g·Si,t, g·Si,t*wyi,t, lnRISRi,t and (lnRISRi,t)2 are not significant, indicating that there may be a unit root in the sequence; hence, the unit root test is carried out after the first-order difference processing. The first-order difference of these two factors is stable belonging to the first-order single integer, namely, d.Si,t~I(1), d.(mftfpi,t*Si,t)~I(1), d.(g·Si,t)~I(1), d.(g·Si,t*wyi,t)~I(1), D.(lnRisri,t)~I(1) and D.(lnRisri,t)2~I(1).
Because some variables do not meet the single integer of the same order, in the model analysis of the impact of RISR on marine fishery quality, this study follows Zhao and Yu (2012) by replacing the original data with variable data after the first-order differenced.
This section mainly uses the differential GMM to test the impact of regional industrial structure evolution on marine fishery economic fluctuations in four models. Models 1 and 2 test the impact of RISA and RISR on economic fluctuations, respectively, whereas model 3 tests the joint effect of RISA and RISR. Model 4 tests the overall effect of regional industrial structure on the economic fluctuations of marine fisheries. Table 3 shows that the overall regression effect of the model is better and there is no pseudo regression. Regarding the validity test of instrumental variables, the corresponding P values of AR (2) and Sargan tests are all greater than 5%, indicating that the instrumental variables selected in models 1–4 are effective and there is no problem of over identification.
Table 3 Impact of regional industrial structure advancement and rationalization on economic fluctuations in the marine fishery industry.
Across the three models, RISA and RISR significantly affect economic fluctuations in the marine fishery industry. RISA’s effect is positive, and thus, it amplifies the economic fluctuations. However, the coefficient of interaction term g·RISAi,t*wyi,t is negative, which indicates that overall, it may restrain the economic fluctuations. Furthermore, RISR has a negative effect, indicating that the improvement of rationalization degree (RISR decrease) will inhibit the economic fluctuation in the marine fishery industry. Furthermore, the coefficient of interactive term g·RISRi,t*wyi,t is positive, indicating that interactive term can expand the economic fluctuation in the marine fishery industry. The results from model 3 are largely consistent with models 1 and 2. In terms of the overall effect (Model 4), the regional industrial structure evolution has a positive impact on the economic fluctuations in marine fisheries, indicating that it can promote the economic fluctuations, which is consistent with the results of Model 1.
In conclusion, the evolution of regional industrial structure significantly affects the fluctuations in the marine fishery economy. RISA’s evolution promotes these fluctuations; that is, it has a “leverage effect.” Thus, hypothesis H1 is supported. By contrast, RISR’s evolution restrains these fluctuations; that is, it has a “mitigating effect.” Thus, hypothesis H3 is supported. We find that while RISR can dampen economic fluctuations, it cannot eliminate them completely.This confirms that regional industrial restructuring causes unstable development of the fishing economy in the short term.
This study uses the dynamic GMM method to test the impact of the evolution of the regional industrial structure on the economic quality of the marine fishery industry. Models 5 and 6 test the effects of RISA and RISR on the economic quality, respectively. Model 7 examines the pathways of regional industrial structure evolution on the economic quality from an overall perspective. The regression results in Table 4 show that the p values of AR (2) and Sargan tests are all greater than 5%, which indicates that the selected variables are effective and there is no problem of overidentification.
Table 4 Regression results of the impact of regional industrial structure evolution on the economic quality of the marine fishery industry.
In model 5, whereas RISA and its squared term are both significant, the former has a negative effect whereas the latter has a positive effect. This shows that the nature of RISA’s relationship with the economic quality of the marine fishery industry is “U-shaped.” Thus, hypothesis H2 is supported. Meanwhile, in model 6, RISR and its squared term both significantly and negatively affect the economic quality of the marine fishery industry. This shows that RISR has an inverted U-shaped relationship with the economic quality of the marine fishery industry. Thus, the “structural dividend” of RISR on the improvement of the economic quality seems to vary across stages, thereby supporting hypothesis H4. The results of Model 7 showed that the primary and secondary terms of the regional industrial structure passed the significance level tests of 1% and 5% respectively. The estimated value of the primary term coefficient is positive, indicating that the regional industrial structure evolution is beneficial to improving the economic quality. The estimated coefficient of the secondary term is negative, indicating that the impact passway of regional industrial structure on the economic quality is an inverted U-shaped relationship.
Our results show that the evolution of regional industrial structure can cause the economic fluctuations in the marine fishery industry. The mechanism is shown in Figure 2. Specifically, RISA’s evolution not only attracts capital, science and technology, talents, and other marine fishery resources from the fishery system to non-fishery industries but also reduces the total amount of marine fishery resources. This weakens the development capacity of marine fisheries and dampens the speed of development. However, it can promote the advancement of the internal industrial structure of marine fishery and stimulate technological innovation and progress in the industry. Technological progress will accelerate the industrialization and service-oriented development of marine fisheries and promote the industry’s transformation from being resource intensive to capital and technology intensive. Meanwhile, greater fishery mechanization and intelligent operation abilities will also lead to the emergence of a surplus labor force, which can be absorbed in the non-fishery industries due to changes induced by RISA. In addition, RISA’s evolution will accelerate the development of science and technology, information, and other service fields. All these activities can cause the economic fluctuations in the marine fishery industry.
Next, RISR’s evolution has an inhibitory effect on these economic fluctuations. This is because RISR can coordinate the allocation of marine fishery resources or production factors in various industries and promote the marine fishery industry’s development from an unbalanced state to a balanced state, thereby inhibiting the fluctuations. RISR’s evolution can guide some non-fishery production factors or resources, especially advanced scientific and technological resources or human capital, into the marine fishery industry. This will promote the industry’s development and thus weaken economic fluctuations. Meanwhile, this evolution will accelerate the flow of regional economic resources or production factors, promote the exchange of information and technology between different industries, guide the flow of advanced production technologies (such as seedling technology, seafood-intensive processing technology, cold chain logistics technology, etc.) into marine fisheries, and improve the market competitiveness and technological strength of marine fisheries. This will mitigate the issues of market information asymmetry and low technology level of marine fisheries. These will promote the stable development of the industry.
First, RISA has a U-shaped relationship with the economic quality of the marine fishery industry. When RISA value is less than 5.108,7 it will inhibit the improvement of the industry’s total factor productivity, diminishing the “structural dividend.” RISA’s 8evolution can attract the surplus labor due to the upgrading of the fishery industry and relieve the pressure on fishermen to switch industries. However, it will also attract marine fishery science and technology resources, human capital, and other resources into the non-fishery industries with high productivity or growth. Consequently, the loss of these high-quality resources will greatly hinder the development of marine fishery economy. This is not conducive to the innovation and progress of marine fishery technology and, ultimately, will reduce the total factor productivity of marine fishery. Thus, at a low level of RISA, the negative effect of RISA’s evolution is greater than the positive effect; that is, RISA’s evolution of RISA is not conducive to the improvement of the economic quality of the marine fishery industry.
When RISA value is greater than 5.108, RISA’s evolution promotes the industry’s total factor productivity, yielding the “structural dividend.” RISA’s evolution can promote the improvement of regional science and technology innovation ability. Technology diffusion and spillover will also increase, with new scientific and technological achievements being applied to the marine fishery industry. This will reinforce the feedback effect of science and technology on the marine fishery industry. Meanwhile, greater cooperation between scientific research departments will further improve the scientific and technological innovation ability of the marine fishery industry. Overall, the continuous progress of fishery technology will help improve the marine fishery industry’s total factor productivity. The transmission effect of RISA’s evolution will promote advancement of the industry’s internal industrial structure, driving the self-innovation of technology. The application of new technology will improve the economic quality of the marine fishery industry.
Interestingly, all sampled areas show RISA values greater than 5.108 (Figure 3). This indicates that RISA’s evolution has a substantial impact on improving the economic quality of the marine fishery industry at the current stage. Hence, with further improvement in RISA, the “structural dividend” will gradually increase.
Second, RISR’s relationship with the economic quality of the marine fishery industry is “inverted U-shaped.” When RISR level reaches 0.3018, RISR’s promotion effect is the highest; at this point, the more reasonable the industrial structure is, the higher the economic quality of the marine fishery industry. Then, improving the industry’s total factor productivity has a dynamic equilibrium effect of industrial structure (Li, 2017). Thus, RISR has a phased effect on the “structural dividend” and there is an optimal level of rationalization. Rather, excessive rationality or irrationality of the regional industrial structure can reduce the marginal efficiency of resource elements.
According to data from 2003 to 2020, the Taier index of China and its coastal areas shows a downward trend (Figure 4), indicating that RISR is constantly improving. In 2003-2020, China's RISR average was 0.364 and higher than the optimal level of 0.301. Hence, this indicated the need to continuously optimise the RISR of China's marine fisheries to reach optimal levels and gradually release structural dividends. In terms of regions, these regions were not at the optimum level of structural rationalization from an average data (2003-2020) or data for 2020. RISR needs needs further adjustment. Hence, we speculate that RISR's evolution will have a potential "structural dividend" for improving economic quality in these regions.
This study analyzes the impact of regional industrial structure's evolution on the fluctuation and quality of the marine fishery economy from the RISA and RISR . The results show that the regional industrial structure's evolution is an important factor. It can cause fluctuations in the marine fisheries economy and improve the economic quality in the long term. The impact of RISA and RISR on economic fluctuations and economic quality in marine fisheries is differentiated. RISA causes economic fluctuations in the marine fishery industry, which has an obvious “leverage effect.” Further, RISA’s impact path on marine fishery quality is “U” shaped. In addition, all RISA values for the selected sample areas exceed 5.108. This indicates that RISA’s evolution has a “structural dividend.” Meanwhile, RISR’s evolution has a significant “mitigating effect” on the fluctuations in the marine fishery economy. Further, its impact path on marine fishery quality is “inverted U” shaped. This indicates that RISR has an optimal level at which it has a significant impact on the improvement of marine fishery quality; thus, if the marine fisheries economy quality is to be improved, we should not be deliberately pursued at highest level of structural rationalization, but at its optimum level. Too low or too high a level of rationalization is detrimental to the quality of fisheries.
In general, RISA’s evolution can improve the quality of the marine fisheries industry despite the fluctuating economic development. Meanwhile, RISR’s evolution can weaken these fluctuations through firm and technological transformations; however, excessive pursuit of structure rationalization is not conducive to improving the quality of the marine fisheries economy.
The raw data supporting the conclusions of this article will be made available by the authors, without undue reservation.
BW: Writing-original draft; Writing-review and editing; Methodology; Formal analysis; HTL: Data curation; PYS: Investigation; SFZ: Funding acquisition; software HZZ: Resources; Validation. All authors contributed to the article and approved the submitted version.
Project Support: The Guangxi Science and Technology Base and Talent Project (Guike AD20159004), the Guangxi Key R&D Plan (GuikeAB1850023), the Startup Project of Doctor Scientific Research of Yantai University (2220004/1103), the Youth Project of National Social Science Foundation of China (19CJY023), and the Youth Project of Natural Science Foundation of Guangxi Science and Technology Department (2018GXNSFBA050010).
The authors declare that the research was conducted in the absence of any commercial or financial relationships that could be construed as a potential conflict of interest.
All claims expressed in this article are solely those of the authors and do not necessarily represent those of their affiliated organizations, or those of the publisher, the editors and the reviewers. Any product that may be evaluated in this article, or claim that may be made by its manufacturer, is not guaranteed or endorsed by the publisher.
The Supplementary Material for this article can be found online at: https://www.frontiersin.org/articles/10.3389/fmars.2022.944630/full#supplementary-material
Ahmad Z., Jun M., Khan I. (2015). Agri. industrial structure and its influence on energy efficiency: A study of Pakistan. Eur. J. Economic Stud. 11 (1), 16–22.
Bah E. M., Brada J. C. (2009). Total factor productivity growth, structural change and convergence in the new members of the European union. Comp. Economic Stud. 51 (4), 421–446. doi: 10.1057/ces.2009.8
Baumol W. J. (1967). Macroeconomics of unbalanced growth: The anatomy of urban crisis. Am. Economic Rev., 415–426.
Baum C. F., Schaffer M. E., Stillman S. (2007). Enhanced routines for instrumental variables generalized method of moments estimation and testing. Stata J. 7 (4), 465–506. doi: 10.1177/1536867X0800700402
Deng C., Zhao K., Yang W. F. (2018). The nonlinear influence of industrial structure changes on economic growth in China–—An empirical study based on PSTR model. J. Quantitative Economics. 9 (02), 1–19. doi: 10.16699/b.cnki.jqe.2018.02.002
Ding Z. H., Zhang M. (2013). Influence of industrial structure transformation on economic fluctuation of Japan: Smooth or not? World Economy Stud. 1), 74–79. doi: 10.13516/j.cnki.wes.2013.01.010
Eggers A., Loannides Y. (2006). The role of output composition in the stabilization of US output growth. J. Macroeconomics. 28 (3), 585–595. doi: 10.1016/j.jmacro.2004.09.007
Fagerberg J. (2000). Technological progress, structural change and productivity growth: A comparative study. Struct. Change Economic Dynamics 11 (4), 393–411. doi: 10.1016/S0954-349X(00)00025-4
Fang F. Q., Zhan X. Y. (2011). Empirical analysis of the stabilizing effect of industrial structure upgrading on the economic fluctuations in China. Economic Theory Business Manage. 9), 5–16.
Frank M. W. (2005). Income inequality and economic growth in the U.S.: a panel cointegration approach, working paper (Texas, USA: Sam Houston State University).
Fu X. M., Wang N., Jiang S. S., Yang F., Li J. M., Wang C. Y. (2019). A research on influencing factors on the international cooperative exploitation for deep-sea bioresources based on the ternary fuzzy DEMATEL method. Ocean Coast. Management. 172, 55–63. doi: 10.1016/j.ocecoaman.2019.01.019
Gan C. H., Zheng R. G., Yu D. F. (2011). An empirical study on the effects of industrial structure on economic growth and fluctuations in China. Economic Res. J. 5), 4–16.
Gómez ,. S., Maynou F. (2020). Economic, sociocultural and ecological dimensions of fishing capacity in NW Mediterranean fisheries. Ocean Coast. Manage., 197. doi: 10.1016/j.ocecoaman.2020.105323
Han L. M. (2018). Study on the "blue granary" strategy in china's marine unemployment development (Beijing: Economic Science Press), pp5.
Han L. M., Guo Y. C., Dong S. L. (2016). Research on establishing a national offshore aquaculture experimental zone based on the development of the yellow Sea cold water mass. Pacific J. 24 (05), 79–85. doi: 10.14015/j.cnki.1004-8049.2016.5.009
Han L. M., Li D. H. (2015). Blue food system: Guarantee of china's food security. Issues Agric. Economy 36 (01), 24–29+110. doi: 10.13246/j.cnki.iae.2015.01.005
Han L. M., Ren G. Y., Qin H. (2007). The basic connotation and particularity of the "fishery, fishermen, fishing village" problem. Issues Agric. Economy 06), 93–96+112.
Hodrick R. J., Prescott E. C. (1997). Postwar U.S.business cycles: An empirical investigation. J. Money Credit Banking 29), 1–16.
Hu A. G., Zhou S. J., Ren H. (2016). The strategic innovations towards adapting to and leading china's new normal status. J. Tsinghua Univ. (Philosophy Soc. Sciences) 31 (02), 17–22+195. doi: 10.13613/j.cnki.qhdz.002419
Jin Y. Y., Liu Y. Y., Lin J. M. (2020). The relationship between the evolution of marine fishery industrial structure and economic growth. Ocean Dev. Manage. 37 (08), 64–68. doi: 10.20016/j.cnki.hykfygl.2020.08.013
Le J. H., Dai Y., Liu W. C. (2019). An empirical analysis of the impact of fishery industry structure on economic growth: Take nine coastal provinces as example. Chin. Fisheries Economics 37 (01), 13–20.
Li X. Y. (2017). An empirical study on production structure changes of china’s marine fishery and its influencing factors (Hangzhou, China: Zhejiang University).
Li D. H., Han L. M. (2019). A study on building a new system of food security guarantee by integrating land and sea. J. Soc. Sci. Ser. 06), 109–117+2.
Liu W. C. (2018). Research into the influence of change of fishery industry structure on the fishery economic growth from the supply side (Shanghai, China: Shanghai Ocean University).
Liu W., Zhang H. (2008). Structural change and technical advance in china's economic growth. Economic Res. J. 43 (11), 4–15.
Meng Q. W. (2015). Analysis on the evolution of china's fishery internal industrial structure and adjustment countermeasures. Dongyue Tribune 36 (05), 126–129. doi: 10.15981/j.cnki.dongyueluncong.2015.05.022
Peneder M. (2003). Industrial structure and aggregate growth. Struct. Change economic dynamics. 14 (4), 427–448. doi: 10.1016/S0954-349X(02)00052-8
Peng C., Li C. F., Li Y. S. (2013). Study on the dynamic effects of industrial structure on economic fluctuation. Ind. Economic Res. 3), 91–100. doi: 10.13269/j.cnki.ier.2013.03.010
Ping Y., Zhao L. R. (2018). Analysis and forecast of contribution of fishery industry economic growth. Chin. Fisheries Economics. 36 (04), 64–69.
Samuels J. D. (2017). Assessing aggregate reallocation effects with heterogeneous inputs, and evidence across countries. Rev. World Economics. 153 (2), 385–410. doi: 10.1007/s10290-016-0273-x
Shen Y. C., Yue S. J., Sun ,. S. Q., Guo M. Q. (2020). Sustainable total factor productivity growth: The case of China. J. Of Cleaner Production, 256. doi: 10.1016/j.jclepro.2020.120727
Tu Y. Z., Liu R., Yang X. Z. (2016). Research on the impact of industrial structure upgrading to TFP in China. Ind. Economic Review. 04), 45–58. doi: 10.14007/j.cnki.cjpl.2016.04.004
Valdemarsen J. W. (2001). Technological trends in capture fisheries. Ocean & coastal management 44, 635–651. doi: 10.1016/S0964-5691(01)00073-4
Wang B., Han L. M. (2017). The impact of china's marine industry structure change on marine economic growth: Based on the regression analysis of panel threshold effects in 11 provinces and cities along the coast. Resource Sci. 06), 1182–1193.
Wang B., Han L. M., Ni G. J. (2019a). Research on the structural effect of marine fisheries economic growth in structural reforms–from the interpretation of the panel threshold mode. J. Agrotechnical Economics. 04), 132–144. doi: 10.13246/j.cnki.jae.2019.04.020
Wang ,. B., Han L., Zhang H. (2021). The impact of regional industrial structure upgrading on the economic growth of marine fisheries in China–the perspective of industrial structure advancement and rationalization. Front. Mar. Sci. 8. doi: 10.3389/fmars.2021.693804
Wang B., Ni G. J., Han L. M. (2019b). Impact of the evolution of industry structure on the economic fluctuation of marine fishery. Resource science. 41 (02), 289–300.
Wang B. (2022). Study on the development strategy of China's marine fishery economy from the perspective of industrial structure evolution. (Beijing: Economic Science Press).
Wang J., Ning L. (2017). Opportunities, predicaments and directions of the supply-side structural reform of fisheries. Northern Economy Trade. 06, 100–101.
Wang D. W., Wang M. Y., Chen L. (2004). China's industrial restructuring, efficiency and its labor allocation. Economic Res. J. 4), 41–49.
Warren ,. C., Steenbergen D. J. (2021). Fisheries decline, local livelihoods and conflicted governance: An Indonesian case. Ocean Coast. Manage. 202, 105498. doi: 10.1016/j.ocecoaman.2020.105498
Xu M., Tao C. Q. (2017). Dual environmental regulation, industrial structure and total factor productivity: Based on system GMM and threshold model. J. Nanjing Univ. Finance Economics. 1), 8–17.
Yang L., Su X. (2010). Research on the objectives and implementation paths of the optimization and upgrading of marine fishery industrial structure from the perspective of industrial ecology. Issues Agric. Economy. 31 (10), 99–105.
Yang ,. W., Yan ,. M. (2018). Regional influence of china's fishery structure adjustment on fishermen's income. Jiangsu Agric. Sci. 46 (21), 324–328.
Zhang C., Ping Y. (2017). The influence of marine industrial structure change on marine economic efficiency. Ocean Dev. Management. 11), 91–96.
Zhao W. J., Yu J. P. (2012). Trade openness, FDI and china's industrial economic growth pattern–empirical analysis based on data of 30 industrial sectors. Economic Res. J. 8), 18–31.
Keywords: regional industrial structure, marine fisheries, economic fluctuations, development quality, structure effect
Citation: Wang B, Li HT, Sun PY, Zhang SF and Zhang HZ (2023) The effects and paths of regional industrial structure transformation on the fluctuation and quality of the marine fisheries economy in China. Front. Mar. Sci. 9:944630. doi: 10.3389/fmars.2022.944630
Received: 15 May 2022; Accepted: 24 October 2022;
Published: 25 January 2023.
Edited by:
You-Shao Wang, South China Sea Institute of Oceanology (CAS), ChinaReviewed by:
Shafi Muhammad, Lasbela University of Agriculture, Water and Marine Sciences, PakistanCopyright © 2023 Wang, Li, Sun, Zhang and Zhang. This is an open-access article distributed under the terms of the Creative Commons Attribution License (CC BY). The use, distribution or reproduction in other forums is permitted, provided the original author(s) and the copyright owner(s) are credited and that the original publication in this journal is cited, in accordance with accepted academic practice. No use, distribution or reproduction is permitted which does not comply with these terms.
*Correspondence: Shaofeng Zhang, emhhbmdzaGFvZmVuZ0A0aW8ub3JnLmNu; Hongzhi Zhang, b3VxZHNub3dAMTYzLmNvbQ==
Disclaimer: All claims expressed in this article are solely those of the authors and do not necessarily represent those of their affiliated organizations, or those of the publisher, the editors and the reviewers. Any product that may be evaluated in this article or claim that may be made by its manufacturer is not guaranteed or endorsed by the publisher.
Research integrity at Frontiers
Learn more about the work of our research integrity team to safeguard the quality of each article we publish.